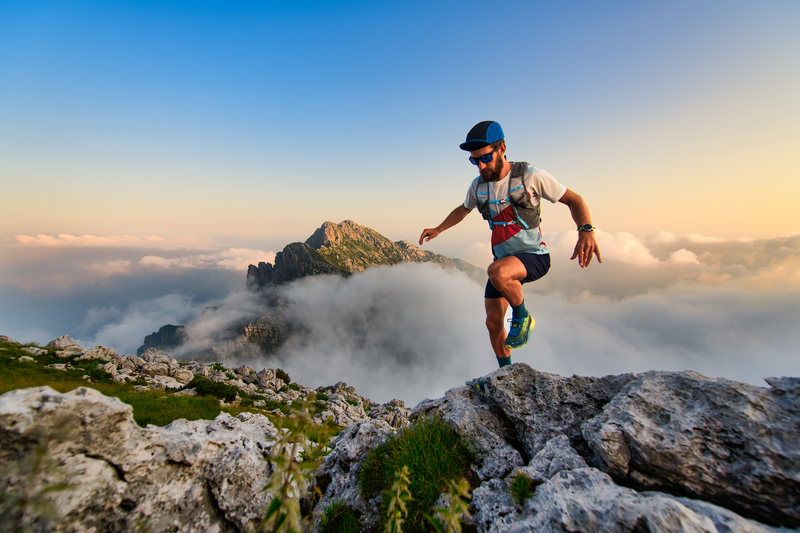
94% of researchers rate our articles as excellent or good
Learn more about the work of our research integrity team to safeguard the quality of each article we publish.
Find out more
ORIGINAL RESEARCH article
Front. Immunol. , 04 July 2022
Sec. Cancer Immunity and Immunotherapy
Volume 13 - 2022 | https://doi.org/10.3389/fimmu.2022.921223
This article is part of the Research Topic RNA Methylation and Tumor Immunity View all 4 articles
Background: Soft-tissue sarcoma (STS) represents a rare and diverse cohort of solid tumors, and encompasses over 100 various histologic and molecular subtypes. In recent years, RNA modifications including m6A, m5C, m1A, and m7G have been demonstrated to regulate immune response and tumorigenesis. Nevertheless, the cross-talk among these RNA modification regulators and related effects upon the tumor microenvironment (TME), immune infiltrates, and immunotherapy in STS remain poorly understood.
Methods: In this study, we comprehensively investigated transcriptional and genetic alterations of 32 RNA modification regulators in STS patients from The Cancer Genome Atlas (TCGA) cohort and validated them in the Gene Expression Omnibus (GEO) cohort. Single-cell transcriptomes were introduced to identify regulators within specific cell types, with own sequencing data and RT-qPCR conducted for biological validation. Distinct regulator clusters and regulator gene subtypes were identified by using unsupervised consensus clustering analysis. We further built the regulator score model based on the prognostic regulator-related differentially expressed genes (DEGs), which could be used to quantitatively assess the risk for individual STS patients. The clinical and biological characteristics of different regulator score groups were further examined.
Results: A total of 455 patients with STS were included in this analysis. The network of 32 RNA modification regulators demonstrated significant correlations within multiple different RNA modification types. Distinct regulator clusters and regulator gene subtypes were characterized by markedly different prognoses and TME landscapes. The low regulator score group in the TCGA-SARC cohort was characterized by poor prognosis. The robustness of the scoring model was further confirmed by the external validation in GSE30929 and GSE17674. The regulator score was negatively correlated with CD4+ T cell, Th2 cell, and Treg cell recruitment and most immunotherapy-predicted pathways, and was also associated with immunotherapy efficacy.
Conclusions: Overall, our study is the first to demonstrate the cross-talk of RNA modification regulators and the potential roles in TME and immune infiltrates in STS. The individualized assessment based on the regulator score model could facilitate and optimize personalized treatment.
Soft-tissue sarcoma (STS) represents a rare and diverse cohort of solid tumors accounting for merely 1% of all adult cancers (1). STS mainly arises from the embryonic mesoderm and encompasses over 100 various histologic and molecular subtypes (2, 3). Previous studies demonstrated that frequently mutated genes including TP53, NF1, and PIK3CA were associated with the prognosis of STS, which suggested potential therapeutic targets (4). In addition, epigenetic regulation also plays a crucial role in tumorigenesis of mesenchymal tumors (5). In recent years, RNA modifications have received increased attention due to their significant effect on gene expression, including DNA transcription to mRNA translation (6, 7).
All RNA bases are capable of hosting different chemical modifications, and RNA modification may contribute to the initiation and development of human diseases (7). Currently, there are over 170 known RNA modifications including but not limited to N6-methyladenosine (m6A), 5-methylcytosine (m5C), N1-methyladenosine (m1A), 7-methylguanosine (m7G), pseudouridine (Ψ), and adenosine-to-inosine RNA editing (A-to-I editing) (8). In most RNA modifications such as m6A and m5C, the process of modification was mediated by the regulator proteins including writers (methyltransferases), readers (binding proteins), and erasers (demethylase) (7, 9, 10). Due to the modulation of RNA metabolism and protein synthesis, RNA modification regulators mediate tumorigenesis on aspects of cell proliferation, differentiation, and pharmacoresistance (11, 12).
The modification of m6A was first found within poly(A) RNA fractions in the 1970s, but growing interests have been paid to this field only from the 2010s with methylated RNA immunoprecipitation-sequencing (MeRIP-Seq) introduced (13, 14). The combination of the next-generation sequencing technology and immunoprecipitation could efficiently map this RNA modification throughout the transcriptome (15). The m6A methyltransferase complexes are mainly composed of methyltransferase-like 3 (METTL3), METTL14, METTL16, Wilms’ tumor 1-associated protein (WTAP), zinc finger CCCH-type containing 13 (ZC3H13), RNA-binding motif protein 15 (RBM15), and the corresponding paralogue RBM15B (9). However, m6A demethylases including fat mass and obesity-associated protein (FTO) and α-ketoglutarate-dependent dioxygenase alkB homolog 5 (ALKBH5) were initially reported only in the recent decade (16, 17). Reader proteins including YT521-B homology (YTH) family and the insulin-like growth factor 2 mRNA-binding proteins (IGF2BP) family could bind m6A-modified mRNA to execute biological functions (18, 19). Significantly, several m6A regulators were reported to be abnormally expressed in human tumor tissues, thus initiating tumorigenesis and metastasis (20).
The presence of m5C, a methylated form in the fifth carbon of cytosine, could occur in both DNA and RNA (21). However, the function of m5C in RNA is less studied. The formation of m5C is primarily introduced by the methyltransferase NOP/SUN (NSUN) protein family, including seven members in human (22, 23). Among them, NSUN2 mainly mediates m5C formation in mRNA (10, 24). Aly/REF export factor (ALYREF) and Y box binding protein 1 (YBX1) act as the readers binding to the mRNA m5C site (10, 24, 25). Increasing evidence indicates that m5C is associated with various cellular activities and tumorigenesis (26).
Studies on the modification of m1A in mRNA have been gradually expanding since the advent of relevant sequencing technologies in recent years (27, 28). Modification regulators including tRNA methyltransferase 6/61A (TRMT6/61A), TRMT61B, and TRMT61C are currently known writers in m1A (29–31). Similar to the modification of m6A, YTH family members could mediate the m1A process by binding to the corresponding mRNA sites (32). Also, similar to ALKBH5 in m6A, methyl groups could also be erased by m1A demethylases including ALKBH1 and ALKBH3 (28, 33). The recent study suggested a specific association between m1A regulators and cell proliferation in gastrointestinal cancers (34).
Moreover, m7G is another significant RNA modification required in most processes of the life cycle of RNA (35). The internal m7G methylation is regulated by METTL1 and WD repeat domain 4 (WDR4) (36). Likewise, RNA guanine-7 methyltransferase (RNMT) could also catalyze the methylation at the guanine N7 position, which is associated with tumor growth (7, 37).
However, the above-mentioned studies only focused solely on specific RNA modifications, while the cross-talk among different patterns of RNA modification regulators in STS remain unclear. In the present study, we comprehensively investigated the cross-talk among RNA modification regulators including m6A, m5C, m1A, and m7G in the STS of The Cancer Genome Atlas (TCGA) and Gene Expression Omnibus (GEO) cohort. Several RNA modification regulator-related patterns were identified, and relative tumor microenvironment (TME) characteristics were intensively studied. Moreover, we established the prognostic regulator-related scoring model for individual STS patients. The RNA modification regulator-related score could help predict chemoimmunotherapy response in STS patients. The findings demonstrated that the cross-talk among different patterns of RNA modification regulators potentially contributed to shaping TME and immune characteristics, which had significant implications for therapeutic guidance.
Overall study workflow is presented in Figure 1. We downloaded gene expression profiles of STS and clinical data corresponding to the samples from the TCGA and GEO databases. The expression matrices of normal adipose and muscle tissue were derived from the Genotype-Tissue Expression (GTEx) database. To maximize compatibility and reduce batch effects between the TCGA and GTEx data, the RNA-Seq data from these two databases were processed and unified following sufficiently rigorous procedures, which consist of the uniform realignment, the quantification of gene expression, and the correction of batch effect (38). All TCGA datasets of RNA sequencing, somatic mutations, copy number variations (CNVs), and clinical data were obtained from the UCSC Xena browser (https://xenabrowser.net/) (39). The mutation data were visualized by utilizing the package “maftools” (version 2.8.0). For the GEO database, two eligible STS cohorts with prognosis data (GSE30929 and GSE17674) and one cohort with single-cell RNA-seq data (GSE131309) were collected for further validation analysis. In total, 455 patients with STS were included in our analysis, and basic information is summarized in Supplementary Table 1.
For pan-cancer analysis, the TCGA pan-cancer FPKM RNA-seq and clinical data were downloaded via the UCSC Xena Browser. The pan-cancer mutation annotation files were obtained from the GDC data portal (https://portal.gdc.cancer.gov/). Furthermore, we also introduced the immunotherapy-treated cohort. The cohort of melanoma patients treated with the combination of anti-PD-1 and anti-CTLA-4 was used to evaluate the association between the regulator score and prognosis after immunotherapy (40).
On the basis of prior studies (8, 9, 24, 41–43), a total of 32 RNA modification regulators including m6A, m5C, m1A, and m7G were included in the current study (Supplementary Table 2). The distribution landscape of selected RNA modification regulators on human chromosomes was plotted by the package “Rcircos” (version 1.2.1). The unsupervised clustering analysis was conducted to recognize RNA modification regulator and gene-related patterns. The R package “ConsensusClusterPlus” (version 1.56.0) was utilized with the key parameters including maxK = 9 and repetitions = 1000, so as to stabilize the identification (44).
As distinct RNA modification regulator patterns were identified via the unsupervised clustering, we further conducted differential gene expression analysis. The R package “limma” (version 3.48.3) was applied to conduct pairwise comparisons in gene expression among distinct patterns. The lmFit and eBayes functions were utilized to ensure accuracy. Multiple comparisons were corrected by using the Benjamini–Hochberg method (45). The differentially expressed genes (DEGs) were filtered with adjusted p-value < 0.05.
In order to probe the biological characteristics of different RNA modification regulator-related patterns, gene set variation analysis (GSVA) was conducted by utilizing the R package “GSVA” (version 1.40.1) (46). Similarly, we used GSVA to compare potential biological differences between low and high regulator score subgroups. The priori-defined gene sets (h.all.v7.5.1 and c2.cp.kegg.v7.4) were downloaded from the Molecular Signatures Database (MSigDB). For differential expression analysis of the hallmark gene sets, GSVA output was submitted to the R package “limma” (version 3.48.3) and tested using moderated t-statistics. The results were further illustrated as bar chats using the R package “ggplot2” (version 3.3.5). The GO annotation analysis was also conducted by utilizing the R package “clusterProfiler” (version 4.0.5), with false discovery rate (FDR) < 0.05 to determine significant enrichments (47).
The interaction of RNA modification regulator expression in STS was assessed by the Spearman correlation test and visualized by the R package “corrplot” (version 0.90). The network of RNA modification regulator combined with prognostic data was further constructed and visualized by utilizing the R package “igraph” (version 1.2.6).
Single-sample gene set enrichment analysis (ssGSEA) was utilized for quantifying specific immune cell infiltration. Marker genes of specific immune cell types for ssGSEA were retrieved from the published study (48). Levels of immune cell infiltration were normalized ranging from 0 to 1. To investigate the association between TME and potential biological processes, we applied the robust tumor mutation burden (TMB) signatures obtained from published data (49). Moreover, we also calculated ESTIMATE scores of samples, a gene signature-based algorithm that estimates stromal and immune infiltration, by using the R package “ESTIMATE” (version 1.0.13) (50).
Furthermore, signatures related to immunotherapy-predicted pathways and cancer-immunity cycles were extracted from published studies (Supplementary Tables 4, 5) (51, 52). The cancer-immunity cycles established the guiding frameworks for cancer immunotherapy (51). The whole cycles included 7 steps: cancer antigen release and presentation (steps 1 and 2), T-cell priming and activation (step 3), immune cell recruitment (step 4), immune cell infiltration into tumors (step 5), T-cell recognition of cancers (step 6), and killing of cancer cells (step 7). The method of calculating the activity of these steps was reported previously (53). In this study, the signature scores of immunotherapy-predicted pathways and cancer-immunity cycles were calculated by GSVA mentioned above. We then used the R package “ggcor” (version 0.9.4.3) to compare the correlations between the regulator score and GSVA scores of these gene sets.
The RNA modification regulator-related scoring system was established as follows. First, distinct RNA modification regulator clusters were identified by the unsupervised clustering, and the overlapping DEGs among these clusters were filtered and selected. Then, the univariate Cox regression analysis was used to estimate the prognostic relevance for each DEG. The significantly prognostic genes were extracted, and the principal component analysis (PCA) was further performed based on these prognostic DEGs. Both PC1 and PC2 of prognostic DEGs were selected to serve as the signature scores. This scoring method has significant strength in focusing on the score of the set with the largest block of well-correlated (or anticorrelated) genes, while downweighing contributions from genes unrelated to most set factors, which was applied in previous studies (54, 55). The formula for the scoring system was as follows: regulator- related score = ∑(PC1i + PC2i) where i represents the expression of the final determined prognostic DEGs.
Single-cell RNA-seq data were acquired from one published study (GSE131309) (56). Based on the package “Seurat” (version 4.0.5), the data were analyzed following the standard pipeline, which was explained in detail on the official website (https://satijalab.org/seurat/). In the current study, the quality control (QC) metrics were consistent with those in the published study. We conducted gene expression normalization by LogNormalize (scale factor = 10,000). Then, 2,000 highly variable genes (HVGs) were identified with the FindVariableGenes function. Following the results of the ElbowPlot, 25 PCs were selected. Then, cell clustering and t-distributed stochastic neighbor embedding (t-SNE) were further performed based on the above analysis. Moreover, we used the same labels from the data resource to annotate specific cell clusters, and detailed annotation approaches were present in corresponding parts in that study (56). The gene expression of RNA modification regulators was further visualized.
The Genomics of Drug Sensitivity in Cancer (GDSC) was accessed to collect drug response data (https://www.cancerrxgene.org/downloads/anova) (57). The drug response data spanned 518 compounds that target 24 pathways. Furthermore, there were nearly 1,000 human cancer cell lines within this database. To assess chemotherapeutic sensitivity, IC50 and drug sensitivity score were used based on the R packages “pRRophetic” (version 0.5) and “oncoPredict” (version 0.2) (58, 59). Moreover, p-value was corrected for multiple comparisons where appropriate.
The human synovial sarcoma cell line (SW-982) and liposarcoma cell line (SW-872) were purchased from Procell Life Science & Technology Co., Ltd. The human skin fibroblast cell line (HSF) was purchased from Fenghui Biotechnology Co., Ltd. The primary human synovial sarcoma cells (hSS-005R) were established as previously described (60). The above cell lines were cultured in Dulbecco’s modified Eagle medium (DMEM) supplemented with 10% fetal bovine serum (FBS) at 37°C in 5% CO2 atmosphere.
Total RNA of cell lines was extracted using the RNA Express Total RNA Kit (M050, NCM Biotech, China). The cDNA synthesis was performed by utilizing the RevertAid First Strand cDNA Synthesis kit (K1622, Thermo Fisher Scientific, United States). The RT-qPCR was performed as previously described (60). The sequences of the primers for RT-qPCR are presented in Table S3.
We validated related gene expression level with our own sequencing data including 4 tumor samples and 4 paired normal tissues. Full-length transcriptome analysis was performed by Biomarker Technologies (Biomarker Technologies Ltd, Beijing, China). All operations were in accordance with Oxford Nanopore Technologies (Oxford Nanopore Technologies, Oxford, United Kingdom). The analysis platform (BMKCloud) performs correlation analysis based on reference sequences and nanopore transcriptome sequencing data.
Statistical analysis was performed by using R software (version 4.1.0). The Spearman correlation test was conducted to calculate the correlations of RNA modification regulators. For pairwise comparisons, data were compared by utilizing Student’s t-tests for parametric comparisons and Wilcoxon signed-rank test for nonparametric comparisons. Similarly, one-way ANOVA and Kruskal–Wallis test were applied when over two groups were analyzed. Survival curve comparison was conducted by Log-rank test. Univariate and multivariate Cox regression were utilized to identify significant prognostic factors, with hazard ratio (HR) and 95% confidence interval (CI) calculated. Receiver operating characteristic (ROC) curves were performed to assess the accuracy of the model by utilizing the R package “timeROC” (version 0.4). The function “surv_cutpoint” of the package “survminer” (version 0.4.9) was repeatedly conducted to determine the optimal cutoff values of the regulator scores in the datasets. The patients in the datasets were further dichotomized into low and high regulator score subgroups. We also used chi-square or Fisher exact tests to compare clinical characteristics between two distinct groups. Statistical significance was assigned with two-sided p-value < 0.05.
In this study, a total of 32 RNA modification regulators were selected. The somatic mutation frequency of RNA modification regulators in STS was first assessed. The mutations were concentrated within 14 RNA modification regulators, and only 18 of 237 STS patients (7.59%) displayed regulator-associated mutations (Figure 2A). For a global view in pan-cancer, we also explored the mutation frequency in 32 other cancer types from the TCGA cohort (Supplementary Figure 1E). It could be found that the proportion of regulator mutation was relatively low in uveal melanoma (UVM), pheochromocytoma and paraganglioma (PCPG), and testicular germ cell tumors (TGCT), while uterine corpus endometrial carcinoma (UCEC) presented more mutations. The analysis of mutation co-occurrence indicated significant correlations among gene mutations including LRPPRC, IGF2BP2, YTHDC2, ALKBH5, and WTAP (Supplementary Figure S1A). However, there was no significant survival difference in overall survival (OS) or disease-free survival (DFS) between STS patients with mutations and without mutations (Supplementary Figures 1B, C). Further examination of the CNV alteration indicated that ALKBH5, METTL1, and METTL3 exhibited relatively evident CNV gain, while METTL16 and ZC3H13 presented a relatively substantial proportion of CNV loss (Figure 2B). Additionally, the chromosome locations of each RNA modification regulator are depicted in Figure 2C. The Gene Ontology (GO) analysis of the regulators indicated that biological processes were mainly enriched in terms of RNA modification (Supplementary Figure S1D).
Figure 2 Transcriptional and genetic alterations of RNA modification regulators in STS. (A) The mutation frequency of RNA modification regulators in 237 STS patients. The upper bar reflects the TMB. Every single column represents a patient. (B) The CNV alteration of RNA modification regulators in STS. The height of the column with a specific color represents the CNV frequency (%). The color represents the CNV status including gain (red) and loss (blue). (C) The location of RNA modification regulators on human chromosomes. (D–G) The expression level of 32 RNA modification regulators between STS samples (red) and normal tissues (blue) in the TCGA-SARC cohort. The box plot extends from the 25th to 75th percentile and the central line indicates the median. (H) Principal component analysis (PCA) of 32 RNA modification regulators for discriminating between tumor and normal samples. ns, p ≥ 0.05; *, 0.01 ≤ p < 0.05; **, 0.001 ≤ p < 0.01; ***, 0.0001 ≤ p < 0.001; ****, p < 0.0001.
Next, we characterized the gene expression of RNA modification regulators in STS samples against normal tissues. Of 32 RNA modification regulators, 30 regulators were significantly differentially expressed (Figures 2D–G). The t-SNE visualization of single cells from STS samples of the GSE131309 dataset was colored by specific cell clusters (Figure 3A). The distribution and level of gene expression were also illustrated (Figure 3B and Supplementary Figures 2A, B). Notably, METTL3, METTL16, and IGF2BP2 mainly clustered in malignant cells, while WTAP, ZC3H13, HNRNPA2B1, YTHDF2, YTHDF3, and YTHDC1 were broadly distributed in all cell clusters. We also conducted RT-qPCR analysis to validate regulator expression in cell lines of STS (Figures 3C–F). The expression levels of METTL14, WTAP, YTHDC1, and LRPPRC were significantly lower in STS cell lines including SW-982, hSS-005R, and SW-872, compared with the expression in HSF. The expression level of RNA modification regulators validated by our own sequencing data is shown in Supplementary Figure 3. In four pairs of tumor and normal samples, the differences in expression levels of several regulators were consistent with those in public datasets. The prognostic roles of these regulators in TCGA pan-caner datasets were also explored (Figure 3H). Furthermore, based on the expression level of 32 RNA modification regulators, we could efficiently discriminate STS samples from normal tissues (Figure 2H).
Figure 3 Validation and interaction of RNA modification regulator expression in STS. (A) The t-SNE plot demonstrating specific cell clusters. Each color corresponds to one cell type. (B) The t-SNE plots illustrating the expression level of specific genes. (C–F) Validation of expression of RNA modification regulators in cell lines. (G) The interaction of RNA modification regulator expression in STS. The colors represent the types of RNA modification. The size of the circles indicated the prognostic effect assessed by p-value. The dots within the circles represent the prognostic roles including protective factor (green) and risk factor (black). (H) The prognostic roles of 32 RNA modification regulators across cancers in TCGA. Red indicates the higher regulator expression related to poor survival, while blue suggests the association with good survival. Only statistically significant prognostic factors were present. *, 0.01 ≤ p < 0.05; **, 0.001 ≤ p < 0.01; ***, 0.0001 ≤ p < 0.001; ****, p < 0.0001.
The network of 32 RNA modification regulators presented a comprehensive landscape of the interactions (Figure 3G). It is noteworthy that the significant correlations were not limited to the single RNA modification but to multiple different RNA modification types. More remarkably, METTL1 showed negative correlation with a substantial proportion of RNA modification regulators. These findings suggested potential cross-talk among these regulators, which might play significant roles in the development of distinct RNA modification patterns.
In order to further elucidate distinct RNA modification regulator patterns, unsupervised consensus clustering was conducted to group STS patients in the TCGA-SARC cohort based on the expression of 32 regulators (Supplementary Figuress 4A–F). We identified K = 3 as the optimal index according to the elbow method (61). Correspondingly, 259 STS patients in the TCGA-SARC cohort were identified into 3 clusters including 120 cases in cluster A, 83 cases in cluster B, and 56 cases in cluster C (Figure 4A). These clusters were further named as Regulator Clusters A–C, respectively (Supplementary Table 6). Analysis of the survival curve indicated a significant difference among 3 distinct regulator clusters, and Regulator Cluster B showed an apparent survival advantage (Figure 4B). For further comparison of pathway enrichment among distinct regulator clusters, GSVA was conducted. As illustrated in Figures 4D, E and Supplementary Figure 4G, Regulator Cluster C was significantly enriched in pathways associated with DNA replication, mismatch repair, and base excision repair. Of note, further ssGSEA demonstrated that Regulator Cluster C was also enriched with innate immune cell infiltrations that include myeloid-derived suppressor cells (MDSCs), macrophages, monocytes, and natural killer (NK) cells (Figure 4C).
Figure 4 Identification of regulator clusters and related biological characteristics. (A) Heatmap of 32 RNA modification regulators among distinct regulator clusters. (B) The OS curve comparing survival of the TCGA-SARC cohort in Regulator Clusters A (blue), Regulator Clusters B (orange), and Regulator Clusters C (red). (C) The infiltration of immune cells within distinct regulator clusters. The box plot extends from the 25th to 75th percentile, and the central parallel line indicates the median. (D, E) The GSVA illustrating pathway enrichment among distinct regulator clusters. ns, p ≥ 0.05; *, 0.01 ≤ p < 0.05; **, 0.001 ≤ p < 0.01; ***, 0.0001 ≤ p < 0.001.
To investigate potential associations between TME cell infiltration and RNA modification regulators, the immune cell compositions were compared among distinct patterns (Supplementary Figure 1F). As illustrated, most regulators showed negative correlations with CD8+ T cells, and were positively correlated with T helper cells and Treg cells. For further exploration of clinical and biological characteristics of distinct regulator clusters, a total of 117 DEGs were identified as RNA modification regulator-related signature, which was illustrated within the overlapping of the Venn diagram (Figure 5A). These DEGs were subsequently evaluated by the univariate Cox regression analysis, and 54 DEGs with a prognostic effect were screened out (Supplementary Table 7). The main terms in biological processes of GO analysis of these 54 DEGs included RNA splicing, RNA catabolic process, and nuclear division (Figure 5G). Consistent with the identification method of regulator clusters, the unsupervised clustering analysis revealed 3 distinct genomic subtypes including Regulator genes S1–S3 based on the 54 DEGs (Supplementary Figures 5A–F and Supplementary Table 6). We found clear distinction of gene expression among these subtypes, and clinical characteristics were also variable as illustrated (Figure 5D). Notably, significant survival differences existed among these regulator gene subtypes, and Regulator gene S3 was correlated with poor prognosis (Figure 5B). The stromal scores of Regulator gene S3 were also relatively lower compared with scores in the other two regulator gene subtypes (Figure 5C). The pathway enrichment analysis demonstrated significant enrichment for DNA replication, homologous recombination, and mismatch repair in Regulator gene S3 (Figure 5E and Supplementary Figure 5G, H). Subsequent analysis based on TMB signatures also showed the enhanced activity of base excision repair, DNA damage response, and epithelial–mesenchymal transition (EMT) in Regulator gene S3 (Figure 5F). For immune cell infiltration, Regulator gene S3 was enriched with activated CD4+ T cells and Th2 cells (Figure S5I).
Figure 5 Identification of distinct genomic subtypes and TME cell infiltration. (A) Venn diagram showing the overlapping of RNA modification regulator-related DEGs. (B) The OS curve comparing survival of the TCGA-SARC cohort in Regulator gene S1 (blue), Regulator gene S2 (orange), and Regulator gene S3 (red). (C) Differences in stromal score among three regulator gene subtypes in the TCGA-SARC cohort. (D)The unsupervised clustering analysis of the 54 overlapping DEGs in the TCGA-SARC cohort. (E) The GSVA illustrating pathway enrichment among distinct regulator gene subtypes. (F) Differences in TMB signatures among three regulator gene subtypes in the TCGA-SARC cohort. (G) GO enrichment analysis of the 54 overlapping DEGs. ns, p ≥ 0.05; *, 0.01 ≤ p < 0.05; **, 0.001 ≤ p < 0.01; ***, 0.0001 ≤ p < 0.001; ****, p < 0.0001.
Although distinct RNA modification-related regulator clusters and regulator gene subtypes were identified, the analysis was limited within the TCGA-SARC cohort. We further built the RNA regulator score model based on the prognostic regulator-related DEGs, which could be used to calculate for each STS patient. The flow diagram of the development of the regulator score is illustrated in Figure 6A. There was a significant difference in the regulator score among distinct regulator gene subtypes (Supplementary Figure 6A). The STS patients were further dichotomized into a high regulator score and a low regulator score group according to the cutoff value calculated by the algorithm. It was worth noting that patients with a low regulator score were associated with better prognosis in the TCGA-SARC cohort (p < 0.001) (Figure 6B). The robustness of the scoring model was further confirmed by the external validation in GSE30929 and GSE17674. The external validation indicated a concordant result in the DFS of GSE30929 (p < 0.001) and OS of GSE17674 (p = 0.003) with that in the TCGA-SARC cohort (Figures 6G, H). The areas under the curve (AUCs) of ROCs for 1-, 3-, and 5-year survival also achieved acceptable values, namely, 0.792, 0.705, and 0.744 in GSE17674, respectively (Figure 6I and Supplementarys Figure 6B, C). Subgroup analysis of regulator scores in different clinical characteristic groups in the TCGA-SARC cohort also yielded stable results (Supplementary Figures 6D–N). In addition, clinical characteristics including gender, age, histology, site, and vital status between the high and low regulator score groups were compared (Figure 6J). Multivariate Cox regression analysis was further conducted to determine the prognostic role of the regulator score in STS patients. As illustrated, the regulator score was identified as a robust independent prognostic indicator in the TCGA-SARC cohort (HR = 4.45, 95% CI 2.65–7.49, p < 0.001) (Figure 6K).
Figure 6 Construction of regulator score and related clinical characteristics. (A) Alluvial diagram illustrating the association among regulator clusters, regulator gene subtypes, regulator score groups, and vital status. (B) The OS curve comparing survival of the TCGA-SARC cohort in low regulator score (blue) and high regulator score (red) groups. (C) Correlations between regulator score and immune cell infiltration by the Spearman correlation test. (D–F) Differences of ESTIMATES score (D), stromal score (E), and tumor purity (F) between high and low regulator score groups. (G, H) The DFS curve of GSE30928 (G) and the OS curve of GSE17674 (H) in low (blue) and high regulator score (red) groups. (I) Time-dependent ROC evaluating the predictive performance of the regulator score in the TCGA-SARC cohort. (J) Clinical characteristics between low and high regulator score groups in the TCGA-SARC cohort. (K) Multivariate Cox regression of clinical characteristics with regulator score. The horizontal line represents the 95% CI for each variable. The vertical dot line represents the HR of STS patients. *, 0.01 ≤ p < 0.05; **, 0.001 ≤ p < 0.01; ***, 0.0001 ≤ p < 0.001.
Because of the significant correlation between the regulator score and the prognosis of STS patients, we further investigated potential biological processes associated with the regulator score. As illustrated in Figure 6C, the regulator score exhibited significant inverse correlations with innate immune cells including CD56bright NK cells, eosinophils, immature dendritic cells, mast cells, and monocytes. Moreover, the ESTIMATE score and the stromal score were significantly higher in the STS patients with a low regulator score, while those with a high regulator score obtained a significantly higher tumor purity score (Figures 6D–F). Higher TMB was also found in the high regulator score group (Figure 7A and Supplementary Figure 5J). In the TCGA-SARC cohort, survival analysis demonstrated that the poor prognosis was associated with lower TMB, which would further deteriorate combined with a higher regulator score (Figures 7B, C). For the frequency of the somatic mutation between these two groups, we observed more mutations in the high regulator score group with a sample mutation proportion of 83.61%, compared with 61.49% in the low regulator score group (Figures 7D, E). It is also noteworthy that the high regulator score group had a significantly higher frequency of arm-level amplification and deletion than the low regulator score group (p < 0.05) (Figure 8A). When comparing the pathway activities between distinct regulator score groups, we found a considerable increase of reactive oxygen species production and oxidative phosphorylation, but the activity level of the Wnt/β-catenin signaling pathway strongly decreased (Figure 7F). As the cancer-immunity cycles were of guiding significance for immunotherapy, the correlation with the regulator score was also explored. There was an inverse correlation among CD4+ T cell, dendritic cell, Th17 cell, Th2 cell, and Treg cell recruitment with the regulator score (Figure 7G). Meanwhile, the regulator score was negatively correlated with most of the immunotherapy-predicted pathways, indicating its potential role in related immunotherapy.
Figure 7 Association between regulator score and biological processes. (A) The difference in the TMB level between low and high regulator score groups in the TCGA-SARC cohort. (B) The OS curve comparing survival of high- and low-TMB groups in the TCGA-SARC cohort. (C) The OS curve illustrating the subgroup analysis of TMB level and regulator score. (D, E) The somatic mutation frequency of high (D) and low (E) regulator score groups in the TCGA-SARC cohort. (F) Differences in pathway activities between low and high regulator score groups. (G) Correlation of the regulator score with immunotherapy-predicted pathways (lower left) and cancer immunity cycle (upper right).
Figure 8 The potential role of regulator score in CNV and chemotherapeutic value. (A) The frequency of arm-level amplification and deletion between low and high regulator score groups. (B) The progression-free survival (PFS) curve comparing survival of high and low regulator score groups in a cohort of melanoma patients treated with the combination of anti-PD-1 and anti-CTLA-4. (C) The proportion of clinical response to anti-PD-1 with anti-CTLA-4 immunotherapy in high and low regulator score groups in the melanoma cohort. (D–F) The box plot of the estimated IC50 of MK-2206 (D), erlotinib (E), and Nutlin-3a (F) between low and high regulator score groups. (G–I) The box plot of the predicted drug sensitivity scores of MK-2206 (G), erlotinib (H), and Nutlin-3a (I) between low and high regulator score groups. A lower drug sensitivity score indicated that this group could be more sensitive to the drug therapy. *, p < 0.05.
The strong link between the regulator score and TME prompted us to further explore the predictive effect of the regulator score on response to checkpoint immunotherapy. As there was no information about immunotherapy in the TCGA-SARC cohort, a cohort of melanoma patients treated with the combination of anti-PD-1 and anti-CTLA-4 was utilized. The regulator score of an individual patient was calculated on the basis of the scoring system mentioned above. Surprisingly, patients with a low regulator score exhibited significant survival advantage compared with those in the high regulator score group (p = 0.040) (Figure 8B). Moreover, the response rates to immunotherapy were also significantly higher in the low regulator score group compared with those in high regulator score group (p < 0.001) (Figure 8C). In addition to immunotherapy, other chemotherapeutic agents might also exert a potential anti-cancer effect. Therefore, the GDSC database was selected because of the large chemotherapeutic agents available. After systematic drug screening, three common chemotherapeutic agents including MK-2206, erlotinib, and Nutlin-3a were identified with IC50 varying significantly between the high and low regulator score groups (Figures 8D–F). Significant differences in the drug sensitivity score of these drugs were also illustrated between two scoring groups, suggesting that the low regulator score group was more sensitive to these drugs (Figures 8G–I).
Numerous studies have demonstrated that RNA modification played an important role in multiple biological processes mediated by various regulators (9, 10, 42, 43). However, considerable studies focused solely on the single type of RNA modification. Furthermore, the overall landscape of TME and immune infiltrates mediated by different patterns of RNA modification regulators have not been studied in depth. Consequently, exploring the cross-talk among RNA modification regulators including m6A, m5C, m1A, and m7G in the STS may help elucidate the characteristics of TME and corresponding subtypes and further develop a therapeutic strategy for STS treatment.
Hence, a total of 32 RNA modification regulators were thoroughly studied on the basis of gene expression, mutation patterns, and CNV profiles. The proportion of mutations within regulators were relatively lower, which was consistent with related studies in other malignancies (62, 63). We also demonstrated that the expression levels of RNA modification regulators were significantly different between STS and normal samples. The expression of several RNA modification regulators was verified by utilizing RT-qPCR in cell lines, which may shed creative lights on further research on STS. The emergence of single-cell transcriptomes contributed to identifying gene expression at high resolution within specific cell types (64). Of note, METTL3, METTL16, and IGF2BP2 were mainly represented in the malignant clusters. According to the study focusing on the role of METTL3 in lung adenocarcinoma, METTL3 could enhance mRNA translation including EGFR and Hippo pathways, further promoting growth and invasion of human lung cancer cells (65). In line with this study, the expression level of METTL3 was significantly upregulated in osteosarcoma tissues and cell lines (66). Moreover, silencing METTL3 could suppress tumor proliferation and migration and was also associated with lymphoid enhancer-binding factor 1 (LEF1) and Wnt/β-catenin signaling pathway. Substantial cross-talk among RNA modification regulators was observed, which was consistent with the results in colorectal cancer (67). As a limited number of studies have been conducted concerning the cross-talk of RNA modification regulators, further studies with biological mechanism research are warranted in the future.
Concerning the distinct characteristics of these regulators, three regulator clusters were identified by utilizing the unsupervised consensus clustering. This method could help discover conformational details that might be masked due to population averages, thus identifying potential meaningful patterns (68, 69). Regulator Cluster C was characterized by poor prognosis compared with the other two clusters. It has been demonstrated that MDSCs were relatively enriched in Regulator Cluster C, which has been extensively studied and considered as immunosuppressive cells (70). The proangiogenic capacity of immature myeloid cells may facilitate tumor growth and metastasis (71). Preliminary evidence suggested promising activity when drugs targeting reprogramming of the metabolism of MDSCs were applied in combination with immune checkpoint inhibitors (72). The DEGs on the basis of distinct regulator clusters were mainly enriched in the biological process of RNA splicing, indicating the significant role of RNA modification in regulating RNA splicing, stabilization, and metabolism (20, 73–75). Similar to the analysis of regulator clusters, three regulator gene subtypes were identified with markedly different prognoses and TME landscapes. The stromal scores were significantly reduced in Regulator gene S3, also suggesting the low level of infiltrating stromal cells in this subtype in STS (50).
Although STS cohorts could be identified into distinct clusters based on the robust clustering algorithm, an accurate approach was needed to quantitatively assess RNA modification-related risk for STS patients at the single individual level. The RNA regulator score model established in the current study has significant clinical values and could guide treatment for STS patients. Firstly, the RNA regulator score could serve as a strong prognostic indicator for STS. As we expected, Regulator gene S3 with a relatively poor prognosis also scored significantly higher. Moreover, the RNA regulator score could efficiently distinguish TME characteristics concerning tumor purity, and stromal and immune cell infiltration in individual STS patients. This study found a mild positive correlation between regulator score and TMB. Notably, the relationship between TMB and survival was controversial and varied across tumor types (76, 77). The TCGA-SARC patients with high TMB presented a better prognosis, which needs to be further explored in future studies. Furthermore, a combined prediction model including TMB and the regulator score could provide better outcome prediction. In this study, the potential role of the regulator score in cancer-immunity cycles cannot be ignored, which also suggested that RNA modification could regulate immunotherapy (78). As is widely known, tumor progression was associated with the driver mutations (79). Moderate differences were identified in multiple mutant genes between groups with different regulator scores. We observed a relatively increased mutation rate of ATRX in the high regulator score group, which was characterized by poor prognosis. Previous studies demonstrated that protein coded by ATRX has been implicated in chromatin remodeling at telomeres. Therefore, ATRX mutations may consequently lead to an abnormal telomeric phenotype, which has been proven in glioma (80, 81).
In the absence of an STS cohort receiving immunotherapy, we introduced an independent melanoma dataset treated with the combination of anti-PD-1 and anti-CTLA-4. The strength of the regulator score was further verified while additional prospective studies of STS concerning immunotherapy are still needed for further verification. Additionally, the regulator score-based drug screening could identify potential chemotherapeutic agents for personalized therapy.
There are growing lines of evidence of widespread cross-talk between RNA modification regulators in a wide variety of tumors (82). In colorectal cancer, the interactions of 26 RNA modification regulators could redefine the characteristics of TME and give a more reliable indication of the prognosis (67). Moreover, the importance of noncoding RNAs with RNA modification has become increasingly appreciated in recent years (83, 84). With further study upon RNA modification, more specific regulators have been identified and a total of 32 RNA modification regulators have been included in the current study. Future research should also focus on the cross-talk between RNA modification regulators in non-neoplastic diseases.
In conclusion, this study, for the first time, represented a comprehensive and systematic analysis of four types of RNA modification regulators in STS. The cross-talk of RNA modification regulators played a significant role in regulating the complexity of TME, which was strongly associated with the prognosis of STS patients. The individualized assessment based on the regulator score model could facilitate and optimize personalized treatment. In a broad perspective, this work reinforces the significance of the cross-talk of RNA modification regulators and sheds new light on the individualized treatment strategies for STS patients.
The datasets presented in this study can be found in online repositories. The names of the repository/repositories and accession number(s) can be found below: NCBI GEO, accession no: GSE198568. https://www.ncbi.nlm.nih.gov/geo/query/acc.cgi?acc=GSE198568.
LQ and ZL conceived and designed this study. WZ, XR, LQ, and ZY performed the data analysis, plotting of figures, and writing. RX, RC, and CT were responsible for the critical reading of the manuscript. All authors participated in interpreting the results and revision of the manuscript and approved the submitted version. All authors read and approved the final manuscript.
This work was supported by grants from the National Natural Science Foundation of China (NSFC; No. 81902745, No. 82172500, and No. 82103228), the Hunan Provincial Research and Development Program in Key Areas (2020DK 2003), and the China Postdoctoral Science Foundation (No. 2021M693557).
The authors declare that the research was conducted in the absence of any commercial or financial relationships that could be construed as a potential conflict of interest.
The reviewer YZ declared a shared parent affiliation with the authors LQ, WZ, XR, RX, RC, CT and ZL to the handling editor at the time of review.
All claims expressed in this article are solely those of the authors and do not necessarily represent those of their affiliated organizations, or those of the publisher, the editors and the reviewers. Any product that may be evaluated in this article, or claim that may be made by its manufacturer, is not guaranteed or endorsed by the publisher.
We are grateful to all of the patients and cooperators for participating in this study, especially for the data from the TCGA and GEO.
The Supplementary Material for this article can be found online at: https://www.frontiersin.org/articles/10.3389/fimmu.2022.921223/full#supplementary-material
Supplementary Table 1 | Basic information of public datasets included in this study.
Supplementary Table 2 | Summary of different RNA modification regulators.
Supplementary Table 3 | Sequences of the primers used in RT-qPCR.
Supplementary Table 4 | Immunotherapy-predicted pathways signatures.
Supplementary Table 5 | Cancer immunity cycles signatures.
Supplementary Table 6 | Samples clustering in TCGA-SARC cohort.
Supplementary Table 7 | Prognostic analysis of 54 RNA modification regulator-related DEGs using a univariate Cox analysis.
Supplementary Figure 1 | Correlation and prognostic analysis of RNA modifications regulators in STS. (A) The mutation co-occurrence and exclusion analysis for 14 mutated regulators. The color represents the correlations including co-occurrence (green) and mutually exclusion (purple). (B) Overall survival (OS) curve of STS patients with (red) and without mutations (green) of RNA modification regulators. (C) Disease-free survival (DFS) curve of STS patients with (red) and without mutations (green)of RNA modification regulators. (D) GO enrichment analysis of 32 RNA modification regulators. The horizontal axis represents the gene ratio. (E) The mutation frequency of 32 RNA modification regulators in other 32 cancer types of TCGA cohort. The sample size of each cancer type was given in the in bracket. (F) The spearman correlation between TME cell infiltration and RNA modification regulators. (G) Association of expression of RNA modification regulators with OS based on univariate Cox regression analysis.
Supplementary Figure 2 | Expression of RNA modification regulators in STS at single-cell resolution. (A) The t-SNE plots illustrating the expression level of specific genes. (B) The stacked violin plots demonstrating the expression level of specific genes across cell clusters.
Supplementary Figure 3 | The expression level of RNA modification regulators verified by sequencing data. (A–L) The expression level of RBM15B, ZC3H13, METTL16, IGF2BP2, FMR1, YTHDF2, TRMT61A, TRMT61B, ALKBH1, ALLYREF, WDR4 and RNMT based on the sequencing data.
Supplementary Figure 4 | Analysis of RNA modification Regulator Clusters in STS. (A–D) Consensus clustering based on RNA modification regulators (K = 2-5). (E) Consensus cumulative distribution function (CDF) Plot based on RNA modification regulators. (F) Delta area plot of consensus clustering based on RNA modification regulators. (G) The GSVA illustrating pathway enrichment among distinct Regulator Clusters.
Supplementary Figure 5 | Analysis of Regulator gene subtypes in STS. (A–D) Consensus clustering based on regulator-related DEGs (K = 2-5). (E) The CDF Plot based on the 54 RNA modification regulator-related DEGs. (F) Delta area plot of consensus clustering based on the DEGs. (G, H) The GSVA illustrating pathway enrichment among distinct Regulator gene subtypes. (I) The infiltration of immune cells within distinct Regulator gene subtypes. (J) Scatter plots depicting the positive correlation between TMB and Regulator Score in TCGA-SARC cohort.
Supplementary Figure 6 | Validation and subgroup analysis of Regulator Score. (A) Differences of Regulator Score among distinct Regulator Clusters. (B, C) Time-dependent ROC evaluating the predictive performance of Regulator Score in GSE17674 (B) and GSE30929 (C). (D–N) Subgroup analysis of Regulator Scores in different clinical characteristics groups including age, gender, site and histology.
STS, soft-tissue sarcoma; m6A, N6-methyladenosine; m5C, 5-methylcytosine; m1A, N1-methyladenosine; m7G, 7-methylguanosine; Ψ, pseudouridine; A-to-I editing, adenosine-to-inosine RNA editing; MeRIP-Seq, methylated RNA immunoprecipitation-sequencing; METTL3, Methyltransferase Like 3; METTL14, Methyltransferase Like 14; METTL16, Methyltransferase Like 16; WTAP, Wilms’ tumor 1-associated protein; ZC3H13, zinc finger CCCH-type containing 13; RBM15, RNA-binding motif protein 15; FTO, obesity-associated protein; ALKBH5, α-ketoglutarate-dependent dioxygenase alkB homolog 5; YTH, YT521-B homology; IGF2BP, insulin-like growth factor 2 mRNA-binding proteins; NSUN, methyltransferases NOP/SUN; ALYREF, Aly/REF export factor; YBX1, Y box binding protein 1; TRMT6/61A, tRNA methyltransferase 6/61A; TRMT61B, tRNA methyltransferase 61B; TRMT61C, tRNA methyltransferase 61C; WDR4, WD repeat domain 4; RNMT, RNA guanine-7 methyltransferase; TCGA, The Cancer Genome Atlas; GEO, Gene Expression Omnibus; TME, tumor microenvironment; GTEx, the Genotype-Tissue Expression; CNVs, copy number variations; DEGs, differentially expressed genes; GSVA, gene set variation analysis; GO, Gene Ontology; MSigDB, Molecular Signatures Database; FDR, false discovery rate; ssGSEA, single-sample gene set enrichment analysis; TMB, tumor mutation burden; PCA, principal component analysis; GGI, gene expression grade index; QC, quality control; HVG, highly variable genes; t-SNE, t-distributed stochastic neighbor embedding; GDSC, The Genomics of Drug Sensitivity in Cancer; HR, hazard ratio; CI, confidence interval; ROC, receiver operating characteristic; UVM, uveal melanoma; PCPG, pheochromocytoma and paraganglioma; TGCT, testicular germ cell tumors; UCEC, uterine corpus endometrial carcinoma; OS, overall survival; DFS, disease-free survival; MDSC, myeloid-derived suppressor cell; EMT, epithelial–mesenchymal transition; LEF1, lymphoid enhancer-binding factor 1.
1. Gamboa AC, Gronchi A, Cardona K. Soft-Tissue Sarcoma in Adults: An Update on the Current State of Histiotype-Specific Management in an Era of Personalized Medicine. CA Cancer J Clin (2020) 70(3):200–29. doi: 10.3322/caac.21605
2. Kasper B, Sleijfer S, Litière S, Marreaud S, Verweij J, Hodge RA, et al. Long-Term Responders and Survivors on Pazopanib for Advanced Soft Tissue Sarcomas: Subanalysis of Two European Organisation for Research and Treatment of Cancer (Eortc) Clinical Trials 62043 and 62072. Ann Oncol (2014) 25(3):719–24. doi: 10.1093/annonc/mdt586
3. Kallen ME, Hornick JL. The 2020 Who Classification: What’s New in Soft Tissue Tumor Pathology? Am J Surg Pathol (2021) 45(1):e1–e23. doi: 10.1097/PAS.0000000000001552
4. Barretina J, Taylor BS, Banerji S, Ramos AH, Lagos-Quintana M, DeCarolis PL, et al. Subtype-Specific Genomic Alterations Define New Targets for Soft-Tissue Sarcoma Therapy. Nat Genet (2010) 42(8):715–21. doi: 10.1038/ng.619
5. Nacev BA, Jones KB, Intlekofer AM, Yu JSE, Allis CD, Tap WD, et al. The Epigenomics of Sarcoma. Nat Rev Cancer (2020) 20(10):608–23. doi: 10.1038/s41568-020-0288-4
6. Delaunay S, Frye M. Rna Modifications Regulating Cell Fate in Cancer. Nat Cell Biol (2019) 21(5):552–9. doi: 10.1038/s41556-019-0319-0
7. Barbieri I, Kouzarides T. Role of Rna Modifications in Cancer. Nat Rev Cancer (2020) 20(6):303–22. doi: 10.1038/s41568-020-0253-2
8. Shi H, Chai P, Jia R, Fan X. Novel Insight Into the Regulatory Roles of Diverse Rna Modifications: Re-Defining the Bridge Between Transcription and Translation. Mol Cancer (2020) 19(1):78. doi: 10.1186/s12943-020-01194-6
9. Yang Y, Hsu PJ, Chen YS, Yang YG. Dynamic Transcriptomic M(6)a Decoration: Writers, Erasers, Readers and Functions in Rna Metabolism. Cell Res (2018) 28(6):616–24. doi: 10.1038/s41422-018-0040-8
10. Guo G, Pan K, Fang S, Ye L, Tong X, Wang Z, et al. Advances in Mrna 5-Methylcytosine Modifications: Detection, Effectors, Biological Functions, and Clinical Relevance. Mol Ther Nucleic Acids (2021) 26:575–93. doi: 10.1016/j.omtn.2021.08.020
11. Delaunay S, Rapino F, Tharun L, Zhou Z, Heukamp L, Termathe M, et al. Elp3 Links Trna Modification to Ires-Dependent Translation of Lef1 to Sustain Metastasis in Breast Cancer. J Exp Med (2016) 213(11):2503–23. doi: 10.1084/jem.20160397
12. Rapino F, Delaunay S, Rambow F, Zhou Z, Tharun L, De Tullio P, et al. Codon-Specific Translation Reprogramming Promotes Resistance to Targeted Therapy. Nature (2018) 558(7711):605–9. doi: 10.1038/s41586-018-0243-7
13. Desrosiers R, Friderici K, Rottman F. Identification of Methylated Nucleosides in Messenger Rna From Novikoff Hepatoma Cells. Proc Natl Acad Sci USA (1974) 71(10):3971–5. doi: 10.1073/pnas.71.10.3971
14. Dominissini D, Moshitch-Moshkovitz S, Schwartz S, Salmon-Divon M, Ungar L, Osenberg S, et al. Topology of the Human and Mouse M6a Rna Methylomes Revealed by M6a-Seq. Nature (2012) 485(7397):201–6. doi: 10.1038/nature11112
15. Meyer KD, Saletore Y, Zumbo P, Elemento O, Mason CE, Jaffrey SR. Comprehensive Analysis of Mrna Methylation Reveals Enrichment in 3' Utrs and Near Stop Codons. Cell (2012) 149(7):1635–46. doi: 10.1016/j.cell.2012.05.003
16. Jia G, Fu Y, Zhao X, Dai Q, Zheng G, Yang Y, et al. N6-Methyladenosine in Nuclear Rna Is a Major Substrate of the Obesity-Associated Fto. Nat Chem Biol (2011) 7(12):885–7. doi: 10.1038/nchembio.687
17. Zheng G, Dahl JA, Niu Y, Fedorcsak P, Huang CM, Li CJ, et al. Alkbh5 Is a Mammalian Rna Demethylase That Impacts Rna Metabolism and Mouse Fertility. Mol Cell (2013) 49(1):18–29. doi: 10.1016/j.molcel.2012.10.015
18. Huang H, Weng H, Sun W, Qin X, Shi H, Wu H, et al. Recognition of Rna N(6)-Methyladenosine by Igf2bp Proteins Enhances Mrna Stability and Translation. Nat Cell Biol (2018) 20(3):285–95. doi: 10.1038/s41556-018-0045-z
19. Zhang Z, Theler D, Kaminska KH, Hiller M, de la Grange P, Pudimat R, et al. The Yth Domain Is a Novel Rna Binding Domain. J Biol Chem (2010) 285(19):14701–10. doi: 10.1074/jbc.M110.104711
20. Lan Q, Liu PY, Haase J, Bell JL, Hüttelmaier S, Liu T. The Critical Role of Rna M(6)a Methylation in Cancer. Cancer Res (2019) 79(7):1285–92. doi: 10.1158/0008-5472.Can-18-2965
21. Edelheit S, Schwartz S, Mumbach MR, Wurtzel O, Sorek R. Transcriptome-Wide Mapping of 5-Methylcytidine Rna Modifications in Bacteria, Archaea, and Yeast Reveals M5c Within Archaeal Mrnas. PloS Genet (2013) 9(6):e1003602. doi: 10.1371/journal.pgen.1003602
22. Reid R, Greene PJ, Santi DV. Exposition of a Family of Rna M(5)C Methyltransferases From Searching Genomic and Proteomic Sequences. Nucleic Acids Res (1999) 27(15):3138–45. doi: 10.1093/nar/27.15.3138
23. Goll MG, Kirpekar F, Maggert KA, Yoder JA, Hsieh CL, Zhang X, et al. Methylation of Trnaasp by the DNA Methyltransferase Homolog Dnmt2. Sci (New York NY) (2006) 311(5759):395–8. doi: 10.1126/science.1120976
24. Yang X, Yang Y, Sun BF, Chen YS, Xu JW, Lai WY, et al. 5-Methylcytosine Promotes Mrna Export - Nsun2 as the Methyltransferase and Alyref as an M(5)C Reader. Cell Res (2017) 27(5):606–25. doi: 10.1038/cr.2017.55
25. Bohnsack KE, Hobartner C, Bohnsack MT. Eukaryotic 5-Methylcytosine (M(5)C) Rna Methyltransferases: Mechanisms, Cellular Functions, and Links to Disease. Genes (Basel) (2019) 10(2). doi: 10.3390/genes10020102
26. Xue C, Zhao Y, Li L. Advances in Rna Cytosine-5 Methylation: Detection, Regulatory Mechanisms, Biological Functions and Links to Cancer. biomark Res (2020) 8(1):43. doi: 10.1186/s40364-020-00225-0
27. Dominissini D, Nachtergaele S, Moshitch-Moshkovitz S, Peer E, Kol N, Ben-Haim MS, et al. The Dynamic N(1)-Methyladenosine Methylome in Eukaryotic Messenger Rna. Nature (2016) 530(7591):441–6. doi: 10.1038/nature16998
28. Li X, Xiong X, Wang K, Wang L, Shu X, Ma S, et al. Transcriptome-Wide Mapping Reveals Reversible and Dynamic N(1)-Methyladenosine Methylome. Nat Chem Biol (2016) 12(5):311–6. doi: 10.1038/nchembio.2040
29. Chujo T, Suzuki T. Trmt61b Is a Methyltransferase Responsible for 1-Methyladenosine at Position 58 of Human Mitochondrial Trnas. RNA (2012) 18(12):2269–76. doi: 10.1261/rna.035600.112
30. Ozanick S, Krecic A, Andersland J, Anderson JT. The Bipartite Structure of the Trna M1a58 Methyltransferase From S. Cerevisiae Is Conserved in Humans. RNA (2005) 11(8):1281–90. doi: 10.1261/rna.5040605
31. Vilardo E, Nachbagauer C, Buzet A, Taschner A, Holzmann J, Rossmanith W. A Subcomplex of Human Mitochondrial Rnase P Is a Bifunctional Methyltransferase–Extensive Moonlighting in Mitochondrial Trna Biogenesis. Nucleic Acids Res (2012) 40(22):11583–93. doi: 10.1093/nar/gks910
32. Dai X, Wang T, Gonzalez G, Wang Y. Identification of Yth Domain-Containing Proteins as the Readers for N1-Methyladenosine in Rna. Anal Chem (2018) 90(11):6380–4. doi: 10.1021/acs.analchem.8b01703
33. Liu F, Clark W, Luo G, Wang X, Fu Y, Wei J, et al. Alkbh1-Mediated Trna Demethylation Regulates Translation. Cell (2016) 167(7):1897. doi: 10.1016/j.cell.2016.11.045
34. Zhao Y, Zhao Q, Kaboli PJ, Shen J, Li M, Wu X, et al. M1a Regulated Genes Modulate Pi3k/Akt/Mtor and Erbb Pathways in Gastrointestinal Cancer. Trans Oncol (2019) 12(10):1323–33. doi: 10.1016/j.tranon.2019.06.007
35. Chen W, Feng P, Song X, Lv H, Lin H. Irna-M7g: Identifying N(7)-Methylguanosine Sites by Fusing Multiple Features. Mol Ther Nucleic Acids (2019) 18:269–74. doi: 10.1016/j.omtn.2019.08.022
36. Alexandrov A, Martzen MR, Phizicky EM. Two Proteins That Form a Complex Are Required for 7-Methylguanosine Modification of Yeast Trna. RNA (2002) 8(10):1253–66. doi: 10.1017/s1355838202024019
37. Tsukamoto T, Shibagaki Y, Niikura Y, Mizumoto K. Cloning and Characterization of Three Human Cdnas Encoding Mrna (Guanine-7-)-Methyltransferase, an Mrna Cap Methylase. Biochem Biophys Res Commun (1998) 251(1):27–34. doi: 10.1006/bbrc.1998.9402
38. Wang Q, Armenia J, Zhang C, Penson AV, Reznik E, Zhang L, et al. Unifying Cancer and Normal Rna Sequencing Data From Different Sources. Sci Data (2018) 5:180061. doi: 10.1038/sdata.2018.61
39. Goldman MJ, Craft B, Hastie M, Repečka K, McDade F, Kamath A, et al. Visualizing and Interpreting Cancer Genomics Data Via the Xena Platform. Nat Biotechnol (2020) 38(6):675–8. doi: 10.1038/s41587-020-0546-8
40. Gide TN, Quek C, Menzies AM, Tasker AT, Shang P, Holst J, et al. Distinct Immune Cell Populations Define Response to Anti-Pd-1 Monotherapy and Anti-Pd-1/Anti-Ctla-4 Combined Therapy. Cancer Cell (2019) 35(2):238–55.e6. doi: 10.1016/j.ccell.2019.01.003
41. Meyer KD, Jaffrey SR. Rethinking M(6)a Readers, Writers, and Erasers. Annu Rev Cell Dev Biol (2017) 33:319–42. doi: 10.1146/annurev-cellbio-100616-060758
42. Varshney D, Lombardi O, Schweikert G, Dunn S, Suska O, Cowling VH. Mrna Cap Methyltransferase, Rnmt-Ram, Promotes Rna Pol Ii-Dependent Transcription. Cell Rep (2018) 23(5):1530–42. doi: 10.1016/j.celrep.2018.04.004
43. Li X, Xiong X, Zhang M, Wang K, Chen Y, Zhou J, et al. Base-Resolution Mapping Reveals Distinct M(1)a Methylome in Nuclear- and Mitochondrial-Encoded Transcripts. Mol Cell (2017) 68(5):993–1005.e9. doi: 10.1016/j.molcel.2017.10.019
44. Wilkerson MD, Hayes DN. Consensusclusterplus: A Class Discovery Tool With Confidence Assessments and Item Tracking. Bioinformatics (2010) 26(12):1572–3. doi: 10.1093/bioinformatics/btq170
45. Benjamini Y, Hochberg Y. Controlling the False Discovery Rate: A Practical and Powerful Approach to Multiple Testing. J R Stat Soc Series B Stat Methodol (1995) 57(1):289–300. doi: 10.1111/j.2517-6161.1995.tb02031.x
46. Hänzelmann S, Castelo R, Guinney J. Gsva: Gene Set Variation Analysis for Microarray and Rna-Seq Data. BMC Bioinf (2013) 14:7. doi: 10.1186/1471-2105-14-7
47. Yu G, Wang LG, Han Y, He QY. Clusterprofiler: An R Package for Comparing Biological Themes Among Gene Clusters. OMICS (2012) 16(5):284–7. doi: 10.1089/omi.2011.0118
48. Bindea G, Mlecnik B, Tosolini M, Kirilovsky A, Waldner M, Obenauf AC, et al. Spatiotemporal Dynamics of Intratumoral Immune Cells Reveal the Immune Landscape in Human Cancer. Immunity (2013) 39(4):782–95. doi: 10.1016/j.immuni.2013.10.003
49. Mariathasan S, Turley SJ, Nickles D, Castiglioni A, Yuen K, Wang Y, et al. Tgfβ Attenuates Tumour Response to Pd-L1 Blockade by Contributing to Exclusion of T Cells. Nature (2018) 554(7693):544–8. doi: 10.1038/nature25501
50. Yoshihara K, Shahmoradgoli M, Martínez E, Vegesna R, Kim H, Torres-Garcia W, et al. Inferring Tumour Purity and Stromal and Immune Cell Admixture From Expression Data. Nat Commun (2013) 4:2612. doi: 10.1038/ncomms3612
51. Chen DS, Mellman I. Oncology Meets Immunology: The Cancer-Immunity Cycle. Immunity (2013) 39(1):1–10. doi: 10.1016/j.immuni.2013.07.012
52. Hu J, Yu A, Othmane B, Qiu D, Li H, Li C, et al. Siglec15 Shapes a Non-Inflamed Tumor Microenvironment and Predicts the Molecular Subtype in Bladder Cancer. Theranostics (2021) 11(7):3089–108. doi: 10.7150/thno.53649
53. Xu L, Deng C, Pang B, Zhang X, Liu W, Liao G, et al. Tip: A Web Server for Resolving Tumor Immunophenotype Profiling. Cancer Res (2018) 78(23):6575–80. doi: 10.1158/0008-5472.Can-18-0689
54. Chong W, Shang L, Liu J, Fang Z, Du F, Wu H, et al. M(6)a Regulator-Based Methylation Modification Patterns Characterized by Distinct Tumor Microenvironment Immune Profiles in Colon Cancer. Theranostics (2021) 11(5):2201–17. doi: 10.7150/thno.52717
55. Zhang B, Wu Q, Li B, Wang D, Wang L, Zhou YL. M(6)a Regulator-Mediated Methylation Modification Patterns and Tumor Microenvironment Infiltration Characterization in Gastric Cancer. Mol Cancer (2020) 19(1):53. doi: 10.1186/s12943-020-01170-0
56. Jerby-Arnon L, Neftel C, Shore ME, Weisman HR, Mathewson ND, McBride MJ, et al. Opposing Immune and Genetic Mechanisms Shape Oncogenic Programs in Synovial Sarcoma. Nat Med (2021) 27(2):289–300. doi: 10.1038/s41591-020-01212-6
57. Yang W, Soares J, Greninger P, Edelman EJ, Lightfoot H, Forbes S, et al. Genomics of Drug Sensitivity in Cancer (Gdsc): A Resource for Therapeutic Biomarker Discovery in Cancer Cells. Nucleic Acids Res (2013) 41(Database issue):D955–61. doi: 10.1093/nar/gks1111
58. Maeser D, Gruener RF, Huang RS. Oncopredict: An R Package for Predicting in Vivo or Cancer Patient Drug Response and Biomarkers From Cell Line Screening Data. Brief Bioinform (2021) 22(6). doi: 10.1093/bib/bbab260
59. Iorio F, Knijnenburg TA, Vis DJ, Bignell GR, Menden MP, Schubert M, et al. A Landscape of Pharmacogenomic Interactions in Cancer. Cell (2016) 166(3):740–54. doi: 10.1016/j.cell.2016.06.017
60. Qi L, Xu R, Wan L, Ren X, Zhang W, Zhang K, et al. Identification and Validation of a Novel Pyroptosis-Related Gene Signature for Prognosis Prediction in Soft Tissue Sarcoma. Front Genet (2021) 12. doi: 10.3389/fgene.2021.773373
61. Krzanowski WJ, Lai YT. A Criterion for Determining the Number of Groups in a Data Set Using Sum-Of-Squares Clustering. Biometrics (1988) 44:23–34. doi: 10.2307/2531893
62. Guo Y, Wang R, Li J, Song Y, Min J, Zhao T, et al. Comprehensive Analysis of M6a Rna Methylation Regulators and the Immune Microenvironment to Aid Immunotherapy in Pancreatic Cancer. Front Immunol (2021) 12. doi: 10.3389/fimmu.2021.769425
63. Zheng F, Du F, Qian H, Zhao J, Wang X, Yue J, et al. Expression and Clinical Prognostic Value of M6a Rna Methylation Modification in Breast Cancer. biomark Res (2021) 9(1):28. doi: 10.1186/s40364-021-00285-w
64. Saliba AE, Westermann AJ, Gorski SA, Vogel J. Single-Cell Rna-Seq: Advances and Future Challenges. Nucleic Acids Res (2014) 42(14):8845–60. doi: 10.1093/nar/gku555
65. Lin S, Choe J, Du P, Triboulet R, Gregory Richard I. The M6a Methyltransferase Mettl3 Promotes Translation in Human Cancer Cells. Mol Cell (2016) 62(3):335–45. doi: 10.1016/j.molcel.2016.03.021
66. Miao W, Chen J, Jia L, Ma J, Song D. The M6a Methyltransferase Mettl3 Promotes Osteosarcoma Progression by Regulating the M6a Level of Lef1. Biochem Biophys Res Commun (2019) 516(3):719–25. doi: 10.1016/j.bbrc.2019.06.128
67. Chen H, Yao J, Bao R, Dong Y, Zhang T, Du Y, et al. Cross-Talk of Four Types of Rna Modification Writers Defines Tumor Microenvironment and Pharmacogenomic Landscape in Colorectal Cancer. Mol Cancer (2021) 20(1):29. doi: 10.1186/s12943-021-01322-w
68. Sawh AN, Shafer MER, Su J-H, Zhuang X, Wang S, Mango SE. Lamina-Dependent Stretching and Unconventional Chromosome Compartments in Early C. Elegans Embryos. Mol Cell (2020) 78(1):96–111.e6. doi: 10.1016/j.molcel.2020.02.006
69. Prosperi MCF, Sahiner UM, Belgrave D, Sackesen C, Buchan IE, Simpson A, et al. Challenges in Identifying Asthma Subgroups Using Unsupervised Statistical Learning Techniques. Am J Respir Crit Care Med (2013) 188(11):1303–12. doi: 10.1164/rccm.201304-0694OC
70. Motz GT, Coukos G. The Parallel Lives of Angiogenesis and Immunosuppression: Cancer and Other Tales. Nat Rev Immunol (2011) 11(10):702–11. doi: 10.1038/nri3064
71. Voron T, Marcheteau E, Pernot S, Colussi O, Tartour E, Taieb J, et al. Control of the Immune Response by Pro-Angiogenic Factors. Front Oncol (2014) 4. doi: 10.3389/fonc.2014.00070
72. Kim SH, Li M, Trousil S, Zhang Y, Pasca di Magliano M, Swanson KD, et al. Phenformin Inhibits Myeloid-Derived Suppressor Cells and Enhances The Anti-Tumor Activity of Pd-1 Blockade In Melanoma. J Invest Dermatol (2017) 137(8):1740–8. doi: 10.1016/j.jid.2017.03.033
73. Zheng Q, Gan H, Yang F, Yao Y, Hao F, Hong L, et al. Cytoplasmic M1a Reader Ythdf3 Inhibits Trophoblast Invasion by Downregulation of M1a-Methylated Igf1r. Cell Discovery (2020) 6(1):12. doi: 10.1038/s41421-020-0144-4
74. Chen Y-S, Yang W-L, Zhao Y-L, Yang Y-G. Dynamic Transcriptomic M5c and Its Regulatory Role in Rna Processing. WIRE RNA (2021) 12(4):e1639. doi: 10.1002/wrna.1639
75. Enroth C, Poulsen LD, Iversen S, Kirpekar F, Albrechtsen A, Vinther J. Detection of Internal N7-Methylguanosine (M7g) Rna Modifications by Mutational Profiling Sequencing. Nucleic Acids Res (2019) 47(20):e126–e. doi: 10.1093/nar/gkz736
76. Samstein RM, Lee C-H, Shoushtari AN, Hellmann MD, Shen R, Janjigian YY, et al. Tumor Mutational Load Predicts Survival After Immunotherapy Across Multiple Cancer Types. Nat Genet (2019) 51(2):202–6. doi: 10.1038/s41588-018-0312-8
77. Riviere P, Goodman AM, Okamura R, Barkauskas DA, Whitchurch TJ, Lee S, et al. High Tumor Mutational Burden Correlates With Longer Survival in Immunotherapy-Naïve Patients With Diverse Cancers. Mol Cancer Ther (2020) 19(10):2139–45. doi: 10.1158/1535-7163
78. Li X, Ma S, Deng Y, Yi P, Yu J. Targeting the Rna M6a Modification for Cancer Immunotherapy. Mol Cancer (2022) 21(1):76. doi: 10.1186/s12943-022-01558-0
79. Roy DM, Walsh LA, Chan TA. Driver Mutations of Cancer Epigenomes. Protein Cell (2014) 5(4):265–96. doi: 10.1007/s13238-014-0031-6
80. Heaphy CM, de Wilde RF, Jiao Y, Klein AP, Edil BH, Shi C, et al. Altered Telomeres in Tumors With Atrx and Daxx Mutations. Science (2011) 333(6041):425. doi: 10.1126/science.1207313
81. Kannan K, Inagaki A, Silber J, Gorovets D, Zhang J, Kastenhuber ER, et al. Whole-Exome Sequencing Identifies Atrx Mutation as a Key Molecular Determinant in Lower-Grade Glioma. Oncotarget (2012) 3(10):1194–203. doi: 10.18632/oncotarget.689
82. Chen YT, Shen JY, Chen DP, Wu CF, Guo R, Zhang PP, et al. Identification of Cross-Talk Between M(6)a and 5mc Regulators Associated With Onco-Immunogenic Features and Prognosis Across 33 Cancer Types. J Hematol Oncol (2020) 13(1):22. doi: 10.1186/s13045-020-00854-w
83. Chen Y, Ling Z, Cai X, Xu Y, Lv Z, Man D, et al. Activation of Yap1 by N6-Methyladenosine-Modified Circcpsf6 Drives Malignancy in Hepatocellular Carcinoma. Cancer Res (2022) 82(4):599–614. doi: 10.1158/0008-5472.Can-21-1628
Keywords: RNA modification regulator, soft-tissue sarcoma, tumor microenvironment, immune infiltrate, drug sensitivity, immunotherapy
Citation: Qi L, Zhang W, Ren X, Xu R, Yang Z, Chen R, Tu C and Li Z (2022) Cross-Talk of Multiple Types of RNA Modification Regulators Uncovers the Tumor Microenvironment and Immune Infiltrates in Soft Tissue Sarcoma. Front. Immunol. 13:921223. doi: 10.3389/fimmu.2022.921223
Received: 15 April 2022; Accepted: 02 June 2022;
Published: 04 July 2022.
Edited by:
Guohui Wan, Sun Yat-sen University, ChinaCopyright © 2022 Qi, Zhang, Ren, Xu, Yang, Chen, Tu and Li. This is an open-access article distributed under the terms of the Creative Commons Attribution License (CC BY). The use, distribution or reproduction in other forums is permitted, provided the original author(s) and the copyright owner(s) are credited and that the original publication in this journal is cited, in accordance with accepted academic practice. No use, distribution or reproduction is permitted which does not comply with these terms.
*Correspondence: Zhihong Li, bGl6aGlob25nQGNzdS5lZHUuY24=; Chao Tu, dHVjaGFvQGNzdS5lZHUuY24=
Disclaimer: All claims expressed in this article are solely those of the authors and do not necessarily represent those of their affiliated organizations, or those of the publisher, the editors and the reviewers. Any product that may be evaluated in this article or claim that may be made by its manufacturer is not guaranteed or endorsed by the publisher.
Research integrity at Frontiers
Learn more about the work of our research integrity team to safeguard the quality of each article we publish.