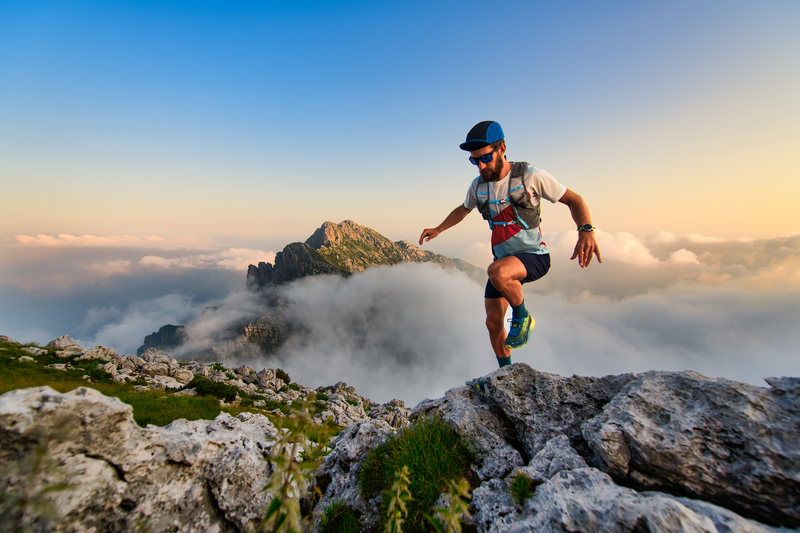
94% of researchers rate our articles as excellent or good
Learn more about the work of our research integrity team to safeguard the quality of each article we publish.
Find out more
ORIGINAL RESEARCH article
Front. Immunol. , 04 July 2022
Sec. Autoimmune and Autoinflammatory Disorders
Volume 13 - 2022 | https://doi.org/10.3389/fimmu.2022.928623
This article is part of the Research Topic Biomarkers and Therapeutic Strategies of Systemic Lupus Erythematosus View all 11 articles
Systemic lupus erythematosus (SLE) is an autoimmune disease that affects multiple body systems with heterogeneous clinical manifestations. Since gene expression analyses have been accomplished on diverse types of samples to specify SLE-related genes, single-cohort transcriptomics have not produced reliable results. Using an integrated multi-cohort analysis framework, we analyzed whole blood cells from SLE patients from three transcriptomics cohorts (n=1222) and identified a five-gene signature that distinguished SLE patients from controls. We validated the diagnostic performance of this five-gene signature in six independent validation cohorts (n= 469), with an area under the receiver operating characteristic curve of 0.88 [95% CI 0.7 − 0.96]. This five-gene signature may be associated with the proportion of SLE immune cells, and generalizable across ages and sample types with real diagnostic value for clinical application.
Systemic lupus erythematosus (SLE) is a classic systemic autoimmune disease characterized by aberrant activity of the immune system and formation of nuclear autoantigens and immune complexes resulting in inflammation of multiple organs (1).
The incidence and prevalence of SLE varies among different ethnicities and minority groups and global prevalence is about 0-241/100000 (2). The Lupus nephritis is the most common target-organ manifestation in about 50% patients with SLE and is the most common cause of morbidity and mortality (3). Moreover, other organs involvement such as CNS involvement, cardiovascular disease, lung and skin damage contribute to mortality of SLE.
Without timely diagnosis and treatment or poor control, it will lead to irreversible damage to organs, even cause death (4). With more autoantibody tests available over the past decades, more than 180 autoantibodies can be detected in patients with SLE (5). A systematic literature search and meta-regression of diagnostic data found that the vast majority of SLE patients (97.8% [95% CI 96.8, 98.5]) have positive anti-nuclear antibodies (ANAs), suggesting ANAs are closely associated with SLE (6). Therefore, ANAs are important for diagnosis of SLE and are used as a screening parameter in routine therapy. In recent years, many novel diagnostic markers for SLE have been reported, such as serum Galectin-9, exosomal miRNAs and non-coding RNAs, further studies are needed to confirm their value in diagnosis of SLE (7, 8). There are no universally accepted diagnostic criteria for SLE because of genetic heterogeneity. However, optimized classification criteria for SLE have been widely used based on clinical manifestations and autoimmune serology.
An interplay between genetic predisposition, epigenetic and environmental factors is involved in SLE development and activity. A fraction of genetic loci with SLE susceptibility has been demonstrated (9). The diagnostic accuracy and therapy for SLE have been dramatically improved over time. However, SLE is still a disease with poor prognosis and cause serious harm to the health of patients. Therefore, diagnosing, treating, and identifying novel therapies for SLE is still challenging and more accurate diagnostic markers are needed to assess the SLE. In essence, SLE is an autoimmune disease and we speculated dysregulated gene expression from whole blood or immune cells may provide new insights in SLE diagnosis.
In the study, we analyzed whole blood or immune cells from SLE patients from transcriptomics cohorts and identified novel biomarkers for SLE diagnosis. Additional biomarkers revealed by new technology may help us improve understanding of pathogenesis of SLE.
We conducted a systematic search for expression profiling by array and whole-genome expression datasets that diagnosed SLE patients. We identified 9 datasets and divided them into 3 discovery cohorts (GSE65391, GSE49454, GSE50635) and 6 validation cohorts (GSE10325, GSE30153, GSE37356, GSE27427, GSE39088, GSE11909) from Gene Expression Omnibus (GEO) repository (Table 1).
In discovery cohorts, three microarray was performed to identify differentially expressed genes in SLE and control group (Control VS SLE). Expression profiling of whole blood samples from two validation cohorts (GSE39088, GSE11909). Additional four validation cohorts (GSE10325, GSE30153, GSE37356, GSE27427), patients samples obtained from CD4 T cells/CD19 B cells, B cells, Monocytes and Neutrophils, respectively.
Affymetrix chips were normalized using GC Robust Multi-Array Average (GCRMA) and all other chips were normalized using normal-exponential correction and quantile normalization. Log2 normalization was performed in all arrays before analysis. DerSimonian-Laird random effects were used to combine gene expression effect sizes via Hedges’g (10). For differential expression, we set significance thresholds of FDR less than 1% and an effect size greater than 0.8 fold (in non-log space). All analyses were executed with R software (version 4.1.3).
Using the MetaIntegrator R package, we ran a forward search to identify the most miserly gene signature that maximizes diagnostic performance (11). Each sample’s SLE score is derived by subtracting the mean expression of the downregulated genes from the mean expression of the upregulated genes.
Through the ImmuneDeconv package, we conducted cell-mixture deconvolution analysis (12). Briefly, each microarray dataset was converted into a gene-expression matrix. The methodology uses a list of genes, which are Characteristics for each cell type, as markers. Based on the expression values of signature genes, each cell type is quantified separately.
We conducted a systematic search for data on whole-genome expression in whole blood from patients with SLE. Our multicohort analysis found 93 significantly differentially expressed genes (FDR < 0.01, effect size > 0.8-fold) between patients who with SLE vs Control in the three discovery cohorts (N = 1222). Using forward search, we specified a signature of five significantly differentially expressed genes in SLE and Control that was optimized for diagnostic performance (Figure 1A). The forest plots showed that the 5 selected genes were differentially expressed (4 up-regulated, 1 down-regulated) according to the forward search (Figures 1B–F). In each sample, we calculated a SLE score by subtracting the mean expression of the one down-regulated gene from the mean expression of the up-regulated genes (Figure 1G and Figure S1). There was a significant difference between patients with SLE and Control in the discovery cohorts with a summary area under the curve (AUC) equal to 0.93 (95% confidence interval 0.74-0.99, Figure 1H).
Figure 1 Discovery of the five-Gene signature diagnosis of SLE. (A) Multi-cohort analysis workflow for identifying and validating the 5-gene signature. (B–F) 5-gene signature from the forward search. (G) A representative violin plot illustrating the performance of the 5-gene signature to differentiate SLE from Control in one of the discovery cohorts (GSE49454, Supplementary Figure S1). (H) ROC curves of patients with SLE versus controls in three discovery datasets.
We then validated the five-gene set in the six validation cohorts (N=469). We validated the up-regulated and down-regulated genes (Figures 2A–E). Also, we calculated the SLE score of each sample in the validation cohorts and meta score of each dataset (Figure 2F and Figure S1). Although the considerable heterogeneity between datasets, including sample type, race, platform, the SLE scores accurately identified SLE patients in all six datasets (summary AUC=0.88 [95% CI 0.7-0.96]) (Figure 2G). Notably, this signature could effectively discriminate the SLE patients for the four-validation dataset (GSE11909, GSE27427, GSE39088, GSE30153). However, the AUC of SLE scores in the GSE37356 (Monocytes) and GSE10325 (CD4 T cells, CD19 B cells) dataset were 0.67 and 0.73, respectively.
Figure 2 Validation of the 5-Gene signature diagnosis of SLE. (A–E) The up-regulated genes and down-regulated genes in independent validation cohorts. (F) A representative violin plot illustrating the performance of the five-gene signature to differentiate SLE from Control in one of the discovery cohorts (GSE39088). The Wilcoxon p value and error bars are shown. (G) ROC curves of patients with SLE versus controls in six independent validation cohorts.
We also examined whether the five-gene signature is enriched in some immune cell types by using ImmuneDeconv analysis, which is based on publicly available whole-genome expression profiles from 10 molecular immune cells. We found that the percentages of NK Cell, B cells and neutrophils were significantly changed in the SLE group. Interestingly, no statistically consistent and reproducible differences in the proportions of T cells and monocytes (Figure 3 and Figure S2).
Figure 3 A representative boxplot illustrating the analysis of the enrichment profiles of immune cells in deconvolution of cell mixtures (GSE65391). * P-value < 0.05; ** P-value < 0.01.
SLE is primarily caused by autoantibodies-induced tissue injury. An irregular immune response and inflammation are also significant pathological processes in SLE. Although numerous studies have been conducted, early diagnosis of SLE remains a challenging diagnostic problem, and many studies have suffered from a reproducibility crisis. The reproducibility crisis has been exacerbated by the fact that results from homogeneous, single-center studies do not generally generalize to heterogeneous populations in the real world. By combining data from various populations into one study, multi-cohort gene expression analysis has improved reproducibility. Here, through the use of a large, multicenter dataset of various types of samples, we identified five diagnostic biomarkers for patients with SLE (IFI27, IFI44L, ANXA3, LY96, ABCB1). Furthermore, we utilized six independent validation sets to verify the diagnostic power of the five-gene signature.
There is strong evidence from previous studies that four of the above five genes are involved in SLE pathogenesis and therapeutics. In patients with SLE, the expression of IFI27, IFI44L, and ANXA3 were up-regulated as compared to controls (13, 14). A previous study demonstrated that IFI44L promoter methylation as a blood biomarker for systemic lupus erythematosus (15). Moreover, IFI27, IFI44L, and ANXA3 are associated with highly expressed interferon-stimulated genes. Up-regulation of IFI27 (the interferon alpha-inducible protein27) expression may be associated with inflammatory response (16), and is involved in the progression of an HIV infection by regulating immune response (17). P-glycoprotein encoded by ABCB1 gene is an ATP-dependent drug efflux pump, which has been detected in some autoimmune disease, such as SLE (18). And ABCB1 gene polymorphisms may be associated with clinical features of SLE (19). Additionally, synovial tissue from SLE patients up-regulated IFI27 and IFI44L (20). Notably, we first demonstrate that LY96 genes are novel markers of SLE in this study. Molecular analysis suggests that the LY96 coding protein binds to toll-like receptor 4 on the cell surface and grants responsiveness to lipopolysaccharide (LPS), thus connecting the receptor and LPS signaling (21). The effectiveness of LY96 to another type of auto-immune disease, namely Rheumatic, has also been reported (22).
Cell-type enrichment analysis suggest that reduced abundances of NK Cell, and B cells in patients with SLE. Interestingly, there was no significant difference in monocyte proportions between SLE and the control group, and diagnostic power was also limited in the independent validation set (AUC=0.67, [95% CI 0.61-0.73]).
In summary, we identified five genes that are significantly associated with SLE diagnosis. In addition, this result was validated across nine datasets and multiple types of human blood-born samples. This suggests that these genes could serve as biomarkers for the diagnosis of SLE in real clinical application. Understanding the mechanism of SLE pathogenesis through the five-gene signature can be beneficial. In a follow-up study, we hope to gain a deeper understanding of their possible functions.
The datasets presented in this study can be found in online repositories. The names of the repository/repositories and accession number(s) can be found in the article/Supplementary Material.
The studies involving human participants were reviewed and approved by Baylor Institute for Immunology Research, UT Southwestern Medical Center, and Mayo Clinic. The patients/participants provided their written informed consent to participate in this study.
MW, QX, and SC established the concept and the investigation. MW, QD, SC, JW collected and processed data. MW, SC drafted the manuscript, and all authors reviewed the manuscript and approved the version for publication. All authors contributed to the article and approved the submitted version.
The authors declare that the research was conducted in the absence of any commercial or financial relationships that could be construed as a potential conflict of interest.
All claims expressed in this article are solely those of the authors and do not necessarily represent those of their affiliated organizations, or those of the publisher, the editors and the reviewers. Any product that may be evaluated in this article, or claim that may be made by its manufacturer, is not guaranteed or endorsed by the publisher.
The Supplementary Material for this article can be found online at: https://www.frontiersin.org/articles/10.3389/fimmu.2022.928623/full#supplementary-material
1. Durcan L, O'Dwyer T, Petri MJTL. Management Strategies and Future Directions for Systemic Lupus Erythematosus in Adults. Lancet (2019) 393:2332–43. doi: 10.1016/S0140-6736(19)30237-5
2. Rees F, Doherty M, Grainge MJ, Lanyon P, Zhang WJR. The Worldwide Incidence and Prevalence of Systemic Lupus Erythematosus: A Systematic Review of Epidemiological Studies. Rheumatology (2017) 56:1945–61. doi: 10.1093/rheumatology/kex260
3. Fava A, Petri M. Systemic Lupus Erythematosus: Diagnosis and Clinical Management. J Autoimmun (2019) 96:1–13. doi: 10.1016/j.jaut.2018.11.001
4. Liang R, Landry I, Saliaj MJC. Systemic Lupus Erythematosus vs. Mixed Connect Tissue Dis Disguised As Scabies (2022) 14(3):e23621. doi: 10.7759/cureus.23621
5. Yaniv G, Twig G, Shor DB-A, Furer A, Sherer Y, Mozes O, et al. A Volcanic Explosion of Autoantibodies in Systemic Lupus Erythematosus: A Diversity of 180 Different Antibodies Found in SLE Patients. Autoimmun Rev (2015) 14:75–9. doi: 10.1016/j.autrev.2014.10.003
6. Leuchten N, Hoyer A, Brinks R, Schoels M, Schneider M, Smolen J, et al. And Research, Performance of Antinuclear Antibodies for Classifying Systemic Lupus Erythematosus: A Systematic Literature Review and Meta-Regression of Diagnostic Data. Arthritis Care Res (2018) 70:428–38. doi: 2010.1002/acr.23292
7. Matsuoka N, Fujita Y, Temmoku J, Furuya MY, Asano T, Sato S, et al. Galectin-9 as a Biomarker for Disease Activity in Systemic Lupus Erythematosus. PloS one (2020) 15. doi: 10.1371/journal.pone.0227069
8. Li W, Liu S, Chen Y, Weng R, Zhang K, He X, et al. Circulating Exosomal microRNAs as Biomarkers of Systemic Lupus Erythematosus. Clinics (2020) 75:e1528. doi: 10.6061/clinics/2020/e1528
9. Kwon Y-C, Chun S, Kim K, Mak AJC. Update on the Genetics of Systemic Lupus Erythematosus: Genome-Wide Association Studies and Beyond. Cells (2019) 8:1180. doi: 10.3390/cells8101180
10. Sweeney TE, Haynes WA, Vallania F, Ioannidis JP, Khatri P. Methods to Increase Reproducibility in Differential Gene Expression via Meta-Analysis. Nucleic Acids Res (2017) 45:e1–1. doi: 10.1093/nar/gkw797
11. Haynes WA, Vallania F, Liu C, Bongen E, Tomczak A, Andres-Terrè M, et al. Empowering multi-Cohort Gene Expression Analysis to Increase Reproducibility. Pacific Symposium On Biocomputing 2017 World Sci (2017), 144–53. doi: 10.1142/9789813207813_0015
12. Sturm G, Finotello F, Petitprez F, Zhang JD, Baumbach J, Fridman WH, et al. Comprehensive Evaluation of Transcriptome-Based Cell-Type Quantification Methods for Immuno-Oncology. Bioinformatics (2019) 35:i436–45. doi: 10.1093/bioinformatics/btz363
13. Bing P-F, Xia W, Wang L, Zhang Y-H, Lei S-F, Deng F.-Y.J.P.O. Common Marker Genes Identified From Various Sample Types for Systemic Lupus Erythematosus. PLoS One (2016) 11 doi: 10.1371/journal.pone.0156234
14. Okada Y, Terao C, Ikari K, Kochi Y, Ohmura K, Suzuki A, et al. Meta-Analysis Identifies Nine New Loci Associated With Rheumatoid Arthritis in the Japanese Population. Meta Anal (2012) 44:511–6. doi: 10.1038/ng.2231
15. Zhao M, Zhou Y, Zhu B, Wan M, Jiang T, Tan Q, et al. IFI44L Promoter Methylation as a Blood Biomarker for Systemic Lupus Erythematosus. Ann Rheumatol Dis (2016) 75:1998–2006. doi: 10.1136/annrheumdis-2015-208410
16. Nagasawa Y, Okuzaki D, Muso E, Yamamoto R, Shinzawa M, Iwasaki Y, et al. IFI27 Is a Useful Genetic Marker for Diagnosis of Immunoglobulin A Nephropathy and Membranous Nephropathy Using Peripheral Blood. PloS One (2016) 11:e0153252. doi: 10.1371/journal.pone.0153252
17. Huang H, Lv J, Huang Y, Mo Z, Xu H, Huang Y, et al. IFI27 Is a Potential Therapeutic Target for HIV Infection. Ann Med (2022) 54:314–25. doi: 10.1080/07853890.2021.1995624
18. Tsujimura S, Saito K, Nakayamada S, Tanaka Y. Relevance of Multidrug Resistance 1 and P-Glycoprotein to Drug Resistance in Patients With Systemic Lupus Erythematosus. Histol Histopathol (2007) 22:465–8. doi: 10.14670/HH-22.465
19. Gonzalez TP, Mucenic T, Brenol JC, Xavier RM, Schiengold M, Chies JA. ABCB1 C1236T, G2677T/A and C3435T Polymorphisms in Systemic Lupus Erythematosus Patients. Braz J Med Biol Res (2008) 41:769–72. doi: 10.1590/S0100-879X2008000900005
20. Toukap AN, Galant C, Theate I, Maudoux A, Lories R, Houssiau F, et al. And Rheumatism, Identification of Distinct Gene Expression Profiles in the Synovium of Patients With Systemic Lupus Erythematosus. Arthritis Rheumatol (2007) 56:1579–88. doi: 10.1002/art.22578
21. Dou T, Fu M, Wang Y, Zhao Y, Wang Z, Bian Z, et al. Signatures of Positive Selection in LY96 Gene in Vertebrates. J Biosci (2013) 38:899–904. doi: 10.1007/s12038-013-9377-9
Keywords: SLE, transcriptome, diagnosis, immune cells, multi-cohort
Citation: Wei M, Dong Q, Chen S, Wang J, Yang H and Xiong Q (2022) Identification of Key Biomarkers in Systemic Lupus Erythematosus by a Multi-Cohort Analysis. Front. Immunol. 13:928623. doi: 10.3389/fimmu.2022.928623
Received: 26 April 2022; Accepted: 09 May 2022;
Published: 04 July 2022.
Edited by:
Xiaoyan Wang, Shanghai Jiao Tong University, ChinaCopyright © 2022 Wei, Dong, Chen, Wang, Yang and Xiong. This is an open-access article distributed under the terms of the Creative Commons Attribution License (CC BY). The use, distribution or reproduction in other forums is permitted, provided the original author(s) and the copyright owner(s) are credited and that the original publication in this journal is cited, in accordance with accepted academic practice. No use, distribution or reproduction is permitted which does not comply with these terms.
*Correspondence: Meilin Wei, d2VpbWVpbGluMTAwN0AxNjMuY29t; Qin Xiong, ZWxpbm9yXzIwQGZveG1haWwuY29t
†These authors have contributed equally to this work
Disclaimer: All claims expressed in this article are solely those of the authors and do not necessarily represent those of their affiliated organizations, or those of the publisher, the editors and the reviewers. Any product that may be evaluated in this article or claim that may be made by its manufacturer is not guaranteed or endorsed by the publisher.
Research integrity at Frontiers
Learn more about the work of our research integrity team to safeguard the quality of each article we publish.