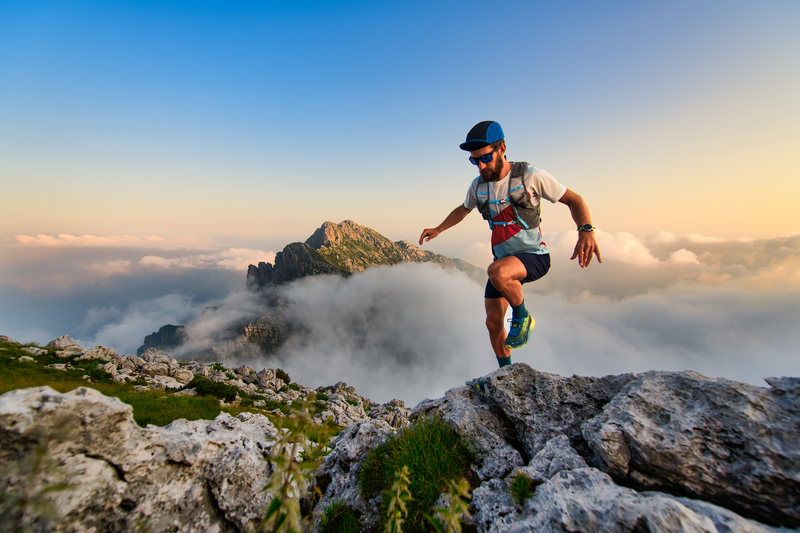
94% of researchers rate our articles as excellent or good
Learn more about the work of our research integrity team to safeguard the quality of each article we publish.
Find out more
ORIGINAL RESEARCH article
Front. Immunol. , 14 June 2022
Sec. Cancer Immunity and Immunotherapy
Volume 13 - 2022 | https://doi.org/10.3389/fimmu.2022.922315
This article is part of the Research Topic Combinational Immunotherapy of Cancer: Novel Targets, Mechanisms, and Strategies View all 85 articles
Background: Ferroptosis is a newly iron-dependent mode of programmed cell death that is involved in a variety of malignancies. But no research has shown a link between ferroptosis-related long non-coding RNAs (FRLs) and uveal melanoma (UM). We aimed to develop a predictive model for UM and explore its potential function in relation to immune cell infiltration.
Methods: Identification of FRLs was performed using the Cancer Genome Atlas (TCGA) and FerrDb databases. To develop a prognostic FRLs signature, univariate Cox regression and least absolute shrinkage and selection operator (LASSO) were used in training cohort. Kaplan-Meier (K-M) and receiver operating characteristic (ROC) curve analyses were used to assess the reliability of the risk model. The immunological functions of FRLs signature were determined using gene set enrichment analysis (GSEA). Immunological cell infiltration and immune treatment were studied using the ESTIMATE, CIBERSORT, and ssGSEA algorithms. Finally, in vitro assays were carried out to confirm the biological roles of FRLs with known primer sequences (LINC00963, PPP1R14B.AS1, and ZNF667.AS1).
Results: A five-genes novel FRLs signature was identified. The mean risk score generated by this signature was used to create two risk groups. The high-risk score UM patients had a lower overall survival rate. The area under the curve (AUC) of ROC and K-M analysis further validated the strong prediction capacity of the prognostic signature. Immune cells such as memory CD8 T cells, M1 macrophages, monocytes, and B cells showed a substantial difference between the two groups. GSEA enrichment results showed that the FRLs signature was linked to certain immune pathways. Moreover, UM patients with high-risk scores were highly susceptible to several chemotherapy drugs, such as cisplatin, imatinib, bortezomib, and pazopanib. Finally, the experimental validation confirmed that knockdown of three identified lncRNA (LINC00963, PPP1R14B.AS1, and ZNF667.AS1) suppressed the invasive ability of tumor cells in vitro.
Conclusion: The five-FRLs (AC104129.1, AC136475.3, LINC00963, PPP1R14B.AS1, and ZNF667.AS1) signature has effects on clinical survival prediction and selection of immunotherapies for UM patients.
Uveal melanoma (UM) is a rare subtype of melanoma that differs significantly from cutaneous and other types of melanoma in terms of biological and clinical characteristics, with extremely high mortality rates (1, 2). The mean incidence of UM in the USA was five per million (3, 4). Approximately half of UM patients will develop hepatic metastasis (5–8). Although various therapies, such as radiotherapy, local resection, immunotherapy, chemotherapy, and phototherapy, increase the possibility of preserving useful vision, the unsatisfied prognosis of UM has not improved appreciably (9, 10). In addition, recent investigations show that prospective diagnostic tools for UM are rare and limited, indicating the challenge of early diagnosis of UM (11). Therefore, novel predictive models and useful biomarkers for UM patients need to be found and used in clinical practice as soon as possible.
Dysfunction of anti-tumor immunity in tumor microenvironment is a hallmark of melanoma and re-balance of immunosuppressive microenvironment is crucial for melanoma treatment (12). Recently, several immune check-point blockage immunotherapies have been approved for the treatment of melanoma, which contributed to survival of UM patients and provided effective disease control in a fraction of patients (13). Recently clinical practices reported that programmed cell death 1(PD-1) inhibitors only achieved 3.6-4.7% of response rate in patients with UM, and blood biomarkers represented a hopeful mean to evaluate the efficacy of immunotherapy in UM (14, 15). These studies recapped the different characteristics of tumor immune microenvironment of UM and highlighted the importance of developing novel prognostic prediction tools for UM immunotherapy.
Recent years, ferroptosis, as a new category of regulated cell death, is triggered by intracellular iron and it participates in a variety of physiological activities, such as lipid peroxidation and iron metabolism (16–18). The abnormalities of ferroptosis have been associated with the development of hepatocellular, cervical, breast, lung, ovarian, prostate, colorectal, renal carcinomas, and melanoma (17, 19–22). The tumor‐suppressive effect of ferroptosis in carcinomatosis is crucial, including UM (23). Oleic acid prevented melanoma cells from entering ferroptosis, and enhanced lymphatic exposure protected melanoma cells against ferroptosis and boosted their ability to survive (24). Moreover, drugs-induced ferroptosis could potentially enhance anti-tumor immunity response by inhibiting the dedifferentiation of melanoma cells (25, 26). So, evaluation of ferroptosis-related genes (FRGs) or FRLs may provide new clues for diagnoses and prognosis prediction of UM.
Long noncoding RNAs (lncRNAs), as a form of non-coding RNA, play a pivotal role in cellular processes, such as metabolism, neurodegenerative dysfunction, cell cycle regulation, and neoplasia (27–29). LncRNAs are involved in essential carcinogenesis pathways in melanoma, such as the PI3K/Akt, NF-kappa B, and MAPK/ERK (30). Currently, fast-growing studies have explored the ferroptosis-related roles of lncRNAs in tumorigenesis (31). For examples, miR-9 promotes ferroptosis through targeting GOT1 in melanoma cells (32). The miR-137 inhibits necroptosis, and the knockdown of miR-137 boosted anticancer efficacy of erastin by enhancing ferroptosis both in vitro and in vivo(33). As a result, lncRNA might be examined as a possible target for novel RNA-based anti-UM treatments (34, 35).
Based on the above facts, we hypothesized that FRLs might benefit in the identification of high-risk UM patients and the development of customized treatment plans for them. Herein, we constructed a FRLs signature and confirmed its prognostic value for UM patients. Then, we evaluated the relationship between the immune infiltration landscape and FRLs signature. Finally, we conducted cell experiments to further verify the biological functions of three identified FRLs with known primer sequences.
The RNA sequencing data in FPKM format and clinicopathological characteristics of UM patients (n=80) were downloaded from TCGA (https://portal.gdc.cancer.gov/). Our research only included UM patients with known survival time and status. Additionally, patients with overall survival of fewer than 30 days in TCGA-UM were excluded to ensure the reliability of the study. The ‘caret’ R package randomly allocated all the selected patients (n=74) into the training and validation cohorts in a 1:1 ratio (36). The ensemble expression matrix was converted into a gene symbol expression matrix using Perl language. Log2 conversion of the data was performed.
In total, 382 FRGs were obtained from the FerrDb website (http://www.zhounan.org/ferrdb/index.html) database (37). Then, we determined the association between FRGs and lncRNAs by the ‘limma’ R package with Spearman correlation coefficient > 0.6 and p < 0.001 as the threshold (38).
Prognostic FRLs were screened using a univariate Cox regression analysis. Those FRLs with p-value < 0.01 were chosen for an overall survival-based LASSO regression analysis to reduce overfit and the number of FRLs by the ‘glmnet’ R package. The risk formula were calculated based on the FRLs expression levels and relevant regression coefficient as follows:
The predictive efficacy of the FRLs signature was assessed using K-M and ROC curve analyses by the ‘survival’ and ‘survminer’ R packages. The principal component analysis (PCA) and t-distributed stochastic neighbor embedding (t-SNE) analysis further examined the clustering ability of risk score. We also compared the prediction ability of the FRLs signature with additional clinical characteristics, such as gender, age, and tumor classification.
The lncRNA-mRNA coexpression network was created to show the relationship between the FRLs and their related mRNAs using the ‘ggalluvial’ R package and Cytoscape software (version 3.9.0) (39).
Background biological enrichment analyses, such as the Kyoto Encyclopedia of Genes and Genomes (KEGG) pathways and GO biology functions, were examined using GSEA (Version 4.1.0) by ‘ggplot2’ R package. Gene sets items with normal p < 0.05 with FDR q < 0.25 were considered significant.
The difference in immune cell infiltration, the stromal, tumor purity, immune, and ESTIMATE score between the two groups was evaluated using the CIBERSORT and ESTIMATE algorithm (40). The immune function and cell subgroups were investigated using the ssGSEA. In addition, the immune checkpoint and m6A-related genes collected from prior publications were also investigated using ‘limma’ and ‘ggplot2’ R package. The IC50 of frequently used chemotherapeutic medicines was calculated by using R package ‘pRRophetic.’ (41). Tumor Mutation Burden (TMB) was also analyzed using the ‘maftools’ R package.
Human highly invasive UM cell line (C918) was purchased from Procell Life Science &Technology, Wuhan, China. C918 cells were cultivated in Roswell Park Memorial Institute medium. Small interfering and negative control RNA were used in knockdown experiments. After 48 hours transfection, cells were collected for RNA extraction or other functional assays. The sequences of siRNA utilized are listed in Supplementary Table 1.
TRIzol (Invitrogen, Carlsbad, CA) was used to extract total RNA from cell samples. The reverse transcription of the RNA into complementary DNA was subsequently performed using a GoScript reverse transcription system (Promega). Quantitative Real Time‐PCR (qRT‐PCR) validated the transfection efficiency. The list of the primer sequences utilized is shown in Supplementary Table 2. The following were the qRT-PCR conditions: initial denaturation takes 5 minutes at 95°C, followed by 40 cycles of denaturation (15 sec at 95°C), annealing (30 sec 59°C), elongation (30 sec at 72°C), and ultimate extension (5 min at 72°C).
Transwell assays were used to assess C918 cell invasion ability. 1.5×104 cells were placed in the top chamber. After a 24-hour incubation period, C918 cells shifted through the membrane were kept for 15 min in methanol and dyed with 10% crystal violet. For invasion assays, Matrigel (Basement Membrane Matrix) was placed into the top chambers 24 h before the trials. The number of C918 cells was counted in ten randomly chosen fields.
The capacity of C918 cells to proliferate was assessed using the Cell Counting Kit-8 (CCK-8) assay (MCE). After 48 h transfection, C918 cells were seeded at a density of 3500 cells per well in 96-well plates in six repetitions. After 12 h, 24 h, 36 h, and 48 h, the C918 cells were incubated with 10% CCK-8 solution for 1 h at 37°C. A 450 nm wavelength was used to measure the absorbance of living cells.
C918 cells were collected and implanted into a six-well plate, where they were grown until 80% fusion. Then the C918 cells were starved in a serum-free medium for 24 hours. The plate was scratched with a one-line design using a 1000μl pipette tip. Scratch healing was examined under the microscope and photographed after a 24-hour culture period.
The R language software was used to conduct all statistical computations in this research. The Wilcoxon method was used for the two-sample tests. The Kruskal-Wallis test was utilized to assess differences in data among the multiple groups.
The FRLs signature was constructed following the flowchart shown in Figure 1. We identified 14,057 lncRNAs and 240 FRGs from the TCGA-UM data. 535 FRLs were identified with the threshold of Pearson correlation coefficients > 0.6 and p < 0.001 (Supplementary Table 3).
49 lncRNAs were uncovered after univariate Cox analysis with p < 0.01 filtering (Figure 2), five of which were determined using LASSO regression and finally included in the prognostic model (Figures 3A, B). The patients were divided into two groups (high-risk and low-risk) according to the median risk score, and the risk score was as follows: risk score = (4.9674 × AC136475.3) + (0.2274 × AC104129.1) + (1.4277 × PPP1R14B.AS1) + (-0.3151 × LINC00963) + (-0.6063 × ZNF667.AS1) (Figure 3C).
Figure 2 Prognosis-related FRLs from the training set screened using univariate Cox regression analysis.
Figure 3 Development of FRLs signature. (A, B) LASSO regression model of the prognostic FRLs. (C) Forest plot of five FRLs revealed by multivariate Cox regression. **p < 0.01.
The predictive value of the five-FRLs signature was investigated. The K-M curves showed that patients with a high risk score had a considerably increased risk of mortality (Figures 4A–C). The areas under the ROC (AUC) values in the training and testing cohorts were 0.904 and 0.740 at 1 year, which showed high accuracy (Figures 4D–F). In addition, the survival status, survival times, and expression patterns of patients with UM are shown in Figures 5A–C. The ROC findings also revealed that the risk signature was a more relatively precise prognostic indicator than other clinical alternative prediction models for UM (Figures 5D–F). Patients with the elevated expression of the discovered FRLs, such as AC104129.1, AC136475.3, LINC00963, and PPP1R14B.AS1, showed a shorter overall life expectancy (p < 0.01) (Figures 6A–C). The lncRNA distribution of the two groups was shown in the PCA and t-SNE analyses (Figures 6D-F), and we obviously judged that the selected UM patients might be better discriminated between the two groups. Furthermore, univariate and multivariate Cox regression analysis showed that only the five FRLs signature could serve as an independent prognostic factor for UM patients (Figures 7A–C). Additionally, as the stages and T classification progressed, the risk score increased (p < 0.05) (Figures 7D–G). This finding implies that the FRL signature is strongly involved in the development and prognosis of UM. Sankey diagram depicted the link between FRLs and FRGs, as well as their risk types in UM (Figure 8A). The blue nodes represent genes, whereas the pink nodes indicate FRLs co-expressed with those genes (Figure 8B).
Figure 4 The K-M survival curve and ROC curve of risk score based on five FRLs signature. (A–C) Differences in the overall survival of UM patients between the high- and low-risk groups in the (A) training, (B) test, and (C) all cohorts. (D–F) ROC curves of risk scores at 1, 2, and 3 years in the (D) training, (E) test, and (F) all sets.
Figure 5 Evaluation of the FRLs signature predictive ability. (A–C) The distribution plots, survival status, and expression trends of the different risk score in the (A) training, (B) test, and (C) all cohorts. (D-F) ROC curves of risk score, age, gender, stage, and T classification in the (D) training, (E) testing, and (F) all sets.
Figure 6 Kaplan–Meier survival analysis of five hub FRLs and discrimination analysis of the risk score. (A–C) The K-M survival curve analysis of the five optimal FRLs signature (AC104129.1 AC136475.3, LINC00963, and PPP1R14B.AS1, and ZNF667.AS1) in the (A) training, (B) test, and (C) all cohorts. (D–F) PCA and t-SNE diagrams of genome-wide expression profiles of TCGA-UM in the (D) training, (E) validation, and (F) all groups, respectively.
Figure 7 The independent prognostic value. (A–C) The forest plots for univariate and multivariate Cox regression analysis in (A) training, (B) validation, and (C) total groups. (D–G) Different levels of risk scores in UM patients stratified by (D) age, (E) gender, (F) stage, and (G) T classification.
Figure 8 Diagram of lncRNA-mRNA coexpression network and functional analysis. (A, B) Alluvial diagram of the relationship between lncRNA and co-expression mRNA with different risk-subgroups. (C) GO function annotation of different risk groups. (D) The significantly enriched KEGG pathways by GSEA using immune gene set.
Immune-related GO terms and KEGG pathways were analyzed by GSEA. The high-risk group had an accumulation of immune-related GO items (Figure 8C), including macrophage activation involved in immune response, immunological memory process, lipopolysaccharide mediated signaling pathway, mature B cell differentiation, and innate immune response. In addition, the KEGG pathways proteasome, cytosolic DNA sensing, Toll-like receptor (TLR), leukocyte transendothelial migration, and Leishmania infection were also enriched in the high-risk group (Figure 8D). The details are presented in Supplementary Table 4. These results suggested that the newly identified FRLs signature was strongly associated with tumor immune function.
We used the CIBERSORT algorithm to examine the immune cell infiltration landscape in UM patients to comprehend more about the association between the FRLs signature and antitumor immune regulation. A heatmap was used to demonstrate the changes in immune cell infiltration between the two groups (Figure 9A). Figure 9B shows the percentage of each typical immune cell. We found that CD8 T cells, M1 macrophages, memory B cells, CD4 memory T cells, Monocytes, and resting mast cells were significantly different between the two groups. The different immune cell correlation was displayed in Figure 9C. Figures 9D–G showed that the immune, ESTIMATE, and stromal score were all considerably higher in the high-risk group. Almost all scores of immune cell proportion and immune-related functions differed substantially between the two groups (Figures 10A, B). As shown in Figure 10C, some validated effective checkpoint immunotherapy targets were overexpressed in the high-risk group, such as PDCD1 (PD-1) and CTLA4. Furthermore, the expression of m6A-related genes YTHDF1 and ALKBH5 was obviously higher in the high-risk group (Figure 10D). These revealed that the immune responses of the two groups differed, which might be applied to anti-tumor immunotherapy in UM.
Figure 9 (A) Heatmap of immune cell expression using a variety of algorithms. (B) The proportion of each typical immune cell between the two groups. (C) The correlation of immune cells shown in a heatmap. (D–G) Comparison of the (D) immune, (E) ESTIMATE, (F) tumor purity, and (G) stromal score. *p < 0.05, **p < 0.01, and ***p < 0.001.
Figure 10 (A, B) The score of (A) immune cell infiltration proportions and (B) immune function by ssGSEA. (C, D) The differential gene expression of (C) checkpoints and (D) m6A between two groups. *p < 0.05, **p < 0.01, and ***p < 0.001. ns, no significance.
We evaluated the estimated IC50 levels of some chemotherapy medicines between the two groups, and ten typical drugs are shown in Figure 11A. The results showed that cisplatin, imatinib, nilotinib, rapamycin, bortezomib, and pazopanib may be potential medications for treating UM patients in the high-risk group.
Figure 11 Differences in sensitivity of chemotherapeutic drugs and TMB. (A) The IC50 levels of two prognostic risk groups for ten common chemotherapy drugs. (B, C) Top 20 gene mutations in (B) low- and (C) high-risk groups. (D) Violin plot of TMB in UM patients between two risk groups. (E) The differences in high- and low-TMB levels between high- and low-risk groups in UM patients. (F) Kaplan–Meier plot of overall survival for UM patients.
Although there was no statistically significant difference in TMB levels between the two groups (Figures 11B–D), we found that higher TMB had a tendency for lower overall survival rate (Figures 11E–F).
The roles of the five identified FRLs have not been reported in UM. In addition, the detailed primer sequences of AC104129.1 and AC136475.3 are unavailable in gene banks. Thus, to further explore the potential cell function of the other three lncRNAs (LINC00963, PPP1R14B.AS1, and ZNF667.AS1), we used the C918 cell line to construct the lncRNAs knockdown phenotypes. The transfection efficiency was confirmed by qRT–PCR (Figure 12A), and both siRNA fragments dramatically reduced the expression of LINC00963, PPP1R14B.AS1, and ZNF667.AS1. Then, we performed a series of assays to study cell function change. CCK-8 assay results suggested that the down-regulation of PPP1R14B.AS1 inhibited the proliferation ability of C918, while the underexpression of ZNF667.AS1 enhanced the proliferation of C918 cells. The knockdown of LINC00963 in the C918 cells could not change the proliferation capacity (Figure 12B). Scratch test suggested that after culture for 24 h, scratches of the knock-down group healed slowly and the area of cell migration decreased, indicating that inhibition of LINC00963, PPP1R14B.AS1, and ZNF667.AS1 expression could reduce the migration ability of C918 cells (Figure 12C). Furthermore, knockdown of LINC00963, PPP1R14B.AS1, and ZNF667.AS1 attenuated the invasion ability of C918 cells via transwell assay (Figure 12D). These findings suggest that LINC00963, PPP1R14B.AS1, and ZNF667.AS1 serve as high-risk predictors in C918 cells, and their high expression promotes cancer growth in some way.
Figure 12 Characteristics of FRLs signature and its functional effect on C918 cells. (A) The transfection efficiency verified by qRT-PCR. (B) The viability of C918 detected through CCK-8 assay after knockdown of three identified FRLs. (C) Cell migration observed under the microscope. (D) The differences in the invasive ability of C918 cells. *p < 0.05, **p < 0.01, and ***p < 0.001. “ns” means no significance.
Patients with advanced stage UM have a death rate of more than 95% within 5 years (42), so discovering new and effective prognostic biomarkers is crucial for UM. Several studies have recently begun to mine the prognostic lncRNA value in tumors from public databases. For examples, a FRLs signature could be utilized to accurately predict the prognosis of glioma (43). BASP1-AS1 might be used as a biomarker to detect cutaneous malignant melanoma (44). However, to our knowledge, there is a scarcity of studies focusing on FRLs in UM. Many cellular processes, including ferroptosis, are regulated by lncRNAs in experimental studies. Several previously identified lncRNAs are related to shape, TNM stages, diagnosis, and progression of melanoma (45). Therefore, developing a FRLs signature may be useful in predicting UM prognosis and optimizing therapeutic methods.
In this study, we first obtained 240 FRGs and 535 FRLs in TCGA-UM data. Patients with UM were allocated to the training and test groups in a 1:1 ratio. We first identified 49 prognostic FRLs using univariate Cox regression analysis. Then We obtained five FRLs signature using LASSO Cox regression. The following K-M survival analysis showed that UM patients with the high-risk score had a terrible prognosis, but those in the low-risk group had a better life expectancy, demonstrating strong prognostic potential of the newly discovered signature. Additionally, AUC analysis suggested stable performance in different risk-level UM patients. As a result, once the expression levels of the five FRLs are identified, we can forecast the probability of mortality in patients with UM. We also found that risk score was significantly higher in advanced stage (IV) and T classification (IV), indicating that the FRLs signature has a significantly discriminable ability for UM patients. Then, we conducted a series of cell tests to verify the function of these discovered FRLs. These findings suggested that the FRL signature might be exploited as a viable UM prognostic biomarker.
Our FRLs signature included AC104129.1, AC136475.3, LINC00963, PPP1R14B.AS1, and ZNF667.AS1. The roles of the five FRLs have not been reported in UM. This research was the first to utilize the lncRNA AC104129.1 as a biomarker for cancer. Nevertheless, further research on AC104129.1 is needed to understand the deeper mechanisms in the future. The other four lncRNAs have been reported as biomarkers for different carcinoma. For instance, AC136475.3 could be considered as a prognostic factor hepatocellular carcinoma (46). LINC00963 could serve as an oncogene by regulating biological processes, including survival, metastasis, and differentiation (47), was up-regulated in prostate cancer (48), hepatocellular carcinoma (49), osteosarcoma (50), and cutaneous squamous cell carcinoma (51, 52). PPP1R14B-AS1 was identified to be highly expressed in 12 malignancies in the TCGA database. The inhibition of PPP1R14B-AS1 can repress growth and migration in human hepatocellular carcinoma (53). ZNF667-AS1, as a tumor suppressor, could inhibit the viability, migration, and invasion of esophageal squamous cell carcinoma (54). In this study, the CCK-8 experiment revealed that the down-regulation of PPP1R14B.AS1 decreased C918 proliferation. In contrast, C918 cells proliferated more when ZNF667.AS1 was underexpressed. In addition, the knockdown of LINC00963 had no obvious effect on the proliferation of C918 cells. For the scratch migration and transwell assay, the knockdown of the three identified FRLs (LINC00963, PPP1R14B.AS1, and ZNF667.AS1) could suppress migration and invasion in the C918 cell line, similar to the above-mentioned findings.
Additionally, we investigated the correlation between the immune infiltration and the five-FRLs signature. We found that memory B cells, M1 macrophages, activated CD4 memory T cells, Monocytes, CD8 T cells, and resting mast cells were significantly different between the two groups. CD8 T cells have the capacity to promote ferroptosis in vivo (55, 56). In a recent case report, the accumulation of CD8 T cells was detected in hepatic metastases lesions in a patient with UM (57). In our study, the percentage of CD8 T cells was greater in patients with a high risk score. Recent findings show that interferon-gamma produced by CD8 T cells has a tumor-suppressing impact when ferroptosis is activated, suggesting that the immune system may help to prevent carcinogenesis through ferroptosis (55). This could provide fresh insights into the association between ferroptosis and tumor immune microenvironment.
Immune checkpoint inhibitors (including PD-1, CTLA-4, PD-L1, and TIM-3) reduced the activation of immune cell, leading to immunosuppression of the tumor immune microenvironment (58, 59). Anti-PD-1 drugs, such as pembrolizumab, nivolumab, or atezolizumab, were already used to treat individuals with metastatic UM, displaying limited response rates and therapeutic effects in UM patients (14, 60, 61). Anti CTLA-4 drugs, such as tremelimumab and ipilimumab, were also used in patients with metastatic UM. A previous meta-analysis showed that immune checkpoint blockade immunotherapy was helpful for the treatment of UM patients in terms of long-term survival (62). In this study, the PD-1 expression was increased in the high-risk group. CD8 T cells could regulate endocytic recycling of PD-1 and exert synergistic effects with anti-PD-1 therapy in hepatocellular carcinoma (63), and inducing immunogenic ferroptosis in cancer cells also potentiates anti-PD-1 therapy (64), highlighting a promising strategy for cancer immunotherapy.
GSEA results showed that immune system hallmarks, such as immunological memory process, activation of innate immune response, mature B cell differentiation, lipopolysaccharide mediated pathway, and TLR, were considerably enriched in the high-risk score group. TLR is an essential protein molecule in innate immunity and a major regulator of ferroptosis (65–67), and the anti-tumor effectiveness of immunotherapy was aided by enhanced ferroptosis (55). TLR stimulation activated immunoinhibitory signaling pathways such as PD-1 expression (68), which indicates that new targets may be developed to improve therapeutic efficacy for UM.
Predicting the drug sensitivity facilitated in avoiding ineffective use of drugs, revealed new applications for existing drugs, and increased the success rate of therapy (69). Chemotherapy may cause clinical benefits in UM, particularly in patients without bulky liver metastases. The combination of nivolumab and ipilimumab was found to be highly beneficial in metastatic UM (70). Chemotherapy regimens adapted from cutaneous melanoma, such as cisplatin, dacarbazine, and temozolomide, have been utilized in UM patients, and response rates vary between 0% and 15% (71, 72). A triple-drug treatment regimen consisting of cisplatin, vinblastine, and dacarbazine also improved UM patient survival (73). Recent studies have shown that the inducing ferroptosis by blocking STAT3/Nrf2/GPx4 signaling makes osteosarcoma cells more sensitive to cisplatin (74). Our research demonstrated that patients in high-risk group were more susceptible to cisplatin, mitomycin C, gefitinib, bortezomib, pazopanib, lapatinib, rapamycin, and temsirolimus. This may provide novel therapeutic strategies targeting ferroptosis in UM patients.
Admittedly, this study has some limitations. Firstly, the research only covered a limited number of patients owing to the low prevalence of UM, and some deviations might occur. Secondly, the stages N and M of UM patients were excluded because of the unavailable data. Therefore, it is unclear whether they are predictive factors. Lastly, the exact process through which these lncRNAs influence ferroptosis is uncertain. The association between these lncRNAs and FRGs has to be investigated further.
In summary, for the first time, we report and confirm a five-FRLs signature that could be used as a potential biomarker and treatment option for UM. Our results may contribute to predicting the prognosis and developing effective chemotherapy and immunotherapy for UM patients.
Publicly available datasets were analyzed in this study. This data can be found in TCGA (https://portal.gdc.cancer.gov/projects/TCGA-UVM). Further inquiries can be directed to the corresponding authors.
XM, JZ, and QL conceived and designed this study. XM, SY, WB, BZ, YC, JZ, QL, and JN downloaded and analyzed the raw data, performed bioinformatics, and wrote the manuscript. BZ, XM, SY, WB, YC, and JN performed the cell experiments. JZ and QL supervised the research and critically reviewed the paper. All of the writers contributed to the essay and gave their approval to the final version. All authors contributed to the article and approved the submitted version.
This study received funding from the Science, Technology and Innovation Commission of Shenzhen Municipality under grant (No. JCYJ20210324113610029 and No. JCYJ20180228164400218).
The authors’ claims in this article are solely their own, and do not necessarily represent the opinions of their affiliated organizations, the publisher, editors, or reviewers.
The authors declare that the research was conducted in the absence of any commercial or financial relationships that could be construed as a potential conflict of interest.
All claims expressed in this article are solely those of the authors and do not necessarily represent those of their affiliated organizations, or those of the publisher, the editors and the reviewers. Any product that may be evaluated in this article, or claim that may be made by its manufacturer, is not guaranteed or endorsed by the publisher.
We are sincerely acknowledging the contributions from the TCGA.
The Supplementary Material for this article can be found online at: https://www.frontiersin.org/articles/10.3389/fimmu.2022.922315/full#supplementary-material
1. Rodrigues M, de Koning L, Coupland SE, Jochemsen AG, Marais R, Stern M-H, et al. So Close, Yet So Far: Discrepancies Between Uveal and Other Melanomas. A Position Paper From Um Cure 2020. Cancers (2019) 11(7):1032. doi: 10.3390/cancers11071032
2. Talty R, Rosenberg M. The Role of Ferroptosis in Melanoma. Pigm Cell Melanom Res (2022) 35(1):18–25. doi: 10.1111/pcmr.13009
3. Singh AD, Turell ME, Topham AK. Uveal Melanoma: Trends in Incidence, Treatment, and Survival. Ophthalmology (2011) 118(9):1881–5. doi: 10.1016/j.ophtha.2011.01.040
4. Ghazawi FM, Darwich R, Le M, Rahme E, Zubarev A, Moreau L, et al. Uveal Melanoma Incidence Trends in Canada: A National Comprehensive Population-Based Study. Br J Ophthalmol (2019) 103(12):1872–6. doi: 10.1136/bjophthalmol-2018-312966
5. Amaro A, Gangemi R, Piaggio F, Angelini G, Barisione G, Ferrini S, et al. The Biology of Uveal Melanoma. Cancer Metast. Rev (2017) 36(1):109–40. doi: 10.1007/s10555-017-9663-3
6. Lorenzo D, Maria Piulats J, Ochoa M, Arias L, Gutierrez C, Catala J, et al. Clinical Predictors of Survival in Metastatic Uveal Melanoma. Japan J Ophthalmol (2019) 63(2):197–209. doi: 10.1007/s10384-019-00656-9
7. Wilson MA, Zhong J, Rosenbaum BE, Utter K, Moran U, Darvishian F, et al. Impact of Initial Stage on Metastatic Melanoma Survival. Melanom. Res (2019) 29(3):281–8. doi: 10.1097/cmr.0000000000000526
8. Branisteanu DC, Bogdanici CM, Branisteanu DE, Maranduca MA, Zemba M, Balta F, et al. Uveal Melanoma Diagnosis and Current Treatment Options (Review). Exp Ther Med (2021) 22(6):1428. doi: 10.3892/etm.2021.10863
9. Damato B. Ocular Treatment of Choroidal Melanoma in Relation to the Prevention of Metastatic Death - a Personal View. Prog Retina Eye Res (2018) 66:187–99. doi: 10.1016/j.preteyeres.2018.03.004
10. Wu M-Y, Lai T-T, Liao W-T, Li C-J. Clinicopathological and Prognostic Significance and Molecular Mechanisms Governing Uveal Melanoma. Ther Adv Med Oncol (2020) 12:1–12. doi: 10.1177/1758835920917566
11. Bol KF, Donia M, Heegaard S, Kiilgaard JF, Svane IM. Genetic Biomarkers in Melanoma of the Ocular Region: What the Medical Oncologist Should Know. Int J Mol Sci (2020) 21(15):5231. doi: 10.3390/ijms21155231
12. Iacono D, Vitale MG, Basile D, Pelizzari G, Cinausero M, Poletto E, et al. Immunotherapy for Older Patients With Melanoma: From Darkness to Light? Pigm. Cell Melanom. Res (2021) 34(3):550–63. doi: 10.1111/pcmr.12917
13. Petrova V, Arkhypov I, Weber R, Groth C, Altevogt P, Utikal J, et al. Modern Aspects of Immunotherapy With Checkpoint Inhibitors in Melanoma. Int J Mol Sci (2020) 21(7):2367. doi: 10.3390/ijms21072367
14. Algazi AP, Tsai KK, Shoushtari AN, Munhoz RR, Eroglu Z, Piulats JM, et al. Clinical Outcomes in Metastatic Uveal Melanoma Treated With Pd-1 and Pd-L1 Antibodies. Cancer (2016) 122(21):3344–53. doi: 10.1002/cncr.30258
15. Heppt MV, Heinzerling L, Kaehler KC, Forschner A, Kirchberger MC, Loquai C, et al. Prognostic Factors and Outcomes in Metastatic Uveal Melanoma Treated With Programmed Cell Death-1 or Combined Pd-1/Cytotoxic T-Lymphocyte Antigen-4 Inhibition. Eur J Cancer (2017) 82:56–65. doi: 10.1016/j.ejca.2017.05.038
16. Dixon SJ, Lemberg KM, Lamprecht MR, Skouta R, Zaitsev EM, Gleason CE, et al. Ferroptosis: An Iron-Dependent Form of Nonapoptotic Cell Death. Cell (2012) 149(5):1060–72. doi: 10.1016/j.cell.2012.03.042
17. Stockwell BR, Angeli JPF, Bayir H, Bush AI, Conrad M, Dixon SJ, et al. Ferroptosis: A Regulated Cell Death Nexus Linking Metabolism, Redox Biology, and Disease. Cell (2017) 171(2):273–85. doi: 10.1016/j.cell.2017.09.021
18. Yan H-f, Zou T, Tuo Q-z, Xu S, Li H, Belaidi AA, et al. Ferroptosis: Mechanisms and Links With Diseases. Signal Transduct Target Ther (2021) 6(1):49. doi: 10.1038/s41392-020-00428-9
19. Tang R, Hua J, Xu J, Liang C, Meng Q, Liu J, et al. The Role of Ferroptosis Regulators in the Prognosis, Immune Activity and Gemcitabine Resistance of Pancreatic Cancer. Ann Trans Med (2020) 8(21):1347. doi: 10.21037/atm-20-2554a
20. Wang H, Cheng Y, Mao C, Liu S, Xiao D, Huang J, et al. Emerging Mechanisms and Targeted Therapy of Ferroptosis in Cancer. Mol Ther (2021) 29(7):2185–208. doi: 10.1016/j.ymthe.2021.03.022
21. Mou Y, Wang J, Wu J, He D, Zhang C, Duan C, et al. Ferroptosis, a New Form of Cell Death: Opportunities and Challenges in Cancer. J Hematol Oncol (2019) 12:34. doi: 10.1186/s13045-019-0720-y
22. Basit F, van Oppen LMPE, Schoeckel L, Bossenbroek HM, van Emst-de Vries SE, Hermeling JCW, et al. Mitochondrial Complex I Inhibition Triggers a Mitophagy-Dependent Ros Increase Leading to Necroptosis and Ferroptosis in Melanoma Cells. Cell Death Dis (2017) 8(3):e2716. doi: 10.1038/cddis.2017.133
23. Ashrafizadeh M, Mohammadinejad R, Tavakol S, Ahmadi Z, Roomiani S, Katebi M. Autophagy, Anoikis, Ferroptosis, Necroptosis, and Endoplasmic Reticulum Stress: Potential Applications in Melanoma Therapy. J Cell Physiol (2019) 234(11):19471–9. doi: 10.1002/jcp.28740
24. Ubellacker JM, Tasdogan A, Ramesh V, Shen B, Mitchell EC, Martin-Sandoval MS, et al. Lymph Protects Metastasizing Melanoma Cells From Ferroptosis. Nature (2020) 585(7823):113–+. doi: 10.1038/s41586-020-2623-z
25. Tsoi J, Robert L, Paraiso K, Galvan C, Sheu KM, Lay J, et al. Multi-Stage Differentiation Defines Melanoma Subtypes With Differential Vulnerability to Drug-Induced Iron-Dependent Oxidative Stress. Cancer Cell (2018) 33(5):890–+. doi: 10.1016/j.ccell.2018.03.017
26. Gagliardi M, Saverio V, Monzani R, Ferrari E, Piacentini M, Corazzari M. Ferroptosis: A New Unexpected Chance to Treat Metastatic Melanoma? Cell Cycle (2020) 19(19):2411–25. doi: 10.1080/15384101.2020.1806426
27. Anastasiadou E, Jacob LS, Slack FJ. Non-Coding Rna Networks in Cancer. Nat Rev Cancer (2018) 18(1):5–18. doi: 10.1038/nrc.2017.99
28. Durante G, Comito F, Lambertini M, Broseghini E, Dika E, Ferracin M. Non-Coding Rna Dysregulation in Skin Cancers. Non-Cod Genome (2021) 65(4):641–55. doi: 10.1042/ebc20200048
29. Bande M, Fernandez-Diaz D, Fernandez-Marta B, Rodriguez-Vidal C, Lago-Baameiro N, Silva-Rodriguez P, et al. The Role of Non-Coding Rnas in Uveal Melanoma. Cancers (2020) 12(10):2944. doi: 10.3390/cancers12102944
30. Safa A, Gholipour M, Dinger ME, Taheri M, Ghafouri-Fard S. The Critical Roles of Lncrnas in the Pathogenesis of Melanoma. Exp Mol Pathol (2020) 117:104558. doi: 10.1016/j.yexmp.2020.104558
31. Zhi Y, Gao L, Wang B, Ren W, Liang KX, Zhi K. Ferroptosis Holds Novel Promise in Treatment of Cancer Mediated by Non-Coding Rnas. Front Cell Dev Biol (2021) 9:686906. doi: 10.3389/fcell.2021.686906
32. Zhang K, Wu L, Zhang P, Luo M, Du J, Gao T, et al. Mir-9 Regulates Ferroptosis by Targeting Glutamic-Oxaloacetic Transaminase Got1 in Melanoma. Mol Carcinogen. (2018) 57(11):1566–76. doi: 10.1002/mc.22878
33. Luo M, Wu L, Zhang K, Wang H, Zhang T, Gutierrez L, et al. Mir-137 Regulates Ferroptosis by Targeting Glutamine Transporter Slc1a5 in Melanoma. Cell Death Differ (2018) 25(8):1457–72. doi: 10.1038/s41418-017-0053-8
34. Barbagallo C, Caltabiano R, Broggi G, Russo A, Puzzo L, Avitabile T, et al. Lncrna Linc00518 Acts as an Oncogene in Uveal Melanoma by Regulating an Rna-Based Network. Cancers (2020) 12(12):3867. doi: 10.3390/cancers12123867
35. Wu X, Li X-F, Wu Q, Ma R-Q, Qian J, Zhang R. Lncrna Snhg15 Predicts Poor Prognosis in Uveal Melanoma and Its Potential Pathways. Int J Ophthalmol (2020) 13(8):1195–201. doi: 10.18240/ijo.2020.08.04
36. Kuhn M. Building Predictive Models in R Using the Caret Package. J Stat Softw. (2008) 28(5):1–26. doi: 10.18637/jss.v028.i05
37. Zhou N, Bao J. Ferrdb: A Manually Curated Resource for Regulators and Markers of Ferroptosis and Ferroptosis-Disease Associations. Database J Biol Database Curation (2020) 2020:baaa021. doi: 10.1093/database/baaa021
38. Ritchie ME, Phipson B, Wu D, Hu Y, Law CW, Shi W, et al. Limma Powers Differential Expression Analyses for Rna-Sequencing and Microarray Studies. Nucleic Acids Res (2015) 43(7):e47. doi: 10.1093/nar/gkv007
39. Shannon P, Markiel A, Ozier O, Baliga NS, Wang JT, Ramage D, et al. Cytoscape: A Software Environment for Integrated Models of Biomolecular Interaction Networks. Genome Res (2003) 13(11):2498–504. doi: 10.1101/gr.1239303
40. Chen B, Khodadoust MS, Liu CL, Newman AM, Alizadeh AA. Profiling Tumor Infiltrating Immune Cells With Cibersort. Methods Mol Biol (Clifton. NJ) (2018) 1711:243–59. doi: 10.1007/978-1-4939-7493-1_12
41. Geeleher P, Cox N, Huang RS. Prrophetic: An R Package for Prediction of Clinical Chemotherapeutic Response From Tumor Gene Expression Levels. PloS One (2014) 9(9):e107468. doi: 10.1371/journal.pone.0107468
42. Kujala E, Makitie T, Kivela T. Very Long-Term Prognosis of Patients With Malignant Uveal Melanoma. Invest Ophthalmol Visual Sci (2003) 44(11):4651–9. doi: 10.1167/iovs.03-0538
43. He Y, Ye Y, Tian W, Qiu H. A Novel Lncrna Panel Related to Ferroptosis, Tumor Progression, and Microenvironment Is a Robust Prognostic Indicator for Glioma Patients. Front Cell Dev Biol (2021) 9:788451. doi: 10.3389/fcell.2021.788451
44. Li Y, Gao Y, Niu X, Tang M, Li J, Song B, et al. Lncrna Basp1-As1 Interacts With Ybx1 to Regulate Notch Transcription and Drives the Malignancy of Melanoma. Cancer Sci (2021) 112(11):4526–42. doi: 10.1111/cas.15140
45. Bian D, Shi W, Shao Y, Li P, Song G. Long Non-Coding Rna Gas5 Inhibits Tumorigenesis Via Mir-137 in Melanoma. Am J Trans Res (2017) 9(3):1509–20.
46. Ye J, Li H, Wei J, Luo Y, Liu H, Zhang J, et al. Risk Scoring System Based on Lncrna Expression for Predicting Survival in Hepatocellular Carcinoma With Cirrhosis. Asian Pacific. J Cancer Prevent.: APJCP (2020) 21(6):1787–95. doi: 10.31557/apjcp.2020.21.6.1787
47. Xu S, Wang H, Pan H, Shi Y, Li T, Ge S, et al. Anril Lncrna Triggers Efficient Therapeutic Efficacy by Reprogramming the Aberrant Ink4-Hub in Melanoma. Cancer Lett (2016) 381(1):41–8. doi: 10.1016/j.canlet.2016.07.024
48. Wang L, Han S, Jin G, Zhou X, Li M, Ying X, et al. Linc00963: A Novel, Long Non-Coding Rna Involved in the Transition of Prostate Cancer From Androgen-Dependence to Androgen-Independence. Int J Oncol (2014) 44(6):2041–9. doi: 10.3892/ijo.2014.2363
49. Wu JH, Tian XY, An QM, Guan XY, Hao CY. Linc00963 Promotes Hepatocellular Carcinoma Progression by Activating Pi3k/Akt Pathway. Eur Rev Med Pharmacol Sci (2018) 22(6):1645–52. doi: 10.26355/eurrev_201803_14574
50. Zhou Y, Yin L, Li H, Liu L-H, Xiao T. The Lncrna Linc00963 Facilitates Osteosarcoma Proliferation and Invasion by Suppressing Mir-204-3p/Fn1 Axis. Cancer Biol Ther (2019) 20(8):1141–8. doi: 10.1080/15384047.2019.1598766
51. Wang J, Li C, Xu L, Yang C, Zhang X. Mir-1193 Was Sponged by Linc00963 and Inhibited Cutaneous Squamous Cell Carcinoma Progression by Targeting Sox4. Pathol Res Pract (2019) 215(10):152600. doi: 10.1016/j.prp.2019.152600
52. Jiao H, Jiang S, Wang H, Li Y, Zhang W. Upregulation of Linc00963 Facilitates Melanoma Progression Through Mir-608/Nacc1 Pathway and Predicts Poor Prognosis. Biochem Biophys Res Commun (2018) 504(1):34–9. doi: 10.1016/j.bbrc.2018.08.115
53. Yang Y, Zhang Y, Miao L, Liao W, Liao W. Lncrna Ppp1r14b-As1 Promotes Tumor Cell Proliferation and Migration Via the Enhancement of Mitochondrial Respiration. Front Genet (2020) 11:557614. doi: 10.3389/fgene.2020.557614
54. Dong Z, Li S, Wu X, Niu Y, Liang X, Yang L, et al. Aberrant Hypermethylation-Mediated Downregulation of Antisense Lncrna Znf667-As1 and Its Sense Gene Znf667 Correlate With Progression and Prognosis of Esophageal Squamous Cell Carcinoma. Cell Death Dis (2019) 10:930. doi: 10.1038/s41419-019-2171-3
55. Wang W, Green M, Choi JE, Gijon M, Kennedy PD, Johnson JK, et al. Cd8(+) T Cells Regulate Tumour Ferroptosis During Cancer Immunotherapy. Nature (2019) 569(7755):270. doi: 10.1038/s41586-019-1170-y
56. Lang X, Green MD, Wang W, Yu J, Choi JE, Jiang L, et al. Radiotherapy and Immunotherapy Promote Tumoral Lipid Oxidation and Ferroptosis Via Synergistic Repression of Slc7a11. Cancer Discov (2019) 9(12):1673–85. doi: 10.1158/2159-8290.cd-19-0338
57. Johansson J, Siarov J, Kiffin R, Molne J, Mattsson J, Naredi P, et al. Presence of Tumor-Infiltrating Cd8(+) T Cells and Macrophages Correlates to Longer Overall Survival in Patients Undergoing Isolated Hepatic Perfusion for Uveal Melanoma Liver Metastasis. Oncoimmunology (2020) 9(1):e1854519. doi: 10.1080/2162402x.2020.1854519
58. Pant A, Medikonda R, Lim M. Alternative Checkpoints as Targets for Immunotherapy. Curr Oncol Rep (2020) 22(12):126. doi: 10.1007/s11912-020-00983-y
59. Tocheva AS, Mor A. Checkpoint Inhibitors: Applications for Autoimmunity. Curr Allergy Asthma Rep (2017) 17(10):72. doi: 10.1007/s11882-017-0740-z
60. Kottschade LA, McWilliams RR, Markovic SN, Block MS, Bisneto JV, Pham AQ, et al. The Use of Pembrolizumab for the Treatment of Metastatic Uveal Melanoma. Melanom Res (2016) 26(3):300–3. doi: 10.1097/cmr.0000000000000242
61. Karydis I, Chan PY, Wheater M, Arriola E, Szlosarek PW, Ottensmeier CH. Clinical Activity and Safety of Pembrolizumab in Ipilimumab Pre-Treated Patients With Uveal Melanoma. Oncoimmunology (2016) 5(5):e1143997. doi: 10.1080/2162402x.2016.1143997
62. Zhao L, Xia W, Zhang Y, Zou P, Zhu Q, Zhang R. Efficacy and Safety of Immune Checkpoint Blockades in the Treatment of Ocular Melanoma: A Systematic Review and Meta-Analysis. Front Oncol (2021) 11:781162. doi: 10.3389/fonc.2021.781162
63. Wang X, He Q, Shen H, Xia A, Tian W, Yu W, et al. Tox Promotes the Exhaustion of Antitumor Cd8(+) T Cells by Preventing Pd1 Degradation in Hepatocellular Carcinoma. J Hepatol (2019) 71(4):731–41. doi: 10.1016/j.jhep.2019.05.015
64. Fan F, Liu P, Bao R, Chen J, Zhou M, Mo Z, et al. A Dual Pi3k/Hdac Inhibitor Induces Immunogenic Ferroptosis to Potentiate Cancer Immune Checkpoint Therapy. Cancer Res (2021) 81(24):6233–45. doi: 10.1158/0008-5472.can-21-1547
65. Akira S, Takeda K. Toll-Like Receptor Signalling. Nat Rev Immunol (2004) 4(7):499–511. doi: 10.1038/nri1391
66. Chen X, Xu S, Zhao C, Liu B. Role of Tlr4/Nadph Oxidase 4 Pathway in Promoting Cell Death Through Autophagy and Ferroptosis During Heart Failure. Biochem Biophys Res Commun (2019) 516(1):37–43. doi: 10.1016/j.bbrc.2019.06.015
67. El-Khalik SRA, Ibrahim RR, Ghafar MTA, Shatat D, El-Deeb OS. Novel Insights Into the Slc7a11-Mediated Ferroptosis Signaling Pathways in Preeclampsia Patients: Identifying Pannexin 1 and Toll-Like Receptor 4 as Innovative Prospective Diagnostic Biomarkers. J Assist Reprod Genet (2022) 39:1115–24. doi: 10.1007/s10815-022-02443-x
68. Sethi T, Kovach AE, Rubinstein SM, Lee L, Wang Y, Morgan DS, et al. Correlation of Toll-Like Receptor (Tlr) and Pd-1 Pathways in Central Nervous System Lymphoma: A Clinicopathological Study on Outcomes. Blood (2016) 128(22):929. doi: 10.1182/blood.V128.22.929.929
69. Shen WX, Zeng X, Zhu F, Wang YL, Qin C, Tan Y, et al. Out-Of-the-Box Deep Learning Prediction of Pharmaceutical Properties by Broadly Learned Knowledge-Based Molecular Representations. Nat Mach Intell (2021) 3(4):334–+. doi: 10.1038/s42256-021-00301-6
70. Maria Piulats J, Espinosa E, de la Cruz-Merino L, Varela M, Alonso Carrion L, Martin-Algarra S, et al. Nivolumab Plus Ipilimumab for Treatment-Naive Metastatic Uveal Melanoma: An Open-Label, Multicenter, Phase Ii Trial by the Spanish Multidisciplinary Melanoma Group (Gem-1402). J Clin Oncol (2021) 39(6):586–+. doi: 10.1200/jco.20.00550
71. Spagnolo F, Grosso M, Picasso V, Tornari E, Pesce M, Queirolo P. Treatment of Metastatic Uveal Melanoma With Intravenous Fotemustine. Melanom Res (2013) 23(3):196–8. doi: 10.1097/CMR.0b013e3283610586
72. Augsburger JJ, Correa ZM, Shaikh AH. Effectiveness of Treatments for Metastatic Uveal Melanoma. Am J Ophthalmol (2009) 148(1):119–27. doi: 10.1016/j.ajo.2009.01.023
73. Schinzari G, Rossi E, Cassano A, Dadduzio V, Quirino M, Pagliara M, et al. Cisplatin, Dacarbazine and Vinblastine as First Line Chemotherapy for Liver Metastatic Uveal Melanoma in the Era of Immunotherapy: A Single Institution Phase Ii Study. Melanom Res (2017) 27(6):591–5. doi: 10.1097/cmr.0000000000000401
Keywords: ferroptosis, lncRNA, immune microenvironment, uveal melanoma, bioinformatics, prognostic value
Citation: Ma X, Yu S, Zhao B, Bai W, Cui Y, Ni J, Lyu Q and Zhao J (2022) Development and Validation of a Novel Ferroptosis-Related LncRNA Signature for Predicting Prognosis and the Immune Landscape Features in Uveal Melanoma. Front. Immunol. 13:922315. doi: 10.3389/fimmu.2022.922315
Received: 17 April 2022; Accepted: 10 May 2022;
Published: 14 June 2022.
Edited by:
Xian Zeng, Fudan University, ChinaCopyright © 2022 Ma, Yu, Zhao, Bai, Cui, Ni, Lyu and Zhao. This is an open-access article distributed under the terms of the Creative Commons Attribution License (CC BY). The use, distribution or reproduction in other forums is permitted, provided the original author(s) and the copyright owner(s) are credited and that the original publication in this journal is cited, in accordance with accepted academic practice. No use, distribution or reproduction is permitted which does not comply with these terms.
*Correspondence: Qinghua Lyu, Lyuqh2020@163.com; Jun Zhao, doctorzhaojun@163.com
†These authors have contributed equally to this work and share first authorship
Disclaimer: All claims expressed in this article are solely those of the authors and do not necessarily represent those of their affiliated organizations, or those of the publisher, the editors and the reviewers. Any product that may be evaluated in this article or claim that may be made by its manufacturer is not guaranteed or endorsed by the publisher.
Research integrity at Frontiers
Learn more about the work of our research integrity team to safeguard the quality of each article we publish.