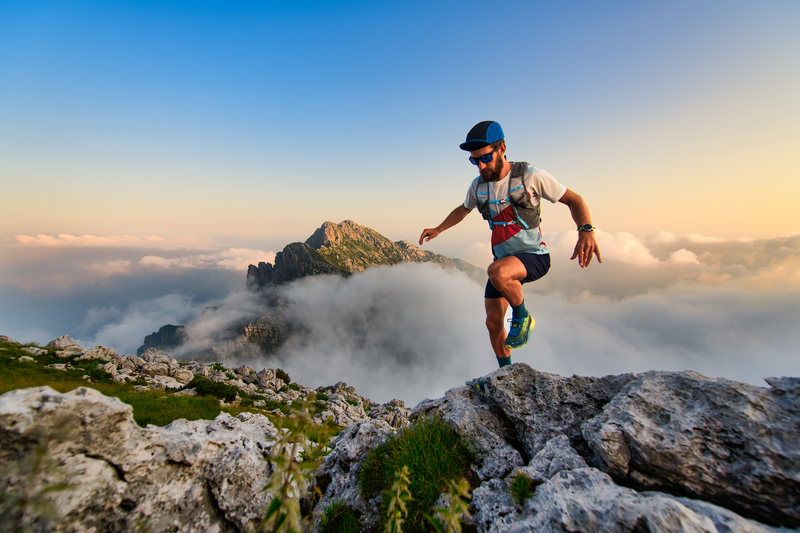
95% of researchers rate our articles as excellent or good
Learn more about the work of our research integrity team to safeguard the quality of each article we publish.
Find out more
MINI REVIEW article
Front. Immunol. , 06 April 2022
Sec. Cancer Immunity and Immunotherapy
Volume 13 - 2022 | https://doi.org/10.3389/fimmu.2022.883989
This article is part of the Research Topic Insights in Cancer Immunity and Immunotherapy: 2021 View all 9 articles
Presentation of tumor-specific or tumor-associated peptides by HLA class I molecules to CD8+ T cells is the foundation of epitope-centric cancer immunotherapies. While often in silico HLA binding predictions or in vitro immunogenicity assays are utilized to select candidates, mass spectrometry-based immunopeptidomics is currently the only method providing a direct proof of actual cell surface presentation. Despite much progress in the last decade, identification of such HLA-presented peptides remains challenging. Here we review typical workflows and current developments in the field of immunopeptidomics, highlight the challenges which remain to be solved and emphasize the importance of direct target validation for clinical immunotherapy development.
Immunotherapy has been established as the fourth pillar of cancer treatment. The immune system’s ability to discriminate self from non-self constitutes the basis of all immunotherapeutic interventions. Essential for this discrimination process are small peptides, termed epitopes, presented via human leukocyte antigen (HLA) complexes at the cell surface allowing constant monitoring of both intra- and extracellular protein expression and thus the cell’s health state. HLA class I molecules are expressed by virtually all nucleated cells and present short peptides (8-15 amino acids) of mainly intracellular origin. HLA class I:peptide complexes are surveyed by CD8+ T cells and allow them to identify and, ultimately, eliminate infected or malignant cells. HLA class II expression is restricted to professional antigen presenting cells and mainly responsible for the display of longer peptides (up to 25 amino acids) of extracellular origin to CD4+ T cells (1). Collectively, all HLA-presented peptides are termed immunopeptidome.
Essential for the development of epitope-specific cancer immunotherapies such as therapeutic vaccines or T cell receptor (TCR)-transgenic T cells is the identification of tumor-associated antigens (TAAs) or tumor-specific antigens (TSAs). Tumor-associated antigens show low expression or are absent in healthy, adult tissue (2). TAAs are categorized into developmental antigens (normally expressed only during embryonal development), cancer-testis antigens (normally expressed only in reproductive tissue), overexpressed antigens, and post-translationally modified antigens. Examples of well-studied TAAs include the developmental antigen CEA (carcinoembryonic antigen), the cancer-testis antigens of the MAGE (melanoma-associated antigen) family and NY-ESO-1, the overexpressed TAAs epidermal growth factor receptor 2 (HER2) in breast cancer or CD19 in B cell malignancies, and the post-translationally modified antigen MUC-1 (3). Importantly, immune responses against TAAs are limited by central tolerance mechanisms and often lack complete specificity to cancer cells. In contrast, tumor-specific antigens represent optimal targets since they are exclusively expressed on cancer cells but not on healthy host cells and are therefore not subject to central tolerance. TSAs can arise from genomic protein-coding alterations such as single nucleotide variants, gene fusions, or InDel mutations (4–9). Collectively, these TSAs have been termed “neoantigens”. More recently, the antigenic repertoire has been extended by “non-canonical tumor antigens”, for which it is not yet clear if they are tumor-associated or truly tumor-specific, and therefore their possible clinical relevance is highly debated. They arise from alternative mRNA splicing (10, 11), RNA editing (12, 13), usage of alternative transcription start sites or reading frames (14, 15), as well as cryptic peptides that are produced by proteasomal splicing and lack exome evidence (16, 17). Other sources of tumor antigens include peptides with post-translational modifications (18, 19) and peptides of viral (20, 21) or bacterial origin (22) presented in a tumor-associated or tumor-specific manner (Figure 1).
Figure 1 Processes involved in antigen processing and presentation and their effect on the generation of a diverse HLA class I peptide repertoire. Peptides for surface presentation by HLA class I molecules are generated by transcription of DNA into mRNA and subsequent translation into proteins. Here, processes such as genetic variation and epigenetic regulation at the gene level, alternative splicing and RNA editing at the transcript level, the translation of alternative open reading frames (ORFs) and non-coding regions and post-transcriptional modifications (PTMs) can enlarge the peptide repertoire. Eventually, proteins are degraded by the proteasome, generating peptides. Here, proteasomal splicing can increase peptide diversity while proteasomal degradation can also reduce the size of the repertoire by destroying some potential HLA binders. Finally, peptides are selectively conveyed via the transporter associated with antigen processing (TAP) into the lumen of the endoplasmic reticulum (ER). Here, some peptides are further trimmed by ER-associated aminopeptidases (ERAP) and then selectively associate with specific human leukocyte antigen (HLA) complexes. Eventually, stable HLA:peptide complexes are transported to the cell surface for interaction with CD8+ T cells. Created with BioRender.com.
The main strategies used today to identify neoantigens are based on next-generation sequencing data and subsequent prioritization of candidates using in silico HLA binding prediction. At best, peptide immunogenicity is further validated using in vitro assays, however, whether these peptides are actually presented via HLA class I molecules on the target cell surface is rarely confirmed. This is all the more important, because sequencing data and even assessment of a potential source protein’s expression level are not directly reflected in epitope presentation. In fact, biogenesis of HLA class I-presented peptides does not obey the “law of mass action”, meaning that the proportion of presented epitopes does not represent the amount of the source proteins within the cell’s proteome (23). While this allows the efficient sampling of a large part of a cell’s proteome and the timely recognition of malignant or infected cells, it hampers the identification of neoantigens solely based on next generation sequencing and in silico HLA binding prediction. Additionally, peptides derived from non-canonical open reading frames constitute a non-neglectable part of the immunopeptidome, which, however, is not completely assessable by basic next-generation sequencing approaches (24, 25).
Currently, the only available technique to provide a direct proof of presentation for clinically relevant target epitopes is mass spectrometry-based immunopeptidomics.
In order to identify tumor-specific peptide sequences in immunopeptidomics assays, these sequences first have to be identified from next-generation sequencing data and included into the “search space” [recently reviewed in (26)]. At the DNA level, data generated by whole-genome sequencing (WGS) and whole-exome sequencing (WES) are commonly used for this task. While WES is less cost-intensive than WGS, it only allows the identification of tumor antigens derived from protein-coding parts of the genome. Analyses deploying RNA sequencing technologies further allow the identification of potential tumor antigens derived from other sources such as alternative splicing, intron retention, RNA editing and other non-canonical sequences. Additionally, RNA sequencing provides information on gene expression levels, which, however, should be handled with caution when prioritizing tumor antigen candidates (see above and “Lessons learned from immunopeptidomics”). Ribosome profiling (Ribo-seq) allows the identification of transcripts undergoing active translation. Using the inherent periodicity of the obtained reads it is possible to generate sample-specific de novo reference proteomes including previously unannotated ORFs potentially harboring tumor antigens (15, 27).
Importantly, all these workflows usually generate large datasets of potential tumor antigens, exceeding the capacity to test all of them in laborious and time-consuming cellular assays. Thus, computational revision of the “search space” is typically performed as a next step.
Since there are a large number of biological processes involved in antigen processing and presentation, there are just as many computational tools to predict if a given peptide is likely to be presented at the cell surface. For HLA class I ligands, ubiquitinated proteins are digested in the cytosol by the proteasome, which can exist in the canonical form and, induced by interferons, as immunoproteasome. Given the different cleavage specificities of these subtypes, some tumor antigens require one or the other form to be generated efficiently (28, 29). Several algorithms exist for the prediction of proteasomal cleavage sites, for example, NetChop20S (30) and ProteaSMM (31) which are trained on in vitro degradation data. After their release from the proteasome into the cytosol, peptides may be further trimmed by cytosolic peptidases such as e.g. TPPII. NetChopCterm (30) is trained on actual HLA ligand data taking into account these other proteases involved in antigen processing and therefore performs best for prediction of HLA ligand processing (32). Resulting peptides are transported into the endoplasmatic reticulum (ER) via transporter associated with antigen processing (TAP) molecules, which again show selectivity for certain peptide characteristics. As for proteasomal cleavage, several algorithms have been developed to model peptide transport into the ER (31, 33, 34). In the ER, peptides can be further trimmed by ER-resident peptidases such as endoplasmatic reticulum aminopeptidases (ERAP), before being loaded onto HLA class I molecules. Both, polygeny and polymorphism of the HLA class I gene locus ensure that a large peptide repertoire can be displayed to CD8+ T cells by forming different peptide binding groves. Conserved amino acids at the anchor positions of the bound peptides allow sequence clustering to identify HLA binding motifs (35–37), defining HLA supertypes and developing algorithms for HLA binding prediction. Since the binding affinity of a peptide to a given HLA molecule is thought to be the most critical determinant of actual peptide presentation, much effort has been invested in the development and constant improvement of such algorithms (38–40). While most of the initial algorithms were based solely on binding affinity data, newer iterations have been much improved by the incorporation of eluted ligand data generated by mass spectrometry (41, 42). Nevertheless, several studies reported discrepancies between predicted binders and actually presented peptides (43–45). Moreover, shortlisting of candidates by HLA binding predictions creates an a priori bias and might exclude actually presented tumor-specific epitopes (46, 47). Both considerations are particularly important when utilizing predictions for rare HLA alleles with little to no data available to train the respective algorithms. Ultimately, tumor-specific peptides presented by HLA class I molecules at the cell surface have to be recognized by CD8+ T cells in order to induce tumor cell elimination. As not all presented tumor-specific epitopes trigger the necessary T cell activation, recent modeling approaches focused on the prediction of immunogenicity based on peptide presentation and recognition characteristics (48, 49) or by structural modeling of HLA:peptide and HLA:peptide:TCR complexes (50, 51). A number of computational pipelines implementing next-generation sequencing data and prediction of HLA ligand processing and binding have been proposed and used to prioritize tumor antigen candidates [(52, 53); for an extensive list see (54)].
The field of immunopeptidomics first emerged in the early 1990s when peptides presented by HLA molecules were characterized using Edman degradation (35, 55) and mass spectrometry (56). More recently, the field gained new momentum with the introduction of high-sensitivity MS instrumentation and the finding that T cells, reactivated by immune checkpoint blockade, specifically recognize tumor-specific epitopes (57–59). In contrast to the enormous technical advancements, the basic principles of immunopeptidomics sample preparation have remained largely the same (60). HLA-presented peptides are either dissociated nonspecifically by mild acid elution (MAE) or HLA:peptide complexes are purified by immunoprecipitation (IP) using a specific antibody with subsequent separation of peptides and HLA molecules. Sample preparation by IP represents the currently preferred method due to its high specificity and increased yield compared to MAE (61).
Different MS acquisition methods have been applied for the interrogation of the immunopeptidome and the identification of tumor antigens: data-dependent acquisition (DDA), targeted data acquisition, and more recently data-independent acquisition (DIA). As most immunopeptidomic experiments aim at the identification of yet unknown epitopes, discovery-driven DDA is the most commonly used method for the generation of tandem mass spectra. The obtained data is then used for the identification of peptides and their amino acid sequence either by comparison to theoretical mass spectra of all precursors in a database with a similar mass-to-charge (m/z) ratio, by de novo sequencing, or a combination of the two approaches. Using DDA, several thousand unique HLA class I-presented peptides can be identified. Since the heuristic selection and subsequent fragmentation of peptide precursors depend on their abundance, low abundant peptides (such as most tumor-specific epitopes) are prone to be missed by DDA, and DDA lacks reproducibility and quantitative accuracy. In contrast, targeted acquisition methods such as selective/multiple reaction monitoring (S/MRM) and parallel reaction monitoring (PRM) are considered the gold standard for identification and quantification, in particular of low abundant peptides. In targeted approaches, only predefined peptides are selected for fragmentation. A predefined subset of fragments in the case of S/MRM or all fragments in the case of PRM are then detected and used for identification and quantification. The major drawbacks of these targeted approaches are the need for a priori knowledge of the analyte of interest, i.e. the epitope, as well as the labor-intensive pre-selection of suitable transitions in the case of S/MRM analyses. Targeted analyses are thus restricted to the detection of pre-defined peptide subsets of limited size and do not offer comprehensive investigation of the complete immunopeptidome. More recently, DIA has been introduced for the analysis of the immunopeptidome leading to improvements in both reproducibility and quantification compared to DDA (62–64). For DIA, all precursors within a defined mass-to-charge window are subjected to fragmentation in an unbiased manner generating highly convoluted mass spectra. This process is iterated across the full mass-to-charge range to generate a “digital map” of the analyzed sample. To identify peptides from such mass spectra, they are compared to a spectral library containing fragmentation and retention time information of the peptides of interest. The generation of a high-quality spectral library usually requires preceding extensive DDA analysis of the same or a related sample. More recently, the introduction of library-free approaches (65–67) and tools for in silico prediction of mass spectra (68–70) have enabled comparable performances without the need of an experimental spectral library.
Besides the major improvements in instrumentation, several other aspects of a typical immunopeptidomics workflow have been subjected to optimization. For example, the analysis of the immunopeptidomes from HLA-monoallelic cell lines does not require deconvolution of HLA binding motifs and was shown to improve predictive power of HLA binding algorithms trained on the respective data (71). Other studies utilized secreted and C-terminally tagged HLA class I molecules to increase the purification yield and reduce the need for detergents during sample preparation (72, 73). In the future, similar approaches for rare and poorly investigated HLA alleles might uncover their binding motifs and further improve HLA binding predictions. Additionally, it has been reported that various parameters such as the usage of different detergents for cell lysis or choice of peptide purification methods can both affect the yield and repertoire of identified peptides (74). Studies employing heavy-labelled HLA:peptide complexes estimated peptide losses of up to 99% during immunoprecipitation (75, 76). Several novel approaches for the sample preparation process have been proposed in order to improve both sensitivity and reproducibility, including a high-throughput immunoprecipitation protocol (77), a semi-automated workflow utilizing TMT labeling (78), as well as a microfluidic-based HLA enrichment protocol (79).
For proteogenomics, and mass spectrometry-based immunopeptidomics in particular, extremely inflated search spaces such as those created for example from three- or six-frame translations of RNA-seq and Ribo-Seq data can significantly increase the rate of false-positive identifications (80). This rate can be controlled by target-decoy approaches. Here, decoy sequences (e.g. reversed canonical protein sequences) are included into the database and score distributions of target and decoy matches can be used to separate false from true identifications and calculate false discovery rates (81–83). The confidence in peptide identifications can be increased by combining the results of different search engines (15) or by multi-round database search approaches searching first against canonical protein databases and subsequently against larger databases containing potential neoepitopes (47). MS-Rescue (84) and DeepRescore (85) are machine learning-based algorithms which increase both sensitivity and reliability of peptide identifications using information such as peptide binding motifs, retention times and mass spectra predictions. Moreover, tools to create HLA-specific, and therefore smaller, peptide databases exist (86, 87) and can be used to decrease the probability of false-positive identifications. Additionally, the quality of an immunopeptidomics dataset can be assessed a posteriori by performing HLA binding predictions as well as peptide sequence clustering (36) or by correlating observed retention times with calculated hydrophobicity indices (88) or predicted retention times (89), respectively. Finally, the ultimate proof of a correct peptide identification is provided by the comparison of retention times and mass spectra recorded from synthetic peptides with those obtained from the biological sample (21, 90, 91).
Much progress has been made in the field of immunopeptidomics in the last decade, however, some limitations still apply. There are tens of thousands of potential binders per HLA allele and although it has been shown that there are “hotspots” for the generation of HLA class I-presented peptides (92, 93), it is unlikely that each and every HLA class I-presented peptide will be identified (94, 95). In the case of mutation-derived neoepitopes, it has been postulated that on average only one such neoepitope is identifiable per 1.8x108 non-synonymous mutations and per 1.1x104 unique HLA class I-presented peptides (96), emphasizing the need for increased sensitivity both in sequence variant calling and mass spectrometry. The latter is best illustrated by comparing the already impressive sensitivity of modern mass spectrometers in the attomolar range to the sensitivity of CD8+ T cells, with early reports suggesting that a single to a few hundred HLA class I-presented peptides per cell are sufficient to trigger an immune response (97–99), effectively rendering T cells 103 to 106 times more sensitive than current generation MS instruments (100). Although often used to prioritize neoepitope candidates, RNA expression data should be handled with caution as several studies showed that there is no clear correlation between the transcriptome and the immunopeptidome (15, 101, 102). Moreover, peptides have been identified without the detection of the corresponding transcript, for example if they are derived from long-lived proteins, defective ribosomal products (DRiPs) or short-lived transcripts that are rapidly degraded by nonsense-mediated RNA decay (101, 103–105).
Using sequencing-based approaches for epitope prioritization, there usually remain hundreds to thousands of candidates depending on the mutational load of the investigated sample – an amount that is unfeasible for validation using classical approaches such as in vitro binding or T cell killing assays. In contrast, mass spectrometry-based identification of target epitopes greatly reduces the number of candidates and additionally limits them to those actually processed and presented by HLA class I molecules at the cell surface. For the clinical application of any epitope-based therapy, proof of immunogenicity, i.e. T cell reactivity, represents the ultimate prerequisite. It can be assessed either functionally by the detection of cytokine secretion using ELISA or ELISPOT assays (106, 107), by the direct detection of T cells recognizing a given epitope using peptide-MHC multimers (108, 109) or by in vitro expansion of T cells in combination with TCR sequencing (110, 111). Other considerations before the clinical application of any epitope-based therapy should include the clonal expression and tumor specificity, as well as the “similarity-to-self” of the target epitope. Clonal expression has recently been shown to be a critical determinant for tumor rejection in both mice and humans (112–114). True tumor specificity is of particular importance to avoid off target effects as exemplified by severe neurological toxicity in a clinical trial utilizing MAGE-A3 TCR-engineered T cells (115). Likewise, “similarity-to-self” should be considered for the selection of actionable target epitopes to both avoid off-target effects and to mount effective T cell responses (116). The recent introduction of the HLA Ligand Atlas (117) provides a comprehensive resource of HLA-presented peptides for various benign tissues which should be considered when selecting tumor-associated target epitopes including those arising from non-canonical sources. Despite the importance of proving the immunogenicity of a given candidate epitope, it has to be emphasized that it only can be of therapeutic benefit if it is actually processed and presented by cancer cells (Figure 2).
Figure 2 The importance of being presented. In typical neoantigen identification workflows, candidate tumor antigens are defined from next generation sequencing data (left side). Subsequent in silico binding predictions and in vitro immunogenicity tests identify potential tumor neoepitopes, which, however, are not necessarily presented on the tumor cells themselves. If the target epitope is not present, CD8+ T cells cannot identify the transformed cells and the tumor proliferates (middle). In contrast, mass spectrometry-based immunopeptidomics identifies truly presented and actionable epitopes. Immunotherapies targeting these epitopes lead to an immune attack by CD8+ T cells and tumor destruction (right side). Created with BioRender.com.
Mass spectrometry-based immunopeptidomics currently is the only unbiased methodology for the identification of target epitopes actually HLA-presented on the cell surface and should be utilized when selecting targets for clinical applications such as adoptive cell transfer and cancer vaccines. Although mass spectrometry is still comparatively insensitive relative to T cells and thus might miss some target epitopes, several studies involving immunopeptidomics have been performed or are under active investigation in clinical trials. Vaccination with IMA901, consisting of multiple tumor-associated epitopes identified and validated by the MS-centric XPRESIDENT approach (118), showed an association between T cell responses to tumor-associated epitopes and increased survival in patients with renal cell carcinoma (119). A personalized dendritic cell vaccine approach was used for the delivery of mutation-derived neoepitopes in melanoma patients and shown to promote both expansion as well as diversity of epitope-specific T cells. HLA class I-mediated presentation of the selected epitopes was confirmed by immunopeptidomics (120). Utilizing a warehouse vaccine concept with 14 HLA class I and class II tumor-associated epitopes, iVAC-XS15-CLL01 is currently under clinical investigation for the treatment of chronic lymphocytic leukemia (121). The GAPVAC-101 trial investigated the combination of a warehouse vaccine and a fully personalized vaccine in glioblastoma patients and showed the induction of both CD8+ and CD4+ T cell responses (122).
Ideally, future therapeutic approaches will target epitopes that are shared between patients. Here, tumor-associated epitopes as well as viral epitopes are intriguing targets, however also tumor-specific epitopes can be targeted in larger cohorts for some indications such as microsatellite-instable cancers (123). The increasing number of non-canonical sources for HLA class I-presented peptides as well as the identification of bacteria-derived peptides presented by HLA molecules in a tumor-specific manner potentially represent a rich pool of targetable epitopes (22, 124). Additionally, new therapeutic approaches targeting multiple HLA allotypes will increase the number of patients benefiting from such interventions (125).
Much progress has been made in the field of immunopeptidomics within the last decade and future technical and computational advancements overcoming the previously described remaining limitations and further pushing the limits of peptide detection will certainly strengthen the importance of mass spectrometry-based identification of target epitopes in the future. As the ultimate and most direct proof of epitope presentation remains reserved for immunopeptidomics, it is expected to become even more integral to the development of epitope-specific immunotherapies.
JB and AR wrote this manuscript together and both approved the submitted version.
The authors declare that the research was conducted in the absence of any commercial or financial relationships that could be construed as a potential conflict of interest.
All claims expressed in this article are solely those of the authors and do not necessarily represent those of their affiliated organizations, or those of the publisher, the editors and the reviewers. Any product that may be evaluated in this article, or claim that may be made by its manufacturer, is not guaranteed or endorsed by the publisher.
1. Rock KL, Reits E, Neefjes J. Present Yourself! By MHC Class I and MHC Class II Molecules. Trends Immunol (2016) 37(11):724–37. doi: 10.1016/j.it.2016.08.010
2. Haen SP, Löffler MW, Rammensee HG, Brossart P. Towards New Horizons: Characterization, Classification and Implications of the Tumour Antigenic Repertoire. Nat Rev Clin Oncol (2020) 17(10):595–610. doi: 10.1038/s41571-020-0387-x
3. Yarchoan M, Johnson BA 3rd, Lutz ER, Laheru DA, Jaffee EM. Targeting Neoantigens to Augment Antitumour Immunity. Nat Rev Cancer (2017) 17(4):209–22. doi: 10.1038/nrc.2016.154
4. Türeci Ö, Vormehr M, Diken M, Kreiter S, Huber C, Sahin U. Targeting the Heterogeneity of Cancer With Individualized Neoepitope Vaccines. Clin Cancer Res (2016) 22(8):1885–96. doi: 10.1158/1078-0432.CCR-15-1509
5. Bassani-Sternberg M, Bräunlein E, Klar R, Engleitner T, Sinitcyn P, Audehm S, et al. Direct Identification of Clinically Relevant Neoepitopes Presented on Native Human Melanoma Tissue by Mass Spectrometry. Nat Commun (2016) 7:13404. doi: 10.1038/ncomms13404
6. Clark RE, Dodi IA, Hill SC, Lill JR, Aubert G, Macintyre AR, et al. Direct Evidence That Leukemic Cells Present HLA-Associated Immunogenic Peptides Derived From the BCR-ABL B3a2 Fusion Protein. Blood (2001) 98(10):2887–93. doi: 10.1182/blood.V98.10.2887
7. Linnebacher M, Gebert J, Rudy W, Wörner S, Yuan YP, Bork P, et al. Frameshift Peptide-Derived T-Cell Epitopes: A Source of Novel Tumor-Specific Antigens. Int J Cancer (2001) 93(1):6–11. doi: 10.1002/ijc.1298
8. Narayan R, Olsson N, Wagar LE, Medeiros BC, Meyer E, Czerwinski D, et al. Acute Myeloid Leukemia Immunopeptidome Reveals HLA Presentation of Mutated Nucleophosmin. PloS One (2019) 14(7):e0219547. doi: 10.1371/journal.pone.0219547
9. van der Lee DI, Reijmers RM, Honders MW, Hagedoorn RS, de Jong RC, Kester MG, et al. Mutated Nucleophosmin 1 as Immunotherapy Target in Acute Myeloid Leukemia. J Clin Invest (2019) 129(2):774–85. doi: 10.1172/JCI97482
10. Smart AC, Margolis CA, Pimentel H, He MX, Miao D, Adeegbe D, et al. Intron Retention Is a Source of Neoepitopes in Cancer. Nat Biotechnol (2018) 36(11):1056–8. doi: 10.1038/nbt.4239
11. Lu SX, De Neef E, Thomas JD, Sabio E, Rousseau B, Gigoux M, et al. Pharmacologic Modulation of RNA Splicing Enhances Anti-Tumor Immunity. Cell (2021) 184(15):4032–47.e31. doi: 10.1016/j.cell.2021.05.038
12. Zhang M, Fritsche J, Roszik J, Williams LJ, Peng X, Chiu Y, et al. RNA Editing Derived Epitopes Function as Cancer Antigens to Elicit Immune Responses. Nat Commun (2018) 9(1):3919. doi: 10.1038/s41467-018-06405-9
13. Bartok O, Pataskar A, Nagel R, Laos M, Goldfarb E, Hayoun D, et al. Anti-Tumour Immunity Induces Aberrant Peptide Presentation in Melanoma. Nature (2020) 590(7845):332–7. doi: 10.1038/s41586-020-03054-1
14. Laumont CM, Daouda T, Laverdure JP, Bonneil E, Caron-Lizotte O, Hardy MP, et al. Global Proteogenomic Analysis of Human MHC Class I-Associated Peptides Derived From Non-Canonical Reading Frames. Nat Commun (2016) 7:10238. doi: 10.1038/ncomms10238
15. Chong C, Müller M, Pak H, Harnett D, Huber F, Grun D, et al. Integrated Proteogenomic Deep Sequencing and Analytics Accurately Identify non-Canonical Peptides in Tumor Immunopeptidomes. Nat Commun (2020) 11(1):1293. doi: 10.1038/s41467-020-14968-9
16. Vigneron N, Stroobant V, Chapiro J, Ooms A, Degiovanni G, Morel S, et al. An Antigenic Peptide Produced by Peptide Splicing in the Proteasome. Science (New York NY) (2004) 304(5670):587–90. doi: 10.1126/science.1095522
17. Liepe J, Marino F, Sidney J, Jeko A, Bunting DE, Sette A, et al. A Large Fraction of HLA Class I Ligands are Proteasome-Generated Spliced Peptides. Science (New York NY) (2016) 354(6310):354–8. doi: 10.1126/science.aaf4384
18. Zarling AL, Ficarro SB, White FM, Shabanowitz J, Hunt DF, Engelhard VH. Phosphorylated Peptides are Naturally Processed and Presented by Major Histocompatibility Complex Class I Molecules In Vivo. J Exp Med (2000) 192(12):1755–62. doi: 10.1084/jem.192.12.1755
19. Malaker SA, Penny SA, Steadman LG, Myers PT, Loke JC, Raghavan M, et al. Identification of Glycopeptides as Posttranslationally Modified Neoantigens in Leukemia. Cancer Immunol Res (2017) 5(5):376–84. doi: 10.1158/2326-6066.Cir-16-0280
20. Riemer AB, Keskin DB, Zhang G, Handley M, Anderson KS, Brusic V, et al. A Conserved E7-Derived Cytotoxic T Lymphocyte Epitope Expressed on Human Papillomavirus 16-Transformed HLA-A2+ Epithelial Cancers. J Biol Chem (2010) 285(38):29608–22. doi: 10.1074/jbc.M110.126722
21. Blatnik R, Mohan N, Bonsack M, Falkenby LG, Hoppe S, Josef K, et al. A Targeted LC-MS Strategy for Low-Abundant HLA Class-I-Presented Peptide Detection Identifies Novel Human Papillomavirus T-Cell Epitopes. Proteomics (2018) 18(11):e1700390. doi: 10.1002/pmic.201700390
22. Kalaora S, Nagler A, Nejman D, Alon M, Barbolin C, Barnea E, et al. Identification of Bacteria-Derived HLA-Bound Peptides in Melanoma. Nature (2021) 592(7852):138–43. doi: 10.1038/s41586-021-03368-8
23. Dersh D, Holly J, Yewdell JW. A Few Good Peptides: MHC Class I-Based Cancer Immunosurveillance and Immunoevasion. Nat Rev Immunol (2020) 21(2):116–28. doi: 10.1038/s41577-020-0390-6
24. Laumont CM, Vincent K, Hesnard L, Audemard E, Bonneil E, Laverdure JP, et al. Noncoding Regions are the Main Source of Targetable Tumor-Specific Antigens. Sci Trans Med (2018) 10(470):eaau5516. doi: 10.1126/scitranslmed.aau5516
25. Erhard F, Dolken L, Schilling B, Schlosser A. Identification of the Cryptic HLA-I Immunopeptidome. Cancer Immunol Res (2020) 8(8):1018–26. doi: 10.1158/2326-6066.CIR-19-0886
26. Roudko V, Greenbaum B, Bhardwaj N. Computational Prediction and Validation of Tumor-Associated Neoantigens. Front Immunol (2020) 11:27. doi: 10.3389/fimmu.2020.00027
27. Ruiz Cuevas MV, Hardy MP, Holly J, Bonneil E, Durette C, Courcelles M, et al. Most non-Canonical Proteins Uniquely Populate the Proteome or Immunopeptidome. Cell Rep (2021) 34(10):108815. doi: 10.1016/j.celrep.2021.108815
28. Morel S, Lévy F, Burlet-Schiltz O, Brasseur F, Probst-Kepper M, Peitrequin AL, et al. Processing of Some Antigens by the Standard Proteasome But Not by the Immunoproteasome Results in Poor Presentation by Dendritic Cells. Immunity (2000) 12(1):107–17. doi: 10.1016/s1074-7613(00)80163-6
29. Sijts AJ, Ruppert T, Rehermann B, Schmidt M, Koszinowski U, Kloetzel PM. Efficient Generation of a Hepatitis B Virus Cytotoxic T Lymphocyte Epitope Requires the Structural Features of Immunoproteasomes. J Exp Med (2000) 191(3):503–14. doi: 10.1084/jem.191.3.503
30. Keşmir C, Nussbaum AK, Schild H, Detours V, Brunak S. Prediction of Proteasome Cleavage Motifs by Neural Networks. Protein Eng (2002) 15(4):287–96. doi: 10.1093/protein/15.4.287
31. Tenzer S, Peters B, Bulik S, Schoor O, Lemmel C, Schatz MM, et al. Modeling the MHC Class I Pathway by Combining Predictions of Proteasomal Cleavage, TAP Transport and MHC Class I Binding. Cell Mol Life Sci (2005) 62(9):1025–37. doi: 10.1007/s00018-005-4528-2
32. Calis JJ, Reinink P, Keller C, Kloetzel PM, Kesmir C. Role of Peptide Processing Predictions in T Cell Epitope Identification: Contribution of Different Prediction Programs. Immunogenetics (2015) 67(2):85–93. doi: 10.1007/s00251-014-0815-0
33. Peters B, Bulik S, Tampe R, Van Endert PM, Holzhutter HG. Identifying MHC Class I Epitopes by Predicting the TAP Transport Efficiency of Epitope Precursors. J Immunol (2003) 171(4):1741–9. doi: 10.4049/jimmunol.171.4.1741
34. Bhasin M, Raghava GP. Analysis and Prediction of Affinity of TAP Binding Peptides Using Cascade SVM. Protein Sci (2004) 13(3):596–607. doi: 10.1110/ps.03373104
35. Falk K, Rötzschke O, Stevanovié S, Jung G, Rammensee H-G. Allele-Specific Motifs Revealed by Sequencing of Self-Peptides Eluted From MHC Molecules. Nature (1991) 351(6324):290–6. doi: 10.1038/351290a0
36. Andreatta M, Alvarez B, Nielsen M. GibbsCluster: Unsupervised Clustering and Alignment of Peptide Sequences. Nucleic Acids Res (2017) 45(W1):W458–w63. doi: 10.1093/nar/gkx248
37. Racle J, Michaux J, Rockinger GA, Arnaud M, Bobisse S, Chong C, et al. Robust Prediction of HLA Class II Epitopes by Deep Motif Deconvolution of Immunopeptidomes. Nat Biotechnol (2019) 37(11):83–6. doi: 10.1038/s41587-019-0289-6
38. Rammensee H, Bachmann J, Emmerich NP, Bachor OA, Stevanovic S. SYFPEITHI: Database for MHC Ligands and Peptide Motifs. Immunogenetics (1999) 50(3-4):213–9. doi: 10.1007/s002510050595
39. Nielsen M, Andreatta M. NetMHCpan-3.0; Improved Prediction of Binding to MHC Class I Molecules Integrating Information From Multiple Receptor and Peptide Length Datasets. Genome Med (2016) 8(1):33. doi: 10.1186/s13073-016-0288-x
40. Shao XM, Bhattacharya R, Huang J, Sivakumar IKA, Tokheim C, Zheng L, et al. High-Throughput Prediction of MHC Class I and II Neoantigens With MHCnuggets. Cancer Immunol Res (2020) 8(3):396–408. doi: 10.1158/2326-6066.CIR-19-0464
41. Jurtz V, Paul S, Andreatta M, Marcatili P, Peters B, Nielsen M. NetMHCpan-4.0: Improved Peptide-MHC Class I Interaction Predictions Integrating Eluted Ligand and Peptide Binding Affinity Data. J Immunol (2017) 199(9):3360–8. doi: 10.4049/jimmunol.1700893
42. Reynisson B, Alvarez B, Paul S, Peters B, Nielsen M. NetMHCpan-4.1 and NetMHCIIpan-4.0: Improved Predictions of MHC Antigen Presentation by Concurrent Motif Deconvolution and Integration of MS MHC Eluted Ligand Data. Nucleic Acids Res (2020) 48(W1):W449–54. doi: 10.1093/nar/gkaa379
43. Andreatta M, Nielsen M. Gapped Sequence Alignment Using Artificial Neural Networks: Application to the MHC Class I System. Bioinformatics (Oxford England) (2016) 32(4):511–7. doi: 10.1093/bioinformatics/btv639
44. Schmidt J, Guillaume P, Dojcinovic D, Karbach J, Coukos G, Luescher I. In Silico and Cell-Based Analyses Reveal Strong Divergence Between Prediction and Observation of T-Cell-Recognized Tumor Antigen T-Cell Epitopes. J Biol Chem (2017) 292(28):11840–9. doi: 10.1074/jbc.M117.789511
45. Löffler MW, Mohr C, Bichmann L, Freudenmann LK, Walzer M, Schroeder CM, et al. Multi-Omics Discovery of Exome-Derived Neoantigens in Hepatocellular Carcinoma. Genome Med (2019) 11(1):28. doi: 10.1186/s13073-019-0636-8
46. Bonsack M, Hoppe S, Winter J, Tichy D, Zeller C, Kupper MD, et al. Performance Evaluation of MHC Class-I Binding Prediction Tools Based on an Experimentally Validated MHC-Peptide Binding Data Set. Cancer Immunol Res (2019) 7(5):719–36. doi: 10.1158/2326-6066.CIR-18-0584
47. Becker JP, Helm D, Rettel M, Stein F, Hernandez-Sanchez A, Urban K, et al. NMD Inhibition by 5-Azacytidine Augments Presentation of Immunogenic Frameshift-Derived Neoepitopes. iScience (2021) 24(4):102389. doi: 10.1016/j.isci.2021.102389
48. Wells DK, van Buuren MM, Dang KK, Hubbard-Lucey VM, Sheehan KCF, Campbell KM, et al. Key Parameters of Tumor Epitope Immunogenicity Revealed Through a Consortium Approach Improve Neoantigen Prediction. Cell (2020) 183(3):818–34.e13. doi: 10.1016/j.cell.2020.09.015
49. Schmidt J, Smith AR, Magnin M, Racle J, Devlin JR, Bobisse S, et al. Prediction of Neo-Epitope Immunogenicity Reveals TCR Recognition Determinants and Provides Insight Into Immunoediting. Cell Rep Med (2021) 2(2):100194. doi: 10.1016/j.xcrm.2021.100194
50. Pierce BG, Weng Z. A Flexible Docking Approach for Prediction of T Cell Receptor–Peptide–MHC Complexes. Protein Sci (2013) 22(1):35–46. doi: 10.1002/pro.2181
51. Riley TP, Keller GLJ, Smith AR, Davancaze LM, Arbuiso AG, Devlin JR, et al. Structure Based Prediction of Neoantigen Immunogenicity. Front Immunol (2019) 10:2047. doi: 10.3389/fimmu.2019.02047
52. Coelho A, Fonseca AL, Martins DL, Lins PBR, da Cunha LM, de Souza SJ. neoANT-HILL: An Integrated Tool for Identification of Potential Neoantigens. BMC Med Genomics (2020) 13(1):30. doi: 10.1186/s12920-020-0694-1
53. Hundal J, Kiwala S, McMichael J, Miller CA, Xia H, Wollam AT, et al. Pvactools: A Computational Toolkit to Identify and Visualize Cancer Neoantigens. Cancer Immunol Res (2020) 8(3):409–20. doi: 10.1158/2326-6066.CIR-19-0401
54. Gopanenko AV, Kosobokova EN, Kosorukov VS. Main Strategies for the Identification of Neoantigens. Cancers (2020) 12(10):2879. doi: 10.3390/cancers12102879
55. Rötzschke O, Falk K, Deres K, Schild H, Norda M, Metzger J, et al. Isolation and Analysis of Naturally Processed Viral Peptides as Recognized by Cytotoxic T Cells. Nature (1990) 348(6298):252–4. doi: 10.1038/348252a0
56. Hunt DF, Henderson RA, Shabanowitz J, Sakaguchi K, Michel H, Sevilir N, et al. Characterization of Peptides Bound to the Class I MHC Molecule HLA-A2.1 by Mass Spectrometry. Science (New York NY) (1992) 255(5049):1261–3. doi: 10.1126/science.1546328
57. van Rooij N, van Buuren MM, Philips D, Velds A, Toebes M, Heemskerk B, et al. Tumor Exome Analysis Reveals Neoantigen-Specific T-Cell Reactivity in an Ipilimumab-Responsive Melanoma. J Clin Oncol (2013) 31(32):e439–e42. doi: 10.1200/JCO.2012.47.7521
58. Gubin MM, Zhang X, Schuster H, Caron E, Ward JP, Noguchi T, et al. Checkpoint Blockade Cancer Immunotherapy Targets Tumour-Specific Mutant Antigens. Nature (2014) 515(7528):577–81. doi: 10.1038/nature13988
59. Linnemann C, van Buuren MM, Bies L, Verdegaal EM, Schotte R, Calis JJ, et al. High-Throughput Epitope Discovery Reveals Frequent Recognition of Neo-Antigens by CD4+ T Cells in Human Melanoma. Nat Med (2015) 21(1):81–5. doi: 10.1038/nm.3773
60. Kuznetsov A, Voronina A, Govorun V, Arapidi G. Critical Review of Existing MHC I Immunopeptidome Isolation Methods. Molecules (2020) 25(22):5409. doi: 10.3390/molecules25225409
61. Lanoix J, Durette C, Courcelles M, Cossette É, Comtois-Marotte S, Hardy M-P, et al. Comparison of the MHC I Immunopeptidome Repertoire of B-Cell Lymphoblasts Using Two Isolation Methods. Proteomics (2018) 18(12):1700251. doi: 10.1002/pmic.201700251
62. Caron E, Espona L, Kowalewski DJ, Schuster H, Ternette N, Alpizar A, et al. An Open-Source Computational and Data Resource to Analyze Digital Maps of Immunopeptidomes. Elife (2015) 4:e07661. doi: 10.7554/eLife.07661
63. Ritz D, Kinzi J, Neri D, Fugmann T. Data-Independent Acquisition of HLA Class I Peptidomes on the Q Exactive Mass Spectrometer Platform. Proteomics (2017) 17(19):10.1002/pmic.201700177. doi: 10.1002/pmic.201700177
64. Pak H, Michaux J, Huber F, Chong C, Stevenson BJ, Müller M, et al. Sensitive Immunopeptidomics by Leveraging Available Large-Scale Multi-HLA Spectral Libraries, Data-Independent Acquisition and MS/MS Prediction. Mol Cell Proteomics (2021) 20:100080. doi: 10.1016/j.mcpro.2021.100080
65. Tsou C-C, Avtonomov D, Larsen B, Tucholska M, Choi H, Gingras A-C, et al. DIA-Umpire: Comprehensive Computational Framework for Data-Independent Acquisition Proteomics. Nat Methods (2015) 12(3):258–64. doi: 10.1038/nmeth.3255
66. Tran NH, Qiao R, Xin L, Chen X, Liu C, Zhang X, et al. Deep Learning Enables De Novo Peptide Sequencing From Data-Independent-Acquisition Mass Spectrometry. Nat Methods (2019) 16(1):63–6. doi: 10.1038/s41592-018-0260-3
67. Demichev V, Messner CB, Vernardis SI, Lilley KS, Ralser M. DIA-NN: Neural Networks and Interference Correction Enable Deep Proteome Coverage in High Throughput. Nat Methods (2020) 17(1):41–4. doi: 10.1038/s41592-019-0638-x
68. Tiwary S, Levy R, Gutenbrunner P, Salinas Soto F, Palaniappan KK, Deming L, et al. High-Quality MS/MS Spectrum Prediction for Data-Dependent and Data-Independent Acquisition Data Analysis. Nat Methods (2019) 16(6):519–25. doi: 10.1038/s41592-019-0427-6
69. Declercq A, Bouwmeester R, Degroeve S, Martens L, Gabriels R. MS2Rescore: Data-Driven Rescoring Dramatically Boosts Immunopeptide Identification Rates. bioRxiv (2021). doi: 10.1101/2021.11.02.466886
70. Wilhelm M, Zolg DP, Graber M, Gessulat S, Schmidt T, Schnatbaum K, et al. Deep Learning Boosts Sensitivity of Mass Spectrometry-Based Immunopeptidomics. Nat Commun (2021) 12(1):3346. doi: 10.1038/s41467-021-23713-9
71. Abelin JG, Keskin DB, Sarkizova S, Hartigan CR, Zhang W, Sidney J, et al. Mass Spectrometry Profiling of HLA-Associated Peptidomes in Mono-Allelic Cells Enables More Accurate Epitope Prediction. Immunity (2017) 46(2):315–26. doi: 10.1016/j.immuni.2017.02.007
72. Finton KAK, Brusniak MY, Jones LA, Lin C, Fiore-Gartland AJ, Brock C, et al. ARTEMIS: A Novel Mass-Spec Platform for HLA-Restricted Self and Disease-Associated Peptide Discovery. Front Immunol (2021) 12:658372. doi: 10.3389/fimmu.2021.658372
73. Hickman HD, Batson CL, Prilliman KR, Crawford DL, Jackson KL, Hildebrand WH. C-Terminal Epitope Tagging Facilitates Comparative Ligand Mapping From MHC Class I Positive Cells. Hum Immunol (2000) 61(12):1339–46. doi: 10.1016/s0198-8859(00)00216-0
74. Nicastri A, Liao H, Muller J, Purcell AW, Ternette N. The Choice of HLA-Associated Peptide Enrichment and Purification Strategy Affects Peptide Yields and Creates a Bias in Detected Sequence Repertoire. Proteomics (2020) 20(12):e1900401. doi: 10.1002/pmic.201900401
75. Hassan C, Kester MG, Oudgenoeg G, de Ru AH, Janssen GM, Drijfhout JW, et al. Accurate Quantitation of MHC-Bound Peptides by Application of Isotopically Labeled Peptide MHC Complexes. J Proteomics (2014) 109:240–4. doi: 10.1016/j.jprot.2014.07.009
76. Stopfer LE, D'Souza AD, White FM. 1,2,3, MHC: A Review of Mass-Spectrometry-Based Immunopeptidomics Methods for Relative and Absolute Quantification of pMHCs. Immuno-Oncol Technol (2021) 11:100042. doi: 10.1016/j.iotech.2021.100042
77. Chong C, Marino F, Pak H, Racle J, Daniel RT, Müller M, et al. High-Throughput and Sensitive Immunopeptidomics Platform Reveals Profound Interferonγ-Mediated Remodeling of the Human Leukocyte Antigen (HLA) Ligandome. Mol Cell Proteomics (2018) 17(3):533–48. doi: 10.1074/mcp.TIR117.000383
78. Pollock SB, Rose CM, Darwish M, Bouziat R, Delamarre L, Blanchette C, et al. Sensitive and Quantitative Detection of MHC-I Displayed Neoepitopes Using a Semiautomated Workflow and TOMAHAQ Mass Spectrometry. Mol Cell Proteomics (2021) 20:100108. doi: 10.1016/j.mcpro.2021.100108
79. Feola S, Haapala M, Peltonen K, Capasso C, Martins B, Antignani G, et al. PeptiCHIP: A Microfluidic Platform for Tumor Antigen Landscape Identification. ACS Nano (2021) 15(10):15992–6010. doi: 10.1021/acsnano.1c04371
80. Nesvizhskii AI. Proteogenomics: Concepts, Applications and Computational Strategies. Nat Methods (2014) 11(11):1114–25. doi: 10.1038/nmeth.3144
81. Elias JE, Gygi SP. Target-Decoy Search Strategy for Mass Spectrometry-Based Proteomics. Methods Mol Biol (2010) 604:55–71. doi: 10.1007/978-1-60761-444-9_5
82. Zhang J, Xin L, Shan B, Chen W, Xie M, Yuen D, et al. PEAKS DB: De Novo Sequencing Assisted Database Search for Sensitive and Accurate Peptide Identification. Mol Cell Proteomics (2012) 11(4):M111 010587. doi: 10.1074/mcp.M111.010587
83. Sinitcyn P, Rudolph JD, Cox J. Computational Methods for Understanding Mass Spectrometry–Based Shotgun Proteomics Data. Annu Rev BioMed Data Sci (2018) 1(1):207–34. doi: 10.1146/annurev-biodatasci-080917-013516
84. Andreatta M, Nicastri A, Peng X, Hancock G, Dorrell L, Ternette N, et al. MS-Rescue: A Computational Pipeline to Increase the Quality and Yield of Immunopeptidomics Experiments. Proteomics (2019) 19(4):e1800357. doi: 10.1002/pmic.201800357
85. Li K, Jain A, Malovannaya A, Wen B, Zhang B. DeepRescore: Leveraging Deep Learning to Improve Peptide Identification in Immunopeptidomics. Proteomics (2020) 20(21-22):e1900334. doi: 10.1002/pmic.201900334
86. Murphy JP, Konda P, Kowalewski DJ, Schuster H, Clements D, Kim Y, et al. MHC-I Ligand Discovery Using Targeted Database Searches of Mass Spectrometry Data: Implications for T-Cell Immunotherapies. J Proteome Res (2017) 16(4):1806–16. doi: 10.1021/acs.jproteome.6b00971
87. Zhang L, Liu G, Hou G, Xiang H, Zhang X, Huang Y, et al. IntroSpect: Motif-Guided Immunopeptidome Database Building Tool to Improve the Sensitivity of HLA Binding Peptide Identification. bioRxiv (2021). doi: 10.1101/2021.08.02.454768
88. Krokhin OV. Sequence-Specific Retention Calculator. Algorithm for Peptide Retention Prediction in Ion-Pair RP-HPLC: Application to 300- and 100-Å Pore Size C18 Sorbents. Anal Chem (2006) 78(22):7785–95. doi: 10.1021/ac060777w
89. Bouwmeester R, Gabriels R, Hulstaert N, Martens L, Degroeve S. DeepLC can Predict Retention Times for Peptides That Carry as-Yet Unseen Modifications. Nat Methods (2021) 18(11):1363–9. doi: 10.1038/s41592-021-01301-5
90. Toprak UH, Gillet LC, Maiolica A, Navarro P, Leitner A, Aebersold R. Conserved Peptide Fragmentation as a Benchmarking Tool for Mass Spectrometers and a Discriminating Feature for Targeted Proteomics. Mol Cell Proteomics (2014) 13(8):2056–71. doi: 10.1074/mcp.O113.036475
91. Ghosh M, Gauger M, Marcu A, Nelde A, Denk M, Schuster H, et al. Guidance Document: Validation of a High-Performance Liquid Chromatography-Tandem Mass Spectrometry Immunopeptidomics Assay for the Identification of HLA Class I Ligands Suitable for Pharmaceutical Therapies. Mol Cell Proteomics (2020) 19(3):432–43. doi: 10.1074/mcp.C119.001652
92. Pearson H, Daouda T, Granados DP, Durette C, Bonneil E, Courcelles M, et al. MHC Class I-Associated Peptides Derive From Selective Regions of the Human Genome. J Clin Invest (2016) 126(12):4690–701. doi: 10.1172/JCI88590
93. Müller M, Gfeller D, Coukos G, Bassani-Sternberg M. 'Hotspots' of Antigen Presentation Revealed by Human Leukocyte Antigen Ligandomics for Neoantigen Prioritization. Front Immunol (2017) 8:1367. doi: 10.3389/fimmu.2017.01367
94. Faridi P, Purcell AW, Croft NP. In Immunopeptidomics We Need a Sniper Instead of a Shotgun. Proteomics (2018) 18(12):e1700464. doi: 10.1002/pmic.201700464
95. Joyce S, Ternette N. Know Thy Immune Self and non-Self: Proteomics Informs on the Expanse of Self and non-Self, and How and Where They Arise. Proteomics (2021) 21(23–24):e2000143. doi: 10.1002/pmic.202000143
96. Freudenmann LK, Marcu A, Stevanovic S. Mapping the Tumour Human Leukocyte Antigen (HLA) Ligandome by Mass Spectrometry. Immunology (2018) 154(3):331–45. doi: 10.1111/imm.12936
97. Christinck ER, Luscher MA, Barber BH, Williams DB. Peptide Binding to Class I MHC on Living Cells and Quantitation of Complexes Required for CTL Lysis. Nature (1991) 352(6330):67–70. doi: 10.1038/352067a0
98. Foote J, Eisen HN. Breaking the Affinity Ceiling for Antibodies and T Cell Receptors. Proc Natl Acad Sci U S A (2000) 97(20):10679–81. doi: 10.1073/pnas.97.20.10679
99. Purbhoo MA, Irvine DJ, Huppa JB, Davis MM. T Cell Killing Does Not Require the Formation of a Stable Mature Immunological Synapse. Nat Immunol (2004) 5(5):524–30. doi: 10.1038/ni1058
100. Bigot J, Lalanne AI, Lucibello F, Gueguen P, Houy A, Dayot S, et al. Splicing Patterns in SF3B1-Mutated Uveal Melanoma Generate Shared Immunogenic Tumor-Specific Neoepitopes. Cancer Discov (2021) 11(8):1938–51. doi: 10.1158/2159-8290.CD-20-0555
101. Weinzierl AO, Lemmel C, Schoor O, Müller M, Krüger T, Wernet D, et al. Distorted Relation Between mRNA Copy Number and Corresponding Major Histocompatibility Complex Ligand Density on the Cell Surface. Mol Cell Proteomics (2007) 6(1):102–13. doi: 10.1074/mcp.M600310-MCP200
102. Caron E, Vincent K, Fortier MH, Laverdure JP, Bramoulle A, Hardy MP, et al. The MHC I Immunopeptidome Conveys to the Cell Surface an Integrative View of Cellular Regulation. Mol Syst Biol (2011) 7:533. doi: 10.1038/msb.2011.68
103. Yewdell JW, Antón LC, Bennink JR. Defective Ribosomal Products (DRiPs): A Major Source of Antigenic Peptides for MHC Class I Molecules? J Immunol (1996) 157(5):1823–6.
104. Apcher S, Daskalogianni C, Lejeune F, Manoury B, Imhoos G, Heslop L, et al. Major Source of Antigenic Peptides for the MHC Class I Pathway is Produced During the Pioneer Round of mRNA Translation. Proc Natl Acad Sci U S A (2011) 108(28):11572–7. doi: 10.1073/pnas.1104104108
105. Rock KL, Farfán-Arribas DJ, Colbert JD, Goldberg AL. Re-Examining Class-I Presentation and the DRiP Hypothesis. Trends Immunol (2014) 35(4):144–52. doi: 10.1016/j.it.2014.01.002
106. Lee PP. T-Cell Responses to Cancer. Methods Cell Biol (2004) 75:513–32. doi: 10.1016/s0091-679x(04)75021-9
107. Slota M, Lim J-B, Dang Y, Disis ML. ELISpot for Measuring Human Immune Responses to Vaccines. Expert Rev Vaccines (2011) 10(3):299–306. doi: 10.1586/erv.10.169
108. Hadrup SR, Bakker AH, Shu CJ, Andersen RS, van Veluw J, Hombrink P, et al. Parallel Detection of Antigen-Specific T-Cell Responses by Multidimensional Encoding of MHC Multimers. Nat Methods (2009) 6(7):520–6. doi: 10.1038/nmeth.1345
109. Peng S, Zaretsky JM, Ng AHC, Chour W, Bethune MT, Choi J, et al. Sensitive Detection and Analysis of Neoantigen-Specific T Cell Populations From Tumors and Blood. Cell Rep (2019) 28(10):2728–38.e7. doi: 10.1016/j.celrep.2019.07.106
110. Danilova L, Anagnostou V, Caushi JX, Sidhom JW, Guo H, Chan HY, et al. The Mutation-Associated Neoantigen Functional Expansion of Specific T Cells (MANAFEST) Assay: A Sensitive Platform for Monitoring Antitumor Immunity. Cancer Immunol Res (2018) 6(8):888–99. doi: 10.1158/2326-6066.CIR-18-0129
111. Arnaud M, Chiffelle J, Genolet R, Navarro Rodrigo B, Perez MAS, Huber F, et al. Sensitive Identification of Neoantigens and Cognate TCRs in Human Solid Tumors. Nat Biotechnol (2021). doi: 10.1038/s41587-021-01072-6
112. McGranahan N, Furness AJ, Rosenthal R, Ramskov S, Lyngaa R, Saini SK, et al. Clonal Neoantigens Elicit T Cell Immunoreactivity and Sensitivity to Immune Checkpoint Blockade. Science (New York NY) (2016) 351(6280):1463–9. doi: 10.1126/science.aaf1490
113. Verdegaal EM, de Miranda NF, Visser M, Harryvan T, van Buuren MM, Andersen RS, et al. Neoantigen Landscape Dynamics During Human Melanoma-T Cell Interactions. Nature (2016) 536(7614):91–5. doi: 10.1038/nature18945
114. Gejman RS, Chang AY, Jones HF, DiKun K, Hakimi AA, Schietinger A, et al. Rejection of Immunogenic Tumor Clones is Limited by Clonal Fraction. Elife (2018) 7:e41090. doi: 10.7554/eLife.41090
115. Morgan RA, Chinnasamy N, Abate-Daga D, Gros A, Robbins PF, Zheng Z, et al. Cancer Regression and Neurological Toxicity Following Anti-MAGE-A3 TCR Gene Therapy. J Immunother (2013) 36(2):133–51. doi: 10.1097/CJI.0b013e3182829903
116. Duan F, Duitama J, Al Seesi S, Ayres CM, Corcelli SA, Pawashe AP, et al. Genomic and Bioinformatic Profiling of Mutational Neoepitopes Reveals New Rules to Predict Anticancer Immunogenicity. J Exp Med (2014) 211(11):2231–48. doi: 10.1084/jem.20141308
117. Marcu A, Bichmann L, Kuchenbecker L, Kowalewski DJ, Freudenmann LK, Backert L, et al. HLA Ligand Atlas: A Benign Reference of HLA-Presented Peptides to Improve T-Cell-Based Cancer Immunotherapy. J Immunother Cancer (2021) 9(4):e002071. doi: 10.1136/jitc-2020-002071
118. Singh-Jasuja H, Emmerich NPN, Rammensee H-G. The Tübingen Approach: Identification, Selection, and Validation of Tumor-Associated HLA Peptides for Cancer Therapy. Cancer Immunol Immunother (2004) 53(3):187–95. doi: 10.1007/s00262-003-0480-x
119. Walter S, Weinschenk T, Stenzl A, Zdrojowy R, Pluzanska A, Szczylik C, et al. Multipeptide Immune Response to Cancer Vaccine IMA901 After Single-Dose Cyclophosphamide Associates With Longer Patient Survival. Nat Med (2012) 18(8):1254–61. doi: 10.1038/nm.2883
120. Carreno BM, Magrini V, Becker-Hapak M, Kaabinejadian S, Hundal J, Petti AA, et al. Cancer Immunotherapy. A Dendritic Cell Vaccine Increases the Breadth and Diversity of Melanoma Neoantigen-Specific T Cells. Science (New York NY) (2015) 348(6236):803–8. doi: 10.1126/science.aaa3828
121. Nelde A, Maringer Y, Bilich T, Salih HR, Rörden M, Heitmann JS, et al. Immunopeptidomics-Guided Warehouse Design for Peptide-Based Immunotherapy in Chronic Lymphocytic Leukemia. Front Immunol (2021) 12:705974. doi: 10.3389/fimmu.2021.705974
122. Hilf N, Kuttruff-Coqui S, Frenzel K, Bukur V, Stevanovic S, Gouttefangeas C, et al. Actively Personalized Vaccination Trial for Newly Diagnosed Glioblastoma. Nature (2019) 565(7738):240–5. doi: 10.1038/s41586-018-0810-y
123. Kloor M, Reuschenbach M, Pauligk C, Karbach J, Rafiyan M-R, Al-Batran S-E, et al. A Frameshift Peptide Neoantigen-Based Vaccine for Mismatch Repair-Deficient Cancers: A Phase I/IIa Clinical Trial. Clin Cancer Res (2020) 26(17):4503–10. doi: 10.1158/1078-0432.Ccr-19-3517
124. Chong C, Coukos G, Bassani-Sternberg M. Identification of Tumor Antigens With Immunopeptidomics. Nat Biotechnol (2022) 40(2):175–88. doi: 10.1038/s41587-021-01038-8
Keywords: immunopeptidomics, T cell epitope, tumor antigen, HLA class I, neoantigen, cancer immunotherapy
Citation: Becker JP and Riemer AB (2022) The Importance of Being Presented: Target Validation by Immunopeptidomics for Epitope-Specific Immunotherapies. Front. Immunol. 13:883989. doi: 10.3389/fimmu.2022.883989
Received: 25 February 2022; Accepted: 16 March 2022;
Published: 06 April 2022.
Edited by:
Catherine Sautes-Fridman, U1138 Centre de Recherche des Cordeliers (CRC) (INSERM), FranceReviewed by:
Arie Admon, Technion Israel Institute of Technology, IsraelCopyright © 2022 Becker and Riemer. This is an open-access article distributed under the terms of the Creative Commons Attribution License (CC BY). The use, distribution or reproduction in other forums is permitted, provided the original author(s) and the copyright owner(s) are credited and that the original publication in this journal is cited, in accordance with accepted academic practice. No use, distribution or reproduction is permitted which does not comply with these terms.
*Correspondence: Angelika B. Riemer, YS5yaWVtZXJAZGtmei5kZQ==
Disclaimer: All claims expressed in this article are solely those of the authors and do not necessarily represent those of their affiliated organizations, or those of the publisher, the editors and the reviewers. Any product that may be evaluated in this article or claim that may be made by its manufacturer is not guaranteed or endorsed by the publisher.
Research integrity at Frontiers
Learn more about the work of our research integrity team to safeguard the quality of each article we publish.