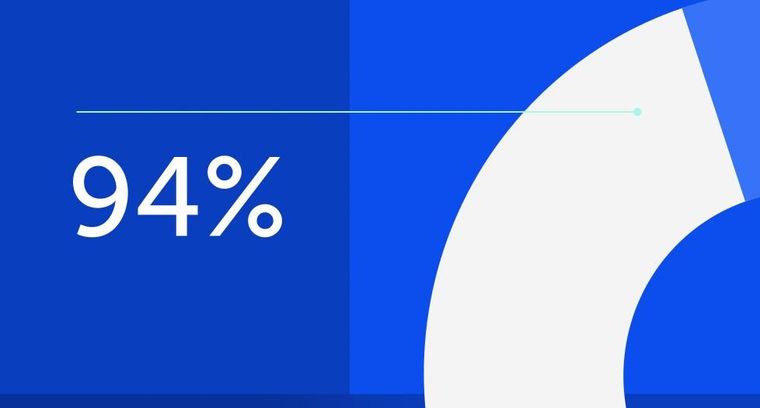
94% of researchers rate our articles as excellent or good
Learn more about the work of our research integrity team to safeguard the quality of each article we publish.
Find out more
ORIGINAL RESEARCH article
Front. Immunol., 19 July 2022
Sec. Viral Immunology
Volume 13 - 2022 | https://doi.org/10.3389/fimmu.2022.888661
This article is part of the Research TopicDefinition of the Immune Parameters Related to COVID-19 SeverityView all 45 articles
The global coronavirus disease 2019 (COVID-19) pandemic has lasted for over 2 years now and has already caused millions of deaths. In COVID-19, leukocyte pyroptosis has been previously associated with both beneficial and detrimental effects, so its role in the development of this disease remains controversial. Using transcriptomic data (GSE157103) of blood leukocytes from 126 acute respiratory distress syndrome patients (ARDS) with or without COVID-19, we found that COVID-19 patients present with enhanced leukocyte pyroptosis. Based on unsupervised clustering, we divided 100 COVID-19 patients into two clusters (PYRcluster1 and PYRcluster2) according to the expression of 35 pyroptosis-related genes. The results revealed distinct pyroptotic patterns associated with different leukocytes in these PYRclusters. PYRcluster1 patients were in a hyperinflammatory state and had a worse prognosis than PYRcluster2 patients. The hyperinflammation of PYRcluster1 was validated by the results of gene set enrichment analysis (GSEA) of proteomic data (MSV000085703). These differences in pyroptosis between the two PYRclusters were confirmed by the PYRscore. To improve the clinical treatment of COVID-19 patients, we used least absolute shrinkage and selection operator (LASSO) regression to construct a prognostic model based on differentially expressed genes between PYRclusters (PYRsafescore), which can be applied as an effective prognosis tool. Lastly, we explored the upstream transcription factors of different pyroptotic patterns, thereby identifying 112 compounds with potential therapeutic value in public databases.
The coronavirus disease 2019 (COVID-19) pandemic caused by the severe acute respiratory syndrome coronavirus 2 (SARS-CoV-2) has killed 6 million people until May 2022 (1). COVID-19 patients present with different clinical symptoms ranging from mild cold-like symptoms to a high fever, pneumonia, and possibly acute respiratory distress syndrome (ARDS). In the development of severe COVID-19 disease, uncontrolled systemic hyperinflammation caused by a dysregulated immune response leads to the release of pro-inflammatory cytokines and chemokines, a condition that is known as cytokine storm (2). COVID-19 patients show elevated blood levels of many cytokines, including IL-1β, IL-2, IL-6, IL-7, IL-8, IL-10, IL-18, G-CSF, IP-10, MCP-1, MIP-1A, and TNF (3–6). This cytokine storm is closely related to lung damage, multiple organ failure, and a poor prognosis, according to recent research (4, 7–11). Concurrently, several studies have also shown that cytokine blockade can improve the survival rate of patients with COVID-19 (12–15).
A possible mechanism linking cytokine storm to organ damage is inflammatory cell death, namely pyroptosis and necroptosis. Pyroptosis has been intensely studied recently. Some patients with severe COVID-19 may develop a systemic cytokine storm because SARS-CoV-2 promotes cytokine storms by inducing pyroptosis in pro-inflammatory blood-born immune cells (16–19). However, only a few studies about necroptosis and cytokine storm in COVID-19 have been published thus far.
Pyroptosis is a mechanism of programmed cell death characterized by the inflow of sodium ions and water mediated by gasdermin proteins, resulting in cell membrane rupture, excessive cell swelling, and spontaneous release of cytosolic contents into the extracellular space (20). Gasdermin proteins, which consist of an N-terminus with membrane pore-forming activity and an inhibitory C-terminus, are the key regulators of pyroptosis. Upon inflammasome activation, caspase proteins, including caspase-1 and other non-canonical inflammasome caspases (e.g., caspase-4, caspase-5, and caspase-11), cleave gasdermin into two parts (21), thereby unleashing the pore-forming activity of the N-terminus. This N-terminus fragment of gasdermin binds to the cell membrane, forming pores and leading to pyroptosis (22). Pyroptosis triggers the rapid release of a slew of alarmins including, cytokines (IL-1β, IL-18), chemokines, and damage-associated molecular patterns (DAMPs), prompting an immediate response from surrounding immune cells and triggering a pyroptotic chain reaction (23). Thus, pyroptosis plays a key role in the emergence of a cytokine storm, according to recent research (17, 19, 24).
Although pyroptosis is crucial for innate immunity (25, 26), extensive pyroptosis can cause tissue inflammation, organ failure and death, as found in various diseases (27). For example, in atherosclerosis, cholesterol crystals and oxidized low-density lipoprotein cause macrophage pyroptosis, which leads to a massive release of cytokines, promoting inflammation and disease progression (28). In line with these findings, NLRP3 inflammasome or ASC inhibition, which prevent macrophage pyroptosis, can lower infarct size and improve heart function in an animal model of myocardial infarction (29, 30). Similar results have also been observed in alcoholic hepatitis (31), lupus erythematosus (32, 33), and even in the central nervous system (34).
In COVID-19 patients, various cells undergo pyroptosis, including leukocytes (monocytes, macrophages, mucosal-associated invariant T cells) and other type of cells (adipocytes, lung epithelial cells and endothelial cells) (16, 35–39). On the one hand, SARS-CoV-2 nucleocapsid can prevent Gasdermin D cleavage, thus reducing host pyroptosis and suppressing the immune response (40), in addition to inhibiting coronavirus infection by promoting non-classical secretion of β-interferon (41). On the other hand, SARS-COV-2 can stimulate macrophage GSDMD-mediated pyroptosis, which leads to the rapid release of pro-inflammatory cytokines and to a cytokine storm (17). In addition, the synergistic effect of TNF-α and IFN-γ can trigger GSDMD-mediated pyroptosis and promote a cytokine storm, thereby increasing mortality among COVID19 patients (42). However, in cats and dogs, deficiencies of the inflammasome and pyroptosis pathways (cats and tigers do not express AIM2 and NLRP1, and dogs do not express AIM2 and have a shorter form of NLRC4 than humans) may provide an evolutionary advantage against SARS-CoV-2 by reducing cytokine storm-induced host damage (43). Therefore, the role of pyroptosis in COVID-19 remains complex, requiring more comprehensive studies.
Based on transcriptome data of patients with or without COVID-19 available in public databases (GSE157103), we found that the leukocytes of ARDS patients with COVID-19 have considerably higher pyroptotic markers than patients without COVID-19. Moreover, at least two different patterns of pyroptosis occur in patients with COVID-19, one correlated with a poor prognosis and the other with a benign prognosis. These two pyroptosis patterns may be regulated by different upstream transcription factor networks, which could prove therapeutically valuable for drug development.
From the GEO dataset, we retrieved RNA-seq data (GSE157103) of 126 ARDS patients, namely 100 COVID-19 patients and 26 non-COVID-19 patients, in addition to their clinical data, including gender, age, underlying disease status (diabetes), coagulation (D-dimer, ferritin, CRP, procalcitonin, fibrinogen), and hospital-free days post 45-day follow-up (HFD45), among other parameters. More specifically, HFD45 is defined as the number of days patients lived outside of a hospital from enrollment through death or the end of follow-up (44). The higher HDF45 is, the milder the disease and the better the prognosis will be.
The label-free quantification (LFQ) intensities of 736 proteins of 126 ARDS patients were collected from Mass Spectrometry Interactive Virtual Environment (MassIVE) (MSV000085703). After calculating the logarithm of the FQL intensities, we used the R package “limma” to calculate the log2(Log2Fold of change) (log2FC) of 736 proteins between two PYRclusters. The 736 proteins were sorted from large to small by log2FC (not absolute value). Then, using the “org.Hs.eg.db” and “clusterProfiler” packages, gene set enrichment analysis (GSEA) was performed based on MSigDB gene sets C2, C5 and C7. Significant gene sets were identified when |Normalized Enrichment Score (NES)|>1 and False Discovery Rate (FDR) < 0.25.
Gene ontology (GO) and Kyoto Encyclopedia of Genes and Genomes (KEGG) analyses were performed using the “org.Hs.eg.db” and “clusterProfiler” packages. GO terms and KEGG terms were identified as significantly enriched when p.adjust < 0.05.
Single-sample gene set enrichment analysis (ssGSEA) and “Cibersort” were used to analyze the immune cell infiltration fractions. The former was based on the list of Pan-cancer Immune Metagenes (45, 46).
Based on RNA-seq data of 35 pyroptosis-related genes including AIM2, CASP1, CASP3, CASP4, CASP5, CASP6, CASP8, CASP9, ELANE, GPX4, GSDMA, GSDMB, GSDMC, GSDMD, GSDME, GZMA, GZMB, IL18, IL1B, IL6, NLRC4, NLRP1, NLRP2, NLRP3, NLRP6, NLRP7, NOD1, NOD2, PJVK, PLCG1, PRKACA, PYCARD, SCAF11, TIRAP, and TNF, we divided 100 COVID-19 patients into two clusters (PYRcluster) using the “nmf” package. We determine the k value based on the consensusMap function.
The differentially expressed genes (DEGs) of two PYRclusters were identified using the “limma” package. Using the median of HFD45 as the cutoff value, COVID-19 patients were divided into two groups. The DEGs in these two groups were calculated using the limma package and subsequently applied for PCA analysis. PC1 and PC2 were used to construct the PYRscore (47).
Based on the log2(TPM) of the 570 DEGs between PYRclusters and the HDF45 of each patient, we used the “glmnet” package to build a PYRsafescore model by LASSO regression. We determine the signatures of the model by selecting the lambda value with the smallest mean-squared error by 20-fold-cross-validation. The coefficients of the final signatures were used to calculate the PYRsafescore as follows: protective score = ∑ Coefficienti × Expression level of signaturei. Using the “caret” package, 100 patients were randomly divided into a training group and a test group with a ratio of 2:1. The model built with the training group data was validated in the test group. We used “ROCR” packages to plot receiver operating characteristic (ROC) curves and to calculate area under the curve (AUC) scores to evaluate model performance.
First, based on the transcription factor targets (TFT) and their gene sets in MSigDB (48), we used the “clusterProfiler” package to enrich the transcription factors from DEGs between PYRclusters.
We then used the “CoRegNet” package to enrich the transcription factor co-regulatory network in COVID-19 patients from PYRcluster1 and PYRcluster2 based on the dataset of transcription factor targets (CHEA, ENCODE, and JASPAR Predicted Transcription Factor Targets, MotifMap and TRANSFAC Predicted Transcription Factor Targets, and TRANSFAC Curated Transcription Factor Targets) from Harmonizome (49, 50). Lastly, the network graph was plotted using the “ggraph” package.
We used the transcription factors that we screened as keywords to search for the corresponding compounds on ChEMBL (51), thus screening active or repressed compounds based on their role in pyroptosis.
The Wilcoxon sum-rank test and the t-test were used to compare different groups, and the Pearson’s product-moment correlation test was used for correlation analysis. All statistical tests were two-sided, and a significant difference was defined as a p-value of 0.05. Power calculations were performed using the following R packages: “pwr” and “rstatix” at sig.level=0.05.
A GEO dataset provided RNA-seq data and clinical data from 126 samples of 100 patients with COVID-19 and 26 patients without COVID-19 (GSE157103) (44). Initially, we assessed the expression levels of pyroptosis-related gene sets of all patients based on prior studies (52).
The expression of the pyroptosis-related genes AIM2, CASP1, CASP3, CASP6, CASP8, CASP9, GSDMA, GSDMC, GZMB, IL6, NLRP3, NLRP7, NOD1, NOD2, SCAF11, and TIRAP was significantly higher in blood leukocytes of COVID-19 patients, indicating that the level of pyroptosis was significantly increased (Figure 1A). NLRP3, NLRP7, NOD1, NOD2 are closely related to caspase activation (53, 54).
Figure 1 (A) Boxplot of 35 pyroptosis-related genes’ relative expression between different types of patients. C: COVID-19 patients; NC: none-COVID-19 patients. (B) The Pearson’s correlation between 35 pyroptosis-related genes in COVID -19 patients, R value represents the Pearson’s correlation coefficient. *p < 0.05, **p < 0.01, ***p < 0.001, ****p < 0.0001.
Subsequently, we outlined the correlation patterns of 35 pyroptosis-related genes in COVID-19 patients to investigate relationships between different pyroptosis-related genes. Although most of the 35 genes have a substantial positive association with other genes, several genes are nevertheless negatively correlated with other pyroptosis-related genes. ELANE, for example, is negatively correlated with AIM2, CASP1, CASP3, CASP4, CASP5, CASP6, CASP8, CASP9, GSDMB, GSDMC, GZMA, IL1B, IL6, NLRP1, NLRP2, NLRP3, NOD1, NOD2, PJVK, PLCG1, PRKACA, SCAF11, TIRAP, and TNF. Therefore, the expression of these pyroptosis-related genes in COVID-19 patients presents complex patterns (Figure 1B). Furthermore, each caspase is strongly and positively correlated with the others. For example, the linkage between GSDMA and caspases suggests that its cleavage is related to caspase-3/-6/-8/-9. CASP3 expression is highly linked to GSDME, in line with previous reports on caspase-3 cleavage of GSDME, releasing its activity (22).
By gene set variation analysis (GSVA), we studied changes in the biological function of leukocytes between the two types of patients. Mismatch repair, homologous recombination, replication, cell cycle, and p53 signaling are more enriched in leukocytes of COVID-19 patients, indicating severe cell damage during viral infection and ongoing damage repair (Figure 2A and Supplementary Table 1).
Figure 2 (A) Gene set variation analysis (GVSA) analysis shows COVID-19 patients’ leukocytes may have been significantly damaged during viral infection and are undergoing damage repair. C: COVID-19 patients; NC: none-COVID-19 patients. (B) The abundance of leukocytes between the different types of patients. *p < 0.05, **p < 0.01, ***p < 0.001, ****p < 0.0001, ns, no significance.
By single-sample gene set enrichment analysis (ssGSEA), we also compared the proportions of 28 immune cell types between the two groups and found that the numbers of various immune cells are much higher in COVID-19 patients than in non-COVID-19 patients, indicating a highly active immune response in COVID-19 patients (46) (Figure 2B and Supplementary Table 2). Surprisingly, the numbers of some immune cells, such as macrophage and cd56dim natural killer cells, were lower in COVID-19 patients than in the control group. This difference could be due to cell death caused by massive viral infection and increased pyroptosis (17, 55). Human dendritic cells and T lymphocytes (including CD4+ and CD8+) undergo pyroptosis via the AIM2-Caspase1-gasdermin D and the CARD8-Caspase1-gasdermin D axes (56, 57), respectively. Pyroptosis has also been identified in macrophages and neutrophils (58, 59). Although NK cells have not been documented to undergo pyroptosis on their own, they can participate in this process, playing a key role (60). These results suggests that blood leukocytes either directly undergo pyroptosis or have a synergistic role with pyroptosis in COVID-19 patients.
In summary, blood leukocytes exhibit substantial pyroptotic characteristics in COVID-19 patients.
By non-negative matrix factorization based on 35 pyroptosis-related genes, we clustered the 100 COVID-19 patients into two clusters, PYRcluster1 and PYRcluster2 (Figure 3A and Supplementary Figure 1A). The best clustering result was found when k = 2, with no differences in age, gender, days admitted before enrollment, replacement therapy (pre-enrollment) or underlying disorders between the two clusters, which we called PYRclusters (Supplementary Figures 1B–E, 3A, B).
Figure 3 (A) Consensus clustering matrix for k = 2. (B) The heatmap of 35 pyroptosis-related genes between the two PYRclusters. Red represents high expression; blue represents low expression. (C) Boxplot of significant pyroptosis-related genes’ relative expression between two PYRclusters. (D–F) The HFD45, ventilator-free days, D-dimer levels between the two PYRclusters. *p < 0.05, **p < 0.01, ***p < 0.001, ****p < 0.0001, ns, no significance.
We first explored differences in the expression of pyroptosis-related genes between the two PYRclusters (Figures 3B,C and Supplementary Table 3) and found that PYRcluster1 has a higher expression of NLRC4, NLRP6, CASP5, CASP8, CASP9, GSDMC, and AIM2, whereas PYRcluster2 has a higher expression of GPX4, GSDMD, PYCARD, TNF, IL6, NLRP2, NLRP7, GSDMA, CASP6, GSDMB, NOD1, GZMA, and GZMB.
Subsequently, we found that PYRcluster2 has a higher hospital-free days post 45-day follow-up (HFD45), ventilator-free days and a lower proportion of mechanical ventilation than PYRcluster1 (Figures 3D, E and Supplementary Figure 3C), which suggests a better prognosis. The blood D-dimer level of PYRcluster1 is significantly higher than that of PYRcluster2 (Figure 3F), indicating hypercoagulation. Although albumin and hemoglobin of the two PYRclusters are mostly below the normal range (green dashed line), PYRcluster1 deviates further from the normal range than PYRcluster2. Other clinical features do not differ significantly between the two clusters (Supplementary Figures 2A–C). Based on these results, the highly expressed pyroptosis-related genes of PYRcluster1 may be associated with a poor prognosis. In line with our results, AIM2 and NLRC4 deficiency in dogs and cats provide a protective effect against SARS-CoV-2 by reducing cytokine storm-induced host damage (43).
We further determined differentially expressed genes (DEGs) using the “limma” package, with cutoff criteria of |logFC| >1 and p=0.05, totaling 570 DEGs, and employed Gene ontology (GO), Kyoto Encyclopedia of Genes and Genomes (KEGG) pathway enrichments (Figure 4A and Supplementary Figure 2E). In addition, we determined the logFC of 736 proteins between two PYRclusters (FC=PYRcluster2/PYRcluster1) using “limma” from the proteomic data from the same study as the transcriptome data. Subsequently, we employed gene set enrichment analysis (GSEA) based on the log2FC of these proteins (Supplementary Figure 2D, Supplementary Table 4).
Figure 4 (A) Kyoto Encyclopedia of Genes and Genomes (KEGG) enrichment of 570 DEGs Between two PYRclusters, “up” means these pathways of PYRcluster2 were upregulated when compared to PYRcluster1; “down” means these pathways were downregulated. (B) Leukocytes with significantly different expression levels among PYRclusters. (C) ImmuneScore calculated by “estimate” package between two PYRclusters. (D) Pearson’s correlation between expressions of 35 pyroptosis-related genes and abundance of leukocytes, R value represents the Pearson’s correlation coefficient. Annotated bars above and to the left indicate in which PYRcluster each pyroptosis-related gene or leukocyte is highly expressed.
Immune responses such as antigen recognition/presentation, immune cell activation, migration, and replication are relatively enhanced in PYRcluster2 (Figure 4A and Supplementary Figure 2E). Both transcriptome and proteomic results revealed hyperinflammation in PYRcluster1 (Supplementary Figure 2D, Supplementary Table 6). KEGG pathway enrichment data showed that “Coronavirus disease-COVID-19” pathways are markedly upregulated in PYRcluster2, underscoring a highly activated immune process unique to PYRcluster2. PYRcluster1 has a higher NO synthesis level than PYRcluster2, which may be related to the antiviral capacity of its patients (61–63). The highly activated stress response pathway may associated with severe damage caused by the virus and lead to higher levels of immune cell apoptosis in PYRcluster1 (Supplementary Figure 2D, Supplementary Table 6). Furthermore, PYRcluster1 has a markedly increased expression of cytokines, including IL-1, IL-6, IL-8, IL-10, and TNF (Supplementary Table 6), which are typical components of a cytokine storm and result in a poor prognosis (64, 65). Consistent with an increased D-dimer (Figure 3F), the neutrophil extracellular traps (NETs) that promote blood coagulation are highly expressed in PYRcluster1, promoting venous thrombosis and leading to poor prognosis (66, 67). In addition, AIM2, CXCR2 and UBE2W, which were significantly highly expressed in PYRcluster1, were included in the 20 genes associated with distinctly methylated CpG sites between mild and severe COVID-19 patients (68). Their odds ratios were all greater than 1, indicating that their downregulation is beneficial to COVID-19 patients. In conclusion, the two PYRclusters of COVID-19 patients exhibited distinct pyroptotic patterns and clinical features.
SARS-CoV-2 viruses infect leukocytes and lead to immunodeficiency (69–71). We speculate that leukocyte pyroptosis helps to destroy the virus protective niche and release viruses from cells, thereby enhancing viral clearance and immune recovery. As a result, viruses released by pyroptosis may be further removed by phagocytic cells via phagocytosis.
We first assessed the proportion of different immune cells in the two PYRclusters by ssGSEA. Immune cells highly associated with antivirals, such as activated B, CD4/8+ T, Treg, and NK cells are highly expressed in PYRcluster2 (Figure 4B and Supplementary Figure 4A, Supplementary Table 7). PYRcluster1 had a higher proportion of pro-inflammatory neutrophils. Using “Cibersort”, we discovered PYRcluster2 had a higher proportion of anti-inflammatory M2 macrophages (Supplementary Figure 4A). The hemogram percentages of several cells, such as neutrophils, lymphocytes and monocytes, were consistent with the results of ssGSEA (Supplementary Figure 3F). We then used the “estimate” package to score the two clusters and found that PYRcluster2 shows a greater increase in immune cell infiltration than PYRcluster1 (Figure 4C). As shown in Figure 4D, the expression of characteristic pyroptosis-related genes of PYRcluster1 were significantly positively correlated with its expression of characteristic immune cells. For example, PYCARD, GPX4, GSDMD, GZMA, TNF, NOD1, IL6, NLRP7, CASP6, GSDME, PJVK, GSDMA, and NLPR2 are positively correlated with NK, NKT, regulatory T, γδ T, activated B, and Th17 cells, MDSCs, and monocytes (Figure 4D). Both these pyroptosis-related genes and immune cells are highly expressed in PYRcluster2 (Figures 3C, 4B). Furthermore, leukocytes have stronger phagocytic activity in GO enrichment results in PYRcluster2 than in PYRcluster1 (Supplementary Table 6). In contrast, pyroptosis in PYRcluster1 may produce several pathogen- and damage-related molecular patterns that increase cytokine storm, leading to multiple organ failure and poor prognosis.
We subsequently performed unsupervised clustering of all COVID-19 patients using 570 DEGs and all genes, respectively, yielding two more clusters: DEG and All-Gene clusters. The heatmap demonstrates that these additional clusters match a previous clustering based on the 35 pyroptosis-related genes (Figure 5A and Supplementary Figure 4B–D). These data suggest that distinct patterns of pyroptosis occur in COVID-19 patients, which can be represented by 35 pyroptosis-related genes.
Figure 5 (A) Heatmap of the DEGs between the gene clusters, different clinical data was shown in the annotation. (B) Pyrscore between two PYRclusters. (C, D) Pearson’s correlations between pyrscore and ventilator-free days(C), HFD45 (D), R value represents the Pearson’s correlation coefficient; grey area represents the 95% confidence interval for the linear fit. The maximum value of ventilator-free days is 28 since this 28-day time frame was initially chosen because most subjects with ARDS will have died or been extubated by Day 28. (E) Mean-squared error (MSE) of different numbers of variables revealed by the LASSO regression model. The red dots represent the MSE values; the grey lines represent the standard error (SE); the two vertical dotted lines on the left and right, respectively, represent optimal values by minimum criteria and 1-SE criteria. “Lambda” is the tuning parameter. (F) AUC of patients in the training group and test group. *p < 0.05, **p < 0.01, ***p < 0.001, ****p < 0.0001, ns, no significance.
To better elucidate differences in pyroptotic patterns between PYRcluster1 and PYRcluster2 and their correlation with prognosis, we created a pyroptosis score (pyrscore) (Supplementary Figure 4E). As shown in Figure 5B, PYRcluster1 has a higher score than PYRcluster2. HFD45 and ventilator-free days are negatively correlated with pyroptosis scores (Figures 5C, D), whereas sofa, APACHE-II, D-dimer, and CRP levels are positively correlated (Supplementary Figures 5A–D). In conclusion, PYRcluster1 and PYRcluster2 have different levels of immune response to SARS-COV-2 and pyroptotic patterns, resulting in distinct prognoses.
Given that different pyroptotic patterns may have a significant impact on the prognosis of COVID-19 patients, we created a PYRsafescore model based on the HFD45 of COVID-19 patients and DEGs across PYRclusters by least absolute shrinkage and selection operator (LASSO) regression analysis. In a ratio of 2:1, 100 COVID-19 patients were divided into a training group and a test group, and the model was obtained in the training group. “Lambda-min” was chosen as the best value in the cross-validation procedure (Figure 5E and Supplementary Figures 5E). Lastly, based on the log2 value of the expression level of 10 genes, we established the following scoring model:
The area under the ROC curve (AUC) values of the model in the training and test groups were 0.907 and 0.879, respectively, indicating that our predictive model performs well (Figure 5F). HFD45 and PYRsafescore are positively correlated (Figure 6A), which suggests that a higher PYRsafescore indicates a better prognosis. In addition to HDF45, the correlation of other clinical variables with PYRsafescore, including sofa, ventilator-free days, APACHE-II, and CRP levels, also demonstrates that our model works effectively (Figures 6B, C and Supplementary Figures 6A, B). Additionally, the expression of these 10 genes between the two PYRclusters also has significant differences (Figure 6D). KEGG analysis indicates that they were closely related to the patient’s immune response (Figure 6E).
Figure 6 (A, B, C) Pearson’s correlations between PYRsafescore and HFD45 (A), ventilator-free days (B), APACHE-II (C), R value represents the Pearson’s correlation coefficient; grey area represents the 95% confidence interval for the linear fit. (D) Heatmap of signature genes of PYRsafescore; expression of these genes was highly correlated with HFD45 and PYRsafescore. (E) Kyoto Encyclopedia of Genes and Genomes (KEGG) enrichment of signature genes of PYRsafescore. (F) Transcription factor enrichment of 570 DEGs between PYRclusters using “clusterProfiler” package based on MSigDB gene set: TFT (transcription factor targets) gene set.
In conclusion, our results proved that the newly created prognosis model has a considerable clinical predictive value.
Using the “clusterProfiler” package, we first enriched DEGs across PYRclusters for transcription factors based on MSigDB Collections: “regulatory target gene sets” (Figure 6F). We further enriched the transcription factor regulatory networks for all transcriptome data of the two PYRclusters using the “CoRegNet” package. PYRcluster1 has the regulatory network with MNDA, TSC22D3, HMGB2, FOS, EEF1A1, TRIM22, NFKBIA as transcription factors, and PYRcluster2 has the regulatory network with FOS, MNDA, EEF1A1, DAZAP2, DDIT3, HCLS1, NFKBIA, TSC22D3, PTMA, and TRIM22 (Figure 7A and Supplementary Figure 6C, Supplementary Table 8). Notably, both PYRcluster1 and PYRcluster2 are regulated by FOS, EEF1A1, MNDA, and TRIM22.
Figure 7 (A) Transcription factors regulatory network of PYRcluster1. “Degree” means the number of edges connected to the node. (B) Pearson’s correlation of differentially expressed pyroptosis-related genes and transcription factors in PYRclusters; R value represents the Pearson’s correlation coefficient. Annotation on the left represents in which PYRcluster each pyroptosis-related gene is significantly highly expressed. (C) Pearson’s correlation between different clinical data and transcription factors; R value represents the Pearson’s correlation coefficient.
Once SARS-COV-2 infects cells, EEF1A1 is critical for viral replication. Drug targeting EEF1A has robust antiviral effects in vitro (72). The nuclear factor-kappaB (NF-kappaB)/REL family of transcription factors are activated in response to DNA damage to regulate inflammation and apoptosis resistance (73, 74). Since NFKBIA is highly expressed in COVID-19 patients (75) and tripartite motif-containing (TRIM) 22 can activate NF-kappaB (76) to protect the host from viral infection (77), these two genes have a huge impact on immune disparities between the two PYRclusters. MNDA is a member of the family of hematopoietic interferon (IFN)-inducible nuclear proteins that promotes the degradation of the anti-apoptotic factor MCL-1 and apoptosis in myeloid cells (78, 79).
We then observed the correlation between these transcription factors and the expression of differentially expressed pyroptosis-related genes between PYRclusters and the clinical characteristics of all patients. The correlations between transcription factors and pyroptosis-related genes vary greatly, depending on the PYRclusters (Figure 7B). Pyroptosis-related genes highly expressed in PYRcluster1 are positively correlated with FOS, MAML1, DAZAP2, STAT6, STAT5B, ETS2, HCLS1, TSC22D3, HMGB2, MNDA, TRIM22, NFKBIA, and DDIT3, whereas genes highly expressed in PYRcluster2 are positively correlated with ZNF623, PSMB5, PTMA, EEF1A1, ETS1, CEBPA, and USF2. Moreover, some transcription factors positively correlated with pyroptosis-related genes in PYRcluster2 also have a positive effect on prognosis (Figure 7C).
Taken together, these findings show that distinct pyroptotic patterns may result from different upstream transcriptional regulation pathways. In light of this, we searched the ChEMBL database for compounds that promote or inhibit appropriate transcription factors based on diverse roles in prognosis (51). We screened a total of 112 compounds (Supplementary Table 9) and found that CHEMBL348436 (also known as Cirsimaritin) has the potential to regulate blood leukocyte pyroptosis in COVID-19 patients while simultaneously improving prognosis through FOS and NFKB1A inhibition. In fact, drugs targeting FOS have therapeutic effects in COVID-19 patients (80).
Pyroptosis, a mechanism of programed cell death which leads to cell swelling and lysis, plays a key role in innate immunity by disrupting the pathogen replication niche and killing intracellular bacteria through pore-induced intracellular traps (25, 26). However, excessive pyroptosis may trigger an overactive inflammatory response, resulting in a cytokine storm and severe organ damage through IL-6, TNF and NETs (81–83).
The occurrence of a cytokine storm is a major factor in the progression of moderate-to-severe COVID-19. In the therapy of COVID-19, multi-organ failure induced by cytokine storm has become a significant issue (84). Despite the relevance of pyroptosis in the treatment of severe COVID-19 patients, research on COVID-19 and pyroptosis is currently limited. Some studies suggest that the elevated pyroptosis is not conducive to the treatment of the disease but closely related to SARS-CoV-2 infection and cytokine storm (17, 35, 42, 43). By contrast, other studies show that pyroptosis can also be beneficial in fighting SARS-CoV-2 infection (40, 41). A dual role for NLRP3 was reported in a recent study according to which inflammasome-dependent pyroptosis contributes to the hyperinflammatory state of the lungs. However, pyroptosis can release infectious virus, preventing a productive viral cycle, which can help to eliminate viruses (85). In conclusion, the role of pyroptosis in COVID-19 remains unclear.
From the transcriptome data of COVID-19 patients, we found that the blood leukocytes of COVID-19 patients have typical characteristics of pyroptosis. Of the 35 pyroptosis-related genes retrieved from the database, 19 are significantly elevated in COVID-19 patients. Using an unsupervised clustering approach with non-negative matrix factorization, we classified the COVID-19 patients into two populations with distinct pyroptosis patterns. PYRcluster1 featured high AIM2, CASP1, CASP4, CASP5, CASP8, GSDMC, IL1B, NLRC4, NLRP3, NLRP6, and SCAF11 expression, whereas PYRcluster2 featured high CASP6, GPX4, GSDMA, GSDMB, GSDMD, GZMA, GZMB, IL6, NLRP2, NLRP7, NOD1, PJVK, PLCG1, PYCARD, and TNF expression.
Although the most well-known pyroptotic pathway in the present study contain NLRP3, CASP1, and GSDMD (21), pyroptosis can be induced by different inflammatory caspases and involves varied gasdermin proteins, such as NLRC4, caspase-3, caspase-8, caspase-11/4/5 and GSDMC (20, 86–89).
In combination with other clinical information, we found that PYRcluster2 patients had a better prognosis, including a longer HFD45 and a lower ICU hospitalization rate. PYRcluster2 patients also had more immune cells and a higher immune score. Furthermore, the expression of immune cells was highly correlated with the expression of pyroptosis-related genes. To better elucidate the pyroptotic patterns and prognosis, we calculated the “pyrscore” to characterize different pyroptotic patterns and the “PYRsafescore” to better predict prognosis and assist clinical treatment. Higher PYRsafescore scores mean a better prognosis.
Lastly, by transcription factor enrichment, we identified the upstream transcription factors that regulate different pyroptotic patterns and screened a series of compounds with therapeutic potential in public databases.
Currently, only a few drugs are available for controlling SARS-CoV-2 infection, including monoclonal antibodies that neutralize viral proteins (90–92), drugs that inhibit viral replication (93), and new oral drugs from Pfizer and Merck, namely PAXLOVID and Molnupiravir, which inhibit viral replication and viral proteases, respectively (94, 95). Methods for managing excessive inflammatory response, which is the leading cause of severe COVID-19, are very limited and less effective (96): For example, heparin is widely used to prevent blood clots, and some immunosuppressants such as dexamethasone, IL-6 monoclonal antibodies and JAK kinase family inhibitors are used to inhibit inflammation (12–15, 97–99). Our results suggest that pyroptosis plays a key role in the generation of a hyperinflammatory immune response. Therefore, therapeutic strategies targeting pyroptosis have potential value in managing inflammation and hence reducing COVID-19 severity and mortality.
In conclusion, our data reveal different patterns of pyroptosis of blood leukocytes in patients with COVID-19, which is closely related to their prognosis. We speculate the mechanism underlying diverse prognoses is shown in Figure 8. Prognosis prediction models developed based on different pyroptosis patterns are highly valuable for COVID-19 treatment. In addition, compounds that target the transcription factor network that regulates the pyroptotic process may help to develop new drugs for the treatment of patients with severe COVID-19.
The dataset GSE157103 used in this study is available from: National Center for Biotechnology Information (NCBI), Gene Expression Omnibus (GEO), https://www.ncbi.nlm.nih.gov/geo/query/acc.cgi?acc=GSE157103. The dataset MSV000085703 is available from: the MassIVE database (accession number MSV000085703; https://doi.org/10.25345/C5F74G).
YT, PZ, and LC designed and supervised the study, PZ collected the data, PZ performed the first part of the analysis, and YT completed the analysis and drafted the manuscript. YT, PZ, and QL prepared the original draft and wrote, reviewed, and edited the manuscript. JX and LC revised the manuscript and provided analytical technical support. All authors contributed to the article and approved the submitted version.
This research was funded by the National Natural Science Foundation of China (32170750).
The authors declare that the research was conducted in the absence of any commercial or financial relationships that could be construed as a potential conflict of interest.
All claims expressed in this article are solely those of the authors and do not necessarily represent those of their affiliated organizations, or those of the publisher, the editors and the reviewers. Any product that may be evaluated in this article, or claim that may be made by its manufacturer, is not guaranteed or endorsed by the publisher.
We acknowledge the GEO and MassIVE databases for providing their platforms and contributors for uploading their meaningful datasets. We also thank the National Natural Science Foundation of China for supporting and funding this research.
The Supplementary Material for this article can be found online at: https://www.frontiersin.org/articles/10.3389/fimmu.2022.888661/full#supplementary-material
1. WHO. Covid-19 Dashboard. (2020) Geneva: World Health Organization, 2020. Available online: https://covid19.who.int/ (last cited: [20 May, 2022]).
2. Behrens EM, Koretzky GA. Review: Cytokine Storm Syndrome: Looking Toward the Precision Medicine Era {Gong, 2020 #1322}. Arthritis Rheumatol (2017) 69(6):1135–43. doi: 10.1002/art.40071
3. Ramasamy S, Subbian S. Critical Determinants of Cytokine Storm and Type I Interferon Response in Covid-19 Pathogenesis. Clin Microbiol Rev (2021) 34(3):e00299–20. doi: 10.1128/CMR.00299-20
4. Huang C, Wang Y, Li X, Ren L, Zhao J, Hu Y, et al. Clinical Features of Patients Infected With 2019 Novel Coronavirus in Wuhan, China. Lancet (2020) 395(10223):497–506. doi: 10.1016/S0140-6736(20)30183-5
5. Gong J, Dong H, Xia Q-S, Huang Z-Y, Wang D-K, Zhao Y, et al. Correlation Analysis between Disease Severity and Inflammation-Related Parameters in Patients with Covid-19: A Retrospective Study. BMC Infect Dis (2020) 20(1):963. doi: 10.1186/s12879-020-05681-5
6. Rodrigues TS, de Sá KSG, Ishimoto AY, Becerra A, Oliveira S, Almeida L, et al. Inflammasomes Are Activated in Response to Sars-Cov-2 Infection and Are Associated With Covid-19 Severity in Patients. J Exp Med (2021) 218(3). doi: 10.1084/jem.e20201707
7. Moore JB, June CH. Cytokine Release Syndrome in Severe Covid-19. Sci (New York NY) (2020) 368(6490):473–4. doi: 10.1126/science.abb8925
8. Sims JT, Krishnan V, Chang C-Y, Engle SM, Casalini G, Rodgers GH, et al. Characterization of the Cytokine Storm Reflects Hyperinflammatory Endothelial Dysfunction in Covid-19. J Allergy Clin Immunol (2021) 147(1):107–11. doi: 10.1016/j.jaci.2020.08.031
9. Kim JS, Lee JY, Yang JW, Lee KH, Effenberger M, Szpirt W, et al. Immunopathogenesis and Treatment of Cytokine Storm in Covid-19. Theranostics (2021) 11(1):316–29. doi: 10.7150/thno.49713
10. Jose RJ, Manuel A. Covid-19 Cytokine Storm: The Interplay Between Inflammation and Coagulation. Lancet Respir Med (2020) 8(6):e46–e7. doi: 10.1016/S2213-2600(20)30216-2
11. Mehta P, McAuley DF, Brown M, Sanchez E, Tattersall RS, Manson JJ. Covid-19: Consider Cytokine Storm Syndromes and Immunosuppression. Lancet (2020) 395(10229):1033–4. doi: 10.1016/S0140-6736(20)30628-0
12. Gupta S, Wang W, Hayek SS, Chan L, Mathews KS, Melamed ML, et al. Association Between Early Treatment With Tocilizumab and Mortality Among Critically Ill Patients With Covid-19. JAMA Intern Med (2021) 181(1):41–51. doi: 10.1001/jamainternmed.2020.6252
13. Xu X, Han M, Li T, Sun W, Wang D, Fu B, et al. Effective Treatment of Severe Covid-19 Patients With Tocilizumab. Proc Natl Acad Sci Unite States America (2020) 117(20):10970–5. doi: 10.1073/pnas.2005615117
14. Cao Y, Wei J, Zou L, Jiang T, Wang G, Chen L, et al. Ruxolitinib in Treatment of Severe Coronavirus Disease 2019 (Covid-19): A Multicenter, Single-Blind, Randomized Controlled Trial. J Allergy Clin Immunol (2020) 146(1):137–46.e3. doi: 10.1016/j.jaci.2020.05.019
15. Ramiro S, Mostard RLM, Magro-Checa C, van Dongen CMP, Dormans T, Buijs J, et al. Historically Controlled Comparison of Glucocorticoids With or Without Tocilizumab Versus Supportive Care Only in Patients With Covid-19-Associated Cytokine Storm Syndrome: Results of the Chic Study. Ann Rheum Dis (2020) 79(9):1143–51. doi: 10.1136/annrheumdis-2020-218479
16. Ferreira AC, Soares VC, de Azevedo-Quintanilha IG, Dias S, Fintelman-Rodrigues N, Sacramento CQ, et al. Sars-Cov-2 Engages Inflammasome and Pyroptosis in Human Primary Monocytes. Cell Death Discovery (2021) 7(1):43–. doi: 10.1038/s41420-021-00428-w
17. Zhang J, Wu H, Yao X, Zhang D, Zhou Y, Fu B, et al. Pyroptotic Macrophages Stimulate the Sars-Cov-2-Associated Cytokine Storm. Cell Mol Immunol (2021) 18(5):1305–7. doi: 10.1038/s41423-021-00665-0
18. Yap JKY, Moriyama M, Iwasaki A. Inflammasomes and Pyroptosis as Therapeutic Targets for Covid-19. J Immunol (2020) 205(2):307–12. doi: 10.4049/jimmunol.2000513
19. Liu Y, Fang Y, Chen X, Wang Z, Liang X, Zhang T, et al. Gasdermin E-Mediated Target Cell Pyroptosis by Car T Cells Triggers Cytokine Release Syndrome. Sci Immunol (2020) 5(43):eaax7969. doi: 10.1126/sciimmunol.aax7969
20. Shi J, Gao W, Shao F. Pyroptosis: Gasdermin-Mediated Programmed Necrotic Cell Death. Trends Biochem Sci (2017) 42(4):245–54. doi: 10.1016/j.tibs.2016.10.004
21. Shi J, Zhao Y, Wang K, Shi X, Wang Y, Huang H, et al. Cleavage of Gsdmd by Inflammatory Caspases Determines Pyroptotic Cell Death. Nature (2015) 526(7575):660–5. doi: 10.1038/nature15514
22. Ding J, Wang K, Liu W, She Y, Sun Q, Shi J, et al. Pore-Forming Activity and Structural Autoinhibition of the Gasdermin Family. Nature (2016) 535(7610):111–6. doi: 10.1038/nature18590
23. Wei Y, Yang L, Pandeya A, Cui J, Zhang Y, Li Z. Pyroptosis-Induced Inflammation and Tissue Damage. J Mol Biol (2022) 434(4):167301. doi: 10.1016/j.jmb.2021.167301
24. Wan X, Li J, Wang Y, Yu X, He X, Shi J, et al. H7n9 Virus Infection Triggers Lethal Cytokine Storm by Activating Gasdermin E-Mediated Pyroptosis of Lung Alveolar Epithelial Cells. Natl Sci Rev (2021) 9(1):nwab137–nwab. doi: 10.1093/nsr/nwab137
25. Jorgensen I, Zhang Y, Krantz BA, Miao EA. Pyroptosis Triggers Pore-Induced Intracellular Traps (Pits) That Capture Bacteria and Lead to Their Clearance by Efferocytosis. J Exp Med (2016) 213(10):2113–28. doi: 10.1084/jem.20151613
26. Miao EA, Leaf IA, Treuting PM, Mao DP, Dors M, Sarkar A, et al. Caspase-1-Induced Pyroptosis Is an Innate Immune Effector Mechanism Against Intracellular Bacteria. Nat Immunol (2010) 11(12):1136–42. doi: 10.1038/ni.1960
27. von Moltke J, Trinidad NJ, Moayeri M, Kintzer AF, Wang SB, van Rooijen N, et al. Rapid Induction of Inflammatory Lipid Mediators by the Inflammasome in Vivo. Nature (2012) 490(7418):107–11. doi: 10.1038/nature11351
28. Duewell P, Kono H, Rayner KJ, Sirois CM, Vladimer G, Bauernfeind FG, et al. Nlrp3 Inflammasomes Are Required for Atherogenesis and Activated by Cholesterol Crystals. Nature (2010) 464(7293):1357–61. doi: 10.1038/nature08938
29. Pan J, Han L, Guo J, Wang X, Liu D, Tian J, et al. Aim2 Accelerates the Atherosclerotic Plaque Progressions in Apoe-/- Mice. Biochem Biophys Res Commun (2018) 498(3):487–94. doi: 10.1016/j.bbrc.2018.03.005
30. Kawaguchi M, Takahashi M, Hata T, Kashima Y, Usui F, Morimoto H, et al. Inflammasome Activation of Cardiac Fibroblasts Is Essential for Myocardial Ischemia/Reperfusion Injury. Circulation (2011) 123(6):594–604. doi: 10.1161/CIRCULATIONAHA.110.982777
31. Khanova E, Wu R, Wang W, Yan R, Chen Y, French SW, et al. Pyroptosis by Caspase11/4-Gasdermin-D Pathway in Alcoholic Hepatitis in Mice and Patients. Hepatology (2018) 67(5):1737–53. doi: 10.1002/hep.29645
32. Shin MS, Kang Y, Lee N, Kim SH, Kang KS, Lazova R, et al. U1-Small Nuclear Ribonucleoprotein Activates the Nlrp3 Inflammasome in Human Monocytes. J Immunol (2012) 188(10):4769–75. doi: 10.4049/jimmunol.1103355
33. Shin MS, Kang Y, Wahl ER, Park H-J, Lazova R, Leng L, et al. Macrophage Migration Inhibitory Factor Regulates U1 Small Nuclear Rnp Immune Complex-Mediated Activation of the Nlrp3 Inflammasome. Arthritis Rheumatol (2019) 71(1):109–20. doi: 10.1002/art.40672
34. Sun R, Peng M, Xu P, Huang F, Xie Y, Li J, et al. Low-Density Lipoprotein Receptor (Ldlr) Regulates Nlrp3-Mediated Neuronal Pyroptosis Following Cerebral Ischemia/Reperfusion Injury. J Neuroinflamm (2020) 17(1):330. doi: 10.1186/s12974-020-01988-x
35. Junqueira C, Crespo Â, Ranjbar S, de Lacerda LB, Lewandrowski M, Ingber J, et al. Fcγr-Mediated Sars-Cov-2 Infection of Monocytes Activates Inflammation. Nature (2021) 606(7914):576–84. doi: 10.1038/s41586-022-04702-4
36. Eisfeld HS, Simonis A, Winter S, Chhen J, Ströh LJ, Krey T, et al. Viral Glycoproteins Induce Nlrp3 Inflammasome Activation and Pyroptosis in Macrophages. Viruses (2021) 13(10):2076. doi: 10.3390/v13102076
37. Shi J, Zhou J, Zhang X, Hu W, Zhao J-F, Wang S, et al. Single-Cell Transcriptomic Profiling of Mait Cells in Patients With Covid-19. Front Immunol (2021) 12:700152. doi: 10.3389/fimmu.2021.700152
38. Sun X, Yu J, Wong SH, Chan MTV, Zhang L, Wu WKK. Sars-Cov-2 Targets the Lysosome to Mediate Airway Inflammatory Cell Death. Autophagy (2022), 1–3. doi: 10.1080/15548627.2021.2021496
39. Sun X, Liu Y, Huang Z, Xu W, Hu W, Yi L, et al. Sars-Cov-2 Non-Structural Protein 6 Triggers Nlrp3-Dependent Pyroptosis by Targeting Atp6ap1. Cell Death Differ (2022) 29:1240–1254. doi: 10.1038/s41418-021-00916-7
40. Ma J, Zhu F, Zhao M, Shao F, Yu D, Ma J, et al. Sars-Cov-2 Nucleocapsid Suppresses Host Pyroptosis by Blocking Gasdermin D Cleavage. EMBO J (2021) 40(18):e108249. doi: 10.15252/embj.2021108249
41. Zhao L, Li L, Xue M, Liu X, Jiang C, Wang W, et al. Gasdermin D Inhibits Coronavirus Infection by Promoting the Noncanonical Secretion of Beta Interferon. mBio (2022) 13:e0360021. doi: 10.1128/mbio.03600-21
42. Karki R, Sharma BR, Tuladhar S, Williams EP, Zalduondo L, Samir P, et al. Synergism of Tnf-A and Ifn-Γ Triggers Inflammatory Cell Death, Tissue Damage, and Mortality in Sars-Cov-2 Infection and Cytokine Shock Syndromes. Cell (2021) 184(1):149–68.e17. doi: 10.1016/j.cell.2020.11.025
43. Cui H, Zhang L. Key Components of Inflammasome and Pyroptosis Pathways Are Deficient in Canines and Felines, Possibly Affecting Their Response to Sars-Cov-2 Infection. Front Immunol (2020) 11:592622. doi: 10.3389/fimmu.2020.592622
44. Overmyer KA, Shishkova E, Miller IJ, Balnis J, Bernstein MN, Peters-Clarke TM, et al. Large-Scale Multi-Omic Analysis of Covid-19 Severity. Cell Syst (2021) 12(1):23–40.e7. doi: 10.1016/j.cels.2020.10.003
45. Barbie DA, Tamayo P, Boehm JS, Kim SY, Moody SE, Dunn IF, et al. Systematic Rna Interference Reveals That Oncogenic Kras-Driven Cancers Require Tbk1. Nature (2009) 462(7269):108–12. doi: 10.1038/nature08460
46. Charoentong P, Finotello F, Angelova M, Mayer C, Efremova M, Rieder D, et al. Pan-Cancer Immunogenomic Analyses Reveal Genotype-Immunophenotype Relationships and Predictors of Response to Checkpoint Blockade. Cell Rep (2017) 18(1):248–62. doi: 10.1016/j.celrep.2016.12.019
47. Zhang B, Wu Q, Li B, Wang D, Wang L, Zhou YL. M(6)a Regulator-Mediated Methylation Modification Patterns and Tumor Microenvironment Infiltration Characterization in Gastric Cancer. Mol Cancer (2020) 19(1):53–. doi: 10.1186/s12943-020-01170-0
48. Subramanian A, Tamayo P, Mootha VK, Mukherjee S, Ebert BL, Gillette MA, et al. Gene Set Enrichment Analysis: A Knowledge-Based Approach for Interpreting Genome-Wide Expression Profiles. Proc Natl Acad Sci (2005) 102(43):15545. doi: 10.1073/pnas.0506580102
49. Nicolle R, Radvanyi F, Elati M. Coregnet: Reconstruction and Integrated Analysis of Co-Regulatory Networks. Bioinformatics (2015) 31(18):3066–8. doi: 10.1093/bioinformatics/btv305
50. Rouillard AD, Gundersen GW, Fernandez NF, Wang Z, Monteiro CD, McDermott MG, et al. The Harmonizome: A Collection of Processed Datasets Gathered to Serve and Mine Knowledge About Genes and Proteins. Database (2016) 2016:baw100. doi: 10.1093/database/baw100
51. Mendez D, Gaulton A, Bento AP, Chambers J, De Veij M, Félix E, et al. Chembl: Towards Direct Deposition of Bioassay Data. Nucleic Acids Res (2019) 47(D1):D930–D40. doi: 10.1093/nar/gky1075
52. Zhou C, Li C, Zheng Y, Liu X. Identification of Pyroptosis-Related Signature for Cervical Cancer Predicting Prognosis. Aging (Albany NY) (2021) 13(22):24795–814. doi: 10.18632/aging.203716
53. Zhao L-R, Xing R-L, Wang P-M, Zhang N-S, Yin S-J, Li X-C, et al. Nlrp1 and Nlrp3 Inflammasomes Mediate Lps/Atp−Induced Pyroptosis in Knee Osteoarthritis. Mol Med Rep (2018) 17(4):5463–9. doi: 10.3892/mmr.2018.8520
54. Correa RG, Milutinovic S, Reed JC. Roles of Nod1 (Nlrc1) and Nod2 (Nlrc2) in Innate Immunity and Inflammatory Diseases. Biosci Rep (2012) 32(6):597–608. doi: 10.1042/BSR20120055
55. Zheng M, Gao Y, Wang G, Song G, Liu S, Sun D, et al. Functional Exhaustion of Antiviral Lymphocytes in Covid-19 Patients. Cell Mol Immunol (2020) 17(5):533–5. doi: 10.1038/s41423-020-0402-2
56. Eichholz K, Bru T, Tran TTP, Fernandes P, Welles H, Mennechet FJD, et al. Immune-Complexed Adenovirus Induce Aim2-Mediated Pyroptosis in Human Dendritic Cells. PloS Pathog (2016) 12(9):e1005871. doi: 10.1371/journal.ppat.1005871
57. Linder A, Bauernfried S, Cheng Y, Albanese M, Jung C, Keppler OT, et al. Card8 Inflammasome Activation Triggers Pyroptosis in Human T Cells. EMBO J (2020) 39(19):e105071–e. doi: 10.15252/embj.2020105071
58. Robinson N, Ganesan R, Hegedűs C, Kovács K, Kufer TA, Virág L. Programmed Necrotic Cell Death of Macrophages: Focus on Pyroptosis, Necroptosis, and Parthanatos. Redox Biol (2019) 26:101239. doi: 10.1016/j.redox.2019.101239
59. Karmakar M, Minns M, Greenberg EN, Diaz-Aponte J, Pestonjamasp K, Johnson JL, et al. N-Gsdmd Trafficking to Neutrophil Organelles Facilitates Il-1β Release Independently of Plasma Membrane Pores and Pyroptosis. Nat Commun (2020) 11(1):2212. doi: 10.1038/s41467-020-16043-9
60. Hage C, Hoves S, Strauss L, Bissinger S, Prinz Y, Pöschinger T, et al. Sorafenib Induces Pyroptosis in Macrophages and Triggers Natural Killer Cell-Mediated Cytotoxicity Against Hepatocellular Carcinoma. Hepatology (2019) 70(4):1280–97. doi: 10.1002/hep.30666
61. Mokry RL, Schumacher ML, Hogg N, Terhune SS. Nitric Oxide Circumvents Virus-Mediated Metabolic Regulation During Human Cytomegalovirus Infection. mBio (2020) 11(6):e02630-20. doi: 10.1128/mBio.02630-20
62. Liu C, Wen L, Xiao Q, He K. Nitric Oxide-Generating Compound Gsno Suppresses Porcine Circovirus Type 2 Infection in Vitro and in Vivo. BMC Vet Res (2017) 13(1):59. doi: 10.1186/s12917-017-0976-9
63. Pieretti JC, Rubilar O, Weller RB, Tortella GR, Seabra AB. Nitric Oxide (No) and Nanoparticles - Potential Small Tools for the War Against Covid-19 and Other Human Coronavirus Infections. Virus Res (2021) 291:198202–. doi: 10.1016/j.virusres.2020.198202
64. Qin C, Zhou L, Hu Z, Zhang S, Yang S, Tao Y, et al. Dysregulation of Immune Response in Patients With Coronavirus 2019 (Covid-19) in Wuhan, China. Clin Infect Dis (2020) 71(15):762–8. doi: 10.1093/cid/ciaa248
65. Gong J, Dong H, Xia Q-S, Huang Z-Y, Wang D-K, Zhao Y, et al. Correlation Analysis Between Disease Severity and Inflammation-Related Parameters in Patients With Covid-19: A Retrospective Study. BMC Infect Dis (2020) 20(1):963. doi: 10.1186/s12879-020-05681-5
66. Mauracher LM, Posch F, Martinod K, Grilz E, Däullary T, Hell L, et al. Citrullinated Histone H3, a Biomarker of Neutrophil Extracellular Trap Formation, Predicts the Risk of Venous Thromboembolism in Cancer Patients. J Thromb Haemost (2018) 16(3):508–18. doi: 10.1111/jth.13951
67. Middleton EA, He X-Y, Denorme F, Campbell RA, Ng D, Salvatore SP, et al. Neutrophil Extracellular Traps Contribute to Immunothrombosis in Covid-19 Acute Respiratory Distress Syndrome. Blood (2020) 136(10):1169–79. doi: 10.1182/blood.2020007008
68. Castro de Moura M, Davalos V, Planas-Serra L, Alvarez-Errico D, Arribas C, Ruiz M, et al. Epigenome-Wide Association Study of Covid-19 Severity With Respiratory Failure. EBioMedicine (2021) 66:103339. doi: 10.1016/j.ebiom.2021.103339
69. Zhang D, Guo R, Lei L, Liu H, Wang Y, Wang Y, et al. Frontline Science: Covid-19 Infection Induces Readily Detectable Morphologic and Inflammation-Related Phenotypic Changes in Peripheral Blood Monocytes. J leukocyte Biol (2021) 109(1):13–22. doi: 10.1002/JLB.4HI0720-470R
70. Zhang Z, Zheng Y, Niu Z, Zhang B, Wang C, Yao X, et al. Sars-Cov-2 Spike Protein Dictates Syncytium-Mediated Lymphocyte Elimination. Cell Death Differ (2021) 28(9):2765–77. doi: 10.1038/s41418-021-00782-3
71. Jing Y, Luo L, Chen Y, Westerberg LS, Zhou P, Xu Z, et al. Sars-Cov-2 Infection Causes Immunodeficiency in Recovered Patients by Downregulating Cd19 Expression in B Cells Via Enhancing B-Cell Metabolism. Signal Trans Target Ther (2021) 6(1):345. doi: 10.1038/s41392-021-00749-3
72. White KM, Rosales R, Yildiz S, Kehrer T, Miorin L, Moreno E, et al. Plitidepsin Has Potent Preclinical Efficacy Against Sars-Cov-2 by Targeting the Host Protein Eef1a. Sci (New York NY) (2021) 371(6532):926–31. doi: 10.1126/science.abf4058
73. Li Q, Verma IM. Nf-Kappab Regulation in the Immune System. Nat Rev Immunol (2002) 2(10):725–34. doi: 10.1038/nri910
74. Kolesnichenko M, Mikuda N, Höpken UE, Kärgel E, Uyar B, Tufan AB, et al. Transcriptional Repression of Nfkbia Triggers Constitutive Ikk- and Proteasome-Independent P65/Rela Activation in Senescence. EMBO J (2021) 40(6):e104296–e. doi: 10.15252/embj.2019104296
75. Li S, Wu B, Ling Y, Guo M, Qin B, Ren X, et al. Epigenetic Landscapes of Single-Cell Chromatin Accessibility and Transcriptomic Immune Profiles of T Cells in Covid-19 Patients. Front Immunol (2021) 12:625881. doi: 10.3389/fimmu.2021.625881
76. Ji J, Ding K, Luo T, Zhang X, Chen A, Zhang D, et al. Trim22 Activates Nf-Kb Signaling in Glioblastoma by Accelerating the Degradation of Iκbα. Cell Death Differ (2021) 28(1):367–81. doi: 10.1038/s41418-020-00606-w
77. Wang Y, Chen Y, Lin Y, Quan Y, Xiao X, Zhang R. Trim22 Inhibits Respiratory Syncytial Virus Replication by Targeting Jak-Stat1/2 Signaling. J Med Virol (2021) 93(6):3412–9. doi: 10.1002/jmv.26436
78. Asefa B, Klarmann KD, Copeland NG, Gilbert DJ, Jenkins NA, Keller JR. The Interferon-Inducible P200 Family of Proteins: A Perspective on Their Roles in Cell Cycle Regulation and Differentiation. Blood Cell Mol Dis (2004) 32(1):155–67. doi: 10.1016/j.bcmd.2003.10.002
79. Bottardi S, Guieze R, Bourgoin V, Fotouhi-Ardakani N, Dougé A, Darracq A, et al. Mnda Controls the Expression of Mcl-1 and Bcl-2 in Chronic Lymphocytic Leukemia Cells. Exp Hematol (2020) 88:68–82.e5. doi: 10.1016/j.exphem.2020.07.004
80. Li X, Qiu Q, Li M, Lin H, Cao S, Wang Q, et al. Chemical Composition and Pharmacological Mechanism of Ephedra-Glycyrrhiza Drug Pair Against Coronavirus Disease 2019 (Covid-19). Aging (Albany NY) (2021) 13(4):4811–30. doi: 10.18632/aging.202622
81. Yang X, Cheng X, Tang Y, Qiu X, Wang Y, Kang H, et al. Bacterial Endotoxin Activates the Coagulation Cascade Through Gasdermin D-Dependent Phosphatidylserine Exposure. Immunity (2019) 51(6):983–96.e6. doi: 10.1016/j.immuni.2019.11.005
82. Wu C, Lu W, Zhang Y, Zhang G, Shi X, Hisada Y, et al. Inflammasome Activation Triggers Blood Clotting and Host Death Through Pyroptosis. Immunity (2019) 50(6):1401–11.e4. doi: 10.1016/j.immuni.2019.04.003
83. Soy M, Keser G, Atagündüz P, Tabak F, Atagündüz I, Kayhan S. Cytokine Storm in Covid-19: Pathogenesis and Overview of Anti-Inflammatory Agents Used in Treatment. Clin Rheumatol (2020) 39(7):2085–94. doi: 10.1007/s10067-020-05190-5
84. Chen G, Wu D, Guo W, Cao Y, Huang D, Wang H, et al. Clinical and Immunological Features of Severe and Moderate Coronavirus Disease 2019. J Clin Invest (2020) 130(5):2620–9. doi: 10.1172/JCI137244
85. Sefik E, Qu R, Junqueira C, Kaffe E, Mirza H, Zhao J, et al. Inflammasome Activation in Infected Macrophages Drives Covid-19 Pathology. Nature (2022) 606:585–593. doi: 10.1038/s41586-022-04802-1
86. Rogers C, Fernandes-Alnemri T, Mayes L, Alnemri D, Cingolani G, Alnemri ES. Cleavage of Dfna5 by Caspase-3 During Apoptosis Mediates Progression to Secondary Necrotic/Pyroptotic Cell Death. Nat Commun (2017) 8:14128. doi: 10.1038/ncomms14128
87. Zhang J-Y, Zhou B, Sun R-Y, Ai Y-L, Cheng K, Li F-N, et al. The Metabolite A-Kg Induces Gsdmc-Dependent Pyroptosis Through Death Receptor 6-Activated Caspase-8. Cell Res (2021) 31(9):980–97. doi: 10.1038/s41422-021-00506-9
88. Chen H, Deng Y, Gan X, Li Y, Huang W, Lu L, et al. Nlrp12 Collaborates With Nlrp3 and Nlrc4 to Promote Pyroptosis Inducing Ganglion Cell Death of Acute Glaucoma. Mol Neurodegener (2020) 15(1):26. doi: 10.1186/s13024-020-00372-w
89. Wang Y, Gao W, Shi X, Ding J, Liu W, He H, et al. Chemotherapy Drugs Induce Pyroptosis Through Caspase-3 Cleavage of a Gasdermin. Nature (2017) 547(7661):99–103. doi: 10.1038/nature22393
90. Gupta A, Gonzalez-Rojas Y, Juarez E, Crespo Casal M, Moya J, Falci DR, et al. Early Treatment for Covid-19 with Sars-Cov-2 Neutralizing Antibody Sotrovimab. N Engl J Med (2021) 385(21):1941–50. doi: 10.1056/NEJMoa2107934
91. Dougan M, Nirula A, Azizad M, Mocherla B, Gottlieb RL, Chen P, et al. Bamlanivimab Plus Etesevimab in Mild or Moderate Covid-19. N Engl J Med (2021) 385(15):1382–92. doi: 10.1056/NEJMoa2102685
92. Jones BE, Brown-Augsburger PL, Corbett KS, Westendorf K, Davies J, Cujec TP, et al. The Neutralizing Antibody, Ly-Cov555, Protects Against Sars-Cov-2 Infection in Nonhuman Primates. Sci Transl Med (2021) 13(593):eabf1906. doi: 10.1126/scitranslmed.abf1906
93. Singh AK, Singh A, Singh R, Misra A. Remdesivir in Covid-19: A Critical Review of Pharmacology, Pre-Clinical and Clinical Studies. Diabetes Metab Syndr (2020) 14(4):641–8. doi: 10.1016/j.dsx.2020.05.018
94. Mahase E. Covid-19: Pfizer's Paxlovid Is 89% Effective in Patients at Risk of Serious Illness, Company Reports. BMJ (2021) 375:n2713. doi: 10.1136/bmj.n2713
95. Kabinger F, Stiller C, Schmitzová J, Dienemann C, Kokic G, Hillen HS, et al. Mechanism of Molnupiravir-Induced Sars-Cov-2 Mutagenesis. Nat Struct Mol Biol (2021) 28(9):740–6. doi: 10.1038/s41594-021-00651-0
96. Mølhave M, Agergaard J, Wejse C. Clinical Management of Covid-19 Patients - an Update. Semin Nucl Med (2022) 52(1):4–10. doi: 10.1053/j.semnuclmed.2021.06.004
97. Malgie J, Schoones JW, Pijls BG. Decreased Mortality in Coronavirus Disease 2019 Patients Treated With Tocilizumab: A Rapid Systematic Review and Meta-Analysis of Observational Studies. Clin Infect Dis (2021) 72(11):e742–e9. doi: 10.1093/cid/ciaa1445
98. Marconi VC, Ramanan AV, de Bono S, Kartman CE, Krishnan V, Liao R, et al. Efficacy and Safety of Baricitinib for the Treatment of Hospitalised Adults With Covid-19 (Cov-Barrier): A Randomised, Double-Blind, Parallel-Group, Placebo-Controlled Phase 3 Trial. Lancet Respir Med (2021) 9(12):1407–18. doi: 10.1016/S2213-2600(21)00331-3
Keywords: COVID-19, pyroptosis, leukocytes, prognosis model, transcription factors
Citation: Tang Y, Zhang P, Liu Q, Cao L and Xu J (2022) Pyroptotic Patterns in Blood Leukocytes Predict Disease Severity and Outcome in COVID-19 Patients. Front. Immunol. 13:888661. doi: 10.3389/fimmu.2022.888661
Received: 03 March 2022; Accepted: 17 June 2022;
Published: 19 July 2022.
Edited by:
Camilla Tincati, University of Milan, ItalyReviewed by:
Talia H Swartz, Icahn School of Medicine at Mount Sinai, United StatesCopyright © 2022 Tang, Zhang, Liu, Cao and Xu. This is an open-access article distributed under the terms of the Creative Commons Attribution License (CC BY). The use, distribution or reproduction in other forums is permitted, provided the original author(s) and the copyright owner(s) are credited and that the original publication in this journal is cited, in accordance with accepted academic practice. No use, distribution or reproduction is permitted which does not comply with these terms.
*Correspondence: Jingsong Xu, jingsong.xu@hotmail.com; Luyang Cao, cao_luyang@grmh-gdl.cn
†These authors have contributed equally to this work and share first authorship
Disclaimer: All claims expressed in this article are solely those of the authors and do not necessarily represent those of their affiliated organizations, or those of the publisher, the editors and the reviewers. Any product that may be evaluated in this article or claim that may be made by its manufacturer is not guaranteed or endorsed by the publisher.
Research integrity at Frontiers
Learn more about the work of our research integrity team to safeguard the quality of each article we publish.