- 1Physical and Life Sciences Directorate, Lawrence Livermore National Laboratory, Livermore, CA, United States
- 2School of Natural Sciences, University of California Merced, Merced, CA, United States
- 3Department of Orthopaedic Surgery, University of California Davis Health, Sacramento, CA, United States
Osteoarthritis (OA) is the most common joint disease, affecting over 300 million people world-wide. Accumulating evidence attests to the important roles of the immune system in OA pathogenesis. Understanding the role of various immune cells in joint degeneration or joint repair after injury is vital for improving therapeutic strategies for treating OA. Post-traumatic osteoarthritis (PTOA) develops in ~50% of individuals who have experienced an articular trauma like an anterior cruciate ligament (ACL) rupture. Here, using the high resolution of single-cell RNA sequencing, we delineated the temporal dynamics of immune cell accumulation in the mouse knee joint after ACL rupture. Our study identified multiple immune cell types in the joint including neutrophils, monocytes, macrophages, B cells, T cells, NK cells and dendritic cells. Monocytes and macrophage populations showed the most dramatic changes after injury. Further characterization of monocytes and macrophages reveled 9 major subtypes with unique transcriptomics signatures, including a tissue resident Lyve1hiFolr2hi macrophage population and Trem2hiFcrls+ recruited macrophages, both showing enrichment for phagocytic genes and growth factors such as Igf1, Pdgfa and Pdgfc. We also identified several genes induced or repressed after ACL injury in a cell type-specific manner. This study provides new insight into PTOA-associated changes in the immune microenvironment and highlights macrophage subtypes that may play a role in joint repair after injury.
Introduction
Osteoarthritis (OA) is a severely debilitating joint disease characterized by progressive cartilage loss, bone remodeling, synovial inflammation, and significant joint pain. Historically, OA was considered a purely mechanical disease caused by cartilage wear and tear or injury (1), while rheumatoid arthritis (RA) was deemed a disease caused by excessive joint inflammation (2). However, recent studies have highlighted that inflammation and immune system changes play a role in OA pathogenesis (3–5). Several innate and adaptive immune cell types have been proposed to contribute to OA pathogenesis including macrophages, neutrophils, B cells, T cells and dendritic cells (DCs) (6–9). However, their precise role in OA initiation or progression is still poorly understood. Identifying and characterizing the immune cell subtypes found in the synovial joint during steady state, after injury and during progression to OA will provide insights into disease etiology and treatment options.
Our immune system is a highly complex defense system, activated in response to infection, illness, and injury. Recent studies employing single-cell RNA-sequence (scRNA-seq) analyses of the immune cell infiltrate in various pathological conditions including RA have shed new light on immune cell heterogeneity and their complex functions (10–12). However, only a limited number of studies have attempted to characterize OA-associated immune changes at single-cell level, longitudinally. Recently, using scRNA-seq Chou et al. identified heterogeneous immune populations in human OA synovium including immune regulatory (IR-MΦ) macrophages, inflammatory macrophages (I-MΦ) expressing high levels of class II MHC genes, DCs, B cells, T cells and mast cells (13). This study was performed on patients with advanced OA, all undergoing knee arthroplasty, which prevented the identification of immune changes associated with OA initiation or early stages of joint degeneration. Determining the functional heterogeneity of various immune cell types and elucidating molecular interactions between the immune system and various other cell types in the joint microenvironment that may drive the progressive intra-articular degradation is crucial to understanding OA pathogenesis.
Joint injury is a major risk factor for the development of OA and post-traumatic osteoarthritis and (PTOA) accounts for about 12% of all OA cases (14). Joint injuries including intra-articular fractures and meniscal, ligamentous and chondral injuries have been identified as initiating factors for PTOA (14). Clinical records indicate that more than 50% of individuals with an anterior cruciate ligament (ACL) injury go on to develop PTOA (15). After trauma, an inflammatory response occurs at the site of injury resulting in cellular and molecular changes including synovial cellular infiltration and inflammatory mediator production (16). Transcriptomic analyses in rodent OA models from our group as well as others have revealed the upregulation of many genes encoding for inflammatory cytokines after ACL rupture or surgical destabilization of the medial meniscus (DMM) including Ccl2, Ccl7, Il6 and Il33 (17–19). Inflammatory cytokines have been shown to promote the expression of enzymatic mediators of cartilage degeneration including MMPs and ADAMs, which can lead to adverse tissue remodeling and joint dysfunction (20). It is likely that PTOA is driven by a dysregulated repair and remodeling process. Therefore, delineating injury-induced immune changes in the joint that lead to the production of pro- and anti-degenerative factors is crucial to understand the molecular mechanisms driving progressive joint degeneration after injury and will help us identify therapeutic targets for blunting these unwarranted effects.
In this study, we investigated the immune cell landscape of the knee in a murine injury model at a single-cell resolution and determined injury-induced changes. Knee joint injury was induced in C57BL/6 (BL6) mice using a non-invasive tibial compression (TC) injury model that closely mimics ACL rupture in humans (21). In this model, BL6 consistently show significant cartilage degeneration by 6 weeks post-injury (22–26). Subsequently, we isolated immune cells from knee joints at day 0 [D0: uninjured], D1, D3, D7, D15 and D30 post-injury and profiled using scRNA-seq to characterize the immune compartment in the joint and determine the ACL-injury induced changes. Special emphasis was given to analyzing monocyte and macrophage populations, which significantly expanded after injury. We identified nine monocyte/macrophage subtypes with unique transcriptomic signatures, including a tissue-resident Lyve1hiFolr2hi macrophage and a tissue-recruited Trem2hiFcrls+ macrophage subpopulation with potential chondro-protective functions, and characterized their gene expression profiles as a function of disease progression. We also examined Trem2-/- mice using bulk RNA-seq to determine the potential role of Trem2 receptor in macrophage differentiation or function after injury. Additionally, we characterized injury-induced changes in neutrophil and lymphocyte populations. The detailed cellular and molecular level characterization provided in our study will improve the understanding of the functions of distinct immune subtypes and will facilitate identification of new prognostic and therapeutic targets for PTOA.
Methods
ACL injury model
10-week-old male C57Bl/6J (BL6) (Jackson Laboratory Bar Harbor, ME, USA; Stock No: 000664) or Trem2-/- mice (C57BL/6J-Trem2em2Adiuj/J; Jackson Laboratory Bar Harbor, ME, USA; Stock No: 027197) were subjected to an ACL injury using a non-invasive single dynamic tibial compressive overload model previously described by Christiansen et al. (21). Briefly, the mouse knee was placed between two vertically aligned plates positioned using an electromagnetic material testing system (ElectroForce 3200, TA Instruments, New Castle, DE, USA) and a compressive force was applied (21). ACL rupture occurred after a total compressive force, between 12N-18N, was administered. For pain relief, buprenorphine (0.01 mg/kg) and sterile saline were administered intraperitoneally immediately post-injury. All animal experiments were approved by the Lawrence Livermore National Laboratory and University of California, Davis Institutional Animal Care and Use Committee and conformed to the Guide for the care and use of laboratory animals.
Histological assessment of the articular joint
Knee joints were collected from uninjured and injured mice at 4-weeks post-injury and processed for histological evaluation as previously described (22). Briefly, whole joints were fixed in 10% Neutral Buffered Formalin (NBF), decalcified using 0.5 M EDTA, and processed for paraffin embedding. Joints were sectioned in the sagittal plane at 6µm and serial medial sections that included the femoral condyles, menisci, and tibial plateaus were prepared for histological assessment of joint tissue integrity. Sections were stained on glass slides using 0.1% Safranin-O (0.1%, Sigma, St. Louis, MO, USA; S8884) and 0.05% Fast Green (0.05%, Sigma, St. Louis, MO, USA; F7252) using standard procedures (IHC World, Woodstock, MD, USA), then imaged using a Leica DM5000 microscope.
Immunohistochemistry
Sagittal sections from D0, D7, D30 knee joints of BL6 mice were used for IHC. Primary antibodies were incubated overnight at 4°C in a dark, humid chamber following antigen retrieval with Unitrieve (NB325 Innovex Biosciences, Richmond, CA. USA). Secondary antibodies were incubated for 2 hours at room temperature in a dark, humid chamber at 1:500. Negative control slides were incubated with secondary antibody-only. Stained slides were mounted with Prolong Gold with DAPI for nuclei staining (Molecular Probes, Eugene, OR. USA). Slides were imaged using a Leica DM5000 microscope. ImagePro Plus V7.0 Software, QIClick CCD camera (QImaging, Surrey, BC, Canada) and ImageJ V1.53 Software were used for imaging and photo editing. Primary antibodies include: Lyve1 [1:100 (5.5µg/mL); ab218535 Abcam, Cambridge, UK], Trem2 [1:100 (5.0µg/mL); ab95470 Abcam, Cambridge, UK], CD206 [1:100 (10µg/mL); 601431IG, ThermoFisher, Waltham, MA. USA]. Secondary Antibodies include Chicken anti-rabbit 594 (1:500; A21442, Thermofisher, Waltham, MA. USA), Chicken anti-Rabbit 488 (1:500; A21441, ThermoFisher, Waltham, MA. USA), Donkey anti-goat 488 (1:500; A11055, ThermoFisher, Waltham, MA. USA) and Goat anti-Mouse IgG2a 594 (1:500; A21135, ThermoFisher, Waltham, MA. USA).
Single cell RNA sequencing
Uninjured joints (day 0) and injured joints from 1-, 3-, 7-, 15- and 30 days post-injury (n=5/group) were used for scRNA-seq analysis. Single-cell isolation protocol was optimized to obtain a maximum number of viable cells from the joint without any immune cell contamination from the bone marrow or superficial tissues. Briefly, hindlimbs were dissected free of superficial tissues such as skin and muscle, taking care to maintain the integrity of the knee joint and retaining synovial fluid between tibia and femur. Joint residing cells (cells from cartilage, synovial fluid, synovium, infrapatellar fat pad, fibrous tissue etc.) were liberated by first dissociating the two bones into 7.5 mL of 3% Collagenase 1 solution (Worthington Biochemical, Lakewood, NJ; CLS-1) and 100 µg/mL DNase I (Roche, Basel, Switzerland; 11284932001) in DMEM/F12 shaking at 37°C for two 1-hour digests followed by PBS wash steps between digests. Cells released from the joint were filtered through a 70 μm nylon cell strainer prior to red blood cell (RBC) lysis using ammonium-chloride-potassium (ACK) lysis buffer (ThermoFisher Scientific, Waltham, MA, USA; A1049201). Separation of immune cells was performed using CD45 conjugated magnetic microbeads followed by Miltenyi Biotech MACS separation with LC columns for CD45+ cell enrichment. Both immune (CD45+) and non-immune (CD45-) cell populations were independently sequenced using a Chromium Single Cell 3’ Reagent Kit and Chromium instrument (10X Genomics, Pleasanton, CA). Library preparation was performed according to manufacturer’s protocol and sequenced on an Illumina NextSeq 500 (Illumina, San Diego, CA, USA).
scRNA-seq data analysis
Raw scRNA-seq data was processed using the 10x Genomics Cell Ranger software (version 6.0.0.) according to manufacturer’s recommended protocols (10X Genomics, Pleasanton, CA, USA). Briefly, raw base call (BCL) files generated by Illumina NextSeq 500 sequencer were demultiplexed into FASTQ files using Cell Ranger ‘mkfastq’. Aligning sequencing data to the mouse reference genome (mm10), barcode counting, and unique molecular identifier (UMI) counting were performed using Cell Ranger ‘count’. Remaining analysis was performed using Seurat R package, which performs quality control and subsequent analyses on the feature-barcode matrices produced by Cell Ranger (27, 28). Output files from Cell Ranger were read into Seurat v3 and cells with fewer than 500 detected genes/cell and genes that were expressed by fewer than 5 cells were filtered out. Dead cells and potential doublets were also excluded from subsequent analysis. Next, the data was normalized by employing a global-scaling normalization method ‘LogNormalize’ and a set of highly variable genes was identified. Then the data from various timepoints were integrated for downstream analysis. After quality control procedures and integration were complete, data was scaled, and the dimensionality of the data was reduced by principal component analysis (PCA). Subsequently, cells were grouped into an optimal number of clusters for de novo cell type discovery using Seurat’s ‘FindNeighbors’ and ‘FindClusters’ functions. A non-linear dimensional reduction was then performed via uniform manifold approximation and projection (UMAP) and various cell clusters were identified and visualized. Marker genes per cluster were calculated using Seurat’s ‘FindAllMarkers’ function. To characterize various immune cell types in detail, clusters expressing respective cell types-specific markers were extracted and analyzed as described above to identity subtypes and cell type-specific changes. Gene expression plots were created using Seurat’s ‘VlnPlot’, ‘DotPlot’, ‘FeaturePlot’ and ‘RidgePlot’ functions. Genes differentially expressed between two timepoints were identified using ‘FindMarkers’ function. Ontology enrichment analysis was performed using Enrichr (29).
Pseudo-time trajectory finding
Pseudo-time trajectory of monocytes and macrophages was constructed with Monocle (30). Expression data, phenotype data, and feature data were extracted from the Seurat object and a Monocle ‘CellDataSet’ object was constructed using the ‘newCellDataSet’ function. Highly variable genes from Seurat object were used as ordering genes. Trajectory construction was then performed after dimensionality reduction and cell ordering with default parameters as described before (31).
Bulk RNA-seq and data analysis
Cells were obtained from D0 and D7 wildtype BL6 and Trem2-/- mice as described in the scRNA-seq section and were stored at −80°C in RLT lysis buffer supplemented with beta-mercaptoethanol (Qiagen, Hilden. Germany) until processed. Total RNA was isolated using RNeasy® Mini Qiagen kits (Hilden, Germany) and concentrations were determined using a Qubit® RNA HS Assay Kit (ThermoFisher Scientific, Waltham, MA, USA). Quality was assessed before sequencing using an RNA 6000 Nano kit run on an Agilent 2100 Bioanalyzer (Agilent Technologies Inc., Santa Clara, CA, USA). cDNA libraries were prepared from 100 ng of total RNA per manufacturer specification for RNA sequencing using an Illumina mRNA Stranded Library Preparation kit (Illumina Inc., San Diego, CA, USA) and run on an Illumina NextSeq 500 using the High Output 75 cycles kit (Illumina Inc., San Diego, CA, USA).
Raw sequence data generated from Illumina NextSeq500 was de-multiplexed and converted into fastq files using Illumina’s bcl2fastq software. Read quality was assessed using FastQC (32). Reads were then aligned to mouse reference genome mm10 with STAR (33). Subsequently, gene-wise read counts were calculated using ‘featureCounts’ (34). Then data was normalized using TMM normalization method from edgeR (35) and differentially expressed genes were identified using limma and voom (36). Genes with p-value less than 0.05 and fold change greater than 1.25 were considered as significantly differentially expressed genes.
Flow cytometry analysis
Single cell suspensions were generated as described in the scRNA-seq section then subsequently incubated with the following antibodies at a 1:100 dilution in PBS+1%FBS for macrophage characterization: Biolegend PE/Cyanine7 anti-mouse CD206 (MMR) Antibody (Clone: C068C2), Biolegend PE anti-mouse F4/80 Antibody (Clone: BM8), Biolegend Brilliant Violet 510™ anti-mouse/human CD11b Antibody (Clone: M1/70), Biolegend APC/Cyanine7 anti-mouse CD45 Antibody (Clone: 30-F11), Invitrogen TREM2 Monoclonal Antibody (Clone 78.18), Biolegend Brilliant Violet 510 anti-mouse Ly-6C (Clone: HK1.4), Biolegend APC anti-mouse Ly-6G (Clone: 1A8)and DAPI for viability staining. Flow cytometric analysis was performed on a BD FACSMelody system.
Statistical analysis
Statistical analyses were performed using R statistical software or GraphPad Prism. One-way ANOVA and post-hoc Tukey’s Test or student’s t-test were used to determine statistically significant differences of mean expression values. Results were considered statistically significant if p-value is less than 0.05.
Results
Single-cell level characterization of injury-induced immune changes in the mouse knee joint
To identify various synovial joint immune cell types and characterize their dynamic alterations during the pathological progression of PTOA, we profiled the RNA of individual CD45+ cells isolated from the knee joints of 10-week-old BL6 mice before ACL injury (D0) and after injury, at various post-injury timepoints (D1, D3, D7, D15 and D30) (Figure 1A). Non-immune cells from D0 were also sequenced to investigate potential molecular interactions between immune and connective tissue-forming cells in the joint (Figure 1A). Analysis of immune populations identified 7 major cell clusters with distinct gene expression profiles (Figure 1B; S1). Identity of each cluster was determined based on the expression of previously published cell-type specific markers (Figure 1B) (37–40). Cluster 0 was identified as neutrophils based on high expression of S100a8, S100a9, Mmp9, Retnlg and Ly6g (Figure 1C; S1). Cluster 1 expressed monocyte/macrophage markers Cd14, Itgam and Csf1r and was labeled ‘Monocyte-Macrophage (Mono-Mac)’. Cells in cluster 2 were identified as B cells based on Cd19, Cd79a and Cd79b expression. Cluster 3 expressed high levels of cell cycle genes Mki67, Cdk1, Stmn1, Top2a and Cenpa; this cluster was annotated as ‘Proliferating cells (Prolif. cells)’ (Figure 1C; S1). Cluster 4 expressed T cell markers Cd3e and Thy1 while cluster 5 expressed markers for natural killer (NK) cells including Nkg7 and Gzma (Figure 1C). Cluster 6 was identified as DCs based on the expression of DC markers Cd209a and Lag3 (Figure 1C).
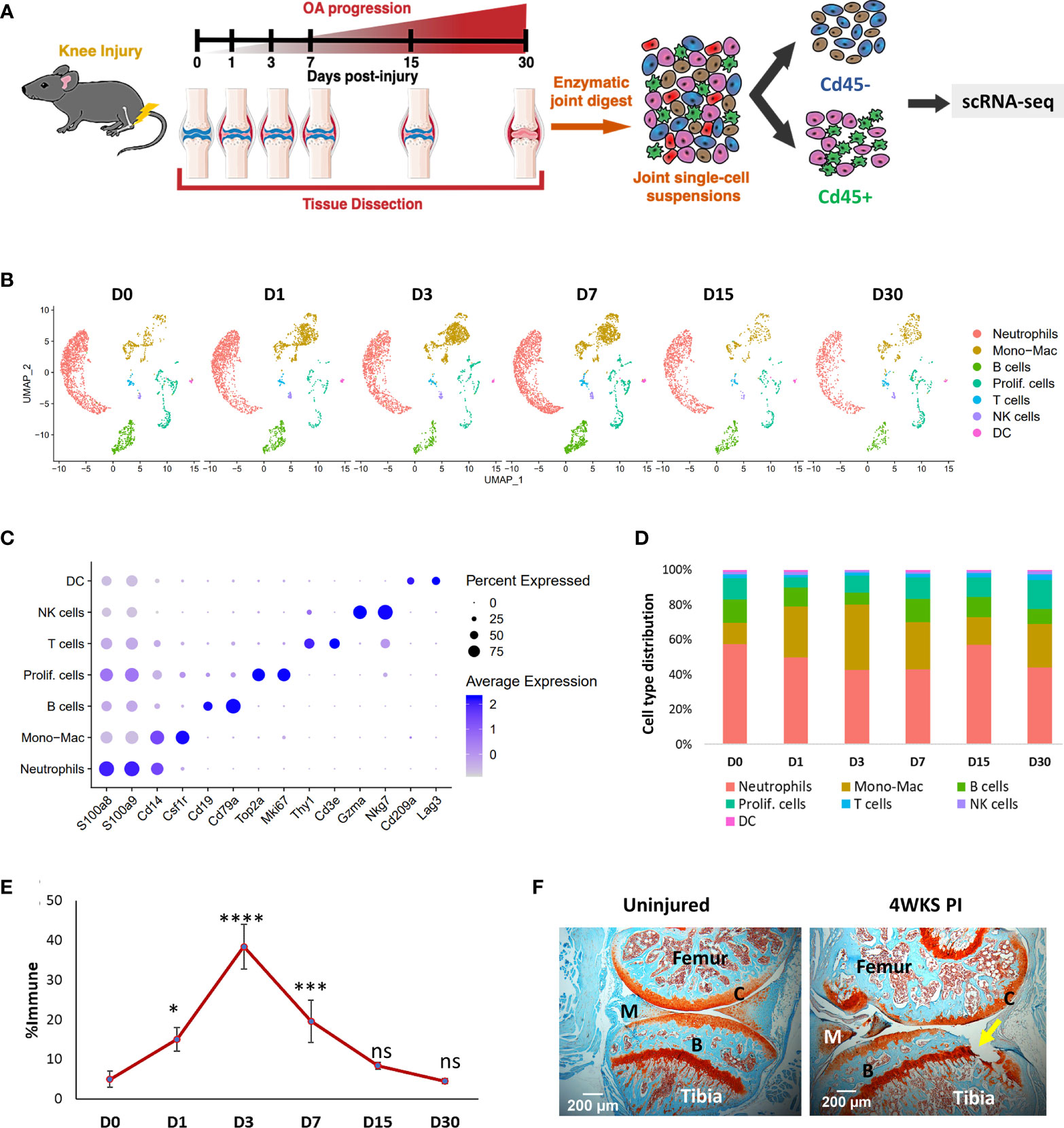
Figure 1 Single-cell analysis of injury-induced immune changes in 10-week-old BL6 mouse knee joints. (A) Graphical representation of the experimental workflow. Uninjured (D0) and injured (D1, D3, D7, D15 and D30) knee joints were dissected, dissociated into single cells and immune and non-immune fractions were sequenced separately using scRNA-seq. (B) Cell clusters from scRNA-seq analysis visualized by Uniform Manifold Approximation and Projection (UMAP). Colors indicate clusters of various cell types. (C) Dot plot showing the expression of selected markers of various cell types. Dot size represents the fraction of cells expressing a specific marker while the intensity of color indicates the average expression level for each gene. (D) Changes in the proportion of various immune cell types in the joint over time. scRNA-seq analysis showed a dramatic increase in Mono-Macs after injury, which peaked at D3. (E) Flow cytometry analysis of injury-induced changes in monocytes/macrophage population. Compared to D0, there was a significant increase in CD45+CD11b+Ly6C+Ly6G- monocytes/macrophages in the knee joints after injury. Data is represented as mean ± standard deviation (SD). *p<0.01; ***p<0.0001; ****p<0.000001; ns, not significant (one-way ANOVA and Tukey’s post hoc test). (F) Safranin-O histological stained sections of uninjured and 4-weeks post-injury joints (4WKS PI). Arrow indicates cartilage damage observed at 4WKS PI. C, cartilage; B, bone; M, meniscus. 5× magnification; scale bars = 200μm.
After injury, the most dramatic changes were observed in the Mono-Mac cluster which increased from ~12% of total immune cells sequenced at D0 to ~37.5% at D3 and then started to decrease (Figure 1D). Consistent with this, flow cytometry analysis showed an increase in CD45+CD11b+Ly6C+Ly6G- monocyte/macrophages (41) in the joint after injury, which peaked at D3 and then reverted to the D0 levels by D30 (Figures 1D–E; S2). The proportion of neutrophils and B lymphocytes were elevated by D7, but at D15 the immune composition was very similar to D0 (Figure 1D). We observed an increase in the proportion of proliferating immune cells at D30 and by then histologically, the joint started to show visible degeneration (Figure 1D, F).
Monocyte and macrophage compartment changes dramatically after knee injury
Previous studies have characterized synovial macrophages in steady state and inflammatory arthritis and have shown that they are a highly heterogeneous population with varying functions and origins (11, 42). To determine the cellular diversity within the Mono-Mac cluster (Figure 1B) and to identify potential developmental relationships between various macrophages and monocyte subtypes we examined the Mono-Mac cluster in more detail. Sub-clustering Mono-Macs resulted in the identification of 9 clusters with distinct gene expression profiles (Figures 2A–C). All clusters expressed Itgam (Cd11b) and Cd14 while Adgre1 (F4/80) and Cd68 expression was enriched in clusters 0, 1, 3 and 5 (Figure 2D; S3A, B), suggesting that these are macrophages. Cluster 0 also showed enrichment for Trem2, Fcrls, Ms4a7, Apoe, Fabp5, Cd63 and several members of complement pathway (C1qa, C1qb and C1qc) (Figure 2A-D; S3C); this cluster was annotated as Trem2hiFcrls+ macrophages. We also detected robust Mrc1 (Cd206) expression in this cluster (Figure 2D; S3C), suggesting that cluster 0 may represent a population of alternatively activated macrophages. Proportion of Trem2hiFcrls+ macrophages increased dramatically after injury, peaking at D3 but showed a significant reduction by D15 (Figure 2E).
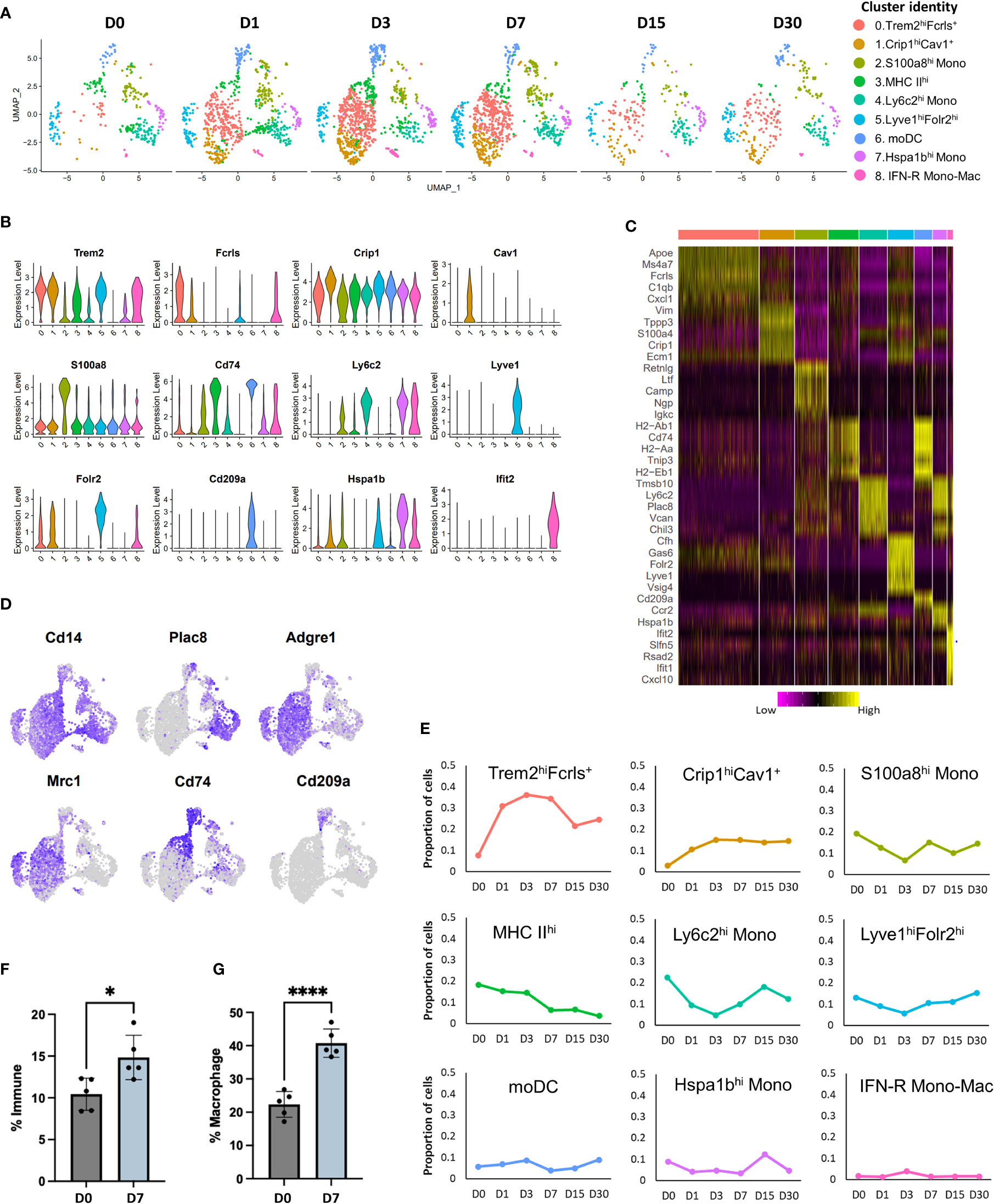
Figure 2 Characterization of injury-induced changes in monocyte/macrophage populations. (A) UMAP plots of nine monocyte and macrophage subtypes identified in mouse knee joints at various timepoints. (B) Violin plots showing the expression of selected markers of various monocyte and macrophage sub-populations (colored based on cluster identities in panel (A). (C) Heatmap showing the scaled expression of top genes enriched in each monocyte/macrophage cluster. (D) Feature plots showing the expression of key monocyte and macrophage markers in various clusters. (E) Changes in the proportion of various monocyte and macrophage populations after injury, determined using scRNA-seq (colored based on cluster identities in panel (A). (F) Flow cytometry analysis of macrophages (CD45+CD11b+F4/80+) in uninjured (D0) and injured (D7) joints. Injured joints at D7 had significantly more macrophages than D0. Data is represented as mean ± SD. *p ≤ 0.05 (two-sided unpaired t-test). (G) Flow cytometry data showing the proportion of M2-like (CD45+CD11b+F4/80+ CD206+) macrophages at D0 and D7, relative to all macrophages. D7 joints showed a significantly higher proportion of M2-like macrophages compared to D0 joints. Data is represented as mean ± SD. ****p ≤ 0.0001 (two-sided unpaired t-test).
Cluster 1 also shared several genes with Trem2hiFcrls+ macrophages including Trem2, Ms4a7 and complement pathway genes C1qa, C1qb and C1qc but had low Mrc1 expression (Figures 2A–D; S3C, Table S1). In addition, this cluster had significantly higher expression of Crip1, S100a4, Vim, Tagln2 and Cspg4 than Trem2hi Fcrls+ macrophages. Unlike cells in Cluster 0, Cluster 1 macrophages expressed high levels of Cav1, a protein known to regulate monocyte differentiation into macrophages (43) and Aqp1, a regulator of macrophage migration (44) suggesting that cluster 1 macrophages may represent a pre-activation, early macrophage differentiation stage population (Figure S3D). This cluster was labeled as Crip1hiCav1+ macrophages. Similar to Trem2hiFcrls+ macrophages, Crip1hiCav1+ macrophage population also showed an increase with time (Figure 2E).
Cluster 2 showed enrichment for granulocyte-associated transcripts S100a8, Lcn2, Retnlg, Mmp8 and Mmp9 along with low to moderate expression of monocyte markers Ly6c2 and Plac8 (Figures 2A-D; S3C, Table S1). This cluster also showed enrichment for inflammatory cytokine Il1b (Figure S3E). Previous studies have described monocytes that express neutrophil markers, suggesting that cluster 2 contains neutrophil-like monocytes (45). This cluster was labelled S100a8hi monocytes. The proportion of S100a8hi monocytes decreased slightly by D3 but reverted back by D7 (Figure 2E). Interestingly, a small subset of cells from cluster 2 also expressed B cell markers including Cd79a and Igkc (Figure S3F).
Cluster 3 represented a population of macrophages (Adgre1+) showing enrichment for class II MHC genes involved in antigen presentation including Cd74, H2-Aa, H2-Ab1 and H2-Eb1 and this cluster was labeled as MHC IIhi. (Figures 2A–D). Proportion of MHC IIhi macrophages gradually decreased after injury (Figure 2E). Cluster 6 also showed enrichment for class II MHC genes including Cd74, H2-Aa, H2-Ab1 and H2-Eb1. This cluster lacked Adgre1 expression but, expressed Cd209a, a marker of monocyte-derived DC (moDC) (Figure 2D) (45, 46). Therefore, this cluster was annotated as moDCs. Both these clusters showed significant enrichment for Il1b (Figure S3E). Cluster 4 showed enrichment for monocyte markers Ly6c2 and Plac8 and was identified as Ly6c2hi monocytes (mono) (Figures 2A–D). Il1b was also enriched in this cluster (Figure S3E). Proportion of Ly6c2hi cluster decreased immediately post-injury and then started to increase by D7 (Figure 2E). Cluster 7 also expressed monocyte markers Ly6c2 and Plac8 but, had significantly higher Hspa1b, Ccr2, Tmpo, and Rhob expression and was annotated as Hspa1bhi mono (Figures 2A–D; S3C).
Cluster 5 contained macrophages (Adgre1+) which expressed Trem2 and several other markers of Trem2hiFcrls+ macrophages but also had unique markers including ‘Lymphatic vessel endothelial hyaluronan receptor 1 (Lyve1)’, Folr2, Timd4, Sparc and Vsig4 (Table S1). Robust Mrc1 (Cd206) expression was also detected in this cluster and this cluster was identified as Lyve1hiFolr2hi macrophages (Figure S3C). A subset of cells in this cluster expressed Aqp1, suggesting the existence of transcriptionally distinct Lyve1hiFolr2hi macrophage subtypes (Figure S3D). The proportion of Lyve1hiFolr2hi macrophages did not change significantly over time (Figure 2E). Genes such as Lyve1, Vsig4, Timd4 and Folr2 have previously been identified as markers of a resident macrophage population in multiple tissues (47), suggesting that cluster 5 may represent a synovial tissue resident macrophage population.
Cluster 8 represented a small population of type I interferon responsive (IFN-R) monocytes and macrophages which expressed genes such as Ifit1, Ifit2, Ifit3 and Isg15 (Figures 2A–C, S3C; Table S1). Both monocyte and macrophage marker expression were observed in cluster 8 and this cluster was identified as IFN-R mono-mac. This cluster also expressed high levels of inflammatory cytokines Cxcl10 (48) and Ccl12 (49) (Figure S3E). Our data showed that there is a dramatic increase in macrophage number after injury which persisted until D7, and a significant proportion of these newly differentiated macrophages acquired an M2-like phenotype (Figures 2A–E) consistent with healing. Flow cytometry analysis further confirmed that there are significantly more macrophages (CD45+CD11b+F4/80+) at D7 compared to D0 and that ~40% of macrophages at D7 were M2-like (CD45+CD11b+F4/80+CD206+) compared to ~18% M2-like macrophages at D0 (Figures 2F, G; S4).
To determine the potential developmental relationships between various monocyte and macrophage clusters, we performed a pseudo-time differentiation trajectory analysis with Monocle (Figures 3A, B; S5A). Cells were plotted on the trajectory according to its pseudo-time value which is the inferred distance along a cell’s developmental lineages from the origin of the lineage. Monocytes were found at the start of the pseudo-time trajectory and macrophage populations expressing high levels of Mrc1 were at the other end (Figures 3A, B; S5A). S100a8hi monocytes and Hspa1bhi monocytes occupied two separate branches while Ly6c2hi monocytes were distributed on both these branches (Figure 3A; S5A). We also observed a significant expansion of Trem2hiFcrls+ population along the trajectory in a monocyte → macrophage direction at D1-D7 post-injury (Figure 3A; S5A). This expansion coincided with an increase in Ly6c2+ cells primarily at D1, Adgre1(F4/80)+ cells at D1-D7, Mrc1(Cd206)+ cells primarily at D3-D7 (Figure 3C). In addition, we observed an increase in Adgre1 and Mrc1 expression and a decrease in the expression of monocyte markers Ly6c2 and Plac8 with time in Trem2hiFcrls+ cluster (Figure 3D). This suggested that Trem2hiFcrls+ macrophage population might have originated from Ly6c2+ monocytes and most cells acquire an M2-like phenotype by D3 (Figures 3A–D). To further confirm this, we extracted Ly6c2-expressing and Mrc1-expressing cells from the scRNA-seq data and found that a significant number of cells in Trem2hiFcrls+ cluster at D1 and D3 are Ly6c2+ cells while a high proportion of cells were Mrc1+ at D3-D7 (Figure S5B).
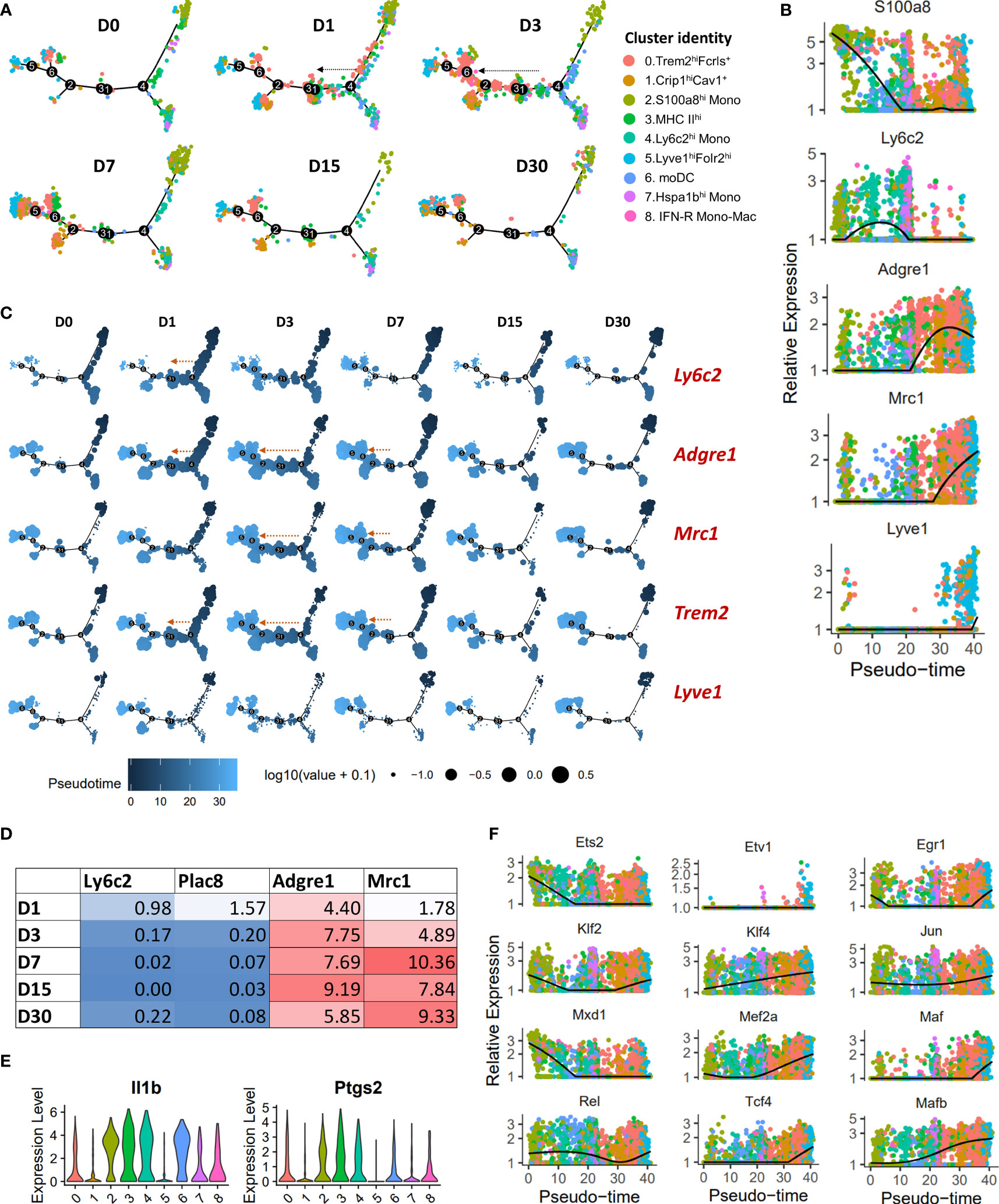
Figure 3 Pseudo-time differentiation trajectory analysis of monocytes and macrophages from D0-D30. (A) The relative position of cells across the pseudo-time differentiation trajectory is depicted. Each point is a cell and is colored according to its cluster identity. Cells along the trajectory were divided into six groupings based on experimental timepoints (D0-D30). An expansion of Trem2hiFcrls+ population in monocytes → macrophage direction was observed after injury, primarily at D1 and D3 (indicated by arrows). (B) Expression of monocyte and macrophage markers on a pseudo-time scale (each point represents a cell and is colored based on cluster identities in panel (A)). Monocyte markers were highly expressed at the beginning of the differentiation trajectory while Mrc1 and Lyve1 had the highest expression towards the end. (C) Superimposition of the expression of selected genes on the pseudo-time trajectory. Each point is a cell and is colored according to its pseudo-time value. Circle size represents the gene expression level. Expansion of cell populations expressing high levels of Adgre1, Trem2 and Mrc1 in the monocyte → macrophage direction was observed after injury (indicated by arrows). (D) Average expression of monocyte and macrophage markers in Trem2hiFcrlshi cluster. (E) Violin plots showing elevated Il1b and Ptgs2 expression in MHC IIhi macrophages (cluster 3). MHC IIhi macrophages and monocytes/moDCs expressed higher levels of Il1b and Ptgs2 compared to other macrophage clusters, including Trem2hiFcrls+ and Lyve1+ macrophages. (F) Plots showing pseudo-time-ordered expression of selected transcription factors (cells are colored based on cluster identities in panel (A)).
MHC IIhi macrophages also showed an expansion along the pseudo-time trajectory (Figure S5A). This subtype constituted a significant proportion of cells at D0 (Figure 2E), suggesting that MHC IIhi macrophages could represent the MHCII+ tissue resident macrophages described in previous studies (47, 50). They also appeared to be replenished by monocytes after injury; this cluster had Ly6c+ cells at D1 and D3 like Trem2hiFcrls+ cluster (Figure S5B). We also found elevated levels of Il1b and Ptgs2 expression in cells of MHC IIhi cluster, like monocytes (Figure 3E). The expression levels were significantly higher than other macrophage clusters, including Trem2hiFcrls+, Crip1hiCav1+ and Lyve1+ macrophages, suggesting that MHC IIhi macrophages have an inflammatory phenotype (Figure 3E). IFN-R Mono-Mac (Ifit2+) showed a slight expansion along the pseudo-time trajectory primarily at D3 indicating increased proliferation/differentiation at this timepoint (Figures S5A, S6A). We also observed a similar expansion of Crip1hiCav1+ macrophages along the trajectory after injury (Figures S5A, S6A) but, not of Lyve1+ macrophages which primarily occupied at the end of the pseudo-time trajectory (Figure 3C; S5A).
We also identified several transcription factors (TFs) differentially expressed in these monocyte/macrophage subpopulations which may play a role in regulating their unique transcriptomic signatures. By plotting TF expression on a pseudo-time scale, we found that TFs Mxd1 and Ets2 had highest expression early in the differentiation trajectory, primarily in monocytes whereas TFs such as Mafb, Mef2a, Mef2c, Klf4 and Tcf4 had the highest expression towards the end of the pseudo-time trajectory, primarily in Mrc1+ macrophage subtypes (Figure 3F; S6B). Etv1 and Maf were mostly expressed in Lyve1hiFolr2hi macrophages whereas Irf7, a key regulator of type-I interferon-dependent immune responses (51), was significantly enriched in IFN-R Mono-Mac (Figure 3F; S6B).
Lyve1 marks resident macrophages with potential anabolic functions
Lyve1hiFolr2hi macrophages were a major Mono-Mac population at all timepoints examined including D0, and this population robustly expressed Mrc1 (CD206) and Cd163, markers of alternatively activated macrophages (Figure 4A). We observed that Lyve1hiFolr2hi macrophages did not show an expansion along the differentiation trajectory after injury like other macrophage subtypes, suggesting that they were not actively differentiated from monocytes. Ccr2 expression in macrophages correlates with a monocyte origin and it has been shown that Ccr2- macrophages are not replenished by monocytes (47, 52). We found that Lyve1hiFolr2hi macrophages had minimal Ccr2 expression while all other clusters robustly expressed Ccr2 (Figure 4B). This suggests that Lyve1hiFolr2hi cluster may represent self-renewing tissue resident macrophages. IHC analysis showed that Lyve1high macrophages are primarily present at the synovial lining at D0 and express CD206, suggesting that they have an M2-like phenotype (Figures 4C–D). The neat lining of Lyve1+ macrophages was disrupted, and the spatial orientation of these cells changed after ACL injury in mice (Figures 5A, B) and these cells started infiltrating the synovium (Figures 5A, C, D). Trem2 receptor expression was also observed in Lyve1+ macrophages (Figure 5).
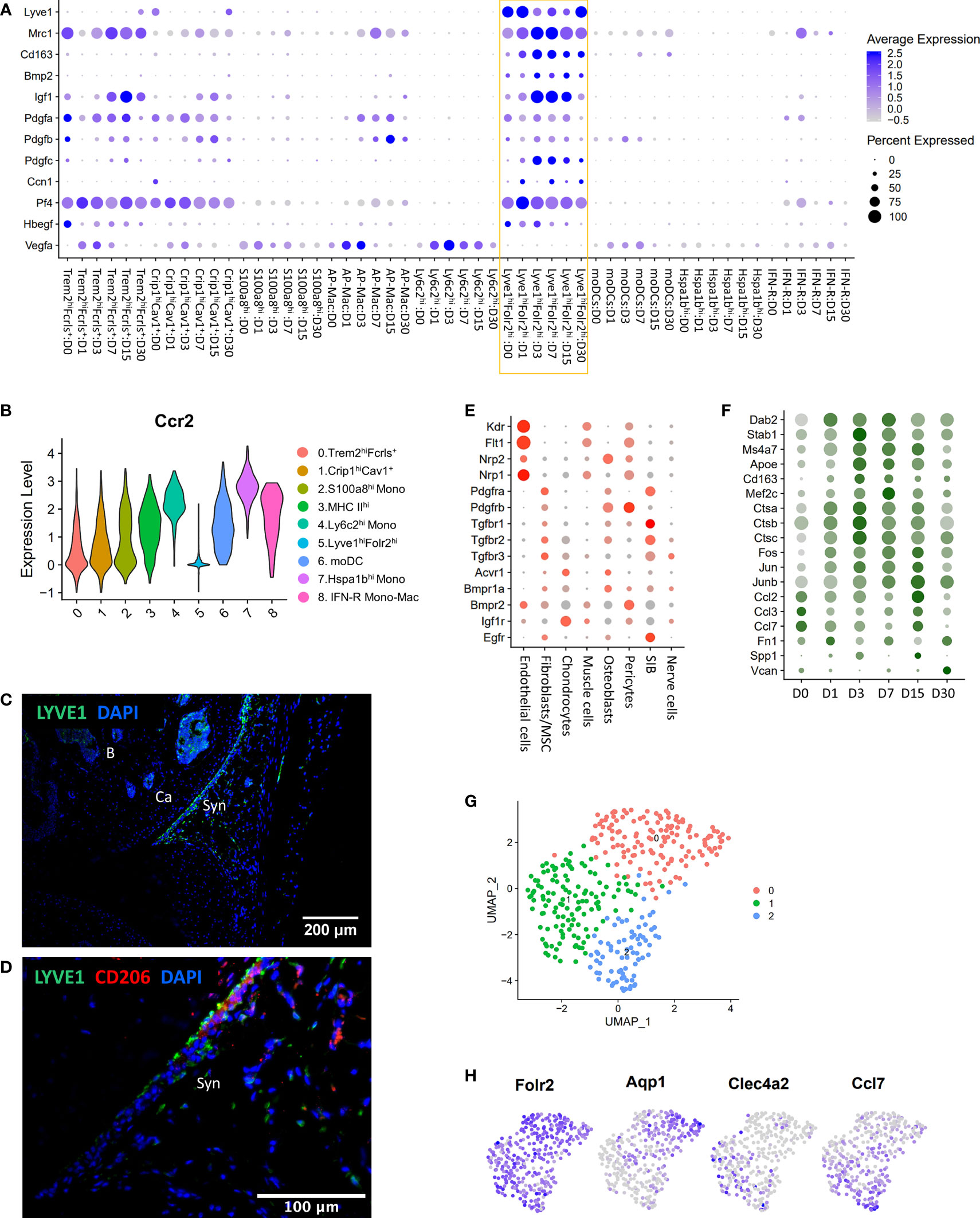
Figure 4 Characterization of Lyve1hiFolr2hi macrophages. (A) Dot plot showing enrichment of growth factors in Lyve1hiFolr2hi macrophages (yellow box). Dot size represents the fraction of cells expressing a specific gene while the intensity of color indicates the average expression level for each gene. (B) Violin plot showing the expression of Ccr2 in various monocyte/macrophage subtypes. Lyve1+ macrophages showed significantly lower Ccr2 expression. (C) IHC analysis of Lyve1+ macrophages at D0 (10× magnification; scale bar = 200μm). Lyve1+ macrophages were primarily present at the synovial lining at D0. B: bone; Ca: cartilage; Syn: synovium. (D) Co-expression of Lyve1 and CD206 at the synovial lining at D0 (40× magnification; scale bar = 100μm). (E) Growth factor receptor expression in connective tissue-forming cells from the joint. Dot size represents the fraction of cells expressing each gene (grey: low expression; red: high expression). (F) Genes upregulated in Lyve1hiFolr2hi macrophages after injury. Dot size represents the fraction of cells expressing each gene (grey: low expression; green: high expression). (G) UMAP plot showing 3 subtypes of Lyve1hiFolr2hi macrophages. (H) Feature plots showing the expression of Folr2 and markers of various subtypes of Lyve1hiFolr2hi macrophages.
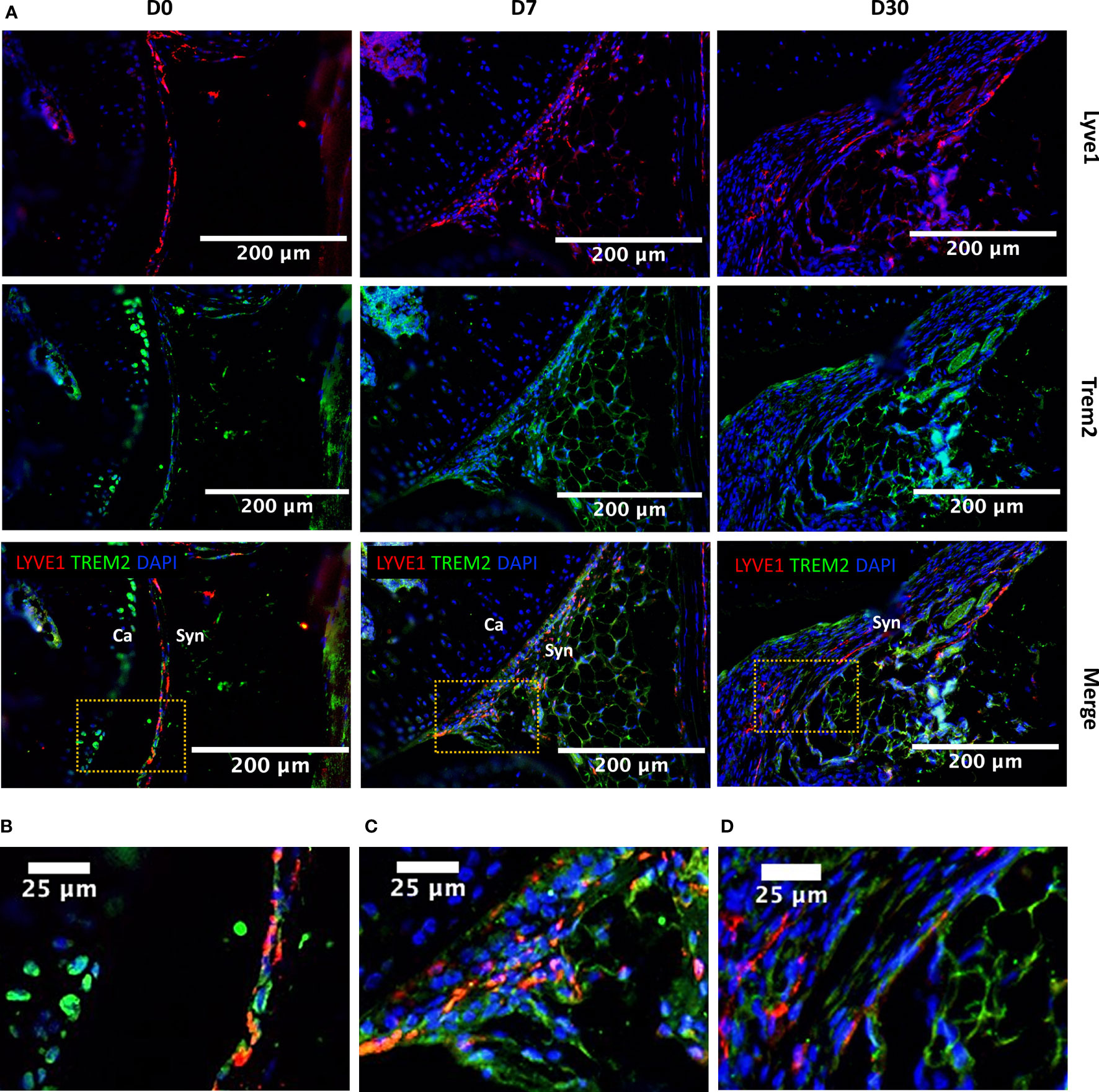
Figure 5 Injury-induced changes in the localization of Lyve1hiFolr2hi macrophages based on Lyve1 expression. (A) IHC showing Lyve1 and Trem2 expression in macrophages at D0, D7 and D30 (20× magnification; scale bar = 200μm), and a closer view of the images for (B) D0, (C) D7 and (D) D30 matching to the highlighted boxes in A (scale bar = 25μm). The Lyve1+ macrophages were primarily observed at the synovial lining at D0. The lining of Lyve1+ macrophages was disrupted after injury and these cells started to infiltrate the synovial membrane. Ca, cartilage; Syn, synovium.
To further understand the potential functions of Lyve1hiFolr2hi macrophages in the joint, we examined the gene expression signature of these macrophages and characterized the temporal molecular changes within this population in response to injury. We found that Lyve1hiFolr2hi macrophages showed enrichment for several growth factors including Bmp2, Igf1, Pdgfa, Pdgfb, Pdgfc and Hbegf as well as Ccn1 which also shows growth factor activities (53) (Figure 4A). We also observed that this macrophage cluster had extremely low expression for Vegfa, an angiogenesis regulating growth factor expressed in several other clusters (Figure 4A). Interestingly, Lyve1hiFolr2hi macrophages also showed enrichment for Pf4, a chemokine commonly considered as megakaryocyte/platelet specific (Figure 4A) (54). We observed a significant increase in Igf1 and Pdgfc expression after injury which then started to decrease by D15 (Figure 4A). To determine the potential targets of Lyve1hiFolr2hi macrophage secreted growth factors, we examined connective tissue forming cells in the joint for growth factor receptor expression and found that chondrocytes had the highest expression of Igf1 receptor Igf1r whereas Vegfa receptors Kdr, Flt1, Nrp1 and Nrp2 were primarily expressed in endothelial cells (Figure 4E; S7A). Bmp2 receptors Acvr1 and Bmpr1a were enriched in osteoblasts, chondrocytes and fibroblasts/MSCs. Hbegf receptor Egfr was primarily expressed in fibroblasts/MSCs and synovial intimal fibroblasts (SIB) (Figure 4E). Previous studies have identified Bmp2 and Igf1 as two cartilage-anabolic cytokines (55–57). Ccn1 has also been identified as an important regulator of chondrogenesis (53, 58). This suggests that Lyve1hiFolr2hi macrophages may have an anabolic function in the joint.
We also identified several genes including Dab2, Cd163, Stab1, Apoe and cathepsins Ctsa, Ctsb and Ctsc upregulated in this cluster after injury. The expression of these genes peaked at D3/D7 and was subsequently reduced (Figure 4F). Dab2 is a regulator of inflammatory signaling during phenotypic polarization of macrophages (59). Stab1 in macrophages has been shown to mediate tissue homeostasis and prevent fibrosis in chronic liver injury (60). Apoe deficiency in macrophages results in impaired clearance of apoptotic bodies (61) and cathepsin inhibition led to a polarization shift from M2- to M1 macrophages (62). Several transcription factors including Fos, Jun, Junb and Mef2c were also upregulated after injury (Figure 4F). We also found that extracellular matrix (ECM) genes including Fn1, Spp1 and Vcan were upregulated at D15/D30 (Figure 4F). Interestingly, Lyve1hiFolr2hi cluster expressed inflammatory cytokines Ccl2, Ccl3 and Ccl7 and their expression was reduced after injury and remained low until D7 (Figure 4F). Ccl7 is known to be expressed by both M1 and M2 macrophages (63). Park et al. has recently described three vascular macrophage subtypes that shared resident macrophage genes including Lyve1, Mrc1, Pf4, Cd163, and Folr2 and one of which expressed inflammatory and chemokine genes including Ccl7, Ccl2 and Cxcl1 (64). To further explore the existence of such Lyve1hiFolr2hi macrophage subtypes, we extracted all cells from Lyve1hiFolr2hi macrophage cluster and reanalyzed using Seurat. Consistent with the findings by Park et al, we identified three Lyve1hiFolr2hi macrophage subtypes enriched for Aqp1, Clec4a1 and cytokines (Ccl2, Ccl7), respectively (Figures 4G, H; S7B) (64). Clec4a1+ subtype also showed enrichment for transcription actors Atf3, Egr1, Jun and Fos (Figure S7B). All three clusters expressed Folr2, Lyve1 and Cd163 (Figure S7B) and it is possible that these subclusters represent various differentiation/activation stages of Lyve1hiFolr2hi macrophages rather than distinct subtypes.
Trem2hiFcrls+ macrophages assume a molecular profile similar to the resident Lyve1hiFolr2hi macrophage population
Trem2hiFcrls+ macrophages were the major macrophage population in the injured joints until D15 and appeared to be derived from monocyte (Figures 2A, 3). We found that Trem2hiFcrls+ macrophages also showed enrichment for Mrc1 and many of the anabolic growth factors highly expressed in Lyve1hiFolr2himacrophages including Igf1, Pdgfa and Pdgfb, suggesting that these two populations might have similar functions (Figure 4A; S7C). Additionally, we observed Trem2 expression in Lyve1+ synovial macrophages (Figure 5). Next, we investigated genes enriched in both these macrophage populations compared to all other monocyte/macrophage clusters. Hundred and fifty-four genes were >1.25 fold enriched (FDR <0.05) in Trem2hiFcrls+ cluster compared to all monocytes, MHC IIhi and Crip1hiCav+ macrophages. Of these, 111 genes were also enriched in Lyve1hiFolr2hi cluster (Figure 6A). In addition to growth factors, genes common to both Trem2hiFcrls+ and Lyve1hiFolr2hi macrophages included Trem2 and its ligand Apoe (65), Dab2, Stab1, Ms4a7, cathepsins (Ctsb, Ctsd and Ctsl), complement pathway genes C1qa, C1qb and C1qc, and Mmp inhibitor Timp2 (Figures 6B, C). We also identified many genes enriched in Trem2hiFcrls+ macrophages compared to Lyve1hiFolr2hi macrophages. These included anti-inflammatory cytokine Il10, Spp1, Gpnmb, Fabp5, Mmp14 and Mmp19 (Figure 6D). Our study also revealed genes activated in Trem2hiFcrls+ macrophages at various timepoints post-injury. Genes including Spp1, Ccr2, Fabp5 and Mmp8 were elevated at D1 while Igf1 had the highest expression at D7 (Figure 6E). Arg1, a marker of M2 macrophages was significantly upregulated at D1 and D3 but was dramatically reduced by D7 (Figures S8A, B). Similar to Lyve1hiFolr2hi macrophages, Ctsa, Ctsb and Ctsc had the peak expression at D3. Complement pathway genes also showed an increase in expression with time (Figure 6E). We also observed significant heterogeneity within the Trem2hiFcrls+ cluster. A subset of cells showed significant enrichment for transcription factors such as Jun and Fos while another subset of cells showed enrichment for cytokines such as Cxcl2 and Il10 (Figure S8C).
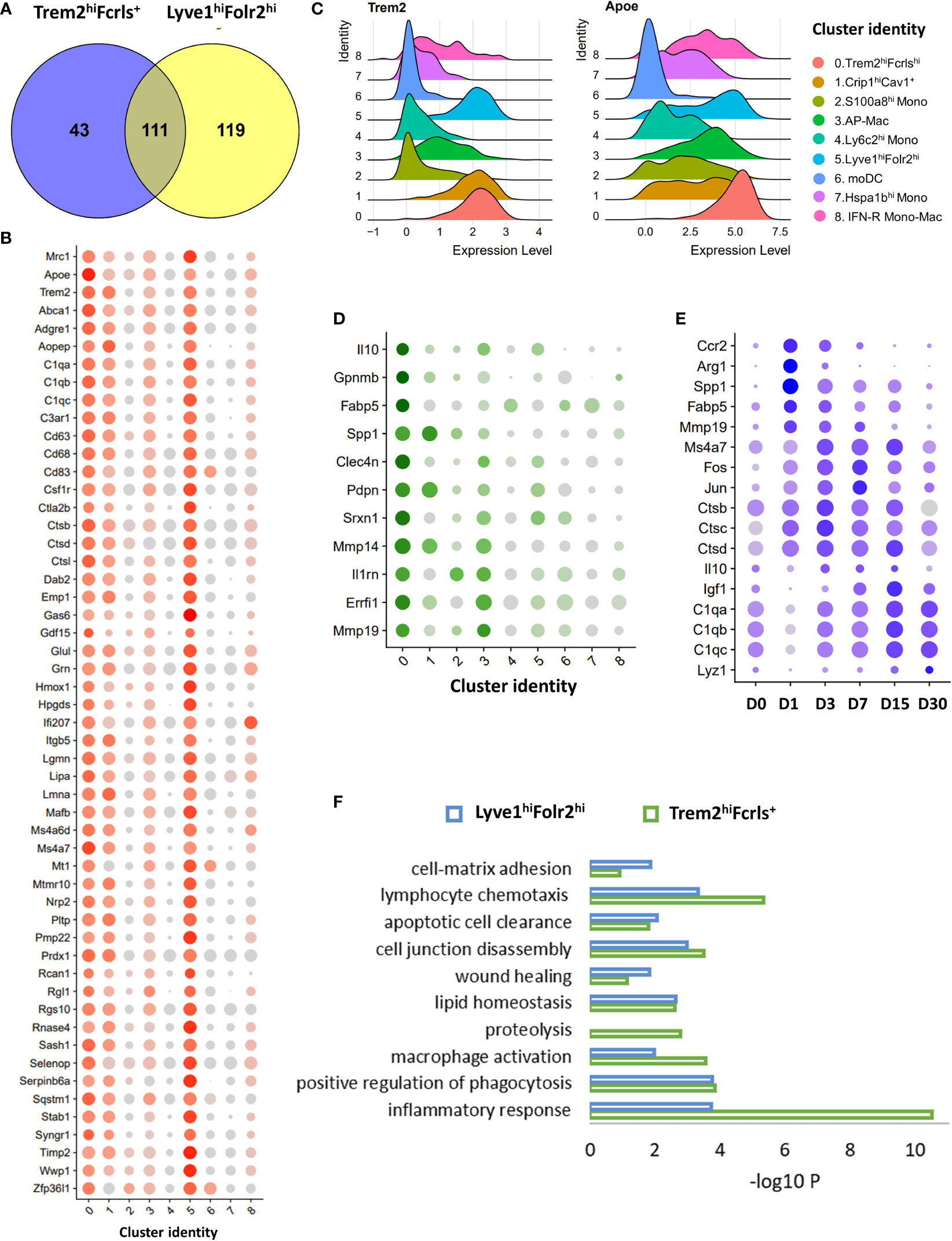
Figure 6 Characterization of Trem2hi M2-like macrophages using scRNA-seq. (A) Venn diagrams showing the overlap between genes enriched in both Trem2hiFcrls+ and Lyve1hiFolr2himacrophages compared to all other clusters. (B) Dot plot showing the expression of a subset of genes enriched in both Trem2hiFcrls+ and Lyve1hiFolr2himacrophages. Dot size represents the fraction of cells expressing each gene (grey: low expression; red: high expression). (C) Ridge plot showing the expression of Trem2 and its ligand Apoe in various clusters. Both Trem2 and Apoe were enriched in Trem2hiFcrls+ and Lyve1hiFolr2hi macrophages. (D) Dot plot showing the expression of a subset of genes enriched in Trem2hiFcrls+ macrophages compared to Lyve1hiFolr2hi macrophages. Dot size represents the fraction of cells expressing each gene (grey: low expression; green: high expression) (E) Genes upregulated in Trem2hiFcrls+ macrophages after injury. Dot size represents the fraction of cells expressing each gene (grey: low expression; blue: high expression). (F) Ontology terms associated with genes enriched in Trem2hiFcrls+ and Lyve1hiFolr2himacrophages compared to other clusters.
Next, we performed an ontology enrichment analysis of genes in Trem2hiFcrls+ and Lyve1hiFolr2hi macrophages. Both macrophage populations showed enrichment for biological processes such as ‘wound healing’, ‘phagocytosis’, ‘apoptotic cell clearance’ to name a few. (Figure 6F). ‘Proteolysis’ was enriched only in the Trem2hiFcrls+ cluster, and this cluster also showed higher enrichment for ‘inflammatory response’ (Figure 6F). Our data suggests that recruited Trem2hiFcrls+ macrophages assume a transcriptional profile similar to Lyve1hiFolr2hi macrophages and both these populations may play a role in tissue remodeling via phagocytosis and clearing of apoptotic cells after injury. In addition, the growth factors secreted by these macrophage populations might also contribute to tissue repair after injury.
TREM2 (triggering receptor expressed on myeloid cells 2) is a cell surface receptor expressed in myeloid lineage cells such as monocytes, macrophages and dendritic cells and microglia in brain (66, 67). We found that Trem2 and its ligand Apoe are robustly expressed in M2-like Trem2hiFcrls+ and Lyve1hiFolr2hi macrophage populations (Figure 6B). Trem2 and Apoe have previously been shown to play a role in phagocytosis (61, 68) and Trem2+ macrophages have been implicated in tissue repair responses in multiple organs (69, 70). To confirm Trem2 expression in M2-like macrophages we performed flow cytometry analysis of synovial macrophages from D0 and D7. Flow cytometry analysis showed that >85% of the M2-like macrophages (CD45+CD11b+F4/80+CD206+) in the joint express Trem2 (Figures 7A–C). Consistent with scRNA-seq data, injured joints at D7 had significantly more Trem2+ M2-like macrophages compared to uninjured D0 joints while macrophages from D0 joints were primarily Trem2-CD206-(Figure 7D). To determine if Trem2 plays a significant role in M2 macrophage differentiation, we analyzed macrophages from uninjured and injured joints of wildtype (WT) and Trem2 deficient mice (Trem2-/-) using flow cytometry. The proportion of M2 macrophages was not significantly different between these mice (Figure S8D), however bulk RNA-seq analysis of cells from uninjured and injured joints of WT and Trem2-/- mice determined that Trem2-/-mice had significantly lower expression of Cx3cr1, Ptprc, Adgre1, Csf1r, Havcr2, Ldlr, P2y12, Fcgr1 and Fcgr3 at D7 compared to WT (Figure 7E). Fcgr1, Fcgr3 and P2y12 play roles in phagocytosis (71, 72) while genes such as Cx3cr1 and Csf1r are involved in macrophage differentiation, recruitment or activation (73–75). Downregulation of these genes in Trem2-/-mice indicates an altered macrophage response after injury and suggests that Trem2 signaling may play a major role in joint repair by modulating macrophage activation and phagocytosis.
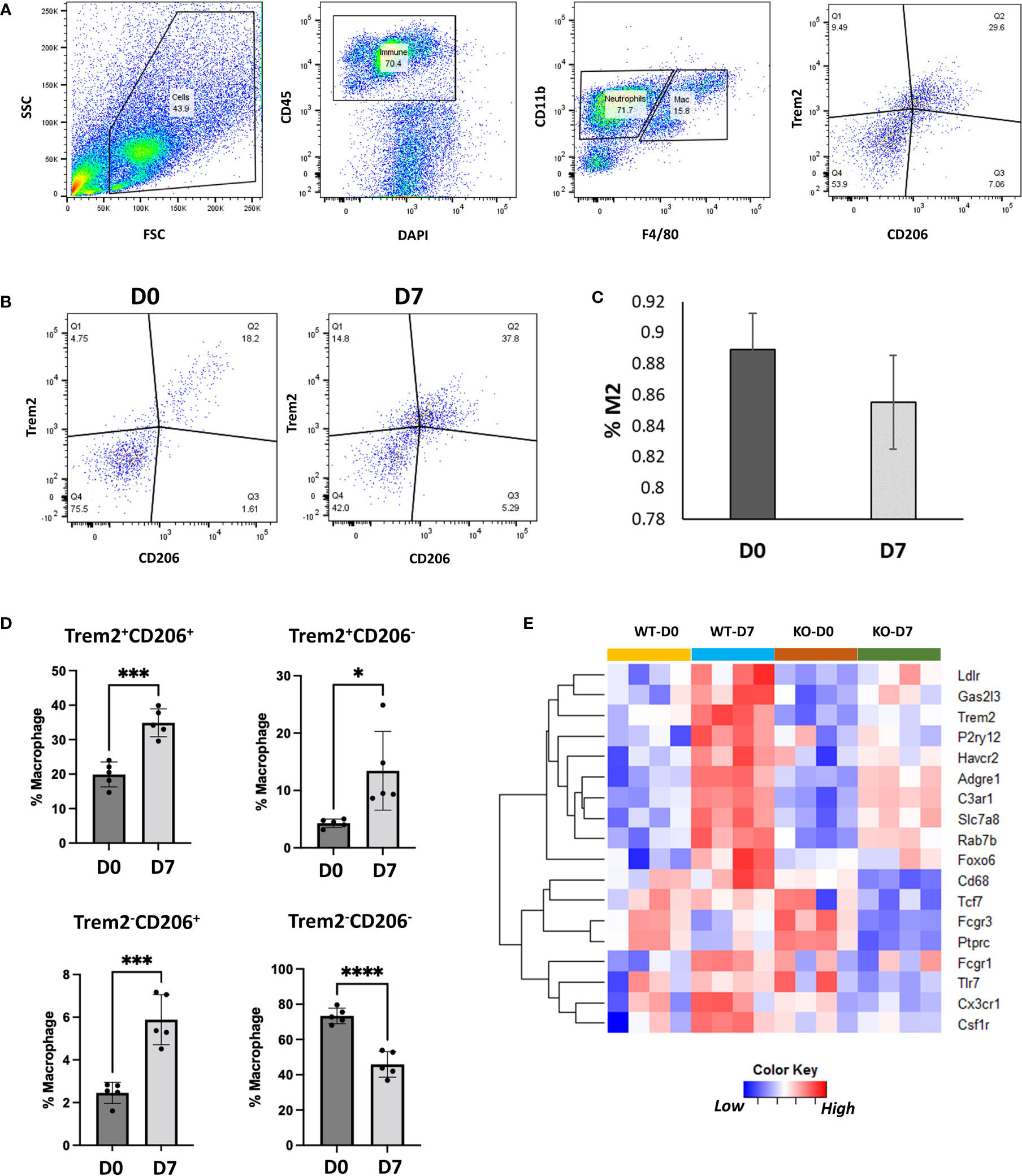
Figure 7 Characterization of Trem2-expressing macrophages using flow cytometry. (A) Flow cytometry gating strategy to identify Trem2-expressing M2-like macrophages. (B) Flow cytometry plots showing Trem2+ M2-like macrophages at D0 and D7. (C) Proportion of Trem2+ macrophages among M2-like macrophages at D0 and D7. Data is represented as mean ± SD. (D) Proportion of Trem2 and CD206- expressing macrophages relative to all macrophages. Data is represented as mean ± SD. *p ≤ 0.05; ***p ≤ 0.001; ****p ≤ 0.0001 (two-sided unpaired t-test). (E) Genes differentially expressed between injured joints of wildtype (WT) and Trem2-/- (KO) mice at D7.
Characterization of neutrophils and lymphocytes
Neutrophils were the most abundant immune population identified at all timepoints examined (Figures 1B–D) and appeared to be a major source of matrix degrading enzymes such as Mmp8 and Mmp9 and several cytokines including Il1a, Il1b, Cxcl2, Cxcl3 and Ccl3 in the joints (Figure 8A). Further analysis of cell from the neutrophil cluster revealed 4 major subtypes with distinct gene expression profiles: 1) Ccrl2hi neutrophils expressing high levels of Ccrl2, Il1b, Ptgs2 and Cxcl2; 2) Mmp8hi neutrophils were enriched for Mmp8, Mmp9, Retnlg and Ly6c2; 3) Chil3hi neutrophils had high expression of Chil3, Ngp and Lcn2 and 4) Interferon (Ifn)-responsive neutrophils expressed high levels of Ifit1 and Isg15 (Figures 8B, C). We also identified several genes up regulated in the neutrophil cluster after injury including Oncostatin M (Osm), Ptgs2, Mmp8, Mmp9 and Adam8 (Figure 8D).
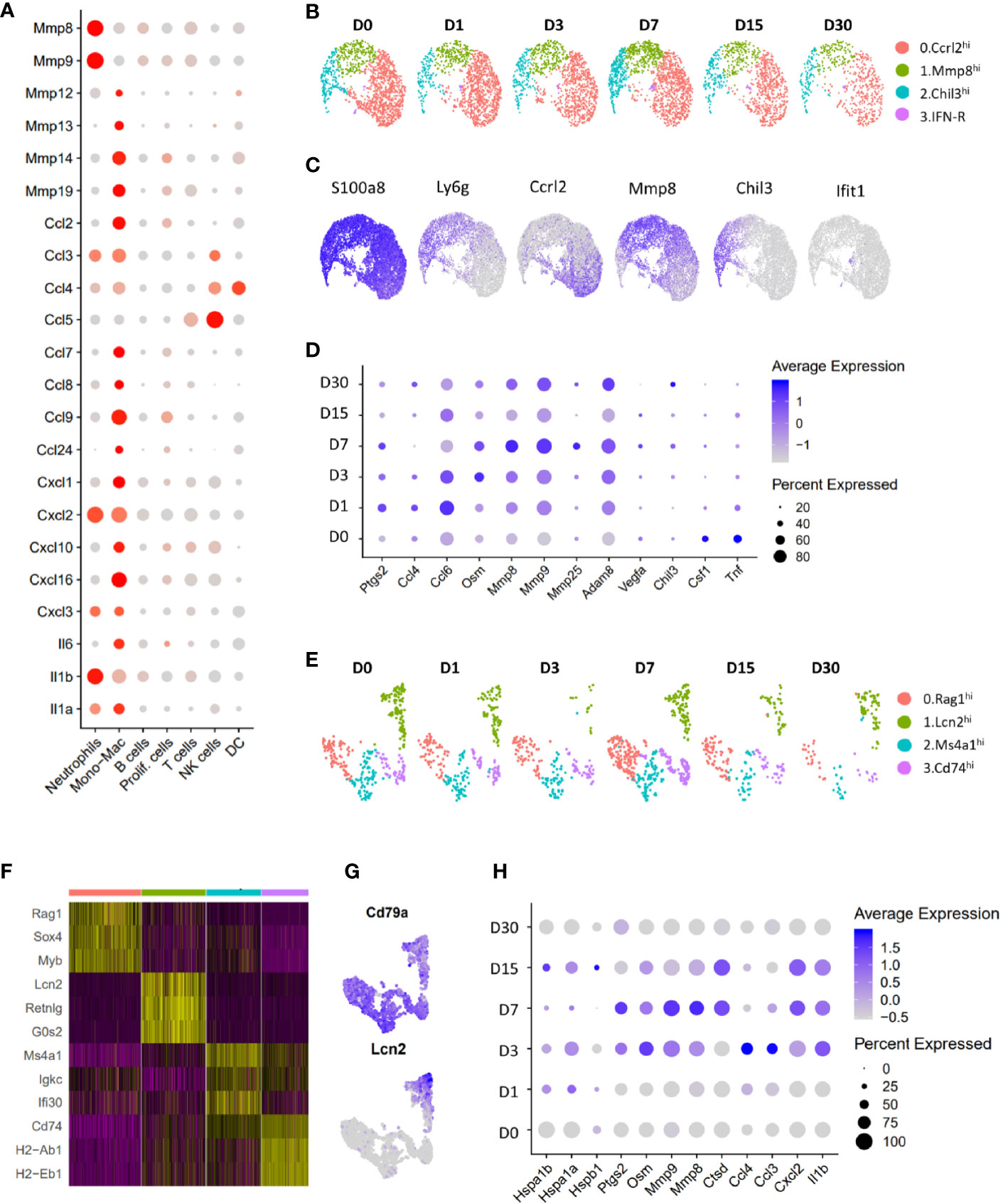
Figure 8 Characterization of neutrophils and B lymphocytes. (A) Dot plot showing the expression of cytokines and proteases in various immune cell clusters. Dot size represents the fraction of cells expressing a specific marker while the intensity of color indicates the average expression level for each gene (grey: low expression; red: high expression). (B) UMAP plot showing various neutrophil sub-clusters. (C) Feature plot showing the expression of various neutrophil sub-cluster markers as well as pan-neutrophil marker S100a8. (D) Dot plot showing genes differentially expressed in neutrophils after injury. Dot size represents the fraction of cells expressing a specific marker while the intensity of color indicates the average expression level for each gene. (E) UMAP plot showing various B cell sub-clusters. (F) Heatmap showing genes enriched in each B cell subtype. (G) Feature plot showing the expression of Lcn2 and B cell marker Cd79a in B cell subtypes. (H) Genes differentially expressed in Lcn2hi B cell-like cells after injury. Dot size represents the fraction of cells expressing a specific marker while the intensity of color indicates the average expression level for each gene.
T, NK and DC clusters were underrepresented in our data which prevented us from identifying significant injury induced changes in these cell types. Analysis of B cell cluster revealed four subtypes of B cells, all expressing Cd79a (Figures 8E–G). Interestingly, one of the B cell subtypes expressed granulocyte markers Lcn2, Retnlg and Mmp8 and was label as Lcn2hi B cell-like cells (Figures 8E–G). We found a significant increase in inflammatory cytokine and matrix-degrading enzyme expression in this cluster after injury (Figure 8H), suggesting that Lcn2hi B cell-like cells may also contribute to PTOA pathogenesis.
Discussion
Using scRNA-seq technology to unbiasedly investigate how injury alters the synovial joint immune compartment, our study revealed a heterogeneity of transcriptional responses within various immune populations including macrophages, neutrophils, and B cells, not previously appreciated by traditional bulk RNA-seq or other gene expression profiling approaches. Neutrophils were identified as a major source of inflammatory cytokines including Il1a and Il1b and matrix degrading enzymes including Mmp8, Mmp9 and Adam8 (Figure 8A), which indicates that persistence of these cells in the joint, post injury may significantly influence PTOA development. We also observed a significant number of B cells in the joint. Interestingly, we identified a B cell subtype expressing both B cell and granulocyte markers (Figures 8E–H). This cluster also showed an increase in cytokine and protease expression after injury and may also contribute to OA pathogenesis. Studies have shown that B cells can differentiate into granulocyte-macrophage progenitor-like cells (76). However, further studies are required to determine the true identity and role in OA pathogenesis of these immune cell subtypes.
Monocyte/macrophage cluster showed the most dramatic changes after injury (Figures 1D, E). Monocytes and macrophages play critical roles in tissue repair after injury by regulating inflammation, removing debris and dead cells, and producing cytokines and growth factors that promote healing (77). Our analysis uncovered nine transcriptionally distinct monocytes/macrophages populations and defined injury-induced changes in these populations. One of the major populations we identified is a tissue resident Lyve1hiFolr2hi macrophage cluster that robustly expressed genes such as Lyve1, Folr2, Timd4, Sparc and Vsig4 along with M2 macrophage markers Mrc1 and Cd163. Previous studies identified Lyve1hi tissue-resident macrophages in multiple organs, including heart, lungs, bladder, blood vessels and fat tissues and suggested that these macrophages play a key role in healing and tissue repair (78–81). Consistent with previous studies, Lyve1hiFolr2hi macrophages appeared to be self-renewing (47, 50) and not be recruited to the site of injury from other parts of the body. Chakarov et al. recently showed that lack of Lyve1+ macrophages exacerbated lung fibrosis (80) while Dick et al. showed that Lyve1+ resident cardiac macrophages have a protective role after myocardial infarction (50). Culemann et al. described a distinct population of synovial tissue-resident macrophages which co-express genes such as Vsig4, Trem2 and Sparc and form an internal immunological barrier at the synovial lining (42). It has also been shown that the Folr2highLyve1pos macrophage population is reduced in patients with treatment-naïve and -resistant RA compared to healthy individuals and it is increased in RA patients under remission (11). Our results are consistent with these previous studies and indicate that Lyve1hiFolr2hi macrophages reside primarily at the synovial lining and are involved in tissue repair and remodeling after injury. We found that Lyve1hiFolr2hi macrophages express Bmp2 and Igf1 and since Igf1 receptor Igfr is highly expressed on the surface of chondrocytes while Bmp2 receptor Acvr1 is enriched in chondrocytes and osteoblasts, we postulate that the secretion of these growth factors by these macrophages promote cartilage growth and repair, consistent with the chondro-protective role of these growth factors in the knee (55–57).
We also identified a subpopulation of macrophages, Trem2hiFcrls+, that we hypothesize to be recruited into the joint, post injury, and to proceed to adopt a transcriptional signature similar to the Lyve1hiFolr2hi macrophage, further aiding in the healing process. This population was dramatically increased after injury. Our data suggest that these macrophages originate from Ly6c+ monocytes. We also identified several transcription factors including Maf, Mafb, Mef2a, Mef2c, Klf4, Etv1 and Tcf4 that may play a role in the differentiation/function of these macrophages (Figure 3F). Maf, Mafb and Klf4 have previously been shown to promote M2 polarization (82–84). Etv1 has previously been identified as a transcription factor enriched in Lyve1hi macrophages and has been implicated in macrophage polarization (85, 86).
Both Lyve1hiFolr2hi and Trem2hiFcrls+ macrophage populations showed enrichment for genes including Trem2, its ligand Apoe, complement genes (C1qa, C1qb and C1qc) and Cathepsins (Ctsb, Ctsd and Ctsl) and multiple growth factors. However, resident macrophage markers such as Lyve1, Vsig4, Timd4 and Folr2 were not adopted by Trem2hiFcrlshi macrophages. Trem2 is a transmembrane receptor which has been shown to bind to high-density lipoproteins (HDL), low-density lipoproteins (LDL), and several apolipoproteins, including Apoe (65). Trem2 enhances the phagocytosis function of microglia and suppress neuroinflammation; in humans, TREM2 loss-of-function variants are associated with Alzheimer’s disease (65, 87). Several prior studies have implicated Trem2+ macrophages in tissue repair (69, 88) and Trem2 has been described as a promotor of macrophage phenotypic switching during tissue repair, tuning down the recruited macrophage inflammatory profile (69). Furthermore, it has been shown that Trem2hi macrophages were reduced in RA synovium compared to healthy synovium, and patients undergoing RA remission showed an increase in Trem2hi macrophages (11). This correlation further stresses the potential protective role of Trem2+ macrophages, in the joint. We also found that >85% of alternatively activated macrophages in the injured knee joints are Trem2+. Injured joints from Trem2-/- mice had significantly lower levels of genes involved in macrophage differentiation, recruitment, activation or phagocytosis such as Cx3cr1, Csf1r, Fcgr1, Fcgr3 and P2y12 play roles in phagocytosis (71–75), suggesting that Trem2 plays a major role in regulating macrophage responses after injury. Future studies will provide more insights into the functions of Trem2+ macrophages in the synovial joint, both in steady state and after injury.
Our studies also identified additional monocyte/macrophage populations including Ly6c+ monocytes, interferon-responsive cells (IFN-R mono-mac), Crip1hiCav1+ macrophages, MHC IIhi macrophages, granulocyte-like monocytes (S100a8hi) and moDCs. It remains to be determined whether all nine monocyte/macrophage clusters found in knee joints are distinct subpopulations or some merely represent a transcriptional state during differentiation. We observed that a subset of cells from S100a8hi cluster expressed B cell markers. It has previously been shown that pre/pro-B cells can generate macrophage populations suggesting that these Cd79a+ cells may have originated from B cells (89). However, further studies are required to confirm the origin of these cells. Monocyte clusters, moDCs and MHC IIhi macrophages showed significant enrichment for Il1b, an inflammatory cytokine involved in OA (90) while IFN-R mono-mac expressed high levels of Cxcl10 and Ccl12. Cxcl10 plays a role in regulating neutrophil-NK cell cross-talk determining the severity of experimental OA (91) and macrophage secretion of Ccl12 has been shown to inhibit fibroblast-mediated cardiac wound healing (49). It is likely that Lyve1hiFolr2hi and Trem2hiFcrls+ macrophages play a chondro-protective role and promote the healing process (92, 93) after joint injury while monocytes, moDCs, MHC IIhi macrophages and neutrophils may have pro-inflammatory functions (Figure 9). Additional studies are required to understand the specific functions of various monocyte/macrophage subtypes in the joint and the cues that drive differentiation of monocytes to a specific fate.
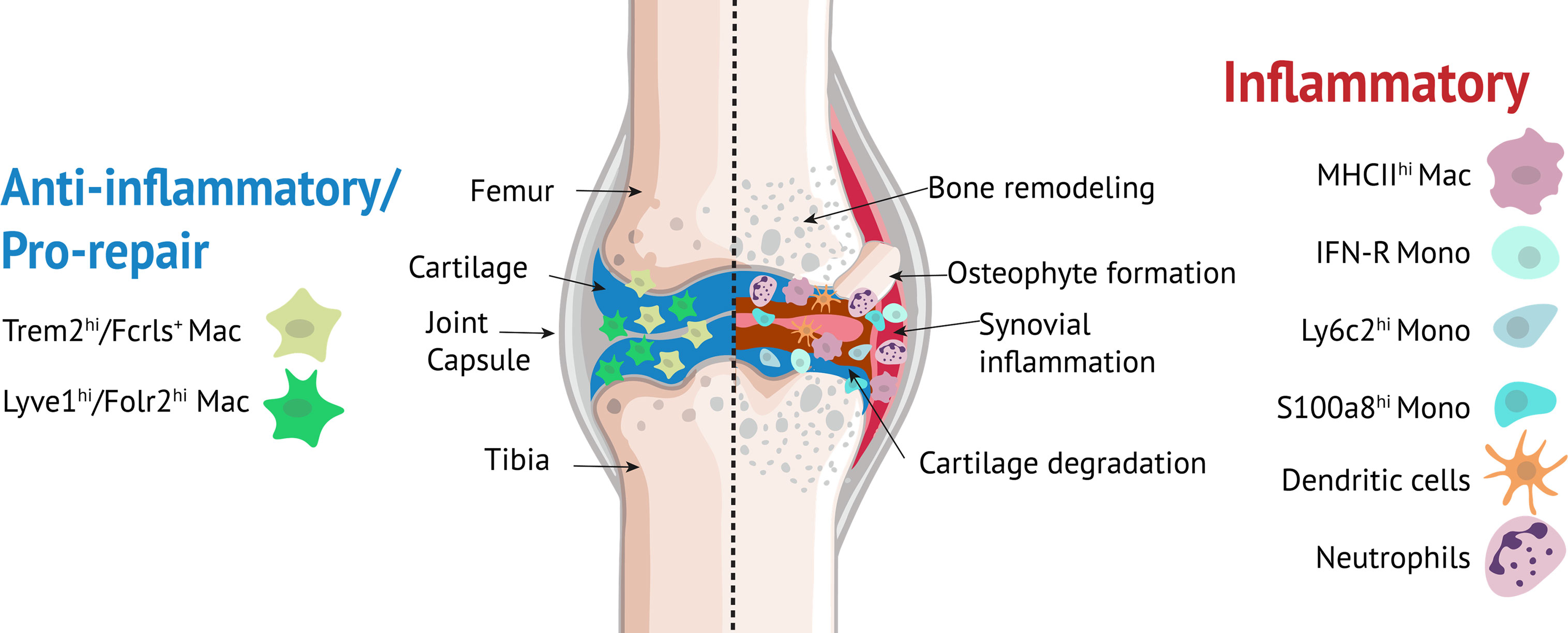
Figure 9 Myeloid cells in the knee joint. Lyve1hiFolr2hi and Trem2hiFcrls+ macrophages play an anti-inflammatory/pro-repair role while monocytes, moDCs, MHC IIhi macrophages and neutrophils may have pro-inflammatory roles.
Our study highlighted several monocyte/macrophage subpopulations that might play significant roles in orchestrating the response to ACL injury. We hypothesize that these immune subpopulations may also play a role in other types of joint injuries including a tibial plateau fracture or a meniscal tear as changes in these monocyte/macrophage subpopulations have also been identified in other tissues after injury and in inflammatory diseases (11, 12, 94). Dysregulated innate immune response is likely a key contributing factor to pathological changes occurring in the joint after injury including synovial inflammation, cartilage degeneration, abnormal bone remodeling and osteophyte formation (Figure 9) and early PTOA progression may be prevented or delayed by interventions with anti-inflammatory/immune modulatory drugs, including the ones currently in use for the treatment of RA (95). Our study identified multiple monocyte/macrophage subtypes that could be potentially targeted to prevent or treat PTOA. However, a thorough understanding of the precise functions of these monocyte/macrophage subpopulations is necessary to effectively target these populations with minimal side effects. Studies focused on detailed molecular and functional characterization of some of these macrophage subtypes in the context of PTOA are currently underway. Findings from these studies could pave the way for the development of novel therapeutic strategies including cell-based therapies for OA such as modulating patient’s own immune cells to prevent or slowdown joint degeneration after injury.
While we were able to identify multiple neutrophil, monocyte/macrophage and B cell subpopulations, we failed to characterize the heterogeneity in T/NK cells and DCs due to underrepresentation of these cell types in our data. There is also a possibility that we missed some neutrophil, monocyte/macrophage and B cell subpopulations present in the joints. As single-cell isolation protocols improve, we will be able to release more viable cells from mineralized tissues such as bone and cartilage and sequence much higher number of cells, which will allow us to characterize rare and novel cell populations. Our study highlights the tremendous complexity and plasticity that underlie the synovial joint immune compartment in steady state, and after ACL injury. Our findings also provide insights into the potential roles of some of these immune subtypes in the joint. A greater understanding of the differential contribution of individual immune subsets to maintaining synovial joint homeostasis and joint tissue repair after injury will open new avenues for developing improved therapeutic strategies for PTOA and other joint diseases.
Data availability statement
The datasets presented in this study can be found in online repositories. The name of the repository and accession numbers can be found below: NCBI GenBank (https://www.ncbi.nlm.nih.gov/genbank); GSE200842 and GSE200843
Ethics statement
The animal study was reviewed and approved by Lawrence Livermore National Laboratory IACUC and UC Davis IACUC.
Author contributions
Conceptualization: AS and GL. Methodology: AS, JM, NH, DM, KM, BC, GL, BA and NR. Formal analysis, AS, NH, SW. Writing- original draft: AS, GL. Writing—review & editing: NH, SW, JM, BC. Project management: AS and GL. All authors contributed to the article and approved the submitted version.
Funding
This study received funding from DOD PR192271, LDRD 2020 20-LW-002 and DOD PR180268.
Acknowledgments
This work was performed under the auspices of the U.S. Department of Energy by Lawrence Livermore National Laboratory under Contract DE-AC52-07NA27344.
Conflict of interest
The authors declare that the research was conducted in the absence of any commercial or financial relationships that could be construed as a potential conflict of interest.
Publisher’s note
All claims expressed in this article are solely those of the authors and do not necessarily represent those of their affiliated organizations, or those of the publisher, the editors and the reviewers. Any product that may be evaluated in this article, or claim that may be made by its manufacturer, is not guaranteed or endorsed by the publisher.
Supplementary material
The Supplementary Material for this article can be found online at: https://www.frontiersin.org/articles/10.3389/fimmu.2022.938075/full#supplementary-material
References
1. de Rezende MU, de Campos GC. Is osteoarthritis a mechanical or inflammatory disease? Rev Bras Ortop (2013) 48(6):471–4. doi: 10.1016/j.rbe.2013.12.002
2. Lin YJ, Anzaghe M, Schulke S. Update on the pathomechanism, diagnosis, and treatment options for rheumatoid arthritis. Cells (2020) 9(4):880. doi: 10.3390/cells9040880
3. Woodell-May JE, Sommerfeld SD. Role of inflammation and the immune system in the progression of osteoarthritis. J Orthop Res (2020) 38(2):253–7. doi: 10.1002/jor.24457
4. Robinson WH, Lepus CM, Wang Q, Raghu H, Mao R, Lindstrom TM, et al. Low-grade inflammation as a key mediator of the pathogenesis of osteoarthritis. Nat Rev Rheumatol (2016) 12(10):580–92. doi: 10.1038/nrrheum.2016.136
5. Chow YY, Chin KY. The role of inflammation in the pathogenesis of osteoarthritis. Mediators Inflamm (2020) 2020:8293921. doi: 10.1155/2020/8293921
6. Hsueh MF, Zhang X, Wellman SS, Bolognesi MP, Kraus VB. Synergistic roles of macrophages and neutrophils in osteoarthritis progression. Arthritis Rheumatol (2021) 73(1):89–99. doi: 10.1002/art.41486
7. Zhu W, Zhang X, Jiang Y, Liu X, Huang L, Wei Q, et al. Alterations in peripheral T cell and b cell subsets in patients with osteoarthritis. Clin Rheumatol (2020) 39(2):523–32. doi: 10.1007/s10067-019-04768-y
8. Li YS, Luo W, Zhu SA, Lei GH. T Cells in osteoarthritis: Alterations and beyond. Front Immunol (2017) 8356. doi: 10.3389/fimmu.2017.00356
9. Alahdal M, Zhang H, Huang R, Sun W, Deng Z, Duan L, et al. Potential efficacy of dendritic cell immunomodulation in the treatment of osteoarthritis. Rheumatol (Oxford) (2021) 60(2):507–17. doi: 10.1093/rheumatology/keaa745
10. Zhang F, Wei K, Slowikowski K, Fonseka CY, Rao DA, Kelly S, et al. Defining inflammatory cell states in rheumatoid arthritis joint synovial tissues by integrating single-cell transcriptomics and mass cytometry. Nat Immunol (2019) 20(7):928–42. doi: 10.1038/s41590-019-0378-1
11. Alivernini S, MacDonald L, Elmesmari A, Finlay S, Tolusso B, Gigante MR, et al. Distinct synovial tissue macrophage subsets regulate inflammation and remission in rheumatoid arthritis. Nat Med (2020) 26(8):1295–306. doi: 10.1038/s41591-020-0939-8
12. Jin K, Gao S, Yang P, Guo R, Li D, Zhang Y, et al. Single-cell RNA sequencing reveals the temporal diversity and dynamics of cardiac immunity after myocardial infarction. Small Methods (2022) 6(3):e2100752. doi: 10.1002/smtd.202100752
13. Chou CH, Jain V, Gibson J, Attarian DE, Haraden CA, Yohn CB, et al. Synovial cell cross-talk with cartilage plays a major role in the pathogenesis of osteoarthritis. Sci Rep (2020) 10(1):10868. doi: 10.1038/s41598-020-67730-y
14. Punzi L, Galozzi P, Luisetto R, Favero M, Ramonda R, Oliviero F, et al. Post-traumatic arthritis: overview on pathogenic mechanisms and role of inflammation. RMD Open (2016) 2(2):e000279. doi: 10.1136/rmdopen-2016-000279
15. Whittaker JL, Woodhouse LJ, Nettel-Aguirre A, Emery CA. Outcomes associated with early post-traumatic osteoarthritis and other negative health consequences 3-10 years following knee joint injury in youth sport. Osteoarthritis Cartilage (2015) 23(7):1122–9. doi: 10.1016/j.joca.2015.02.021
16. Lieberthal J, Sambamurthy N, Scanzello CR. Inflammation in joint injury and post-traumatic osteoarthritis. Osteoarthritis Cartilage (2015) 23(11):1825–34. doi: 10.1016/j.joca.2015.08.015
17. Sebastian A, Murugesh DK, Mendez ME, Hum NR, Rios-Arce ND, McCool JL, et al. Global gene expression analysis identifies age-related differences in knee joint transcriptome during the development of post-traumatic osteoarthritis in mice. Int J Mol Sci (2020) 21(1):364. doi: 10.3390/ijms21010364
18. Sebastian A, Chang JC, Mendez ME, Murugesh DK, Hatsell S, Economides AN, et al. Comparative transcriptomics identifies novel genes and pathways involved in post-traumatic osteoarthritis development and progression. Int J Mol Sci (2018) 19(9):2657. doi: 10.3390/ijms19092657
19. Loeser RF, Olex AL, McNulty MA, Carlson CS, Callahan MF, Ferguson CM, et al. Microarray analysis reveals age-related differences in gene expression during the development of osteoarthritis in mice. Arthritis Rheumatol (2012) 64(3):705–17. doi: 10.1002/art.33388
20. Goldring MB, Otero M. Inflammation in osteoarthritis. Curr Opin Rheumatol (2011) 23(5):471–8. doi: 10.1097/BOR.0b013e328349c2b1
21. Christiansen BA, Anderson MJ, Lee CA, Williams JC, Yik JH, Haudenschild DR. Musculoskeletal changes following non-invasive knee injury using a novel mouse model of post-traumatic osteoarthritis. Osteoarthritis Cartilage (2012) 20(7):773–82. doi: 10.1016/j.joca.2012.04.014
22. Chang JC, Sebastian A, Murugesh DK, Hatsell S, Economides AN, Christiansen BA, et al. Global molecular changes in a tibial compression induced ACL rupture model of post-traumatic osteoarthritis. J Orthop Res (2017) 35(3):474–85. doi: 10.1002/jor.23263
23. Chang JC, Christiansen BA, Murugesh DK, Sebastian A, Hum NR, Collette NM, et al. SOST/Sclerostin improves posttraumatic osteoarthritis and inhibits MMP2/3 expression after injury. J Bone Miner Res (2018) 33(6):1105–13. doi: 10.1002/jbmr.3397
24. Mendez ME, Sebastian A, Murugesh DK, Hum NR, McCool JL, Hsia AW, et al. LPS-induced inflammation prior to injury exacerbates the development of post-traumatic osteoarthritis in mice. J Bone Miner Res. (2020) 35(11):2229–41. doi: 10.1002/jbmr.4117
25. Mendez ME, Murugesh DK, Sebastian A, Hum NR, McCloy SA, Kuhn EA, et al. Antibiotic treatment prior to injury improves post-traumatic osteoarthritis outcomes in mice. Int J Mol Sci (2020) 21(17):6424. doi: 10.3390/ijms21176424
26. Rios-Arce ND, Murugesh DK, Hum NR, Sebastian A, Jbeily EH, Christiansen BA, et al. Preexisting type 1 diabetes mellitus blunts the development of posttraumatic osteoarthritis. JBMR Plus (2022) 6(5):e10625. doi: 10.1002/jbm4.10625
27. Butler A, Hoffman P, Smibert P, Papalexi E, Satija R. Integrating single-cell transcriptomic data across different conditions, technologies, and species. Nat Biotechnol (2018) 36(5):411–20. doi: 10.1038/nbt.4096
28. Stuart T, Butler A, Hoffman P, Hafemeister C, Papalexi E, Mauck WM 3rd, et al. Comprehensive integration of single-cell data. Cell (2019) 177(7):1888–902 e21. doi: 10.1016/j.cell.2019.05.031
29. Xie Z, Bailey A, Kuleshov MV, Clarke DJB, Evangelista JE, Jenkins SL, et al. Gene set knowledge discovery with enrichr. Curr Protoc (2021) 1(3):e90. doi: 10.1002/cpz1.90
30. Trapnell C, Cacchiarelli D, Grimsby J, Pokharel P, Li S, Morse M, et al. The dynamics and regulators of cell fate decisions are revealed by pseudotemporal ordering of single cells. Nat Biotechnol (2014) 32(4):381–6. doi: 10.1038/nbt.2859
31. Sebastian A, McCool JL, Hum NR, Murugesh DK, Wilson SP, Christiansen BA, et al. Single-cell RNA-seq reveals transcriptomic heterogeneity and post-traumatic osteoarthritis-associated early molecular changes in mouse articular chondrocytes. Cells (2021) 10(6):1462. doi: 10.3390/cells10061462
32. Brown J, Pirrung M, McCue LA. FQC dashboard: integrates FastQC results into a web-based, interactive, and extensible FASTQ quality control tool. Bioinformatics (2017) 33(19):3137–9. doi: 10.1093/bioinformatics/btx373
33. Dobin A, Davis CA, Schlesinger F, Drenkow J, Zaleski C, Jha S, et al. STAR: ultrafast universal RNA-seq aligner. Bioinformatics (2013) 29(1):15–21. doi: 10.1093/bioinformatics/bts635
34. Liao Y, Smyth GK, Shi W. featureCounts: an efficient general purpose program for assigning sequence reads to genomic features. Bioinformatics (2014) 30(7):923–30. doi: 10.1093/bioinformatics/btt656
35. Robinson MD, McCarthy DJ, Smyth GK. edgeR: a bioconductor package for differential expression analysis of digital gene expression data. Bioinformatics (2010) 26(1):139–40. doi: 10.1093/bioinformatics/btp616
36. Ritchie ME, Phipson B, Wu D, Hu Y, Law CW, Shi W, et al. Limma powers differential expression analyses for RNA-sequencing and microarray studies. Nucleic Acids Res (2015) 43(7):e47. doi: 10.1093/nar/gkv007
37. Zilionis R, Engblom C, Pfirschke C, Savova V, Zemmour D, Saatcioglu HD, et al. Single-cell transcriptomics of human and mouse lung cancers reveals conserved myeloid populations across individuals and species. Immunity (2019) 50(5):1317–34 e10. doi: 10.1016/j.immuni.2019.03.009
38. Martini E, Kunderfranco P, Peano C, Carullo P, Cremonesi M, Schorn T, et al. Single-cell sequencing of mouse heart immune infiltrate in pressure overload-driven heart failure reveals extent of immune activation. Circulation (2019) 140(25):2089–107. doi: 10.1161/CIRCULATIONAHA.119.041694
39. Sebastian A, Hum NR, Martin KA, Gilmore SF, Peran I, Byers SW, et al. Single-cell transcriptomic analysis of tumor-derived fibroblasts and normal tissue-resident fibroblasts reveals fibroblast heterogeneity in breast cancer. Cancers (Basel) (2020) 12(5):1307. doi: 10.3390/cancers12051307
40. Scott RE, Ghule PN, Stein JL, Stein GS. Cell cycle gene expression networks discovered using systems biology: Significance in carcinogenesis. J Cell Physiol (2015) 230(10):2533–42. doi: 10.1002/jcp.24990
41. Pang J, Urao N, Koh TJ. Proliferation of Ly6C+ monocytes/macrophages contributes to their accumulation in mouse skin wounds. J Leukoc Biol (2020) 107(4):551–60. doi: 10.1002/JLB.3HI1119-389RRRR
42. Culemann S, Gruneboom A, Nicolas-Avila JA, Weidner D, Lammle KF, Rothe T, et al. Locally renewing resident synovial macrophages provide a protective barrier for the joint. Nature (2019) 572(7771):670–5. doi: 10.1038/s41586-019-1471-1
43. Fu Y, Moore XL, Lee MK, Fernandez-Rojo MA, Parat MO, Parton RG, et al. Caveolin-1 plays a critical role in the differentiation of monocytes into macrophages. Arterioscler Thromb Vasc Biol (2012) 32(9):e117–25. doi: 10.1161/ATVBAHA
44. Tyteca D, Nishino T, Debaix H, van der Smissen P, N'Kuli F, Hoffmann D, et al. Regulation of macrophage motility by the water channel aquaporin-1: crucial role of M0/M2 phenotype switch. PloS One (2015) 10(2):e0117398. doi: 10.1371/journal.pone.0117398
45. Yanez A, Coetzee SG, Olsson A, Muench DE, Berman BP, Hazelett DJ, et al. Granulocyte-monocyte progenitors and monocyte-dendritic cell progenitors independently produce functionally distinct monocytes. Immunity (2017) 47(5):890–902 e4. doi: 10.1016/j.immuni.2017.10.021
46. Menezes S, Melandri D, Anselmi G, Perchet T, Loschko J, Dubrot J, et al. The heterogeneity of Ly6C(hi) monocytes controls their differentiation into iNOS(+) macrophages or monocyte-derived dendritic cells. Immunity (2016) 45(6):1205–18. doi: 10.1016/j.immuni.2016.12.001
47. Dick SA, Wong A, Hamidzada H, Nejat S, Nechanitzky R, Vohra S, et al. Three tissue resident macrophage subsets coexist across organs with conserved origins and life cycles. Sci Immunol (2022) 7(67):eabf7777. doi: 10.1126/sciimmunol.abf7777
48. Gao B, Lin J, Jiang Z, Yang Z, Yu H, Ding L, et al. Upregulation of chemokine CXCL10 enhances chronic pulmonary inflammation in tree shrew collagen-induced arthritis. Sci Rep (2018) 8(1):9993. doi: 10.1038/s41598-018-28404-y
49. DeLeon-Pennell KY, Iyer RP, Ero OK, Cates CA, Flynn ER, Cannon PL, et al. Periodontal-induced chronic inflammation triggers macrophage secretion of Ccl12 to inhibit fibroblast-mediated cardiac wound healing. JCI Insight (2017) 2(18):e94207. doi: 10.1172/jci.insight.94207
50. Dick SA, Macklin JA, Nejat S, Momen A, Clemente-Casares X, Althagafi MG, et al. Self-renewing resident cardiac macrophages limit adverse remodeling following myocardial infarction. Nat Immunol (2019) 20(1):29–39. doi: 10.1038/s41590-018-0272-2
51. Honda K, Yanai H, Negishi H, Asagiri M, Sato M, Mizutani T, et al. IRF-7 is the master regulator of type-I interferon-dependent immune responses. Nature (2005) 434(7034):772–7. doi: 10.1038/nature03464
52. Bajpai G, Bredemeyer A, Li W, Zaitsev K, Koenig AL, Lokshina I, et al. Tissue resident CCR2- and CCR2+ cardiac macrophages differentially orchestrate monocyte recruitment and fate specification following myocardial injury. Circ Res (2019) 124(2):263–78. doi: 10.1161/CIRCRESAHA.118.314028
53. Chen PC, Cheng HC, Yang SF, Lin CW, Tang CH. The CCN family proteins: modulators of bone development and novel targets in bone-associated tumors. BioMed Res Int (2014) 2014:437096. doi: 10.1155/2014/437096
54. Field DJ, Aggrey-Amable AA, Blick SK, Ture SK, Johanson A, Cameron SJ, et al. Platelet factor 4 increases bone marrow b cell development and differentiation. Immunol Res (2017) 65(5):1089–94. doi: 10.1007/s12026-017-8951-x
55. Zhang Z, Li L, Yang W, Cao Y, Shi Y, Li X, et al. The effects of different doses of IGF-1 on cartilage and subchondral bone during the repair of full-thickness articular cartilage defects in rabbits. Osteoarthritis Cartilage (2017) 25(2):309–20. doi: 10.1016/j.joca.2016.09.010
56. An C, Cheng Y, Yuan Q, Li J. IGF-1 and BMP-2 induces differentiation of adipose-derived mesenchymal stem cells into chondrocytes-like cells. Ann BioMed Eng (2010) 38(4):1647–54. doi: 10.1007/s10439-009-9892-x
57. Ruan S, Deng J, Yan L, Huang W. Evaluation of the effects of the combination of BMP-2-modified BMSCs and PRP on cartilage defects. Exp Ther Med (2018) 16(6):4569–77. doi: 10.3892/etm.2018.6776
58. Zhang Y, Sheu TJ, Hoak D, Shen J, Hilton MJ, Zuscik MJ, et al. CCN1 regulates chondrocyte maturation and cartilage development. J Bone Miner Res Mar (2016) 31(3):549–59. doi: 10.1002/jbmr.2712
59. Adamson SE, Griffiths R, Moravec R, Senthivinayagam S, Montgomery G, Chen W, et al. Disabled homolog 2 controls macrophage phenotypic polarization and adipose tissue inflammation. J Clin Invest (2016) 126(4):1311–22. doi: 10.1172/JCI79590
60. Rantakari P, Patten DA, Valtonen J, Karikoski M, Gerke H, Dawes H, et al. Stabilin-1 expression defines a subset of macrophages that mediate tissue homeostasis and prevent fibrosis in chronic liver injury. Proc Natl Acad Sci U S A. (2016) 113(33):9298–303. doi: 10.1073/pnas.1604780113
61. Grainger DJ, Reckless J, McKilligin E. Apolipoprotein e modulates clearance of apoptotic bodies in vitro and in vivo, resulting in a systemic proinflammatory state in apolipoprotein e-deficient mice. J Immunol (2004) 173(10):6366–75. doi: 10.4049/jimmunol.173.10.6366
62. Oelschlaegel D, Weiss Sadan T, Salpeter S, Krug S, Blum G, Schmitz W, et al. Cathepsin inhibition modulates metabolism and polarization of tumor-associated macrophages. Cancers (Basel) (2020) 12(9):2579. doi: 10.3390/cancers12092579
63. Orecchioni M, Ghosheh Y, Pramod AB, Ley K. Macrophage polarization: Different gene signatures in M1(LPS+) vs. classically and M2(LPS-) vs. alternatively activated macrophages. Front Immunol (2019) 10:1084. doi: 10.3389/fimmu.2019.01084
64. Park I, Goddard ME, Cole JE, Zanin N, Lyytikainen LP, Lehtimaki T, et al. C-type lectin receptor CLEC4A2 promotes tissue adaptation of macrophages and protects against atherosclerosis. Nat Commun (2022) 13(1):215. doi: 10.1038/s41467-021-27862-9
65. Gratuze M, Leyns CEG, Holtzman DM. New insights into the role of TREM2 in alzheimer's disease. Mol Neurodegener (2018) 13(1):66. doi: 10.1186/s13024-018-0298-9
66. Zhong L, Chen XF, Zhang ZL, Wang Z, Shi XZ, Xu K, et al. DAP12 stabilizes the c-terminal fragment of the triggering receptor expressed on myeloid cells-2 (TREM2) and protects against LPS-induced pro-inflammatory response. J Biol Chem (2015) 290(25):15866–77. doi: 10.1074/jbc.M115.645986
67. Liu W, Taso O, Wang R, Bayram S, Graham AC, Garcia-Reitboeck P, et al. Trem2 promotes anti-inflammatory responses in microglia and is suppressed under pro-inflammatory conditions. Hum Mol Genet (2020) 29(19):3224–48. doi: 10.1093/hmg/ddaa209
68. Gervois P, Lambrichts I. The emerging role of triggering receptor expressed on myeloid cells 2 as a target for immunomodulation in ischemic stroke. Front Immunol (2019) 10:1668. doi: 10.3389/fimmu.2019.01668
69. Coelho I, Duarte N, Barros A, Macedo MP, Penha-Goncalves C. Trem-2 promotes emergence of restorative macrophages and endothelial cells during recovery from hepatic tissue damage. Front Immunol (2020) 11:616044. doi: 10.3389/fimmu.2020.616044
70. Seno H, Miyoshi H, Brown SL, Geske MJ, Colonna M, Stappenbeck TS. Efficient colonic mucosal wound repair requires Trem2 signaling. Proc Natl Acad Sci U S A. (2009) 106(1):256–61. doi: 10.1073/pnas.0803343106
71. McKenzie SE, Schreiber AD. Fc gamma receptors in phagocytes. Curr Opin Hematol (1998) 5(1):16–21. doi: 10.1097/00062752-199801000-00003
72. Blume ZI, Lambert JM, Lovel AG, Mitchell DM. Microglia in the developing retina couple phagocytosis with the progression of apoptosis via P2RY12 signaling. Dev Dyn (2020) 249(6):723–40. doi: 10.1002/dvdy.163
73. Donnelly DJ, Longbrake EE, Shawler TM, Kigerl KA, Lai W, Tovar CA, et al. Deficient CX3CR1 signaling promotes recovery after mouse spinal cord injury by limiting the recruitment and activation of Ly6Clo/iNOS+ macrophages. J Neurosci (2011) 31(27):9910–22. doi: 10.1523/JNEUROSCI.2114-11.2011
74. Ishida Y, Kimura A, Nosaka M, Kuninaka Y, Hemmi H, Sasaki I, et al. Essential involvement of the CX3CL1-CX3CR1 axis in bleomycin-induced pulmonary fibrosis via regulation of fibrocyte and M2 macrophage migration. Sci Rep Dec 4 (2017) 7(1):16833. doi: 10.1038/s41598-017-17007-8
75. Stanley ER, Chitu V. CSF-1 receptor signaling in myeloid cells. Cold Spring Harb Perspect Biol (2014) 6(6):a021857. doi: 10.1101/cshperspect.a021857
76. Cirovic B, Schonheit J, Kowenz-Leutz E, Ivanovska J, Klement C, Pronina N, et al. C/EBP-induced transdifferentiation reveals granulocyte-macrophage precursor-like plasticity of b cells. Stem Cell Rep (2017) 8(2):346–59. doi: 10.1016/j.stemcr.2016.12.015
77. Wynn TA, Vannella KM. Macrophages in tissue repair, regeneration, and fibrosis. Immunity (2016) 44(3):450–62. doi: 10.1016/j.immuni.2016.02.015
78. Schyns J, Bai Q, Ruscitti C, Radermecker C, De Schepper S, Chakarov S, et al. Non-classical tissue monocytes and two functionally distinct populations of interstitial macrophages populate the mouse lung. Nat Commun (2019) 10(1):3964. doi: 10.1038/s41467-019-11843-0
79. Farbehi N, Patrick R, Dorison A, Xaymardan M, Janbandhu V, Wystub-Lis K, et al. Single-cell expression profiling reveals dynamic flux of cardiac stromal, vascular and immune cells in health and injury. Elife (2019) 8:e43882. doi: 10.7554/eLife.43882
80. Chakarov S, Lim HY, Tan L, Lim SY, See P, Lum J, et al. Two distinct interstitial macrophage populations coexist across tissues in specific subtissular niches. Science (2019) 363(6432):eaau0964. doi: 10.1126/science.aau0964
81. Lim HY, Lim SY, Tan CK, Thiam CH, Goh CC, Carbajo D, et al. Hyaluronan receptor LYVE-1-Expressing macrophages maintain arterial tone through hyaluronan-mediated regulation of smooth muscle cell collagen. Immunity (2018) 49(2):326–41 e7. doi: 10.1016/j.immuni.2018.06.008
82. Kim H. The transcription factor MafB promotes anti-inflammatory M2 polarization and cholesterol efflux in macrophages. Sci Rep (2017) 7(1):7591. doi: 10.1038/s41598-017-07381-8
83. Liu M, Tong Z, Ding C, Luo F, Wu S, Wu C, et al. Transcription factor c-maf is a checkpoint that programs macrophages in lung cancer. J Clin Invest (2020) 130(4):2081–96. doi: 10.1172/JCI131335
84. Liao X, Sharma N, Kapadia F, Zhou G, Lu Y, Hong H, et al. Kruppel-like factor 4 regulates macrophage polarization. J Clin Invest (2011) 121(7):2736–49. doi: 10.1172/JCI45444
85. Summers KM, Bush SJ, Hume DA. Network analysis of transcriptomic diversity amongst resident tissue macrophages and dendritic cells in the mouse mononuclear phagocyte system. PloS Biol (2020) 18(10):e3000859. doi: 10.1371/journal.pbio.3000859
86. Sanin DE, Matsushita M, Klein Geltink RI, Grzes KM, van Teijlingen Bakker N, Corrado M, et al. Mitochondrial membrane potential regulates nuclear gene expression in macrophages exposed to prostaglandin E2. Immunity (2018) 49(6):1021–33 e6. doi: 10.1016/j.immuni.2018.10.011
87. Yeh FL, Hansen DV, Sheng M. TREM2, microglia, and neurodegenerative diseases. Trends Mol Med (2017) 23(6):512–33. doi: 10.1016/j.molmed.2017.03.008
88. Hou J, Zhang J, Cui P, Zhou Y, Liu C, Wu X, et al. TREM2 sustains macrophage-hepatocyte metabolic coordination in nonalcoholic fatty liver disease and sepsis. J Clin Invest (2021) 131(4):e135197. doi: 10.1172/JCI135197
89. Audzevich T, Bashford-Rogers R, Mabbott NA, Frampton D, Freeman TC, Potocnik A, et al. Pre/pro-b cells generate macrophage populations during homeostasis and inflammation. Proc Natl Acad Sci U S A. (2017) 114(20):E3954–E63. doi: 10.1073/pnas.1616417114
90. Daheshia M, Yao JQ. The interleukin 1beta pathway in the pathogenesis of osteoarthritis. J Rheumatol (2008) 35(12):2306–12. doi: 10.3899/jrheum.080346
91. Benigni G, Dimitrova P, Antonangeli F, Sanseviero E, Milanova V, Blom A, et al. CXCR3/CXCL10 axis regulates neutrophil-NK cell cross-talk determining the severity of experimental osteoarthritis. J Immunol (2017) 198(5):2115–24. doi: 10.4049/jimmunol.1601359
92. Henn D, Chen K, Fehlmann T, Trotsyuk AA, Sivaraj D, Maan ZN, et al. Xenogeneic skin transplantation promotes angiogenesis and tissue regeneration through activated Trem2(+) macrophages. Sci Adv (2021) 7(49):eabi4528. doi: 10.1126/sciadv.abi4528
93. Kieu TQ, Tazawa K, Kawashima N, Noda S, Fujii M, Nara K, et al. Kinetics of LYVE-1-positive M2-like macrophages in developing and repairing dental pulp in vivo and their pro-angiogenic activity in vitro. Sci Rep (2022) 12(1):5176. doi: 10.1038/s41598-022-08987-3
94. Cochain C, Vafadarnejad E, Arampatzi P, Pelisek J, Winkels H, Ley K, et al. Single-cell RNA-seq reveals the transcriptional landscape and heterogeneity of aortic macrophages in murine atherosclerosis. Circ Res (2018) 122(12):1661–74. doi: 10.1161/CIRCRESAHA.117.312509
Keywords: osteoarthritis, ptoa, scRNA-seq, immune cells, macrophages, inflammation, knee injury, Trem2
Citation: Sebastian A, Hum NR, McCool JL, Wilson SP, Murugesh DK, Martin KA, Rios-Arce ND, Amiri B, Christiansen BA and Loots GG (2022) Single-cell RNA-Seq reveals changes in immune landscape in post-traumatic osteoarthritis. Front. Immunol. 13:938075. doi: 10.3389/fimmu.2022.938075
Received: 06 May 2022; Accepted: 06 July 2022;
Published: 29 July 2022.
Edited by:
Michael V. Volin, Midwestern University, United StatesReviewed by:
Cleo Selina Bonnet, Cardiff University, United KingdomOran D. Kennedy, Royal College of Surgeons in Ireland, Ireland
Copyright © 2022 Sebastian, Hum, McCool, Wilson, Murugesh, Martin, Rios-Arce, Amiri, Christiansen and Loots. This is an open-access article distributed under the terms of the Creative Commons Attribution License (CC BY). The use, distribution or reproduction in other forums is permitted, provided the original author(s) and the copyright owner(s) are credited and that the original publication in this journal is cited, in accordance with accepted academic practice. No use, distribution or reproduction is permitted which does not comply with these terms.
*Correspondence: Aimy Sebastian, c2ViYXN0aWFuNEBsbG5sLmdvdg==; Gabriela G. Loots, bG9vdHMxQGxsbmwuZ292