- 1Department of Rheumatology and Clinical Immunology, Amsterdam Rheumatology and Immunology Centre, Amsterdam University Medical Centers, University of Amsterdam, Amsterdam, Netherlands
- 2Department of Experimental Immunology, Amsterdam University Medical Centers, University of Amsterdam, Amsterdam, Netherlands
- 3Amsterdam Rheumatology Center, Amsterdam, Netherlands
- 4Department of Neurology, MS Center Amsterdam, Amsterdam University Medical Center (UMC), Vrije Universiteit Amsterdam, Amsterdam Neuroscience, Amsterdam, Netherlands
- 5Department of Endocrinology and Metabolism, Amsterdam University Medical Centers, University of Amsterdam, Amsterdam, Netherlands
- 6Amsterdam Rheumatology and Immunology Center, Reade, Amsterdam, Netherlands
The preclinical phase of autoimmune disorders is characterized by an initial asymptomatic phase of varying length followed by nonspecific signs and symptoms. A variety of autoimmune and inflammatory manifestations can be present and tend to increase in the last months to years before a clinical diagnosis can be made. The phenotype of an autoimmune disease depends on the involved organs, the underlying genetic susceptibility and pathophysiological processes. There are different as well as shared genetic or environmental risk factors and pathophysiological mechanisms between separate diseases. To shed more light on this, in this narrative review we compare the preclinical disease course of four important autoimmune diseases with distinct phenotypes: rheumatoid arthritis (RA), Systemic Lupus Erythematosus (SLE), multiple sclerosis (MS) and type 1 diabetes (T1D). In general, we observed some notable similarities such as a North-South gradient of decreasing prevalence, a female preponderance (except for T1D), major genetic risk factors at the HLA level, partly overlapping cytokine profiles and lifestyle risk factors such as obesity, smoking and stress. The latter risk factors are known to produce a state of chronic systemic low grade inflammation. A central characteristic of all four diseases is an on average lengthy prodromal phase with no or minor symptoms which can last many years, suggesting a gradually evolving interaction between the genetic profile and the environment. Part of the abnormalities may be present in unaffected family members, and autoimmune diseases can also cluster in families. In conclusion, a promising strategy for prevention of autoimmune diseases might be to address adverse life style factors by public health measures at the population level.
Introduction
Autoimmune disorders are diseases in which the immune system recognizes and reacts against self-antigens. Clinical onset is often preceded by low grade inflammation (1), disease-specific autoimmune features and nonspecific signs and symptoms. Little is known about similarities and differences between these diseases concerning the time course, nature and extent of inflammatory or autoimmune events. In the last 20 years, the number of individuals affected by autoimmune diseases has increased, especially in the more economically developed countries (2–4). Various mechanisms have been proposed to explain the increased incidence and prevalence, some of which might be shared between different autoimmune diseases.
Individuals in the pre-clinical phase have an initial asymptomatic phase of varying length in which the immune system is activated and the autoimmune process is started. Oftentimes, this phase is followed by nonspecific signs and symptoms and it might take years for the disease to manifest itself. To shed more light on this matter, we here compare the preclinical disease course of a selection of four important autoimmune diseases: rheumatoid arthritis (RA), Systemic Lupus Erythematosus (SLE), multiple sclerosis (MS) and type 1 diabetes (T1D). Although these are clinically distinct diseases, involving different autoimmune reactions and target organs, in some cases they appear to share certain genetic and environmental risk factors as well as pathophysiological mechanisms (5, 6). These insights may help to design strategies to prevent the development or progression of autoimmune diseases in general.
This review describes the evolution of disease manifestations from the pre-clinical phase up to clinical disease when the diagnosis can be made. We thereby focus on similarities and differences between the selected diseases rather than provide an in-depth review per disease. The review is not intended to give an overview of intervention studies in the at-risk phase, since these are discussed in another article of the present issue. The data were collected from literature via PubMed and Medline (Box 1).
Overview of Autoimmune Diseases
RA, SLE, MS and T1D are autoimmune diseases which affect specific organs (Figure 1), with a later shift towards systemic compromise due to complications and comorbidity. They may also be associated to varying degrees with systemic inflammation. In the pre-clinical stage of autoimmune diseases, individuals have risk factors, both genetic and environmental, which predispose them to the disease. In the next paragraphs, we’ll present an overview of those risk factors, and how they might be similar or differ between diseases.
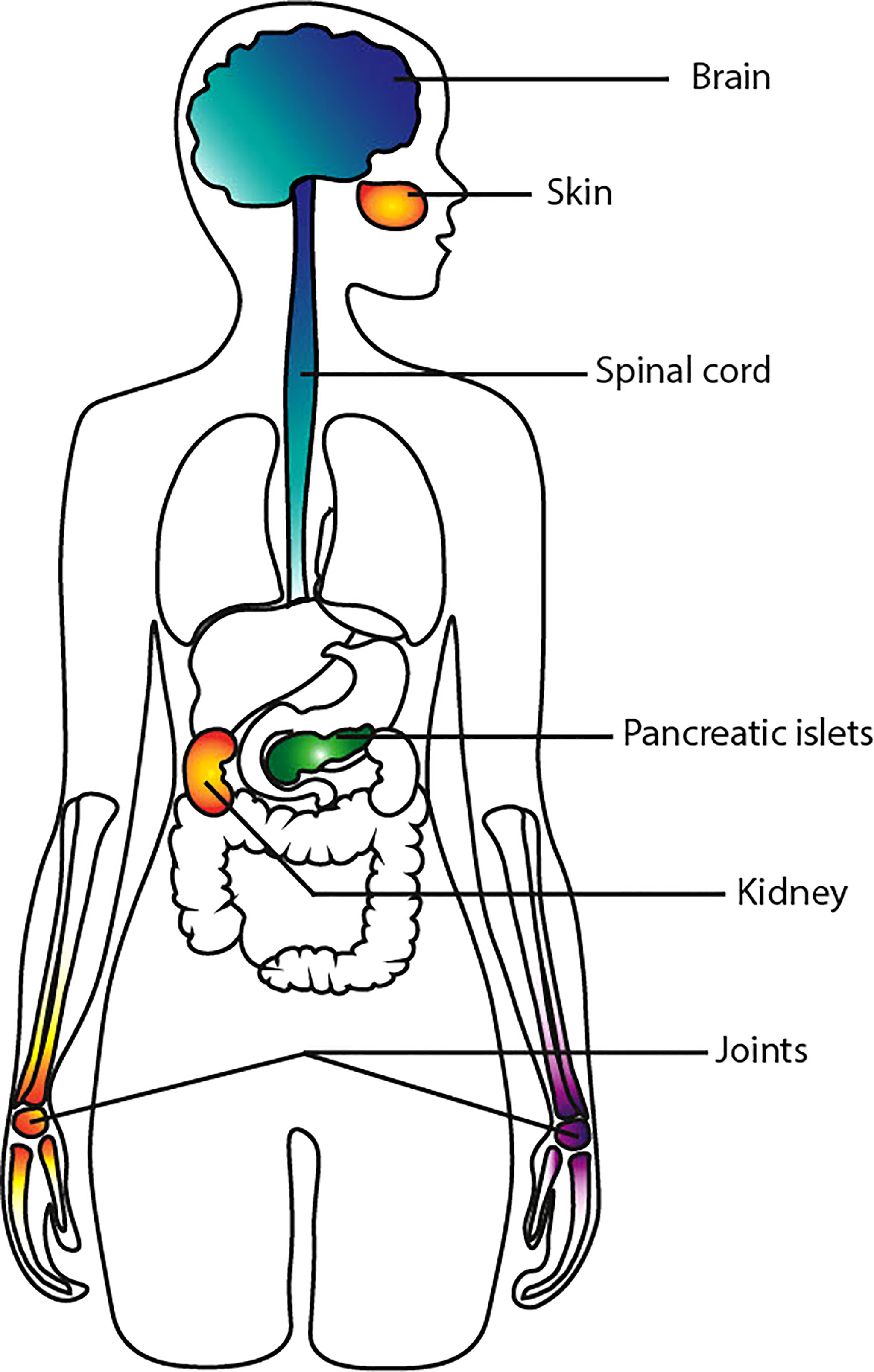
Figure 1 Primary site of onset. This illustration shows the primary site of initiation of the autoimmune process in RA, SLE, MS, and T1D. RA is represented in purple, SLE in orange, MS in blue and T1D in green.
Rheumatoid Arthritis
RA is an organ-specific autoimmune disease mainly characterized by a symmetrical peripheral polyarthritis, in which systemic inflammation and other manifestations may also be associated. RA affects 0,5-1% of the population worldwide, with a higher prevalence in regions at greater distance from the equator (7, 8). RA is seen as an autoimmune disease due to the presence of autoantibodies such as rheumatoid factor (RF) and anti-citrullinated protein antibodies (ACPA) in the majority of cases, which is then associated with a more severe disease course (9, 10).
In RA there is a familial clustering of disease, and a family history of RA increases the risk of disease by three to ten times (11, 12). This indicates an important role of genetic factors in disease risk. Indeed, more than 100 loci have been found associated to RA (13). The most relevant alleles are the ‘shared epitope’ (SE) at the HLA-DRB1 locus and Protein tyrosine phosphatase (PTPN22) (9, 14, 15). HLA-DRB1 codes for a cell surface molecule with a peptide-binding groove that has high affinity towards citrullinated proteins (16, 17). Other important genetic factors in RA are CLTA4 and PADI4, involved in the immune system regulation and post-translational conversion of arginine to citrulline residues, respectively (9, 18). RA has also higher incidence in women, with a female-to-male ration of 2-3:1 (19).
Several environmental factors contribute to the risk of RA. The most prominent one is smoking (20–22), which as a risk factor interacts with SE (17, 23, 24). The increased risk of RA associated with smoking requires long term exposure to manifest, but moderate cigarette consumption is enough to affect disease risk and individuals will have a high RA risk even years after smoking cessation (22, 25). Similarly, other airway irritants such as silica and textile dust exposure are associated with increased risk of RA (21, 26, 27). Additional lifestyle behavior, such as lack of exercise, stress, and an unhealthy diet, all contribute to increasing the risk of developing RA (28–33). Studies that have shown an association between high birth weight and RA suggest that even environmental exposures in utero may contribute to the risk for RA (34, 35).
The average age of onset of clinically manifest RA is around 50 years old. The onset is preceded in many cases by a preclinical phase characterized by activation of the immune system and production of autoantibodies. Circulating autoantibodies together with low level inflammation as measured by high sensitive CRP are found on average 5 years before the onset of symptoms (36, 37). In one prediction model (38) using demographic, clinical and serological characteristics, individuals in the highest risk category had an 80% probability of developing RA within 5 years. Immune cell recruitment is usually followed by non-specific musculoskeletal symptoms and fatigue (39). Moreover, pain and transient swelling of the joints are common symptoms in at risk individuals (30-60% of seropositive individuals) (40).
In the years preceding symptoms, the autoantibody response broadens to include more and more ACPA specificities (37, 41–43), anti-acetylated peptide antibodies (AAPA), anti-carbamylated (anti-CarP), and RF, which is referred to as epitope spreading (44–48). In the months before clinical onset, ACPA additionally undergo glycosylation changes both of the Fc part and the Fab part of the ACPA-IgG molecule, leading to a more pro-inflammatory phenotype (49–51). It has been hypothesized that environmental exposure related to the respiratory tract (smoking, dust, respiratory infections) might be involved in antibody production and disease pathogenesis (21, 26, 27). Those environmental triggers could cause low-level inflammation of the lung mucosae, leading to protein citrullination (23, 52).
In patients with early RA, studies of low level inflammation at mucosal sites such as the gums and the lungs have revealed that these inflammatory lesions can be involved in local ACPA production. Transfer of ACPA to the joints may then be one mechanism that incites inflammation at the joint level. Soluble factors also regulate the immune response, and both pro-inflammatory and anti-inflammatory cytokine levels are altered in the preclinical phase (53). Markers of inflammation, such as C-Reactive Protein (CRP), are also increased up to 5 years before RA onset and positively correlate with antibody levels. Individuals with both elevated CRP and autoantibodies are more likely to develop RA (36, 37, 54). Increased plasma levels of polyunsaturated fatty acid-derived lipid mediators such as 5-Hydroxyeicosatetraenoic acid (5-HETE) are seen in ACPA positive individuals who later develop inflammatory arthritis (IA), further increasing the risk and pointing to a low omega3 fatty acid status. Cytokines associated with 5-HETE, such as IL-1β, IL-6, IL-8, and TNF, are also altered in preclinical RA individuals (55, 56).
In the phase with vague symptoms such as stiffness or arthralgia, inflammation of the joints can sometimes already be detected by imaging modalities as ultrasound, MRI and PET scan. In particular, subclinical inflammation of the joints detected by MRI predicted RA onset by a few months (57–60). At the time RA is diagnosed, patients usually have symmetrical polyarthritis in the hands and/or feet, which when left untreated can progress to joint destruction (61, 62). In established RA there is an overlap with other diseases, or comorbidity, including cardiovascular disease, chronic lung disease and periodontitis (63, 64).
Systemic Lupus Erythematosus
SLE is characterized by a great variety of clinical manifestations, including inflammatory skin lesions, arthritis, pleurisy and pericarditis, inflammation in the internal organs, involvement of the central or peripheral nervous system, hematological manifestations, and others. The disease course is highly variable, some patients experiencing long periods of remission (the absence of disease manifestations), but many more experiencing frequent flares of disease activity and/or chronic symptoms. For many patients, the general feeling of illness, accompanied by fatigue, lassitude, and minor cognitive difficulties is the most burdensome feature of the disease.
SLE is an uncommon disease with wide geographic variation in distribution, with high frequency in North America; SLE also has higher frequency in the Afro-American population compared to Caucasians, which may be due to both genetic and environmental differences (65). There is a genetic component of disease, with concordance in monozygotic twins of 24-35% as compared to 2-5% in dizygotic twins (66). In the Caucasian population, HLA-DRB1*1501 and HLA-DRB1*0301 are associated with a 2-to-3 fold increase risk of SLE (67, 68). Other genes strongly associated with SLE are those coding for the complement system and the Fc-γ receptor (FcγR), all of which have a role in immune regulation (69–71). Genes that are involved in the IFN pathway, such as Interferon Regulatory Factor 8 (IRF8), IFIH1, Toll-like receptor 7 (TLR7), and Tyrosine Kinase 2 (TYK2) are also risk loci for the disease (72, 73). SLE also affects women much more frequently than men (female-to-male ratio 9:1) (65, 74, 75).
Smoking is a risk factor for SLE and is associated with higher anti-double strand (anti-dsDNA) antibody production (76). In the Nurses’ Health Study, nurses that smoked had a 67% increased risk of developing SLE compared to non-smokers, although the intensity of smoking did not influence disease risk. This association is time-sensitive, and the increased SLE risk persists for up to five years after quitting (77). It has been suggested that vitamin D may have a role in SLE pathogenesis and progression, and vitamin D supplementation might ameliorate inflammatory and hemostatic markers, however, this is controversial (78, 79). Lack of sleep is also associated with the transition to SLE in one study (80).
SLE occurs at all ages but the peak incidence is in the 3rd and 4th decades of life, and men have a later peak incidence compared to women (81, 82). Studies of the evolution of SLE from a healthy state through a preclinical phase to full-blown disease are complicated by the fact that the diagnosis of SLE cannot be made until sufficient clinical manifestations have occurred, to give the clinician the confidence that the diagnosis is correct. Thus, it is quite common for individuals to experience some joint pains and skin lesions for several years without a diagnosis. But then, an episode of pleurisy and the discovery of antinuclear (ANA) and anti-dsDNA antibodies leads to the diagnosis of SLE. No serious observer can doubt that the earlier joint and skin symptoms were manifestations of the same disease process, yet it would not have been correct to make the diagnosis of SLE at that time. In some cases, intermediate disease categories are used, such as “incomplete lupus” or “undifferentiated connective tissue disease”, but lack of uniform definitions and the variety of clinical and laboratory manifestations that are seen have hampered further progress.
Thus, it has been challenging to investigate the pre-clinical phase of SLE. A landmark study by Arbuckle et al. found that the emergence of autoantibodies preceded the clinical disease by many years, and there seemed to be a strict order by which they manifest: the first antibodies to appear are ANA, antiphospholipid, anti-Ro (SS-A) and anti-La antibodies (SS-B), which manifest at the same time. Anti-Ro antibodies are detectable in the serum approximately four years before SLE clinical manifestations. Subsequently, anti-dsDNA antibodies become manifest months before clinical diagnosis, followed by anti-Sm and anti-nuclear ribonucleoprotein (anti-RNP) antibodies, whose levels start increasing exponentially up to a year before diagnosis and are highest just before the disease is diagnosed (83, 84).
SLE also has alterations in levels of pro-inflammatory cytokines long before the onset of clinical signs and symptoms, with increased type I and II interferon (IFN-I and IFN-II), IL-5, IL-6, IL-17, and TNF (84, 85).
Multiple Sclerosis
MS is an inflammatory demyelinating disorder of the central nervous system (CNS) with a presumed autoimmune pathogenesis. Several genetic, environmental and lifestyle risk factors are reported. A latitudinal gradient has been found, i.e. the farther away from the equator the frequency of MS increases. This latitudinal risk factor may reflect differences in UV radiation, sun exposure, vitamin D levels and epigenetic interactions. Migration from a higher to a lower latitude after puberty has an impact on disease risk: migrants retain its original risk (6, 86, 87). Genetic predisposition has a role in disease susceptibility, with 5% disease concordance in dizygotic twins that increases to 25% in monozygotic twins (87). HLA alleles exert the most common genetic risk factors, in particular the HLA-DRB1*1501 haplotype has been demonstrated to be the most significant genetic risk factor to develop MS (odds ratio approximately 3) (5, 88). More than 500 small nucleotide polymorphisms (SNPs) are associated with MS risk, involving mostly immune associated genes, such as IL-2 receptor subunit alpha (IL2RA), IL7R, CLEC16A and CD226 (5). MS is more frequent in women, with a female-to-male ratio of 2-3 (89).
Cigarette smoking contributes to the risk of MS, with a 50% higher risk in ever smokers compared to never smokers (86, 87). Two environmental factors that influence MS risk are vitamin D levels and Epstein-Barr virus (EBV) infection (6, 86, 87, 90, 91). Vitamin D deficiency in earliest stages of life is associated with increased risk of MS, while high sun exposure during childhood correlates with lower risk of disease (5, 6). In addition, the Nurses’ Health Study showed a 40% decrease risk of MS in women that had at least 400 international unit (IU) of vitamin D intake per day. Childhood obesity is associated with a higher risk to develop MS (86, 87, 92). Although the mechanism of action has not been fully elucidated yet, EBV infection seems to be a causative and necessary but not sufficient agent to develop MS (5, 86, 87, 90, 91). Recently, Lanz et al. demonstrated a high-affinity molecular mimicry between the glial cell adhesion molecule (GlialCAM) in the CNS and EBV nuclear antigen 1 (EBNA1). Considering that nearly 100% of MS patients has detectable anti-EBNA1 antibodies before clinical symptoms, it suggests that molecular mimicry may play a role in the pathophysiological mechanism to induce MS (93). Age of infection also influences disease risk, with 2-to-3 fold higher risk in individuals with EBV infection at later age (87).
In general patients are identified when they first manifest signs and symptoms characteristic for this disease (94). Most patients are diagnosed between age 20 and 40 year, however children and people of older age may also be diagnosed with MS (89). The clinical phase of MS is preceded by a latent period, in which a prodromal phase of MS can be identified (95). The prodromal phase can manifest 10-15 years before symptom onset, even up to 20 years in primary progressive MS (PP-MS). In this phase, an early set of sign and symptoms that predates classical MS symptoms start to manifest (96). A subclinical inflammation (SCIN) phase seems to be the first step of disease pathogenesis (94). While no formal biomarkers of the prodromal stage are available, the radiologically isolated syndrome (RIS) might be considered a neuroimaging biomarker (96, 97). In RIS, the CNS shows lesions similar to those identified in MS patients without clinical symptoms suggestive of MS, with areas of the brain and the spinal cord that show signs of damage and scarring (97).
Serum neurofilament light chain (sNfl) is indicative of ongoing neuraxonal degeneration, and can be used as a biomarker for neuronal injury. MS patients usually have high levels of sNfl that decrease after treatment with disease modifying therapies, and MS risk positively correlates with higher sNfl levels in a time-dependent manner, starting several years before MS (median of 6 years) (96, 98). In the earliest stages of disease, the adaptive immune system is mostly involved in pathogenesis, in particular with autoreactive T cells, B cells, and autoantibody production against myelin proteins (99, 100). T and B cell in spinal fluid are altered in prodromal MS, and present a pro-inflammatory cluster, with high percentage of expanded CD8+ T cells within the neuronal lesion (94).
MS can either manifest as episodes of inflammation with neurological symptoms followed by partial or total remission (relapsing remitting MS, RRMS, 85% of patients), or as a gradually progressive disease (PPMS). In time, RRMS may evolve into a progressive phase of the disease called secondary progressive MS (SPMS). Depending on the site of the lesion, patients may have different clinical pictures. Common presenting symptoms in RRMS are optic neuritis and ascending sensory symptoms, whereas PPMS in general presents with progressive motor impairment (88).
Type 1 Diabetes
In T1D autoimmunity targets the beta-cells of the pancreas eventually resulting in absolute insulin deficiency. Similarly to the diseases mentioned above, T1D incidence is also affected by the latitudinal gradient and migration, with increased disease risk when populations move from low-incidence to high-incidence countries (6, 101). However, genes have a relevant role in disease risk, and relatives of T1D patients have a 15-20 times higher risk of developing T1D, rising from about 0,4% in the general population to 25-50% in monozygotic twins (102). Familial risk is mostly linked to HLA genes, and decreases to 1% in non-HLA genes (5). HLA are the most common alleles involved in T1D, but other relevant genetic risk factors include genes involved in the insulin and metabolism, as well as regulators of the immune response (5, 103).
In contrast with the other diseases discussed here, there is no demonstrated association between smoking and T1D (6), which might be explained more by the young age of patients at disease onset than by a true lack of a role of smoking in disease pathogenesis. Low physical activity, psychological stress and psychological trauma are associated with T1D risk (101). Vitamin D supplementation leads to lower autoantibody levels which may be beneficial in the early stages of disease (5, 15).
Diet may also influence T1D risk, as there is an increased risk in overweight children (101). Cow’s milk consumption is associated with islet autoimmunity (IA) and pancreatic beta cell destruction (15, 101). Other possible risk factors for T1D are viral infections, such as enterovirus, Coxsackie B viruses (CBVs), and respiratory viruses. Viral infections seem to correlate with incidence of islet autoimmunity (5, 101).
T1D present two peaks of incidence at 4-7 years old and – more commonly – 10-14 years of age (104, 105). T1D pathogenesis is characterized by three stages, two of which compose the preclinical phase. The first, asymptomatic stage involves immune recognition and activation with autoantibody production, initial beta cell destruction, but absence of dysglycaemia. In the second stage, progressive islet destruction and loss of beta cell mass leads to impaired insulin production and eventually dysglycaemia. Individuals in this stage are still asymptomatic (15), however, this stage evolves gradually. When approximately 80% of beta cell mass is destructed, glucose will rise and patients will become symptomatic. The percentage beta cell loss needed before symptoms arise decreases with age (106). The insulitis, persistent inflammation of pancreatic cells, is associated with functional impairment in the latest stages of preclinical disease (107). However, functional biochemical testing might already show impaired glucose tolerance.
Biomarkers of the T1D preclinical phase, and its progression towards clinical manifestation, are also the proinsulin to c-peptide (PI:C) ratio and reduced pancreatic volume. The first is indicative of beta cell stress, while the latter seems to correlate with reduced pancreatic islets and loss of exocrine volume. At-risk individuals, especially children younger than 10 years old, that progress to T1D have higher serum PI:C ratio than those who never progress to T1D. Moreover, FDR of T1D patients have reduced pancreatic volume compared to seronegative individuals, although it is still higher than patients with recent onset T1D (108, 109).
High levels of CD4+ and CD8+ T cells with specificity for beta cell autoantigens are now found in the islets of asymptomatic individuals. This antigen recognition might be mediated by B cell antigen presentation to T cells (107, 110). As said, autoantibodies are the first markers of disease. There are five main autoantibodies directed against insulin and islet cells. They precede clinical manifestations of T1D and are markers of beta-cell autoimmunity: autoantibodies against insulin (IAA), autoantibodies against insulinoma-associated antigen-2 (IA-2), autoantibodies against glutamic acid decarboxylase (GAD or GADA), autoantibodies against zinc-transporter 8 (ZnT8), and islet cell antibodies (ICA). The distribution of the different antibodies is age-related as IAA is the main antibody found in children, while GADA is most commonly found in young adults (111). Post-translation modification of insulin causes the formation of new epitopes that are recognized by autoantibodies involved in T1D pathogenesis (15, 103, 112, 113). The probability of diabetes development is dependent on the number of islet antibodies found in one person (114).
The symptomatic stage of T1D manifests as polyuria, polydipsia due to hyperglycemia, and eventually ketoacidosis caused by excessive lipolysis due to insulin deficiency and can only be treated with insulin replacement therapy (106).
Comparison Between Diseases
The four diseases included in this review can affect a wide range of organs and tissues, that may be the initial site of an attack by the immune system. In line, the resulting pathology is diverse and one could easily conclude that the diseases have little in common. However, when one looks beyond the clinical manifestations to the genetic, environmental and behavioral determinants, it appears that apart from the differences there are also some notable similarities (Table 1). These include (in the majority of diseases) aspects such as a North-South gradient of decreasing prevalence (6, 86, 87, 101), a female preponderance (19, 89, 115), major genetic risk factors at the HLA level, partly overlapping cytokine profiles and lifestyle risk factors such as obesity, smoking and stress. Of note, T1D has predominance in males (116).
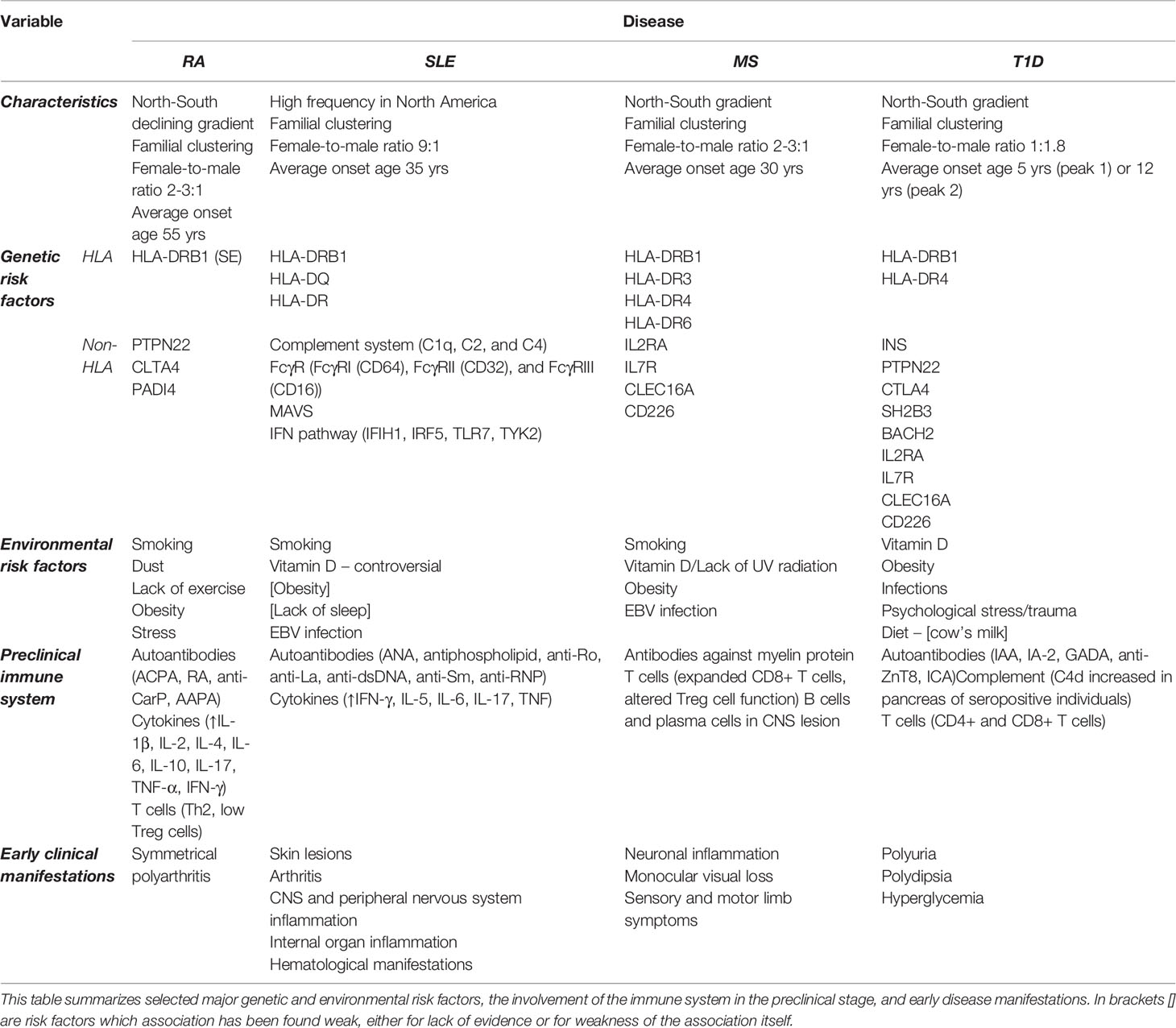
Table 1 Overview of selected major characteristics, risk factors, immunological and clinical features of four autoimmune diseases.
The North-South gradient may point to genetic differences, but can also be partly due to different climatic influences or dietary habit differences between more Northern and more Southern regions. Likewise, the observed female preponderance may be related to reproductive hormonal factors or alternatively to X-linked genetic factors. For both explanations, the available data do not fully explain the predominance of females (117). The importance of the environment is illustrated by the effect of migration, as an example children that move from Nordic countries to southern countries in younger years have the same prevalence of MS and T1D as is present in the new country (6, 86, 87). Moreover, an increased prevalence of RA was observed after migration from rural to urban areas in South Africa (118). A central characteristic remains the lengthy period of asymptomatic to undifferentiated disease which can cover many years, suggesting a gradually evolving interaction between the genetic profile and the environment. Differently from RA, SLE, and MS, T1D symptomatology is dependent on the amount of beta cell destruction, with residual hormonal function preceding symptoms (106).
When we thus suppose there may be a partly shared pathophysiology between the four diseases, one might expect this to become apparent in a clustering of diseases in the same individual. RA and SLE can indeed occur together, a situation called “rhupus”, however, this is quite uncommon (119). MS and T1D also tend to have a lower overlap than expected by their prevalences, partially due to an opposite role of HLA haplotypes (5, 6). T1D on the other hand seems to predispose affected persons to develop RA, possibly due to shared genetic risk factors (120).
On the level of antibodies, we see that RA patients might express ANA antibodies, while SLE and T1D patients can also express RF and/or ACPA (121). Relatives of patients also have risk of developing autoimmunity, not necessarily the same as their affected relative. This might be due to both shared genetic and environmental risk factors, and a more pro-inflammatory state of the immune system. Indeed, the presence of autoantibodies and their related autoimmune disease predispose patients to manifest non-disease specific antibodies, in a process called poly-autoimmunity. Hence, the mechanisms involved and the timeline of autoantibody production are still not clear, but both genetic and environmental factors might be involved (121). Taken together, the four diseases show a modest overlap in occurrence but more overlap in autoimmune phenomena.
In this sections below, we look further into these overlapping aspects.
Genetic Risk Factors
RA, SLE, MS, and T1D all have a genetic component, with familial clustering and higher risk of disease in first degree relatives of patients (FDR) (12, 66, 87, 102, 122). Several of these genetic risk factors are shared between the diseases, with similarities being most apparent between RA and SLE on one hand, and between MS and T1D on the other hand.
The most prominent genetic risk factors are alleles within the HLA class, in particular HLA-DRB1. HLA contributes to nearly 33% of RA risk (123).The HLA-associated risk in RA with an odds ratio of around 6 is almost entirely due to a small peptide sequence present in a number of HLA-DRB1 haplotypes, the ‘shared epitope’ (124, 125). In the Caucasian population, HLA-DRB1 alleles are associated with a 2-to-3 fold increase risk of SLE, however, this association has not been seen consistently in the Afro-American population (67, 68, 126). On the other hand, specific HLA haplotypes might have a protective role in MS and T1D, such as HLA-DRB1*01, HLA-DRB1*10, HLA-DRB1*11 and HLA-DRB1*14 (5). HLA-DRB1*04 is a risk factor in both RA and T1D (5, 24), while HLA-DRB1*1501/DQB1*0602 have an opposite effect in MS and T1D, with an increased risk for MS but a protective role in T1D (5, 87). However, several other SNPs of the HLA gene associated with T1D also seem to be associated with MS (5, 6). Both MS and T1D have an epistasis effect, with haplotype-specific interactions between alleles of different parental origins (5).
RA, SLE, and T1D also share non-HLA risk factors with other autoimmune diseases, such as celiac disease, psoriasis, and autoimmune thyroid disease (5, 127, 128). Moreover, loci on the chromosome 3 have a 16% relative contribution to the risk of RA (123). A large number of non-HLA genes involved in autoimmune diseases are interlinked in a network that regulates interferon signaling and dendritic cell (DC) and T cell function. The tyrosine kinase cell-surface receptor FLT3, also known as CD135, is expressed on DC, and lymphoid and myeloid progenitors, and is involved in the regulation of monocyte and DC maturation. A specific intron variation in FLT3 causes the production of a truncated protein, with decreased levels of FLT3 receptor and increased circulating FLT3 ligand, which could lead to autoimmunity. FLT3 is associated with increased risk of RA, SLE, and T1D, and high levels of FLT3 ligand are found in both serum and synovial fluid of inflamed joints of RA patients (127).
Both RA and SLE show an association between disease risk and genes that are involved in type I interferon production, signaling, and response, such as IRF5, Interleukin 1 receptor associated kinase 1 (IRAK1), and Signal transducer and activator of promoter 4 (STAT4) (9, 14). In SLE, in presence of anti-RNA binding proteins (RBP) and anti-dsDNA antibodies, IRF5 is associated with higher levels of circulating type I interferon activity. Additionally, IRF5 variants are associated with higher antibody production predisposition in healthy individuals, which could form immune complexes that activate innate immune cells through over activation of the toll-like receptor (14).
A SNP haplotype of the STAT4 gene in the third intron is associated with both RA and SLE, with higher risk when this SNP is present in both alleles. STAT4 is involved in the signaling of cytokines, such as IFN-I, IL-12, and IL-23, which promote differentiation of effector T cells towards a Th17 phenotype. However, STAT4 has different roles in RA and SLE at least according to animal models: while in RA STAT4 deficiency in mice is protective, with inability of those mice to develop RA, in SLE STAT4-deficient mice have accelerated nephritis and higher mortality (129).
PTPN22, which codes for a protein involved in both T and B cell signaling, is also an important risk factor for RA, SLE, and T1D (9, 14, 15). In RA, PTPN22 has a stronger association risk in male compared to female seropositive individuals, and gene carriers have an earlier onset of disease (9). PTPN22 is one of the common non-HLA genes associated with T1D, together with IL2RA, which in turn is also associated with SLE and MS (15, 128). IL2RA is involved in lymphocyte activity regulation and confers a 28% and 33% increased risk of developing MS and T1D, respectively (6, 130).
Other non-HLA genetic factors involved in autoimmunity are small nucleotide polymorphisms (SNP) in immune associated genes, such as IL7R, SH2B3, CTLA4, BACH2, CLEC16A and CD226, and the latter are involved in both MS and T1D risk (5, 6, 9, 131–134). These SNP can either give a predisposition to both diseases, or be mutually exclusive, and some of the shared genetic risk factors between MS and T1D are directly associated with disease development (5, 6). Both in MS and T1D, the weight of the genetic predisposition in disease development depends on the family member affected by the disease, with a parent-of-origin effect (5, 135). In T1D, there is an higher risk associated with paternal heredity, while in MS the increased risk is associated with maternal heredity (5).
Lifestyle and Environmental Factors
Although genetic factors play an important role in risk of autoimmunity, genetic predisposition is able to explain only up to 50% of the risk of developing RA and T1D, leaving half of the patients without any known genetic marker (122, 135). Numerous studies have investigated the role of environmental factors in disease development including lifestyle factors, comorbidities, external agent exposure and bacterial and viral infections (77, 136, 137).
Smoking is one of the most prominent environmental risk factors, and has a role in RA, SLE, and MS (20, 77, 87). Although no association has been described between smoking and T1D, this is more likely due to the young age of T1D onset. Smoking causes citrulline autoimmunity in the lung in genetically susceptible individuals (24, 52, 138) and also triggers the production of RF (20, 22, 23), explaining an association between smoking and seropositive RA. In SLE, smoking is a risk factor for anti-dsDNA production (77), while in MS smoking induces an increased axonal demyelination and disruption of the blood-brain barrier, in parallel with an immunomodulatory effect mediated by increasing both nitric oxide levels and its metabolites (87). Both in RA and MS, but not in SLE, smoking has a dose-response relation with disease risk (22, 25, 86). After smoking cessation, the increased risk for RA and SLE remains present for several years (22, 25).
Occupational exposure seems also to be a risk factor for autoimmune diseases; silica and other inorganic dust exposure have been reported to increase the risk of RA and SLE (27, 139, 140). However, these associations are not as strong as for smoking.
Additional lifestyle factors are exercise, alcohol consumption, diet and body mass index (BMI). Exercise and moderate alcohol consumption have been associated with decreased risk of RA and SLE (33, 141), while obesity is associated with higher risk of RA, SLE, MS and T1D (76, 141–145). In persons at risk for RA, the combination of obesity and smoking seems to synergistically increase the risk of RA (146). In the Nurses’ Health Study, overweight and obese women had higher risk of developing RA, MS, and T1D (86, 147, 148). Similarly, being overweight is associated with higher risk of T1D (101). Consequently, dietary factors may be expected to play a role in disease risk. The overall dietary quality influences the risk for RA, amounting to a 40% decrease in risk for seropositive RA in women in the highest versus the lowest quartile of dietary quality (32). As for MS, a highly enriched fish diet seems to be protective; populations in Northern countries with a diet high in fish and fish oils show a similar MS incidence to those in lower-latitude countries (86, 87). In the case of T1D, cow’s milk has been suggested to trigger an autoimmune response in genetically at-risk individuals that leads to the destruction of pancreatic beta cells (15, 101). This correlation has been also found in the Diabetes Autoimmunity Study in the Young (DAISY), in which children with low and moderate genetic risk that had higher cow’s milk intake also had higher risk of islet autoimmunity (IA) (149).
Either chronic stress or the presence or post-traumatic stress disorder (PTSD) have both been related to the subsequent occurrence of autoimmune diseases (28). In a study covering the whole population of Sweden, a diagnosis of a stress-related disorder increased the risk of any autoimmune disease by 50% in the whole period of 35 years thereafter, including the diseases discussed here. Furthermore, a large study on US veterans of the Iraq war showed a doubled risk of RA, SLE and MS in individuals affected by PTSD (150). An increased risk for RA was also found by the Nurses’ Health Study in nurses that had PTSD symptoms (151), and chronic stress and psychological trauma had also been suggested to be associated with T1D risk. At least for the effect of stress, this might be due to higher levels of cortisol, inducing insulin resistance while also modulating the immune response (101).
It is important to consider that many of the associations mentioned above have a tendency to cluster within the population. Unhealthy diet, lack of physical activity, obesity, chronic stress, as well as environmental exposure, low socio-economic status and low income, all co-segregate, making it hard to identify if the causal association found by observational studies is caused by one specific factor or a combination of them.
Vitamin D levels have been suggested to influence disease severity in both MS and T1D in a seasonal way, with higher relapses in MS and diagnostic rate in T1D linked to vitamin D status (5). The mechanisms behind this association are not clear. However, 25-hydroxy vitamin D (25(OH)D) levels, which reflect vitamin D absorption by UV light exposure, inversely correlate with MS risk in white individuals (86, 87). 25(OH)D levels also inversely correlate with BMI, especially above 30, which might suggest an indirect mechanism of BMI as a risk factor (86). The onset of the first demyelinating event in at-risk-of-MS individuals correlates with both sun exposure and vitamin D levels. Sun exposure is measured by the degree of actin damage, which was lower at the time of onset of disease (87). While there is no correlation between T1D and 25(OH)D levels at birth, a birth-cohort study in Finland showed that 1 year of supplementation of dietary vitamin D, at a dose of 2000 IU daily was associated with a reduced risk of developing T1D in children. This might indicate a role of vitamin D in the pathogenesis of T1D between birth and early childhood (152, 153).
Another factor that may play a role in disease risk are viral infections. In RA there is no consistent evidence of infections involved in the pathogenesis. Epstein-Barr (EBV) infection has been suggested to increase the risk of SLE (154, 155) and is a major environmental risk factor for MS development (91, 96). While individuals with elevated immunoglobulin levels against EBV have a 2-fold increased risk of developing MS, EBV seronegative individuals have a disease risk near zero. Moreover, this mechanism seems to be specific to EBV, since cytomegalovirus infection does not influence MS risk, suggesting that EBV infection may be partially necessary for MS onset (5, 86, 96, 156). It has been postulated that EBV infection either increases activation and expansion of T and B cells, or is responsible for B cell immortalization, in particular of B cells that produce antibodies against EBV, leading to antigen presentation to pathogenic T cells (87).
While the association between MS and EBV infection is strong, the role of infections inT1D pathogenesis is not yet well defined. The Diabetes Prediction and Prevention (DIPP) study demonstrated a correlation between first autoantibody appearance and enterovirus infection, and serological studies suggest a link between Coxsackie B virus, in particular CBV4 serotype, and T1D. Moreover, the Teddy study described a possible correlation between respiratory infections, with a common peak between 6 and 9 months of age, and increased risk of islet autoimmunity, which follow a similar trend (15, 157). In summary, there is evidence for a role of viral infections in the pathogenesis mainly of MS and T1D, with a very specific role of EBV in MS.
Activation of the Immune System
RA, SLE, MS, and T1D all have a latent phase that precedes formal clinical diagnosis (Figure 2). The length of this phase can vary between diseases and within individuals at risk for the same disease, but a common feature is the activation of the immune system, which is visible to a varying degree in the different diseases and precedes the onset of symptoms.
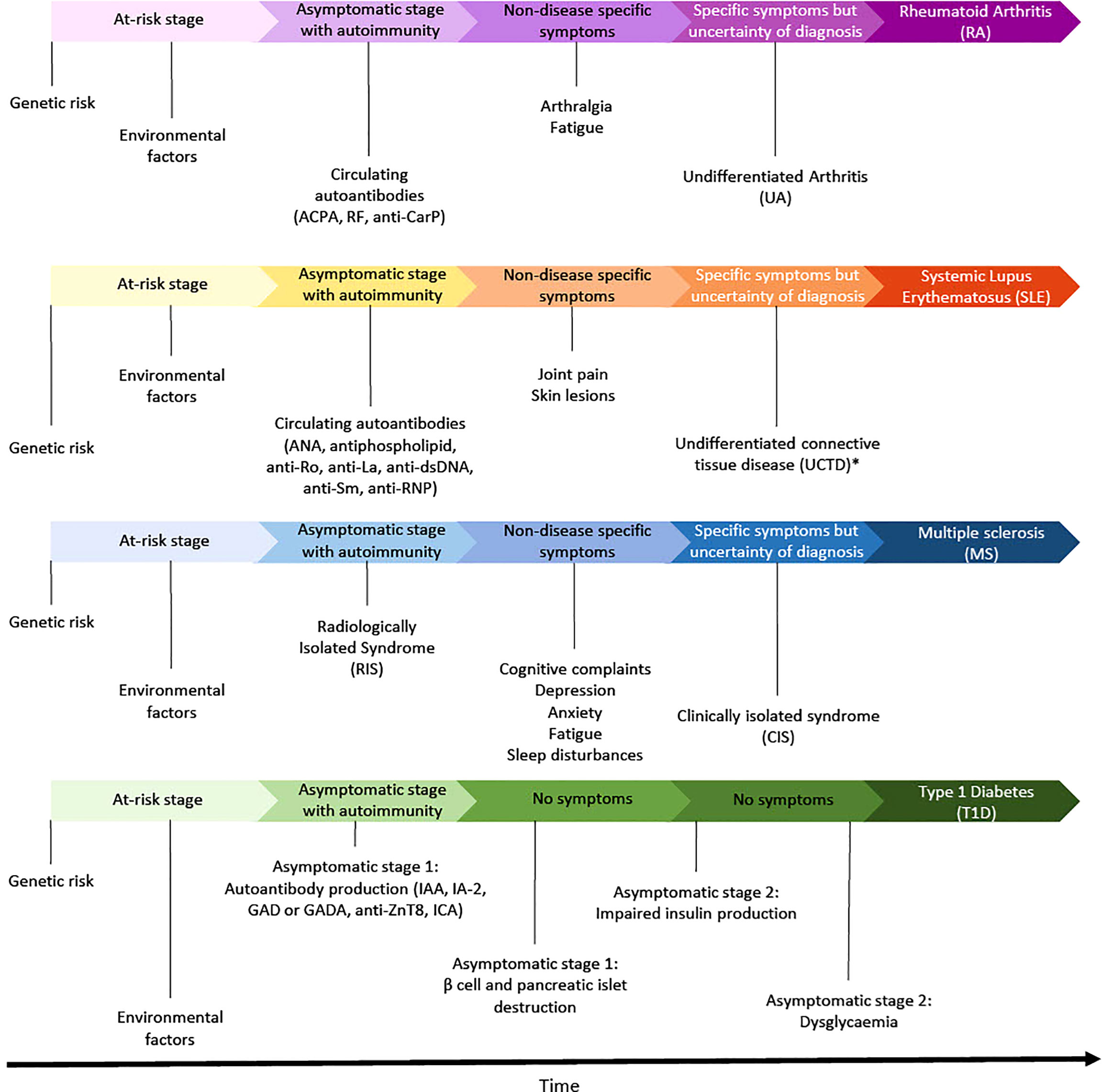
Figure 2 This illustration shows an overview of the transition from at-risk to disease diagnosis. In purple is represented RA, in orange SLE, in blue MS, and in green T1D. *Also known as “incomplete Lupus”. ACPA, Anti-citrullinated protein antibody; RF, Rheumatoid factor; anti-CarP, anti-carbamylated; ANA, antinuclear antibody; anti-dsDNA, anti-double strand DNA; anti-RNP, anti-nuclear ribonucleoprotein; IAA, autoantibodies against insulin; IA-2, autoantibodies against insulinoma-associated antigen-2; GAD or GADA, autoantibodies against glutamic acid decarboxylase; anti-ZnT8, autoantibodies against zinc-transporter 8; ICA, islet cell antibodies.
Humoral Immunity
As described above, the majority of RA patients is seropositive, and these antibodies develop over many years before the clinical disease, with increasing concentrations as well as specificities (59, 158). In particular ACPA are thought to be involved in the development of synovitis and bony erosions.
In contrast, in SLE autoantibodies are uniformly found in all patients. This is in part due to the conceptions and definitions used for making the diagnosis of SLE in clinical practice, codified by the recent EULAR/ACR classification criteria for SLE where the presence of ANA is required (159).
Some autoantibodies in SLE play an important role in the pathogenesis; this is most convincing for anti-DNA antibodies. Furthermore, there is a strong association between the combination of multiple antibodies, such as anti-dsDNA and anti-C1q, decreased complement levels, and lupus nephritis (LN). The most reproducible autoantibodies for diagnostic purposes are those reflecting renal involvement (160, 161).
So far, in MS no specific autoantibody has been found, however, autoantibodies against several CNS cells have been reported in this disease (162, 163).
As noted above, also persons at risk for T1D can develop several types of autoantibodies. There is a combination effect of multiple antibodies, with 70% risk of disease in children with multiple (three or four) circulating antibodies. Young children preferably develop IAA, while GAD autoantibodies are most commonly found in teenagers. However, the conversion from single to multiple antibodies can be slow (111, 114, 164–166).
Nearly 60% of children with single autoantibodies will lose antibody production over time and convert to seronegative (15). This mechanism is unique to T1D and differs from RA and SLE.
Of note, FDR of RA, SLE, and T1D patients may have autoantibodies detectable in their serum in absence of any signs or symptoms of disease (83, 121).
Cellular Immunity
The cellular component of the immune system also has an active role in disease pathogenesis. In preclinical RA, ACPA+ individuals have decreased T regulatory (Treg) cell levels and a shift of CD4+ T cells towards pro-inflammatory subsets, in particular T helper (Th) 2 cells (53, 167). In SLE, numerous abnormalities of cellular immunity have been described (168) but it has been difficult to determine whether these are necessary elements of the pathophysiology of the disease itself or the consequences of long-standing inflammation or of the treatments used to control it. In established MS altered activity and levels of Treg cells and predominance of CD8+ T cells are found within the neuronal lesion (94, 169, 170). In the prodromal phase of MS, the frequency of expanded CD8+ T cells within the CNS increases, and cells show alterations of their markers towards a more pro-inflammatory phenotype (94). Autoreactive T cells target the myelin in MS and the pancreatic islets autoantigens in T1D patients and T1D relatives, respectively (171). In the asymptomatic phase of T1D, the high levels of CD4+ and CD8+ T cells that are specific for beta cell autoantigens cause persistent inflammation of the pancreatic islet, called insulitis. This antigen recognition might be mediated by B cell antigen presentation to T cells (107, 110).
The B cell component is also altered in individuals at risk for RA, who have higher levels of IgA plasmablasts than the general population (172). The importance of the B cell component in the evolution from at-risk individuals to RA has also been demonstrated in the PRAIRI study, a clinical trial in ACPA-positive at-risk individuals, in which B cell depletion through a single dose of rituximab significantly delayed disease onset compared to placebo (173). In SLE, patients have decreased levels of CD27-IgD-IgM B cells, which represent an activated and auto-reactive state (174). Expanded B cells are also found in the neuronal lesions of prodromal MS individuals, where they correlate with oligoclonal immunoglobulin bands (94). Moreover, B cells also have a role in the pathogenesis of T1D, as demonstrated by B cell depletion after 1 year treatment with rituximab. Patients that received the treatment had reduced impairment of beta cell function compared to placebo, and required less insulin for disease management (175).
Soluble Factors
Soluble factors have a role in disease pathogenesis, inducing immune activation, recruitment, and regulation of the immune response. They are also responsible for direct pathogenic manifestations and can be used in some cases as biomarkers of disease progression. Soluble factors involve a variety of molecules, such as cytokines, complement and markers of inflammation.
Preclinical RA individuals on average have increased levels of both pro-inflammatory and anti-inflammatory cytokines, such as IL-1β, IL-2, IL-4, IL-6, IL-10, IL-12, TNF-α, and IFN-γ. Cytokine levels change over time, IL-4 and IL-14 levels being higher at the earliest stages of disease, and IL-17 levels increasing before disease onset and decreasing after RA becomes established (53, 176). Type I interferon (IFN-I) is detectable in the blood of both at-risk and established RA individuals, and has also a role in SLE initiation of SLE. While in RA there is higher production of IFNβ, in SLE there is abundance of circulating IFNα. Treatment of viral hepatitis with INF-α has been associated with de novo onset of SLE, the symptoms of which would improve after the treatment is stopped. Serum levels of IFN-I increase drastically one year before SLE onset, and circulating IFN-I is considered an hereditary risk factor for SLE (14). IFN-γ is also increased in SLE individuals more than 3.5 years before diagnosis and is associated with increased anti-RNA antibody production, inflammation, and transition from undifferentiated disease to connective tissue disease (84, 177). First-degree relatives of patients with MS have on average a more pro-inflammatory cytokine profile (higher TNFα, lower IL-10), this suggests that differences in cytokine profile may contribute to the pathogenesis of MS (178)
The complement system is involved in both SLE and T1D. The presence of C1q deficiency in at-risk SLE individuals, together with increased IgG : IgM anti-dsDNA ratio, may be indicative of disease development (179). C4d has been found to be increased in the pancreas of 25% of T1D patients, while in non-diabetic individuals this percentage decreases to 7% of T1D associated autoantibody positive and 2% of autoantibody negative individuals (180).
Preclinical Signs and Symptoms
In RA, autoantibody production precedes the first disease manifestations by years (158). In time, the low lover inflammation and ACPA and/or RF titers increase, followed by non-specific musculoskeletal symptoms (39). Other common symptoms in pre-RA are arthralgia, fatigue, reduced mental health due to limited functionality and work absence, and non-articular manifestations, such as cardiovascular diseases (181, 182). More than 60% of seropositive individuals tend to have pain, stiffness and swelling of the joint, and nearly 30% had joint tenderness, even before RA onset (40). Another study showed an increased frequency of primary care visits for musculoskeletal symptoms, infections and comorbidities in the years prior to the diagnosis of IA (183).
As explained above, the identification of a preclinical stage of SLE and the diagnosis itself is complicated by the need of sufficient clinical manifestation and the time elapse that this entails. Individuals in this phase may experience joint or skin symptoms for several years, associated with ANA and anti-DNA antibody production.
During the prodromal phase of MS decreased cognitive performance, fatigue, pain, depression, anxiety, bowel, and bladder disorders are more often reported in the 5 years before the diagnosis of MS. Individuals in the prodromal phase are also more likely to seek healthcare and present health deterioration 5-10 years before the first clinical event (96, 98, 184). Nearly one third of RIS individuals develop MS-related neurological symptoms within 5 years. Age younger than 35 years old, male gender, thoracic or cervical spinal cord lesion, and the presence of oligoclonal bands in the cerebrospinal fluid are major predictors of RIS conversion to MS (98, 185).
The preclinical phase of T1D can be divided into two stages, with an initial stage of immune recognition and antibody production, beta cell and pancreatic destruction, followed by an exacerbation of islet destruction that leads to insulin production impairment and dysglycaemia (15). Functional tests are able to detect an impairment of insulin production and dysregulation of glucose metabolism in the preclinical phase, however specific signs or symptoms are only shown with manifest hyperglycemia, the clinical stage (15, 186, 187).
Discussion
The necessarily incomplete overview of the preclinical phase of four distinct autoimmune diseases presented in this narrative review naturally highlights several differences in pathophysiology and clinical manifestations, but also shows that many of their etiologic and pathophysiological features actually overlap. The picture that emerges of these autoimmune diseases is that of a genetically determined increased sensitivity to breach immune tolerance to certain body parts, that is triggered under the influence of often multiple environmental factors during many years. Highly prevalent environmental factors such as smoking, obesity and stress are related to all four of these diseases and are known in general to produce a state of chronic systemic low grade inflammation (1). Thus, although the genetic basis and clinical features of the diseases are quite specific, the trigger for their manifestation in many cases is quite general.
The preclinical or prodromal phase of these diseases is characterized by nonspecific symptoms and in some cases more specific signs of autoimmunity at laboratory testing, which increase towards the onset of clinically manifest disease and subsequent diagnosis. Thus a high risk of future clinical disease can mostly be measured accurately only shortly, typically in the last year or so, before onset of clinical disease. Such a high risk of imminent disease then provides the setting in which preventive interventions with drug therapy could be tested, a situation resembling very early treatment of the same disease.
Attempts at prevention at an earlier stage would then involve interventions directed at life style factors. However, since it is difficult to identify individuals with an only slightly increased risk for autoimmune diseases, preventive efforts for autoimmune diseases would then become part of the public health domain. Indeed, increased public health or legislation actions to reduce smoking and obesity, as well as other unhealthy behaviors, while being completely nonspecific, could have a huge impact on the incidence and burden of not only the autoimmune diseases discussed here, but chronic non-communicable diseases in general. Meanwhile, physicians treating persons with increased risk of these diseases will have to await further advances in the prediction of clinical disease and in the (cost-)effectiveness of preventive therapy in high risk individuals.
Author Contributions
All authors listed have made a substantial, direct, and intellectual contribution to the work, and approved it for publication.
Funding
The project has received funding from the European Union’s Horizon 2020 research and innovation programme under the Marie Skłodowska-Curie grant agreement no. 847551 (GF).
Conflict of Interest
The authors declare that the research was conducted in the absence of any commercial or financial relationships that could be construed as a potential conflict of interest.
Box 1. Search strategy and selection – We searched MEDLINE for publications in English using the terms “rheumatoid arthritis”, “systemic lupus erythematosus”, “multiple sclerosis”, and “type 1 diabetes”, “risk factors”, “preclinical”, “prodromal”, “asymptomatic”, and MEDLINE subheadings. We selected articles based on our opinion of their scientific importance. We focused on original research articles, and selected reviews from highly authorative journals. We provide an overview of four autoimmune diseases, comparing their similarities and differences in their preclinical stage.
Publisher’s Note
All claims expressed in this article are solely those of the authors and do not necessarily represent those of their affiliated organizations, or those of the publisher, the editors and the reviewers. Any product that may be evaluated in this article, or claim that may be made by its manufacturer, is not guaranteed or endorsed by the publisher.
Acknowledgments
We are grateful to Remke Albers for providing expert assistance with the literature review.
References
1. Furman D, Campisi J, Verdin E, Carrera-Bastos P, Targ S, Franceschi C, et al. Chronic Inflammation in the Etiology of Disease Across the Life Span. Nat Med (2019) 25(12):1822–32. doi: 10.1038/s41591-019-0675-0
2. Bach JF. The Hygiene Hypothesis in Autoimmunity: The Role of Pathogens and Commensals. Nat Rev Immunol (2018) 18(2):105–20. doi: 10.1038/nri.2017.111
3. Koch-Henriksen N, Sørensen PS. The Changing Demographic Pattern of Multiple Sclerosis Epidemiology. Lancet Neurol (2010) 9(5):520–32. doi: 10.1016/S1474-4422(10)70064-8
4. Myasoedova E, Crowson CS, Kremers HM, Therneau TM, Gabriel SE. Is the Incidence of Rheumatoid Arthritis Rising? Results From Olmsted County, Minnesota, 1955-2007. Arthritis Rheum (2010) 62(6):1576–82. doi: 10.1002/art.27425
5. Handel AE, Handunnetthi L, Ebers GC, Ramaǵopalan SV. Type 1 Diabetes Mellitus and Multiple Sclerosis: Common Etiological Features. Nat Rev Endocrinol (2009) 5(12):655–64. doi: 10.1038/nrendo.2009.216
6. Tettey P, Simpson S, Taylor BV, van der Mei IAF. The Co-Occurrence of Multiple Sclerosis and Type 1 Diabetes: Shared Aetiologic Features and Clinical Implication for MS Aetiology. J Neurol Sci (2015) 348(1-2):126–31. doi: 10.1016/j.jns.2014.11.019
7. Doran MF, Pond GR, Crowson CS, O’Fallon WM, Gabriel SE. Trends in Incidence and Mortality in Rheumatoid Arthritis in Rochester, Minnesota, Over a Forty-Year Period. Arthritis Rheum (2002) 46(3):625–31. doi: 10.1002/art.509
8. Rossini M, Rossi E, Bernardi D, Viapiana O, Gatti D, Idolazzi L, et al. Prevalence and Incidence of Rheumatoid Arthritis in Italy. Rheumatol Int (2014) 34(5):659–64. doi: 10.1007/s00296-014-2974-6
9. Plenge RM, Padyukov L, Remmers EF, Purcell S, Lee AT, Karlson EW, et al. Replication of Putative Candidate-Gene Associations With Rheumatoid Arthritis in >4,000 Samples From North America and Sweden: Association of Susceptibility With PTPN22, CTLA4, and PADI4. Am J Hum Genet (2005) 77(6):1044–60. doi: 10.1086/498651
10. Burgers LE, Van Steenbergen HW, Ten Brinck RM, Huizinga TWJ, van der Helm-van Mil AHM. Differences in the Symptomatic Phase Preceding ACPA-Positive and ACPA-Negative RA: A Longitudinal Study in Arthralgia During Progression to Clinical Arthritis. Ann Rheum Dis (2017) 76(10):1751–4. doi: 10.1136/annrheumdis-2017-211325
11. Burton PR, Clayton DG, Cardon LR, Craddock N, Deloukas P, Duncanson A, et al. The Wellcome Trust Case Control Consortium. Genome-Wide Association Study of 14,000 Cases of Seven Common Diseases and 3,000 Shared Controls. Nature (2007) 447(7145):661–78. doi: 10.1038/nature05911
12. Hemminki K, Li X, Sundquist J, Sundquist K. Familial Associations of Rheumatoid Arthritis With Autoimmune Diseases and Related Conditions. Arthritis Rheum (2009) 60(3):661–8. doi: 10.1002/art.24328
13. Kim K, Bang SY, Lee HS, Bae SC. Update on the Genetic Architecture of Rheumatoid Arthritis. Nat Rev Rheumatol (2017) 13(1):13-24. doi: 10.1038/nrrheum.2016.176
14. Muskardin TLW, Niewold TB. Type I Interferon in Rheumatic Diseases. Nat Rev Rheumatol (2018) 14(4):214–28. doi: 10.1038/nrrheum.2018.31
15. Primavera M, Giannini C, Chiarelli F. Prediction and Prevention of Type 1 Diabetes. Front Endocrinol (2020) 15:11. doi: 10.3389/fendo.2020.00248
16. Bos WH, Wolbink GJ, Boers M, Tijhuis GJ, De Vries N, van der Horst-Bruinsma IE, et al. Arthritis Development in Patients With Arthralgia is Strongly Associated With Anti-Citrullinated Protein Antibody Status: A Prospective Cohort Study. Ann Rheum Dis (2010) 69(3):490–4. doi: 10.1136/ard.2008.105759
17. Lundstrüom E, Källberg H, Alfredsson L, Klareskog L, Padyukov L. Gene-Environment Interaction Between the DRB1 Shared Epitope and Smoking in the Risk of Anti-Citrullinated Protein Antibody-Positive Rheumatoid Arthritis: All Alleles are Important. Arthritis Rheum (2009) 60(6):1597–603. doi: 10.1002/art.24572
18. Suzuki A, Yamada R, Chang X, Tokuhiro S, Sawada T, Suzuki M, et al. Functional Haplotypes of PADI4, Encoding Citrullinating Enzyme Peptidylarginine Deiminase 4, are Associated With Rheumatoid Arthritis. Nat Genet (2003) 34(4):395–402. doi: 10.1038/ng1206
19. van Vollenhoven RF. Sex Differences in Rheumatoid Arthritis: More Than Meets the Eye. BMC Med (2009) 7:12. doi: 10.1186/1741-7015-7-12
20. Heliovaara M, Aho K, Aromaa A, Knekt P, Reunanen A. Smoking and Risk of Rheumatoid Arthritis. J Rheumatol (1993) 33(4):652–8.
21. Silman AJ, Newman J, MacGregor AJ. Cigarette Smoking Increases the Risk of Rheumatoid Arthritis: Results From a Nationwide Study of Disease-Discordant Twins. Arthritis Rheum (1996) 39(5):732–5. doi: 10.1002/art.1780390504
22. Liu X, Tedeschi SK, Barbhaiya M, Leatherwood CL, Speyer CB, Lu B, et al. Impact and Timing of Smoking Cessation on Reducing Risk of Rheumatoid Arthritis Among Women in the Nurses’ Health Studies. Arthritis Care Res (2019) 71(7):914–4. doi: 10.1002/acr.23837
23. Padyukov L, Suva C, Stolt P, Alfredsson L, Klareskog L. A Gene-Environment Interaction Between Smoking and Shared Epitope Genes in HLA-DR Provides a High Risk of Seropositive Rheumatoid Arthritis. Arthritis Rheumatol (2004) 50(10):3085–92. doi: 10.1002/art.20553
24. Van Der Helm-Van Mil AHM, Verpoort KN, Le CS, Huizinga TWJ, De Vries RRP, Toes REM. The HLA-DRB1 Shared Epitope Alleles Differ in the Interaction With Smoking and Predisposition to Antibodies to Cyclic Citrullinated Peptide. Arthritis Rheum (2007) 56(2):425–32. doi: 10.1002/art.22373
25. Stolt P, Bengtsson C, Nordmark B, Lindblad S, Lundberg I, Klareskog L, et al. Quantification of the Influence of Cigarette Smoking on Rheumatoid Arthritis: Results From a Population Based Case-Control Study, Using Incident Cases. Ann Rheum Dis (2003) 62(9):835–41. doi: 10.1136/ard.62.9.835
26. Yahya A, Bengtsson C, Larsson P, Too CL, Mustafa AN, Abdullah NA, et al. Silica Exposure is Associated With an Increased Risk of Developing ACPA-Positive Rheumatoid Arthritis in an Asian Population: Evidence From the Malaysian MyEIRA Case–Control Study. Mod Rheumatol (2013). doi: 10.1007/s10165-013-0890-3
27. Too CL, Muhamad NA, Ilar A, Padyukov L, Alfredsson L, Klareskog L, et al. Occupational Exposure to Textile Dust Increases the Risk of Rheumatoid Arthritis: Results From a Malaysian Population-Based Case-Control Study. Ann Rheum Dis (2016) 75(6):997–1002. doi: 10.1136/annrheumdis-2015-208278
28. Song H, Fang F, Tomasson G, Arnberg FK, Mataix-Cols D, de la Cruz LF, et al. Association of Stress-Related Disorders With Subsequent Autoimmune Disease. JAMA (2018) 319(23):2388–400. doi: 10.1001/jama.2018.7028
29. Di Giuseppe D, Bottai M, Askling J, Wolk A. Physical Activity and Risk of Rheumatoid Arthritis in Women: A Population-Based Prospective Study. Arthritis Res Ther (2015) 17(1):40. doi: 10.1186/s13075-015-0560-2
30. De Jong Z, Munneke M, Zwinderman AH, Kroon HM, Jansen A, Ronday KH, et al. Is a Long-Term High-Intensity Exercise Program Effective and Safe in Patients With Rheumatoid Arthritis? Results of a Randomized Controlled Trial. Arthritis Rheum (2003) 48(9):2415–24. doi: 10.1002/art.11216
31. Hurkmans E, van der Giesen FJ, Vlieland TPMV, Schoones J, Van Den Ende ECHM. Dynamic Exercise Programs (Aerobic Capacity and/or Muscle Strength Training) in Patients With Rheumatoid Arthritis. Cochrane Database Systematic Rev (2009) 2009(4):CD006853. doi: 10.1002/14651858.CD006853.pub2
32. Hu Y, Sparks JA, Malspeis S, Costenbader KH, Hu FB, Karlson EW, et al. Long-Term Dietary Quality and Risk of Developing Rheumatoid Arthritis in Women. Ann Rheum Dis (2017) 76(8):1357–64. doi: 10.1136/annrheumdis-2016-210431
33. Lahiri M, Luben RN, Morgan C, Bunn DK, Marshall T, Lunt M, et al. Using Lifestyle Factors to Identify Individuals at Higher Risk of Inflammatory Polyarthritis (Results From the European Prospective Investigation of Cancer-Norfolk and the Norfolk Arthritis Register-The EPIC-2-NOAR Study). Ann Rheum Dis (2014) 73(1):219–26. doi: 10.1136/annrheumdis-2012-202481
34. Jacobsson LTH, Jacobsson ME, Askling J, Knowler WC. Perinatal Characteristics and Risk of Rheumatoid Arthritis. Br Med J (2003) 326(7398):1068–9. doi: 10.1136/bmj.326.7398.1068
35. Mandl LA, Costenbader KH, Simard JF, Karlson EW. Is Birthweight Associated With Risk of Rheumatoid Arthritis? Data From a Large Cohort Study. Ann Rheum Dis (2009) 68(4):514–8. doi: 10.1136/ard.2007.080937
36. Nielen MMJ, Van Schaardenburg D, Reesink HW, Twisk JWR, Van De Stadt RJ, van der Horst-Bruinsma IE, et al. Increased Levels of C-Reactive Protein in Serum From Blood Donors Before the Onset of Rheumatoid Arthritis. Arthritis Rheum (2004) 50(8):2423–7. doi: 10.1002/art.20431
37. Rantapää-Dahlqvist S, De Jong BAW, Berglin E, Hallmans G, Wadell G, Stenlund H, et al. Antibodies Against Cyclic Citrullinated Peptide and IgA Rheumatoid Factor Predict the Development of Rheumatoid Arthritis. Arthritis Rheum (2003) 48(10):2741–9. doi: 10.1002/art.11223
38. Van De Stadt LA, Witte BI, Bos WH, Van Schaardenburg D. A Prediction Rule for the Development of Arthritis in Seropositive Arthralgia Patients. Ann Rheum Dis (2013) 72(12):1920–6. doi: 10.1136/annrheumdis-2012-202127
39. Rakieh C, Nam J L, Hunt L, Hensor EMA, Das S, Bissell LA, et al. Predicting the Development of Clinical Arthritis in Anti-CCP Positive Individuals With non-Specific Musculoskeletal Symptoms: A Prospective Observational Cohort Study. Ann Rheum Dis (2015) 74(9):1659–66. doi: 10.1136/annrheumdis-2014-205227
40. Bemis EA, Demoruelle MK, Seifert JA, Polinski KJ, Weisman MH, Buckner JH, et al. Factors Associated With Progression to Inflammatory Arthritis in First-Degree Relatives of Individuals With RA Following Autoantibody Positive Screening in a non-Clinical Setting. Ann Rheum Dis (2021) 80(2):154–61. doi: 10.1136/annrheumdis-2020-217066
41. Brink M, Hansson M, Mathsson L, Jakobsson PJ, Holmdahl R, Stenlund H, et al. Multiplex Analyses of Antibodies Against Citrullinated Peptides in Individuals Prior to Development of Rheumatoid Arthritis. Arthritis Rheum (2013) 65(4):899–910. doi: 10.1002/art.37835
42. Sokolove J, Bromberg R, Deane KD, Lahey LJ, Derber LA, Chandra PE, et al. Autoantibody Epitope Spreading in the Pre-Clinical Phase Predicts Progression to Rheumatoid Arthritis. PLoS One (2012) 7(5):e35296. doi: 10.1371/annotation/2e462817-ab93-4d78-95a4-1d8b9d172971
43. Van De Stadt LA, De Koning MHMT, Van De Stadt RJ, Wolbink G, Dijkmans BAC, Hamann D, et al. Development of the Anti-Citrullinated Protein Antibody Repertoire Prior to the Onset of Rheumatoid Arthritis. Arthritis Rheum (2011) 63(11):3226-33. doi: 10.1002/art.30537
44. Verheul MK, Böhringer S, van Delft MAM, Jones JD, Rigby WFC, Gan RW, et al. Triple Positivity for Anti–Citrullinated Protein Autoantibodies, Rheumatoid Factor, and Anti–Carbamylated Protein Antibodies Conferring High Specificity for Rheumatoid Arthritis: Implications for Very Early Identification of At-Risk Individuals. Arthritis Rheumatol (2018) 70(11):1721–1731. doi: 10.1002/art.40562
45. Grönwall C, Liljefors L, Bang H, Hensvold AH, Hansson M, Mathsson-Alm L, et al. A Comprehensive Evaluation of the Relationship Between Different IgG and IgA Anti-Modified Protein Autoantibodies in Rheumatoid Arthritis. Front Immunol (2021) 12. doi: 10.3389/fimmu.2021.627986
46. Gan RW, Trouw LA, Shi J, Toes REM, Huizinga TWJ, Demoruelle MK, et al. Anti-Carbamylated Protein Antibodies are Present Prior to Rheumatoid Arthritis and are Associated With its Future Diagnosis. J Rheumatol (2015) 42(4):572–9. doi: 10.3899/jrheum.140767
47. Kolfenbach JR, Deane KD, Derber LA, O’Donnell CI, Gilliland WR, Edison JD, et al. Autoimmunity to Peptidyl Arginine Deiminase Type 4 Precedes Clinical Onset of Rheumatoid Arthritis. Arthritis Rheum (2010) 62(9):2633–9. doi: 10.1002/art.27570
48. Juarez M, Bang H, Hammar F, Reimer U, Dyke B, Sahbudin I, et al. Identification of Novel Antiacetylated Vimentin Antibodies in Patients With Early Inflammatory Arthritis. Ann Rheum Dis (2016) 75(6):1099–107. doi: 10.1136/annrheumdis-2014-206785
49. Kerkman PF, Rombouts Y, van der Voort EIH, Trouw LA, Huizinga TWJ, Toes REM, et al. Circulating Plasmablasts/Plasmacells as a Source of Anticitrullinated Protein Antibodies in Patients With Rheumatoid Arthritis. Ann Rheum Dis (2013) 72(7):1259–63. doi: 10.1136/annrheumdis-2012-202893
50. Hafkenscheid L, de Moel E, Smolik I, Tanner S, Meng X, Jansen BC, et al. N-Linked Glycans in the Variable Domain of IgG Anti–Citrullinated Protein Antibodies Predict the Development of Rheumatoid Arthritis. Arthritis Rheumatol (2019) 71(10):1626–1633. doi: 10.1002/art.40920
51. Rombouts Y, Ewing E, Van De Stadt LA, Selman MHJ, Trouw LA, Deelder AM, et al. Anti-Citrullinated Protein Antibodies Acquire a Pro-Inflammatory Fc Glycosylation Phenotype Prior to the Onset of Rheumatoid Arthritis. Ann Rheum Dis (2015) 74(1):234–41. doi: 10.1136/annrheumdis-2013-203565
52. Makrygiannakis D, Hermansson M, Ulfgren AK, Nicholas AP, Zendman AJW, Eklund A, et al. Smoking Increases Peptidylarginine Deiminase 2 Enzyme Expression in Human Lungs and Increases Citrullination in BAL Cells. Ann Rheum Dis (2008) 67(10):1488–92. doi: 10.1136/ard.2007.075192
53. Kokkonen H, Söderström I, Rocklöv J, Hallmans G, Lejon K, Dahlqvist SR. Up-Regulation of Cytokines and Chemokines Predates the Onset of Rheumatoid Arthritis. Arthritis Rheumatol (2010) 62(2):383–91. doi: 10.1002/art.27186
54. Nielen MMJ, Van Schaardenburg D, Reesink HW, Twisk JWR, Van De Stadt RJ, van der Horst-Bruinsma IE, et al. Simultaneous Development of Acute Phase Response and Autoantibodies in Preclinical Rheumatoid Arthritis. Ann Rheum Dis (2006) 65(4):535–7. doi: 10.1136/ard.2005.040659
55. Polinski KJ, Bemis EA, Yang F, Crume T, Demoruelle MK, Feser M, et al. Association of Lipid Mediators With Development of Future Incident Inflammatory Arthritis in an Anti–Citrullinated Protein Antibody–Positive Population. Arthritis Rheumatol (2021) 73(6):955–962. doi: 10.1002/art.41631
56. Gan RW, Bemis EA, Demoruelle MK, Striebich CC, Brake S, Feser ML, et al. The Association Between Omega-3 Fatty Acid Biomarkers and Inflammatory Arthritis in an Anti-Citrullinated Protein Antibody Positive Population. Rheumatol (United Kingdom) (2017) 56(12):2229–2236. doi: 10.1093/rheumatology/kex360
57. Van Steenbergen HW, Van Nies JAB, Huizinga TWJ, Bloem JL, Reijnierse M, van der Helm-Van Mil AHM, et al. Characterising Arthralgia in the Preclinical Phase of Rheumatoid Arthritis Using MRI. Ann Rheum Dis (2015) 74(6):1225–32. doi: 10.1136/annrheumdis-2014-205522
58. van der Ven M, van der Veer-Meerkerk M, Ten Cate DF, Rasappu N, Kok MR, Csakvari D, et al. Absence of Ultrasound Inflammation in Patients Presenting With Arthralgia Rules Out the Development of Arthritis. Arthritis Res Ther (2017) 19(1):202. doi: 10.1186/s13075-017-1405-y
59. Van Steenbergen HW, Mangnus L, Reijnierse M, Huizinga TWJ, van der Helm-Van Mil AHM. Clinical Factors, Anticitrullinated Peptide Antibodies and MRI-Detected Subclinical Inflammation in Relation to Progression From Clinically Suspect Arthralgia to Arthritis. Ann Rheum Dis (2016) 75(10):1824–30. doi: 10.1136/annrheumdis-2015-208138
60. Gent YYJ, Voskuyl AE, Kloet RW, Van Schaardenburg D, Hoekstra OS, Dijkmans BAC, et al. Macrophage Positron Emission Tomography Imaging as a Biomarker for Preclinical Rheumatoid Arthritis: Findings of a Prospective Pilot Study. Arthritis Rheum (2012) 64(1):62–6. doi: 10.1002/art.30655
61. Van De Sande MGH, De Hair MJH, van der Leij C, Klarenbeek PL, Bos WH, Smith MD, et al. Different Stages of Rheumatoid Arthritis: Features of the Synovium in the Preclinical Phase. Ann Rheum Dis (2011) 70(5):772–7. doi: 10.1136/ard.2010.139527
62. Scherer HU, Häupl T, Burmester GR. The Etiology of Rheumatoid Arthritis. J Autoimmun (2020) 110:102400. doi: 10.1016/j.jaut.2019.102400
63. Semb AG, Ikdahl E, Wibetoe G, Crowson C, Rollefstad S. Atherosclerotic Cardiovascular Disease Prevention in Rheumatoid Arthritis. Nat Rev Rheumatol (2020) 16(7):361–379. doi: 10.1038/s41584-020-0428-y
64. Pappas DA, Nyberg F, Kremer JM, Lampl K, Reed GW, Horne L, et al. Prevalence of Cardiovascular Disease and Major Risk Factors in Patients With Rheumatoid Arthritis: A Multinational Cross-Sectional Study. Clin Rheumatol (2018) 15(4):R96. doi: 10.1007/s10067-018-4113-3
65. Feldman CH, Hiraki LT, Liu J, Fischer MA, Solomon DH, Alarcõn GS, et al. Epidemiology and Sociodemographics of Systemic Lupus Erythematosus and Lupus Nephritis Among US Adults With Medicaid Coverage, 2000-2004. Arthritis Rheum (2013) 65(3):753–63. doi: 10.1002/art.37795
66. Deafen D, Escalante A, Weinrib L, Horwitz D, Bachman B, Roy-Burman P, et al. A Revised Estimate of Twin Concordance in Systemic Lupus Erythematosus. Arthritis Rheum (1992) 35(3):311–8. doi: 10.1002/art.1780350310
67. Graham RR, Ortmann WA, Langefeld CD, Jawaheer D, Selby SA, Rodine PR, et al. Visualizing Human Leukocyte Antigen Class II Risk Haplotypes in Human Systemic Lupus Erythematosus. Am J Hum Genet (2002) 71(3):543–53. doi: 10.1086/342290
68. Tsuchiya N, Kawasaki A, Tsao BP, Komata T, Grossman JM, Tokunaga K. Analysis of the Association of HLA-DRB1, Tnfα Promoter and TNFR2 (TNFRSF1B) Polymorphisms With SLE Using Transmission Disequilibrium Test. Genes Immun (2001) 2(6):317–22. doi: 10.1038/sj.gene.6363783
69. Salmon JE, Millard S, Schachter LA, Arnett FC, Ginzler EM, Gourley MF, et al. Fcγriia Alleles are Heritable Risk Factors for Lupus Nephritis in African Americans. J Clin Invest (1996) 97(5):1348–54. doi: 10.1172/JCI118552
70. Duits AJ, Bootsma H, Derksen RHWM, Spronk PE, Kater L, Kallenberg CGM, et al. Skewed Distribution of IGG FC Receptor Iia (CD32) Polymorphism is Associated With Renal Disease in Systemic Lupus Erythematosus Patients. Arthritis Rheum (1995) 38(12):1832–6. doi: 10.1002/art.1780381217
71. Lee HS, Chung YH, Kim TG, Kim TH, Jun JB, Jung S, et al. Independent Association of HLA-DR and Fcγ Receptor Polymorphisms in Korean Patients With Systemic Lupus Erythematosus. Rheumatology (2003) 42(12):1501–7. doi: 10.1093/rheumatology/keg404
72. Graham DS, Morris DL, Bhangale TR, Criswell LA, Syvänen AC, Rönnblom L, et al. Association of NCF2, IKZF1, IRF8, IFIH1, and TYK2 With Systemic Lupus Erythematosus. PLoS Genet (2011) 7(10):e1002341. doi: 10.1371/journal.pgen.1002341
73. Raafat II, El Guindy N, Shahin RMH, Samy LA, El Refai RM. Toll-Like Receptor 7 Gene Single Nucleotide Polymorphisms and the Risk for Systemic Lupus Erythematosus: A Case-Control Study. Z fur Rheumatologie (2018) 13(2):R41. doi: 10.1007/s00393-017-0283-7
74. Somers EC, Marder W, Cagnoli P, Lewis EE, DeGuire P, Gordon C, et al. Population-Based Incidence and Prevalence of Systemic Lupus Erythematosus: The Michigan Lupus Epidemiology and Surveillance Program. Arthritis Rheumatol (2014) 66(2):369–78. doi: 10.1002/art.38238
75. Chakravarty EF, Bush TM, Manzi S, Clarke AE, Ward MM. Prevalence of Adult Systemic Lupus Erythematosus in California and Pennsylvania in 2000: Estimates Obtained Using Hospitalization Data. Arthritis Rheum (2007) 56(6):2092–4. doi: 10.1002/art.22641
76. Choi MY, Hahn J, Malspeis S, Stevens EF, Karlson EW, Sparks JA, et al. A Combination of Healthy Lifestyle Behaviors Reduces Risk of Incident Systemic Lupus Erythematosus. Arthritis Rheumatol (2021) 74(2):274–283. doi: 10.1002/art.41935
77. Barbhaiya M, Tedeschi SK, Lu B, Malspeis S, Kreps D, Sparks JA, et al. Cigarette Smoking and the Risk of Systemic Lupus Erythematosus, Overall and by Anti-Double Stranded DNA Antibody Subtype, in the Nurses’ Health Study Cohorts. Ann Rheum Dis (2018) 77(2):196–202. doi: 10.1136/annrheumdis-2017-211675
78. Schoindre Y, Jallouli M, Tanguy ML, Ghillani P, Galicier L, Aumaître O, et al. Lower Vitamin D Levels are Associated With Higher Systemic Lupus Erythematosus Activity, But Not Predictive of Disease Flare-Up. Lupus Sci Med (2014) 1(1):e000027. doi: 10.1136/lupus-2014-000027
79. Young KA, Munroe ME, Guthridge JM, Kamen DL, Niewold TB, Gilkeson GS, et al. Combined Role of Vitamin D Status and CYP24A1 in the Transition to Systemic Lupus Erythematosus. Ann Rheum Dis (2017) 76(1):153–158. doi: 10.1136/annrheumdis-2016-209157
80. Young KA, Munroe ME, Harley JB, Guthridge JM, Kamen DL, Gilkensen GS, et al. Less Than 7 Hours of Sleep Per Night is Associated With Transitioning to Systemic Lupus Erythematosus. Lupus (2018) 27(9):1524–1531. doi: 10.1177/0961203318778368
81. Sassi RH, Hendler JV, Piccoli GF, Gasparin AA, da Silva Chakr RM, Brenol JCT, et al. Age of Onset Influences on Clinical and Laboratory Profile of Patients With Systemic Lupus Erythematosus. Clin Rheumatol (2017) 36(1):89–95. doi: 10.1007/s10067-016-3478-4
82. Ohta A, Nagai M, Nishina M, Tomimitsu H, Kohsaka H. Age at Onset and Gender Distribution of Systemic Lupus Erythematosus, Polymyositis/Dermatomyositis, and Systemic Sclerosis in Japan. Mod Rheumatol (2013) 23(4):759–64. doi: 10.3109/s10165-012-0733-7
83. Arbuckle MR, McClain MT, Rubertone MV, Scofield RH, Dennis GJ, James JA, et al. Development of Autoantibodies Before the Clinical Onset of Systemic Lupus Erythematosus. N Engl J Med (2003) 349(16):1526–33. doi: 10.1056/NEJMoa021933
84. Lu R, Munroe ME, Guthridge JM, Bean KM, Fife DA, Chen H, et al. Dysregulation of Innate and Adaptive Serum Mediators Precedes Systemic Lupus Erythematosus Classification and Improves Prognostic Accuracy of Autoantibodies. J Autoimmun (2016) 74:182–193. doi: 10.1016/j.jaut.2016.06.001
85. Slight-Webb S, Lu R, Ritterhouse LL, Munroe ME, Maecker HT, Fathman CG, et al. Autoantibody-Positive Healthy Individuals Display Unique Immune Profiles That May Regulate Autoimmunity. Arthritis Rheumatol (2016) 68(10):2492–502. doi: 10.1002/art.39706
86. Hagan KA, Munger KL, Ascherio A, Grodstein F. Epidemiology of Major Neurodegenerative Diseases in Women: Contribution of the Nurses’ Health Study. Am J Public Health (2016) 106(9):1650–5. doi: 10.2105/AJPH.2016.303324
87. Ascherio A, Munger KL, Lünemann JD. The Initiation and Prevention of Multiple Sclerosis. Nat Rev Neurol (2012) 8(11):602–12. doi: 10.1038/nrneurol.2012.198
88. Compston A, Coles A. Multiple Sclerosis. Lancet (2008) 372(9648):1502–17. doi: 10.1016/S0140-6736(08)61620-7
89. Liguori M, Nuzziello N, Licciulli F, Consiglio A, Simone M, Viterbo RG, et al. Combined microRNA and mRNA Expression Analysis in Pediatric Multiple Sclerosis: An Integrated Approach to Uncover Novel Pathogenic Mechanisms of the Disease. Hum Mol Genet (2018) 27(1):66–79. doi: 10.1093/hmg/ddx385
90. Bjornevik K, Cortese M, Healy BC, Kuhle J, Mina MJ, Leng Y, et al. Longitudinal Analysis Reveals High Prevalence of Epstein-Barr Virus Associated With Multiple Sclerosis. Science (2022) 375(6578):296–301. doi: 10.1126/science.abj8222
91. Giovannoni G, Hawkes CH, Lechner-Scott J, Levy M, Yeh EA, Gold J. Is EBV the Cause of Multiple Sclerosis? Mult Scler Relat Disord (2022) 58:103636. doi: 10.1016/j.msard.2022.103636
92. Thompson AJ, Baranzini SE, Geurts J, Hemmer B, Ciccarelli O. Multiple Sclerosis. Lancet (2018) 391(10130):1622–1636. doi: 10.1016/S0140-6736(18)30481-1
93. Lanz TV, Brewer RC, Ho PP, Moon J-S, Jude KM, Fernandez D, et al. Clonally Expanded B Cells in Multiple Sclerosis Bind EBV EBNA1 and GlialCAM. Nature (2022) 603(7900):321–327. doi: 10.21203/rs.3.rs-1239863/v1
94. Beltrán E, Gerdes LA, Hansen J, Flierl-Hecht A, Krebs S, Blum H, et al. Early Adaptive Immune Activation Detected in Monozygotic Twins With Prodromal Multiple Sclerosis. J Clin Invest (2019) 129(11):4758–4768. doi: 10.1172/JCI128475
95. Giovannoni G. The Neurodegenerative Prodrome in Multiple Sclerosis. Lancet Neurol (2017) 16(6):413–414. doi: 10.1016/S1474-4422(17)30127-8
96. Tremlett H, Munger KL, Makhani N. The Multiple Sclerosis Prodrome: Evidence to Action. Front Neurol (2022) 12. doi: 10.3389/fneur.2021.761408
97. Okuda DT, Mowry EM, Beheshtian A, Waubant E, Baranzini SE, Goodin DS, et al. Incidental MRI Anomalies Suggestive of Multiple Sclerosis: The Radiologically Isolated Syndrome. Neurology (2009) 72(9):800–5. doi: 10.1212/01.wnl.0000335764.14513.1a
98. Bjornevik K, Munger KL, Cortese M, Barro C, Healy BC, Niebuhr DW, et al. Serum Neurofilament Light Chain Levels in Patients With Presymptomatic Multiple Sclerosis. JAMA Neurol (2020) 77(1):58–64. doi: 10.1001/jamaneurol.2019.3238
99. Crawford MP, Yan SX, Ortega SB, Mehta RS, Hewitt RE, Price DA, et al. High Prevalence of Autoreactive, Neuroantigen-Specific CD8+ T Cells in Multiple Sclerosis Revealed by Novel Flow Cytometric Assay. Blood (2004) 103(11):4222–31. doi: 10.1182/blood-2003-11-4025
100. Baranzini SE, Jeong MC, Butunoi C, Murray RS, Bernard CC, Oksenberg JR. B Cell Repertoire Diversity and Clonal Expansion in Multiple Sclerosis Brain Lesions. J Immunol (1999) 163(9):5133–44.
101. Rewers M, Ludvigsson J. Environmental Risk Factors for Type 1 Diabetes. Lancet (2016) 387(10035):2340–2348 doi: 10.1016/S0140-6736(16)30507-4
102. Hyttinen V, Kaprio J, Kinnunen L, Koskenvuo M, Tuomilehto J. Genetic Liability of Type 1 Diabetes and the Onset Age Among 22, 650 Young Finnish Twin Pairs: A Nationwide Follow-Up Study. Diabetes (2003) 52(4):1052–5. doi: 10.2337/diabetes.52.4.1052
103. Krischer JP, Lynch KF, Schatz DA, Ilonen J, Lernmark Å, Hagopian WA, et al. The 6 Year Incidence of Diabetes-Associated Autoantibodies in Genetically at-Risk Children: The TEDDY Study. Diabetologia (2015) 58(5):980–7. doi: 10.1007/s00125-015-3514-y
104. Variation and Trends in Incidence of Childhood Diabetes in Europe. Lancet (2000) 355(9207):873–6. doi: 10.1016/S0140-6736(99)07125-1
105. Dabelea D, Bell RA, D’Agostino RB, Imperatore G, Johansen JM, Linder B, et al. Incidence of Diabetes in Youth in the United States. J Am Med Assoc (2007) 297(24):2716–24. doi: 10.1001/jama.297.24.2716
106. Klinke DJ. Extent of Beta Cell Destruction is Important But Insufficient to Predict the Onset of Type 1 Diabetes Mellitus. PLoS One (2008) 3(1):e1374. doi: 10.1371/journal.pone.0001374
107. Eizirik DL, Colli ML, Ortis F. The Role of Inflammation in Insulitis and β-Cell Loss in Type 1 Diabetes. Nat Rev Endocrinol 2009 5. doi: 10.1038/nrendo.2009.21
108. Sims EK, Chaudhry Z, Watkins R, Syed F, Blum J, Ouyang F, et al. Elevations in the Fasting Serum Proinsulin-To-C-Peptide Ratio Precede the Onset of Type 1 Diabetes. Diabetes Care (2016) 39(9):1519–26. doi: 10.2337/dc15-2849
109. Campbell-Thompson ML, Filipp SL, Grajo JR, Nambam B, Beegle R, Middlebrooks EH, et al. Relative Pancreas Volume is Reduced in First-Degree Relatives of Patients With Type 1 Diabetes. In: Diabetes Care (2019).
110. Lehuen A, Diana J, Zaccone P, Cooke A. Immune Cell Crosstalk in Type 1 Diabetes. Nat Rev Immunol (2010) 10(7):501–13. doi: 10.1038/nri2787
111. Bosi E, Boulware DC, Becker DJ, Buckner JH, Geyer S, Gottlieb PA, et al. Impact of Age and Antibody Type on Progression From Single to Multiple Autoantibodies in Type 1 Diabetes Relatives. J Clin Endocrinol Metab (2017) 102(8):2881–2886. doi: 10.1210/jc.2017-00569
112. Ilonen J, Hammais A, Laine AP, Lempainen J, Vaarala O, Veijola R, et al. Patterns of β-Cell Autoantibody Appearance and Genetic Associations During the First Years of Life. Diabetes (2013) 62(10):3636–40. doi: 10.2337/db13-0300
113. Strollo R, Vinci C, Napoli N, Pozzilli P, Ludvigsson J, Nissim A. Antibodies to Post-Translationally Modified Insulin as a Novel Biomarker for Prediction of Type 1 Diabetes in Children. Diabetologia (2017) 60(8):1467–1474. doi: 10.1007/s00125-017-4296-1
114. Ziegler AG, Rewers M, Simell O, Simell T, Lempainen J, Steck A, et al. Seroconversion to Multiple Islet Autoantibodies and Risk of Progression to Diabetes in Children. JAMA (2013) 309(23):2473–9. doi: 10.1001/jama.2013.6285
115. Rees F, Doherty M, Grainge MJ, Lanyon P, Zhang W. The Worldwide Incidence and Prevalence of Systemic Lupus Erythematosus: A Systematic Review of Epidemiological Studies. Rheumatol (United Kingdom) (2017) 56(11):1945–1961. doi: 10.1093/rheumatology/kex260
116. Östman J, Lönnberg G, Arnqvist HJ, Blohmé G, Bolinder J, Schnell AE, et al. Gender Differences and Temporal Variation in the Incidence of Type 1 Diabetes: Results of 8012 Cases in the Nationwide Diabetes Incidence Study in Sweden 1983-2002. J Intern Med (2008) 263(4):386–94. doi: 10.1111/j.1365-2796.2007.01896.x
117. Viatte S, Plant D, Raychaudhuri S. Genetics and Epigenetics of Rheumatoid Arthritis. Nat Rev Rheumatol (2013) 9(3):141–53. doi: 10.1038/nrrheum.2012.237
118. Solomon L, Robin G, Valkenburg HA. Rheumatoid Arthritis in an Urban South African Negro Population. Ann Rheum Dis (1975) 34(2):128–35. doi: 10.1136/ard.34.2.128
119. Tani C, D’Aniello D, Sedie AD, Carli L, Cagnoni M, Possemato N, et al. Rhupus Syndrome: Assessment of its Prevalence and its Clinical and Instrumental Characteristics in a Prospective Cohort of 103 SLE Patients. Autoimmun Rev (2013) 12(4):537–41. doi: 10.1016/j.autrev.2012.09.004
120. Kronzer VL, Crowson CS, Sparks JA, Myasoedova E, Davis JM. Comorbidities As Risk Factors for Rheumatoid Arthritis and Their Accrual After Diagnosis. Mayo Clin Proc (2019) 94(12):2488–2498. doi: 10.1136/annrheumdis-2019-eular.4460
121. James JA, Chen H, Young KA, Bemis EA, Seifert J, Bourn RL, et al. Latent Autoimmunity Across Disease-Specific Boundaries in at-Risk First-Degree Relatives of SLE and RA Patients. EBioMedicine (2019) 42:76–85. doi: 10.1016/j.ebiom.2019.03.063
122. Frisell T, Saevarsdottir S, Askling J. Family History of Rheumatoid Arthritis: An Old Concept With New Developments. Nat Rev Rheumatol (2016) 12(6):335–43. doi: 10.1038/nrrheum.2016.52
123. Cornélis F, Fauré S, Martinez M, Prud’homme JF, Fritz P, Dib C, et al. New Susceptibility Locus for Rheumatoid Arthritis Suggested by a Genome-Wide Linkage Study. Proc Natl Acad Sci U S A (1998) 95(18):10746–50. doi: 10.1073/pnas.95.18.10746
124. Gregersen PK, Silver J, Winchester RJ. The Shared Epitope Hypothesis. An Approach to Understanding the Molecular Genetics of Susceptibility to Rheumatoid Arthritis. Arthritis Rheum (1987) 30(11):1205–13. doi: 10.1002/art.1780301102
125. Van Der Woude D, Houwing-Duistermaat JJ, Toes REM, Huizinga TWJ, Thomson W, Worthington J, et al. Quantitative Heritability of Anti-Citrullinated Protein Antibody-Positive and Anti-Citrullinated Protein Antibody-Negative Rheumatoid Arthritis. Arthritis Rheum (2009) 60(4):916–23. doi: 10.1002/art.24385
126. Ramos PS, Brown EE, Kimberly RP, Langefeld CD. Genetic Factors Predisposing to Systemic Lupus Erythematosus and Lupus Nephritis. Semin Nephrol (2010) 30(2):164–76. doi: 10.1016/j.semnephrol.2010.01.007
127. Saevarsdottir S, Olafsdottir TA, Ivarsdottir EV, Halldorsson GH, Gunnarsdottir K, Sigurdsson A, et al. FLT3 Stop Mutation Increases FLT3 Ligand Level and Risk of Autoimmune Thyroid Disease. Nature (2020) 584(7822):619–623. doi: 10.1038/s41586-020-2436-0
128. Törn C, Hadley D, Lee HS, Hagopian W, Lernmark Å, Simell O, et al. Role of Type 1 Diabetes- Associated Snps on Risk of Autoantibody Positivity in the TEDDY Study. Diabetes (2015) 64(5):1818–29. doi: 10.2337/db14-1497
129. Remmers EF, Plenge RM, Lee AT, Graham RR, Hom G, Behrens TW, et al. STAT4 and the Risk of Rheumatoid Arthritis and Systemic Lupus Erythematosus. N Engl J Med (2007) 357(10):977–86. doi: 10.1056/NEJMoa073003
130. Maier LM, Lowe CE, Cooper J, Downes K, Anderson DE, Severson C, et al. IL2RA Genetic Heterogeneity in Multiple Sclerosis and Type 1 Diabetes Susceptibility and Soluble Interleukin-2 Receptor Production. PLoS Genet (2009) 5(1):e1000322. doi: 10.1371/journal.pgen.1000322
131. Smyth DJ, Plagnol V, Walker NM, Cooper JD, Downes K, Yang JHM, et al. Shared and Distinct Genetic Variants in Type 1 Diabetes and Celiac Disease. N Engl J Med (2008) 359(26):2767–77. doi: 10.1056/NEJMoa0807917
132. Coenen MJH, Trynka G, Heskamp S, Franke B, van Diemen CC, Smolonska J, et al. Common and Different Genetic Background for Rheumatoid Arthritis and Coeliac Disease. Hum Mol Genet (2009) 18(21):4195–203. doi: 10.1093/hmg/ddp365
133. Morris DL, Sheng Y, Zhang Y, Wang YF, Zhu Z, Tombleson P, et al. Genome-Wide Association Meta-Analysis in Chinese and European Individuals Identifies Ten New Loci Associated With Systemic Lupus Erythematosus. Nat Genet (2016) 48(8):940–946. doi: 10.1038/ng.3603
134. Cooper JD, Smyth DJ, Smiles AM, Plagnol V, Walker NM, Allen JE, et al. Meta-Analysis of Genome-Wide Association Study Data Identifies Additional Type 1 Diabetes Risk Loci. Nat Genet (2008) 40(12):1399–401. doi: 10.1038/ng.249
135. Pociot F, Lernmark Å. Genetic Risk Factors for Type 1 Diabetes. Lancet (2016) 387(10035):2331–2339. doi: 10.1016/B978-0-12-374279-7.15001-5
136. Colditz GA, Philpott SE, Hankinson SE. The Impact of the Nurses’ Health Study on Population Health: Prevention, Translation, and Control. Am J Public Health (2016) 106(9):1540–5. doi: 10.2105/AJPH.2016.303343
137. Rewers M, Hyöty H, Lernmark Å, Hagopian W, She JX, Schatz D, et al. The Environmental Determinants of Diabetes in the Young (TEDDY) Study: 2018 Update. Curr Diabetes Rep (2018) 18(12):136. doi: 10.1007/s11892-018-1113-2
138. Klareskog L, Padyukov L, Lorentzen J, Alfredsson L. Mechanisms of Disease: Genetic Susceptibility and Environmental Triggers in the Development of Rheumatoid Arthritis. Nat Clin Pract Rheumatol (2006) 2(8):425–33. doi: 10.1038/ncprheum0249
139. Blanc PD, Järvholm B, Torén K. Prospective Risk of Rheumatologic Disease Associated With Occupational Exposure in a Cohort of Male Construction Workers. Am J Med (2015) 128(10):1094–101. doi: 10.1016/j.amjmed.2015.05.001
140. Stolt P, Källberg H, Lundberg I, Sjögren B, Klareskog L, Alfredsson L. Silica Exposure is Associated With Increased Risk of Developing Rheumatoid Arthritis: Results From the Swedish EIRA Study. Ann Rheum Dis (2005) 64(4):582–6. doi: 10.1136/ard.2004.022053
141. Ye D, Mao Y, Xu Y, Xu X, Xie Z, Wen C. Lifestyle Factors Associated With Incidence of Rheumatoid Arthritis in US Adults: Analysis of National Health and Nutrition Examination Survey Database and Meta-Analysis. BMJ Open (2021) 11(1):e038137. doi: 10.1136/bmjopen-2020-038137
142. Liu X, Tedeschi SK, Lu B, Zaccardelli A, Speyer CB, Costenbader KH, et al. Long-Term Physical Activity and Subsequent Risk for Rheumatoid Arthritis Among Women: A Prospective Cohort Study. Arthritis Rheumatol (2019) 71(9):1460–1471. doi: 10.1002/art.40899
143. Barbhaiya M, Lu B, Sparks JA, Malspeis S, Chang SC, Karlson EW, et al. Influence of Alcohol Consumption on the Risk of Systemic Lupus Erythematosus Among Women in the Nurses’ Health Study Cohorts. Arthritis Care Res (2017) 69(3):384–392. doi: 10.1002/acr.22945
144. Ljung L, Rantapää-Dahlqvist S. Abdominal Obesity, Gender and the Risk of Rheumatoid Arthritis - a Nested Case-Control Study. Arthritis Res Ther (2016) 18(1):277. doi: 10.1186/s13075-016-1171-2
145. Crowson CS, Matteson EL, Davis JM, Gabriel SE. Contribution of Obesity to the Rise in Incidence of Rheumatoid Arthritis. Arthritis Care Res (2013) 65(1):71–7. doi: 10.1002/acr.21660
146. De Hair MJH, Landewé RBM, Van De Sande MGH, Van Schaardenburg D, Van Baarsen LGM, Gerlag DM, et al. Smoking and Overweight Determine the Likelihood of Developing Rheumatoid Arthritis. Ann Rheum Dis (2013) 72(10):1654–8. doi: 10.1136/annrheumdis-2012-202254
147. Lu B, Hiraki LT, Sparks JA, Malspeis S, Chen CY, Awosogba JA, et al. Being Overweight or Obese and Risk of Developing Rheumatoid Arthritis Among Women: A Prospective Cohort Study. Ann Rheum Dis (2014) 73(11):1914–22. doi: 10.1136/annrheumdis-2014-205459
148. Marchand NE, Sparks JA, Tedeschi SK, Malspeis S, Costenbader KH, Karlson EW, et al. Abdominal Obesity in Comparison With General Obesity and Risk of Developing Rheumatoid Arthritis in Women. J Rheumatol (2021) 48(2):165–173. doi: 10.3899/jrheum.200056
149. Lamb MM, Miller M, Seifert JA, Frederiksen B, Kroehl M, Rewers M, et al. The Effect of Childhood Cow’s Milk Intake and HLA-DR Genotype on Risk of Islet Autoimmunity and Type 1 Diabetes: The Diabetes Autoimmunity Study in the Young. Pediatr Diabetes (2015) 16(1):31–8. doi: 10.1007/s00125-015-3657-x
150. O’Donovan A, Cohen BE, Seal KH, Bertenthal D, Margaretten M, Nishimi K, et al. Elevated Risk for Autoimmune Disorders in Iraq and Afghanistan Veterans With Posttraumatic Stress Disorder. Biol Psychiatry (2015) 77(4):365–74. doi: 10.1016/j.biopsych.2014.06.015
151. Lee YC, Agnew-Blais J, Malspeis S, Keyes K, Costenbader K, Kubzansky LD, et al. Post-Traumatic Stress Disorder and Risk for Incident Rheumatoid Arthritis. Arthritis Care Res (2016) 68(3):292–8. doi: 10.1002/acr.22683
152. Makinen M, Loyttyniemi E, Koskinen M, Vaha-Makila M, Siljander H, Nurmio M, et al. Serum 25-Hydroxyvitamin D Concentrations at Birth in Children Screened for HLA-DQB1 Conferred Risk for Type 1 Diabetes. J Clin Endocrinol Metab (2019) 104(6):2277–2285. doi: 10.1210/jc.2018-02094
153. Hyppönen E, Läärä E, Reunanen A, Järvelin MR, Virtanen SM. Intake of Vitamin D and Risk of Type 1 Diabetes: A Birth-Cohort Study. Lancet (2001) 358(9292):1500–3. doi: 10.1016/S0140-6736(01)06580-1
154. Harley JB, James JA. Epstein-Barr Virus Infection may be an Environmental Risk Factor for Systemic Lupus Erythematosus in Children and Teenagers [3]. Arthritis Rheumatism (1999) 42(8):1782–3. doi: 10.1002/1529-0131(199908)42:8<1782::AID-ANR36>3.0.CO;2-X
155. McClain MT, Poole BD, Bruner BF, Kaufman KM, Harley JB, James JA. An Altered Immune Response to Epstein-Barr Nuclear Antigen 1 in Pediatric Systemic Lupus Erythematosus. Arthritis Rheum (2006) 54(1):360–8. doi: 10.1002/art.21682
156. Levin LI, Munger KL, O’Reilly EJ, Falk KI, Ascherio A. Primary Infection With the Epstein-Barr Virus and Risk of Multiple Sclerosis. Ann Neurol (2010) 67(6):824–30. doi: 10.1002/ana.21978
157. Lönnrot M, Lynch KF, Elding Larsson H, Lernmark Å, Rewers MJ, Törn C, et al. Respiratory Infections are Temporally Associated With Initiation of Type 1 Diabetes Autoimmunity: The TEDDY Study. Diabetologia (2017) 60(10):1931–1940. doi: 10.1007/s00125-017-4365-5
158. Nielen MMJ, Van Schaardenburg D, Reesink HW, Van De Stadt RJ, van der Horst-Bruinsma IE, De Koning MHMT, et al. Specific Autoantibodies Precede the Symptoms of Rheumatoid Arthritis: A Study of Serial Measurements in Blood Donors. Arthritis Rheum (2004) 50(2):380–6. doi: 10.1002/art.20018
159. Aringer M, Costenbader K, Daikh D, Brinks R, Mosca M, Ramsey-Goldman R, et al. European League Against Rheumatism/American College of Rheumatology Classification Criteria for Systemic Lupus Erythematosus. Arthritis Rheumatol (2019) 2019:1151–9. doi: 10.1002/art.40930
160. Mok CC, Ho LY, Leung HW, Wong LG. Performance of Anti-C1q, Antinucleosome, and anti-dsDNA Antibodies for Detecting Concurrent Disease Activity of Systemic Lupus Erythematosus. Transl Res (2010) 156(6):320–5. doi: 10.1016/j.trsl.2010.07.009
161. Lhotta K, Klotz W, Lhotta K. Sensitivity and Specificity of Autoantibody Tests in the Differential Diagnosis of Lupus Nephritis. Lupus (2009) 18(14):1276–80. doi: 10.1177/0961203309345753
162. Breij ECW, Brink BP, Veerhuis R, Van Den Berg C, Vloet R, Yan R, et al. Homogeneity of Active Demyelinating Lesions in Established Multiple Sclerosis. Ann Neurol (2008) 63(1):16–25. doi: 10.1002/ana.21311
163. Comi G, Bar-Or A, Lassmann H, Uccelli A, Hartung HP, Montalban X, et al. Role of B Cells in Multiple Sclerosis and Related Disorders. Ann Neurol (2021) 89(1):13–23. doi: 10.1002/ana.25927
164. Endesfelder D, Hagen M, Winkler C, Haupt F, Zillmer S, Knopff A, et al. A Novel Approach for the Analysis of Longitudinal Profiles Reveals Delayed Progression to Type 1 Diabetes in a Subgroup of Multiple-Islet-Autoantibody-Positive Children. Diabetologia (2016) 59(10):2172–80. doi: 10.1007/s00125-016-4050-0
165. Parikka V, Näntö-Salonen K, Saarinen M, Simell T, Ilonen J, Hyöty H, et al. Early Seroconversion and Rapidly Increasing Autoantibody Concentrations Predict Prepubertal Manifestation of Type 1 Diabetes in Children at Genetic Risk. Diabetologia (2012) 55(7):1926–36. doi: 10.1007/s00125-012-2523-3
166. Kimpimäki T, Kupila A, Hämäläinen A-M, Kukko M, Kulmala P, Savola K, et al. The First Signs of $β$-Cell Autoimmunity Appear in Infancy in Genetically Susceptible Children From the General Population: The Finnish Type 1 Diabetes Prediction and Prevention Study. J Clin Endocrinol Metab (2001) 86(10):4782–8. doi: 10.1210/jcem.86.10.7907
167. Hunt L, Hensor EM, Nam J, Burska AN, Parmar R, Emery P, et al. T Cell Subsets: An Immunological Biomarker to Predict Progression to Clinical Arthritis in ACPA-Positive Individuals. Ann Rheum Dis (2015) 75(10):1884–9. doi: 10.1136/annrheumdis-2015-207991
168. Kaul A, Gordon C, Crow MK, Touma Z, Urowitz MB, Van Vollenhoven R, et al. Systemic Lupus Erythematosus. Nat Rev Dis Prim (2016) 2:16039. doi: 10.1038/nrdp.2016.39
169. Sambucci M, Gargano F, De Rosa V, De Bardi M, Picozza M, Placido R, et al. FoxP3 Isoforms and PD-1 Expression by T Regulatory Cells in Multiple Sclerosis. Sci Rep (2018) 8(1):3674. doi: 10.1038/s41598-018-21861-5
170. Dominguez-Villar M, Baecher-Allan CM, Hafler DA. Identification of T Helper Type 1-"Like, Foxp3 + Regulatory T Cells in Human Autoimmune Disease. Nat Med (2011) 17(6):673–5. doi: 10.1038/nm.2389
171. Marrosu MG, Cocco E, Lai M, Spinicci G, Pischedda MP, Contu P. Patients With Multiple Sclerosis and Risk of Type 1 Diabetes Mellitus in Sardinia, Italy: A Cohort Study. Lancet (2002) 359(9316):1461–5. doi: 10.1016/S0140-6736(02)08431-3
172. Kinslow JD, Blum LK, Deane KD, Demoruelle MK, Okamoto Y, Parish MC, et al. Elevated IgA Plasmablast Levels in Subjects at Risk of Developing Rheumatoid Arthritis. Arthritis Rheumatol (2016) 68(10):2372–83. doi: 10.1002/art.39771
173. Gerlag DM, Safy M, Maijer KI, Tang MW, Tas SW, Starmans-Kool MJF, et al. Effects of B-Cell Directed Therapy on the Preclinical Stage of Rheumatoid Arthritis: The PRAIRI Study. Ann Rheum Dis (2019) 78(2):179–85. doi: 10.1136/annrheumdis-2017-212763
174. Rodríguez-Bayona B, Ramos-Amaya A, Pérez-Venegas JJ, Rodríguez C, Brieva JA. Decreased Frequency and Activated Phenotype of Blood CD27 IgD IgM B Lymphocytes is a Permanent Abnormality in Systemic Lupus Erythematosus Patients. Arthritis Res Ther (2010) 12(3):R108. doi: 10.1186/ar3042
175. Pescovitz MD, Greenbaum CJ, Krause-Steinrauf H, Becker DJ, Gitelman SE, Goland R, et al. Rituximab, B-Lymphocyte Depletion, and Preservation of Beta-Cell Function. N Engl J Med (2009) 361(22):2143–52. doi: 10.1056/NEJMoa0904452
176. Deane KD, O’Donnell CI, Hueber W, Majka DS, Lazar AA, Derber LA, et al. The Number of Elevated Cytokines and Chemokines in Preclinical Seropositive Rheumatoid Arthritis Predicts Time to Diagnosis in an Age-Dependent Manner. Arthritis Rheumatol (2010) 62(11):3161–72. doi: 10.1002/art.27638
177. Yusof MYM, Psarras A, El-Sherbiny YM, Hensor EMA, Dutton K, Ul-Hassan S, et al. Prediction of Autoimmune Connective Tissue Disease in an at-Risk Cohort: Prognostic Value of a Novel Two-Score System for Interferon Status. Ann Rheum Dis (2018) 77(10):1432–1439. doi: 10.1136/annrheumdis-2018-213386
178. De Jong BA, Schrijver HM, Huizinga TWJ, Bollen ELEM, Polman CH, Uitdehaag BMJ, et al. Innate Production of Interleukin-10 and Tumor Necrosis Factor Affects the Risk of Multiple Sclerosis. Ann Neurol (2000) 48(4):641–6. doi: 10.1002/1531-8249(200010)48:4<641::AID-ANA11>3.0.CO;2-Z
179. Bhattacharya J, Pappas K, Toz B, Aranow C, Mackay M, Gregersen PK, et al. Serologic Features of Cohorts With Variable Genetic Risk for Systemic Lupus Erythematosus. Mol Med (2018) 24(1):24. doi: 10.1186/s10020-018-0019-4
180. Rowe P, Wasserfall C, Croker B, Campbell-Thompson M, Pugliese A, Atkinson M, et al. Increased Complement Activation in Human Type 1 Diabetes Pancreata. Diabetes Care (2013) 36(11):3815–7; . doi: 10.2337/dc13-0203
181. Stack JRJ, van Tuyl LHD, Sloots M, Van De Stadt LA, Hoogland W, Maat B, et al. Symptom Complexes in Patients With Seropositive Arthralgia and in Patients Newly Diagnosed With Rheumatoid Arthritis: A Qualitative Exploration of Symptom Development. Rheumatol (United Kingdom) (2014) 53(9):1646–53. doi: 10.1093/rheumatology/keu159
182. Marrie RA, Walld R, Bolton JM, Sareen J, Walker JR, Patten SB, et al. Rising Incidence of Psychiatric Disorders Before Diagnosis of Immune-Mediated Inflammatory Disease. Epidemiol Psychiatr Sci (2019) 28(3):333–342. doi: 10.1017/S2045796017000579
183. Van Beers-Tas M, Nielen MMJ, Twisk JWR, Korevaar J, Van Schaardenburg D. Increased Primary Care Use for Musculoskeletal Symptoms, Infections and Comorbidities in the Years Before the Diagnosis of Inflammatory Arthritis. RMD Open (2020) 6(2):e001163. doi: 10.1136/rmdopen-2019-001163
184. Makhani N, Tremlett H. The Multiple Sclerosis Prodrome. Nat Rev Neurol (2021) 17(8):515–521. doi: 10.3389/fneur.2021.761408
185. Okuda DT, Mowry EM, Cree BAC, Crabtree EC, Goodin DS, Waubant E, et al. Asymptomatic Spinal Cord Lesions Predict Disease Progression in Radiologically Isolated Syndrome. Neurology (2011) 76(8):686–92. doi: 10.1212/WNL.0b013e31820d8b1d
186. Sosenko JM, Palmer JP, Rafkin-Mervis L, Krischer JP, Cuthbertson D, Mahon J, et al. Incident Dysglycemia and Progression to Type 1 Diabetes Among Participants in the Diabetes Prevention Trial-Type 1. Diabetes Care (2009) 32(9):1603–7. doi: 10.2337/dc08-2140
Keywords: rheumatoid arthritis (RA), systemic lupus erythematosus (SLE), multiple sclerosis (MS), type 1 diabetes (T1D), prodromal phase, genetic risk factors, environmental risk factors, pathophysiological process
Citation: Frazzei G, van Vollenhoven RF, de Jong BA, Siegelaar SE and van Schaardenburg D (2022) Preclinical Autoimmune Disease: a Comparison of Rheumatoid Arthritis, Systemic Lupus Erythematosus, Multiple Sclerosis and Type 1 Diabetes. Front. Immunol. 13:899372. doi: 10.3389/fimmu.2022.899372
Received: 18 March 2022; Accepted: 30 May 2022;
Published: 30 June 2022.
Edited by:
Darin T. Okuda, University of Texas Southwestern Medical Center, United StatesReviewed by:
Karen Cerosaletti, Benaroya Research Institute, United StatesAaron Michels, University of Colorado, United States
Copyright © 2022 Frazzei, van Vollenhoven, de Jong, Siegelaar and van Schaardenburg. This is an open-access article distributed under the terms of the Creative Commons Attribution License (CC BY). The use, distribution or reproduction in other forums is permitted, provided the original author(s) and the copyright owner(s) are credited and that the original publication in this journal is cited, in accordance with accepted academic practice. No use, distribution or reproduction is permitted which does not comply with these terms.
*Correspondence: Giulia Frazzei, Zy5mcmF6emVpQGFtc3RlcmRhbXVtYy5ubA==