- 1Guangdong Medical University, Zhanjiang, China
- 2Department of Burn Surgery, The First People’s Hospital of Foshan, Foshan, China
- 3The Second Affiliated Hospital, School of Medicine, Zhejiang University, Hangzhou, China
- 4Department of Dermatology, The First People’s Hospital of Foshan, Foshan, China
- 5Neijiang Health Vocational College, Neijiang, China
- 6The Second School of Medicine, Wenzhou Medical University, Wenzhou, China
- 7Department of Burn and Plastic Surgery, Guangzhou First People’s Hospital, South China University of Technology, Guangzhou, China
A keloid is a fibroproliferative disorder of unknown etiopathogenesis that requires ill-defined treatment. Existing evidence indicates that the immune system plays an important role in the occurrence and development of keloid. However, there is still a lack of research on the immune-related signatures of keloid. Here we identified immune-related signatures in keloid and explored their pathological mechanisms. Transcriptomic datasets (GSE7890, GSE92566, and GSE44270) of keloid and normal skin tissues were obtained from the Gene Expression Omnibus database. The overlap of differentially expressed genes and immune-related genes was considered as differentially expressed immune-related genes (DEIGs). Functional analysis, expression, and distribution were applied to explore the function and characteristics of DEIGs, and the expression of these DEIGs in keloid and normal skin tissues was verified by immunohistochemistry. Finally, we conducted interactive network analysis and immune infiltration analysis to determine the therapeutic potential and immune correlation. We identified four DEIGs (LGR5, PTN, JAG1, and DKK1). In these datasets, only GSE7890 met the screening criteria. In the GSE7890 dataset, DKK1 and PTN were downregulated in keloid, whereas JAG1 and LGR5 were upregulated in keloid. In addition, we obtained the same conclusion through immunohistochemistry. Functional analysis indicated that these four DEIGs were mainly involved in stem cell, cell cycle, UV response, and therapy resistance. Through interactive network analysis, we found that these DEIGs were associated with drugs currently used to treat keloid, such as hydrocortisone, androstanolone, irinotecan, oxaliplatin, BHQ-880, and lecoleucovorin. Finally, many immune cells, including CD8+ T cells, resting memory CD4+ T cells, and M1 macrophages, were obtained by immune infiltration analysis. In conclusion, we identified four immune signaling molecules associated with keloid (LGR5, PTN, JAG1, and DKK1). These immune-related signaling molecules may be important modules in the pathogenesis of keloid. Additionally, we developed novel therapeutic targets for the treatment of this challenging disease.
Introduction
Keloid, one of the benign dermal tumors, is a pathological scar caused by abnormal hyperplasia of the connective tissue in the skin. It is a special type of skin scar; the specific formation mechanism is unclear, but it only occurs as a result of long-term abnormal wound healing (1, 2). Relevant literature shows that susceptibility to keloid formation occurs predominantly in Africans and Asians (1, 3). Keloid generally occurs between 10 and 30 years of age and affects both sexes equally. Keloid may grow slowly for weeks, months, or years, and it can gradually increase and invade the normal skin beyond the edge of the original wound, showing invasive growth, which is the most important feature of keloid (4). They eventually stop growing but do not disappear on their own. Once a keloid develops, it is permanent unless removed or treated successfully (5).
In addition to keloid, hypertrophic scar is another type of abnormal scar on the skin (6). A hypertrophic scar is a wide, thickened, and often raised scar that develops at the site of skin injury and that can be itchy and typically does not show invasive growth. Over time, it extends beyond the boundary of the scar and gradually becomes mature and flat, which is very original wound (7). A leading cause of hypertrophic scar formation is the mechanical tension on the wound (8), but a hypertrophic scar ceases to grow and spontaneously regresses without treatment ranging from months to years (6, 9), which are different from what occurs in keloid (10, 11). From an aesthetic point of view, keloid is thought of as unpleasant, and from a functional point of view, they cause pain and itching. At present, keloid treatment mainly includes surgical and non-surgical treatments, which include laser treatment, radiotherapy, pressure therapy, and hormone therapy. Surgical resection of keloid is the most direct method. Recent literature reports that fat transplantation and botulinum toxin A have certain effects on keloid treatment (12, 13). However, recurrence after conservative treatment or surgical resection is very common, causing a psychological burden on the patients (14, 15).
Inflammation is a defensive response of the human body to damage caused by various inflammatory irritants. The exudation stage includes hemodynamic changes, increased vascular permeability, and inflammatory cell response and limits. It eliminates and rejects foreign pathogenic factors and cells killed by injury through local tissue degeneration, exudation, and proliferation. After the wound is healed (usually 1 to 3 months), scars begin to proliferate on the edge of the wound, sometimes with dilated capillaries and rough surface accompanied by discomfort such as itching and pain. This stage is the collagen stage. Usually, the keloid growth reaches a peak at about 6 months, and then the keloid gradually matures and softens. The first sign of a mature keloid is a change in color, from bright red to dark red, then to purple or brown, and finally to the color of the skin around the keloid; wrinkles appear on the surface of the keloid, and capillaries are reduced until they disappear, the hardness softens, the thickness becomes thinner, and the pain symptoms eventually disappear, but the itching can persist for a long time until the keloid is fully mature (16). The maturation process of keloid is relatively slow; usually it takes half a year to 2 years, and in some patients it can take as long as 3 and 4 years, and the length of time for a keloid to mature in different parts is different (17). The treatment of a keloid needs to be comprehensively considered according to the patient’s age and its nature, size, anatomical location, and distribution as well as the existence of infection foci and function and other factors to establish an individualized treatment plan that suits a patient’s condition (18–20). A treatment plan for keloid includes an injection of corticosteroids, surgical removal (keloid surgery), pressure earring, dressing or garment, laser treatment, silicone sheets and gels, cryotherapy, radiation treatments, and ligature, but these treatments are based on appearance or symptoms. The lack of an exact treatment is mainly due to the unclear specific pathogenesis of keloid, and early literature reports showed that the levels of IgG, IgA, and IgM in keloid were higher than those in normal skin, indicating that keloid formation is a local immune response (21, 22). Moreover, the number of T cells, Langerhans cells, mast cells, and macrophages in keloid is higher than those in normal tissues, which is related to skin fibrosis (23, 24). The levels of circulating immune complexes and cells in patients with keloid are significantly higher than those in normal individuals. All the above-mentioned results show that keloid growth is closely related to immunity. Therefore, it can be inferred that immune treatment methods may have significant prospects in keloid (25).
Inflammation and immune responses have dramatic impacts on the pathogenesis of keloid, and there is a theory that the formation of a keloid is due to the abnormal response of fibroblasts to inflammation (11, 26). A previous study performed nine-color flow cytometry with computational analysis to detect recurrence-related epidermal cellular subsets in keloid (27), further confirming the importance of the immune system. Immune-related genes are helpful in the prognosis and mechanisms of various diseases (28–31). However, little research has been conducted on keloid. Currently, bioinformatic analysis is an important tool for analyzing expression data and screening for target genes in many diseases. Here we first explored the differentially expressed immune-related genes (DEIGs) among different keloid datasets, conducted multiple functional enrichment analyses, constructed a protein–protein interaction (PPI) network, and explored immune infiltration in the keloid microenvironment. Through immunohistochemical verification of keloid and normal skin tissues, we identified immune-related features. Finally, four genes related to keloid (LGR5, PTN, JAG1, and DKK1) were identified, and we further studied keloid pathogenesis, which may provide new ideas and insights for keloid treatment. The design and workflow of this study are shown in Figure 1.
Materials and methods
Data acquisition
Three public datasets, GSE7890 (32), GSE92566 (33), and GSE44270 (34), from the Gene Expression Omnibus database (35) were retrospectively analyzed to obtain the corresponding data, as described previously (36). GSE7890 and GSE92566 were regarded as the training cohort, and GSE44270 was considered as the validation cohort (Table 1). Moreover, hallmark gene sets (37), which are coherently expressed signatures derived by aggregating many gene sets to represent well-defined biological states or processes, and immunologic signature gene sets (38), which represent cell states and perturbations within the immune system, were downloaded from the Molecular Signatures Database (version 7.4) (37, 39). Immune-related genes were obtained from ImmPort (40) (Supplementary Table S1).
Identification of differentially expressed genes (DEGs)
We preprocessed the original data of the training and validation cohorts using the affy package (version 1.68.0) (41) in R (version 3.6.2) to generate the corresponding gene expression profiles. DEGs between the keloid and normal groups were obtained using the limma package (version 3.46.0) (42) with a threshold of log2|fold change| > 1 and P <0.05. The pheatmap package (version 1.0.12) and the ggplot2 package (version 3.3.3) were used for visualization.
Identification of DEIGs
The DEGs obtained from the training cohort were crossed with immune-related genes to obtain the DEIGs. In addition, we computed the semantic similarity among Gene Ontology patterns to evaluate the functional enrichment similarity among DEIGs using the GOSemSim package (version 2.16.1) (43). At the same time, the STRING database (version 11.0) (44) was used to construct the protein–protein interaction (PPI) network. Moreover, we used the MCODE plug-in (version 1.32) (45) in Cytoscape (version 3.8.2) (46) to identify densely connected networks, as described previously (47, 48).
Enrichment analysis
Each training cohort was divided into high and low groups according to the median expression of DEIGs, and the limma package was used for differential analysis. The clusterProfiler package (version 3.18.1) (49) was used for gene set enrichment analysis (50), disease ontology (51), gene ontology (52), and Kyoto Encyclopedia of Genes and Genomes (53) enrichment analysis to understand the biological processes involved in these DEIGs.
Expression and distribution
We analyzed the expression and distribution of each DEIG based on the Human Protein Atlas (54) and Gene Expression Profiling Interactive Analysis (55) to gain a deeper understanding of each DEIG.
Interactive network analysis
Furthermore, the interaction networks of DEIGs was analyzed from three aspects: DEIG–transcription factor, DEIG–microRNA (miRNA), and DEIG–drug through CHIP-X Enrichment Analysis Version 3 (56), Encyclopedia of RNA Interactomes (57), and Drug Gene Interaction Database (58), respectively. The interaction networks were visualized by Cytoscape (46).
Immune infiltration analysis
We conducted immune infiltration landscape analysis based on CIBERSORT (59) to estimate the abundance of member cell types in a mixed keloid cell population using DEIG expression data in the training and validation cohorts.
Clinical validation
Fifteen patients with keloid were enrolled for clinical validation. Keloid and adjacent normal skin tissue samples were collected. The study was approved by the ethics committee of Foshan First People’s Hospital (Foshan, China), and the patients provided signed written informed consent before enrollment. Immunohistochemistry assay was conducted according to the standard procedure (60, 61). The antibodies used were as follows: anti-JAG1 (1:150, SAB, United States), anti-DKK1 (1:150, SAB, United States), anti-PTN (1:150, SAB, United States), anti-LGR5 (1:1050, Affinity, United States), and secondary antibody conjugated with horseradish peroxidase (1:500, Affinity, United States). The process of histological scoring and analysis was conducted as described in our previous study (62).
Results
Identification of DEIGs
We included nine keloid samples and 8 normal samples in the training cohort and 18 keloid samples and 14 normal samples in the validation cohort, respectively (Table 1). There were 48 upregulated and 157 downregulated DEGs in GSE7890 (Figures 2A, B) as well as 801 upregulated DEGs and 908 downregulated DEGs in GSE92566 (Figures 2C, D). A total of 1,793 immune-related genes were obtained from ImmPort, and four DEIGs (LGR5, PTN, JAG1, and DKK1) were obtained by crossing the DEGs from the training cohort (Figure 3A). Detailed information on the four DEIGs is presented in Table 2. Despite the functional enrichment similarity, we found that the score of each DEIG was more than 0.5, and JAG1 had the highest score (Figure 3B). The PPI network with 94 nodes and 1,402 edges indicated the interaction of these genes (Figure 3C), and DKK1 may be the most important gene among these genes (Figure 3D). Moreover, using the MCODE plug-in, we observed DKK1 in a densely connected network with 35 nodes and 570 edges (Figure 3E).
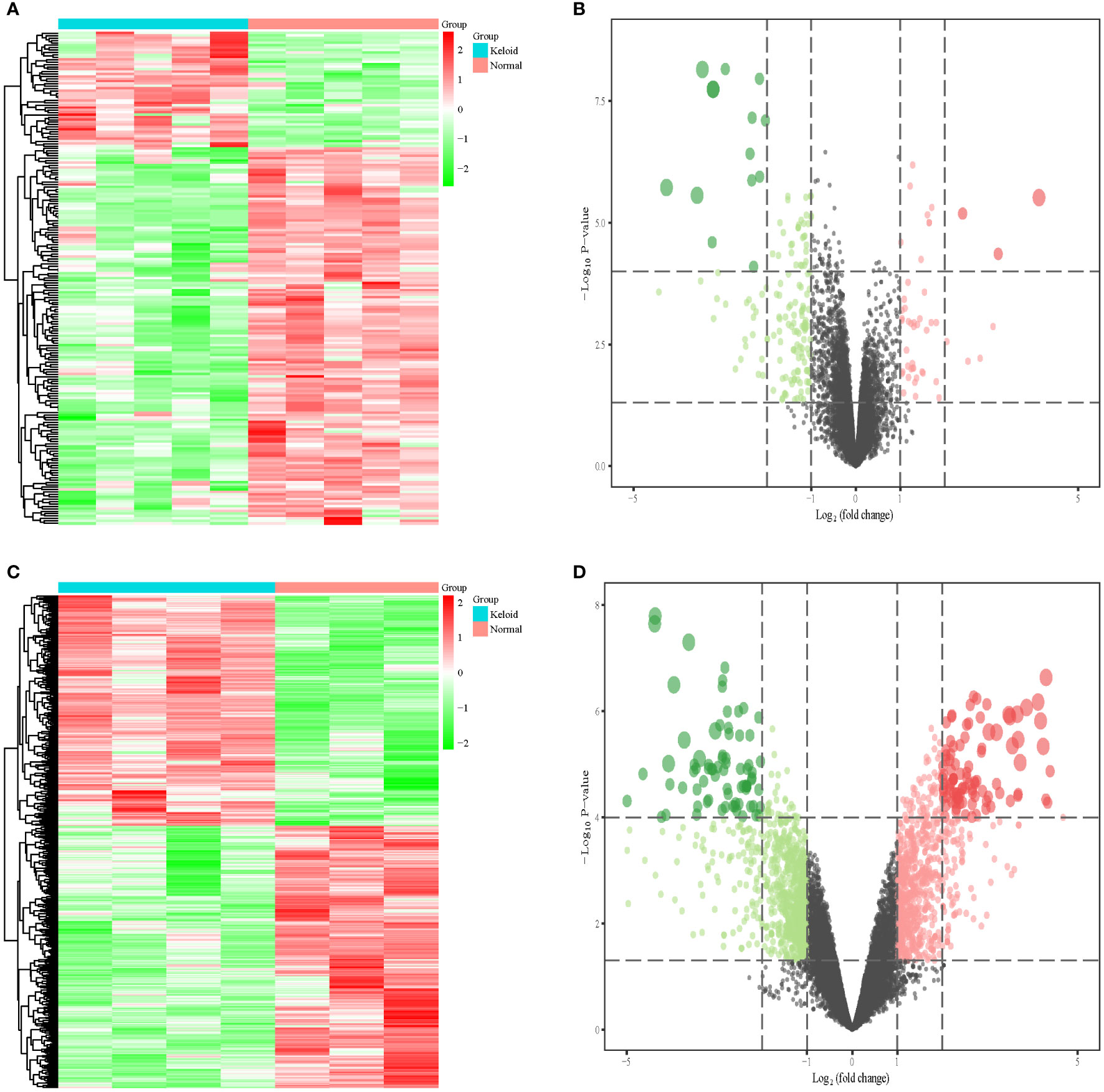
Figure 2 Differential expression analysis in three datasets. (A) Heat map of DEGs in GSE7890. (B) Volcano plot of DEGs in GSE7890. (C) Heat map of DEGs in GSE92566. (D) Volcano plot of DEGs in GSE92566.
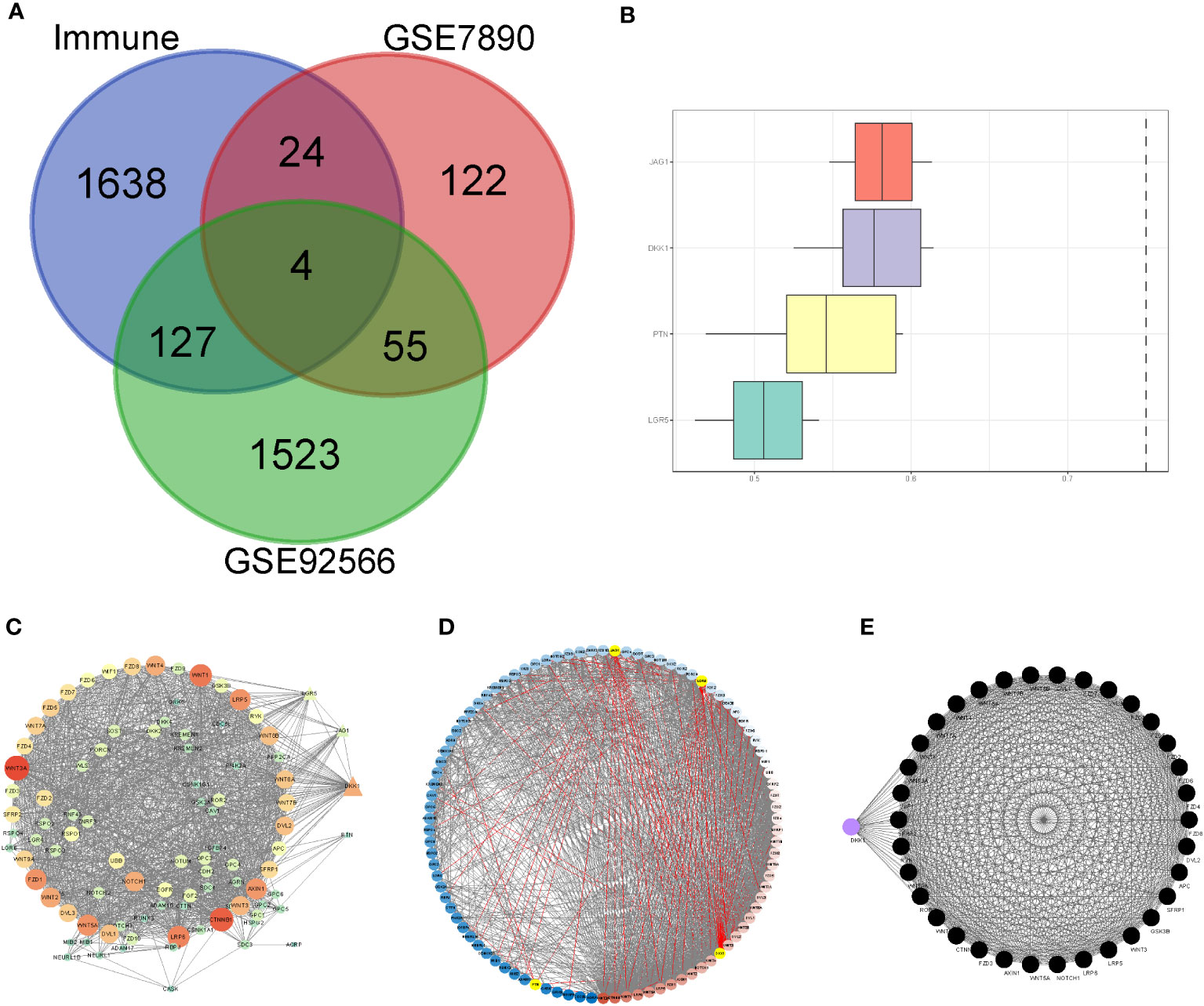
Figure 3 Principal component analysis and identification of differentially expressed immune-related gene (DEIGs). (A) Intersection of differentially expressed genes and immune-related genes. (B) Summary of the functional similarities of four DEIGs. (C) protein–protein interaction (PPI) network sized by degree. (D) PPI network colored by degree. (E) MCODE.
Enrichment analysis
The gene set enrichment analysis indicated that four DEIGs were mainly involved in stem cells and the cell cycle in GSE7890 (Figure 4) and UV response and therapy resistance in GSE92566 (Figure 5). Meanwhile, we conducted disease ontology, gene ontology, and Kyoto Encyclopedia of Genes and Genomes functional analyses in GSE7890 (Figure 6) and GSE92566 (Figure 7). The results indicated that DKK1, JAG1, LGR5, and PTN were enriched in immune-related terms (Figures 6, 7).
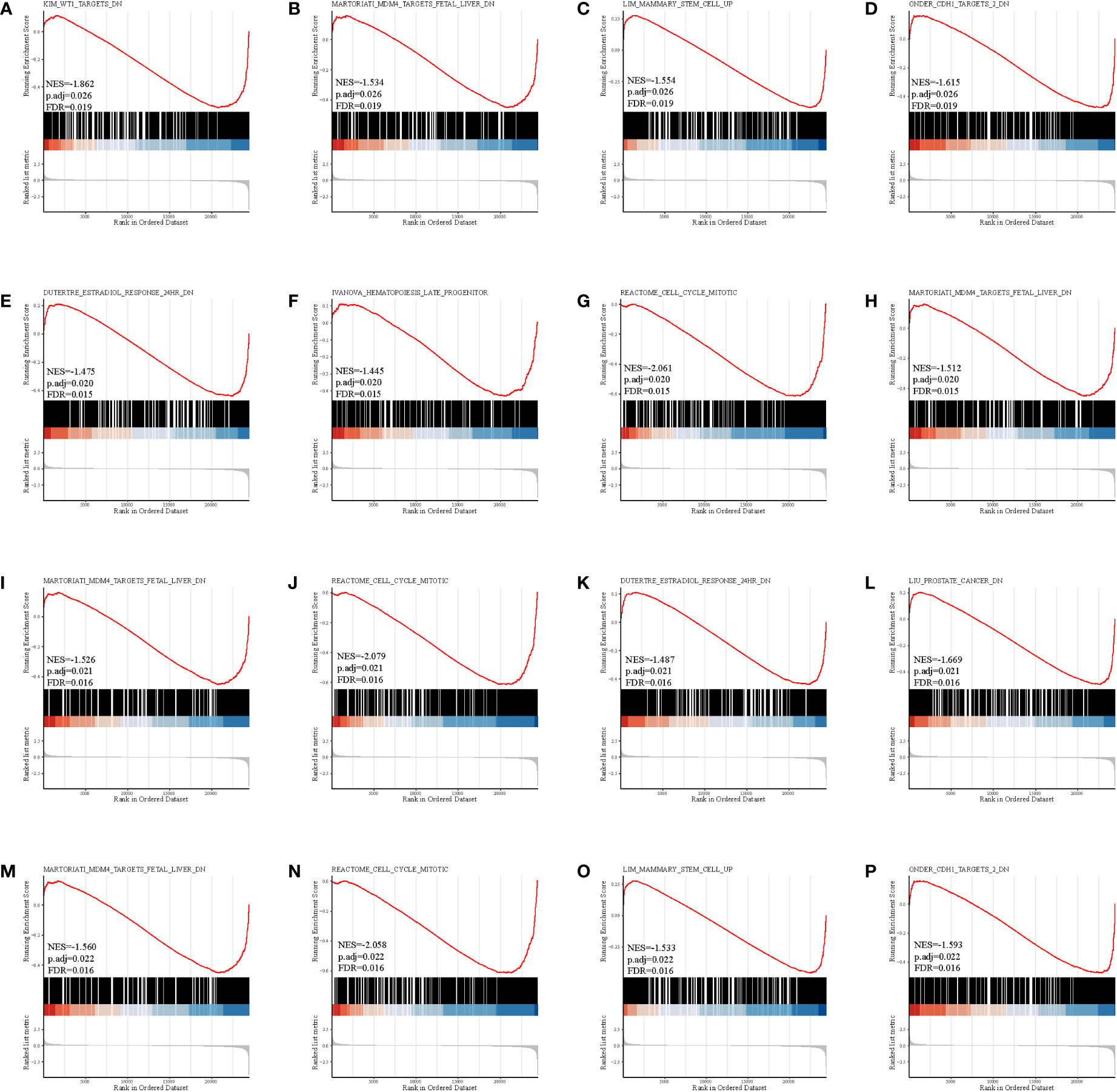
Figure 4 Gene set enrichment analysis in GSE7890. (A–D) DKK1, (E–H) JAG1, (I–L) LGR5, and (M–P) PTN.
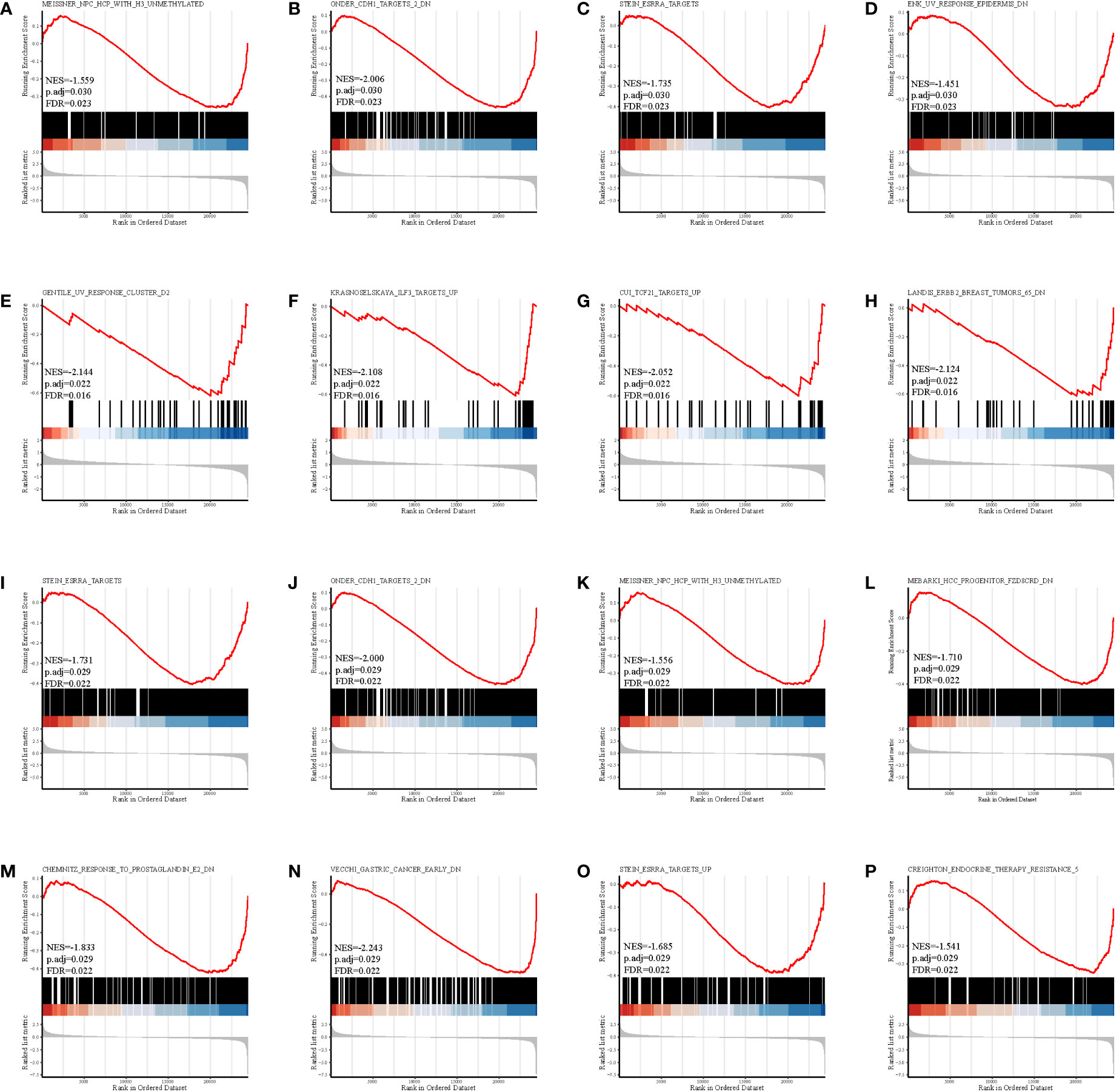
Figure 5 Gene set enrichment analysis in GSE92566. (A–D) DKK1, (E–H) JAG1, (I–L) LGR5, and (M–P) PTN.
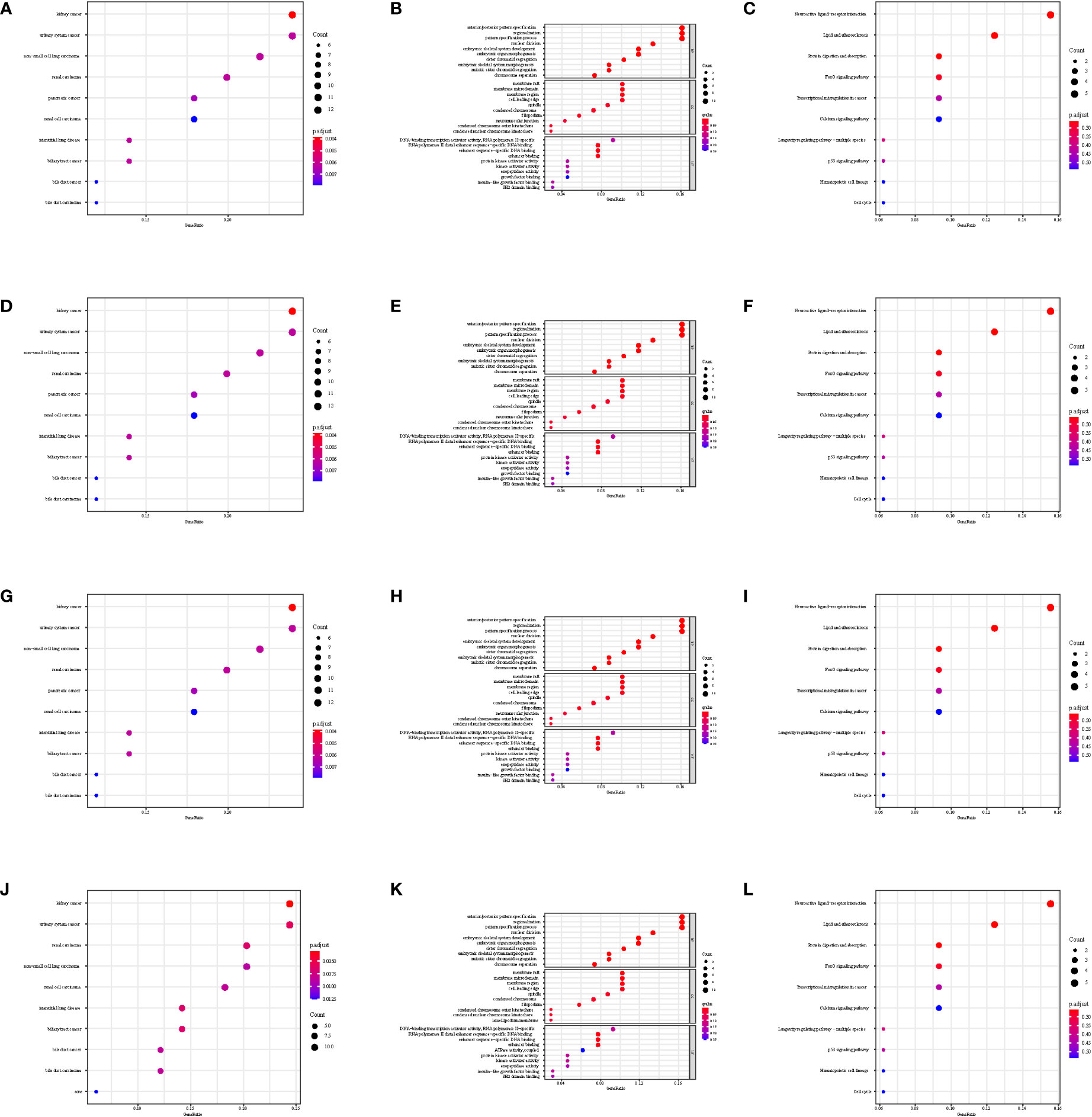
Figure 6 Disease Ontology, Gene Ontology, and Kyoto Encyclopedia of Genes and Genomes functional analysis in GSE7890. (A–C) DKK1, (D–F) JAG1, (G–I) LGR5, and (J–L) PTN.
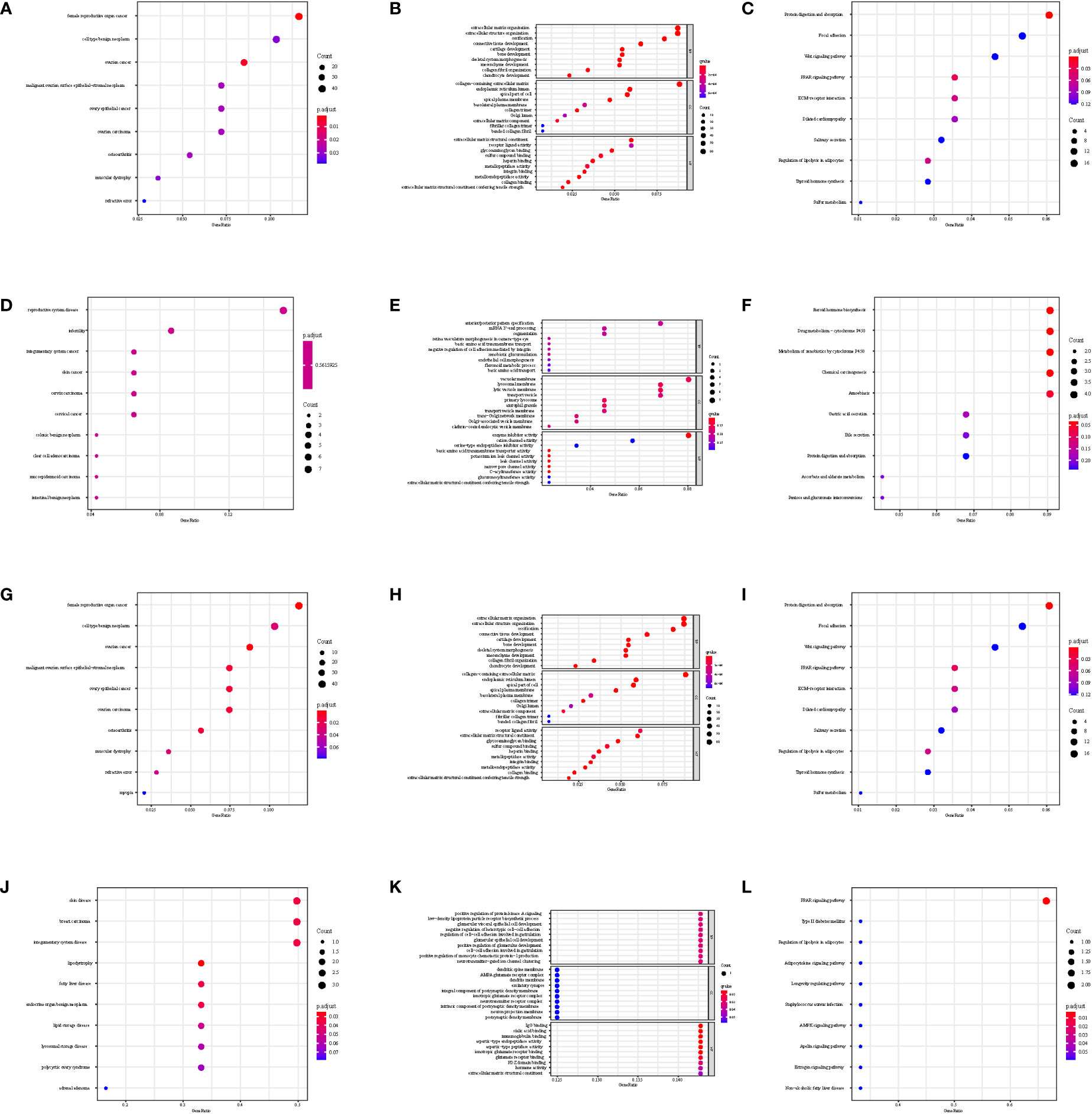
Figure 7 Disease Ontology, Gene Ontology, and Kyoto Encyclopedia of Genes and Genomes functional analysis in GSE92566. (A–C) DKK1, (D–F) JAG1, (G–I) LGR5, and (J–L) PTN.
Expression and distribution
Through the Human Protein Atlas, we found that LGR5, PTN, JAG1, and DKK1 were expressed in the skin (Figures 8A–D).
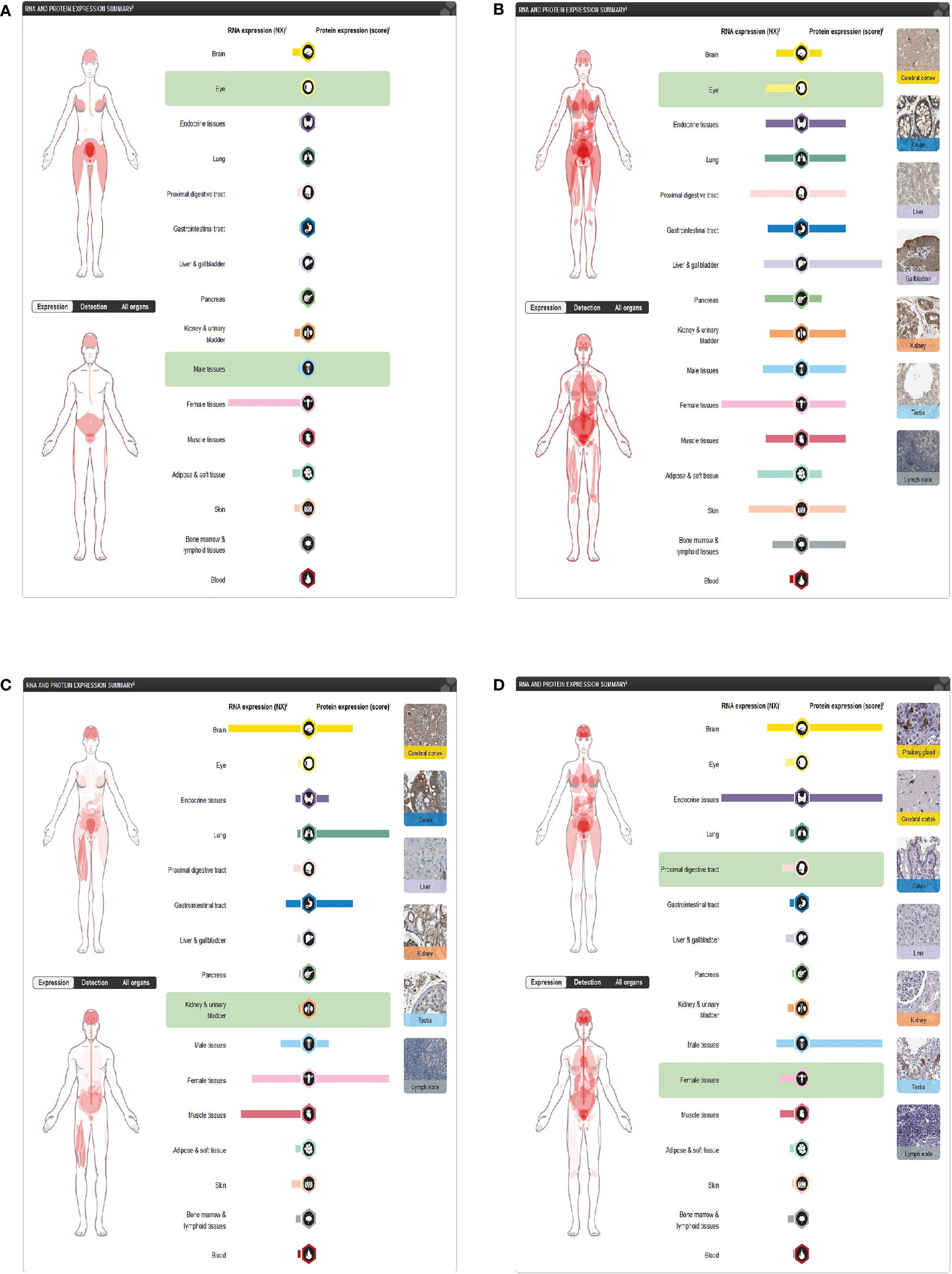
Figure 8 Expression of four differentially expressed immune-related genes. (A) DKK1 in the Human Protein Atlas. (B) JAG1 in the Human Protein Atlas. (C) LGR5 in the Human Protein Atlas. (D) PTN in the Human Protein Atlas.
Interactive network analysis
The DEIG–drug network showed that JAG1 was involved in hydrocortisone, and DKK1 and LGR5 were involved in fluorouracil (Figure 9A). Besides this, DKK1 was also involved in androstanolone, irinotecan, oxaliplatin, BHQ-880 (anti-DKK1 mAb), and lecoleucovorin (Figure 9A). The DEIG–transcription factor network indicated that the four DEIGs had the same transcription factors (Figure 9B). The DEIG–miRNA networks also demonstrated the same miRNAs (Figures 9C–F).
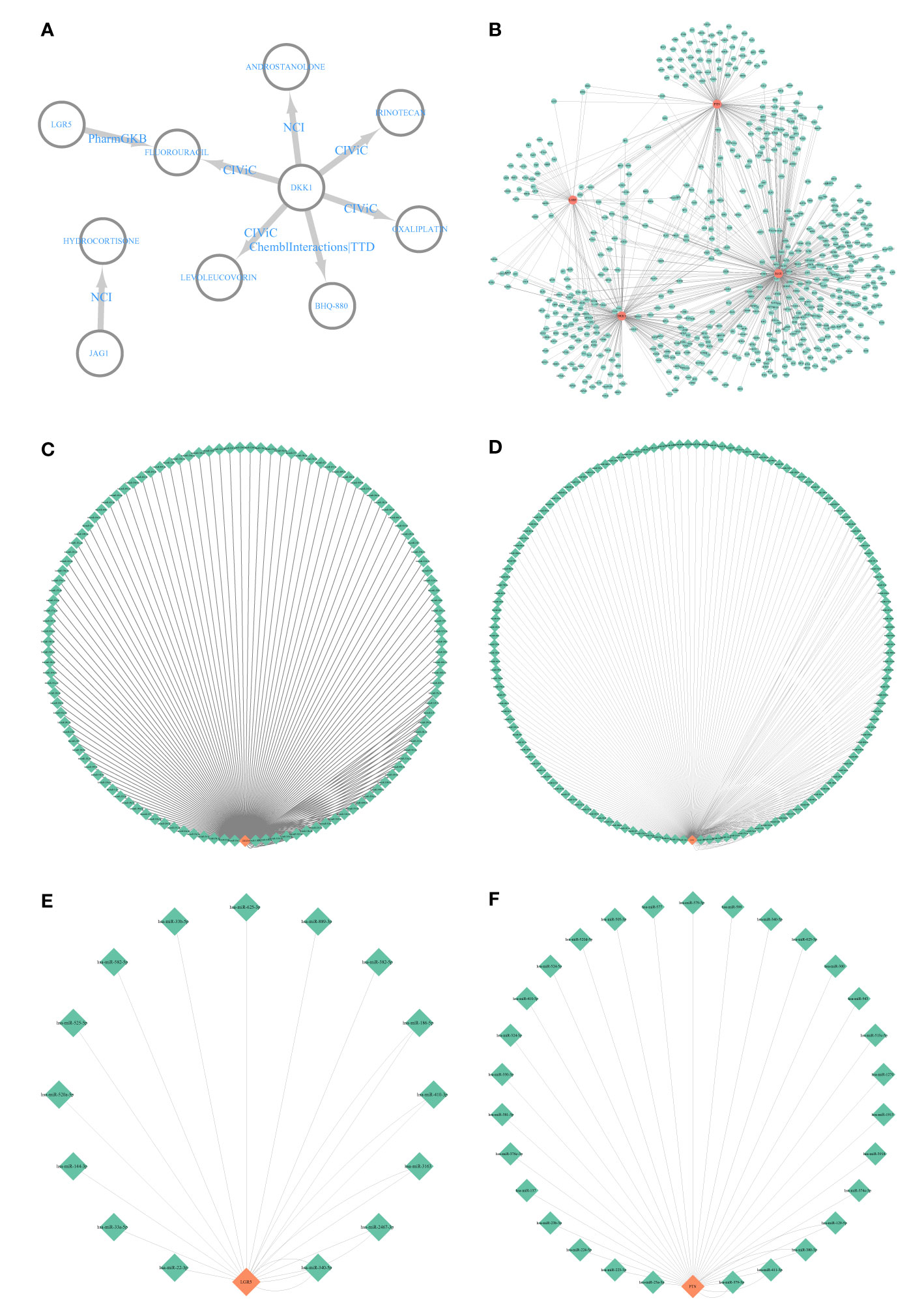
Figure 9 Interactive network analysis. (A) Differentially expressed immune-related gene (DEIG)–drug network; (B) DEIG–transcription factor network. (C) DKK1–miRNA network. (D) JAG1–miRNA network. (E) LGR5–miRNA network. (F) PTN–miRNA network.
Immune infiltration analysis
Using CIBERSORT, we found that CD8+ T cells infiltrated the low-DKK1 group, resting memory CD4+ T cells infiltrated the high-DKK1 group, and M1 macrophages infiltrated the low-DKK1 group in GSE7890 (Figure 10A). CD8+ T cells infiltrated the high-JAG1 group, resting memory CD4+ T cells infiltrated the low-JAG1 group, and M1 macrophages infiltrated the high-JAG1 group in GSE7890 (Figure 10B). CD8+ T cells infiltrated the high-LGR5 group, resting memory CD4+ T cells infiltrated the low-LGR5 group, and M1 macrophages infiltrated the high-LGR5 group in GSE7890 (Figure 10C). CD8+ T cells infiltrated the low-PTN group, resting memory CD4+ T cells infiltrated the high-PTN group, and M1 macrophages infiltrated the low-PTN group in GSE7890 (Figure 10D). Interestingly, similar results were obtained for GSE44270 and GSE92566 (Figures 10E–L).
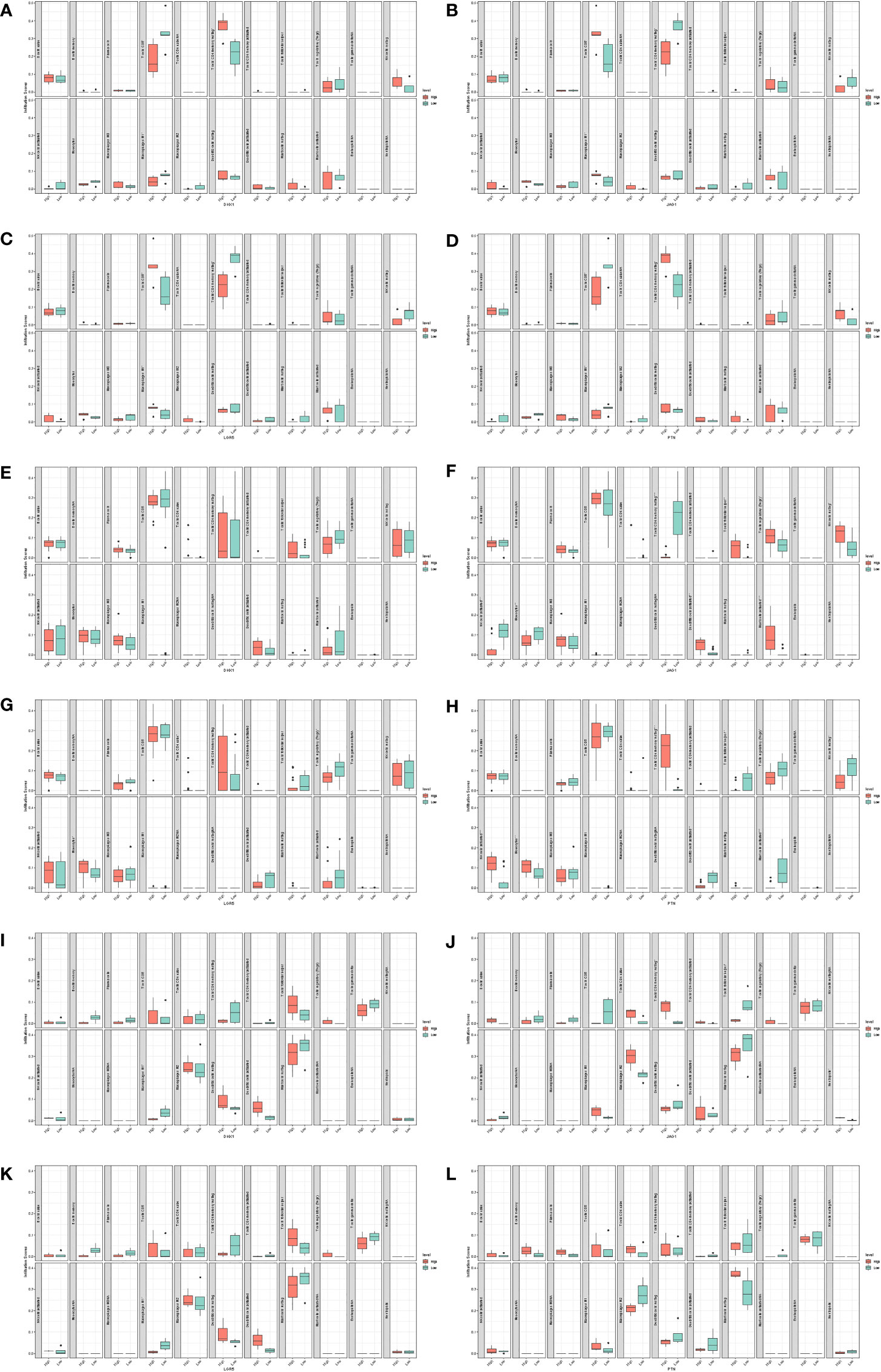
Figure 10 Immune infiltration analysis. (A) DKK1 in GSE7890. (B) JAG1 in GSE7890. (C) LGR5 in GSE7890. (D) PTN in GSE7890. (E) DKK1 in GSE44270. (F) JAG1 in GSE44270. (G) LGR5 in GSE44270. (H) PTN in GSE44270. (I) DKK1 in GSE92566. (J) JAG1 in GSE92566. (K) LGR5 in GSE92566. (L) PTN in GSE92566.
Preliminary immunohistochemistry validation
Through immunohistochemistry staining, we found that the expression of DKK1 and PTN was lower in the keloid (Figures 11A, B), while JAG1 and LGR5 showed a higher expression in the keloid (Figures 11C, D). In the keloid tissue, the brownish-yellow particles with a positive expression of the four molecules were mainly distributed in the nucleus and cytoplasm of fibroblasts. In the normal tissues, the brownish-yellow particles with positive expression of the four molecules were mainly distributed in the nucleus and cytoplasm of fibroblasts (Figure 11).
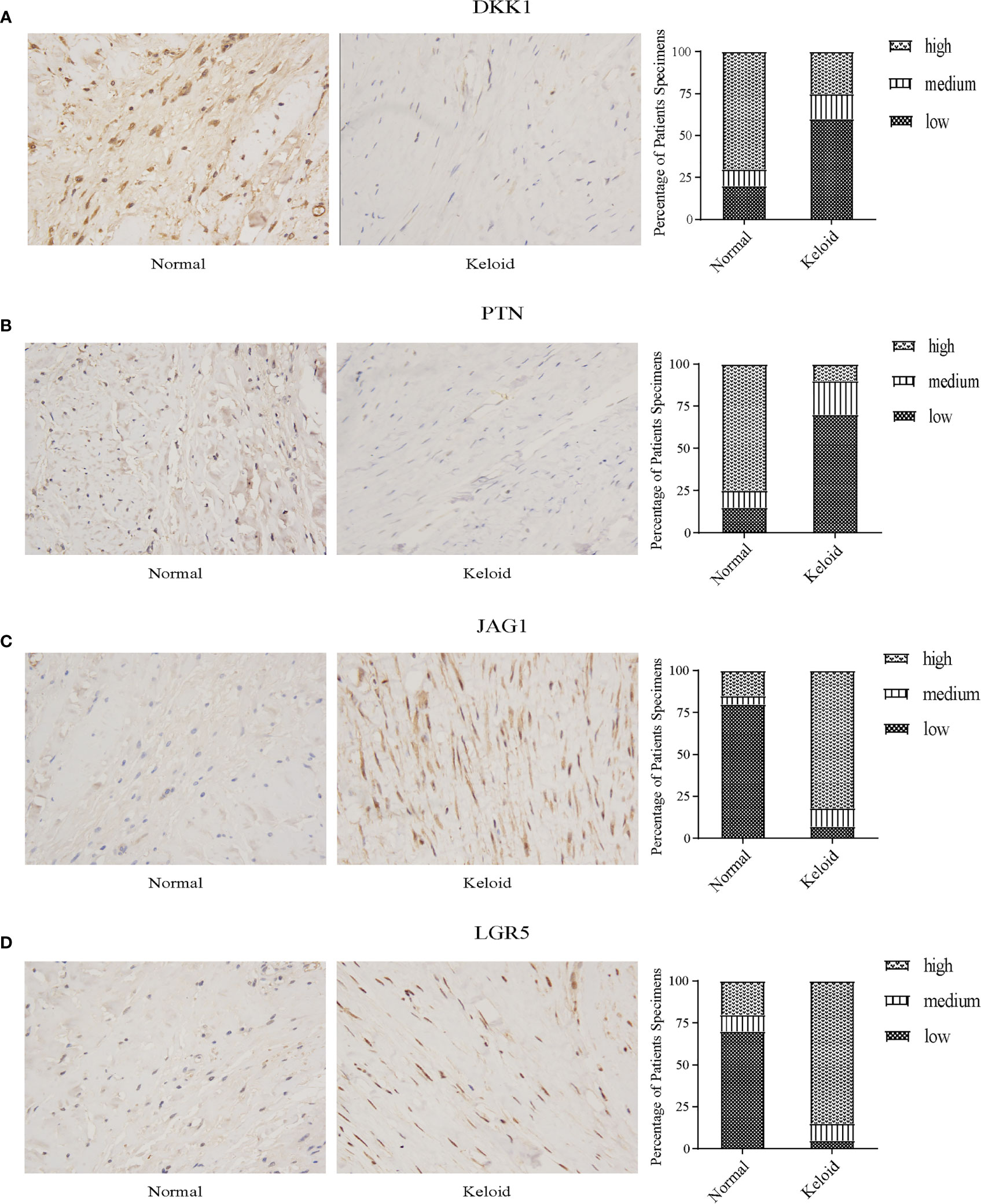
Figure 11 Immunohistochemistry staining and histologic scoring. (A) Expression of DKK1 in normal tissue and keloid. (B) Expression of PTN in normal tissue and keloid. (C) Expression of JAG1 in normal tissue and keloid. (D) Expression of LGR5 in normal tissue and keloid.
Discussion
Keloid is formed from collagen that is produced during a protracted wound healing process (63). They can be divided into the exudation, collagen, and maturation stages (64). In the exudation stage, necrotic tissue is cleared through phagocytosis, removal, and absorption, and the wound is bonded together by the network structure formed by cellulose. In the collagen fiber stage, fibroblasts polymerize to form collagen fibers with the participation of glycine, hydroxyproline, and other substances, which increase the tensile strength of the wound. In the mature stage, collagen fibers are continuously synthesized. At the same time, they are constantly decomposed under the action of collagenase, resulting in degenerative changes in skin scars. Most previous studies have focused on fibroblasts and collagen, but few have emphasized the importance of immunity in keloid Despite intensive research, treatment remains empirical and unsatisfactory. Therefore, it is necessary to identify diagnostic markers that inhibit keloid proliferation and reduce keloid formation. We conducted a comprehensive bioinformatics analysis of 27 keloid samples and 22 normal tissue samples and finally determined that four immune-related signatures were closely related to keloid, and these signatures were related to immunity, stress, and inflammation. A previous study demonstrated that immune-related cells, such as T cells, Th2 cells, and Th1 cells, were upregulated in lesional versus normal skin (65). Here we aimed to explore the potential pathogenesis of keloid-associated immune molecules. We further explained the important role of immunity in keloid through multiple dataset analyses. We found that the expression of DKK1 and PTN was downregulated, while the expression of JAG1 and LGR5 was upregulated in keloid. We obtained the same conclusion through immunohistochemistry. This provides an important reference for future scientific research. We can promote or inhibit the expression of these four molecules through corresponding mechanisms to inhibit the growth of keloid, which will contribute to follow-up clinical research.
The immune system plays an important role in preventing pathogen infiltration, inducing inflammation, initiating downstream processes, and recruiting fibrocytes in keloid (66, 67). Keloid formation is usually considered to be the result of long-term proliferation and a delayed remodeling stage, which is caused by increased fibroblast proliferation and excessive collagen deposition (68, 69). In the dermal thickening of keloid, versican, syndecan-1, fibronectin, thrombospondin-1, tenascin C, CD44, integrin β1, and N-cadherin were immunolocalized in the elongated fibroblasts that were close to the immune cell infiltrate, attached to collagen bundles, and around the microvasculature as well as in some immune cells. Galectin-1/3 was present in the cytoplasm and along the cell membrane of some immune cells and fibroblasts, suggesting that galectin-1/3, in concert with some of the extracellular matrix molecules, was produced to counteract the immune response in keloid. Recent studies have found that the degree of CD3+ cell infiltration in keloid tissue was higher (70). The number of T cells in the tissue is significantly higher than that in peripheral blood and is related to the size of the keloid, suggesting that the occurrence of a keloid is closely related to the role of T cells (71, 72). Macrophages also play an important role in keloid formation. There are two types of macrophages: M1 and M2. M1 plays a role in the early stages of keloid formation, while M2 plays a role in the late stages of keloid formation. In the process of keloid formation, M1 constantly transforms into M2, resulting in the accumulation of a large number of collagen fibers; however, the specific mechanism needs to be further clarified (12). At the same time, we often use glucocorticoids, such as triamcinolone acetonide, locally to treat keloid. Triamcinolone acetonide inhibits the migration of inflammatory cells and reduces fibroblast activity. Most importantly, it can induce lymphocyte DNA degradation and induce lymphocyte apoptosis, thus playing an immunosuppressive role (71, 72). In conclusion, the immune system plays an indispensable role in keloid development. Additionally, keloid formation is closely related to inflammatory responses, especially inflammation-related factors, such as interleukin 6, transforming growth factor-β, and tumor necrosis factor-α and inflammation-related cells, such as neutrophils, mast cells, and lymphocytes (73). The inflammatory response is an adaptive response to adverse stimuli. It can promote fibroblast proliferation and collagen synthesis and eventually develop into a keloid. However, the relationship between inflammatory response and keloid is still in the scientific research stage, and research on the specific mechanism and transformation of the clinical application needs to be further explored.
Here we first focused on immune-related genes and obtained four DEIGs after merging them with the DEGs of GSE7890 and GSE92566, namely LGR5, PTN, JAG1, and DKK1. LGR5 encodes a component of the Wnt receptor complex, which specifically acts as a receptor for a family of Wnt signal agonists named R-spondins. LGR5 is a newly discovered Wnt signal transduction target gene. It is considered a tumor stem cell marker. It is often overexpressed in tumor cells and is strongly associated with tumor formation and development. However, the specific mechanism between LGR5 and the immune-related tumor microenvironment is unclear (74–76). Through the study of keloid in the immunosuppressive microenvironment, exogenous TGF-β1 was induced and LGR5 was highly expressed, but the specific mechanism was not further confirmed. However, this is a potential emerging research direction, which provides new insights for targeted immunotherapy in the future (77). PTN is a member of the heparin-binding cytokine family, which is highly conserved, secretes proteins related to the extracellular matrix, and plays a significant role in cell growth and survival, cell migration, angiogenesis, wound healing, and tumorigenesis (78–80). PTN combined with midkine inactivates the phosphatase activity of receptor protein tyrosine phosphatase to trigger immune responses (81). Huang et al. found that PTN is one of the seven significantly dysregulated genes, which is downregulated in human keloid, but most other genes, such as TGF-β1, TGF-β3, and various collagens, are upregulated in keloid (82, 83). Lee et al. further found that, in keloid, not only the expression of PTN decreased but also the expression of its functional receptor RPTPB/F and upstream regulators was decreased, including PDGF-B, PDGFR-A, and PDGFR-B, which may be a potential novel treatment for keloid in humans (32, 84). JAG1, a ligand for the Notch1 receptor, is involved in Notch signaling. JAG1 participates in the regulation of homeostasis in human tissues and plays an important role in the proliferation, migration, and invasion of fibroblasts (85–87), which can lead to changes in Notch receptor expression in keloid (88). It was found that JAG1 was significantly overexpressed at the mRNA and protein levels in keloid as well as in immune cells. More than 30 years ago, Russell et al. used quantitative real-time polymerase chain reaction to detect the expression level of keloid tissue and normal skin tissue at the same site; increased JAG1 expression was observed in the fibroblasts of keloid nodules (89). Resistance to related drugs, such as hydrocortisone, can be observed in fibroblasts of keloid nodules, which can change the chemistry and morphology of connective tissue cells and hinder the production of intercellular substances, such as collagen fibers. Hydrocortisone can be used to treat keloid by targeting JAG1 (90, 91). DKK1 is an inhibitor of the Wnt signaling pathway, which can regulate Wnt through negative feedback and participates in tumor cell proliferation, apoptosis, migration, and angiogenesis (92, 93). DKK1 binds to the LRP6 co-receptor and inhibits β-catenin-dependent Wnt signaling, and the downregulation of β-catenin is crucial to human immune function (94, 95). Furthermore, DKK1 can regulate immune cells and suppress immune reactions through the GSK3β/E2F1/T-bet axis in CD8+ T cells (96, 97). In a series of studies, it was found that the expression of DKK1 decreased in tumor cells. Keloid is benign skin tumor; therefore, it can be inferred that DKK1 is present at a low level in keloid, which was also confirmed by our previous data analysis and experimental detection (98–100). Moreover, DKK1 regulates the activity of immune cells and participates in T-cell differentiation. Therefore, DKK1 is a promising target for keloid immunotherapy. DKK1 has attracted extensive attention as a mechanism for keloid and immune cells (101). Moreover, in immune infiltration, we identified some immune cells, which were consistent with the available source (67, 102, 103). In a word, we established that four DEIGs played a vital role in the pathogenesis and treatment of keloid; however, the specific mechanism of these four genes in keloid has not been studied. Therefore, it is necessary to conduct further analyses to evaluate the effects of these genes.
The current study has several highlights. First, few studies have linked keloid to immune-related molecules. The expression profiles of normal skin and keloid tissues help us gain a comprehensive understanding of their pathological processes and identify keloid diagnostic biomarkers. Second, DEGs are used to screen immune molecules, which have special advantages in gene expression datasets because they can estimate the connectivity between modules and clinical features. However, our study had some limitations. First, even though we included three datasets, the sample size was small. The data used in this study was obtained from different platforms. Despite the fact that homogenization was conducted, the source bias still be ignored. Finally, this was an in silico analysis, and there is an urgent need for molecular biology experiments and prospective, well-designed, multicenter studies to verify these findings. The specific role of these biomarkers in keloid, their specific association with clinical features (keloid in different periods), and their diagnostic role require further investigation and verification in a large number of clinical patients.
Data availability statement
The datasets presented in this study can be found in online repositories. The names of the repositories and accession numbers can be found in the article.
Ethics statement
Ethical approval was obtained from the review committee of Foshan First People’s Hospital, written informed consent was obtained from the participants prior to sample collection.
Author contributions
XC, SZ, RY, XW, and BL conceived, designed, or planned the idea. XW, BL, JL, XP, PZ, and XZ collected the data. XC, SZ, RY, XW, and BL analyzed the data. All authors interpreted the results. XW and BL drafted the manuscript, and XC, SZ, and RY revised the manuscript. All authors contributed to the article and approved the submitted version.
Funding
This study was supported by the National Natural Science Foundation of China (82002913), Guangdong Basic and Applied Basic Research Foundation (2021A1515011453, 2022A1515012160, 2021B1515120036, and 2022A1515012245) and the Medical Scientific Research Foundation of Guangdong Province (A2022293).
Acknowledgments
We thank the databases mentioned in our study.
Conflict of interest
The authors declare that the research was conducted in the absence of any commercial or financial relationships that could be construed as a potential conflict of interest.
Publisher’s note
All claims expressed in this article are solely those of the authors and do not necessarily represent those of their affiliated organizations, or those of the publisher, the editors and the reviewers. Any product that may be evaluated in this article, or claim that may be made by its manufacturer, is not guaranteed or endorsed by the publisher.
Supplementary material
The Supplementary Material for this article can be found online at: https://www.frontiersin.org/articles/10.3389/fimmu.2022.942446/full#supplementary-material.
Supplementary Table 1 | Details of the immune-related genes.
Abbreviations
DEG, Differentially expressed gene; DEIG, Differentially expressed immune-related gene; DKK1, Dickkopf WNT signaling pathway inhibitor 1; JAG1, Jagged canonical notch ligand 1; LGR5, Leucine-rich repeat containing G protein-coupled receptor 5; miRNA, microRNA; PPI, Protein–protein interaction; PTN, Pleiotrophin.
References
1. Appleton I, Brown N, Willoughby DA. Apoptosis, necrosis, and proliferation: possible implications in the etiology of keloids. Am J Pathol (1996) 149(5):1441–7.
2. Huang C, Akaishi S, Hyakusoku H, Ogawa R. Are keloid and hypertrophic scar different forms of the same disorder? a fibroproliferative skin disorder hypothesis based on keloid findings. Int Wound J (2014) 11(5):517–22. doi: 10.1111/j.1742-481X.2012.01118.x
3. Niessen FB, Spauwen PH, Schalkwijk J, Kon M. On the nature of hypertrophic scars and keloids: a review. Plast Reconstr Surg (1999) 104(5):1435–58. doi: 10.1097/00006534-199910000-00031
4. Fong CY, Biswas A, Subramanian A, Srinivasan A, Choolani M, Bongso A. Human keloid cell characterization and inhibition of growth with human wharton's jelly stem cell extracts. J Cell Biochem (2014) 115(5):826–38. doi: 10.1002/jcb.24724
5. Rabello FB, Souza CD, Farina Junior JA. Update on hypertrophic scar treatment. Clinics (Sao Paulo) (2014) 69(8):565–73. doi: 10.6061/clinics/2014(08)11
6. Ogawa R, Dohi T, Tosa M, Aoki M, Akaishi S. The latest strategy for keloid and hypertrophic scar prevention and treatment: The Nippon medical school (NMS) protocol. J Nippon Med School (2021) 88(1):2–9. doi: 10.1272/jnms.JNMS.2021_88-106
7. Zhang T, Wang X-F, Wang Z-C, Lou D, Fang Q-Q, Hu Y-Y, et al. Current potential therapeutic strategies targeting the TGF-β/Smad signaling pathway to attenuate keloid and hypertrophic scar formation. Biomed Pharmacother (2020) 129, 110287. doi: 10.1016/j.biopha.2020.110287
8. Yagmur C, Akaishi S, Ogawa R, Guneren E. Mechanical receptor–related mechanisms in scar management: A review and hypothesis. Plast Reconstr Surg (2010) 126(2):426–34. doi: 10.1097/PRS.0b013e3181df715d
9. Wang X-Q, Song F, Liu Y-K. Hypertrophic scar regression is linked to the occurrence of endothelial dysfunction. PloS One (2017) 12(5):e0176681. doi: 10.1371/journal.pone.0176681
10. Bond JS, Duncan JAL, Mason T, Sattar A, Boanas A, O’Kane S, et al. Scar redness in humans: How long does it persist after incisional and excisional wounding? Plast Reconstructive Surg (2008) 121(2):487–96. doi: 10.1097/01.prs.0000299183.88334.37
11. Wang Z-C, Zhao W-Y, Cao Y, Liu Y-Q, Sun Q, Shi P, et al. The roles of inflammation in keloid and hypertrophic scars. Front Immunol (2020), 11, 603187. doi: 10.3389/fimmu.2020.603187
12. Williams EA, Thaller SR. The role of fat grafting in the treatment of keloid scars and venous ulcers. J Craniofac Surg (2019) 30(3):696–7. doi: 10.1097/SCS.0000000000005208
13. Scala J, Vojvodic A, Vojvodic P, Vlaskovic-Jovicevic T, Peric-Hajzler Z, Matovic D, et al. Botulin toxin use in Scars/Keloids treatment. Open Access Maced J Med Sci (2019) 7(18):2979–81. doi: 10.3889/oamjms.2019.783
14. Brown BC, McKenna SP, Siddhi K, McGrouther DA, Bayat A. The hidden cost of skin scars: quality of life after skin scarring. J Plast Reconstr Aesthet Surg (2008) 61(9):1049–58. doi: 10.1016/j.bjps.2008.03.020
15. Bayat A, McGrouther DA, Ferguson MW. Skin scarring. BMJ (2003) 326(7380):88–92. doi: 10.1136/bmj.326.7380.88
16. Berman B, Maderal A, Raphael B. Keloids and hypertrophic scars: Pathophysiology, classification, and treatment. Dermatol Surg (2017), 43, S3–S18. doi: 10.1097/DSS.0000000000000819
17. Lee JY-Y, Yang C-C, Chao S-C, Wong T-W. Histopathological differential diagnosis of keloid and hypertrophic scar. Am J Dermatopathol (2004) 26(5):379–84. doi: 10.1097/00000372-200410000-00006
18. Gold MH, Berman B, Clementoni MT, Gauglitz GG, Nahai F, Murcia C. Updated international clinical recommendations on scar management: part 1–evaluating the evidence. Dermatol Surg (2014) 40(8):817–24. doi: 10.1111/dsu.0000000000000049
19. Gold MH, McGuire M, Mustoe TA, Pusic A, Sachdev M, Waibel J, et al. Updated international clinical recommendations on scar management: part 2–algorithms for scar prevention and treatment. Dermatol Surg (2014) 40(8):825–31. doi: 10.1111/dsu.0000000000000050
20. Kim S, Choi TH, Liu W, Ogawa R, Suh JS, Mustoe TA. Update on scar management: guidelines for treating Asian patients. Plast Reconstr Surg (2013) 132(6):1580–9. doi: 10.1097/PRS.0b013e3182a8070c
21. Janssen de Limpens AM, Cormane RH. Studies on the immunologic aspects of keloids and hypertrophic scars. Arch Dermatol Res (1982) 274(3-4):259–66. doi: 10.1007/BF00403728
22. Kischer CW, Shetlar MR, Shetlar CL, Chvapil M. Immunoglobulins in hypertrophic scars and keloids. Plast Reconstr Surg (1983) 71(6):821–5. doi: 10.1097/00006534-198306000-00015
23. Bagabir R, Byers RJ, Chaudhry IH, Muller W, Paus R, Bayat A. Site-specific immunophenotyping of keloid disease demonstrates immune upregulation and the presence of lymphoid aggregates. Br J Dermatol (2012) 167(5):1053–66. doi: 10.1111/j.1365-2133.2012.11190.x
24. Jiao H, Fan J, Cai J, Pan B, Yan L, Dong P, et al. Analysis of characteristics similar to autoimmune disease in keloid patients. Aesthetic Plast Surg (2015) 39(5):818–25. doi: 10.1007/s00266-015-0542-4
25. Klotz T, Munn Z, Aromataris EC, Greenwood JE. Imiquimod to prevent keloid recurrence postexcision: A systematic review and meta-analysis. Wound Repair Regener (2020) 28(1):145–56. doi: 10.1111/wrr.12766
26. Sandulache VC, Parekh A, Li-Korotky H, Dohar JE, Hebda PA. Prostaglandin E2 inhibition of keloid fibroblast migration, contraction, and transforming growth factor (TGF)-beta1-induced collagen synthesis. Wound Repair Regener (2007) 15(1):122–33. doi: 10.1111/j.1524-475X.2006.00193.x
27. Zhang L, Luo H, Meng W, Cen Y, Huang Q, Li H, et al. Integration of flow cytometry and computational analysis to dissect the epidermal cellular subsets in keloids that correlate with recurrence. J Invest Dermatol (2021) 141, 2521–29e4. doi: 10.1016/j.jid.2021.03.022
28. Shen R, Li P, Li B, Zhang B, Feng L, Cheng S. Identification of distinct immune subtypes in colorectal cancer based on the stromal compartment. Front Oncol (2019) 91497:1497. doi: 10.3389/fonc.2019.01497
29. Tang L, Peng C, Zhu SS, Zhou Z, Liu H, Cheng Q, et al. Tre2-Bub2-Cdc16 family proteins based nomogram serve as a promising prognosis predicting model for melanoma. Front Oncol (2020) 10579625:579625. doi: 10.3389/fonc.2020.579625
30. Zhang Z, Li J, He T, Ding J. Bioinformatics identified 17 immune genes as prognostic biomarkers for breast cancer: Application study based on artificial intelligence algorithms. Front Oncol (2020) 10330:330. doi: 10.3389/fonc.2020.00330
31. Zhao J, Guo C, Xiong F, Yu J, Ge J, Wang H, et al. Single cell RNA-seq reveals the landscape of tumor and infiltrating immune cells in nasopharyngeal carcinoma. Cancer Lett (2020), 10, 330. doi: 10.1016/j.canlet.2020.02.010
32. Smith JC, Boone BE, Opalenik SR, Williams SM, Russell SB. Gene profiling of keloid fibroblasts shows altered expression in multiple fibrosis-associated pathways. J Invest Dermatol (2008) 128(5):1298–310. doi: 10.1038/sj.jid.5701149
33. Fuentes-Duculan J, Bonifacio KM, Suarez-Farinas M, Kunjravia N, Garcet S, Cruz T, et al. Aberrant connective tissue differentiation towards cartilage and bone underlies human keloids in African americans. Exp Dermatol (2017) 26(8):721–7. doi: 10.1111/exd.13271
34. Hahn JM, Glaser K, McFarland KL, Aronow BJ, Boyce ST, Supp DM. Keloid-derived keratinocytes exhibit an abnormal gene expression profile consistent with a distinct causal role in keloid pathology. Wound Repair Regener (2013) 21(4):530–44. doi: 10.1111/wrr.12060
35. Barrett T, Wilhite SE, Ledoux P, Evangelista C, Kim IF, Tomashevsky M, et al. NCBI GEO: archive for functional genomics data sets–update. Nucleic Acids Res (2013) 41(Database issue):D991–995. doi: 10.1093/nar/gks1193
36. Zhou JG, Liang B, Jin SH, Liao HL, Du GB, Cheng L, et al. Development and validation of an RNA-Seq-Based prognostic signature in neuroblastoma. Front Oncol (2019) 91361:1361. doi: 10.3389/fonc.2019.01361
37. Liberzon A, Birger C, Thorvaldsdottir H, Ghandi M, Mesirov JP, Tamayo P. The molecular signatures database (MSigDB) hallmark gene set collection. Cell Syst (2015) 1(6):417–25. doi: 10.1016/j.cels.2015.12.004
38. Godec J, Tan Y, Liberzon A, Tamayo P, Bhattacharya S, Butte AJ, et al. Compendium of immune signatures identifies conserved and species-specific biology in response to inflammation. Immunity (2016) 44(1):194–206. doi: 10.1016/j.immuni.2015.12.006
39. Subramanian A, Tamayo P, Mootha VK, Mukherjee S, Ebert BL, Gillette MA, et al. Gene set enrichment analysis: a knowledge-based approach for interpreting genome-wide expression profiles. Proc Natl Acad Sci U.S.A. (2005) 102(43):15545–50. doi: 10.1073/pnas.0506580102
40. Bhattacharya S, Dunn P, Thomas CG, Smith B, Schaefer H, Chen J, et al. ImmPort, toward repurposing of open access immunological assay data for translational and clinical research. Sci Data (2018) 5, 80015. doi: 10.1038/sdata.2018.15
41. Gautier L, Cope L, Bolstad BM, Irizarry RA. Affy–analysis of affymetrix GeneChip data at the probe level. Bioinformatics (2004) 20(3):307–15. doi: 10.1093/bioinformatics/btg405
42. Ritchie ME, Phipson B, Wu D, Hu Y, Law CW, Shi W, et al. Limma powers differential expression analyses for RNA-sequencing and microarray studies. Nucleic Acids Res (2015) 43(7):e47. doi: 10.1093/nar/gkv007
43. Yu G, Li F, Qin Y, Bo X, Wu Y, Wang S. GOSemSim: an r package for measuring semantic similarity among GO terms and gene products. Bioinformatics (2010) 26(7):976–8. doi: 10.1093/bioinformatics/btq064
44. von Mering C, Huynen M, Jaeggi D, Schmidt S, Bork P, Snel B. STRING: a database of predicted functional associations between proteins. Nucleic Acids Res (2003) 31(1):258–61. doi: 10.1093/nar/gkg034
45. Bader GD andHogue CWV. An automated method for finding molecular complexes in large protein interaction networks. BMC Bioinf (2003) 4, 2. doi: 10.1186/1471-2105-4-2
46. Shannon P, Markiel A, Ozier O, Baliga NS, Wang JT, Ramage D, et al. Cytoscape: a software environment for integrated models of biomolecular interaction networks. Genome Res (2003) 13(11):2498–504. doi: 10.1101/gr.1239303
47. Liang B, Zhang XX, Gu N. Virtual screening and network pharmacology-based synergistic mechanism identification of multiple components contained in guanxin V against coronary artery disease. BMC Complement Med Ther (2020) 20(1):345. doi: 10.1186/s12906-020-03133-w
48. Liang B, Liang Y, Gu N. Pharmacological mechanisms of sodium-glucose co-transporter 2 inhibitors in heart failure with preserved ejection fraction. BMC Cardiovasc Disord (2022) 22(1):261. doi: 10.1186/s12872-022-02693-8
49. Yu G, Wang LG, Han Y, He QY. clusterProfiler: an r package for comparing biological themes among gene clusters. OMICS (2012) 16(5):284–7. doi: 10.1089/omi.2011.0118
50. Hanzelmann S, Castelo R, Guinney J. GSVA: gene set variation analysis for microarray and RNA-seq data. BMC Bioinf (2013) 14, 7. doi: 10.1186/1471-2105-14-7
51. Bello SM, Shimoyama M, Mitraka E, Laulederkind SJF, Smith CL, Eppig JT, et al. Disease ontology: improving and unifying disease annotations across species. Dis Model Mech (2018) 11(3). doi: 10.1242/dmm.032839
52. Ashburner M, Ball CA, Blake JA, Botstein D, Butler H, Cherry JM, et al. Gene ontology: tool for the unification of biology. Gene Ontol Consortium Nat Genet (2000) 25(1):25–9. doi: 10.1038/75556
53. Kanehisa M, Furumichi M, Tanabe M, Sato Y, Morishima K. KEGG: new perspectives on genomes, pathways, diseases and drugs. Nucleic Acids Res (2017) 45(D1):D353–61. doi: 10.1093/nar/gkw1092
54. Uhlen M, Fagerberg L, Hallstrom BM, Lindskog C, Oksvold P, Mardinoglu A, et al. Proteomics. tissue-based map of the human proteome. Science (2015) 347(6220):1260419. doi: 10.1126/science.1260419
55. Tang Z, Li C, Kang B, Gao G, Li C, Zhang Z. GEPIA: a web server for cancer and normal gene expression profiling and interactive analyses. Nucleic Acids Res (2017) 45(W1):W98–W102. doi: 10.1093/nar/gkx247
56. Keenan AB, Torre D, Lachmann A, Leong AK, Wojciechowicz ML, Utti V, et al. ChEA3: transcription factor enrichment analysis by orthogonal omics integration. Nucleic Acids Res (2019) 47(W1):W212–24. doi: 10.1093/nar/gkz446
57. Li JH, Liu S, Zhou H, Qu LH, Yang JH. starBase v2.0: decoding miRNA-ceRNA, miRNA-ncRNA and protein-RNA interaction networks from large-scale CLIP-seq data. Nucleic Acids Res (2014) 42(Database issue):D92–97. doi: 10.1093/nar/gkt1248
58. Cotto KC, Wagner AH, Feng YY, Kiwala S, Coffman AC, Spies G, et al. DGIdb 3.0: a redesign and expansion of the drug-gene interaction database. Nucleic Acids Res (2018) 46(D1):D1068–73. doi: 10.1093/nar/gkx1143
59. Chen B, Khodadoust MS, Liu CL, Newman AM, Alizadeh AA. Profiling tumor infiltrating immune cells with CIBERSORT. Methods Mol Biol (2018), 1711, 243–59. doi: 10.1007/978-1-4939-7493-1_12
60. Liang B, Zhang X-X, Li R, Gu N. Guanxin V protects against ventricular remodeling after acute myocardial infarction through the interaction of TGF-β1 and vimentin. Phytomedicine (2022), 95, 153866. doi: 10.1016/j.phymed.2021.153866
61. Zhang X-X, Shao C-L, Cheng S-Y, Zhu Y, Liang B, Gu N. Effect of guanxin V in animal model of acute myocardial infarction. BMC Complement Med Ther (2021) 21(1):72. doi: 10.1186/s12906-021-03211-7
62. Zhou S, Liang Y, Zhang X, Liao L, Yang Y, Ouyang W, et al. SHARPIN promotes melanoma progression via Rap1 signaling pathway. J Invest Dermatol (2020) 140(2):395–403 e396. doi: 10.1016/j.jid.2019.07.696
63. Limandjaja GC, Niessen FB, Scheper RJ, Gibbs S. The keloid disorder: Heterogeneity, histopathology, mechanisms and models. Front Cell Dev Biol (2020) 8360:360. doi: 10.3389/fcell.2020.00360
64. Monstrey S, Middelkoop E, Vranckx JJ, Bassetto F, Ziegler UE, Meaume S, et al. Updated scar management practical guidelines: non-invasive and invasive measures. J Plast Reconstr Aesthet Surg (2014) 67(8):1017–25. doi: 10.1016/j.bjps.2014.04.011
65. Wu J, Del Duca E, Espino M, Gontzes A, Cueto I, Zhang N, et al. RNA Sequencing keloid transcriptome associates keloids with Th2, Th1, Th17/Th22, and JAK3-skewing. Front Immunol (2020) 11597741:597741. doi: 10.3389/fimmu.2020.597741
66. Chen Y, Jin Q, Fu X, Qiao J, Niu F. Connection between T regulatory cell enrichment and collagen deposition in keloid. Exp Cell Res (2019) 383(2):111549. doi: 10.1016/j.yexcr.2019.111549
67. Murao N, Seino K, Hayashi T, Ikeda M, Funayama E, Furukawa H, et al. Treg-enriched CD4+ T cells attenuate collagen synthesis in keloid fibroblasts. Exp Dermatol (2014) 23(4):266–71. doi: 10.1111/exd.12368
68. Funayama E, Chodon T, Oyama A, Sugihara T. Keratinocytes promote proliferation and inhibit apoptosis of the underlying fibroblasts: an important role in the pathogenesis of keloid. J Invest Dermatol (2003) 121(6):1326–31. doi: 10.1111/j.1523-1747.2003.12572.x
69. Chipev CC, Simman R, Hatch G, Katz AE, Siegel DM, Simon M. Myofibroblast phenotype and apoptosis in keloid and palmar fibroblasts in vitro. Cell Death Differ (2000) 7(2):166–76. doi: 10.1038/sj.cdd.4400605
70. Arciniegas E, Carrillo LM, Rojas H, Ramirez R, Chopite M. Galectin-1 and galectin-3 and their potential binding partners in the dermal thickening of keloid tissues. Am J Dermatopathol (2019) 41(3):193–204. doi: 10.1097/DAD.0000000000001284
71. Zhuang Z, Li Y, Wei X. The safety and efficacy of intralesional triamcinolone acetonide for keloids and hypertrophic scars: A systematic review and meta-analysis. Burns (2021) 47(5):987–98. doi: 10.1016/j.burns.2021.02.013
72. Hochman B, Locali RF, Matsuoka PK, Ferreira LM. Intralesional triamcinolone acetonide for keloid treatment: a systematic review. Aesthetic Plast Surg (2008) 32(4):705–9. doi: 10.1007/s00266-008-9152-8
73. Nangole FW, Ouyang K, Anzala O, Ogengo J, Agak GW. Multiple cytokines elevated in patients with keloids: Is it an indication of auto-inflammatory disease? J Inflammation Res (2021), 14, 2465–70. doi: 10.2147/JIR.S312091
74. Hsu SY, Liang SG, Hsueh AJ. Characterization of two LGR genes homologous to gonadotropin and thyrotropin receptors with extracellular leucine-rich repeats and a G protein-coupled, seven-transmembrane region. Mol Endocrinol (1998) 12(12):1830–45. doi: 10.1210/mend.12.12.0211
75. Barker N, van Es JH, Kuipers J, Kujala P, van den Born M, Cozijnsen M, et al. Identification of stem cells in small intestine and colon by marker gene Lgr5. Nature (2007) 449(7165):1003–7. doi: 10.1038/nature06196
76. Kemper K, Prasetyanti PR, De Lau W, Rodermond H, Clevers H, Medema JP. Monoclonal antibodies against Lgr5 identify human colorectal cancer stem cells. Stem Cells (2012) 30(11):2378–86. doi: 10.1002/stem.1233
77. Liu XS, Lin XK, Mei Y, Ahmad S, Yan CX, Jin HL, et al. Regulatory T cells promote overexpression of Lgr5 on gastric cancer cells via TGF-beta1 and confer poor prognosis in gastric cancer. Front Immunol (2019) 101741:1741. doi: 10.3389/fimmu.2019.01741
78. Papadimitriou E, Mikelis C, Lampropoulou E, Koutsioumpa M, Theochari K, Tsirmoula S, et al. Roles of pleiotrophin in tumor growth and angiogenesis. Eur Cytokine Netw (2009) 20(4):180–90. doi: 10.1684/ecn.2009.0172
79. Giddings BH, Kwong SL, Parikh-Patel A, Bates JH, Snipes KP. Going against the tide: increasing incidence of colorectal cancer among koreans, filipinos, and south asians in California, 1988-2007. Cancer Causes Control (2012) 23(5):691–702. doi: 10.1007/s10552-012-9937-6
80. Mikelis C, Koutsioumpa M, Papadimitriou E. Pleiotrophin as a possible new target for angiogenesis-related diseases and cancer. Recent Pat Anticancer Drug Discovery (2007) 2(2):175–86. doi: 10.2174/157489207780832405
81. Herradon G, Ramos-Alvarez MP, Gramage E. Connecting metainflammation and neuroinflammation through the PTN-MK-RPTPbeta/zeta axis: Relevance in therapeutic development. Front Pharmacol (2019) 10377:377. doi: 10.3389/fphar.2019.00377
82. Huang C, Nie F, Qin Z, Li B, Zhao X. A snapshot of gene expression signatures generated using microarray datasets associated with excessive scarring. Am J Dermatopathol (2013) 35(1):64–73. doi: 10.1097/DAD.0b013e31825ba13f
83. Lee DH, Jin CL, Kim Y, Shin MH, Kim JE, Kim M, et al. Pleiotrophin is downregulated in human keloids. Arch Dermatol Res (2016) 308(8):585–91. doi: 10.1007/s00403-016-1678-z
84. Arjunan S, Gan SU, Choolani M, Raj V, Lim J, Biswas A, et al. Inhibition of growth of Asian keloid cells with human umbilical cord wharton's jelly stem cell-conditioned medium. Stem Cell Res Ther (2020) 11(1):78. doi: 10.1186/s13287-020-01609-7
85. Bolos V, Grego-Bessa J, de la Pompa JL. Notch signaling in development and cancer. Endocr Rev (2007) 28(3):339–63. doi: 10.1210/er.2006-0046
86. Chigurupati S, Arumugam TV, Son TG, Lathia JD, Jameel S, Mughal MR, et al. Involvement of notch signaling in wound healing. PloS One (2007) 2(11):e1167. doi: 10.1371/journal.pone.0001167
87. Allenspach EJ, Maillard I, Aster JC, Pear WS. Notch signaling in cancer. Cancer Biol Ther (2002) 1(5):466–76. doi: 10.4161/cbt.1.5.159
88. Syed F, Bayat A. Notch signaling pathway in keloid disease: enhanced fibroblast activity in a jagged-1 peptide-dependent manner in lesional vs. extralesional fibroblasts. Wound Repair Regener (2012) 20(5):688–706. doi: 10.1111/j.1524-475X.2012.00823.x
89. Russell SB, Russell JD, Trupin KM, Gayden AE, Opalenik SR, Nanney LB, et al. Epigenetically altered wound healing in keloid fibroblasts. J Invest Dermatol (2010) 130(10):2489–96. doi: 10.1038/jid.2010.162
90. Wang H, Quan L, Liang J, Shi J, Qiu T, Zhang Y, et al. Gene expression profiling analysis of keloids with and without hydrocortisone treatment. Exp Ther Med (2017) 14(6):5283–8. doi: 10.3892/etm.2017.5263
91. Condorelli AG, Logli E, Cianfarani F, Teson M, Diociaiuti A, El Hachem M, et al. MicroRNA-145-5p regulates fibrotic features of recessive dystrophic epidermolysis bullosa skin fibroblasts. Br J Dermatol (2019) 181(5):1017–27. doi: 10.1111/bjd.17840
92. Wang K, Zhang Y, Li X, Chen L, Wang H, Wu J, et al. Characterization of the kremen-binding site on Dkk1 and elucidation of the role of kremen in dkk-mediated wnt antagonism. J Biol Chem (2008) 283(34):23371–5. doi: 10.1074/jbc.M802376200
93. Grotewold L, Theil T, Ruther U. Expression pattern of dkk-1 during mouse limb development. Mech Dev (1999) 89(1-2):151–3. doi: 10.1016/s0925-4773(99)00194-x
94. Ueland T, Astrup E, Otterdal K, Lekva T, Janardhanan J, Prakash JAJ, et al. Secreted wnt antagonists in scrub typhus. PloS Negl Trop Dis (2021) 15(4):e0009185. doi: 10.1371/journal.pntd.0009185
95. Aslam N, Abusharieh E, Abuarqoub D, Ali D, Al-Hattab D, Wehaibi S, et al. Anti-oncogenic activities exhibited by paracrine factors of MSCs can be mediated by modulation of KITLG and DKK1 genes in glioma SCs in vitro. Mol Ther Oncolytics (2021), 20, 147–65. doi: 10.1016/j.omto.2020.11.005
96. Sui Q, Liu D, Jiang W, Tang J, Kong L, Han K, et al. Dickkopf 1 impairs the tumor response to PD-1 blockade by inactivating CD8+ T cells in deficient mismatch repair colorectal cancer. J Immunother Cancer (2021) 9(3). doi: 10.1136/jitc-2020-001498
97. D'Amico L, Mahajan S, Capietto AH, Yang Z, Zamani A, Ricci B, et al. Dickkopf-related protein 1 (Dkk1) regulates the accumulation and function of myeloid derived suppressor cells in cancer. J Exp Med (2016) 213(5):827–40. doi: 10.1084/jem.20150950
98. Kikuchi A, Matsumoto S, Sada R. Dickkopf signaling, beyond wnt-mediated biology. Semin Cell Dev Biol (2022), 125, 55–65. doi: 10.1016/j.semcdb.2021.11.003
99. Aguilera O, Fraga MF, Ballestar E, Paz MF, Herranz M, Espada J, et al. Epigenetic inactivation of the wnt antagonist DICKKOPF-1 (DKK-1) gene in human colorectal cancer. Oncogene (2006) 25(29):4116–21. doi: 10.1038/sj.onc.1209439
100. Maehata T, Taniguchi H, Yamamoto H, Nosho K, Adachi Y, Miyamoto N, et al. Transcriptional silencing of dickkopf gene family by CpG island hypermethylation in human gastrointestinal cancer. World J Gastroenterol (2008) 14(17):2702–14. doi: 10.3748/wjg.14.2702
101. Chu HY, Chen Z, Wang L, Zhang ZK, Tan X, Liu S, et al. Dickkopf-1: A promising target for cancer immunotherapy. Front Immunol (2021), 12, 658097. doi: 10.3389/fimmu.2021.658097
102. Jin Q, Gui L, Niu F, Yu B, Lauda N, Liu J, et al. Macrophages in keloid are potent at promoting the differentiation and function of regulatory T cells. Exp Cell Res (2018) 362(2):472–6. doi: 10.1016/j.yexcr.2017.12.011
Keywords: immune, signature, keloid, GEO, immune infiltration
Citation: Wang X, Liang B, Li J, Pi X, Zhang P, Zhou X, Chen X, Zhou S and Yang R (2022) Identification and characterization of four immune-related signatures in keloid. Front. Immunol. 13:942446. doi: 10.3389/fimmu.2022.942446
Received: 12 May 2022; Accepted: 27 June 2022;
Published: 27 July 2022.
Edited by:
Lucienne Chatenoud, Université Paris Descartes, FranceReviewed by:
Xie Julin, First Affiliated Hospital, Sun Yat-sen University, ChinaKun Xiong, Central South University, China
Xiao Long, Peking Union Medical College Hospital (CAMS), China
Copyright © 2022 Wang, Liang, Li, Pi, Zhang, Zhou, Chen, Zhou and Yang. This is an open-access article distributed under the terms of the Creative Commons Attribution License (CC BY). The use, distribution or reproduction in other forums is permitted, provided the original author(s) and the copyright owner(s) are credited and that the original publication in this journal is cited, in accordance with accepted academic practice. No use, distribution or reproduction is permitted which does not comply with these terms.
*Correspondence: Xiaodong Chen, Y3hkMjM0QDE2My5jb20=; Sitong Zhou, c2l0b25nemhvdUBob3RtYWlsLmNvbQ==; Ronghua Yang, MjE3MjAwOTFAcXEuY29t
†These authors have contributed equally to this work