Corrigendum: Immunoinformatics approach toward the introduction of a novel multi-epitope vaccine against Clostridium difficile
- 1Infection Control Center, Xiangya Hospital, Central South University, Changsha, China
- 2Center of Respiratory Medicine, Xiangya Hospital, Central South University, Changsha, China
- 3National Clinical Research Center for Geriatric Disorders (XiangYa Hospital), Changsha, China
Clostridium difficile (C.difficile) is an exclusively anaerobic, spore-forming, and Gram-positive pathogen that is the most common cause of nosocomial diarrhea and is becoming increasingly prevalent in the community. Because C. difficile is strictly anaerobic, spores that can survive for months in the external environment contribute to the persistence and diffusion of C. difficile within the healthcare environment and community. Antimicrobial therapy disrupts the natural intestinal flora, allowing spores to develop into propagules that colonize the colon and produce toxins, thus leading to antibiotic-associated diarrhea and pseudomembranous enteritis. However, there is no licensed vaccine to prevent Clostridium difficile infection (CDI). In this study, a multi-epitope vaccine was designed using modern computer methods. Two target proteins, CdeC, affecting spore germination, and fliD, affecting propagule colonization, were chosen to construct the vaccine so that it could simultaneously induce the immune response against two different forms (spore and propagule) of C. difficile. We obtained the protein sequences from the National Center for Biotechnology Information (NCBI) database. After the layers of filtration, 5 cytotoxic T-cell lymphocyte (CTL) epitopes, 5 helper T lymphocyte (HTL) epitopes, and 7 B-cell linear epitopes were finally selected for vaccine construction. Then, to enhance the immunogenicity of the designed vaccine, an adjuvant was added to construct the vaccine. The Prabi and RaptorX servers were used to predict the vaccine’s two- and three-dimensional (3D) structures, respectively. Additionally, we refined and validated the structures of the vaccine construct. Molecular docking and molecular dynamics (MD) simulation were performed to check the interaction model of the vaccine–Toll-like receptor (TLR) complexes, vaccine–major histocompatibility complex (MHC) complexes, and vaccine–B-cell receptor (BCR) complex. Furthermore, immune stimulation, population coverage, and in silico molecular cloning were also conducted. The foregoing findings suggest that the final formulated vaccine is promising against the pathogen, but more researchers are needed to verify it.
1 Introduction
Clostridium difficile (C. difficile) was isolated by Hall and O’Toole in 1935 and recently reclassified as Clostridioides difficile (1). In the late 1970s, Bartlett etal. (2) identified C. difficile as a pathogen related to antibiotic-associated diarrhea. Clostridium difficile infection (CDI) not only places a heavy burden on the healthcare system but also has a significant negative impact on the community population, causing nearly 230,000 CDIs in the United States each year, resulting in over 12,000 deaths and more than $1 billion in economic burden (3). The CDI epidemic occurs not only in the United States but also in Europe (4), Asia (5), and Australia (6). A significant issue with CDI is that it not only has a high incidence rate but also has a high recurrence rate. CDI occurs in approximately 15%–30% of patients treated for the first time and in about 45%–65% of patients previously diagnosed with recurrent Clostridium difficile infection (rCDI) (7). To better address the aforementioned issues, vaccination may be a viable option.
In recent years, several vaccines have been developed, and some are in phases of clinical testing, which can be roughly divided into two broad categories, toxin-based vaccines and nontoxic vaccines (8, 9). Currently, three toxin-based vaccines have entered phase II and above clinical studies. Both CDIFFENSE, a formalin-inactivated toxin vaccine, and VLE84, a recombinant toxin-based vaccine, have achieved satisfactory results in phase I and phase II trials (10, 11). Another formalin-inactivated toxin-based vaccine, developed by Pfizer, has completed phase III trials (12). However, Spencer etal. (13) found that toxin-based vaccines have no effect on C. difficile colonization or spore spreading from the host to the environment but may lead to an increased number of asymptomatic carriers (13, 14). Considering these limitations, several passive immunotherapies against CDI (15, 16) targeting other pathogenic factors, such as flagellum, S-layer proteins (SLPs), Cell wall protein 84 (Cwp84), CD0873, Pilin, and spore proteins, have been taken into consideration. However, some of these vaccine candidates failed to induce a sufficient level of immunity and provided only partial protection against CDI-related death (15, 17–19).
Immunoinformatics development has opened a new door for vaccine research. Compared with traditional vaccines, the multi-epitope vaccine has many advantages. For instance, they do not require microbial culture, saving time and cost; highly promiscuous epitopes can be recognized and combined by multiple alleles at a time to overcome the differences of alleles among the human population; biological harmfulness and toxicity reversion of traditional inactivated or attenuated vaccines can be avoided. At present, multi-epitope vaccines for different diseases, such as HIV-1 (20), cancer (21), Chlamydia trachomatis (22), and parasites (23), have been investigated.
Because C. difficile is strictly anaerobic, it can only survive outside the host intestine as a spore (24). Spores that are resistant to heat, dryness, and a plethora of commonly used disinfectants can transfer to disease-causing vegetative cells when exposed to an available environment (25). Thus, an ideal vaccine should target not only C. difficile propagules but also C. difficile spores.
The outermost layer of C. difficile spores plays a pivotal role in spore–host interactions and contributes to the persistence of the spore in the host (26). CdeC, a cysteine-rich spore exosporium protein, not only plays an essential role in exosporium morphogenesis and the correctness of spore coat assembly but also affects the physicochemical properties of the spore (27, 28). Studies have indicated that CdeC is an immunogenic protein that protects mice against C. difficile UK1 and C. difficile 630Δerm (29, 30).
The flagellum is equipped with multiple biological functions, contributing to biofilm formation, not merely playing an important role in pathogen motility but also participating in adhesion to host cells. Moreover, recent studies have shown that flagellum also affects the expression of toxin genes (31). Flagellum, as the ligand of Toll-like receptor (TLR)5 (31), can trigger the secretion of inflammatory cytokines through the TLR5 signaling pathway, thus playing the role of the immune modulator. Due to its unique immunocompetence, the flagellum has already been introduced as a favorable vaccine candidate, and some of these vaccines have already entered clinical trials (32). FliC (flagellin) and fliD (cap) proteins are two indispensable components of flagellum. FliD shows little variability from different C. difficile strains (33), unlike fliC, which has pronounced variability in the central domain that constitutes the exposed antigen part of the surface of the flagellum filament (34). According to a study, 87% of patients diagnosed with CDI were found to have fliD-specific antibodies, which was higher than the level of fliC-specific antibodies (21%) in Clostridium difficile associated diarrhea (CDAD) patients (35). Hence, in this study, CdeC and fliD were selected as target proteins to design a multi-epitope vaccine that attempted to defend against CDI by blocking spore and C. difficile propagant adherence to the host gut tract.
2 Methodology
2.1 Obtaining Target Protein Amino Acid Sequences
Not only the amino acid sequences of CdeC (CBE03081.1) and fliD (CBE01872.1) in Fasta format were retrieved from the protein database of the National Center for Biotechnology Information (NCBI) (https://www.ncbi.nlm.nih.gov/) but also the protein sequence of adjuvant LT-IIb (ID: 5G3L_H). The protein structure of TLR2 (ID: 2Z7X), TLR4 (ID:4G8A), TLR5 (ID:3J0A), B-cell receptor (BCR) (5DRW), major histocompatibility complex (MHC) I (ID:4U6Y), and MHC II (ID:5JLZ) molecules were downloaded from the Protein Data Bank using accession numbers (https://www.rcsb.org/).
2.2 Cytotoxic T-Cell Lymphocyte Epitope Prediction
The CTL epitopes were predicted by the NetCTL 1.2 server (http://www.cbs.dtu.dk/services/NetCTL/) that integrates the prediction of peptide MHC class I binding, proteasomal C terminal cleavage, and Transporter associated with Antigen processing (TAP) transport efficiency across 12 MHC class I supertypes (A1, A2, A3, A24, A26, B7, B8, B27, B39, B44, B58, and B62) in the human population (36). The antigenicity of predicted CTL epitopes was assessed using Vaxijen v2.0 (http://www.ddg-pharmfac.net/vaxijen/VaxiJen/VaxiJen.html) (37), and epitopes with an antigenicity greater than 0.4 were considered antigens (38). To enhance the antigenicity of the vaccine, only the epitopes with antigenicity greater than 1 were finally selected for the construction of the vaccine. Immunogenicity was further calculated by the Immune Epitope Database (IEDB) server (http://tools.iedb.org/immunogenicity/) (39). Additionally, the AllerTOP v. 2.0 (https://www.ddg-pharmfac.net/AllerTOP/index.html) (40) and ToxinPred (https://webs.iiitd.edu.in/raghava/toxinpred/index.html) (41) were employed to check the allergenicity and toxicity of epitopes, respectively. MHC class I allelic partners of the antigenic epitopes were predicted via Tepitool (http://tools.iedb.org/tepitool/) (42), and alleles with inhibitory concentration (IC50) values ≤500 nM and percentile rank scores ≤2 were considered in this study.
2.3 Helper T Lymphocyte Epitope Prediction
The NetMHC II pan 3.2 server (http://www.cbs.dtu.dk/services/2.5NetMHCIIpan/) (43) was utilized to predict epitopes with an affinity to MHC class II alleles. Based on the affinity for 14 human leukocyte antigen (HLA) DR alleles (HLA-DRB1*01:01; HLA-DRB1*03:01; HLA-DRB1*04:01; HLA-DRB1*04:04; HLA-DRB1*04:05; HLA-DRB1*07:01; HLA-DRB3*02:02; HLA-DRB1*09:01; HLA-DRB1*11:01; HLA-DRB1*13:02; HLA-DRB1*15:01; HLA-DRB3*01:01; HLA-DRB4*01:01; HLA-DRB5*01:01), all HTL epitopes were classified as three-level, and 2% and 10% were selected as the thresholds for the strong and weak binders, respectively. The HTL epitopes with a strong affinity to HLA DR alleles and inhibitory concentration (IC50) ≤500 nM were further tested for antigenicity. Similar to CTL epitopes, the allergenicity and toxicity of HTL epitopes were also predicted by the AllerTOP and ToxinPred servers, respectively. Interferon-gamma (IFN-γ) and interleukin-4 (IL-4) play a significant role in regulating immune cell development and differentiation as well as organism immune response (44, 45). Thus, antigenic (with a threshold value of >0.4), nonallergic, and nontoxic epitopes were tested to see if they could induce IFN-γ and IL-4 secretion using the INFepitope server (http://crdd.osdd.net/raghava/ifnepitope/) (46) and the IL-4pred server (https://webs.iiitd.edu.in/raghava/il4pre) (47), respectively. Only those IFN-γ- and IL-4-inducing epitopes were covered in the vaccine construct. In addition to IFN-γ and IL-4, there are still many cytokines involved in the development, maturation, activation, and effector processes of different immune cell subpopulations (48). Therefore, the ProInflam server (http://metagenomics.iiserb.ac.in/proinflam/index.html) (49), which predicts the pro-inflammatory response of peptides based on machine learning-based models, was used to predict the capacity of HTL epitopes to induce the secretion of pro-inflammatory cytokines like tumor necrosis factor (TNF), IL-1, IL-18, IL-12, or IL-23. IL-2, which was initially called T-cell growth factor, has been demonstrated to promote the proliferation of T cells and B cells and activate the cytotoxic function of natural killer (NK) cells, T lymphocytes, and monocytes (50). Therefore, we also have checked the capacity of selected HTL epitopes to induce the generation of IL-2 by the IL-2pred server (https://webs.iiitd.edu.in/raghava/il2pred/predict.php).
2.4 B-Cell Epitope Prediction
We used the ABCpred server (https://webs.iiitd.edu.in/raghava/abcpred/) (51) to predict linear B-cell epitopes using an artificial neural network, and a window length of 16 was selected as the predicted epitope length. Then, these selected epitopes were tested for antigenicity with VaxiJen v2.0, and epitopes with antigenicity equal to or greater than 0.9 were finally considered in this study. As before, AllerTOP v. 2.0 and ToxinPred were applied to predict the allergenicity and toxicity, respectively.
2.5 Prediction of Epitope Similarity With Human Proteins
To ensure that the final vaccine was incapable of inducing autoimmune disorders and cross-reactivity, all selected epitopes by the above steps were checked for their similarity to human proteins through the Blast (https://blast.ncbi.nlm.nih.gov/Blast.cgi) (52).
2.6 Multi-Epitope Vaccine Designation
The ultimately selected epitopes screened from CdeC and fliD in the previous steps were used for constructing the multi-epitope vaccine. The CTL and HTL epitopes were connected through KK linkers, while B-cell epitopes were linked together by GPGPG linkers. LT-IIb, a type II heat-labile enterotoxin (HLT), enables the formation of a greater proportion of effector memory cells by slowing CD8+ T-cell expansion and contraction dynamics (53). Thus, to improve the immunogenic capacity of the vaccine, LT-IIb was chosen as an adjuvant to attach to the N-terminus of the vaccine construct via the EAAAK linker.
2.7 Multi-Epitope Vaccine Features
2.7.1 The Prediction of Physicochemical Properties of the Vaccine
We employed the ProtParam server (https://web.expasy.org/protparam/) (54) to predict the physical chemistry properties of the multi-epitope vaccine, including molecular weight, the number of amino acids, theoretical isoelectric point (pI), estimated half-life, instability index, aliphatic index, and grand average of hydropathicity (GRAVY). To comparatively evaluate the candidate vaccine’s properties, Brother of Regulator of Imprinted Sites (BORIS) antigens from both human and murine (C1) (55), a multi-epitope vaccine with immunodominant epitopes from six SARS-CoV-2 non-structural proteins and SARS-CoV-2 S protein (C2) (56), transmembrane protein 31 (TMEM31) (C3) (57), and a multi-epitope vaccine composed of immunodominant epitopes of (SYCP1) and ACRBP antigens (C4) (58) were chosen as positive controls.
2.7.2 The Prediction of Antigenicity, Allergenicity, and Toxicity of the Vaccine
Antigenicity prediction of the final vaccine construct was performed using the Vaxijen v2.0, ANTIGENpro (http://scratch.proteomics.ics.uci.edu/), and Secret-AAR servers (http://microbiomics.ibt.unam.mx/tools/aar/) (59). According to cross-validation studies, the ANTIGENpro server generates protein antigenicity indices with a 76% accuracy using specific microarray data (60). The Secret-AAR servers evaluate the protein’s antigenic density and antigenic potential based on its Abundance of Antigenic Regions (AAR) value. And a lower AAR value implies better protein antigenicity. Additionally, both AlgPred (http://crdd.osdd.net/raghava/algpred/) and AllerTOP v2.0 were used to check the allergenicity of the vaccine, and the toxicity of the vaccine was predicted by the ToxinPred server.
2.7.3 The Prediction of Transmembrane Domains, Probability of Solubility, and Signal Peptide of the Vaccine
We employed the TMHMM-2.0 to predict the transmembrane helices in the vaccine sequence. Based on multiple representations of the primary sequence, the SOLpro server (http://scratch.proteomics.ics.uci.edu/) predicted the propensity of a protein to be soluble upon overexpression in E. coli using a two-stage support vector machine (SVM) architecture with a precision of more than 74% (61). The signalP-6.0 online server (https://services.healthtech.dtu.dk/service.php?SignalP-6.0) (62) was employed to predict the signal peptide of the vaccine construct.
2.7.4 The Prediction of Immunoglobulin A-Specific B-Cell Epitopes of the Vaccine
As is well-known, C. difficile is an intestinal pathogen, and intestinal mucosal immunity is very important for protection against CDI (63). Mucosal immunity is mainly mediated by secretory immunoglobulin A (s1gA) secreted by IgA plasma cells (64, 65). Thus, to clarify the potential of the vaccine to induce the generation of s1gA, the IgPred server (https://webs.iiitd.edu.in/raghava/igpred/index.html#) (66) was used to predict the presence of IgA-specific B-cell epitopes in the vaccine. The length of the epitope was set at 20 amino acid residues, the default length of the server, and the screening threshold of the epitope was set at 0.5. The server has a filter threshold of -1 to 1, with a higher threshold implying a high probability of correct prediction but poor coverage/sensitivity.
2.8 Secondary Structure Prediction
Since the protein secondary structure is a key indicator of protein folding, the Prabi server was employed (https://npsa-prabi.ibcp.fr/cgi-bin/npsa_automat.pl?page=/NPSA/npsa.gor4.html) to predict the secondary structure of the vaccine sequences based on the Garnier-Osguthorpe-Robson 4 (GOR4) method with an average accuracy of 64.4% (67).
2.9 Three-Dimensional Modeling, Refinement, and Validation
In this study, the 3D structure of the designed vaccine was predicted with the help of RaptorX (http://raptorx.uchicago.edu/), which was more suitable for the prediction of targets without close homologs in the protein structure, using an ultradeep convolutional residual neural network from a primary sequence or multiple sequence alignments (68). Then, the obtained models were refined using the GalaxyWEB server (http://galaxy.seoklab.org/), which could refine the non-conserved and less reliable zones of the protein’s 3D structure and further generate five refined models of each primary 3D model (69). Based on the model’s Global Distance Test-High Accuracy (GDT-HA), the root-mean-square deviation (RMSD), Molprobity, the best model was selected and further submitted to the PROCHECK, ERRAT (https://saves.mbi.ucla.edu/), and ProSA Web servers (https://prosa.services.came.sbg.ac.at/prosa.php) to verify its quality. The Ramachandran plot generated by PROCHECK reflects the stereochemical quality of a protein structure, and a superior model has more residues in the Ramachandran-favored region. ERRAT (70) assesses the protein structures by analyzing the statistics of non-bonded interactions between different atom types. A model with an ERRAT score of more than 85 is considered to be of high quality, and a higher score indicates a better model. The Z score generated by the ProSA Web server evaluates the overall quality of the protein’s 3D structures, and a positive value indicates that there are errors in the 3D structures of the protein (71).
2.10 Molecular Docking
MHC molecules, which are involved in the recognition and presentation of antigenic peptides, are important for the activation of CD8+ T cells and CD4+ T cells (72). TLRs recognize pathogen-associated molecular patterns (PAMPs) on microorganisms when interacting with organisms, thus activating innate immune cells and inducing the expression and the secretion of a variety of pro-inflammatory cytokines. TLR4 and TLR2, which are expressed on the surface of a subgroup of immunological cells, are capable of recognizing a wide range of bacterial surface proteins, such as lipoteichoic acid (LTA), peptidoglycan (PGN), and lipoarabinomannan (73, 74). TLR5 is known to recognize flagellin proteins (75). B cells are responsible for the body’s humoral immune response (76). BCR acts as a gatekeeper on the surface of B cells; only those epitopes recognized and bound by BCR can further activate B cells. Therefore, predicting the interactions between the designed vaccine and TLRs (TLR2, TLR4, and TLR5), MHC (HLA-A*02:01 and HLA-DRB1*0401) molecules and BCR were strongly necessary. In this study, we first used the HADDOCK 2.4 server (https://wenmr.science.uu.nl/haddock2.4/) (77) to perform the molecular docking between vaccine and TLRs and MHC molecules. The clusPro server (78) has an antibody mode that provides a receptor mask of the non-complementarity determining regions (non-CDRs) that can be precisely complementary to the antigenic determinant of the protein, which indicated that the clusPro was more suitable to perform the molecular docking between vaccine and BCR. Thus, clusPro (https://cluspro.org/tutantibody.php) was selected to conduct the molecular docking for vaccine and BCR in this study. Meanwhile, to further verify vaccine affinity between vaccine and receptors, the docking between vaccine and receptors (TLRs, MHC molecules, and BCR) was also performed by Rosetta software (79). The TLRs and MHC molecules act as receptors, and the designed vaccine acts as a ligand. The detailed docking steps were as follows: (1) A box with the greatest range around the receptor’s geometric center was formed, and amino acids within 1.2 nm of the binding region on the vaccine were placed into the grid box for docking. The different types of atoms were then used as probes to scan and calculate the grid energy; (2) Rosetta software performed a conformational search for the ligand within the range of the box, and the final rankings were determined according to the scores based on the conformation, orientation, position, and energy of the ligand; (3) A total of 1,000 intermolecular interaction phases were obtained, and the ranking was performed based on the scores of molecular docking energy; (4) Cluster analysis was conducted by the built-in cluster analysis module of Rosetta software, and the complex system with the maximum clustering phase and the lowest binding energy score was chosen for further research.
2.11 Molecular Dynamics Simulation
Molecular dynamics (MD) simulation was conducted by Amber18 software (80, 81) to better understand the dynamics and stability of the optional vaccine–receptor docking complexes, and it runs a total of 80 ns. Initially, the Amber99SB force field (82) was applied to generate the parameters of vaccine and receptors, and the force field of heavy atoms with different bond angles was set. Then, taking the protein complex system as the center, adding a cubic water box of 1 nm and Na+ to make the system electrically neutral and preserve the topology and coordinate structure stable. During the preprocessing stage, two-step energy minimization was performed, first restricting the protein to minimize the energy of the water molecule and then releasing the protein to minimize the energy of the whole system. The first energy minimization was performed over 5,000 cycles, with 1,500 cycles using the fastest descent method; the second energy minimization was run over 5,000 cycles, with 2,000 cycles using the fastest descent method. The system temperature gradually increased from 0 to 300 K, and the system pressure was equilibrated under the protocol. The Langevin temperature control method and Berendsen pressure control method were employed to balance the temperature and pressure for 100 ps, respectively. The MD simulation was performed for 80 ns. The cutoff distance between van der Waals energy and short-range electrostatic energy was set at 10 Å, and the long-range electrostatic energy was calculated employing the particle mesh Ewald (PME) method during the run time. Moreover, the RMSD value of the complex system were monitored in real time until the expected average structure of the protein complexes was obtained for subsequent binding mode analysis.
2.12 Population Coverage
HLA alleles are extremely polymorphic, and different HLA types are expressed at significantly different frequencies in different races. Since the selected epitope will induce an immune response only in individuals expressing HLA alleles that can bind to the specific epitope, we used the IEDB tool (http://tools.iedb.org/population/) to predict the population coverage of the designed vaccine in different areas with an algorithm based on HLA allele binding and HLA allele frequencies (83).
2.13 Immune Simulation
To clarify whether the designed vaccine can induce the protective immune response of the host, we employed the C-ImmSim server (https://kraken.iac.rm.cnr.it/CIMMSIM/index.php?page=0) (84) that utilizes position-specific scoring matrices (PSSMs) to describe the immune response between the host and antigen to conduct an in silico immune simulation. The detailed immunization regimen included three injections of 1,000 vaccine units each, with a 4-week interval between each injection. In this investigation, the whole immune simulation process ran for 1,050 time steps, and each time step represents 8 h, with the injection points set at time steps 1, 84, and 168 in this study. HLA-A*02:01, HLA-B*07:02, and HLA-DRB1*0101 were selected as host MHC molecular combinations, and other parameters remained at the default.
2.14 In Silico Molecular Cloning
It is well known that E. coli is the most commonly used prokaryotic expression system (85). E. coli (K12 strains) was selected as the host organism in this study. To achieve better expression of the vaccine in E. coli, the JCAT online server (http://jcat.de/) (86) performed reverse translation and codon optimization of the vaccine. Two parameters of the JCAT server, codon adaptive index (CAI) and the GC content, were used to evaluate the expression potential of vaccine in host. The CAI refers to the consistency between the use frequency of synonymous codons and the optimal codon. The CAI value ranges from 0 to 1, and the closer it is to 1, the better the consistency between the codon and the optimal codon. The GC content refers to the ratio of guanine (G) and cytosine (C) in the nucleotide sequence, and its value range should be between 30% and 70%; if not, the transcription and translation efficiencies will be greatly discounted. XhoI and BamHI restriction sites were added to the N- and C-terminal sites of optimized codon sequences, respectively. The pET28a(+) plasmid, one of the most popular expression plasmids on the market (described in >40,000 published articles) (87), is good for cloning and subcloning heterologous proteins with the E. coli as the host (21, 88). The pET28a(+) plasmid enables high titers of recombinant protein production—as much as 50% of the total expression product after a few hours of induction because of containing T7 promoter (89). Meanwhile, the pET-28a(+) can easily weaken the protein expression by reducing the concentration of inducers [Isopropyl â-D-1-thiogalactopyranoside (IPTG)]. Under non-inducible conditions, the target gene can be silenced completely without transcription (89). Additionally, pET-28a(+) not only provides different tags (N-terminal: His/Thrombin/T7 protein tag and C-terminal: His tag) but also has appropriate restriction sites, which were beneficial to the purification of the target protein and cloning of different genes (90). However, Shilling etal. (87) discovered two design flaws in pET vectors. One is the lack of a complete T7 consensus sequence (T7pCONS) of the T7lac promoter in the traditional pET28a(+) vector, and the other is that the compatibility of the TIR, recognized by the 30S ribosomal subunit during translation initiation, in the traditional pET28a(+) vector with the ribosome of E. coli, is not at its best (87); both defects would impact the production efficiency of the protein. Furthermore, leaky expression may occur in some hosts. There may still be some low-level expression of T7 RNA polymerase from the LacUV5 promoter in some expression host strains even if IPTG is not used (89), which may result in some genes, harmful to target bacteria, expressed in some host strains. Finally, the vaccination sequence was inserted into pET28a(+) expression vectors with the help of SnapGene in this study. The flowchart of the multi-epitope vaccine construction is shown in Figure 1.
3 Results
3.1 Prediction and Screening of Epitopes
CTLs, HTLs, and B cells are important participants in the human protective immune response. CTLs indirectly orchestrate human adaptive immune responses by secreting and aggregating a variety of cytokines, in addition to directly mediating the death of target cells (91). HTLs not only can activate T cells and B cells by recognizing presented foreign antigens and secreting certain cytokines but also contribute to B-cell differentiation into memory B cells (92). B cells are important for the generation of humoral immunity and long-lasting immunity (93). Hence, an ideal vaccine should include both T-cell epitopes (CTL and HTL epitopes) and B-cell epitopes. After layers of filtration, 5 CTL epitopes (Table 1), 5 HTL epitopes (Table 2), and 7 linear B-cell epitopes (Table 3) were finally selected for vaccine construction, and detailed screening materials are provided in the supplementary materials (Tables S1–S3). Among the 5 final selected HTL epitopes, which were all IFN-γ-inducing and IL-4-inducing epitopes, 3 of 5 HTL epitopes were predicted to be inducing pro-inflammatory cytokines. Similarly, 3 of 5 selected HTL epitopes were predicted as IL-2 inducing. From these, we can know that relatively few HTL epitopes among our selected ones are responsible for inducing multiple immune factors, but the combination of multiple epitopes would induce a strong immune response in the body.
3.2 The Multi-Epitope Vaccine Features
3.2.1 The Prediction of Physicochemical Properties of the Vaccine
The final vaccine construct (Figure 2A) is composed of four parts, CTL epitopes, HTL epitopes, linear B-cell epitopes, and LT-IIb (adjuvant), containing 389 amino acids, and its molecular weight was 41,632.89 Da. The vaccine pI is 9.11, and its negatively charged residues and positively charged residues are 43 and 53, respectively. The designed vaccine was considered stable and thermostable with an instability index II of 31.98 and an aliphatic index score of 59.43. Generally, a protein with an instability index below 40 was stable. Additionally, the higher aliphatic index of the protein results in better thermal stability (94). The GRAVY score was -0.585, and a negative sign indicated that it was hydrophilic (95). The estimated half-life of the designed vaccine is 30 h in mammalian reticulocytes, greater than 20 h in yeast, and greater than 10 h in E. coli. The physicochemical parameters of the final vaccine construct were compared to those of positive controls (Table 4). Compared with C1, C2, and C3, the aliphatic index of the final vaccine construct is relatively small, but it is better than C4 and some other multi-epitope vaccines (96–98). Overall, the final vaccine construct revealed appropriate physicochemical properties, including well solubility and stability, which contributed to enhancing the vaccine’s bioavailability and immunogenicity and lessening side effects.
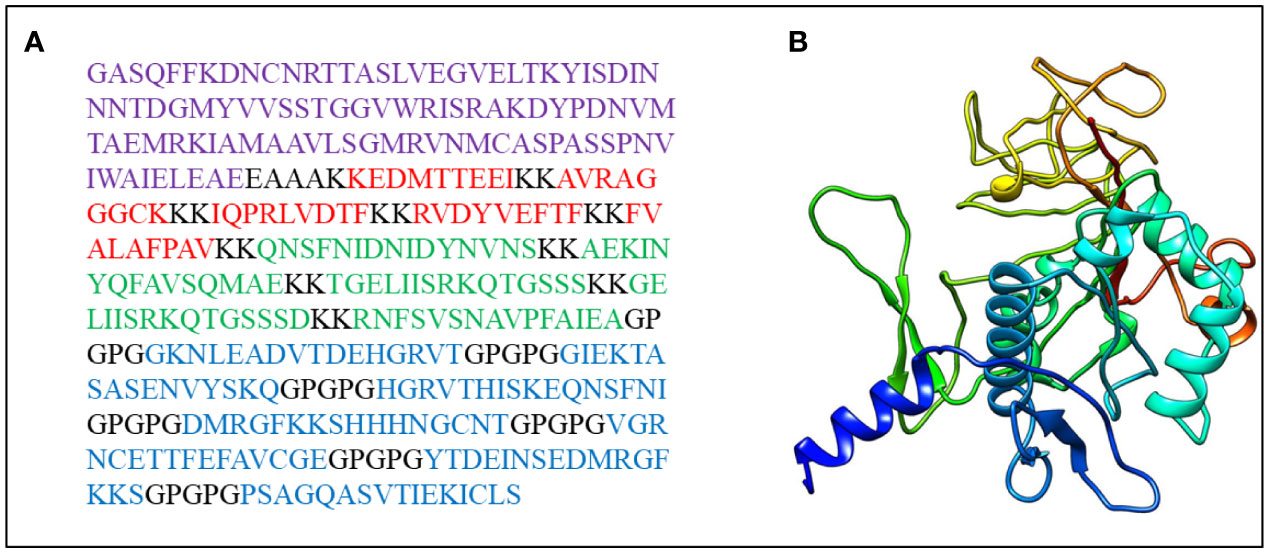
Figure 2 (A) The final vaccine sequence; purple denotes adjuvant, Cytotoxicity T Lymphocytes (CTL) epitopes are colored red, Helper T lymphocyte (HTL) epitopes are colored green, B-cell epitopes are colored blue, and all of them are coupled via different connectors. (B) The three dimensional (3D) structure of the vaccine.
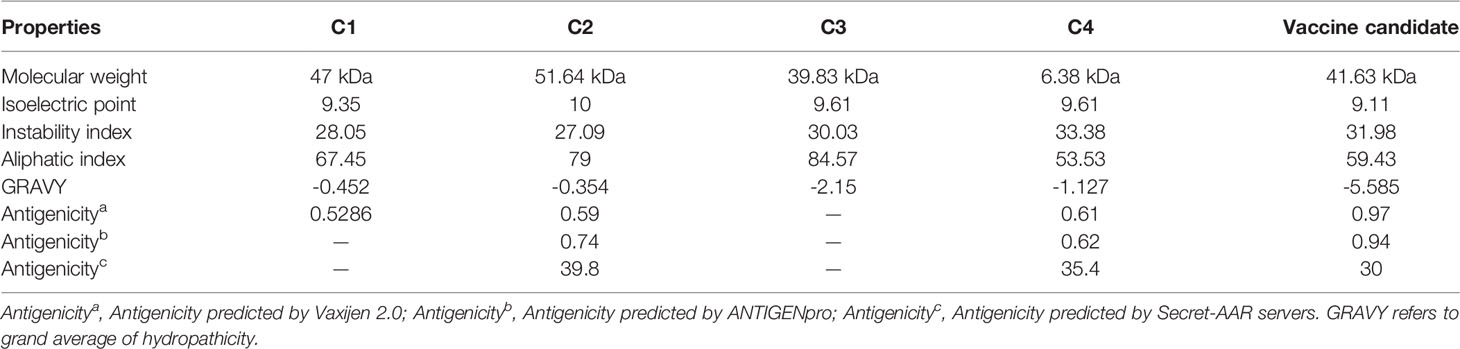
Table 4 Comparison of several physicochemical properties of the positive vaccine controls and C. difficile candidate vaccine.
3.2.2 The Prediction of Antigenicity, Allergenicity, and Toxicity of the Vaccine
The antigenicity scores of the designed vaccine were 0.97, 0.94, and 30 as predicted by the Vaxijen 2.0, ANTIGENpro, and Secret-AAR servers, respectively, showing that the vaccine has a strong affinity to the host immune system products (Table 4). And the vaccine was non-allergenic and nontoxic.
3.2.3 The Prediction of Transmembrane Domains, Solubility, and Signal Peptide of the Vaccine
The designed vaccine detected no transmembrane helix (Figure S1), The SQLpro server predicted that the probability of solubility of the multi-epitope vaccine upon overexpression in E. coli was 0.61 and the prediction results of the TMHMM-2.0 server showed that the vaccine was an extracellular protein, which indicated that the multi-epitope vaccine protein could be soluble expressed and secreted efficiently in E.coli. The predicted results of server signalP-6.0 indicated that the vaccine construct did not have a signal peptide (Figure S2).
3.2.4 The Prediction of Immunoglobulin A-Specific B-Cell Epitopes of the Vaccine
When the screening threshold was fixed to 0.5, the IgPred server predicted that the vaccine contained 22 IgA-specific B-cell epitopes with a length of 20 amino acid residues, indicating that the vaccination was capable of inducing the secretion of IgA. The detailed results were shown in Table S4.
3.3 Secondary Structure Prediction
The secondary structure of the vaccine sequence was predicted by the Prabi server, containing 25.19% alpha-helices, 55.27% random coils, and 19.54% extended strands (Figure S3).
3.4 Three-Dimensional Modeling and Validation of the Vaccine
RaptorX was used to predict the primary 3D (Figure 2B) structures of the vaccine that were further refined via the GalaxyWeb server. Based on the criteria that the higher GDT-HA, the higher RMSD, and the lower MolProbity resulted in the better model, the best model was selected for further validation. The Ramachandran plot of the greatest model showed that 83% of residues situated in the most favored region, and only 0.9% lie in the disallowed region (Figure 3A). The Z score was -5.01 (Figure 3B), and the ERRAT score was 92.42 (Figure 3C). The Ramachandran plot, Z score, and ERRAT score of the greatest model of CdeC and FliD were shown in Figures S4, S5. The surface locations of the final antigen epitopes included in the vaccine construct were visualized on the 3D structures of the CdeC and FliD proteins (Figure 4).
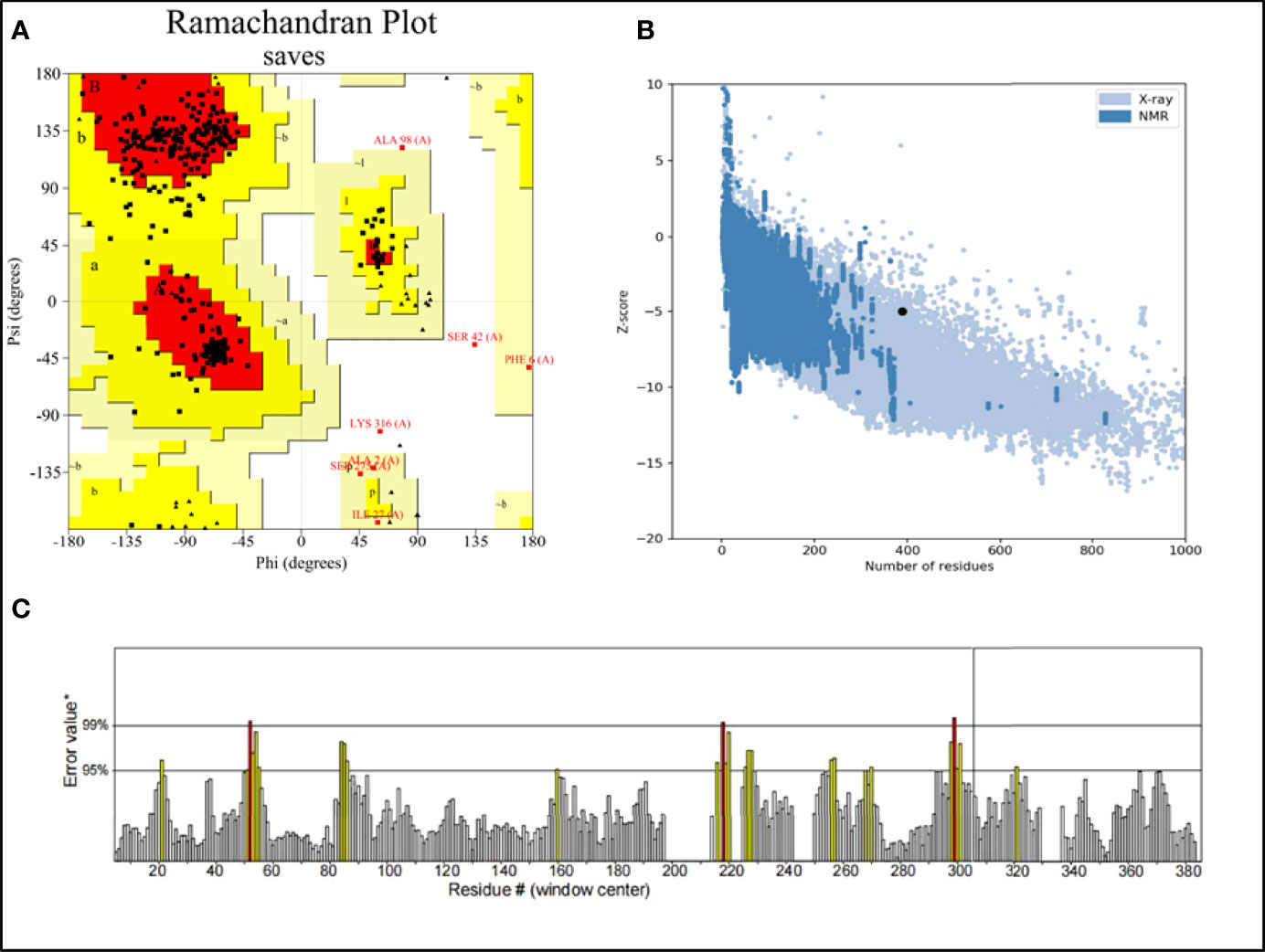
Figure 3 (A) Ramachandran plot of the vaccine. Red denotes the most favored region, dark yellow denotes the additional allowed region, light yellow denotes the generally allowed region, and white denotes the disallowed region; (B) three dimensional (3D) structure of the vaccine was validated by ProSA with a Z score of -5.01; (C) 3D structure of the vaccine was validated by ERRAT with an ERRAT score of 92.42%.
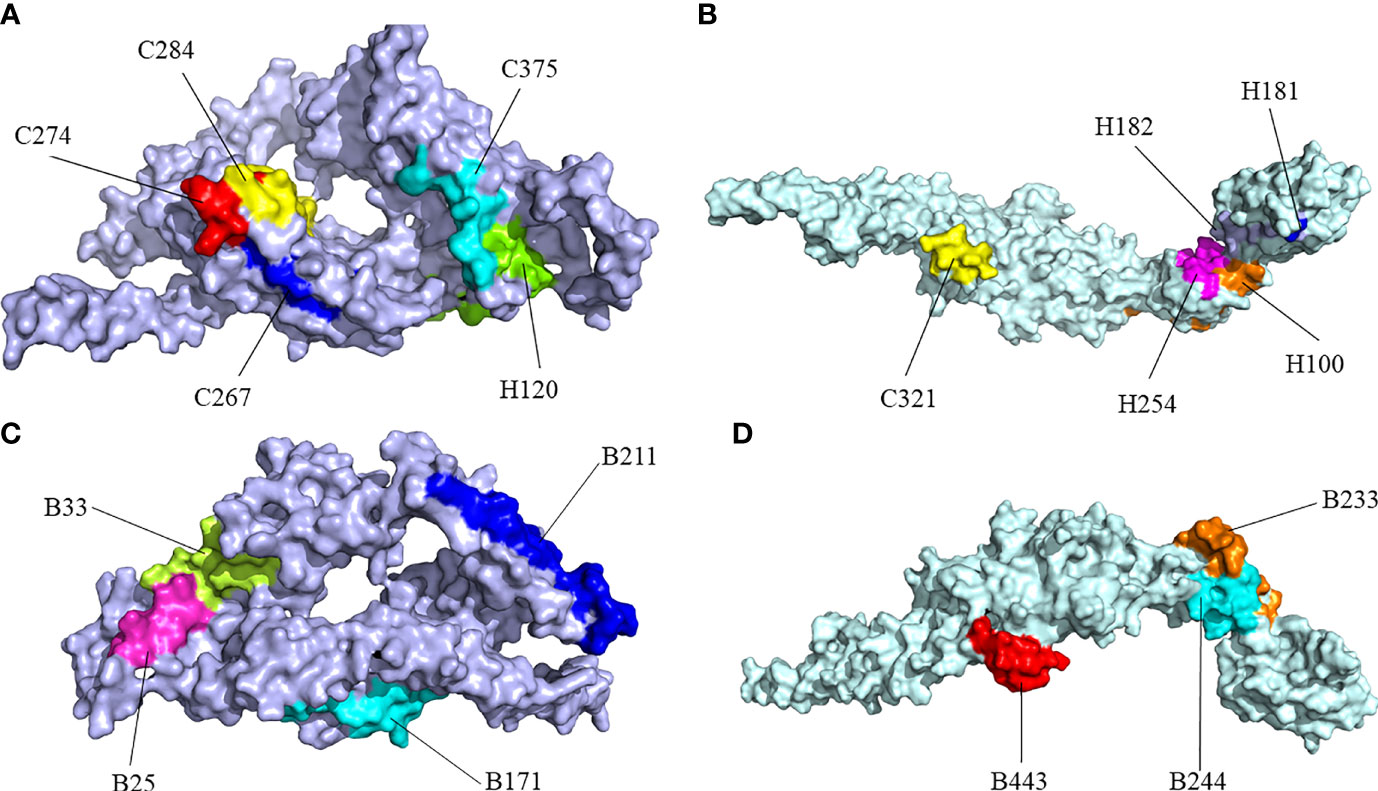
Figure 4 Surface positions of final selected epitopes on the three-dimensional (3D) models of the protein. (A) The Cytotoxicity T Lymphocytes (CTL) and Helper T lymphocyte (HTL) epitopes of CdeC. (B) The CTL and HTL epitopes of FliD. (C) The B-cell epitopes of CdeC. (D) The B-cell epitopes of FliD. Every CTL epitope is represented by a combination of C and its starting position. Every HTL epitope is represented by a combination of H and its starting position. Every B-cell epitope is represented by a combination of B and its starting position.
3.5 Molecular Docking
As shown in Table S5 and Figure S6, HADDOCK V2.4 docking data demonstrated that the vaccination had a good affinity for TLRs and MHC molecules. Also, the docking data from the clusPro server showed that there was also a good affinity between the vaccine and BCR. The surface mode of the vaccine–BCR complex docked by the clusPro server with the lowest binding energy score was shown in Figure S7. A total of 29 model complexes of vaccine and BCR were discovered, and the lowest binding energy score was -347.3, and the center energy was -249.6. Additionally, to reconfirm the binding mode of the vaccine with TLRs, MHC molecules, and BCR, the Rosetta was also employed. The estimated binding free energy of the best docking vaccine–TLR2 complex system was -92.0331 kcal/mol, which was divided into the hydrogen bond energy (-34.2227 kcal/mol), van der Waals energy (-21.2804 kcal/mol), and electrostatic energy (-36.5300 kcal/mol) (Table 5). We analyzed the binding mode of the vaccine–TLR2 complex (Figure 5A). The vaccine–TLR2 binding interface was shown in Figure 5B. Seventeen (K561, T532, K488, N468, H449, E369, E344, K126, K125, K121, E95, S83, N79, D166, E356, N358, S359) hydrophilic amino acids of the TLR2 formed intensive hydrogen-bond networks with 15 (S42, T43, N9, S3, K53, R32, N33, D31, K37, S39, D58, N61, R63, N89, K137) amino acids of the vaccine protein (Figure 5B), and the black dotted lines at the bonding interface referred to hydrogen bonds (Figure 5B). Among the polar interactions between the two proteins, there were several strong salt bridge interactions, including R63-D166, K137-E356, E369-K53, and E344-K53 (Figure 5B). The strength of the salt bridge is significantly stronger than that of the general hydrogen bonding, and it made a significant contribution to the stability of the protein complexes. The estimated binding free energy of the optimal vaccine–HLA-A* 02:01 complex system was -38.6189 kcal/mol, which was split into H-bond energy (-7.9353 kcal/mol), van der Waals energy (-12.3127 kcal/mol), and electrostatic energy (-18.3709 kcal/mol) (Table 5). The binding mode of the vaccine–HLA-A* 02:01 complex was shown in Figure 6A. There were 13 hydrogen bonds (K124-A149, N32-Y84, G122-E154, K124-R157, R48-T73, V46-T73, T13-R44, N9-R44, T43-E63, T43-K66, S42-K66, G44-R97, G44-H70) and 3 salt briges (R48-D77, E63-R65, E19-R170) in the binding interface of the vaccine–HLA-A*02:01 complex (Figure 6B). Fourteen residues have participated in the formation of hydrophobic interactions between the two proteins as shown in Figure 6B. Additionally, molecular docking modes between the vaccine and TLR4, TLR5, and HLA-DRB1*0401 were also performed in this study, and the binding interface diagrams of the complexes among them are shown in the supplementary material (Figure S8). The estimated binding free energy of the optimal vaccine–BCR complex system was -49.0037 kcal/mol, which was subdivided into H-bond energy (-12.9112 kcal/mol), van der Waals energy (-13.4489 kcal/mol), and electrostatic energy (-22.6436 kcal/mol) (Table 5). The binding mode of the vaccine–BCR complex was shown in Figure 7A. Thirteen hydrogen bonds were formed between the binding interface between the vaccine and BCR, which were the main contributing force to the binding between the two proteins (Figure 7B). The polar amino acids involved in the formation of hydrogen bonds include N32, D29, T34, R48, S50, K53, and Y55 in the vaccine and T104, T102, D101, S30, H53, T73, S28, K75, and Q77 in BCR (Figure 7B). In addition, there was good hydrophobic contact between the vaccine and BCR, such as a good hydrophobic contact between F6 and Y25 and a good hydrophobic interaction between W47 and surrounding amino acids such as Y32 and Y111 (Figure 7B).
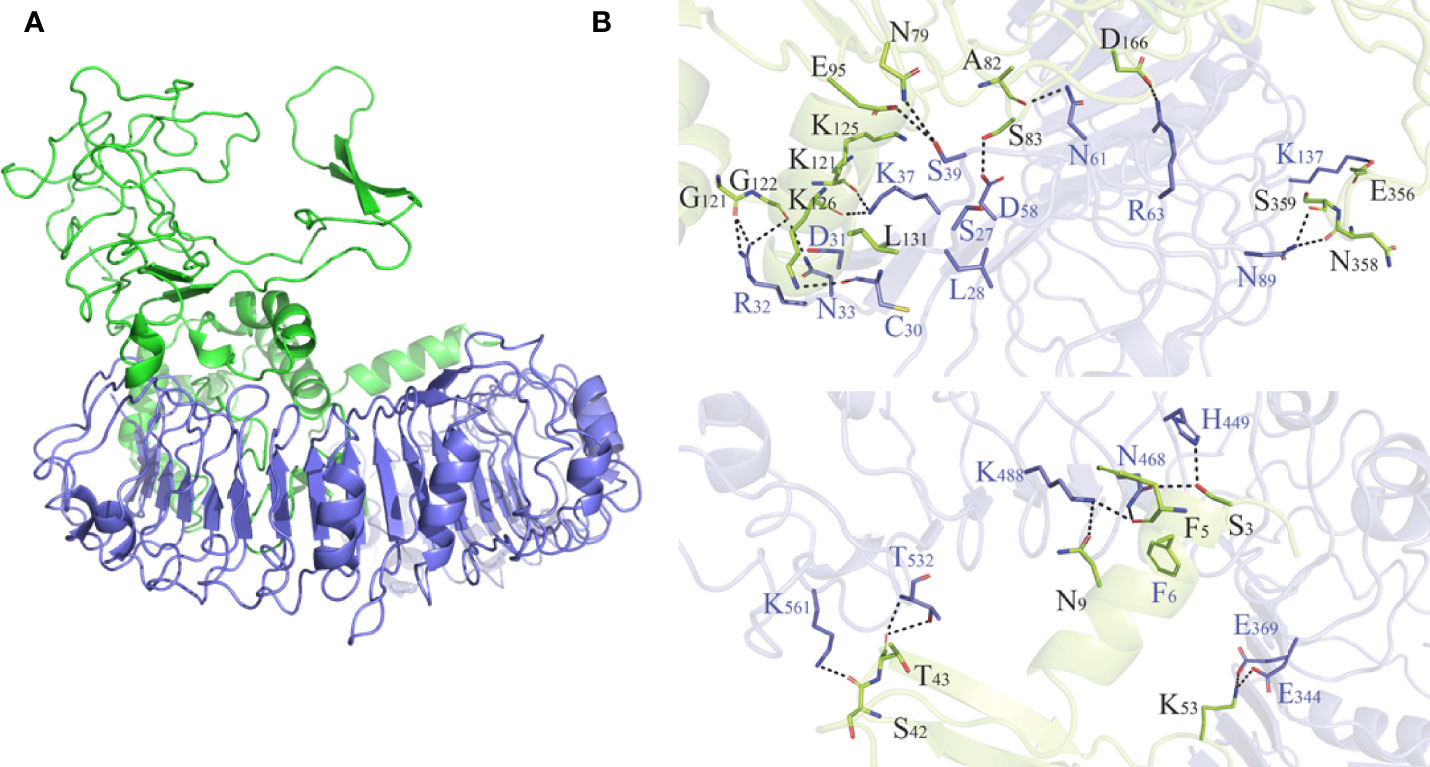
Figure 5 (A) Diagram of the lowest energy docking mode of the vaccine-Toll like receptor (TLR) 2 complex. (B) Pattern diagram of the binding interface of the vaccine–TLR2 complex, the TLR2 is shown in blue, the vaccine is shown in yellow-green. The binding interface amino acid of TLR2 is shown in blue, the binding interface amino acids of vaccine are shown in black. The sticks refer to binding interface amino acids, and the black dotted lines refer to hydrogen bonds.
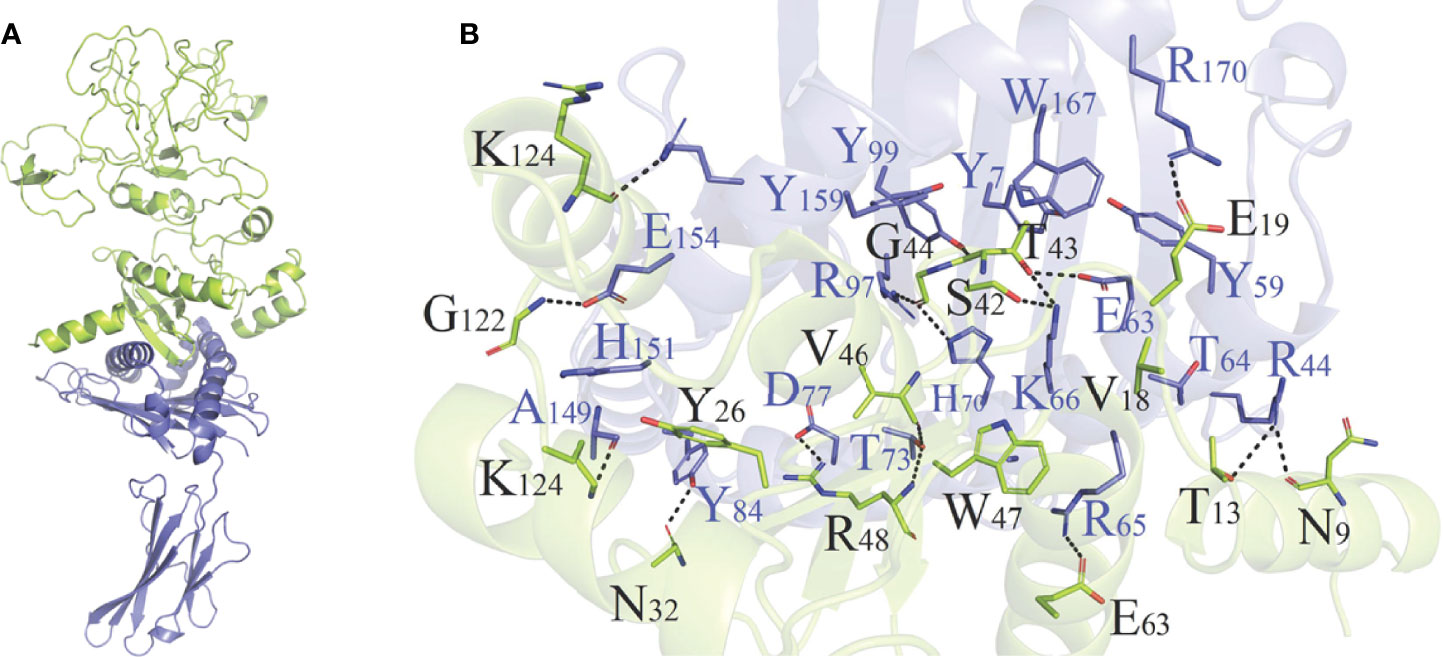
Figure 6 (A) Diagram of the lowest energy docking mode of the vaccine–HLA-A*02:01 complex. (B) Pattern diagram of the binding interface of the vaccine–HLA-A*02:01 complex, the HLA-A*02:01 is shown in blue, the vaccine is shown in yellow-green. The binding interface amino acid of HLA-A*02:01 is shown in blue, the binding interface amino acids of vaccine are shown in black. The sticks refer to binding interface amino acids, and the black dotted lines refer to hydrogen bonds.
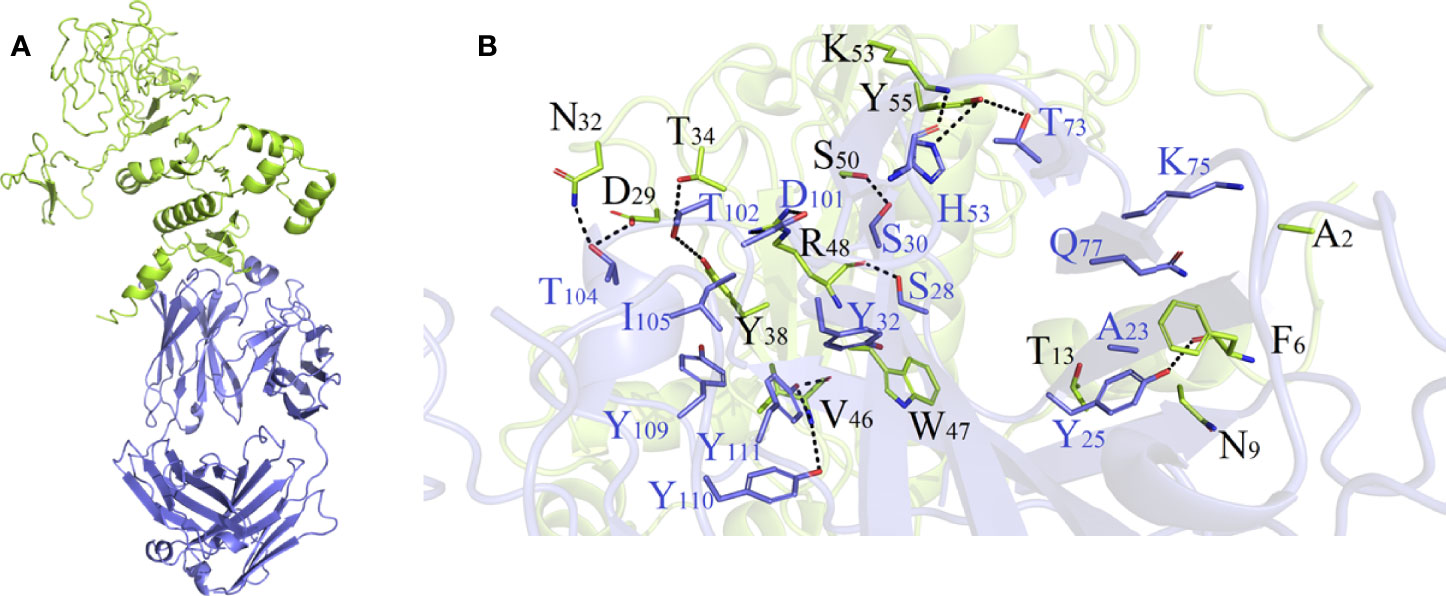
Figure 7 (A) Diagram of the lowest energy docking mode of the vaccine-B cell receptor (BCR) complex. (B) Pattern diagram of the binding interface of the vaccine–BCR complex, the BCR is shown in blue, the vaccine is shown in yellow-green. The binding interface amino acid of BCR is shown in blue, the binding interface amino acids of vaccine are shown in black. The sticks refer to binding interface amino acids, and the black dotted lines refer to hydrogen bonds.
3.6 Molecular Dynamics Simulation
The binding free energy of vaccine–TLR2 (Table 6) and vaccine–HLA-*A0201 (Table 6) was calculated by the MMPBSA module of AMBER. In comparison to the vaccine–HLA-A*0201 complex, the vaccine–TLR2 complex was more stable, which might be explained by the fact that the larger the protein system, the more binding surfaces there are, resulting in better binding ability.
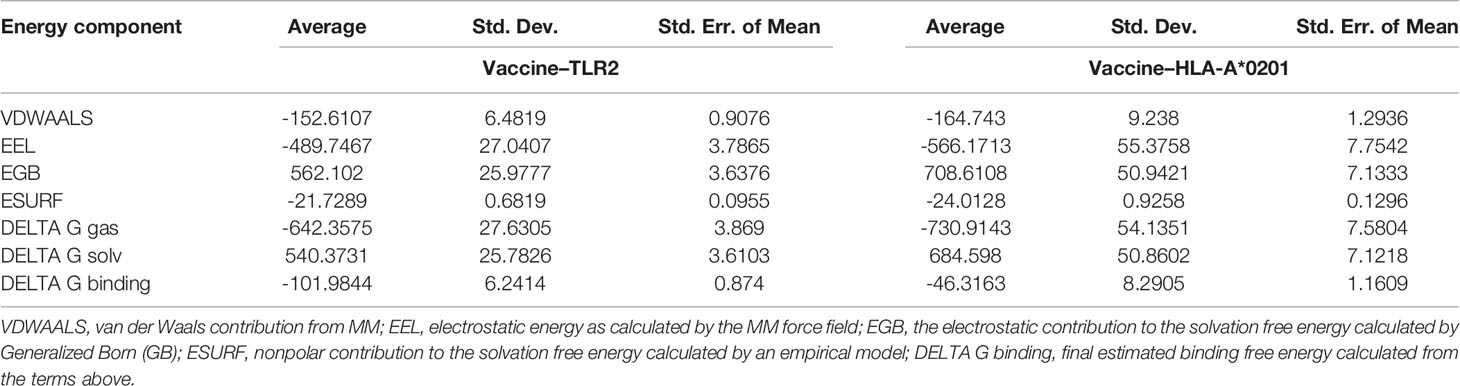
Table 6 Energy analysis of the vaccine–TLR2 and vaccine–HLA-A*02:01 complexes in the molecular dynamics simulation.
The RMSD value represents the dispersion of the average value of protein centroid coordinates during the protein centroid movement, reflecting the change in the overall flexible structure of the complex system. The RMSD values (Figure 8A) of the vaccine–HLA-A*02:01 protein complex were relatively stable and remained almost at 5 Å within the range of 10–80 ns, indicating that the overall complex system reached a relative equilibrium state. For the vaccine–TLR2 protein complex system, the initial up and down fluctuations were relatively violent. The RMSD value (Figure 8A) varied most sharply within the 20–30-ns range, indicating that the complex system was extremely unstable in this interval. However, in the range of 40–80 ns, the system was stable, indicating that the protein vaccine–TLR2 structure reached a relatively balanced and stable state. The RG describes the distribution characteristics of atoms along a specific axis, elucidating the compactness of molecules. To some extent, it was capable of characterizing the overall changes in protein structure using the initial structure as a reference. In this project, the RG cyclotron radius values of vaccine–TLR2 (Figure 8B) and vaccine–HLA-A*02:01 protein complex systems (Figure 8B) were kept at a constant level, almost unchanged, indicating that the protein complexes after binding were relatively stable, which showed a similar trend to RMSD. The stability of the two complex systems was also validated by their hydrogen bonds (Figure 8C). The RMSF value characterizes the flexibility of amino acid residues in the protein complex. The maximum and minimum RMSF values (Figure 8D) of the HLA-A*02:01 molecule in the vaccine–HLA-A*02:01 complex were 3.1298 and 0.8721, respectively. RMSF showed a sustained high level in the region after 168 and was much higher in regions 192–199 and 219–228, indicating that the flexibility of the amino acid residues in these two regions was higher. The maximum and minimum RMSF values of the vaccine in the vaccine–HLA-A*02:01 complex (Figure 8D) were 5.4769 and 0.9279, respectively. The RMSF value of the TLR2 molecule in the vaccine–TLR2 complex was higher and fluctuated less, and its maximum and minimum RMSF values were 3.106 and 0.9486, respectively. The maximum and minimum RMSF values of the vaccine in the vaccine–TLR2 complex were 4.858 and 1.0717, respectively. The RMSF values of the vaccine showed a large fluctuation in the 201–209 region, with the maximum value reaching 4.5632.
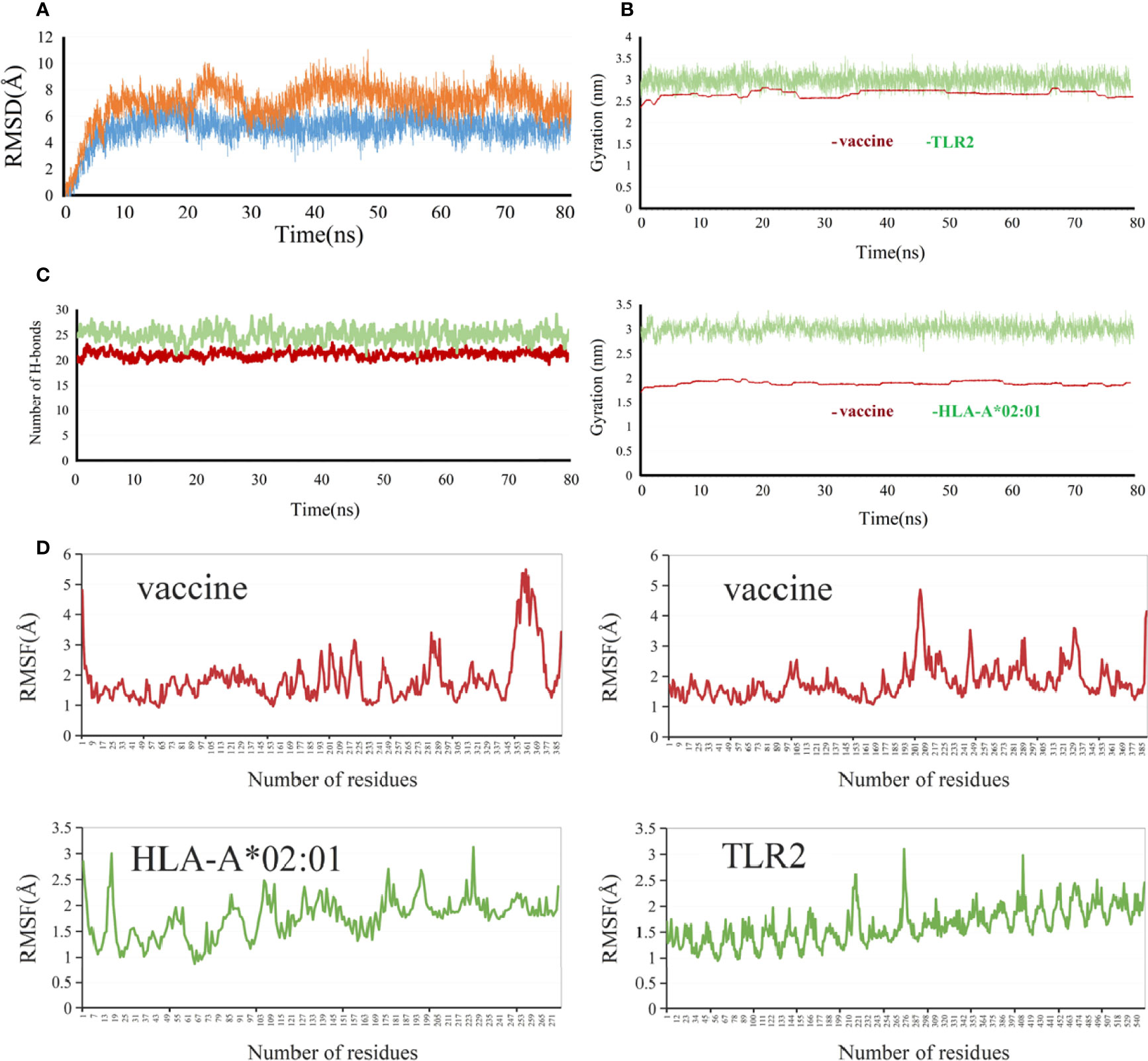
Figure 8 Molecular dynamics simulation analysis of the vaccine with TLR2 and HLA-A*0201. (A) RMSD (Root-mean-square deviation), vaccine-TLR2 (orange), vaccine-HLA-A*0201(blue) (B) RG (radius of gyration), (C) Hydrogen bonds, vaccine-TLR2 (green), vaccine-HLA-A*0201 (brown ) (D) RMSF (root medium square fluctuation).
The structures of the protein complexes of molecular docking and MD were superimposed together, and then the analysis of the changes in protein structure pre and post-MD simulation was performed (Figure S9). As observed, the MD simulation had a significant structural optimization effect on the protein structure complex, particularly at the protein–protein interaction interface, where the structure fluctuated significantly. The MD simulation eliminated the unfavorable factors, such as steric hindrance and binding phase difference, in the docking structure, which made the protein–protein interaction more reasonable. The post-dynamic structures were used for the following binding mode analysis, as detailed in the supplementary materials (Figures S10, S11). All structures in this article were visualized by Discovery Studio Visualizer 2021, UCSF Chimera1.15, and Pymol2.4.
3.7 Population Coverage
In this study, the multi-epitope vaccine covered 95.54% of the world’s population. In addition, in Europe and the United States, where C. difficile was prevalent, the population coverage also reached 96.84% and 96.86%, respectively. In China, its population coverage was relatively low, but it also reached 89.01% (Figure S12).
3.8 Immune Simulation
The immunogenicity of the designed vaccine was assessed through the C-ImmSim online server. Notably, the antibody titers increased significantly following the injection of the vaccine, and the increase was significantly higher after the second and third doses of injection than after the first (Figure 9A). After the first dose injection of the candidate vaccine, an increase in IgM antibody and the B-cell isotype IgM population and a slight elevation of B-cell isotype IgG1 population (Figure 9B) were detected. Following the second injection, there was a relatively large increase in B-cell isotype IgG1 and a slight increase in B-cell isotype IgG2 populations (Figure 9B). IgG1 + IgG2 antibodies had a higher titer than IgM antibodies in the tertiary response (Figure 9A). This demonstrated the Ig heavy-chain class switching, which was important for effective vaccination. Also, with the increasing immunoglobulin concentrations (i.e., IgM, IgG1, and IgG2), the concentration of antigen decreased (Figure 9A). Furthermore, doses of cytokines, such as IFN-γ, IL-2, and IL-12, were also seen, which showed that the proposed vaccine could cause an effective immune response (Figure 9C). The generation and amplification of TC (cytotoxic) cells were observed (Figure 9D). The increases in the TH (helper) cell population and corresponding memory cells were detected (Figure 9E), and TH1 was the dominant subtype (Figure 9F). During exposure, the macrophages (MA), natural killer (NK) cells, and dendritic cells (DCs) were also activated (Figure S13). Overall, these simulations demonstrated that the multi-epitope vaccine was capable of activating both cellular and humoral immunity and eliciting immune memory to induce a strong immune response to the reinvasion of the antigen.
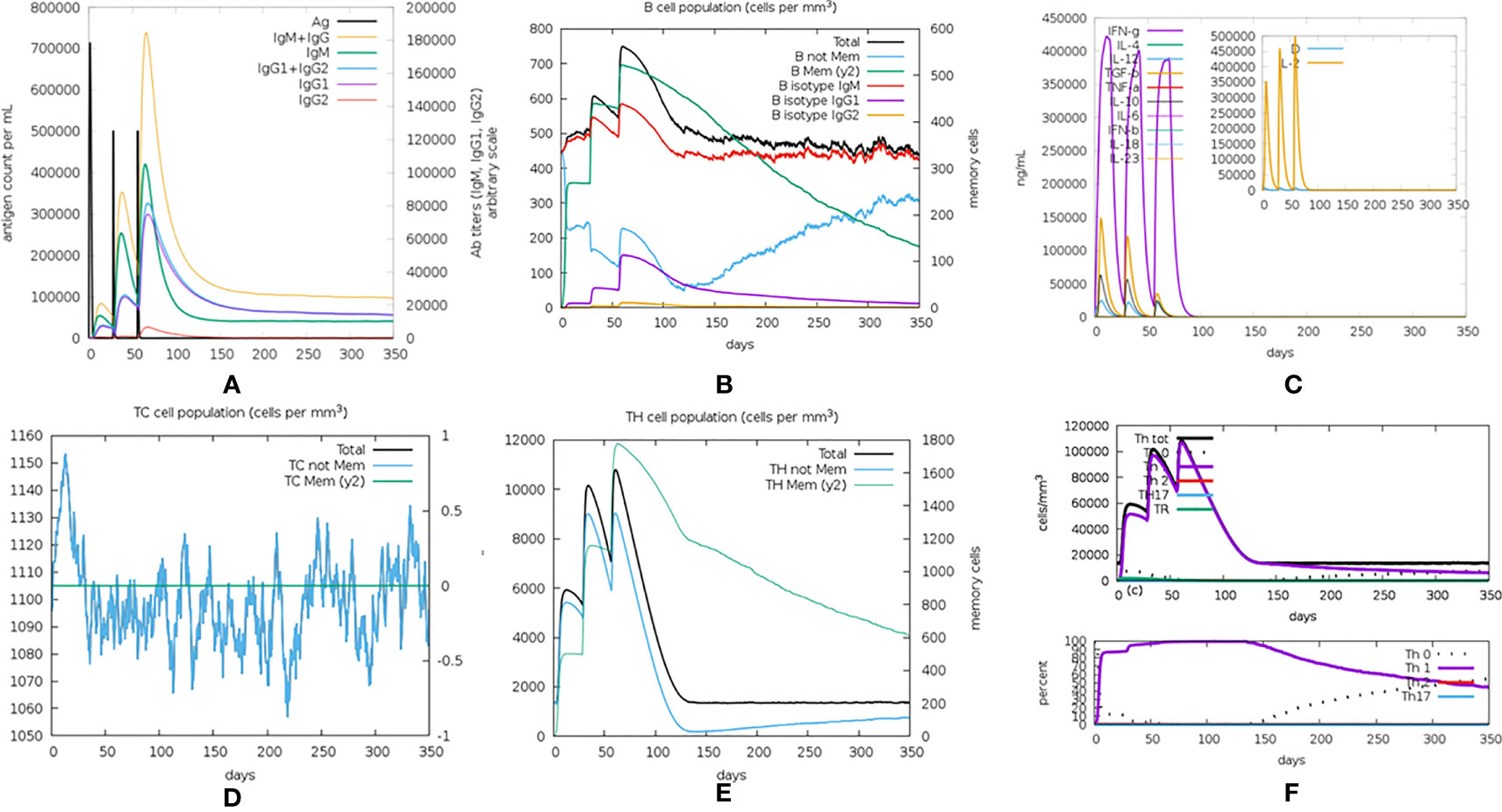
Figure 9 The in silico immune simulation results. (A) Immunoglobulin levels with respect to antigen concentration. (B) Cytokine level in response to the vaccine. (C) B-cell population. (D) TC (cytotoxic) cell population. (E) TH (helper) cell population. (F) The population of different subtypes of TH cells.
3.9 In Silico Molecular Cloning
The vaccine protein was reversely translated and optimized by JCAT to acquire the codons that were highly expressed in E. coli. The length of the optimized codon sequences was 1,167 nucleotides with a CAI value of 1, and the GC content was 50.73%, indicating that the vaccine can be well expressed in E. coli and provides the possibility for the production of the vaccine (Figure S14). Finally, the codon sequences were successfully inserted into the pET28a(+) vector (Figure 10).
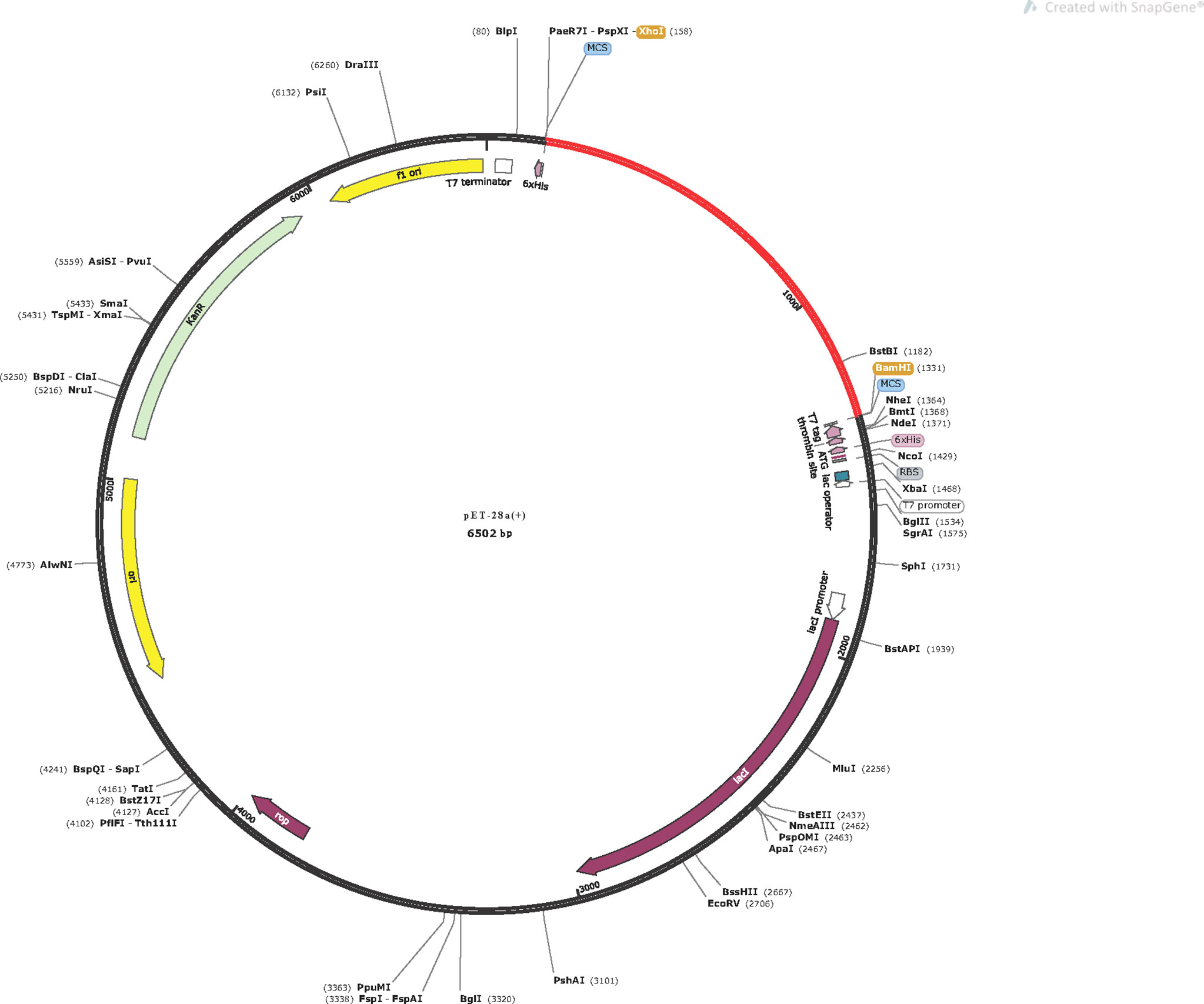
Figure 10 In silico cloning of the vaccine in the pET28a(+). Red area represents the optimized nucleotide sequence of the vaccine, the black areas represent the expression vector, pET28a(+), the XhoI and BamHI represent the cleavage sites of the restriction enzyme.
4 Discussion
CDI not only is associated with substantial morbidity and mortality worldwide but also significantly prolongs the length of hospital stay; as a result, the average cost of hospitalization greatly increases (99). Moreover, the resistance rate of C. difficile to its first-line treatment antibiotics is increasing, and the recurrence rate after antibiotic treatment remains at a high level (100, 101). To effectively prevent CDI, researchers have accelerated the pace of vaccine development.
Because TcdA and TcdB are the main factors associated with symptoms of CDI (102), the bulk of the earlier vaccine studies on C. difficile are mainly based on TcdA and TcdB. However, the failure of Sanofiofi’s vaccine during the phase 3 trial, which was once considered a potential candidate, makes it necessary to take a long, hard look at whether toxin-based vaccines work. To find another way against C. difficile, several surface proteins were evaluated as vaccine candidates. The SLPs have been evaluated as a vaccine component in combination with a variety of adjuvants. Different combinations induced different titers of antibodies, but no of them provided comprehensive protection for the infected model (103). Studies have shown that antibodies against several surface proteins, such as adhesin Cwp66, the protease Cwp84, the fliC and fliD, and the Fbp protein, can be observed in infected patients, which indicates that these proteins are potential vaccine candidates (35). Additionally, the protective efficacy of C. difficile spore proteins was also investigated (29). However, the general of these vaccination candidates failed to generate a satisfactory immunity (15, 17, 18, 29, 35). The protective efficiency of candidate vaccines designed with a single protein is limited. Therefore, can vaccines designed with multiple proteins induce a stronger protective effect?
In recent years, multi-epitope vaccines designed by an immunoinformatic method have gained increasing attention from researchers, and several multi-epitope vaccines dedicated to other pathogens have been evaluated in animal trials, and good immune effects have been achieved (22, 23). The selection of target proteins is the first and crucial step in designing a multi-epitope vaccine. It is well known that spores are the key morphological type of C. difficile transmission and recurrence (25). Following spore germination, adhesion and colonization in the intestinal mucosa are the initial steps for the pathogen’s settlement in the gastrointestinal tract. CdeC, a spore protein, was shown to be located in the exosporium of C. difficile and contributed to spore coat assembly, spore germination, and spore resistance (27, 28). According to bioinformatics analysis about the recorded genome of C. difficile, CdeC was highly homogeneous across C. difficile strains, with the lowest identity at the amino acid level exceeding 90% (104). FliD is a flagellum cap protein that has been less frequently employed in vaccine research than fliC but is more conservative (33), and it has been demonstrated to be efficient at eliciting protective immunity (35). Hence, we designed a multi-epitope vaccine combining CdeC and fliD by a bioinformatics method in this study.
In comparison to most previously studied vaccinations that only provide protective immunity to the vegetative form of C. difficile, this multi-epitope vaccine also produced an additional immune response against C. difficile spores. Moreover, to confirm that a strong immune response would be induced in the host, the designed vaccine included not only T-cell epitopes (CTL epitopes and HTL epitopes) but also B-cell epitopes. Cytokines play an important role in the host immune response (48). IFN-γ and IL-4 are two important cytokines for the activation of host immune cells; the former contributes to the differentiation of TH1 cells, while the latter plays a vital role during TH2 cell differentiation and participates in the activation of macrophage cells (45, 105). IL-2 also was a promoter of immune cell growth (50). Therefore, all selected HTL epitopes were checked for the ability to induce the generation of IFN-γ, IL-2, IL-4, and other pro-inflammatory cytokines (such as IL-1α, IL-1β, TNF-α, IL-12, IL-18, and IL-23). Based on the comprehensive consideration of the basic quantity standard and ability to induce cytokine production of the final selected HTL epitopes, only 5 HTL epitopes were included in this study. All of these 5 epitopes are IL-4-inducing and IFN-γ-inducing epitopes, and 3 of them were predicted to be inducing pro-inflammatory cytokines and IL-2. The immunity of the multi-epitope vaccine is relatively weak; the choice of adjuvant that can improve the vaccine’s immunogenicity is very important for the construction of the vaccine. HLT is a widely recognized vaccine adjuvant (106), and one of them, LT-IIb, was chosen as a vaccine adjuvant to enhance the vaccine’s immunogenicity in this study (107). The selection of linkers also is an important part for the vaccine construction, and the improper selection or inappropriate location will lead to changes in the structure of the vaccine. KK linkers were used to connect CTL epitopes and HTL epitopes, while GPGPG linkers were used to connect B-cell epitopes (108). EAAAK linker was used to connect the vaccine and adjuvant to achieve an effective connection and a fixed space between them (109, 110). We also have predicted the physicochemical properties of the designed vaccine, and its results showed that the vaccine was stable, highly soluble, and thermostable. To assess the potential of the vaccine to induce the production of IgA, we predicted the vaccine’s IgA-specific B-cell epitopes. We also performed molecular docking between the vaccine and BCR to check the interaction affinity between them, and the results showed that the vaccine could be recognized and bound by BCR. Given that antigen-activated B cells can further differentiate into sIgA-secreting plasma cells with the help of transforming growth factor-β (TGF-β) (111). Therefore, one could hypothesize that the vaccine may induce an IgA mucosal immune response. The results of molecular docking of the vaccine with TLRs and MHC molecules suggested that the designed vaccine could successfully enter the body, initiate nonspecific immunity, and subsequently initiate specific immunity. Moreover, to determine whether the vaccine construct could stably bind MHC molecules and TLRs in the free state, MD simulations were conducted. The RMSD analysis revealed that the vaccine–HLA-A*0201 complex stabilized within 10 ns, but the vaccine–TLR2 complex took longer to stabilize. The RG of the two complexes showed little fluctuation during simulation, indicating that the two complexes were stabilized during simulation, and the RMSF results demonstrated that the two complexes were both flexible. Another important part was that the immune simulation was performed in this study, and the results suggested that the designed vaccine could effectively induce a human protective immune response in theory. Additionally, population coverage suggested that the designed vaccines could reach the vast majority of the world’s population.
5 Conclusion
The high incidence and recurrence rate of CDI have brought great negative effects on the human economy and society. However, due to the limitations of current treatment methods and the lack of effective prevention measures, it is particularly important to develop a vaccine preventing CDI as soon as possible. In this study, a multi-epitope vaccine targeting pathogenic adhesion, sporogenesis, and spore adhesion was designed using a time-saving and low-cost approach. The antigenicity, toxicity, allergenicity, and other physicochemical properties of the vaccine were checked. The results of molecular docking and MD simulation showed that the vaccine could stably bind to TLRs and MHC molecules. Furthermore, the immune simulation suggested that the vaccine could induce a strong immune response. Finally, we evaluated the expression potential of the vaccine in E. coli. On all these counts, the vaccine has good efficacy against C. difficile in theory.
6 Limitations
Firstly, compared with traditional vaccines, the immunogenicity of multi-epitope vaccines is relatively weak even with the addition of adjuvants. The application of additional adjuvants such as aluminum hydroxide may resolve this problem. Secondly, CDI is an intestinal infection, but the ability of the developed vaccine to stimulate intestinal immunity remains to be further verified. Additionally, the vaccine designed in this study mainly targets the epidemic Clostridium difficile R20291, and the conserved epitopes of additional antigenic proteins will be considered in the future to improve the protective effect of the vaccine within the limited protein length. Finally, its antigenicity and immunogenicity need to be further verified in in vivo and in vitro tests.
Data Availability Statement
All datasets presented in this study are included in the article and Supplementary Material.
Author Contributions
CT, CL, and AW designed the research and analyzed the data. CT charged the drawing. CT, FZ, YX, YW, XM, SL, TL, SC, and JZ wrote the paper. CL and AW reviewed and edited the paper. CL and AW acquired the funding. All authors have read and agreed to the published version of the article.
Funding
This research was funded by the Natural Science Foundation of Hunan Province (Award No. 2021JJ31071).
Conflict of Interest
The authors declare that the research was conducted in the absence of any commercial or financial relationships that could be construed as a potential conflict of interest.
Publisher’s Note
All claims expressed in this article are solely those of the authors and do not necessarily represent those of their affiliated organizations, or those of the publisher, the editors and the reviewers. Any product that may be evaluated in this article, or claim that may be made by its manufacturer, is not guaranteed or endorsed by the publisher.
Acknowledgments
We extend the sincerest appreciation to University of California, San Francisco and Lanzhou University, for their technical assistance.
Supplementary Material
The Supplementary Material for this article can be found online at: https://www.frontiersin.org/articles/10.3389/fimmu.2022.887061/full#supplementary-material
References
1. Lawson PA, Citron DM, Tyrrell KL, Finegold SM. Reclassification of Clostridium Difficile as Clostridioides Difficile (Hall and O’Toole 1935) Prévot 1938. Anaerobe (2016) 40:95–9. doi: 10.1016/j.anaerobe.2016.06.008
2. Bartlett JG, Chang TW, Gurwith M, Gorbach SL, Onderdonk AB. Antibiotic-Associated Pseudomembranous Colitis Due to Toxin-Producing Clostridia. N Engl J Med (1978) 298(10):531–4. doi: 10.1056/nejm197803092981003
3. CDC. About Cdc’s Urgent Threats (2019). Available at: https://www.cdc.gov/drugresistance/biggest-threats.html#cdiff.
4. Czepiel J, Krutova M, Mizrahi A, Khanafer N, Enoch DA, Patyi M, et al. Mortality Following Clostridioides Difficile Infection in Europe: A Retrospective Multicenter Case-Control Study. Antibiotics (Basel) (2021) 10(3). doi: 10.3390/antibiotics10030299
5. Borren NZ, Ghadermarzi S, Hutfless S, Ananthakrishnan AN. The Emergence of Clostridium Difficile Infection in Asia: A Systematic Review and Meta-Analysis of Incidence and Impact. PloS One (2017) 12(5):e0176797. doi: 10.1371/journal.pone.0176797
6. Eyre DW, Tracey L, Elliott B, Slimings C, Huntington PG, Stuart RL, et al. Emergence and Spread of Predominantly Community-Onset Clostridium Difficile PCR Ribotype 244 Infection in Australia 2010 to 2012. Euro Surveill (2015) 20(10):21059. doi: 10.2807/1560-7917.es2015.20.10.21059
7. Song JH, Kim YS. Recurrent Clostridium Difficile Infection: Risk Factors, Treatment, and Prevention. Gut Liver (2019) 13(1):16–24. doi: 10.5009/gnl18071
8. Leuzzi R, Adamo R, Scarselli M. Vaccines Against Clostridium Difficile. Hum Vaccin Immunother (2014) 10(6):1466–77. doi: 10.4161/hv.28428
9. Bruxelle JF, Péchiné S, Collignon A. Immunization Strategies Against Clostridium Difficile. Adv Exp Med Biol (2018) 1050:197–225. doi: 10.1007/978-3-319-72799-8_12
10. Bézay N, Ayad A, Dubischar K, Firbas C, Hochreiter R, Kiermayr S, et al. Safety, Immunogenicity and Dose Response of VLA84, a New Vaccine Candidate Against Clostridium Difficile, in Healthy Volunteers. Vaccine (2016) 34(23):2585–92. doi: 10.1016/j.vaccine.2016.03.098
11. de Bruyn G, Saleh J, Workman D, Pollak R, Elinoff V, Fraser NJ, et al. Defining the Optimal Formulation and Schedule of a Candidate Toxoid Vaccine Against Clostridium Difficile Infection: A Randomized Phase 2 Clinical Trial. Vaccine (2016) 34(19):2170–8. doi: 10.1016/j.vaccine.2016.03.028
12. Pfizer. A Phase 3, Randomized Observer-Blinded Study To Evaluate the Immunogenicity, Safety, and Tolerability of 2 Doses Compared to 3 Doses of Clostridium Difficile Vaccine in Adults 50 Years of Age and Older (2020). Available at: https://clinicaltrials.gov/ct2/show/NCT03918629.
13. Spencer J, Leuzzi R, Buckley A, Irvine J, Candlish D, Scarselli M, et al. Vaccination Against Clostridium Difficile Using Toxin Fragments: Observations and Analysis in Animal Models. Gut Microbes (2014) 5(2):225–32. doi: 10.4161/gmic.27712
14. Siddiqui F, O’Connor JR, Nagaro K, Cheknis A, Sambol SP, Vedantam G, et al. Vaccination With Parenteral Toxoid B Protects Hamsters Against Lethal Challenge With Toxin A-Negative, Toxin B-Positive Clostridium Difficile But Does Not Prevent Colonization. J Infect Dis (2012) 205(1):128–33. doi: 10.1093/infdis/jir688
15. Maldarelli GA, Matz H, Gao S, Chen K, Hamza T, Yfantis HG, et al. Pilin Vaccination Stimulates Weak Antibody Responses and Provides No Protection in a C57Bl/6 Murine Model of Acute Clostridium Difficile Infection. J Vaccines Vaccin (2016) 7(3). doi: 10.4172/2157-7560.1000321
16. Péchiné S, Bruxelle JF, Janoir C, Collignon A. Targeting Clostridium Difficile Surface Components to Develop Immunotherapeutic Strategies Against Clostridium Difficile Infection. Front Microbiol (2018) 9:1009. doi: 10.3389/fmicb.2018.01009
17. Sandolo C, Péchiné S, Le Monnier A, Hoys S, Janoir C, Coviello T, et al. Encapsulation of Cwp84 Into Pectin Beads for Oral Vaccination Against Clostridium Difficile. Eur J Pharm Biopharm (2011) 79(3):566–73. doi: 10.1016/j.ejpb.2011.05.011
18. Péchiné S, Hennequin C, Boursier C, Hoys S, Collignon A. Immunization Using GroEL Decreases Clostridium Difficile Intestinal Colonization. PloS One (2013) 8(11):e81112. doi: 10.1371/journal.pone.0081112
19. Ghose C, Eugenis I, Sun X, Edwards AN, McBride SM, Pride DT, et al. Immunogenicity and Protective Efficacy of Recombinant Clostridium Difficile Flagellar Protein FliC. Emerg Microbes Infect (2016) 5(2):e8. doi: 10.1038/emi.2016.8
20. Toledo H, Baly A, Castro O, Resik S, Laferté J, Rolo F, et al. A Phase I Clinical Trial of a Multi-Epitope Polypeptide TAB9 Combined With Montanide ISA 720 Adjuvant in Non-HIV-1 Infected Human Volunteers. Vaccine (2001) 19(30):4328–36. doi: 10.1016/s0264-410x(01)00111-6
21. Safavi A, Kefayat A, Sotoodehnejadnematalahi F, Salehi M, Modarressi MH. Production, Purification, and In Vivo Evaluation of a Novel Multiepitope Peptide Vaccine Consisted of Immunodominant Epitopes of SYCP1 and ACRBP Antigens as a Prophylactic Melanoma Vaccine. Int Immunopharmacol (2019) 76:105872. doi: 10.1016/j.intimp.2019.105872
22. Jiang P, Cai Y, Chen J, Ye X, Mao S, Zhu S, et al. Evaluation of Tandem Chlamydia Trachomatis MOMP Multi-Epitopes Vaccine in BALB/c Mice Model. Vaccine (2017) 35(23):3096–103. doi: 10.1016/j.vaccine.2017.04.031
23. Yu M, Zhu Y, Li Y, Chen Z, Sha T, Li Z, et al. Design of a Novel Multi-Epitope Vaccine Against Echinococcus Granulosus in Immunoinformatics. Front Immunol (2021) 12:668492. doi: 10.3389/fimmu.2021.668492
24. Jump RL, Pultz MJ, Donskey CJ. Vegetative Clostridium Difficile Survives in Room Air on Moist Surfaces and in Gastric Contents With Reduced Acidity: A Potential Mechanism to Explain the Association Between Proton Pump Inhibitors and C. Difficile-Associated Diarrhea? Antimicrob Agents Chemother (2007) 51(8):2883–7. doi: 10.1128/aac.01443-06
25. Barra-Carrasco J, Paredes-Sabja D. Clostridium Difficile Spores: A Major Threat to the Hospital Environment. Future Microbiol (2014) 9(4):475–86. doi: 10.2217/fmb.14.2
26. Pizarro-Guajardo M, Calderón-Romero P, Paredes-Sabja D. Ultrastructure Variability of the Exosporium Layer of Clostridium Difficile Spores From Sporulating Cultures and Biofilms. Appl Environ Microbiol (2016) 82(19):5892–8. doi: 10.1128/aem.01463-16
27. Barra-Carrasco J, Olguín-Araneda V, Plaza-Garrido A, Miranda-Cárdenas C, Cofré-Araneda G, Pizarro-Guajardo M, et al. The Clostridium Difficile Exosporium Cysteine (CdeC)-Rich Protein is Required for Exosporium Morphogenesis and Coat Assembly. J Bacteriol (2013) 195(17):3863–75. doi: 10.1128/jb.00369-13
28. Calderón-Romero P, Castro-Córdova P, Reyes-Ramírez R, Milano-Céspedes M, Guerrero-Araya E, Pizarro-Guajardo M, et al. Clostridium Difficile Exosporium Cysteine-Rich Proteins are Essential for the Morphogenesis of the Exosporium Layer, Spore Resistance, and Affect C. Difficile Pathogenesis. PloS Pathog (2018) 14(8):e1007199. doi: 10.1371/journal.ppat.1007199
29. Ghose C, Eugenis I, Edwards AN, Sun X, McBride SM, Ho DD. Immunogenicity and Protective Efficacy of Clostridium Difficile Spore Proteins. Anaerobe (2016) 37:85–95. doi: 10.1016/j.anaerobe.2015.12.001
30. Pizarro-Guajardo M, Ravanal MC, Paez MD, Callegari E, Paredes-Sabja D. Identification of Clostridium Difficile Immunoreactive Spore Proteins of the Epidemic Strain R20291. Proteomics Clin Appl (2018) 12(5):e1700182. doi: 10.1002/prca.201700182
31. Duan Q, Zhou M, Zhu L, Zhu G. Flagella and Bacterial Pathogenicity. J Basic Microbiol (2013) 53(1):1–8. doi: 10.1002/jobm.201100335
32. Döring G, Meisner C, Stern M. A Double-Blind Randomized Placebo-Controlled Phase III Study of a Pseudomonas Aeruginosa Flagella Vaccine in Cystic Fibrosis Patients. Proc Natl Acad Sci USA (2007) 104(26):11020–5. doi: 10.1073/pnas.0702403104
33. Tasteyre A, Karjalainen T, Avesani V, Delmée M, Collignon A, Bourlioux P, et al. Molecular Characterization of fliD Gene Encoding Flagellar Cap and its Expression Among Clostridium Difficile Isolates From Different Serogroups. J Clin Microbiol (2001) 39(3):1178–83. doi: 10.1128/jcm.39.3.1178-1183.2001
34. Tasteyre A, Karjalainen T, Avesani V, Delmée M, Collignon A, Bourlioux P, et al. Phenotypic and Genotypic Diversity of the Flagellin Gene (Flic) Among Clostridium Difficile Isolates From Different Serogroups. J Clin Microbiol (2000) 38(9):3179–86. doi: 10.1128/jcm.38.9.3179-3186.2000
35. Péchiné S, Gleizes A, Janoir C, Gorges-Kergot R, Barc MC, Delmée M, et al. Immunological Properties of Surface Proteins of Clostridium Difficile. J Med Microbiol (2005) 54(Pt 2):193–6. doi: 10.1099/jmm.0.45800-0
36. Larsen MV, Lundegaard C, Lamberth K, Buus S, Lund O, Nielsen M. Large-Scale Validation of Methods for Cytotoxic T-Lymphocyte Epitope Prediction. BMC Bioinf (2007) 8:424. doi: 10.1186/1471-2105-8-424
37. Doytchinova IA, Flower DR. Identifying Candidate Subunit Vaccines Using an Alignment-Independent Method Based on Principal Amino Acid Properties. Vaccine (2007) 25(5):856–66. doi: 10.1016/j.vaccine.2006.09.032
38. Doytchinova IA, Flower DR. VaxiJen: A Server for Prediction of Protective Antigens, Tumour Antigens and Subunit Vaccines. BMC Bioinf (2007) 8:4. doi: 10.1186/1471-2105-8-4
39. Calis JJ, Maybeno M, Greenbaum JA, Weiskopf D, De Silva AD, Sette A, et al. Properties of MHC Class I Presented Peptides That Enhance Immunogenicity. PloS Comput Biol (2013) 9(10):e1003266. doi: 10.1371/journal.pcbi.1003266
40. Dimitrov I, Bangov I, Flower DR, Doytchinova I. AllerTOP V.2–a Server for in Silico Prediction of Allergens. J Mol Model (2014) 20(6):2278. doi: 10.1007/s00894-014-2278-5
41. Gupta S, Kapoor P, Chaudhary K, Gautam A, Kumar R, Raghava GP. In Silico Approach for Predicting Toxicity of Peptides and Proteins. PloS One (2013) 8(9):e73957. doi: 10.1371/journal.pone.0073957
42. Paul S, Sidney J, Sette A, Peters B. TepiTool: A Pipeline for Computational Prediction of T Cell Epitope Candidates. Curr Protoc Immunol (2016) 114:18.19.11–18.19.24. doi: 10.1002/cpim.12
43. Reynisson B, Barra C, Kaabinejadian S, Hildebrand WH, Peters B, Nielsen M. Improved Prediction of MHC II Antigen Presentation Through Integration and Motif Deconvolution of Mass Spectrometry MHC Eluted Ligand Data. J Proteome Res (2020) 19(6):2304–15. doi: 10.1021/acs.jproteome.9b00874
44. Steinke JW, Borish L. Th2 Cytokines and Asthma. Interleukin-4: Its Role in the Pathogenesis of Asthma, and Targeting it for Asthma Treatment With Interleukin-4 Receptor Antagonists. Respir Res (2001) 2(2):66–70. doi: 10.1186/rr40
45. Ivashkiv LB. Ifnγ: Signalling, Epigenetics and Roles in Immunity, Metabolism, Disease and Cancer Immunotherapy. Nat Rev Immunol (2018) 18(9):545–58. doi: 10.1038/s41577-018-0029-z
46. Dhanda SK, Vir P, Raghava GP. Designing of Interferon-Gamma Inducing MHC Class-II Binders. Biol Direct (2013) 8:30. doi: 10.1186/1745-6150-8-30
47. Dhanda SK, Gupta S, Vir P, Raghava GP. Prediction of IL4 Inducing Peptides. Clin Dev Immunol (2013) 2013:263952. doi: 10.1155/2013/263952
48. Dinarello CA. Historical Insights Into Cytokines. Eur J Immunol (2007) 37 Suppl 1(Suppl 1):S34–45. doi: 10.1002/eji.200737772
49. Gupta S, Madhu MK, Sharma AK, Sharma VK. ProInflam: A Webserver for the Prediction of Proinflammatory Antigenicity of Peptides and Proteins. J Transl Med (2016) 14(1):178. doi: 10.1186/s12967-016-0928-3
50. Damoiseaux J. The IL-2 - IL-2 Receptor Pathway in Health and Disease: The Role of the Soluble IL-2 Receptor. Clin Immunol (2020) 218:108515. doi: 10.1016/j.clim.2020.108515
51. Saha S, Raghava GP. Prediction of Continuous B-Cell Epitopes in an Antigen Using Recurrent Neural Network. Proteins (2006) 65(1):40–8. doi: 10.1002/prot.21078
52. Altschul SF, Madden TL, Schäffer AA, Zhang J, Zhang Z, Miller W, et al. Gapped BLAST and PSI-BLAST: A New Generation of Protein Database Search Programs. Nucleic Acids Res (1997) 25(17):3389–402. doi: 10.1093/nar/25.17.3389
53. Hu JC, Greene CJ, King-Lyons ND, Connell TD. The Divergent CD8+ T Cell Adjuvant Properties of LT-IIb and LT-IIc, Two Type II Heat-Labile Enterotoxins, Are Conferred by Their Ganglioside-Binding B Subunits. PloS One (2015) 10(11):e0142942. doi: 10.1371/journal.pone.0142942
54. Wilkins MR, Gasteiger E, Bairoch A, Sanchez JC, Williams KL, Appel RD, et al. Protein Identification and Analysis Tools in the ExPASy Server. Methods Mol Biol (1999) 112:531–52. doi: 10.1385/1-59259-584-7:531
55. Mahdevar E, Safavi A, Abiri A, Kefayat A, Hejazi SH, Miresmaeili SM, et al. Exploring the Cancer-Testis Antigen BORIS to Design a Novel Multi-Epitope Vaccine Against Breast Cancer Based on Immunoinformatics Approaches. J Biomol Struct Dyn (2021) 18:1–18. doi: 10.1080/07391102.2021.1883111
56. Safavi A, Kefayat A, Mahdevar E, Abiri A, Ghahremani F. Exploring the Out of Sight Antigens of SARS-CoV-2 to Design a Candidate Multi-Epitope Vaccine by Utilizing Immunoinformatics Approaches. Vaccine (2020) 38(48):7612–28. doi: 10.1016/j.vaccine.2020.10.016
57. Safavi A, Kefayat A, Abiri A, Mahdevar E, Behnia AH, Ghahremani F. In Silico Analysis of Transmembrane Protein 31 (TMEM31) Antigen to Design Novel Multiepitope Peptide and DNA Cancer Vaccines Against Melanoma. Mol Immunol (2019) 112:93–102. doi: 10.1016/j.molimm.2019.04.030
58. Safavi A, Kefayat A, Sotoodehnejadnematalahi F, Salehi M, Modarressi MH. In Silico Analysis of Synaptonemal Complex Protein 1 (SYCP1) and Acrosin Binding Protein (ACRBP) Antigens to Design Novel Multiepitope Peptide Cancer Vaccine Against Breast Cancer. Int J Pept Res Ther (2019) 25(4):1343–59. doi: 10.1007/s10989-018-9780-z
59. Cornejo-Granados F, Hurtado-Ramírez JM, Hernández-Pando R, Ochoa-Leyva A. Secret-AAR: A Web Server to Assess the Antigenic Density of Proteins and Homology Search Against Bacterial and Parasite Secretome Proteins. Genomics (2019) 111(6):1514–6. doi: 10.1016/j.ygeno.2018.10.007
60. Magnan CN, Zeller M, Kayala MA, Vigil A, Randall A, Felgner PL, et al. High-Throughput Prediction of Protein Antigenicity Using Protein Microarray Data. Bioinformatics (2010) 26(23):2936–43. doi: 10.1093/bioinformatics/btq551
61. Magnan CN, Randall A, Baldi P. SOLpro: Accurate Sequence-Based Prediction of Protein Solubility. Bioinformatics (2009) 25(17):2200–7. doi: 10.1093/bioinformatics/btp386
62. Nielsen H. SignalP 6.0 Predicts All Five Types of Signal Peptides Using Protein Language Models. Available at: https://bioengineeringcommunity.nature.com/posts/signalp-6-0-predicts-all-five-types-of-signal-peptides-using-protein-language-models (Accessed 2022).
63. Hong HA, Hitri K, Hosseini S, Kotowicz N, Bryan D, Mawas F, et al. Mucosal Antibodies to the C Terminus of Toxin A Prevent Colonization of Clostridium Difficile. Infect Immun (2017) 85(4):e01060–16. doi: 10.1128/iai.01060-16
64. Mantis NJ, Rol N, Corthésy B. Secretory IgA’s Complex Roles in Immunity and Mucosal Homeostasis in the Gut. Mucosal Immunol (2011) 4(6):603–11. doi: 10.1038/mi.2011.41
65. Brandtzaeg P. Secretory IgA: Designed for Anti-Microbial Defense. Front Immunol (2013) 4:222. doi: 10.3389/fimmu.2013.00222
66. Gupta S, Ansari HR, Gautam A, Raghava GPS, Open Source Drug Discovery C. Identification of B-Cell Epitopes in an Antigen for Inducing Specific Class of Antibodies. Biol Direct (2013) 8(1):27. doi: 10.1186/1745-6150-8-27
67. Garnier J, Gibrat JF, Robson B. GOR Method for Predicting Protein Secondary Structure From Amino Acid Sequence. Methods Enzymol (1996) 266:540–53. doi: 10.1016/s0076-6879(96)66034-0
68. Källberg M, Margaryan G, Wang S, Ma J, Xu J. RaptorX Server: A Resource for Template-Based Protein Structure Modeling. Methods Mol Biol (2014) 1137:17–27. doi: 10.1007/978-1-4939-0366-5_2
69. Ko J, Park H, Heo L, Seok C. GalaxyWEB Server for Protein Structure Prediction and Refinement. Nucleic Acids Res (2012) 40(Web Server issue):W294–297. doi: 10.1093/nar/gks493
70. Colovos C, Yeates TO. Verification of Protein Structures: Patterns of Nonbonded Atomic Interactions. Protein Sci (1993) 2(9):1511–9. doi: 10.1002/pro.5560020916
71. Wiederstein M, Sippl MJ. ProSA-Web: Interactive Web Service for the Recognition of Errors in Three-Dimensional Structures of Proteins. Nucleic Acids Res (2007) 35(Web Server issue):W407–410. doi: 10.1093/nar/gkm290
72. Miller JF, Vadas MA. Antigen Activation of T Lymphocytes: Influence of Major Histocompatibility Complex. Cold Spring Harb Symp Quant Biol (1977) 41 Pt 2:579–88. doi: 10.1101/sqb.1977.041.01.067
73. Takeda K, Akira S. Toll-Like Receptors. Curr Protoc Immunol (2015) 109:14.12.11–14.12.10. doi: 10.1002/0471142735.im1412s109
74. Vijay K. Toll-Like Receptors in Immunity and Inflammatory Diseases: Past, Present, and Future. Int Immunopharmacol (2018) 59:391–412. doi: 10.1016/j.intimp.2018.03.002
75. Feuillet V, Medjane S, Mondor I, Demaria O, Pagni PP, Galán JE, et al. Involvement of Toll-Like Receptor 5 in the Recognition of Flagellated Bacteria. Proc Natl Acad Sci USA (2006) 103(33):12487–92. doi: 10.1073/pnas.0605200103
76. LeBien TW, Tedder TF. B Lymphocytes: How They Develop and Function. Blood (2008) 112(5):1570–80. doi: 10.1182/blood-2008-02-078071
77. van Zundert GCP, Rodrigues J, Trellet M, Schmitz C, Kastritis PL, Karaca E, et al. The HADDOCK2.2 Web Server: User-Friendly Integrative Modeling of Biomolecular Complexes. J Mol Biol (2016) 428(4):720–5. doi: 10.1016/j.jmb.2015.09.014
78. Desta IT, Porter KA, Xia B, Kozakov D, Vajda S. Performance and Its Limits in Rigid Body Protein-Protein Docking. Structure (2020) 28(9):1071–1081.e1073. doi: 10.1016/j.str.2020.06.006
79. Biesiada M, Purzycka KJ, Szachniuk M, Blazewicz J, Adamiak RW. Automated RNA 3d Structure Prediction With RNAComposer. Methods Mol Biol (2016) 1490:199–215. doi: 10.1007/978-1-4939-6433-8_13
80. Gray JJ, Moughon S, Wang C, Schueler-Furman O, Kuhlman B, Rohl CA, et al. Protein-Protein Docking With Simultaneous Optimization of Rigid-Body Displacement and Side-Chain Conformations. J Mol Biol (2003) 331(1):281–99. doi: 10.1016/s0022-2836(03)00670-3
81. Case DA, Cheatham TE, Darden T, Gohlke H, Luo R, Merz KM Jr., et al. The Amber Biomolecular Simulation Programs. J Comput Chem (2005) 26(16):1668–88. doi: 10.1002/jcc.20290
82. Cordomí A, Caltabiano G, Pardo L. Membrane Protein Simulations Using AMBER Force Field and Berger Lipid Parameters. J Chem Theory Comput (2012) 8(3):948–58. doi: 10.1021/ct200491c
83. Bui HH, Sidney J, Dinh K, Southwood S, Newman MJ, Sette A. Predicting Population Coverage of T-Cell Epitope-Based Diagnostics and Vaccines. BMC Bioinf (2006) 7:153. doi: 10.1186/1471-2105-7-153
84. Rapin N, Lund O, Bernaschi M, Castiglione F. Computational Immunology Meets Bioinformatics: The Use of Prediction Tools for Molecular Binding in the Simulation of the Immune System. PloS One (2010) 5(4):e9862. doi: 10.1371/journal.pone.0009862
85. Baneyx F. Recombinant Protein Expression in Escherichia Coli. Curr Opin Biotechnol (1999) 10(5):411–21. doi: 10.1016/s0958-1669(99)00003-8
86. Grote A, Hiller K, Scheer M, Münch R, Nörtemann B, Hempel DC, et al. JCat: A Novel Tool to Adapt Codon Usage of a Target Gene to its Potential Expression Host. Nucleic Acids Res (2005) 33(Web Server issue):W526–531. doi: 10.1093/nar/gki376
87. Shilling PJ, Mirzadeh K, Cumming AJ, Widesheim M, Köck Z, Daley DO. Improved Designs for pET Expression Plasmids Increase Protein Production Yield in Escherichia Coli. Commun Biol (2020) 3(1):214. doi: 10.1038/s42003-020-0939-8
88. Safavi A, Kefayat A, Mahdevar E, Ghahremani F, Nezafat N, Modarressi MH. Efficacy of Co-Immunization With the DNA and Peptide Vaccines Containing SYCP1 and ACRBP Epitopes in a Murine Triple-Negative Breast Cancer Model. Hum Vaccin Immunother (2021) 17(1):22–34. doi: 10.1080/21645515.2020.1763693
89. Rosano GL, Ceccarelli EA. Recombinant Protein Expression in Escherichia Coli: Advances and Challenges. Front Microbiol (2014) 5:172. doi: 10.3389/fmicb.2014.00172
90. Pereira LM, Baroni L, Bezerra MA, Yatsuda AP. A Hybrid Plasmid pGEM-Pet28 Applied for Heterologous Expression of Neospora Caninum Actin. Matters (2017) 3. doi: 10.19185/matters.201702000012
91. Doherty PC, Allan W, Eichelberger M, Carding SR. Roles of Alpha Beta and Gamma Delta T Cell Subsets in Viral Immunity. Annu Rev Immunol (1992) 10:123–51. doi: 10.1146/annurev.iy.10.040192.001011
92. Panina-Bordignon P, Tan A, Termijtelen A, Demotz S, Corradin G, Lanzavecchia A. Universally Immunogenic T Cell Epitopes: Promiscuous Binding to Human MHC Class II and Promiscuous Recognition by T Cells. Eur J Immunol (1989) 19(12):2237–42. doi: 10.1002/eji.1830191209
93. Getzoff ED, Tainer JA, Lerner RA, Geysen HM. The Chemistry and Mechanism of Antibody Binding to Protein Antigens. Adv Immunol (1988) 43:1–98. doi: 10.1016/s0065-2776(08)60363-6
94. Ikai A. Thermostability and Aliphatic Index of Globular Proteins. J Biochem (1980) 88(6):1895–8.
95. Kyte J, Doolittle RF. A Simple Method for Displaying the Hydropathic Character of a Protein. J Mol Biol (1982) 157(1):105–32. doi: 10.1016/0022-2836(82)90515-0
96. Naz A, Shahid F, Butt TT, Awan FM, Ali A, Malik A. Designing Multi-Epitope Vaccines to Combat Emerging Coronavirus Disease 2019 (COVID-19) by Employing Immuno-Informatics Approach. Front Immunol (2020) 11:1663. doi: 10.3389/fimmu.2020.01663
97. Chauhan V, Rungta T, Rawat M, Goyal K, Gupta Y, Singh MP. Excavating SARS-Coronavirus 2 Genome for Epitope-Based Subunit Vaccine Synthesis Using Immunoinformatics Approach. J Cell Physiol (2021) 236(2):1131–47. doi: 10.1002/jcp.29923
98. Shahid F, Zaheer T, Ashraf ST, Shehroz M, Anwer F, Naz A, et al. Chimeric Vaccine Designs Against Acinetobacter Baumannii Using Pan Genome and Reverse Vaccinology Approaches. Sci Rep (2021) 11(1):13213. doi: 10.1038/s41598-021-92501-8
99. Sierocka A, Kiersnowska Z, Lemiech-Mirowska E, Marczak M. Costs Associated With the Treatment of Clostridioides Difficile Infections. Int J Environ Res Public Health (2021) 18(14). doi: 10.3390/ijerph18147647
100. Cornely OA, Miller MA, Louie TJ, Crook DW, Gorbach SL. Treatment of First Recurrence of Clostridium Difficile Infection: Fidaxomicin Versus Vancomycin. Clin Infect Dis (2012) 55 Suppl 2(Suppl 2):S154–161. doi: 10.1093/cid/cis462
101. Spigaglia P, Mastrantonio P, Barbanti F. Antibiotic Resistances of Clostridium Difficile. Adv Exp Med Biol (2018) 1050:137–59. doi: 10.1007/978-3-319-72799-8_9
102. Hernandez LD, Kroh HK, Hsieh E, Yang X, Beaumont M, Sheth PR, et al. Epitopes and Mechanism of Action of the Clostridium Difficile Toxin A-Neutralizing Antibody Actoxumab. J Mol Biol (2017) 429(7):1030–44. doi: 10.1016/j.jmb.2017.02.010
103. DB NE, O’Brien JB, McCabe MS, Athié-Morales V, Kelleher DP. Active Immunization of Hamsters Against Clostridium Difficile Infection Using Surface-Layer Protein. FEMS Immunol Med Microbiol (2008) 52(2):207–18. doi: 10.1111/j.1574-695X.2007.00363.x
104. Romero-Rodríguez A, Troncoso-Cotal S, Guerrero-Araya E, Paredes-Sabja D. The Clostridioides Difficile Cysteine-Rich Exosporium Morphogenetic Protein, CdeC, Exhibits Self-Assembly Properties That Lead to Organized Inclusion Bodies in Escherichia Coli. mSphere (2020) 5(6):e01065–20. doi: 10.1128/mSphere.01065-20
105. Hsieh CS, Heimberger AB, Gold JS, O’Garra A, Murphy KM. Differential Regulation of T Helper Phenotype Development by Interleukins 4 and 10 in an Alpha Beta T-Cell-Receptor Transgenic System. Proc Natl Acad Sci USA (1992) 89(13):6065–9. doi: 10.1073/pnas.89.13.6065
106. Connell TD. Cholera Toxin, LT-I, LT-IIa and LT-IIb: The Critical Role of Ganglioside Binding in Immunomodulation by Type I and Type II Heat-Labile Enterotoxins. Expert Rev Vaccines (2007) 6(5):821–34. doi: 10.1586/14760584.6.5.821
107. Hu JC, Mathias-Santos C, Greene CJ, King-Lyons ND, Rodrigues JF, Hajishengallis G, et al. Intradermal Administration of the Type II Heat-Labile Enterotoxins LT-IIb and LT-IIc of Enterotoxigenic Escherichia Coli Enhances Humoral and CD8+ T Cell Immunity to a Co-Administered Antigen. PloS One (2014) 9(12):e113978. doi: 10.1371/journal.pone.0113978
108. Livingston B, Crimi C, Newman M, Higashimoto Y, Appella E, Sidney J, et al. A Rational Strategy to Design Multiepitope Immunogens Based on Multiple Th Lymphocyte Epitopes. J Immunol (2002) 168(11):5499–506. doi: 10.4049/jimmunol.168.11.5499
109. Amet N, Lee HF, Shen WC. Insertion of the Designed Helical Linker Led to Increased Expression of Tf-Based Fusion Proteins. Pharm Res (2009) 26(3):523–8. doi: 10.1007/s11095-008-9767-0
110. Ahmad S, Shahid F, Tahir Ul Qamar M, Rehman HU, Abbasi SW, Sajjad W, et al. Immuno-Informatics Analysis of Pakistan-Based HCV Subtype-3a for Chimeric Polypeptide Vaccine Design. Vaccines (Basel) (2021) 9(3). doi: 10.3390/vaccines9030293
Keywords: Clostridium difficile, multi-epitope vaccine, molecular docking, molecular dynamics simulation, immunoinformatics
Citation: Tan C, Zhu F, Xiao Y, Wu Y, Meng X, Liu S, Liu T, Chen S, Zhou J, Li C and Wu A (2022) Immunoinformatics Approach Toward the Introduction of a Novel Multi-Epitope Vaccine Against Clostridium difficile. Front. Immunol. 13:887061. doi: 10.3389/fimmu.2022.887061
Received: 02 March 2022; Accepted: 05 April 2022;
Published: 26 May 2022.
Edited by:
Jeremy P. McAleer, Marshall University, United StatesReviewed by:
William Petri, University of Virginia, United StatesAmirhosein Kefayat, Isfahan University of Medical Sciences, Iran
Copyright © 2022 Tan, Zhu, Xiao, Wu, Meng, Liu, Liu, Chen, Zhou, Li and Wu. This is an open-access article distributed under the terms of the Creative Commons Attribution License (CC BY). The use, distribution or reproduction in other forums is permitted, provided the original author(s) and the copyright owner(s) are credited and that the original publication in this journal is cited, in accordance with accepted academic practice. No use, distribution or reproduction is permitted which does not comply with these terms.
*Correspondence: Chunhui Li, bGljaHVuaHVpQGNzdS5lZHUuY24=; Anhua Wu, eHl3dWFuaHVhQGNzdS5lZHUuY24=