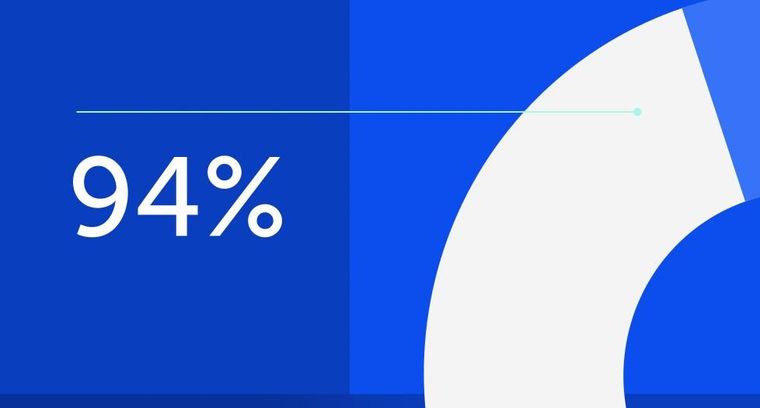
94% of researchers rate our articles as excellent or good
Learn more about the work of our research integrity team to safeguard the quality of each article we publish.
Find out more
ORIGINAL RESEARCH article
Front. Immunol., 19 August 2022
Sec. Inflammation
Volume 13 - 2022 | https://doi.org/10.3389/fimmu.2022.936662
Objective: Platelet (PLT) engages in immune and inflammatory responses, all of which are related to the prognosis of critically ill patients. Although thrombocytopenia at ICU admission contributes to in-hospital mortality, PLT is repeatedly measured during ICU hospitalization and the role of longitudinal PLT trajectory remains unclear. We aimed to identify dynamic PLT trajectory patterns and evaluate their relationships with mortality risk and thrombocytopenia.
Methods: We adopted a three-phase, multi-cohort study strategy. Firstly, longitudinal PLT trajectory patterns within the first four ICU days and their associations with 28-day survival were tested in the eICU Collaborative Research Database (eICU-CRD) and independently validated in the Medical Information Mart for Intensive Care IV (MIMIC-IV) database. Secondly, the relationships among PLT trajectory patterns, thrombocytopenia, and 28-day mortality were explored and validated. Finally, a Mortality GRade system for ICU dynamically monitoring patients (Mortality-GRID) was developed to quantify the mortality risk based on longitudinal PLT, which was further validated in the Molecular Epidemiology of Acute Respiratory Distress Syndrome (MEARDS) cohort.
Results: A total of 35,332 ICU patients were included from three cohorts. Trajectory analysis clustered patients into ascending (AS), stable (ST), or descending (DS) PLT patterns. DS patients with high baseline PLT decline quickly, resulting in poor prognosis. AS patients have low baseline PLT but recover quickly, favoring a better prognosis. ST patients maintain low PLT, having a moderate prognosis in between (HRST vs AS = 1.26, 95% CI: 1.14–1.38, P = 6.15 × 10−6; HRDS vs AS = 1.58, 95% CI: 1.40–1.79, P = 1.41 × 10−13). The associations remained significant in patients without thrombocytopenia during the entire ICU hospitalization and were robust in sensitivity analyses and stratification analyses. Further, the trajectory pattern was a warning sign of thrombocytopenia, which mediated 27.2% of the effects of the PLT trajectory on 28-day mortality (HRindirect = 1.11, 95% CI: 1.06–1.17, P = 9.80 × 10−6). Mortality-GRID well predicts mortality risk, which is in high consistency with that directly estimated in MEARDS (r = 0.98, P = 1.30 × 10−23).
Conclusion: Longitudinal PLT trajectory is a complementary predictor to baseline PLT for patient survival, even in patients without risk of thrombocytopenia. Mortality-GRID could identify patients at high mortality risk.
The intensive care unit (ICU) patients are exposed to pneumonia, sepsis, and other inflammation (1, 2), which hampers the treatment and leads to high mortality rates ranging from 11% to 18% (3, 4). Survivors may suffer from chronic and life-changing physical, psychosocial, and cognitive sequelae (5, 6). Such conditions deteriorate rapidly under the ongoing novel coronavirus disease 2019 (COVID-19) pandemic (7) and cause a considerable burden on public health (8, 9).
Platelets engage in coagulation, inflammation, and the immune response, all of which have close connections with the prognosis of critically ill patients (10, 11). Platelets play a central role in regulating immune responses and interactions with various cells of the innate and adaptive immune systems (12, 13). Functional or quantitative platelet abnormalities may lead to immune dysregulation, prolonged ICU stay, and death (14). Indeed, thrombocytopenia (low platelet count) at ICU admission is associated with poor clinical outcomes and prognosis (15, 16). Accumulating pieces of evidence show that platelet counts vary over ICU hospitalization, with levels dropping after ICU admission and reaching a nadir on the fourth day (17, 18). In addition to the baseline platelet count at admission, such platelet dynamics might affect ICU patient prognosis (19). However, the role of the longitudinal platelet count trajectory has not been elucidated.
Taking advantage of large-scale databases including the eICU Collaborative Research Database (eICU-CRD) and the Medical Information Mart for Intensive Care IV (MIMIC-IV) database, we applied an unsupervised machine learning approach to identify longitudinal platelet count trajectory patterns among ICU patients and evaluate their associations with clinical outcomes. Further, we developed a Mortality Grade system for ICU dynamically monitoring patients (Mortality-GRID) and validated it in the Molecular Epidemiology of Acute Respiratory Distress Syndrome (MEARDS) cohort.
Study samples were collected from eICU-CRD v2.0 (2014–2015) (20) and MIMIC-IV v1.0 (2008–2019) (21) at PhysioNet (22) (certification number: 33755029), as well as the MEARDS cohort (1998–2014) (16, 23). In accordance with previous studies, we excluded ICU admissions if they met any of the following criteria: (i) repeated ICU admissions; (ii) age <18 years; (iii) missing baseline platelet count; (iv) length of ICU stay <48 h (24, 25); (v) daily platelet count measurements <4 times (because of the requirement for trajectory analysis). Detailed cohort descriptions are summarized in the Supplementary Methods. Finally, 19,361, 14,239, and 1,732 ICU patients who met the recruitment criteria were collected from the eICU-CRD, MIMIC-IV, and MEARDS cohorts, respectively.
The primary outcome was 28-day in-hospital survival, defined as a time-to-event outcome from ICU admission to death or loss to follow-up at the end of the study, whichever occurred first. The primary independent variable was daily platelet count measurements in the first four consecutive days after ICU admission. The lowest platelet count was used if the patient had multiple platelet count measures in a single day. Additionally, demographic information, clinical characteristics, laboratory tests, vital signs, and comorbidities were extracted for covariate adjustment and stratified analyses, summarized in Supplementary Tables 1–5. Only variables with a missing proportion of less than 10% were included in further analyses (Supplementary Figures 1, 2).
Trajectory analysis was performed to identify dynamic trends based on longitudinal repeatedly measured platelet count in the first four ICU days using the R package traj, as proposed by Leffondré etal. (26). We followed three steps to identify trajectory patterns. Firstly, we extracted 24 features to depict platelet count dynamic trajectories for each individual (Supplementary Table 6). Secondly, correlations between these 24 features were tested, and factor analysis was conducted to select a subset of features that best described the dynamic changes of the trajectories. Finally, based on the selected features, cluster analysis was used to classify patients with similar dynamic changes into the same group. The optimal number of clusters was determined based on the lowest −2*log-likelihood value, the Akaike information criterion, and Bayesian information criteria of the corresponding models.
Thrombocytopenia is an important prognostic predictor of in-hospital mortality in ICU patients. To test whether the dynamic platelet count trajectory could provide warning signs for thrombocytopenia and further affect patient survival, the Vander Weele causal mediation analysis was performed to evaluate the indirect effect of the dynamic trajectory on 28-day survival (27).
To quantify the mortality risk of dynamic platelet count changes, we computed the average daily change in platelet count after ICU admission. Considering that patients with different baseline platelet counts at admission may have different mortality risks, we classified patients according to the normal range of platelet counts in the ICU (100–300 × 109/L) (28). Patients with no change in platelet count were set as the reference group, and the effects of platelet count changes on 28-day mortality were evaluated using hazard ratios (HRs) derived from restricted cubic spline using the R package rms. Mortality-GRID was developed based on the risk quantization to provide warnings and prophylactic therapy for vulnerable ICU patients.
We conducted a three-phase, multi-cohort study. Firstly, longitudinal platelet count trajectory patterns within the first four ICU days and their associations with 28-day survival were tested in eICU-CRD and further independently validated in MIMIC-IV. Furthermore, a series of stratified and sensitivity analyses were performed to evaluate the robustness of the associations.
Secondly, based on eICU-CRD and MIMIC-IV databases, we explored and validated whether the longitudinal trajectory patterns could provide a warning sign of thrombocytopenia and, through which, mediate the effect of trajectory patterns on patient survival. Additionally, although thrombocytopenia is common in the ICU, considerable patients without thrombocytopenia remain hospitalized during their entire ICU hospitalization. We further clarified the role of dynamic trajectory patterns in such a population.
Finally, we quantified the mortality risk based on the average platelet count change per day and developed Mortality-GRID by combining the eICU-CRD and MIMIC-IV databases to achieve robust parameter estimates. Moreover, the proposed Mortality-GRID was applied in an independent MEARDS cohort. Patients with MEARDS were classified into low-, middle-, and high-risk subgroups by the death hazard tertiles predicted by Mortality-GRID. Then, the survival differences between the subgroups were compared.
The survival differences between trajectory patterns were assessed using multivariable Cox proportional hazards models and illustrated by Kaplan–Meier survival curves. Statistical analyses were performed using the R version 3.6.3. The significance level was defined as two-sided 0.05. The source code was deposited on GitHub: https://github.com/JiajinChen/trajPLT.
Trajectory analysis identified three distinct longitudinal platelet count trajectory patterns in eICU-CRD (Supplementary Table 7): (i) ascending (AS), in which the platelet count was elevated after ICU admission; (ii) stable (ST), in which the platelet count remained relatively unchanged; and (iii) descending (DS), in which the platelet count declined quickly after ICU admission (Figure 1A). Similar patterns were observed upon validation in the MIMIC-IV dataset using the model trained in eICU-CRD (Figure 1B).
Figure 1 Trajectory plot and Kaplan–Meier survival curves of patients with three dynamic platelet count trajectory patterns. (A, B) Trajectory plot of platelet count changes within the first four days after ICU admission in eICU-CRD and MIMIC-IV databases. (C, D) Kaplan–Meier curves of 28-day overall survival for patients with three different dynamic platelet count trajectory patterns in the eICU-CRD and MIMIC-IV databases.
A broad list of demographics, clinical characteristics, laboratory measurements, and vital signs collected at ICU admission were compared across the three trajectory patterns in eICU-CRD (Supplementary Tables 8–11) and MIMIC-IV databases (Supplementary Tables 12–15). Significantly different characteristics across these three patterns were considered potential covariates and adjusted in subsequent models.
A univariate Cox proportional hazards model indicated that patients with ST or DS patterns had significantly worse 28-day survival than those with an AS pattern in eICU-CRD (Figure 1C) and MIMIC-IV datasets (Figure 1D). Further, we verified the robustness of the results by developing six models adjusted for a list of sequentially adding-on covariates (Model1–5 and ModelPS in Table 1). The model was adjusted first for demographics and baseline platelet count (Model1), followed by step-forward add-on adjustments for predispositions and supports (Model2), vital signs (Model3), unevenly distributed laboratory measurements (Model4), and comorbidities (Model5). All models retained consistently significant associations (Table 1). Further, the propensity score model incorporating all these covariates retained consistent results (HRST vs AS = 1.26, 95% CI: 1.14–1.38, P = 6.15 × 10−6; HRDS vs AS = 1.58, 95% CI: 1.40–1.79, P = 1.41 × 10−13) (ModelPS in Table 1). Moreover, the associations remained significant in subgroup analyses (Supplementary Figure 3).
Table 1 Sensitivity analyses for association between platelet count trajectories and 28-day overall survival.
Furthermore, a series of analyses stratified by demographics, severity score, support within 24 h, and comorbidities were performed. Significant associations between platelet count dynamic trajectory pattern and 28-day mortality were observed in most strata in the eICU-CRD and MIMIC-IV databases (Figure 2 and Supplementary Figures 4–5), except for a few strata probably due to insufficient sample size (Supplementary Figures 6–11). Notably, significant heterogeneity in the association was observed between the age subgroups. ICU patients aged <65 years with either an ST (PHeterogeneity = 0.024) or DS (PHeterogeneity = 0.005) dynamic platelet count trend had higher mortality than patients ≥65 years old (Figure 2). Also, significant heterogeneity was observed in the different baseline platelet count subgroups at ICU admission (PHeterogeneity <0.05) (Figure 2).
Figure 2 Forest plots of stratified associations between platelet count trajectory patterns and 28-day survival of ICU patients. Meta-analysis was conducted to pool the results from the eICU-CRD and MIMIC-IV databases. The effects across strata were tested using heterogeneity test.
Thrombocytopenia is an important prognostic predictor of ICU patient mortality, regardless of when it occurs (Supplementary Table 16). Furthermore, we observed the dynamic platelet count trajectory during the first four ICU days as a powerful predictor of thrombocytopenia risk during the following days of ICU hospitalization in the eICU-CRD (ORST vs AS = 3.20, 95% CI: 2.36–4.33, P = 5.73 × 10−14; ORDS vs AS = 6.84, 95% CI: 4.95–9.45, P = 1.73 × 10−31) and the MIMIC-IV dataset (ORST vs AS = 2.58, 95% CI: 1.84–3.61, P = 3.47 × 10−8; ORDS vs AS = 6.27, 95% CI: 4.27–9.20, P = 7.61 × 10−21). The associations were robust in six models, adjusting for a list of sequentially adding-on covariates (Supplementary Table 17).
Furthermore, to test whether dynamic platelet count trajectory in the first four days after ICU admission could affect patient survival via thrombocytopenia, we performed a causal mediation analysis and a significant indirect effect on 28-day survival was observed in both eICU-CRD and MIMIC-IV datasets (HRIndirect = 1.11, 95% CI: 1.06–1.17, P = 9.80 × 10−6, 27.2% of effects mediated) (Figure 3). On the other hand, there were still over 70% of the effects of platelet count trajectory affecting patient mortality through underlying mechanisms rather than thrombocytopenia.
Figure 3 Causal mediation analysis for platelet count dynamic trajectory, thrombocytopenia, and 28-day survival. (A) Mediation model for the effect of platelet count dynamic trajectory on 28-day survival through thrombocytopenia. (B) Results are described as average causal mediated effect (indirect hazard ratio), 95% confidence interval and the proportion of effect mediated (M%).
Indeed, though thrombocytopenia is common in critically ill patients (31.2% in eICU-CRD and 30.1% in MIMIC-IV), a considerable proportion of patients without thrombocytopenia remain during the entire course of ICU hospitalization. Hence, we further conducted a subgroup analysis among patients with no history of thrombocytopenia during the whole ICU hospitalization. The associations between platelet count dynamic trajectory and 28-day mortality remained significant in eICU-CRD (HRST vs AS = 1.22, 95% CI: 1.02–1.47, P = 3.02 × 10−2; HRDS vs AS = 1.73, 95% CI: 1.39–2.15, P = 9.41 × 10−7) and MIMIC-IV (HRST vs AS = 1.48, 95% CI: 1.17–1.87, P = 9.11 × 10−4; HRDS vs AS = 2.13, 95% CI: 1.57–2.88, P = 1.13 × 10−6), respectively (Supplementary Figure 12), suggesting that dynamic platelet count trajectory was an independent prognostic predictor of 28-day mortality of ICU patients, even in patients who never developed thrombocytopenia.
Considering the heterogeneity between patients with different baseline platelet counts at ICU admission, we stratified patients according to the normal platelet count range. A significant dose–response relationship between daily platelet count change and 28-day mortality was observed in the eICU-CRD and MIMIC-IV datasets (Supplementary Figure 13). Compared to patients with no change in platelet count, patients with a daily decline in platelet count in the first four days by 10 × 109/L and a baseline platelet count of <100, between 100 and 300, or >300 had a 51% (HR = 1.51, 95% CI: 1.25–1.83), 16% (HR = 1.16, 95% CI: 1.12–1.20), or 8% (HR = 1.08, 95% CI: 1.03–1.13) increased risk of death, respectively. On the other hand, patients with a baseline platelet count below 100 × 109/L and a daily platelet count increment of 10 × 109/L, a baseline platelet count in the normal range with a daily increment reaching 22 × 109/L, or a baseline platelet count more than 300 × 109/L with an increment of 31 × 109/L, had a 25% decreased risk of death (Supplementary Figure 13).
Based on the mortality quantization, we derived Mortality-GRID using the baseline platelet count at ICU admission and daily platelet count change in the first four days of ICU hospitalization to indicate patient death hazards (Figure 4A). Using this model, one can assign a hazard risk to a patient by their average daily platelet count change. For example, suppose ICU patients with a baseline platelet count of 200 × 109/L have an average platelet count decline of 30 × 109/L, which might cause a 1.58-times mortality risk compared to those with an average platelet count change equal to 0 (stable status). Subsequently, we independently estimated the corresponding risk of daily platelet count change across different baseline platelet count groups in MEARDS (Supplementary Figure 14). Mortality risks predicted by Mortality-GRID were consistent with those directly estimated in MEARDS (r = 0.98, P = 1.30 × 10−23) (Figure 4B), indicating the clinical applicability of Mortality-GRID. Furthermore, we applied Mortality-GRID to the MEARDS cohort and predicted patients’ hazard risk. Patients were then categorized into high-, middle-, and low-risk groups using the tertiles of their hazard risk values. Based on the significant survival differences between groups, the proposed Mortality-GRID successfully identified patients with unfavorable survival (Figure 4C).
Figure 4 Mortality-GRID and validation in the MEARDS database. (A) Mortality-GRID. Patients with no change in platelet count per day were set as the reference group, the values in each cell represent hazard ratios of platelet count changes per day derived from restricted cubic spline regression. (B) Consistency between mortality risks estimated by Mortality-GRID and MEARDS database presented by scatter plot. (C) Estimated survival curves for patients in the MEARDS database. The mortality risks of MEARDS patients were independently predicted by Mortality-GRID and categorized into low-, medium-, and high-risk groups using the tertiles.
To our knowledge, this is the first study to integrate multiple large-scale databases and apply machine learning methods to identify longitudinal platelet count trajectory patterns and validate their relationship with the prognosis of ICU patients. Such associations were robust in sensitivity analyses, and even remained significant among patients without thrombocytopenia during the entire ICU hospitalization. Moreover, the trajectory is a warning sign of thrombocytopenia, which affects patient survival.
Compared to the absolute platelet count at a specific time, longitudinal data provide more information on disease progression (29, 30). Trajectory analysis can uncover the hidden values of repeated measurements. Our study indicated that patients without thrombocytopenia at ICU admission but who had a rapidly declining platelet count during hospitalization might eventually have a poor prognosis.
Among the three trajectory patterns, the descending pattern was characterized as having a high mortality risk, whereas ICU patients with an elevated platelet count had a favorable clinical outcome. Platelets are well recognized in hemostasis by promoting vasoconstriction and platelet aggregation. Moreover, platelets can release proangiogenic factors (such as VEGF) that induce the proliferation and migration of fibroblasts and endothelial cells, thereby contributing to the repair of vascular epithelial damage (31). Most ICU patients experience inflammatory stress or infection, while platelets induce the proliferation, maturation, and activation of immune cells and interact with Toll-like receptors (TLRs) (32), which can recognize pathogen or virus, and are involved in the immune response (33). Thrombocytopenia is a common sign of poor prognosis in ICU patients (34). The decline of platelet count may result in weakened immune function, while the increased platelet count may reflect the elimination of pathogens and the recovery of immune defense (35–37), which contributes to the recovery of ICU patients. Nevertheless, excess platelets are a risk factor for thromboembolism (38), and special attention is required for ICU patients at risk of thromboembolic diseases.
A series of stratified analyses showed robust associations across strata. Notably, we found the risk of descending platelet count was lower among elderly adults (≥65 years). In older adults, platelet activation, aggregation, and secretion were enhanced, and maintaining a relatively low platelet count may relieve the risk of thrombosis and inflammation (39, 40). Further platelet count decline may exacerbate the incidence of adverse events among patients with a low platelet count at admission (41). Moreover, platelet engage in the antivirus and anti-infection processes by forming neutrophil extracellular traps (NET), which enhance leukocyte recruitment and inflammatory factor activation (42). These mechanisms might induce plasma coagulation factors, resulting in pro-inflammatory responses and thrombosis (43). Our results also indicated the heterogeneous effects of platelet trajectory between patients with and without thromboembolic diseases, suggesting the necessity of maintaining a relatively balanced platelet count in patients with thromboembolic disease. Although the associations between platelet trajectory and 28-day mortality were not observed in the subgroup of patients with thromboinflammatory diseases, that might be due to the insufficient sample size. The further heterogeneity suggested no heterogeneity between patients with and without thromboinflammatory diseases. Nevertheless, emerging pieces of evidence have underlined the unique role of platelets in COVID-19, a thromboinflammatory disease (44, 45). During COVID-19, platelets get activated through multiple mechanisms involving the global inflammatory reaction, the dysfunctional endothelium, increased thrombin, or plausible direct viral infection (44). Moreover, the activated platelets can interact with neutrophils, which mediated the inflammation and thrombosis (46). The platelet–neutrophil interaction may trigger the release of NETs (47), which contribute to acute organ failure, ARDS, and mortality in COVID-19 (48). Further data is needed for validation of our model in populations with COVID-19. In addition, several factors (i.e., platelet treatment, transfusion amount, hematologic diseases) may affect the associations. We therefore adjusted them in the models and performed sensitivity analyses by excluding patients with platelet treatment. The associations remained significant in the sensitivity analyses, indicating the robustness of our results.
Additionally, our results showed that the dynamic trajectory of platelet count could be a warning sign for thrombocytopenia and affect ICU patient mortality. For about 30% of the effect of platelet count decline in mortality that is mediated by thrombocytopenia, the trajectory patterns could provide considerable information through other underlying mechanisms. Platelet count can reflect the function and stress response of organs, such as the lung, liver, and kidney (49, 50). Notably, even in patients without thrombocytopenia during their entire ICU hospitalization, the dynamic change in platelet count retained a significant effect on prognosis, which might benefit nearly 70% of ICU patients without thrombocytopenia. Although the daily platelet change seems small, it reflects the dynamic trend, which powerfully predicts mortality. Consequently, the Mortality-GRID system based on accessible daily platelet count could help identify high-risk patients, aid clinical decisions, and guide therapy.
Our study has several strengths. First, we analyzed two large-scale databases, providing robust results. Moreover, these two databases have extensive documentation and public code for researchers. We also released the source code for the audit and tried to ensure reliable data quality and results. Second, we used longitudinal platelet count measures over the first four days of ICU hospitalization to identify three novel dynamic trajectory patterns, which could predict patient prognosis and would considerably benefit clinical decision-making. Third, we used the causal inference method and confirmed the applicability of trajectory patterns for warning signs in patients without thrombocytopenia. Fourth, we adopted a three-phase study design and unsupervised trajectory analysis to better identify the underlying information, along with a series of sensitivity analyses and stratified analyses to guarantee the robustness of the findings. Finally, we provided Mortality-GRID to assist physicians with warning sign detection based on platelet count changes, dynamical assessment for mortality hazards, and early identification of high-risk patients, and then provide personalized prophylactic therapy, i.e., platelet therapy.
We acknowledge some limitations in our study. First, since our samples are mainly Caucasian, a large dataset with other ethnicities is warranted to confirm the applicability of our findings across different ethnicities. Second, more patients had sepsis predisposition in MIMIC-IV than in eICU-CRD. The database constructor provided sepsis 3.0 diagnostics in MIMIC-IV; this variable was not provided in the eICU-CRD database and was manually extracted from the diagnostic texts; thus, sepsis was probably under-diagnosed. However, no heterogeneity was observed between sepsis and non-sepsis subgroups in the two databases. Thus, the potential under-diagnosis of sepsis in eICU-CRD had no impact on the findings of this study. Third, although our study included three databases, heterogeneity might exist across these databases. Nevertheless, the association remained significant across these heterogeneous populations, indicating the robustness of our results.
In summary, we identified three distinct longitudinal platelet count trajectory patterns within the first four ICU days. The platelet count trajectory is a better predictor of patient survival than merely the baseline platelet count. These associations are partially mediated by thrombocytopenia and remain significant in patients without risk of thrombocytopenia. The proposed Mortality-GRID could facilitate prognostic warnings for vulnerable ICU patients.
The MIMIC-IV and eICU-CRD databases are available in PhysioNet (https://physionet.org). MEARDS cohort is available from the corresponding authors on reasonable request.
eICU and MIMIC databases were approved by the Institutional Review Boards (IRB) of the Massachusetts Institute of Technology, and the informed consent has been waived as they do not contain protected health information. MEARDS cohort was reviewed and approved by IRB of Harvard T. H. Chan School of Public Health, MGH and BIDMC, and all participants provided their written informed consent.
YW and RZ designed and supervised the study. JC and LS curated and harmonized the data. JC, XG, SS, and ZS performed statistical analyses. ZD and RL double-checked all analysis code. JC, XG, and LS drafted the manuscript. FC and YW critically reviewed all statistical methods, procedures, and results. DC provided data from MEARDS and reviewed all clinical interpretations. SS, RZ, YW, FC, and DC critically revised the manuscript. All authors listed have made a substantial, direct, and intellectual contribution to the work and approved it for publication.
This study was supported by the National Natural Science Foundation of China (81973142 to YW), the Natural Science Foundation of Jiangsu Province (BK20191354 to RZ), the China Postdoctoral Science Foundation (2020M681671 to SS), and the Jiangsu Planned Projects for Postdoctoral Research Funds (2020Z019 to SS). RZ was partially supported by the Qing Lan Project of the Higher Education Institutions of Jiangsu Province and the Outstanding Young Level Academic Leadership Training Program of Nanjing Medical University.
The authors acknowledge all participants in the eICU, MIMIC, and MEARDS studies, and the Laboratory for Computational Physiology team from the Massachusetts Institute of Technology (LCP-MIT) who maintain the eICU-CRD and MIMIC-IV databases.
The authors declare that the research was conducted in the absence of any commercial or financial relationships that could be construed as a potential conflict of interest.
All claims expressed in this article are solely those of the authors and do not necessarily represent those of their affiliated organizations, or those of the publisher, the editors and the reviewers. Any product that may be evaluated in this article, or claim that may be made by its manufacturer, is not guaranteed or endorsed by the publisher.
The Supplementary Material for this article can be found online at: https://www.frontiersin.org/articles/10.3389/fimmu.2022.936662/full#supplementary-material
eICU-CRD, eICU Collaborative Research Database; MIMIC-IV, Medical Information Mart for Intensive Care IV database; ICU, intensive care unit; MEARDS, Molecular Epidemiology of Acute Respiratory Distress Syndrome; PLT, platelet count; Mortality-GRID, Mortality Grade system for ICU Dynamically monitoring patients; CI, confidence interval; HR, hazard ratios; OR, odds ratio; PS, propensity score.
1. Van Vught LA, Klein Klouwenberg PM, Spitoni C, Scicluna BP, Wiewel MA, Horn J, et al. Incidence, risk factors, and attributable mortality of secondary infections in the intensive care unit after admission for sepsis. JAMA (2016) 315:1469–79. doi: 10.1001/jama.2016.2691
2. Cillóniz C, Torres A, Niederman MS. Management of pneumonia in critically ill patients. BMJ (2021) 375:e065871. doi: 10.1136/bmj-2021-065871
3. Zimmerman JE, Kramer AA, Knaus WA. Changes in hospital mortality for united states intensive care unit admissions from 1988 to 2012. Crit Care (2013) 17:R81. doi: 10.1186/cc12695
4. Pirracchio R, Petersen ML, Carone M, Rigon MR, Chevret S, van der Laan MJ. Mortality prediction in intensive care units with the super ICU learner algorithm (SICULA): a population-based study. Lancet Respir Med (2015) 3:42–52. doi: 10.1016/S2213-2600(14)70239-5
5. Sareen J, Olafson K, Kredentser MS, Bienvenu OJ, Blouw M, Bolton JM, et al. The 5-year incidence of mental disorders in a population-based ICU survivor cohort. Crit Care Med (2020) 48:e675–83. doi: 10.1097/CCM.0000000000004413
6. Geense WW, Zegers M, Peters M, Ewalds E, Simons KS, Vermeulen H, et al. New physical, mental, and cognitive problems 1 year after ICU admission: A prospective multicenter study. Am J Respir Crit Care Med (2021) 203:1512–21. doi: 10.1164/rccm.202009-3381OC
7. Leisman DE, Deutschman CS, Legrand M. Facing COVID-19 in the ICU: vascular dysfunction, thrombosis, and dysregulated inflammation. Intensive Care Med (2020) 46:1105–8. doi: 10.1007/s00134-020-06059-6
8. Adhikari NK, Fowler RA, Bhagwanjee S, Rubenfeld GD. Critical care and the global burden of critical illness in adults. Lancet (2010) 376:1339–46. doi: 10.1016/S0140-6736(10)60446-1
9. Schell CO, Gerdin Wärnberg M, Hvarfner A, Höög A, Baker U, Castegren M, et al. The global need for essential emergency and critical care. Crit Care (2018) 22:284. doi: 10.1186/s13054-018-2219-2
10. Middleton EA, Weyrich AS, Zimmerman GA. Platelets in pulmonary immune responses and inflammatory lung diseases. Physiol Rev (2016) 96:1211–59. doi: 10.1152/physrev.00038.2015
11. Brummel NE, Hughes CG, Thompson JL, Jackson JC, Pandharipande P, Mcneil JB, et al. Inflammation and coagulation during critical illness and long-term cognitive impairment and disability. Am J Respir Crit Care Med (2021) 203:699–706. doi: 10.1164/rccm.201912-2449OC
12. Semple JW, Italiano JE Jr, Freedman J. Platelets and the immune continuum. Nat Rev Immunol (2011) 11:264–74. doi: 10.1038/nri2956
13. Koupenova M, Clancy L, Corkrey HA, Freedman JE. Circulating platelets as mediators of immunity, inflammation, and thrombosis. Circ Res (2018) 122:337–51. doi: 10.1161/CIRCRESAHA.117.310795
14. Blumberg N, Cholette JM, Schmidt AE, Phipps RP, Spinelli SL, Heal JM, et al. Management of platelet disorders and platelet transfusions in ICU patients. Transfus Med Rev (2017) 31:252–7. doi: 10.1016/j.tmrv.2017.04.002
15. Williamson DR, Lesur O, Tétrault JP, Nault V, Pilon D. Thrombocytopenia in the critically ill: prevalence, incidence, risk factors, and clinical outcomes. Can J Anaesth (2013) 60:641–51. doi: 10.1007/s12630-013-9933-7
16. Wei Y, Tejera P, Wang Z, Zhang R, Chen F, Su L, et al. A missense genetic variant in LRRC16A/CARMIL1 improves acute respiratory distress syndrome survival by attenuating platelet count decline. Am J Respir Crit Care Med (2017) 195:1353–61. doi: 10.1164/rccm.201605-0946OC
17. Akca S, Haji-Michael P, De Mendonça A, Suter P, Levi M, Vincent JL. Time course of platelet counts in critically ill patients. Crit Care Med (2002) 30:753–6. doi: 10.1097/00003246-200204000-00005
18. Greinacher A, Selleng K. Thrombocytopenia in the intensive care unit patient. Hematol Am Soc Hematol Educ Program (2010) 2010:135–43. doi: 10.1182/asheducation-2010.1.135
19. Nelson B, Mcgorry PD, Wichers M, Wigman JTW, Hartmann JA. Moving from static to dynamic models of the onset of mental disorder: A review. JAMA Psychiatry (2017) 74:528–34. doi: 10.1001/jamapsychiatry.2017.0001
20. Pollard TJ, Johnson AEW, Raffa JD, Celi LA, Mark RG, Badawi O. The eICU collaborative research database, a freely available multi-center database for critical care research. Sci Data (2018) 5:180178. doi: 10.1038/sdata.2018.178
21. Johnson AE, Pollard TJ, Shen L, Lehman LW, Feng M, Ghassemi M, et al. MIMIC-III, a freely accessible critical care database. Sci Data (2016) 3:160035. doi: 10.1038/sdata.2016.35
22. Goldberger AL, Amaral LA, Glass L, Hausdorff JM, Ivanov PC, Mark RG, et al. PhysioBank, PhysioToolkit, and PhysioNet: components of a new research resource for complex physiologic signals. Circulation (2000) 101:e215–20. doi: 10.1161/01.CIR.101.23.e215
23. Zhang R, Wang Z, Tejera P, Frank AJ, Wei Y, Su L, et al. Late-onset moderate to severe acute respiratory distress syndrome is associated with shorter survival and higher mortality: a two-stage association study. Intensive Care Med (2017) 43:399–407. doi: 10.1007/s00134-016-4638-3
24. Serpa Neto A, Deliberato RO, Johnson AEW, Bos LD, Amorim P, Pereira SM, et al. Mechanical power of ventilation is associated with mortality in critically ill patients: an analysis of patients in two observational cohorts. Intensive Care Med (2018) 44:1914–22. doi: 10.1007/s00134-018-5375-6
25. Zhao GJ, Xu C, Ying JC, Lü WB, Hong GL, Li MF, et al. Association between furosemide administration and outcomes in critically ill patients with acute kidney injury. Crit Care (2020) 24:75. doi: 10.1186/s13054-020-2798-6
26. Leffondré K, Abrahamowicz M, Regeasse A, Hawker GA, Badley EM, Mccusker J, et al. Statistical measures were proposed for identifying longitudinal patterns of change in quantitative health indicators. J Clin Epidemiol (2004) 57:1049–62. doi: 10.1016/j.jclinepi.2004.02.012
27. Vanderweele TJ. Causal mediation analysis with survival data. Epidemiology (2011) 22:582–5. doi: 10.1097/EDE.0b013e31821db37e
28. Drews RE, Weinberger SE. Thrombocytopenic disorders in critically ill patients. Am J Respir Crit Care Med (2000) 162:347–51. doi: 10.1164/ajrccm.162.2.ncc3-00
29. Gerig G, Fishbaugh J, Sadeghi N. Longitudinal modeling of appearance and shape and its potential for clinical use. Med Image Anal (2016) 33:114–21. doi: 10.1016/j.media.2016.06.014
30. Zhao L, Murray S, Mariani LH, Ju W. Incorporating longitudinal biomarkers for dynamic risk prediction in the era of big data: A pseudo-observation approach. Stat Med (2020) 39:3685–99. doi: 10.1002/sim.8687
31. Cognasse F, Laradi S, Berthelot P, Bourlet T, Marotte H, Mismetti P, et al. Platelet inflammatory response to stress. Front Immunol (2019) 10:1478. doi: 10.3389/fimmu.2019.01478
32. Hally K, Fauteux-Daniel S, Hamzeh-Cognasse H, Larsen P, Cognasse F. Revisiting platelets and toll-like receptors (TLRs): At the interface of vascular immunity and thrombosis. Int J Mol Sci (2020) 21:6150. doi: 10.3390/ijms21176150
33. Marcoux G, Laroche A, Hasse S, Bellio M, Mbarik M, Tamagne M, et al. Platelet EVs contain an active proteasome involved in protein processing for antigen presentation via MHC-I molecules. Blood (2021) 138:2607–20. doi: 10.1182/blood.2020009957
34. Jonsson AB, Rygård SL, Hildebrandt T, Perner A, Møller MH, Russell L. Thrombocytopenia in intensive care unit patients: A scoping review. Acta Anaesthesiol Scand (2021) 65:2–14. doi: 10.1111/aas.13699
35. Eisinger F, Patzelt J, Langer HF. The platelet response to tissue injury. Front Med (2018) 5:317. doi: 10.3389/fmed.2018.00317
36. Margraf A, Zarbock A. Platelets in inflammation and resolution. J Immunol (2019) 203:2357–67. doi: 10.4049/jimmunol.1900899
37. Mcdonald B, Dunbar M. Platelets and intravascular immunity: Guardians of the vascular space during bloodstream infections and sepsis. Front Immunol (2019) 10:2400. doi: 10.3389/fimmu.2019.02400
38. Panova-Noeva M, Wagner B, Nagler M, Koeck T, Ten Cate V, Prochaska JH, et al. Comprehensive platelet phenotyping supports the role of platelets in the pathogenesis of acute venous thromboembolism - results from clinical observation studies. EBioMedicine (2020) 60:102978. doi: 10.1016/j.ebiom.2020.102978
39. Mohebali D, Kaplan D, Carlisle M, Supiano MA, Rondina MT. Alterations in platelet function during aging: clinical correlations with thromboinflammatory disease in older adults. J Am Geriatr Soc (2014) 62:529–35. doi: 10.1111/jgs.12700
40. Le Blanc J, Lordkipanidzé M. Platelet function in aging. Front Cardiovasc Med (2019) 6:109. doi: 10.3389/fcvm.2019.00109
41. Hui P, Cook DJ, Lim W, Fraser GA, Arnold DM. The frequency and clinical significance of thrombocytopenia complicating critical illness: a systematic review. Chest (2011) 139:271–8. doi: 10.1378/chest.10-2243
42. Kim SJ, Jenne CN. Role of platelets in neutrophil extracellular trap (NET) production and tissue injury. Semin Immunol (2016) 28:546–54. doi: 10.1016/j.smim.2016.10.013
43. Stevens H, Mcfadyen JD. Platelets as central actors in thrombosis-reprising an old role and defining a new character. Semin Thromb Hemost (2019) 45:802–9. doi: 10.1055/s-0039-1698829
44. Taus F, Salvagno G, Canè S, Fava C, Mazzaferri F, Carrara E, et al. Platelets promote thromboinflammation in SARS-CoV-2 pneumonia. Arterioscler Thromb Vasc Biol (2020) 40:2975–89. doi: 10.1161/ATVBAHA.120.315175
45. Baumann Kreuziger L, Sholzberg M, Cushman M. Anticoagulation in hospitalized patients with COVID-19. Blood (2022). doi: 10.1182/blood.2021014527
46. Ahmad F, Kannan M, Ansari AW. Role of SARS-CoV-2 -induced cytokines and growth factors in coagulopathy and thromboembolism. Cytokine Growth Factor Rev (2022) 63:58–68. doi: 10.1016/j.cytogfr.2021.10.007
47. Mcfadyen JD, Stevens H, Peter K. The emerging threat of (Micro)Thrombosis in COVID-19 and its therapeutic implications. Circ Res (2020) 127:571–87. doi: 10.1161/CIRCRESAHA.120.317447
48. Barnes BJ, Adrover JM, Baxter-Stoltzfus A, Borczuk A, Cools-Lartigue J, Crawford JM, et al. Targeting potential drivers of COVID-19: Neutrophil extracellular traps. J Exp Med (2020) 217:e20200652. doi: 10.1084/jem.20200652
49. Lambert MP. Platelets in liver and renal disease. Hematol Am Soc Hematol Educ Program (2016) 2016:251–5. doi: 10.1182/asheducation-2016.1.251
Keywords: platelet count, inflammation, immunity, critical care, longitudinal trajectory, multi-cohort, prognosis
Citation: Chen J, Gao X, Shen S, Xu J, Sun Z, Lin R, Dai Z, Su L, Christiani DC, Chen F, Zhang R and Wei Y (2022) Association of longitudinal platelet count trajectory with ICU mortality: A multi-cohort study. Front. Immunol. 13:936662. doi: 10.3389/fimmu.2022.936662
Received: 05 May 2022; Accepted: 15 July 2022;
Published: 19 August 2022.
Edited by:
Fabrice Cognasse, INSERM U1059 SAnté INgéniérie BIOlogie, FranceReviewed by:
Chenyu Fan, Shanghai Jiao Tong University, ChinaCopyright © 2022 Chen, Gao, Shen, Xu, Sun, Lin, Dai, Su, Christiani, Chen, Zhang and Wei. This is an open-access article distributed under the terms of the Creative Commons Attribution License (CC BY). The use, distribution or reproduction in other forums is permitted, provided the original author(s) and the copyright owner(s) are credited and that the original publication in this journal is cited, in accordance with accepted academic practice. No use, distribution or reproduction is permitted which does not comply with these terms.
*Correspondence: Ruyang Zhang, emhhbmdydXlhbmdAbmptdS5lZHUuY24=; Yongyue Wei, eXdlaUBuam11LmVkdS5jbg==
†These authors have contributed equally to this work
Disclaimer: All claims expressed in this article are solely those of the authors and do not necessarily represent those of their affiliated organizations, or those of the publisher, the editors and the reviewers. Any product that may be evaluated in this article or claim that may be made by its manufacturer is not guaranteed or endorsed by the publisher.
Research integrity at Frontiers
Learn more about the work of our research integrity team to safeguard the quality of each article we publish.