- 1Department of Laboratory Medicine, Hallym University College of Medicine, Seoul, South Korea
- 2Department of Laboratory Medicine, Hallym University College of Medicine, Hangang Sacred Heart Hospital, Seoul, South Korea
Coronavirus disease 2019 (COVID-19) has been a pandemic for the past two years. Predicting patient prognosis is critical. Although immune checkpoints (ICs) were shown to be involved in severe acute respiratory syndrome coronavirus 2 (SARS-CoV-2) infection, quantitative studies of ICs in clinical practice are limited. In this study, various soluble ICs (sICs) and cytokine levels in patients with SARS-CoV-2 infection at different time points were compared between survivors and deaths; we also examined whether sICs are useful for predicting prognosis. sICs and cytokines were measured in serum samples from 38 patients diagnosed with COVID-19 in the first and second week post-diagnosis. All assays were performed by bead-based multiplexed immunoassay system using Luminex Bio-Plex 200 system. The correlation of sICs and cytokines with laboratory markers was evaluated, and the levels of sICs in survivors were compared with those in deaths. Among the sICs, the second-week levels of soluble cluster of differentiation (sCD27, p = 0.012), sCD40 (p< 0.001), cytotoxic T-lymphocyte-associated protein 4 (sCTLA-4, p< 0.001), herpes virus entry mediator (sHVEM, p = 0.026), and T-cell immunoglobulin and mucin-domain containing-3 (sTIM-3, p = 0.002) were significantly higher in deaths than in survivors. The levels of nine cytokines assessed in the second week of deaths were significantly higher than those in survivors. The sICs sCD27, sCD40, sCTLA-4, and sTIM-3 and cytokines chemokine CC motif ligand 2 (CCL2), GM-CSF, IL-10, and IL-8 showed significant positive correlations with the levels of C-reactive protein (CRP) and procalcitonin and were negatively correlated with the absolute lymphocyte count and platelet values. Increased levels of sICs including sCD27, sCD40, sCTLA-4, and sTIM-3 and cytokines were significant factors for poor prognosis. sICs, together with cytokines and inflammatory markers, may be useful as prognostic stratification markers in SARS-CoV-2-infected patients.
Introduction
The coronavirus disease 2019 (COVID-19) pandemic has continued for more than two years, highlighting the importance of disease diagnosis, evaluation of the patient’s status and prognosis, screening for severely ill patients, and implementing effective treatment methods. In this context, immune checkpoint (IC) molecules have emerged as important in the mechanism of severe acute respiratory syndrome coronavirus 2 (SARS-CoV-2) infection and its progression. Several review articles reported the potential of using ICs as biomarkers of viral infections, including SARS-COV-2 infection (1, 2). ICs are co-stimulatory and co-inhibitory signaling molecules expressed on immune cells and are involved in regulating T-cell activation. Once triggered, these molecules function in either the potent immune response by releasing proinflammatory mediators or by breaking the immune system, maintaining self-tolerance, and preventing immunopathology in the body. In patients with cancer or viral infections, the IC pathway causes T-cell dysfunction to affect immune escape (2–6). Additionally, IC-targeted treatments have been suggested as alternatives for SARS-CoV-2 infection (7–9).
Although ICs were shown to play important roles in SARS-CoV-2 infection, few clinical studies have focused on quantitatively evaluating ICs in patients with SARS-CoV-2 infection. Additionally, the pattern of changes in IC values with disease progression and their prognostic significance remain unclear. Measurement of soluble ICs (sICs) and/or staining of tissue samples revealed a moderate to severe association between increased levels of ICs and disease severity in patients with COVID-19. However, few sICs, such as programmed cell death protein 1 (PD-L1), were measured, and overall survival (OS) was not analyzed because patients who died were excluded from the study (10, 11). Moreover, the sequential changes in sICs during hospitalization are not well-understood (10), and the time point at which sICs were measured was ambiguous in most studies (11). The associations of sICs with common inflammatory markers and cytokines are unclear. Therefore, studies are needed to measure various ICs at different time points in patients with COVID-19.
This study was conducted to determine the importance of sICs as prognostic predictors in patients with SARS-COV-2 infection. The levels of sICs and cytokines in patients with SARS-CoV-2 infection were compared between survivors and deaths. In addition, the levels of sICs and cytokines in each patient were measured in the first and second weeks post-diagnosis to investigate their changes over time. Furthermore, different sICs were evaluated at varying time points to identify the ideal measurement time for determining the patient’s status and disease prognosis. Finally, the cytokines values and laboratory test results were obtained at the time of sIC measurements, and the relationship and prognostic effect of these markers were investigated through an integrated analysis of various prognostic factors.
Materials and methods
Study population
From September 2020 to July 2021, 38 patients diagnosed with COVID-19 and treated in the intensive care unit of Kangnam Sacred Heart Hospital were enrolled in the study. The inclusion criteria for this study were as follows: age > 20 years, confirmed for SARS-COV-2 infection, treated in the intensive care unit (ICU). Patients with insufficient quantities of residual serum samples were excluded. COVID-19 was diagnosed in patients with SARS-CoV-2 RNA using real-time reverse transcription-polymerase chain reaction from nasopharyngeal and/or throat swab samples. Residual serum samples were collected from these patients during the first and second weeks after the diagnosis of COVID-19. A total of 76 specimens were collected from 38 patients. An aliquot (1.0 mL) of each sample was transferred into microtubes and stored at -70°C prior to measuring the concentration of sICs and cytokines. The study was approved by the Institutional Review Board of Kangnam Sacred Heart Hospital of Hallym University, Seoul, Korea (No. 2020-08-004-003). The requirement for informed consent for this study was waived by the institutional review board because the anonymity of personal information was maintained.
Quantification of sIC regulators and cytokines
sICs in the serum were quantified using Milliplex Human Immuno-Oncology Checkpoint Protein Premixed 17-plex Panel 1 (Millipore, Billerica, MA, USA). The sIC panel included soluble cluster of differentiation 27 (sCD27), sCD40, sCD80/B7-1, sCD86/B7-1, soluble B-lymphocyte and T-lymphocyte attenuator (sICOS), soluble cytotoxic T-lymphocyte-associated protein 4 (sCTLA-4), sBTLA, soluble B-lymphocyte and T-lymphocyte attenuator (sBTLA), soluble herpes virus entry mediator (sHVEM), soluble programmed cell death protein 1 (sPD-1), soluble programmed death-ligand 1 (sPD-L1), sPD-L2, sPD-L3, soluble glucocorticoid-induced TNFR-related protein (sGITR), soluble ligand for receptor TNFRSF18/AITR/GITR (sGITRL), soluble Toll-like receptor 2 (sTLR-2), soluble T-cell immunoglobulin and mucin-domain containing-3 (sTIM-3), and lymphocyte activation gene 3 (sLAG-3). Cytokines levels were measured using a Human XL Cytokine Luminex Performance Panel Premixed Kit (R&D Systems, Minneapolis, MN, USA). The cytokine panel included chemokine CC motif ligand 2 (CCL2), CCL3, CCL4, C-X-C motif chemokine ligand (CXCL10), granulocyte-macrophage colony-stimulating factor (GM-CSF), interferon (IFN)-α, IFN-γ, interleukin (IL)-10, IL-12p70, IL-13, IL-17A, IL-1α, IL-1β, IL-4, IL-6, IL-8, and tumor necrosis factor-α (TNF-α). All assays were conducted using Luminex-based multiplex technology according to the manufacturer’s protocols on a Luminex 200 Bio-Plex instrument (Bio-Rad, Hercules, CA, USA).
Laboratory assessments
Clinical data were retrospectively investigated using medical records, and quantitative values were measured at the same time as sICs/cytokines estimation in the first and second weeks. Blood urine nitrogen (BUN), creatinine, lactate dehydrogenase (LD), procalcitonin, and C-reactive protein (CRP) levels were determined using an Atellica IM (Siemens Healthcare Diagnostics Manufacturing Ltd., Munich, Germany). The hemoglobin, platelet, total white blood cell (WBC), absolute neutrophil count (ANC), and lymphocyte counts were obtained using an ADVIA 2120i (Siemens Healthcare Diagnostics Manufacturing Ltd.). SARS-CoV-2 RNA was extracted using the MagNa Pure 96 System (Roche Diagnostics, Basel, Switzerland) and subjected to a real-time polymerase chain reaction using the STANDARD M nCoV Real-Time Detection kit (SD Biosensor, Gyeonggi, South Korea) and Bio–Rad CFX96 analyzer (Bio–Rad Laboratories) for quantification.
Statistical analyses
The clinical characteristics, sICs levels, and cytokines levels of the enrolled patients were compared between survivors and deaths. Moreover, differences between the survivor and death groups were compared based on the values measured in the first and second weeks. Mann–Whitney U test was used to evaluate statistical significance. Pearson’s correlation coefficient was used to analyze the correlation between laboratory markers and various sIC and cytokines levels. The degree of correlation was considered weak for values of 0.10 ≤ r< 0.30, moderate for values of 0.30 ≤ r<0.50, and strong for values of r ≥ 0.50, as previously described (12). The Cox proportional hazards model was used to evaluate the prognostic impact of laboratory tests and sIC and cytokines levels on OS. Age, sex, ANC, and underlying diseases as factors that may influence prognosis were adjusted through multivariable Cox analysis (13). Cumulative OS curves for the cluster groups were calculated using the Kaplan–Meier method and compared using the log-rank test. The cutoff for continuous values was estimated using the Youden index. Pearson’s correlation coefficient and OS were analyzed using the values measured in the second week for all patients. Several factors affecting the survival rate of patients with SARS-CoV-2 infection were identified and classified using the K-means clustering method. Differences were considered statistically significant at p< 0.05. A corrected p-value using Bonferroni-adjustment was applied with the number of comparisons made (14). All statistical analyses were conducted using the statistical R-project program (version 3.6.2), PASW statistics version 18 (SPSS, Inc., Chicago, IL, USA), and MedCalc version 12.0 (MedCalc Software, Mariakerke, Belgium).
Results
Patient characteristics, sIC regulators, and cytokines in patients with COVID-19
Among the 38 patients with SARS-CoV-2 infection, 23 survivors and 15 deaths were included in the study. The median ages of the survivors and deaths were 67.0 and 76.0 years, respectively (p = 0.018). Among the survivors, creatinine (p< 0.001), CRP (p< 0.001), and sPD-L2 (p = 0.040) levels were significantly lower in the second week than in the first week. And, sCD80 (p = 0.029), sGITRL (p = 0.039), and sICOS (p = 0.018) levels were significantly higher in the second week than in the first week (Table 1). After adjusted by Bonferroni’s correction, creatinine (p = 0.019) and CRP (p = 0.005) remained for statistically significant factors. Among the deaths, the total WBC (p = 0.004), ANC (p = 0.002), and sCD40 (p = 0.024) levels were significantly higher in the second week than in the first week. The above items were not statistically significant after adjusted by Bonferroni’s correction. When comparing the survivors with deaths, WBC (p = 0.004), BUN (p = 0.008), LD (p< 0.001), and CRP (p< 0.001) levels measured in the second week were significantly higher in survivors than in those who died. Of the sICs, sCD27 (p = 0.012), sCD40 (p< 0.001), sCTLA-4 (p< 0.001), sHVEM (p = 0.026), and sTIM-3 (p = 0.002) assessed in the second week were significantly increased in deaths compared to in survivors. In contrast, sLAG-3 (p = 0.038) levels decreased in the deaths. After adjusted by Bonferroni’s correction, CRP (p = 0.004), sCD40 (p<0.001), and CTLA-4 (p = 0.024) showed stastistical significance (Table 1). The differences in sex, Ct values for SARS-CoV-2 real-time reverse transcription-polymerase chain reaction tests, lymphocyte count, hemoglobin, and procalcitonin levels between the survivors and deaths and/or first and second weeks were not significant (Table 1). The levels of nine cytokines (CCL2, CCL4, CXCL10, GM-CSF, IL-10, IL-17A, IL-6, IL-8, and TNF-α) in deaths were significantly increased in the second week compared to those in survivors. CCL2 (p< 0.001), CXCL10 (p = 0.002), GM-CSF (p = 0.008), IL-10 (p = 0.007), and IL-8 (p = 0.009) showed stastistical significance after adjusted with Bonferroni’s correction. Among the survivals, after adjusted by Bonferroni’s correction, CXCL10 (p< 0.001), GM-CSF (p = 0.002), and IL-10 (p = 0.012) levels were significantly decreased in the second week compared to those in the first week (Table 2).
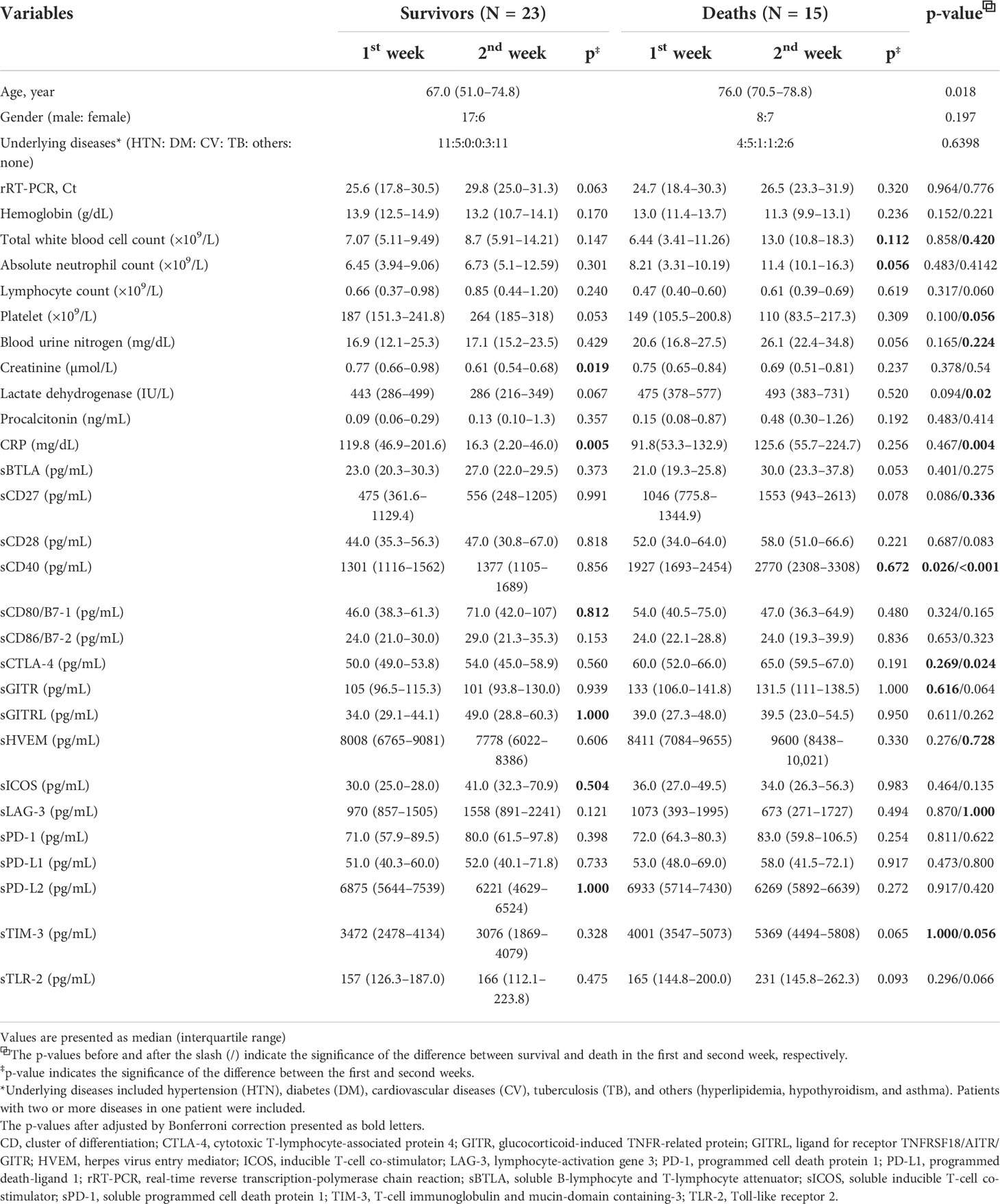
Table 1 Characteristics and levels of soluble type immune checkpoint regulators in survivors and deaths with SARS-CoV-2 infection.
Correlation of laboratory test markers with cytokines and sIC regulators
Inflammatory markers in patients infected with SARS-CoV-2 were positively correlated with the levels of some sICs and cytokines. The levels of sCD27 (r = 0.452, p = 0.006), sCD40 (r = 0.649, p< 0.001), sCTLA-4 (r = 0.452, p = 0.006), sTIM-3 (r = 0.498, p = 0.002), soluble Toll-like receptor-2 (r = 0.468, p = 0.004), CXCL10 (r = 0.495, p = 0.002), GM-CSF (r = 0.544, p = 0.0019), and IL-10 (r = 0.395, p = 0.031) were positively correlated with CRP levels (Figure 1). In contrast, LAG-3 levels were negatively correlated with CRP levels (r = -0.387, p = 0.020). In addition, sCD27 (r = 0.762, p = 0.006) and sCD40 (r = 0.643, p = 0.033) levels were positively correlated with procalcitonin. Fourteen sICs and 2 cytokines were positively correlated with the BUN and/or creatinine levels. Analysis of the CBC showed that sICs, including sCD27 (r = -0.416, p = 0.009), sCD28 (r = -0.352, p = 0.03), sCD40 (r = -0.367, p = 0.023), sHVEM (r = -0.345, p = 0.034), and sTIM-3 (r = -0.572, p< 0.001), were negatively correlated with the lymphocyte count. In addition, sCD27 (r = -0.471, p = 0.003), sCD28 (r = -0.327, p = 0.045), sCD40 (r = -0.511, p = 0.001), sHVEM (r = -0.392, p = 0.0154), sTIM-3 (r = -0.655, p< 0.001), CCL2 (r = -0.327, p = 0.045), CTLA4 (r = -0.457, p = 0.004), GM-CSF (r = -0.451, p = 0.01), IL-10 (r = -0.444, p = 0.011), and IL-8 (r = -0.399, p = 0.013) were negatively correlated with the platelet count. sCD40 was positively correlated with the total WBC count (r = 0.341, p = 0.036) and ANC (r = 0.346, p = 0.034). sLAG-3 expression was positively correlated with the platelet count (r = 0.584, p< 0.001) (Figure 1).
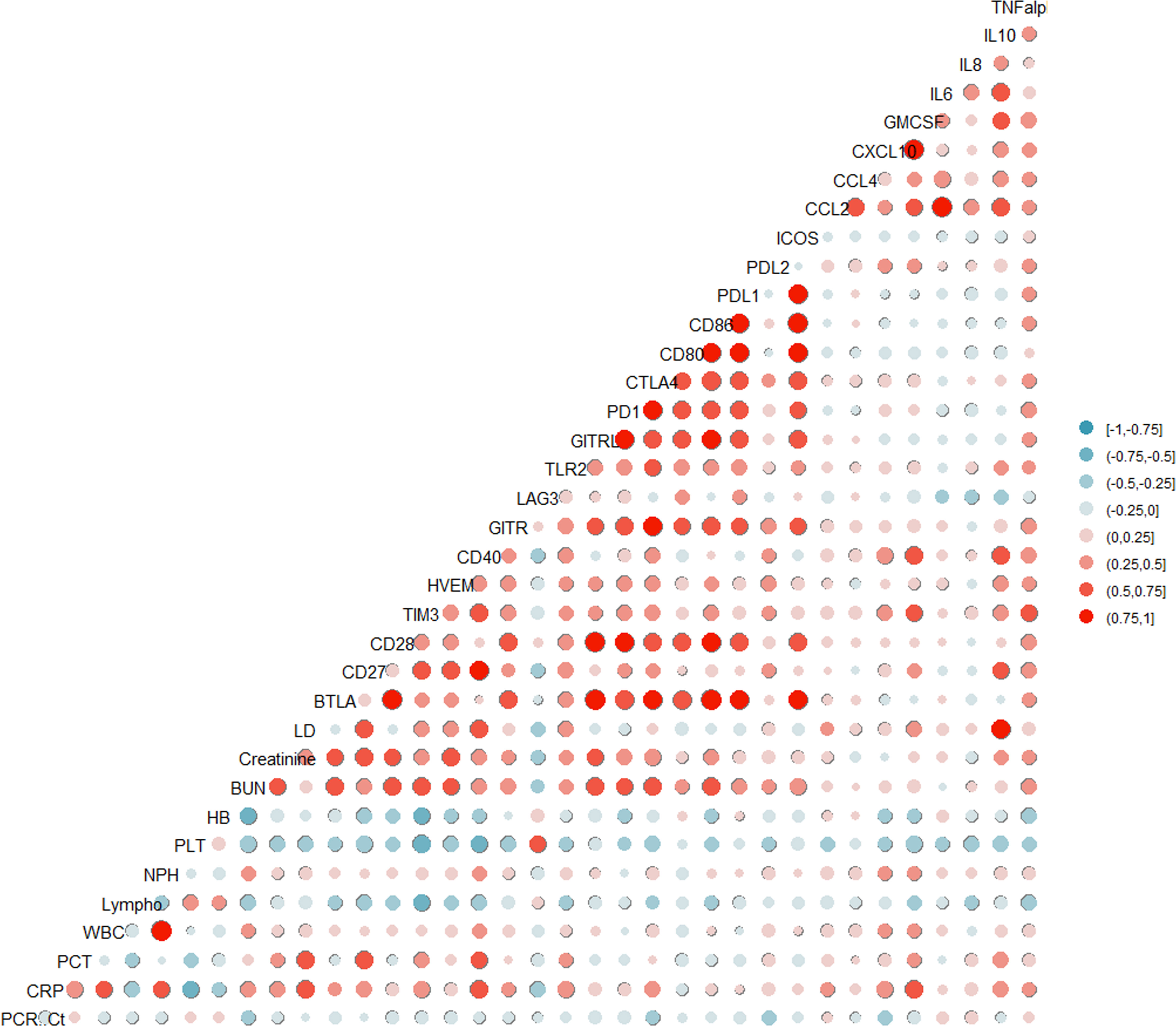
Figure 1 Pairwise association between clinical laboratory markers with soluble type immune checkpoint (IC) regulators and cytokines.
Prognostic impact of IC regulators and cytokines
Univariate analysis revealed that increased levels of sICs, including sCD27, sCD40, sTIM3, and sCTLA-4, and increased levels of cytokines, such as CCL2, CCL4, CXCL10, GM-CSF, IL-10, and IL-8, were associated with poor OS in patients with SARS-CoV-2 infection, whereas increased sLAG-3 levels were associated with a favorable prognosis. Increased age and total WBC, ANC, LD, and CRP levels were also associated with a poor prognosis. These sICs and cytokines were adjusted for age, sex, ANC, and comorbidities including hypertension, diabetes, cardiovascular disease, tuberculosis, and others (hyperlipidemia, hypothyroidism, and asthma) for multivariate analysis; all five sICs (sCD27, sCD40, sTIM3, sCTLA-4, and LAG-3) and four cytokines (CCL2, GM-CSF, IL-10, and IL-8) were significantly associated with OS. Increased LD and CRP levels were also associated with a poor OS in multivariate analysis (Supplemental Table 1). Using the Youden index, each factor was divided into two groups according to the prognosis. Increased level of sCD40 over 1804 pg/mL (Hazard ration (HR) = 6.36, p = 0.031), sCTLA-4 over 60 pg/mL (HR= 5.46, p=0.0097), sTIM-3 over 4752 pg/mL (HR= 5.13, p=0.014), CCL2 over 344.26 pg/mL (HR= 23.6, p=0.006), CXCL10 over 157.54 pg/mL (HR= 14.221, p=0.015), GM-CSF over 61.95 pg/mL (HR= 14.221, p=0.015), and IL-10 over 100.01 pg/mL (HR= 3.95, p=0.027) and decreased sLAG-3 not greater than 761 pg/mL (HR= 5.46, p=0.0097) were associated with poor OS (Table 3). Kaplan–Meier plots of patients with increased or decreased sICs and cytokine levels, classified using the Youden index, are shown in Figure 2. Patients with increased levels of sTIM3 (p< 0.0001), sCTLA4 (p< 0.0001), CCL4 (p = 0.035), GM-CSF (p = 0.0002), and IL-10 (p = 0.0001) exhibited a significantly poor prognosis, and those with decreased sLAG (p = 0.0003) levels showed a significantly poor prognosis. Additionally, increased levels of sCD27 (>355 pg/mL, p = 0.0113), sCD40 (>1804 pg/mL, p< 0.0001), CCL-2 (>344.26 pg/mL, p< 0.0001), and IL-8 (>21.86 pg/mL, p = 0.0005), with lesser than three survivors, were associated with poor prognosis.
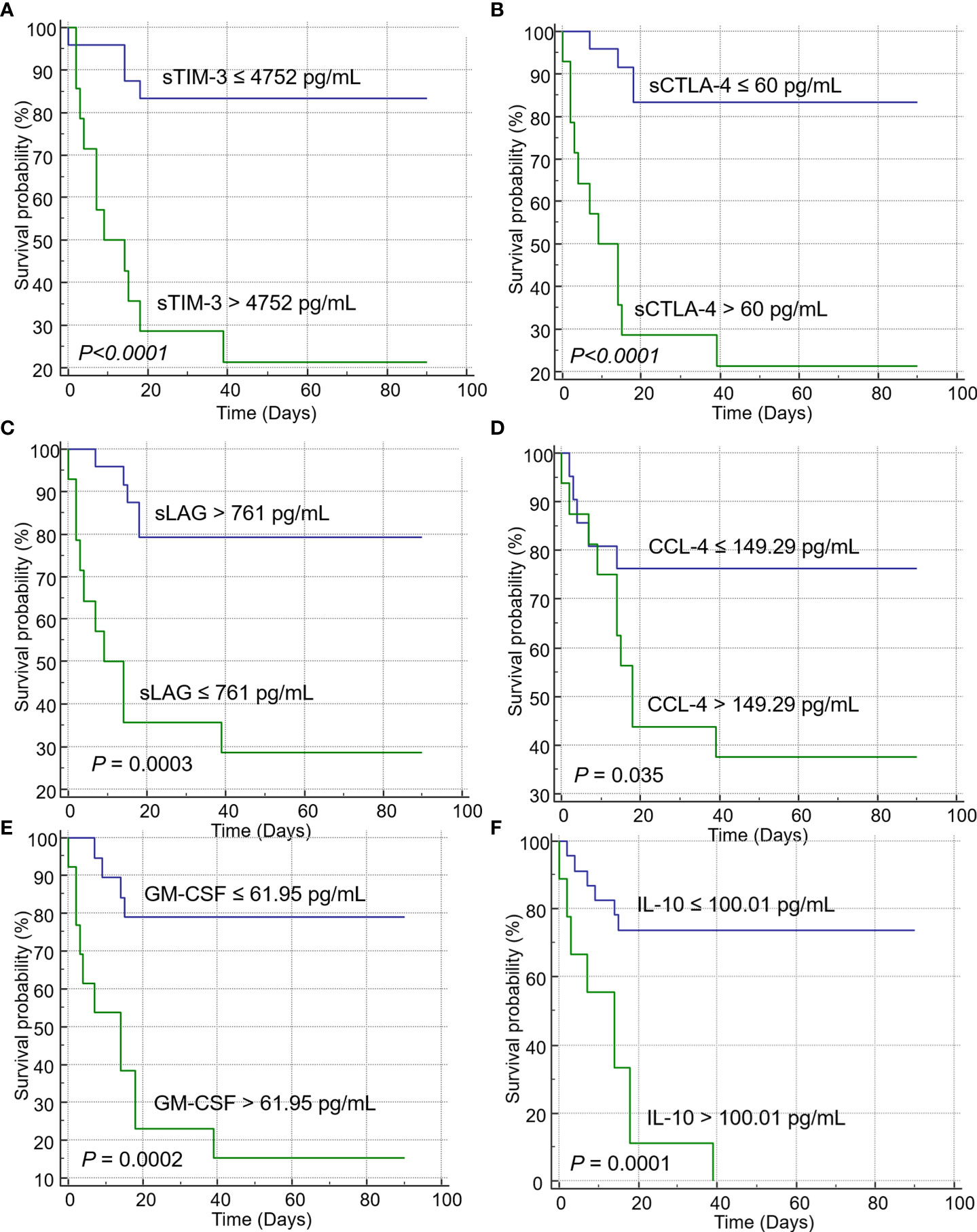
Figure 2 Kaplan–Meier curves showing the overall survival of patients with immune checkpoints (ICs) and cytokines. Increased levels of ICs such as (A) sTIM-3, and (B) sCTLA-4 were significantly associated with poor prognosis in the log-rank test. Decreased levels of (C) sLAG were correlated with poor prognosis. Increased levels of the cytokines (D) CCL-4, (E) GM-CSF, and (F) IL-10 were associated with poor prognosis.
OS of clusters classified based on K-means analysis
Various factors affecting the survival probability of patients with SARS-CoV-2 infection, identified in multivariate analysis, were classified using the K-means clustering method. Upon classification into two clusters, sCD27, sTIM3, sCD40, sCTLA-4, LD, CRP, CXCL10, and GM-CSF were identified as significant clustering factors (Table 4, Figure 3A). Cluster 2, which included increased levels of sICs and cytokines, along with the laboratory results listed above, was associated with poor prognosis (p< 0.001, Figure 3B). In this group, the clustering centers for sCD27, sTIM-3, sCD40, and sCTLA-4 were 2967.4, 5973.2, 3361.1, and 75.3 pg/mL, respectively. The clustering centers of significant cytokines such as CXCL10 and GM-CSF in the cluster 2 group were 637.05 and 102 pg/mL, respectively. In contrast, cluster 1, which showed decreased levels of sICs such as sCD27 (clustering center 818.9 pg/mL), sTIM-3 (3208.2 pg/mL), sCD40 (1558.2 pg/mL), and sCTLA-4 (54.2 pg/mL), and cytokines including CXCL10 (clustering center 272.44 pg/mL) and GM-CSF (55 pg/mL) showed a favorable prognosis (p< 0.001, Figure 3B).
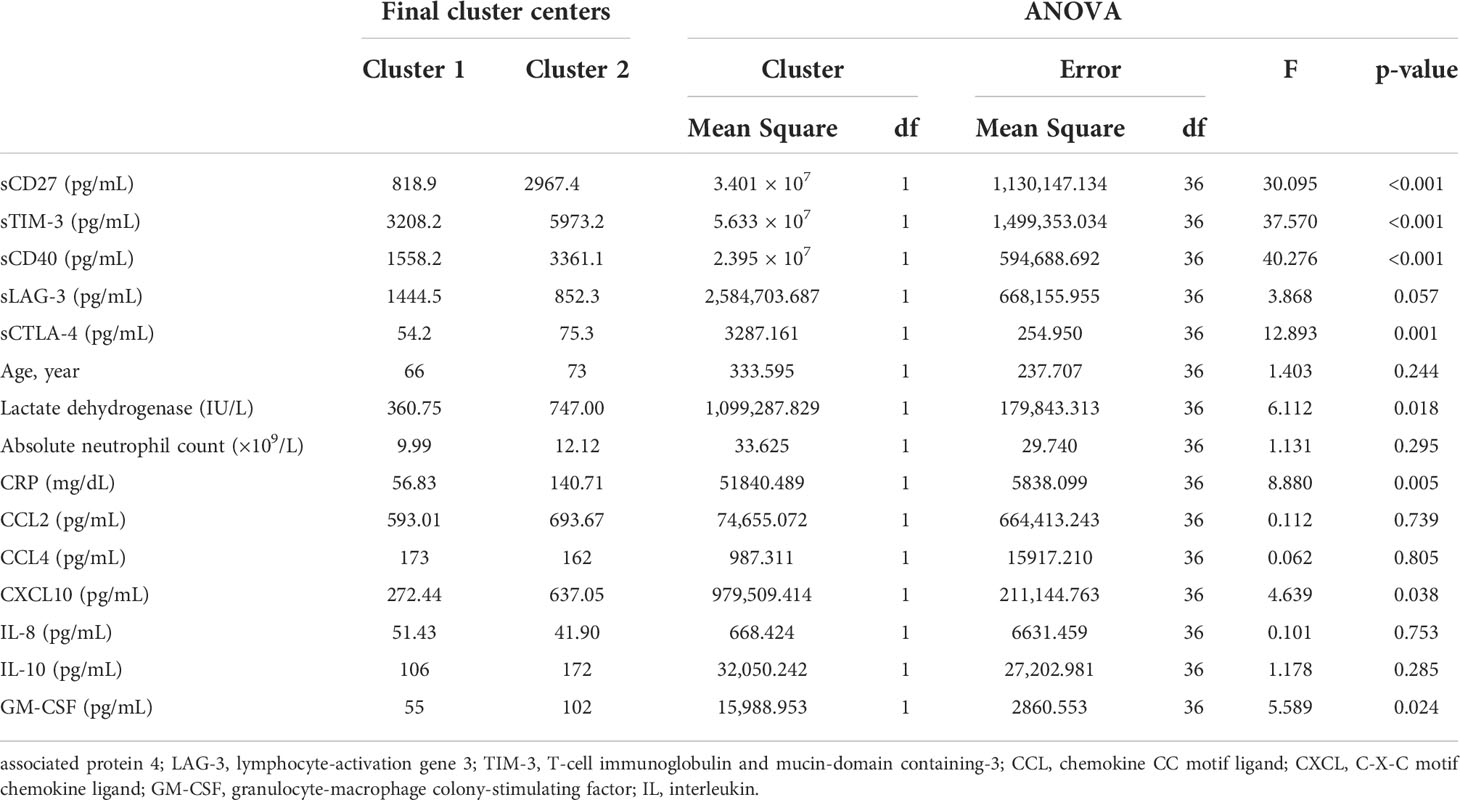
Table 4 Final cluster centers for variables after K-means analysis and their significance of clustering.
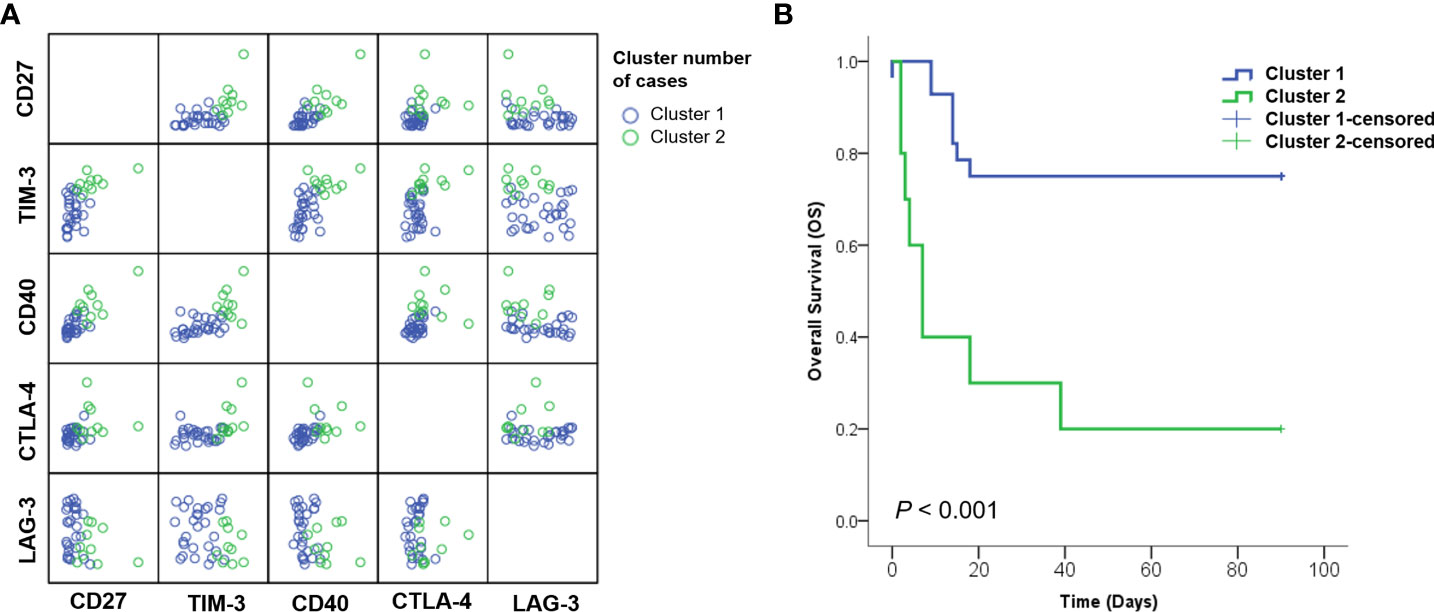
Figure 3 Analysis of patients subdivided into clustering groups. (A) Distribution of patients with SARS-CoV-2 classified according to K-means clustering methods. (B) Kaplan–Meier curves for overall survival (OS) showed that cluster 2 group with increased sICs, including sCD27, sCD40, sTIM3, and sCTLA-4; cytokines, including CXCL-10 and GM-CSF; and laboratory markers, including CRP and LD exhibited significantly poor prognosis than cluster 1.
Discussion
We found that increased sICs, such as sCD40, sCTLA-4, and sTIM-3, and increased cytokines levels, including CCL2, GM-CSF, and IL-10 were significantly poor prognostic factors. These values were significantly correlated with inflammatory markers, such as CRP and procalcitonin, and negatively correlated with the absolute lymphocyte count and platelet count. Cluster analysis of existing laboratory markers with significant sICs and cytokines revealed that patients with higher values of sCD27, sCD40, sCTLA-4, sTIM-3, LD, CRP, CXCL-10, and GM-CSF had a poor prognosis.
We measured the levels of various sICs and cytokines and followed up with each patient at the first and second weeks post-diagnosis. In most previous studies, the days on which sICs or cytokine values were estimated were inaccurate, and the values were measured in the first week of symptom onset (10, 11, 15). Sequential measurement of ICs and cytokine values revealed trends in the changes in IC and cytokine levels and the most favorable measurement time for prognosis prediction. The survivor group showed a significant decrease in the levels of CRP, creatinine and cytokines, such as CXCL10, GM-CSF, and IL-10 in the second week compared with those in the first week. In contrast, in the non-survival group, there was no significant decrease in the levels of laboratory markers, cytokines, or sICs in the first and second weeks. Although no significant differences were observed between the survivor and death groups in the first week, the levels of most markers showed a significant increase in the death group in the second week. Among the laboratory markers, LD, and CRP, and sICs, such as sCD40, and sCTLA-4, and some cytokines (5/11) were significantly higher in the death group than in the survivor group.
ICs play important roles in implanting immune responses that trigger effector functions in various immune cells (3). Although sICs significantly impact viral infection (2, 3, 16), various sICs in patients with SARS-COV-2 infection have not been extensively investigated. Kong et al. recently measured 14 sICs in patients with COVID-19 within three days of hospitalization (10), which showed that sIDO, s4-1BB, sTIM-3, and sCD27 were predictive biomarkers of disease severity. The results showing that sTIM-3 and sCD27 are poor prognostic factors agree with those of the present study. In contrast, we observed an effect on OS by including a large number of deceased patients and by measuring the values at both the first and second weeks; the values measured in the second week were more meaningful than those determined in the first week. Moreover, sCD40 and sCTLA4, which were not included or showed low significance in the above study, were significantly related to OS.
Most studies of sICs in patients with COVID-19 demonstrated the implications of PD-1 or PD-L1 (1, 8, 11, 17). Sabbatino et al. showed that serum PD-L1 levels are prognostic markers in patients with COVID-19 and that PD-L1 dysregulation is associated with COVID-19 pathogenesis (11). However, in our study, the median values of PD-1 and PD-L1 in non-surviving patients were higher than those in surviving patients; however, the difference was not significant. This can be explained by the small sample size of this study. In addition, in a previous study, comparative analysis was performed with healthy controls or non-intensive care unit patients (17), whereas we compared the survival and non-survival of patients with SARS-CoV-2 infection. In another study, the difference between discharged and deceased patients showed a higher p-value than the difference between healthy and discharged patients (11). The low significance of PD-1 and PD-L1 in our study suggests that sICs other than PD-1 and PD-L1 are more useful for predicting the prognosis of SARS-CoV-2-infected patients.
The impact of other sICs has not been widely reported. According to previous studies other sICs, such as CD27, CD40, and T cell exhaustion makers including TIM-3 have been reported to play significant roles in immune-mediated infection control in viral infections. As a member of the TNF superfamily, CD27 includes both costimulatory and apoptosis-inducing molecules, and its stimulation promotes natural killer/T cell survival and effector functions, which are required for the proliferation of various viruses (18–20). In addition, CD40 is important in the IFN-I response, parasitemia control, and host survival (21), and its signaling in macrophages inhibit acute viral replication at the early stages of infection (22). In SARS-CoV-2 infection, CD40 has been used as a subunit vaccine targeting viral antigens to CD40-expressing antigen-presenting cells (23). The newly developed vaccine significantly improved immunity in convalescent macaques, resulting in a reduction in viral load following re-exposure to the virus to levels that may avoid secondary transmission. Finally, as an indicator of CD8+ T cell exhaustion, both PD-1 and TIM-3, CTLA-4, and LAG-3 may be associated with the disease severity of COVID-19. SARS-CoV-2 can lead to limited T-cell function as a result of T-cell exhaustion during long-term infection, which is related to overexpression of immune-inhibitory factors (17, 24). The Gal-9/TIM-3 axis has also been reported to be critical for stratifying patients with SARS-CoV-2 infection with poor prognosis through serial measurements of two patients with COVID-19 (25). In our study, sICs, such as sCD27, sCD40, sCTLA-4, sHVEM, and sTIM-3, but not PD-1 or PD-L1, were significant prognostic factors in patients with SARS-CoV-2 infection. The roles and mechanisms of these sICs in prognosis require further analysis. Particularly, soluble ICs are easy to measure, and serial confirmation of the patient’s status is possible; thus, this approach can be applied in clinical practice.
In this study, various cytokines were measured together with sICs, and clustering analysis was performed by integrating these measured values. Many studies have reported a relationship between the mechanism and prognosis of cytokines in patients with COVID-19 (15, 26–30). However, few studies have focused on the correlation between cytokines and sICs or analyzed them in an integrated manner. In this study, the prognosis-related cytokines, sICs, and clinical laboratory test results were highly correlated. In addition, dividing the patient cluster by integrating various prognostic factors can predict patient mortality. Integrated analysis of various sICs and clinical data can help to accurately determine a patient’s status and auxiliary markers can be used to predict the prognosis of patients with SARS-CoV-2 infection.
One limitation of this study is that only sICs were measured. Further studies are needed to evaluate the levels of IC RNA expression in tissue cultures or tissue biopsy samples in vitro. Second, some cytokines could not be analyzed because their levels in patient samples were below the limit of detection. Patients whose serum samples could not be quantified because their cytokines, such as IFN-γ and IL-4, were below the limit of detection were excluded from analysis. This may vary from the results of other studies on cytokines, which is important for prognosis. Third, a comparison with healthy controls was not performed. In a follow-up study, the differences between the SARS-CoV-2-infected group and the healthy control group must be compared and the characteristics of patients with mild symptoms should be analyzed. In addition, during the study period, the vaccination rate in Koreans was 37.4, and 20 out of 38 study participants (52.6%) were sampled after the start date of vaccination against COVID-19. The number of vaccinated patients that can be inferred from this study was less than half and it was difficult to demonstrate the relationship between the status of vaccination and its impact on sICs through this study. Further studies investigating the association with vaccination state would be required.
With the progression of COVID-19, the lack of beds for patients with severe SARS-CoV-2 infection has emerged as an important concern in many countries. It is important to screen patients with COVID-19, predict disease prognosis, identify patients with severe disease, and effectively implement intensive care. Therefore, the markers identified in this study can be used to predict disease severity in patients with SARS-CoV-2 infection. Various sICs such as sCD27, sCD40, sCTLA-4, and sTIM-3 are significant independent prognostic factors and are helpful in prognosis prediction when they are analyzed together with the cytokines and inflammatory markers currently used in clinical practice.
Data availability statement
The datasets presented in this study can be found in online repositories. The names of the repository/repositories and accession number(s) can be found below: The dataset analyzed in this study can be found in [HARVARD Dataverse] [https://doi.org/10.7910/DVN/TAS2PH].
Ethics statement
The study was approved by the Institutional Review Board of the Kangnam Sacred Heart Hospital of Hallym University, Seoul, Korea (No. 2020-08-004-003). The ethics committee waived the requirement of written informed consent for participation.
Author contributions
NL and SJ designed the study; WS supervised the manuscript writing and participated in editing; NL collected the data, performed the statistical analysis, and wrote the manuscript; SJ and KJ participated in manuscript editing; M-JP reviewed the statistical analysis; and all authors reviewed and approved the manuscript.
Funding
This research was funded by a National Research Foundation of Korea (NRF) grant (grant number NRF-2022R1A2C1003503).
Acknowledgments
The authors appreciate medical technicians for collecting samples to perform the experiments.
Conflict of interest
The authors declare that the research was conducted in the absence of any commercial or financial relationships that could be construed as a potential conflict of interest.
Publisher’s note
All claims expressed in this article are solely those of the authors and do not necessarily represent those of their affiliated organizations, or those of the publisher, the editors and the reviewers. Any product that may be evaluated in this article, or claim that may be made by its manufacturer, is not guaranteed or endorsed by the publisher.
Supplementary material
The Supplementary Material for this article can be found online at: https://www.frontiersin.org/articles/10.3389/fimmu.2022.903419/full#supplementary-material
References
1. Aghbash PS, Eslami N, Shamekh A, Entezari-Maleki T, Baghi HB. SARS-CoV-2 infection: The role of PD-1/PD-L1 and CTLA-4 axis. Life Sci (2021) 270:119124. doi: 10.1016/j.lfs.2021.119124
2. Cai H, Liu G, Zhong J, Zheng K, Xiao H, Li C, et al. Immune checkpoints in viral infections. Viruses (2020) 12:1051. doi: 10.3390/v12091051
3. Joseph J, Rahmani B, Cole Y, Puttagunta N, Lin E, Khan ZK, et al. Can soluble immune checkpoint molecules on exosomes mediate inflammation? J Neuroimmune Pharmacol (2021) Epub ahead of print. doi: 10.1007/s11481-021-10018-3
4. Nagai S, Azuma M. The CD28-B7 family of co-signaling molecules. Adv Exp Med Biol (2019) 1189:25–51. doi: 10.1007/978-981-32-9717-3_2
5. Marin-Acevedo JA, Dholaria B, Soyano AE, Knutson KL, Chumsri S, Lou Y. Next generation of immune checkpoint therapy in cancer: new developments and challenges. J Hematol Oncol (2018) 11:39. doi: 10.1186/s13045-018-0582-8
6. Zahavi DJ, Weiner LM. Targeting multiple receptors to increase checkpoint blockade efficacy. Int J Mol Sci (2019) 20:158. doi: 10.3390/ijms20010158
7. Gatto L, Franceschi E, Nunno VD, Brandes AA. Potential protective and therapeutic role of immune checkpoint inhibitors against viral infections and COVID-19. Immunotherapy (2020) 12:1111–4. doi: 10.2217/imt-2020-0109
8. Awadasseid A, Yin Q, Wu Y, Zhang W. Potential protective role of the anti-PD-1 blockade against SARS-CoV-2 infection. BioMed Pharmacother (2021) 142:111957. doi: 10.1016/j.biopha.2021.111957
9. Gambichler T, Reuther J, Scheel CH, Becker JC. On the use of immune checkpoint inhibitors in patients with viral infections including COVID-19. J Immunother Cancer (2020) 8(2):e001145. doi: 10.1136/jitc-2020-001145
10. Kong Y, Wang Y, Wu X, Han J, Li G, Hua M, et al. Storm of soluble immune checkpoints associated with disease severity of COVID-19. Signal Transduct Target Ther (2020) 5:192. doi: 10.1038/s41392-020-00308-2
11. Sabbatino F, Conti V, Franci G, Sellitto C, Manzo V, Pagliano P, et al. PD-L1 dysregulation in COVID-19 patients. Front Immunol (2021) 12:695242. doi: 10.3389/fimmu.2021.695242
12. Parker RI, Hagan-Burke S. Useful effect size interpretations for single case research. Behav Ther (2007) 38:95–105. doi: 10.1016/j.beth.2006.05.002
13. Williamson EJ, Walker AJ, Bhaskaran K, Bacon S, Bates C, Morton CE, et al. Factors associated with COVID-19-related death using OpenSAFELY. Nature (2020) 584:430–6. doi: 10.1038/s41586-020-2521-4
14. Menyhart O, Weltz B, Győrffy B. MultipleTesting.com: A tool for life science researchers for multiple hypothesis testing correction. PloS One (2021) 16(6):e0245824. doi: 10.1371/journal.pone.0245824
15. Han H, Ma Q, Li C, Liu R, Zhao L, Wang W, et al. Profiling serum cytokines in COVID-19 patients reveals IL-6 and IL-10 are disease severity predictors. Emerg Microbes Infect (2020) 9:1123–30. doi: 10.1080/22221751.2020.1770129
16. Dyck L, Mills KHG. Immune checkpoints and their inhibition in cancer and infectious diseases. Eur J Immunol (2017) 47:765–79. doi: 10.1002/eji.201646875
17. Diao B, Wang C, Tan Y, Chen X, Liu Y, Ning L, et al. Reduction and functional exhaustion of T cells in patients with coronavirus disease 2019 (COVID-19). Front Immunol (2020) 11:827. doi: 10.3389/fimmu.2020.00827
18. Bullock TN. Stimulating CD27 to quantitatively and qualitatively shape adaptive immunity to cancer. Curr Opin Immunol (2017) 45:82–8. doi: 10.1016/j.coi.2017.02.001
19. Remedios KA, Meyer L, Zirak B, Pauli ML, Truong H-A, Boda D, et al. CD27 promotes CD4(+) effector T cell survival in response to tissue self-antigen. J Immunol (Baltimore Md: 1950) (2019) 203:639–46. doi: 10.4049/jimmunol.1900288
20. Deng Y, Chatterjee B, Zens K, Zdimerova H, Müller A, Schuhmachers P, et al. CD27 is required for protective lytic EBV antigen-specific CD8+ T-cell expansion. Blood (2021) 137:3225–36. doi: 10.1182/blood.2020009482
21. Yao X, Wu J, Lin M, Sun W, He X, Gowda C, et al. Increased CD40 expression enhances early STING-mediated type I interferon response and host survival in a rodent malaria model. PloS Pathog (2016) 12:e1005930. doi: 10.1371/journal.ppat.1005930
22. Rogers KJ, Shtanko O, Stunz LL, Mallinger LN, Arkee T, Schmidt ME, et al. Frontline science: CD40 signaling restricts RNA virus replication in mϕs, leading to rapid innate immune control of acute virus infection. J Leukoc Biol (2021) 109:309–25. doi: 10.1002/JLB.4HI0420-285RR
23. Marlin R, Godot V, Cardinaud S, Galhaut M, Coleon S, Zurawski S, et al. Targeting SARS-CoV-2 receptor-binding domain to cells expressing CD40 improves protection to infection in convalescent macaques. Nat Commun (2021) 12:5215. doi: 10.1038/s41467-021-25382-0
24. Wherry EJ, Kurachi M. Molecular and cellular insights into T cell exhaustion. Nat Rev Immunol (2015) 15:486–99. doi: 10.1038/nri3862
25. Martín-Quirós A, Maroun-Eid C, Avendaño-Ortiz J, Lozano-Rodríguez R, Valentín Quiroga J, Terrón V, et al. Potential role of the galectin-9/TIM-3 axis in the disparate progression of SARS-CoV-2 in a married couple: a case report. BioMed Hub (2021) 6:48–58. doi: 10.1159/000514727
26. Costela-Ruiz VJ, Illescas-Montes R, Puerta-Puerta JM, Ruiz C, Melguizo-Rodríguez L. SARS-CoV-2 infection: The role of cytokines in COVID-19 disease. Cytokine Growth Factor Rev (2020) 54:62–75. doi: 10.1016/j.cytogfr.2020.06.001
27. Coperchini F, Chiovato L, Ricci G, Croce L, Magri F, Rotondi M. The cytokine storm in COVID-19: Further advances in our understanding the role of specific chemokines involved. Cytokine Growth Factor Rev (2021) 58:82–91. doi: 10.1016/j.cytogfr.2020.12.005
28. Huang L, Zhao X, Qi Y, Li H, Ye G, Liu Y, et al. Sepsis-associated severe interleukin-6 storm in critical coronavirus disease 2019. Cell Mol Immunol (2020) 17:1092–4. doi: 10.1038/s41423-020-00522-6
29. Mahmoudi S, Rezaei M, Mansouri N, Marjani M, Mansouri D. Immunologic features in coronavirus disease 2019: functional exhaustion of T cells and cytokine storm. J Clin Immunol (2020) 40:974–6. doi: 10.1007/s10875-020-00824-4
Keywords: immune checkpoint, COVID-19, cytokine release syndrome, SARS-CoV-2, prognosis
Citation: Lee N, Jeong S, Jeon K, Park M-J and Song W (2022) Prognostic impacts of soluble immune checkpoint regulators and cytokines in patients with SARS-CoV-2 infection. Front. Immunol. 13:903419. doi: 10.3389/fimmu.2022.903419
Received: 24 March 2022; Accepted: 27 July 2022;
Published: 15 August 2022.
Edited by:
Vijayakumar Velu, Emory University, United StatesReviewed by:
Sivaram Gunisetty, School of Medicine, Emory University, United StatesMorteza Jafarinia, Shiraz University of Medical Sciences, Iran
Sibtain Ahmed, Aga Khan University, Pakistan
Copyright © 2022 Lee, Jeong, Jeon, Park and Song. This is an open-access article distributed under the terms of the Creative Commons Attribution License (CC BY). The use, distribution or reproduction in other forums is permitted, provided the original author(s) and the copyright owner(s) are credited and that the original publication in this journal is cited, in accordance with accepted academic practice. No use, distribution or reproduction is permitted which does not comply with these terms.
*Correspondence: Seri Jeong, aGVoZWJveEBuYXZlci5jb20=