- 1Department of Internal Medicine and Hematology, Laboratory of Flow Cytometry, Military Institute of Medicine, Warsaw, Poland
- 2Department of Internal Medicine, Pulmonology, Allergology and Clinical Immunology, Military Institute of Medicine, Warsaw, Poland
- 3Department of Internal Medicine and Hematology, Military Institute of Medicine, Warsaw, Poland
- 4Department of Internal Medicine, Pulmonary Diseases and Allergy, Medical University of Warsaw, Warsaw, Poland
Lymphocytes play a leading role in regulation of the immune system in lung cancer patients. The recognition of T cells profile may help in prediction of effectiveness of anticancer immunotherapy. The aim of the study was to determine the dominant subpopulation of CD4+ and CD8+ lymphocytes in metastatic and non-metastatic lymph nodes (LNs) of lung cancer patients. LNs aspirates were obtained during EBUS/TBNA procedure and cells were analyzed by flow cytometry. We showed a higher percentage of CD4+ and CD8+ effector memory T cells in the metastatic than in the non-metastatic LNs (28.6 vs. 15.3% and 28.6 vs. 14.0%, p< 0.05). The proportion of CD45RO+ T regulatory cells (CD45RO+ Tregs) was higher in the metastatic LNs than in the non-metastatic ones (65.6 vs. 31%, p< 0.05). We reported the significant differences in T cell subsets depending on the lung cancer metastatic process. We observed that the effector memory T cells were predominant subpopulations in metastatic LNs. Lymphocyte profile in LNs is easy to evaluate by flow cytometry of EBUS/TBNA samples and may reflect the immune status in lung cancer.
Introduction
Lung Cancer
Lung cancer is the most common cause of cancer death in the world, with 2,200,000 new cases a year (1). Taking into account the different clinical course, cell biology, and treatment method, two main histological types of lung cancer are distinguished: non-small cell lung cancer (NSCLC) and small cell lung cancer (SCLC). According to the classification, the NSCLC group includes squamous cell carcinoma (SQCLC), adenocarcinoma (ADC) and the NOS subtype (not otherwise specified, recognized in a small fraction of samples) (2). As a solid tumor with low antigen specificity, lung cancer escapes immune surveillance and attacks by cytotoxic lymphocytes cells which play a major role in the anti-neoplastic defense of the immune system (3).
The presence of neoplastic metastases in the mediastinal lymph nodes (LNs) is one of the most important elements in determining the optimal treatment strategy in patients with NSCLC (4). In recent years, the mediastinal LNs are increasingly researched by use of ultrasound-guided transbronchial needle aspiration (EBUS/TBNA) technique with, routinely, evaluation in light microscopy, but it can provide high diagnostic performance with using flow cytometry analysis (5, 6). It is known that tests performed with the use of peripheral blood (PB) do not show local changes in lung cancer microenvironment. PB serves as a material for the assessment of systemic changes while EBUS/TBNA node biopsy is the material that allows to obtain rich cellular material from the nearest disease area reflecting the tumor microenvironment (TME) (7).
LNs and Lymphocyte Phenotype
LNs act as an initiation site for the immune response and primary site for antigen presentation and lymphocyte maturation (8). Migrating naive thymic T cells called recent thymic emigrants (RTEs) mature into naive T lymphocytes after reaching the node and upon contact with the antigen presented by dendritic cells (DCs).
T naïve cells CD3+ CD45RA+ CD45RO- CD197+ differentiate into short−term living effector T cells phenotypically characterized as CD3+ CD45RA+ CD45RO- CD197-. They are heterogeneous population cells which produce cytokines and cytotoxic molecules, show activation markers and are involved in the inflammatory response (9). These cells have the potential to differentiate into long-lived memory cells which subsets were originally defined based on homing and migration markers along with effector functions. Central memory cells are phenotypically characterized as CD3+ CD45RA- CD45RO+ CD197+ (10, 11), express the LNs homing receptors CCR7 and CD62L, possess a high proliferative capacity but low cytotoxicity and after restimulation are activated, proliferate, and form immune memory. They differentiate into T effector cells (reaching inflammatory tissues) and a smaller population of central memory T cells (circulating between lymphatic organs) (12, 13). Memory T effector cells with the phenotype CD3+ CD45RA- CD45RO+ CD197- with inflammatory capacity, migration potential, and effector function generally do not migrate to LNs and only a very small fraction has been recently found in the LNs (9). Recent reports indicate a large variety of memory cells, including: terminally differentiated effector memory (Temra) cells, memory stem (Tscm) cells, and tissue-resident memory (Trm) cells (14, 15). Temra cells are a heterogeneous population, and although they are memory effector cells, they acquire expression of the CD45RA antigen upon antigenic stimulation and are involved in the immune response (16).
It has been found that infiltration of metastatic lymph nodes by memory T cells is an independent positive prognostic factor in patients with NSCLC (17). It is known that tumor infiltrating naive T cells differentiate into functional effector cells in the tumor microenvironment and promote tumor cells destruction (18, 19). CD8+ T cells with effector markers have been identified in cancer patients and appear to overlap with depleted T cells at tumor sites (20, 21).
The human CD4+ T cell is a heterogeneous population, divided into sub-populations depending on the functions performed. The activities of individual subpopulations result from the type of transcription factors and receptors contained in cells, as well as secreted cytokines, specific for each subpopulation (22).
In addition to the well-known Th1 and Th2 subpopulations, as well as Treg regulatory lymphocytes, additional subpopulations have been described. Th9, Th17, and Th22 are marked according to the type of secreted “main” cytokine and T follicular helper occurring in lymphoid organs (23).
T Cells of Regulatory Properties
Studies conducted in recent years have shown that other subpopulations of CD4+ T lymphocytes also participate in the polarization of the specific response to tumor cells: T regulatory cells (Tregs) and Th17 cells. Tregs play the most important role in regulating the immune response in tumors to inhibit the activity of T cells (CD4+, CD8+), dendritic cells, and NK cells (24). Tregs play a key role in tumor immunology, having an important impact on the outcome of cancer patients (25). The negative prognostic significance of the presence of Tregs has been confirmed in numerous studies on lung cancer (26). Studies reported significantly higher percentage of Tregs in patients with metastatic NSCLC compared to healthy donors with correlation to more advanced stage of disease (27–29). It has been proposed to differentiate Tregs to naive, effector, and terminal effector subtype on the basis of the expression of CD45RO marker (30). However, the prognosis impact of different Tregs subsets in lung cancer prognosis it is limited, sometimes controversial, and additional studies on the Tregs subsets appear to be interesting. In addition, it is also interesting to evaluate Th17 cells, which may play a role in the anti-tumor response. These cells belong to the group of CCR6 + (CD196 +) memory T-helper cells (CCR6 + memTh). CCR6 + memTh is a heterogeneous population that contains Th17/Th22 and Th17/Th1 cells (31). These cells produce pro-inflammatory cytokines such as interleukin (IL)-17A, tumor necrosis factor alpha (TNFα), and interferon-gamma (IFNγ) (32–34). From the above population of T lymphocytes, Th17 may remain an important prognostic factor due to some reports on the role of these cells in the neoplastic environment. Th17 lymphocytes play an important role in antimicrobial and antifungal defense but have weak proliferative and cytotoxic properties (35). Their role in autoimmune processes has been proven, but there are no unambiguous data on the anti-tumor activity of Th17 lymphocytes, although studies show the presence of these cells in the tumor microenvironment (36). It seems that the function of Th17 cells and interleukin-17 (IL-17) produced by Th17 cells, due to its pleiotropism, may have an inhibitory or stimulating effect. The stimulatory effect may be related to the pro-angiogenic effect of IL-17 (37). The antitumor activity of these cells has been demonstrated in numerous studies which showed that IL-17 induces a specific antitumor response and leads to tumor growth inhibition (38). The cells with the phenotype are CD3+ CD4+ CD45RO+ CD196+ which we assessed belong to the memTh CCR6 + cell group. Due to the lack of functional tests to assess cytokines or assay of cell cultures, we called them Th17-related cells.
Aim of the Study
The cellular characteristics of the lymph node and the definition of T-lymphocyte subpopulation in the affected lymph node and in the metastatic-free node seem interesting. Based on previous studies, it is known that the lung environment shows a certain dissimilarity in terms of the nature of the immune response (26, 39–41).
The aim of this study was to determine the dominant subtypes of CD4+ and CD8+ lymphocytes in metastatic and non-metastatic LNs of lung cancer patients. Accurate cellular assessment of lymph nodes offers the possibility of setting a new direction in the pre-therapeutic evaluation of lung cancer patients and the development of additional prognostic markers. Understanding cellular elements that play a role in the immediate disease environment that enables EBUS aspirate testing may have important therapeutic implications.
Materials and Methods
Patients
Patients with histologically confirmed primary lung cancer were included in the study groups (n = 30) (the current histological classification (2) and the 8th edition of TNM classification of lung cancer were used (42)). The study group consisted of patients undergoing diagnostic procedures for lung tumors. Patients without any type of previous or recent anticancer therapy, clinical signs of infection, autoimmune diseases, or immunosuppressive treatment were qualified for the study.
Each patient had provided written informed consent (the Military Institute of Medicine Ethics Committee: 25/WIM/2018) before the diagnostic procedure which included EBUS/TBNA and PB collection. The individual clinical characteristic of the lung cancer patients is summarized in Table 2.
Before EBUS/TBNA, chest computed tomography was performed. During EBUS, suspected metastatic LNs were punctured beginning from the most distal node station. When the quality of the sample was appropriate, the material was divided for cytopathology staining, molecular testing, and flow cytometry analysis.
LNs were considered metastatic when the standard cytopathology samples were classified as positive, histology was consistent with primary tumor, or results of EBUS/TBNA provided a clear diagnosis of cancer.
After precise determination of histological type of lung cancer, the evaluation of biomarkers for further therapy was done according to recommendations (43, 44). Molecular analysis was performed in 4 patients with adenocarcinoma, 1 with not otherwise specified NOS type of lung cancer, 1 adenosquamous, and 2 with squamous type. PD-L1 expression was performed in 9 patients. The decision of evaluation of molecular tests or PD-L1 expression depended on therapeutic plan and patient performance status. Thus, the number of patients with biomarker analysis was low (presented for individual patients in Table 2).
The consecutive samples were collected for flow cytometry analysis and only those that fulfilled inclusion criteria were chosen for further analysis. Next, we divided the samples according to the presence of LNs metastases to metastatic LNs and those without cancer cells (non-metastatic).
Materials
The aspirates from the LNs group 4, 7, 10, and 11 were obtained during the routine EBUS/TBNA procedure of lung cancer diagnosis. After diagnostic aspiration, an additional sample was taken for a flow cytometry analysis. About 1 ml of LNs aspirates was diluted in 0.9% NaCl, collected in tubes containing K2EDTA, and processed for flow cytometry. 2 ml of peripheral blood (PB) was taken and placed it in tubes containing K2EDTA and processed for flow cytometry.
Flow Cytometry Analysis
For flow cytometric analysis 100 μL of LNs aspirate, the same volume of PB and 4 μL of specific monoclonal antibodies were added to each cytometric tube for surface marker detection. Cells were stained with fluorescently labeled antibodies for 20 min at room temperature. Erythrocytes were lysed with Pharm Lyse Lysing Buffer (BD Biosciences, Franklin Lakes, NJ, USA) for 10 min. After washing, cells were analyzed within 2 h. For each sample, a minimum of 100,000 events were collected using the FACS Canto II BD flow cytometry (BD Biosciences). The data were analyzed with DIVA Analysis software 8.0.1 (BD Biosciences) and Infinicyt 1.8 Flow Cytometry (Cytognos, Salamanca, Spain).
To evaluate the T lymphocyte subpopulation and main leukocytes, subsets of the following antibodies were used:
CD45-V500-C (catalog number 655873, clone number: 2D1, BD Biosciences), CD3-PerCP-Cy5.5 (catalog number: 332771, clone number: SK7, BD Biosciences), CD4-FITC (catalog number: 345768, clone number: SK3, BD Biosciences), CD8-V450 (catalog number: 560347, clone number: RPA-T8, BD Biosciences), CD19-PE-Cy7 (catalog number: 341113, clone number: SJ25C1, BD Biosciences), CD56-PE (catalog number: 345810, clone number: MY31, BD Biosciences), CD16-APC-H7 (catalog number: 560195, clone number: 3G8, BD Biosciences), HLA-DR-V450 (catalog number: 655874, clone number: L243, BD Biosciences) CD123-APC (catalog number: 560087, clone number: 7G3, BD Biosciences), CD196-PE (catalog number: 551773, clone number: -), CD197-PerCP-Cy5.5 (catalog number: 353220, clone number: G043H7 BioLegend San Diego, CA, United States), CD45RO-PE-Cy7 (catalog number: 560608, clone number: UCHL1, BD Biosciences), CD45RA-APC (catalog number: 550855, clone number: -, BD Biosciences), CD62L-PE (catalog number: 555544, clone number: -, BD Biosciences), CD31-PerCP-Cy5.5 (catalog number: 303132, clone number: WM59, BioLegend San Diego, CA, United States), CD127-FITC (catalog number: 560549, clone number: HL-7R-M21, BD Biosciences), CD25- APC (catalog number: 340907, clone number: 2A3, BD Biosciences).
Using the appropriate combination of the above antibodies, we distinguished the following of CD4+ or CD8+ T cell subtypes (11) Table 1.
T lymphocytes subsets gating strategy in LNs of lung cancer patients is presented in Figure 1.
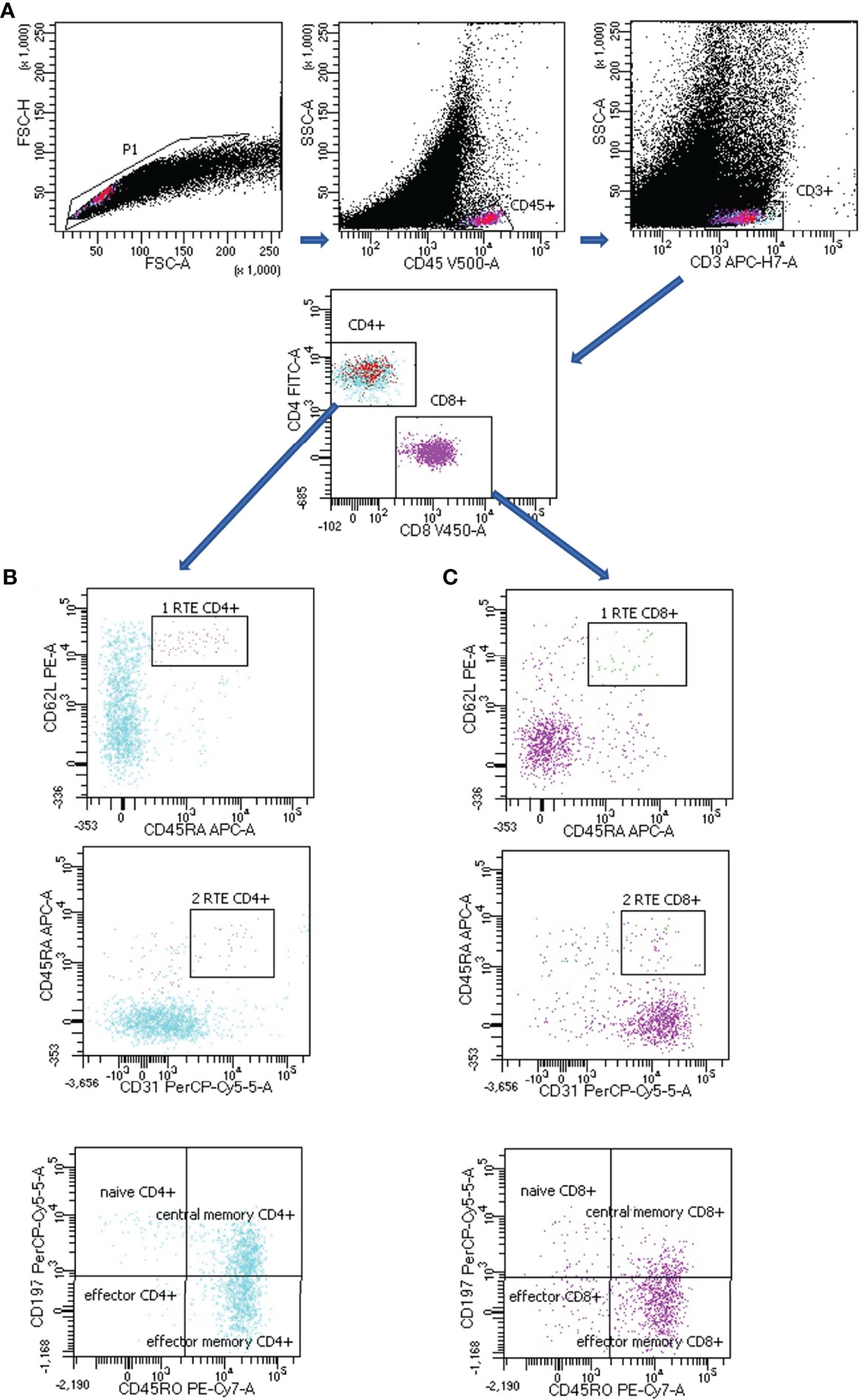
Figure 1 Representative T subsets gating strategy in lymph node (LN) aspirate of lung cancer patient. (A) CD4+ or CD8+ T lymphocytes: FSC-H vs. FSC-A plot: Gating the cells and removing clumps (greater FSC-A relative to FSC-H) and debris (very low FSC), SSC-A vs. CD45 plot: Selection of lymphocytes based on their CD45 positive properties, SSC-A vs. CD3 plot: Selection of lymphocytes T based on their CD3 positive properties. CD4 vs. CD8 plot: Selection of lymphocytes T CD4+ (turquoise) and CD8 + (violet) based on their CD4 positive or CD8 positive properties. (B) CD4+ T lymphocytes subsets: CD62L vs. CD45RA plot together with CD45RA vs. CD31 plot: Selection of recent thymic emigrants T CD4+ cells (RTE CD4+) based on their CD62L positive, CD45RA positive and CD31 positive properties, CD197 vs. CD45RO plot: Selection of naïve CD4+ T cells, effector CD4+ T cells, central memory CD4+ T cells and effector memory CD4+ T cells based on their CD197/CD45RO properties (the exact antigenic characterization can be found in the text in Materials and Methods section, Flow cytometry analysis). (C) CD8+ T lymphocytes subsets: CD62L vs. CD45RA plot together with CD45RA vs. CD31 plot: Selection of recent thymic emigrants T CD8+ cells (RTE CD8+) based on their CD62L positive, CD45RA positive and CD31 positive properties, CD197 vs. CD45RO plot: Selection of naïve CD8+ T cells, effector CD8+ T cells, central memory CD8+ T cells and effector memory CD8+ T cells based on their CD197/CD45RO properties (the exact antigenic characterization can be found in the text in Materials and Methods section, Flow cytometry analysis).
In this study the other subpopulations of T lymphocytes were also analyzed:
- Th17- related cells (CD3+ CD4+ CD45RO+ CD196+),
- T regulatory cells (Tregs CD3+ CD4+ CD25+high CD127−) and
- Tregs with expression of CD45RO+ (Tregs: CD3+ CD4+ CD25+high CD127- CD45RO+).
Tregs, CD45RO+ Tregs and Th17- related cells lymphocytes gating strategy in LNs of lung cancer patients is presented in Figure 2.
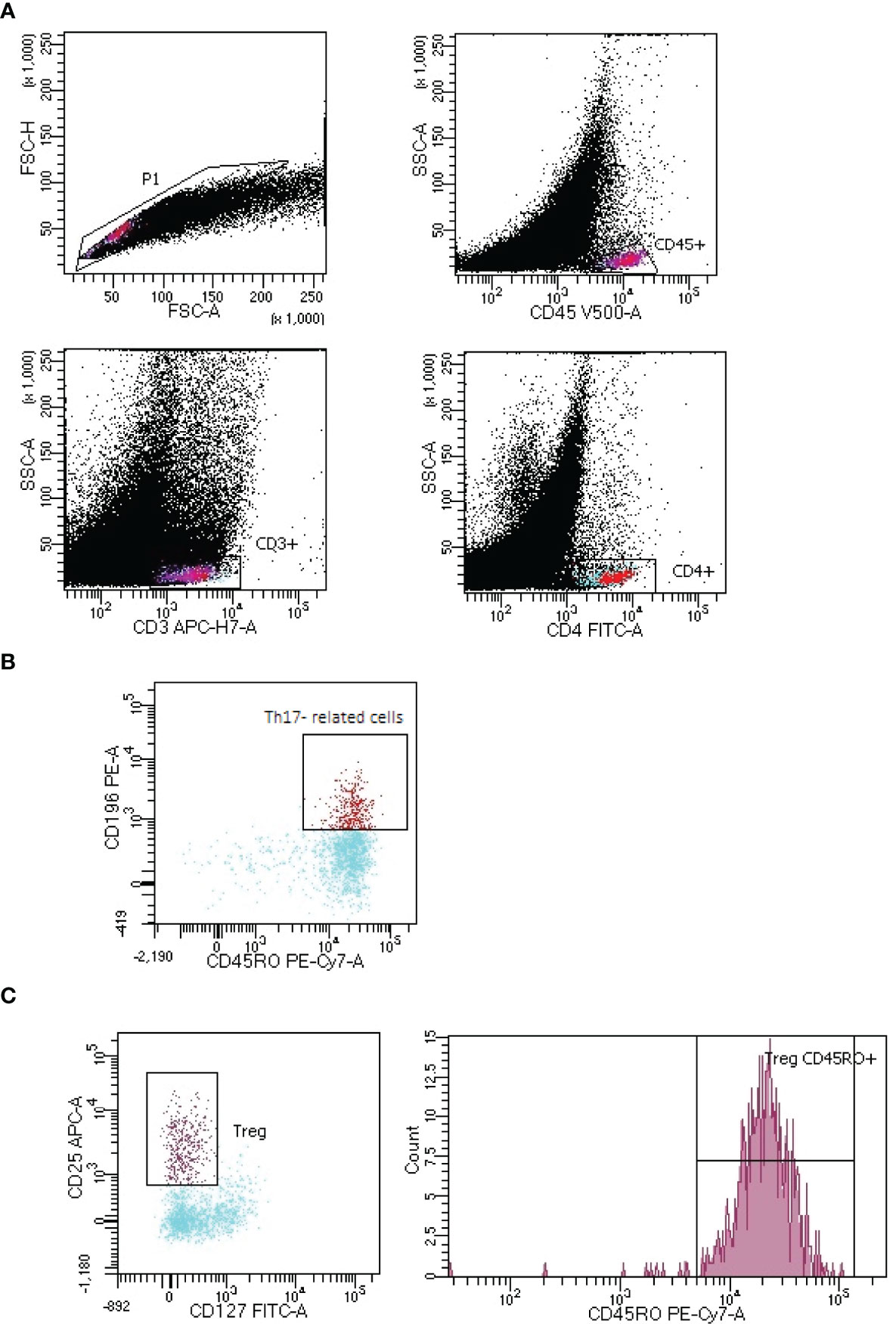
Figure 2 Representative gating strategy of Th17- related and T regulatory cells (Tregs) with expression of CD45RO+ in lymph node (LNs) aspirate of lung cancer patient. (A) CD4+ T lymphocytes:. FSC-H vs. FSC-A plot: Gating the cells and removing clumps (greater FSC-A relative to FSC-H) and debris (very low FSC), CD45 vs. SSC-A plot: Selection of lymphocytes based on their CD45 positive properties, CD3 vs. SSC-A plot: Selection of lymphocytes T based on their CD3 positive properties. CD4 vs. SSC-A plot: Selection of lymphocytes T CD4+ (turquoise) based on their CD4 positive properties. (B) Th17 lymphocytes: CD196 vs. CD45RO plot: Selection of Th17 cells based on their CD196 positive and CD45RO positive properties. (C) Tregs with CD45RO+ expression: CD25 vs. CD127 plot: Selection of Tregs based on their CD25 high positive and CD127 positive properties, count vs. CD45RO+ histogram: Selection of lymphocytes Tregs with CD45RO+ expression.
Moreover, we distinguished the following subpopulations of main leukocytes: lymphocytes (CD45+bright SSC low), lymphocytes T (CD45+bright SSC low CD3+), lymphocytes B (CD45+bright SSC low CD19+), NK cells (CD45+bright SSC low CD3- CD16+), neutrophils (CD45+ SSC bright CD16+), dendritic cells (CD45+ CD123+ bright HLA-DR+), monocytoid line cells: monocytes (in PB: CD45+bright SSC+ HLA-DR+), and macrophages (in EBUS/TBNA: CD45+bright SSC+bright HLA-DR+bright).
Internal quality control was performed daily by checking the optical detector, aligning lasers, and fluid systems using CS&T IVD Beads BD FACS Diva (BD Biosciences, San Jose, CA USA), respectively, according to the manufacturer’s guidelines.
Lymphocyte counts were obtained using a SYSMEX XN-1500 (Sysmex Corp., Kobe, Japan) haematological analyzer.
Results
Patients’ Characteristics
In all patients with suspicion of lung cancer, with the presence of mediastinal lymphadenopathy, a chest X-ray was performed. Computed tomography was performed showing altered LNs. EBUS/TBNA was made for cytological evaluation of the lesion and obtaining material for evaluation by flow cytometry. Histopathological examination of the changed lymph node made the final diagnosis.
There were 30 patients with newly diagnosed and confirmed lung cancer (clinical patient characteristic is presented in Table 2). There were 21 men (the mean age: 65 years, the range min-max: 47-79) and 9 women (the mean age: 67 years, the range min-max: 60-74). There were 13 smokers, 5 ex-smokers, and 12 never smokers in the group. Most patients in the study group were in an advanced stage of lung cancer (stage I: 5 (16.7%), II: 4 (13.3%), III: n = 12 (40.0%) and stage IV: n = 9 (30.0%)). Histological examination revealed: squamous cell lung cancer (SQCLC), 36.7%; small cell lung cancer (SCLC), 30.0%; adenocarcinoma (ADC), 23.3%; not otherwise specified (NOS), 6.7%; and adenosquamous carcinoma (ADSQ), 3.3%. Due to a small number of patients, we did not perform a comparison between the groups with different NSCLC types or between different stages of the disease.
For further comparative analysis using flow cytometry, we selected patients: with the presence of metastases in LN based on histological confirmation (n= 18)and without metastases (n = 12).
There were no differences in the median proportion of main leukocyte subpopulations and T subsets between PB from patients with non-metastatic LNs and PB from patients with metastatic LNs, therefore, PB was not considered in the further analysis (Tables S1, S2, Supplementary data section).
T Lymphocytes Subsets
CD4+ Subsets
In analyzing CD4+ T cells, we observed higher median proportion of effector memory CD4+ T cells in metastatic LNs than non-metastatic (28.7 vs. 15.3%, p= 0.047865). No other differences were observed for the CD4 subtypes of lymphocytes, such as effector, central memory, naïve, or RTE, between metastatic LNs and non-metastatic LNs (Table 3 and Figure 3). The ratio of CD4 naive cells to CD4 memory effector cells did not differ between metastatic and non-metastatic LN (2.59 vs. 0.81, p> 0.05) (data not shown).
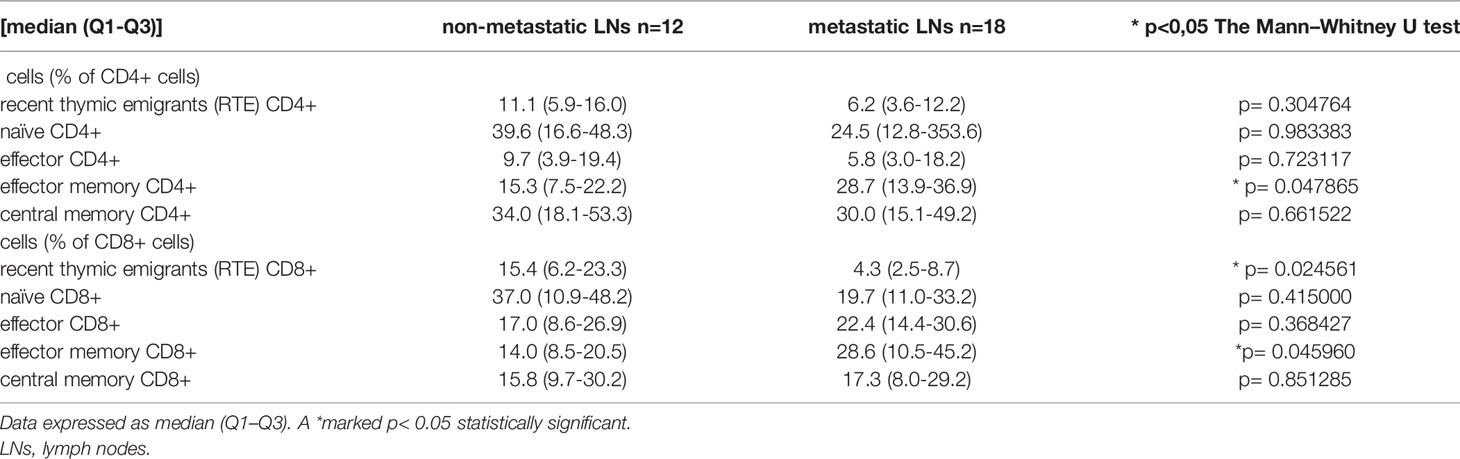
Table 3 Differences in the median proportion of T lymphocytes subsets in lung cancer patients between non-metastatic lymph nodes (LNs) and metastatic LNs.
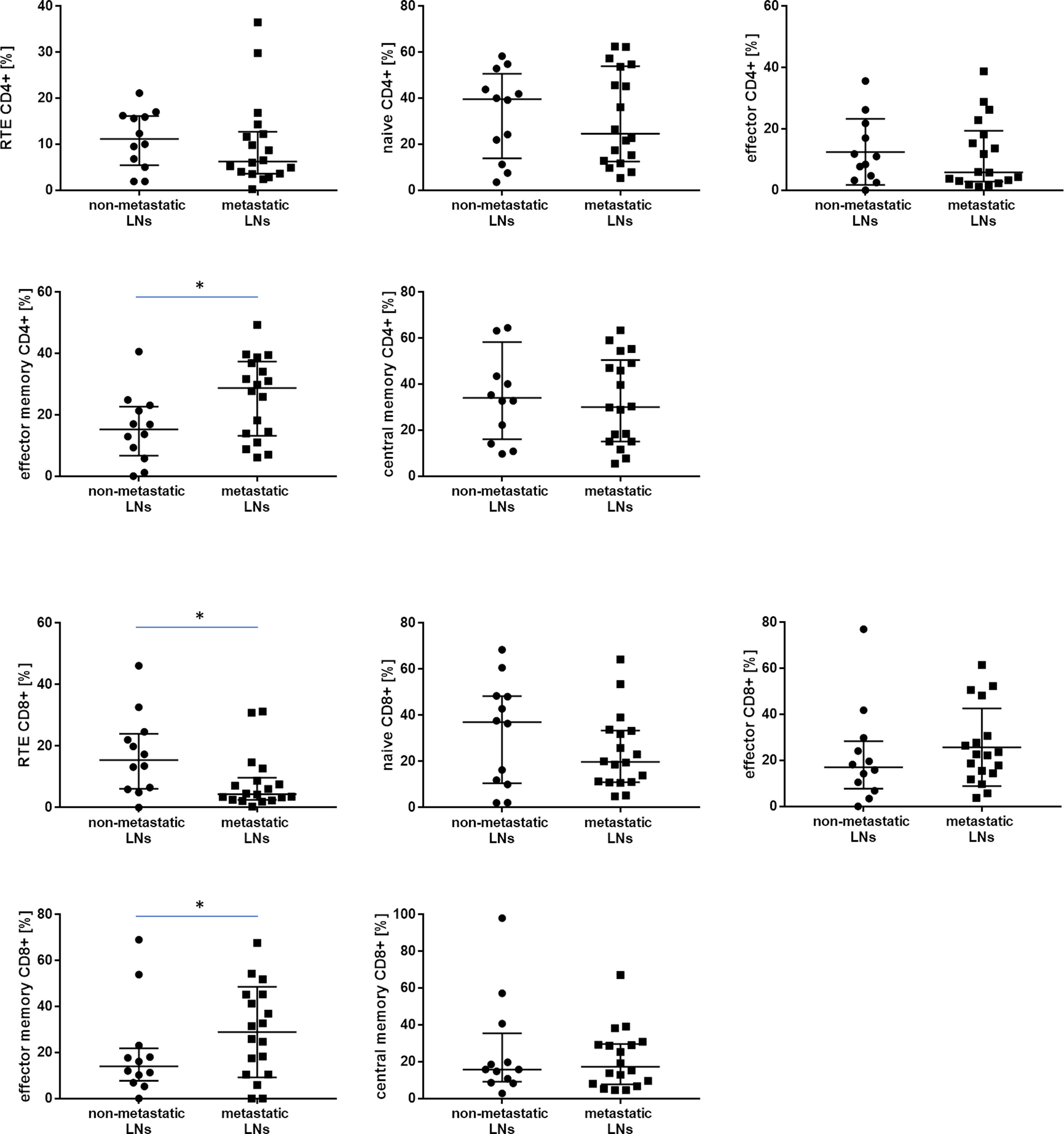
Figure 3 The differences in the median proportion of T lymphocytes subsets (CD4+ and CD8+): Recent thymic emigrants T cells (RTE), naïve T cells, effector T cells, central memory T cells and effector memory T cells between metastatic lymph nodes (LNs) and non-metastatic LNs of the patients with lung cancer. Graphs show the median values (Min-Max). Significant differences in the cell proportion between the non-metastatic lymph nodes (LNs) and the metastatic LNs in the Mann–Whitney U test presented as * (p<0.05).
CD8+ Subsets
In analyzing CD8+ T cells, we observed higher median proportion of effector memory CD8+ T cells in metastatic LNs than non-metastatic LNs (28.6 vs. 14.0%, p= 0.045960). We observed lower median proportion of RTE CD8+ cells in metastatic LNs than non-metastatic LNs (4.3 vs. 15.4, p= 0.024561) (Table 3 and Figure 3). The ratio of CD8 naive cells to CD8 memory effector cells did not differ between metastatic and non-metastatic LN (2.37 vs. 0.58, p> 0.05) (data not shown).
Other T Lymphocytes Subsets
In this study, the proportion of following T lymphocyte subsets was analyzed such as Th17- related cells, Tregs, and Tregs with expression of CD45RO+ (Table 4). We observed a higher median proportion of CD45RO+ Tregs in metastatic LNs than in non-metastatic LNs (65.6 vs. 31.0%, p= 0.01040, result is presented as % of CD45RO+ cells among Tregs) (Figure 4).

Table 4 Differences in the median proportion of T lymphocytes subpopulation: Th17- related and regulatory T cells with CD45RO+ expression in lung cancer patients between non-metastatic lymph nodes (LNs) and metastatic LNs.
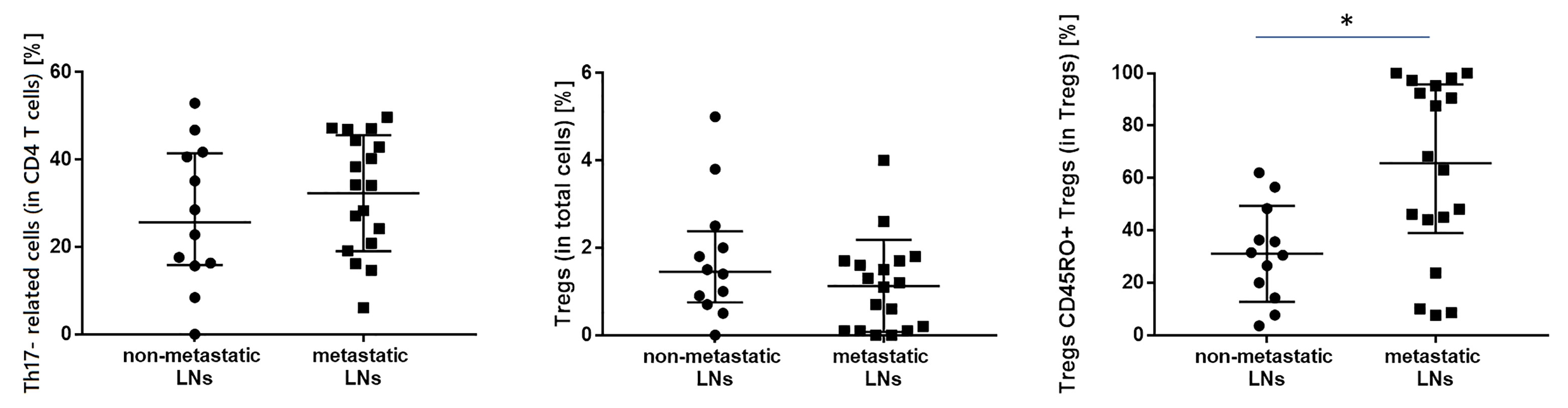
Figure 4 The differences in the median proportion of T regulatory cells (Tregs) (as a % of T cells), Tregs with CD45RO+ expression and Th17- related (as a % of CD4+ cells) between metastatic lymph nodes (LNs) and non-metastatic LNs of the patients with lung cancer. Graphs show the median values (Min-Max). Significant differences in the cell proportion between the non-metastatic lymph nodes (LNs) and the metastatic LNs in the Mann–Whitney U test presented as * (p<0.05).
Correlation Between T Cells Subsets
Considering the correlations between the CD4+ T cell subsets, a significant negative correlation was observed between effector memory CD4+ cells and naïve CD4+ cells (r= -0.5, p< 0.05).
Effector memory CD8+ negatively correlated with effector CD4+ (r= -0.6, p< 0.05), with naïve CD8+ (r= -0.8, p< 0.05), and with effector CD8+ (r= -0.6, p< 0.05).
In the metastatic LN group, there was a significantly negative correlation between the proportion of effector CD4+ cells and Tregs with CD45RO+ expression (r = -0.6, p < 0.05).
In the metastatic LN group, there was a high positive correlation between the proportion of effector memory CD8+ and Tregs with CD45RO+ expression (R = 0.6, p < 0.05).
There was no correlation between T cell populations and Th17- related cells.
All correlations between the proportion of effector memory CD4+, effector memory CD8+ cells, Th17- related cells, and Tregs with CD45RO+ expression with T subset in metastatic LNs are presented in heat maps (Figure 5).
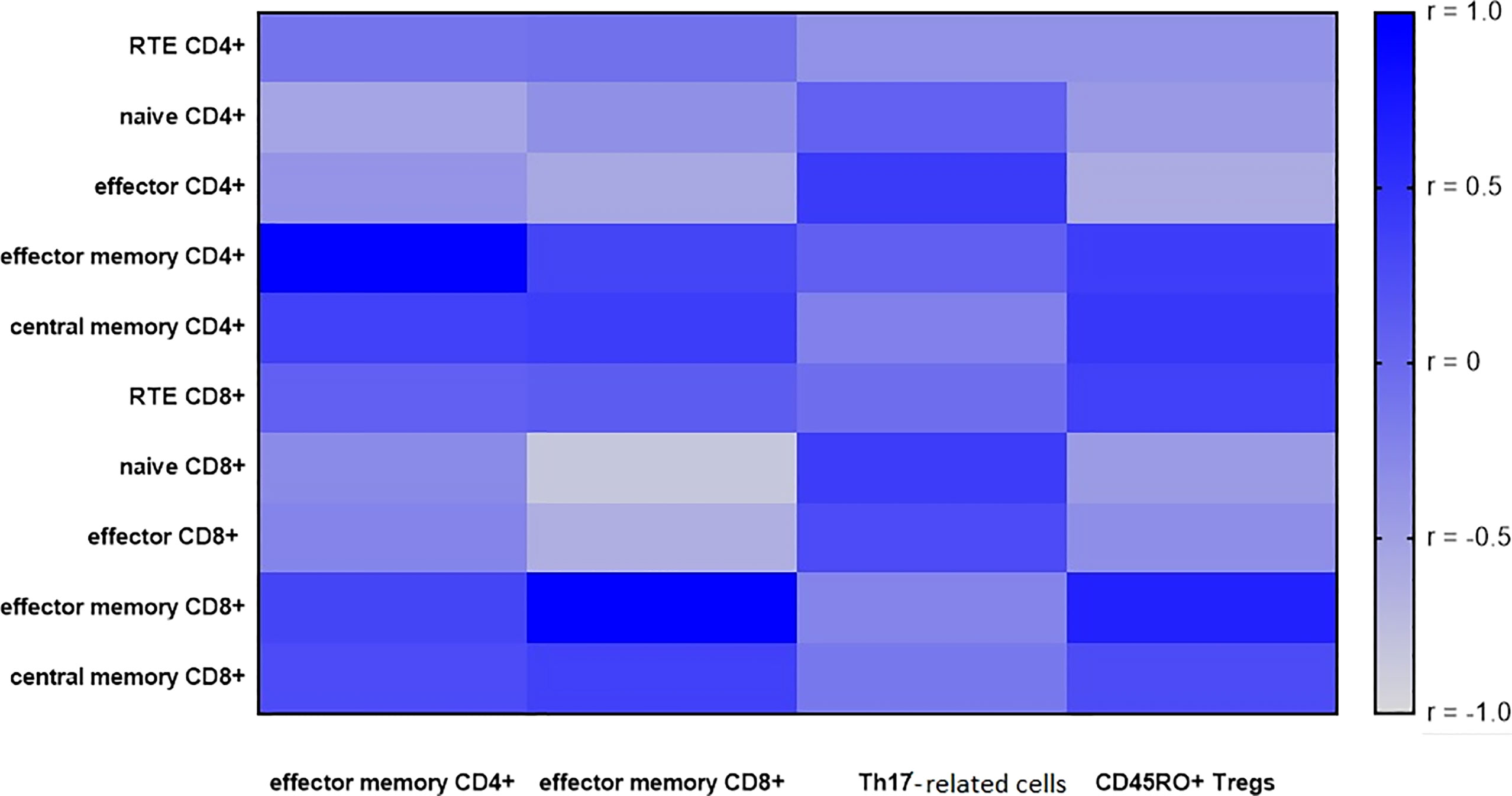
Figure 5 A heatmap of Spearman correlation coefficients for the effector memory T cells (CD4+ and CD8+), Th17- related and Tregs with CD45RO+ expression with T subsets in metastatic lymph nodes (LNs). Correlations with an absolute value more than 0.5 are associated with p < 0.05, blue—positive correlations, gray—negative correlations.
Leukocyte Subsets in Lymph Nodes
We also analyzed the main leukocyte profile in LNs in patients with lung cancer depending on presence of metastases in LNs. We observed a lower median proportion of lymphocytes in metastatic LNs than in non-metastatic LNs (Table 5). There was a higher median proportion of monocyte/macrophage lineage cells and dendritic cells (DCs) in metastatic LNs than non-metastatic LNs.
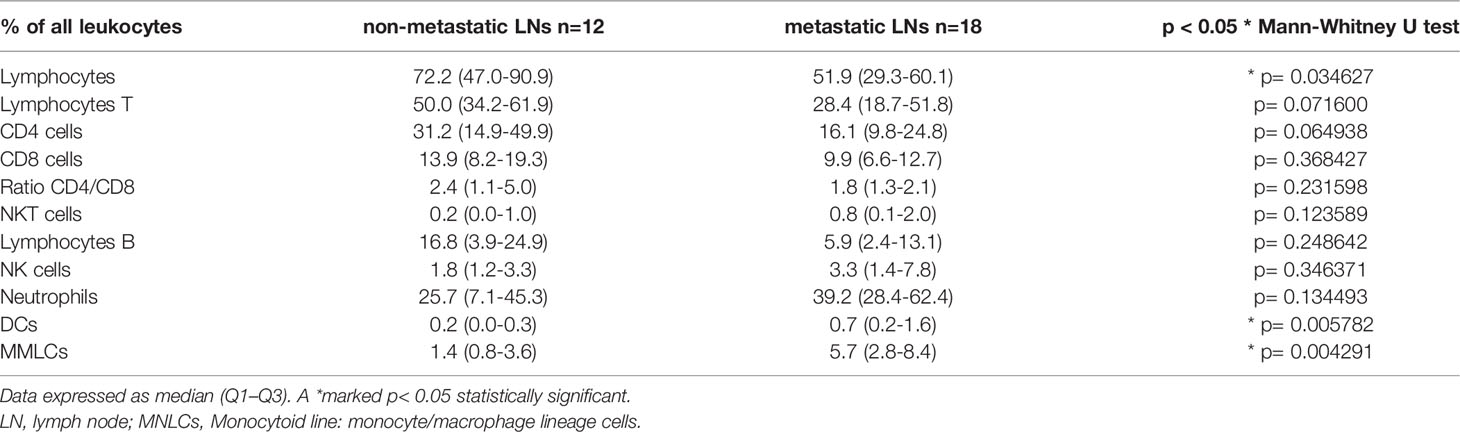
Table 5 The differences in the median proportion of leukocytes and main lymphocytes subpopulation between non-metastatic lymph nodes (LNs) and metastatic LNs.
Cells not expressing CD45 antigen (non-hematopoietic cells), including tumor cells, were not included in this study.
Discussion
In this study, we focused on assessing the LNs cell profile of lung cancer patients with an emphasis on distinguishing the dominant status of T-cell subsets. We focused on the possibility of a practical and accessible assessment of the main subpopulations of T lymphocytes, before possible immunotherapy, as a potential biomarker. Despite the fact that there are many mechanisms which allow cancer cell to escape from immune surveillance (45, 46), the cellular defense is the basis of anti-cancer resistance, used among others, in immunotherapy. It is known that lymphocytes T are the most essential inflammatory cells infiltrating the tumor, exerting a direct cytotoxic effect, or leading to tumor lysis by cytokine release (47–49).
We assumed that it would be important to analyze the process of maturation and differentiation of T cells in LNs of lung cancer patients. The results of our study show, for the first time, the important differences between metastatic and non-metastatic LNs evaluated using EBUS/TBNA and flow cytometry.
T Lymphocyte Subsets Profile in Lung Cancer LNs
In our study the T lymphocytes subsets profile revealed higher median proportion of effector memory CD4+ T cells and effector memory CD8+ T cells in metastatic LNs than non-metastatic LNs.
Moreover, effector memory T cells turned out the dominant population among the total CD8+ cells population. Additionally, we found lower median proportion of RTE CD8+ cells in metastatic than non-metastatic LNs.
It is known that effector memory cells produce many inflammatory cytokines and their most important role is related to the activation of the immune response taking place in peripheral lymphoid organs. These cells do not pass through the lymph nodes but flow to the inflamed sites (12). The function of effector memory T cells depends on the presence of a persistent antigen in the organism and when reaching the site of inflammation, they act through the secretion of cytokines or by a cytotoxic effect (50). Effector memory T cells express higher levels of receptors responsible for migration to inflamed tissues and have a stronger immediate effector function than central memory T (12, 51). In several solid tumors, tumor-specific T cell memory responses likely play an important role in keeping tumors in check, limiting cancer cell dissemination and reducing risk of relapse.
Our results may indicate the cytotoxic reaction to cancer cells in metastatic LNs. Other researchers confirm the presence of effector lymphocytes at the site of the ongoing neoplastic process and their prognostic importance. Our present and former findings indicate specificity of the character of the environment of the metastatic tumor site (52–55). The high number of CD8+ CD45RO+ effector memory T cells found in the primary tumor lesion significantly correlates with a positive clinical outcome in various cancers. In a meta-analysis of 25 published studies with 4,720 patients, researchers assessed the prognostic effect of tumor infiltrating CD45RO+ T cells in human solid tumors (56). It was shown that the infiltration of CD45RO+ T cells was significantly associated with improved overall survival (OS) and disease-free survival (DFS) in all types of solid tumors. Moreover, the high density of CD45RO+ T cells inside the tumor was inversely correlated with the TNM stage of the solid tumor.
Recent research studies on cancer immunotherapy have focused on the use of anti-tumor CD8+ cytotoxic T-cell responses. Principe N. et al. suggest that increased effector memory tumor antigen-specific cytotoxic lymphocytes T CD8+, in the presence of reduced immunosuppression within tumors, is part of a successful immune check point therapy response (57). Other researchers propose an assessment the T central memory to T effector cells (Tcm/Teff) ratio as a predictive biomarker of response to checkpoint inhibitors in NSCLC patients (58).
Interestingly, in our study, despite the expected increase in effector memory cells, we also noticed a decrease in the number of naive RTE CD8+ cells in metastatic LNs. This phenomenon may mean that the tumor has silenced the immune response, stopped the influx of cells into the LNs, or redirected the body’s reaction to the effector ones.
Other T Lymphocyte Subsets
There is evidence that tumor cells form an immunosuppressant TME, including factors secreted by tumor or stromal cells and inhibitory immune cells such as myeloid suppressor cells (MDSC) and Tregs, affecting the activity of T cells (59). The development of the tumor and the spread of tumor cells is facilitated by the so-called immune tolerance, i.e., the lack of response to antigens. Tregs play the most important role in immune surveillance and tolerance mechanisms. The number of circulating Tregs are increased in patients with cancer, including lung cancer (25, 26, 60). Interestingly, in the present study, the proportion of Treg cells did not differ between metastatic and non-metastatic LNs, but the expression of the CD45RO+ antigen on these cells differed significantly. The proportion of CD45RO+ Tregs was higher in the metastatic than in the non-metastatic LNs. This observation confirms the importance of studies of various Tregs subtypes, showing different proliferation and migration potential. Amarillo D. et al. has shown that there was a significant difference between the percentage of memory Tregs (CD45RO+) in control patients and lung cancer patients (19.52 vs. 86.77% p <0.001) and confirmed that circulating Tregs are a potential prognostic factor in lung cancer patients (61).
It was found that increased tumor infiltrating Tregs are associated with poor overall survival in SCLC (62). Moreover, Kotsakis A. et al. determined that the increased presence of terminal effector Tregs predicts improved overall and progression-free survival, whereas an increase in naïve or effector Tregs number was associated with worse survival (25). The expression of the CD45RO marker on effector Treg (CD4+ CD25high CD127low CD45RO+) suggests activation and ability to proliferate but also could indicate a short-lived, terminally diverse population that is rapidly fragmenting and declining (63, 64). As confirmed by the above observation, the collective study of the Tregs population in metastatic LNs may not be sufficient, without precise differentiation, and the role of these cells seems to be very complex in lung cancer patients.
In this study, no difference in the median proportion of Th17- related cells was observed between the metastatic and non-metastatic LNs. Th17 participate in antimicrobial and fungal immunity, allergic, and autoimmune diseases, as well as anti-inflammatory responses (65, 66). Th17 cells are key producers of IL-17, and this cytokine is known to contribute to the induction of lung cancer prometastatic factor expression (67). Many studies reveal the potential functional effects of IL-17 on cancer progression and metastasis. In humans, the increased density of IL-17-positive cells in NSCLC tumors correlated with the density of lymphatic vessels. In addition, loss of IL-17 has been shown to reduce metastasis (68, 69).
The role of Th17 cells in promoting or inhibiting various human cancers seems to be context-dependent (70, 71). The influence of environmental cues may be one of the major determinants to modulate Th17 cell recruitment and function. Recently, growing evidence indicates that tumor-intrinsic genetics determine the corresponding immune profile and Th17 cell function.
Numerous studies have shown that the cytokine IL-17, directly or indirectly, promotes tumor angiogenesis and tumor cell proliferation and inhibits apoptosis by activating inflammatory signaling pathways. Thus, IL-17 contributes to the progression of lung cancer (69, 72, 73).
The above-mentioned studies confirm the robust role of Th17 cells in the course of lung cancer. In our study we found no differences in the proportion of Th17- related cells in non-metastatic and metastatic LNs. Perhaps not number of these cells alone is important, but their degree of stimulation and the ability to produce IL-17. The lack of differences in our work between metastatic and non-metastatic LN may also result from the imprecise phenotypic definition of these cells and the lack of application of functional methods, which is a limitation of our study.
In our study, the main leukocyte population was also divided for the purpose of precisely defining the T lymphocyte subpopulations. However, it was not the subject of the present study but it should be emphasized that the differences in the number of DCs and monocyte/macrophage lineage cells between the metastatic and non-metastatic LNs were presented. We showed higher median proportion of monocyte/macrophage lineage cells, and DCs in metastatic LNs than non-metastatic LNs. This observation confirms the current state of knowledge that, in addition to lymphocytes, important cells within the tumor area are antigen presenting cells (APC), such as DCs and monocytes. In our other work, we present the subtypes of DCs cells (55) and discuss their involvement in metastatic processes together with heterogeneous monocytes (74).
Relationships Between T Lymphocytes Subset in Metastatic LNs
Next, we analyzed the relationship between the effector memory CD4+ and CD8+ cells with other subsets of T cells, CD45RO+ Tregs and Th17- related using correlation in metastatic LNs. Presented correlations confirm the important role of effector memory cells, mainly effector memory CD8+ cells in lung cancer. We noticed more correlations among the subpopulations of T lymphocytes with CD8+ cells than with CD4+ cells in LNs with metastases of lung cancer patients. We showed negative correlation of effector memory T CD8+ cells with effector CD4+, with naïve CD8+, with effector CD8+ cell.
By analyzing the differences between metastatic and non-metastatic LNs, we found that effector memory T cells are an interesting parameter to use for the evaluation of immune status patients with lung cancer. Given the relationships in metastatic LNs between T cell subpopulations as assessed by the correlation, we can also hypothesize about the cytotoxic response of the organism to the tumor and the importance of effector memory cells. Interestingly, in our study, we also observed a high positive correlation between effector memory CD8+ cells and CD45RO+ Tregs. Perhaps these two parameters together differentiate patients with and without metastases and a positive correlation with each other could be used in the evaluation of patients with lung cancers.
To summarize, the LN is known to be a common site of metastasis and lymph node disease predicts increased mortality in many types of cancer. Moreover, LNs are critical in initiating anti-cancer immune responses (75, 76). Metastatic cells in lymphoid organs are important in the induction of anti-cancer CD8+ T cells and tumor rejection (77, 78). Understanding the mechanisms of survival, tumor growth, and immune system response in LNs is key to designing an effective therapy to eradicate LN metastasis. It has been hypothesized that the correlation of effector memory cells with other subsets of T lymphocytes would allow recognition of significant cells and their relationship in the course of lung cancer all of which fits in the progress of prognosis assessment and the determination of new immunological therapeutic points.
Based on the above results, we confirm that using EBUS/TBNA methods with flow cytometry is possible to obtain diagnostic material from the LN, which in the metastatic LNs, have a characteristic T-lymphocyte profile.
Conclusions
In conclusion, our findings show that assessment T cell profile in relation to other T cell subsets by flow cytometry analysis of EBUS/TBNA samples can provide insight into the immune status of metastatic LNs and thus reflect local TME. It is possible during diagnostic procedure to inform about the state of the anti-tumor immune response and further identify potential biomarkers of clinical response to modern therapies of lung cancer.
Data Availability Statement
The raw data supporting the conclusions of this article will be made available by the authors, without undue reservation.
Ethics Statement
The studies involving human participants were reviewed and approved by Military Institute of Medicine Ethics Committee: 25/WIM/2018. The patients/participants provided their written informed consent to participate in this study.
Author Contributions
IK and ER: Conceptualization and methodology; IK, RS, and JB: Data curation; IK and ER: Writing—original draft preparation; IK, KJ-R, PR, and JD-K: Writing—review and editing; AR: Visualization; JD-K: Supervision. All authors contributed to the article and approved the submitted version.
Funding
This study was funded by Military Institute of Medicine, grant number 559.
Conflict of Interest
The authors declare that the research was conducted in the absence of any commercial or financial relationships that could be construed as a potential conflict of interest.
Publisher’s Note
All claims expressed in this article are solely those of the authors and do not necessarily represent those of their affiliated organizations, or those of the publisher, the editors and the reviewers. Any product that may be evaluated in this article, or claim that may be made by its manufacturer, is not guaranteed or endorsed by the publisher.
Supplementary Material
The Supplementary Material for this article can be found online at: https://www.frontiersin.org/articles/10.3389/fimmu.2022.864497/full#supplementary-material
References
1. Ferlay J, Colombet M, Soerjomataram I, Parkin DM, Pineros M, Znaor A, et al. Cancer Statistics for the Year 2020: An Overview. Int J Cancer (2021) 149:1–12. doi: 10.1002/ijc.33588
2. Travis WD, Brambilla E, Nicholson AG, Yatabe Y, Austin JHM, Beasley MB, et al. The 2015 World Health Organization Classification of Lung Tumors: Impact of Genetic, Clinical and Radiologic Advances Since the 2004 Classification. J Thorac Oncol (2015) 10(9):1243–60. doi: 10.1097/JTO.0000000000000630
3. Kunimasa K, Goto T. Immunosurveillance and Immunoediting of Lung Cancer: Current Perspectives and Challenges. Int J Mol Sci (2020) 21(2):597. doi: 10.3390/ijms21020597
4. Wu Y, Han C, Gong L, Wang Z, Liu J, Liu X, et al. Metastatic Patterns of Mediastinal Lymph Nodes in Small-Size Non-Small Cell Lung Cancer (T1b). Front Surg (2020) 7:580203. doi: 10.3389/fsurg.2020.580203
5. Herth FJ, Rabe KF, Gasparini S, Annema JT. Transbronchial and Transoesophageal (Ultrasound-Guided) Needle Aspirations for the Analysis of Mediastinal Lesions. Eur Respir J (2006) 28(6):1264–75. doi: 10.1183/09031936.00013806
6. Rutkowska E, Kwiecien I, Bednarek J, Sokolowski R, Raniszewska A, Jahnz-Rozyk K, et al. T Lymphocyte Maturation Profile in the Ebus-Tbna Lymph Node Depending on the Dlco Parameter in Patients With Pulmonary Sarcoidosis. Cells (2021) 10(12):3404. doi: 10.3390/cells10123404
7. Guarize J, Rocco EG, Marinis F, Sedda G, Bertolaccini L, Donghi SM, et al. Prospective Evaluation of Ebus-Tbna Specimens for Programmed Death-Ligand 1 Expression in Non-Small Cell Lung Cancer Patients: A Pilot Study. J Brasileiro pneumologia Publicacao Oficial da Sociedade Bras Pneumologia e Tisilogia (2021) 47(4):e20200584. doi: 10.36416/1806-3756/e20200584
8. Hampton HR, Chtanova T. Lymphatic Migration of Immune Cells. Front Immunol (2019) 10:1168. doi: 10.3389/fimmu.2019.01168
9. Muroyama Y, Wherry EJ. Memory T-Cell Heterogeneity and Terminology. Cold Spring Harb Perspect Biol (2021) 13(10):a037929. doi: 10.1101/cshperspect.a037929
10. Fink PJ. The Biology of Recent Thymic Emigrants. Annu Rev Immunol (2013) 31:31–50. doi: 10.1146/annurev-immunol-032712-100010
11. Boldt A, Borte S, Fricke S, Kentouche K, Emmrich F, Borte M, et al. Eight-Color Immunophenotyping of T-, B-, and Nk-Cell Subpopulations for Characterization of Chronic Immunodeficiencies. Cytomet Part B Clin Cytomet (2014) 86(3):191–206. doi: 10.1002/cyto.b.21162
12. Sallusto F, Geginat J, Lanzavecchia A. Central Memory and Effector Memory T Cell Subsets: Function, Generation, and Maintenance. Annu Rev Immunol (2004) 22:745–63. doi: 10.1146/annurev.immunol.22.012703.104702
13. Romero P, Zippelius A, Kurth I, Pittet MJ, Touvrey C, Iancu EM, et al. Four Functionally Distinct Populations of Human Effector-Memory Cd8+ T Lymphocytes. J Immunol (2007) 178(7):4112–9. doi: 10.4049/jimmunol.178.7.4112
14. Chang JT, Wherry EJ, Goldrath AW. Molecular Regulation of Effector and Memory T Cell Differentiation. Nat Immunol (2014) 15(12):1104–15. doi: 10.1038/ni.3031
15. Jameson SC, Masopust D. Understanding Subset Diversity in T Cell Memory. Immunity (2018) 48(2):214–26. doi: 10.1016/j.immuni.2018.02.010
16. Tian Y, Babor M, Lane J, Schulten V, Patil VS, Seumois G, et al. Unique Phenotypes and Clonal Expansions of Human Cd4 Effector Memory T Cells Re-Expressing Cd45ra. Nat Commun (2017) 8(1):1473. doi: 10.1038/s41467-017-01728-5
17. Kilvaer TK, Paulsen EE, Khanehkenari MR, Al-Saad S, Johansen RM, Al-Shibli K, et al. The Presence of Intraepithelial Cd45ro+ Cells in Resected Lymph Nodes With Metastases From Nsclc Patients Is an Independent Predictor of Disease-Specific Survival. Br J Cancer (2016) 114(10):1145–51. doi: 10.1038/bjc.2016.92
18. Yu P, Lee Y, Liu W, Chin RK, Wang J, Wang Y, et al. Priming of Naive T Cells Inside Tumors Leads to Eradication of Established Tumors. Nat Immunol (2004) 5(2):141–9. doi: 10.1038/ni1029
19. Thompson ED, Enriquez HL, Fu YX, Engelhard VH. Tumor Masses Support Naive T Cell Infiltration, Activation, and Differentiation Into Effectors. J Exp Med (2010) 207(8):1791–804. doi: 10.1084/jem.20092454
20. Djenidi F, Adam J, Goubar A, Durgeau A, Meurice G, de Montpreville V, et al. Cd8+Cd103+ Tumor-Infiltrating Lymphocytes Are Tumor-Specific Tissue-Resident Memory T Cells and a Prognostic Factor for Survival in Lung Cancer Patients. J Immunol (2015) 194(7):3475–86. doi: 10.4049/jimmunol.1402711
21. Franciszkiewicz K, Le Floc'h A, Jalil A, Vigant F, Robert T, Vergnon I, et al. Intratumoral Induction of Cd103 Triggers Tumor-Specific Ctl Function and Ccr5-Dependent T-Cell Retention. Cancer Res (2009) 69(15):6249–55. doi: 10.1158/0008-5472.CAN-08-3571
22. Shih HY, Sciume G, Poholek AC, Vahedi G, Hirahara K, Villarino AV, et al. Transcriptional and Epigenetic Networks of Helper T and Innate Lymphoid Cells. Immunol Rev (2014) 261(1):23–49. doi: 10.1111/imr.12208
23. Saravia J, Chapman NM, Chi H. Helper T Cell Differentiation. Cell Mol Immunol (2019) 16(7):634–43. doi: 10.1038/s41423-019-0220-6
24. Okeke EB, Uzonna JE. The Pivotal Role of Regulatory T Cells in the Regulation of Innate Immune Cells. Front Immunol (2019) 10:680. doi: 10.3389/fimmu.2019.00680
25. Kotsakis A, Koinis F, Katsarou A, Gioulbasani M, Aggouraki D, Kentepozidis N, et al. Prognostic Value of Circulating Regulatory T Cell Subsets in Untreated Non-Small Cell Lung Cancer Patients. Sci Rep (2016) 6:39247. doi: 10.1038/srep39247
26. Kwiecien I, Stelmaszczyk-Emmel A, Polubiec-Kownacka M, Dziedzic D, Domagala-Kulawik J. Elevated Regulatory T Cells, Surface and Intracellular Ctla-4 Expression and Interleukin-17 in the Lung Cancer Microenvironment in Humans. Cancer Immunol Immunother CII (2017) 66(2):161–70. doi: 10.1007/s00262-016-1930-6
27. Chen C, Chen D, Zhang Y, Chen Z, Zhu W, Zhang B, et al. Changes of Cd4+Cd25+Foxp3+ and Cd8+Cd28- Regulatory T Cells in Non-Small Cell Lung Cancer Patients Undergoing Surgery. Int Immunopharmacol (2014) 18(2):255–61. doi: 10.1016/j.intimp.2013.12.004
28. Erfani N, Mehrabadi SM, Ghayumi MA, Haghshenas MR, Mojtahedi Z, Ghaderi A, et al. Increase of Regulatory T Cells in Metastatic Stage and Ctla-4 Over Expression in Lymphocytes of Patients With Non-Small Cell Lung Cancer (Nsclc). Lung Cancer (2012) 77(2):306–11. doi: 10.1016/j.lungcan.2012.04.011
29. Okita R, Saeki T, Takashima S, Yamaguchi Y, Toge T. Cd4+Cd25+ Regulatory T Cells in the Peripheral Blood of Patients With Breast Cancer and Non-Small Cell Lung Cancer. Oncol Rep (2005) 14(5):1269–73. doi: 10.3892/or.14.5.1269
30. Sakaguchi S, Miyara M, Costantino CM, Hafler DA. Foxp3+ Regulatory T Cells in the Human Immune System. Nat Rev Immunol (2010) 10(7):490–500. doi: 10.1038/nri2785
31. Dankers W, den Braanker H, Paulissen SMJ, van Hamburg JP, Davelaar N, Colin EM, et al. The Heterogeneous Human Memory Ccr6+ T Helper-17 Populations Differ in T-Bet and Cytokine Expression But All Activate Synovial Fibroblasts in an Ifngamma-Independent Manner. Arthritis Res Ther (2021) 23(1):157. doi: 10.1186/s13075-021-02532-9
32. Paulissen SM, van Hamburg JP, Dankers W, Lubberts E. The Role and Modulation of Ccr6+ Th17 Cell Populations in Rheumatoid Arthritis. Cytokine (2015) 74(1):43–53. doi: 10.1016/j.cyto.2015.02.002
33. Restorick SM, Durant L, Kalra S, Hassan-Smith G, Rathbone E, Douglas MR, et al. Ccr6(+) Th Cells in the Cerebrospinal Fluid of Persons With Multiple Sclerosis Are Dominated by Pathogenic Non-Classic Th1 Cells and Gm-Csf-Only-Secreting Th Cells. Brain Behav Immun (2017) 64:71–9. doi: 10.1016/j.bbi.2017.03.008
34. Maggi L, Mazzoni A, Cimaz R, Liotta F, Annunziato F, Cosmi L. Th17 and Th1 Lymphocytes in Oligoarticular Juvenile Idiopathic Arthritis. Front Immunol (2019) 10:450. doi: 10.3389/fimmu.2019.00450
35. Li Y, Wei C, Xu H, Jia J, Wei Z, Guo R, et al. The Immunoregulation of Th17 in Host Against Intracellular Bacterial Infection. Mediators Inflamm (2018) 2018:6587296. doi: 10.1155/2018/6587296
36. Najafi S, Mirshafiey A. The Role of T Helper 17 and Regulatory T Cells in Tumor Microenvironment. Immunopharmacol Immunotoxicol (2019) 41(1):16–24. doi: 10.1080/08923973.2019.1566925
37. Pan B, Shen J, Cao J, Zhou Y, Shang L, Jin S, et al. Interleukin-17 Promotes Angiogenesis by Stimulating Vegf Production of Cancer Cells Via the Stat3/Giv Signaling Pathway in Non-Small-Cell Lung Cancer. Sci Rep (2015) 5:16053. doi: 10.1038/srep16053
38. Yang B, Kang H, Fung A, Zhao H, Wang T, Ma D. The Role of Interleukin 17 in Tumour Proliferation, Angiogenesis, and Metastasis. Mediators Inflammation (2014) 2014:623759. doi: 10.1155/2014/623759
39. Kwiecien I, Polubiec-Kownacka M, Dziedzic D, Wolosz D, Rzepecki P, Domagala-Kulawik J. Cd163 and Ccr7 as Markers for Macrophage Polarization in Lung Cancer Microenvironment. Central-European J Immunol (2019) 44(4):395–402. doi: 10.5114/ceji.2019.92795
40. Kwiecien I, Rutkowska E, Polubiec-Kownacka M, Raniszewska A, Rzepecki P, Domagala-Kulawik J. Blood Monocyte Subsets With Activation Markers in Relation With Macrophages in Non-Small Cell Lung Cancer. Cancers (2020) 12(9):2513. doi: 10.3390/cancers12092513
41. Kwiecien I, Skirecki T, Polubiec-Kownacka M, Raniszewska A, Domagala-Kulawik J. Immunophenotype of T Cells Expressing Programmed Death-1 and Cytotoxic T Cell Antigen-4 in Early Lung Cancer: Local Vs. Systemic Immune Response. Cancers (2019) 11(4):567. doi: 10.3390/cancers11040567
42. Detterbeck FC, Boffa DJ, Kim AW, Tanoue LT. The Eighth Edition Lung Cancer Stage Classification. Chest (2017) 151(1):193–203. doi: 10.1016/j.chest.2016.10.010
43. Postmus PE, Kerr KM, Oudkerk M, Senan S, Waller DA, Vansteenkiste J, et al. Early and Locally Advanced Non-Small-Cell Lung Cancer (Nsclc): Esmo Clinical Practice Guidelines for Diagnosis, Treatment and Follow-Up. Ann Oncol (2017) 28(suppl_4):iv1–iv21. doi: 10.1093/annonc/mdx222
44. Planchard D, Popat S, Kerr K, Novello S, Smit EF, Faivre-Finn C, et al. Metastatic Non-Small Cell Lung Cancer: Esmo Clinical Practice Guidelines for Diagnosis, Treatment and Follow-Up. Ann Oncol (2018) 29(Suppl 4):iv192–237. doi: 10.1093/annonc/mdy275
45. Domagala-Kulawik J, Osinska I. Immune Alterations in Lung Cancer - the New Therapeutic Approach. Pneumonol Alergol Pol (2014) 82(3):286–99. doi: 10.5603/PiAP.2014.0034
46. Domagala-Kulawik J, Osinska I, Hoser G. Mechanisms of Immune Response Regulation in Lung Cancer. Trans Lung Cancer Res (2014) 3(1):15–22. doi: 10.3978/j.issn.2218-6751.2013.11.03
47. Aerts JG, Hegmans JP. Tumor-Specific Cytotoxic T Cells Are Crucial for Efficacy of Immunomodulatory Antibodies in Patients With Lung Cancer. Cancer Res (2013) 73(8):2381–8. doi: 10.1158/0008-5472.CAN-12-3932
48. Kataki A, Scheid P, Piet M, Marie B, Martinet N, Martinet Y, et al. Tumor Infiltrating Lymphocytes and Macrophages Have a Potential Dual Role in Lung Cancer by Supporting Both Host-Defense and Tumor Progression. J Lab Clin Med (2002) 140(5):320–8. doi: 10.1067/mlc.2002.128317
49. Martinez-Lostao L, Anel A, Pardo J. How Do Cytotoxic Lymphocytes Kill Cancer Cells? Clin Cancer Res (2015) 21(22):5047–56. doi: 10.1158/1078-0432.CCR-15-0685
50. Martin MD, Badovinac VP. Defining Memory Cd8 T Cell. Front Immunol (2018) 9:2692. doi: 10.3389/fimmu.2018.02692
51. Geginat J, Sallusto F, Lanzavecchia A. Cytokine-Driven Proliferation and Differentiation of Human Naive, Central Memory, and Effector Memory Cd4(+) T Cells. J Exp Med (2001) 194(12):1711–9. doi: 10.1084/jem.194.12.1711
52. Raniszewska A, Kwiecien I, Sokolowski R, Rutkowska E, Domagala-Kulawik J. Immunomodulatory Molecules on Lung Cancer Stem Cells From Lymph Nodes Aspirates. Cancers (Basel) (2020) 12(4):838. doi: 10.3390/cancers12040838
53. Raniszewska A, Vroman H, Dumoulin D, Cornelissen R, Aerts J, Domagala-Kulawik J. Pd-L1(+) Lung Cancer Stem Cells Modify the Metastatic Lymph-Node Immunomicroenvironment in Nsclc Patients. Cancer Immunol Immunother (2021) 70(2):453–61. doi: 10.1007/s00262-020-02648-y
54. Raniszewska A, Kwiecien I, Rutkowska E, Rzepecki P, Domagala-Kulawik J. Lung Cancer Stem Cells-Origin, Diagnostic Techniques and Perspective for Therapies. Cancers (Basel) (2021) 13(12). doi: 10.3390/cancers13122996
55. Kwiecien I, Rutkowska E, Raniszewska A, Sokolowski R, Bednarek J, Jahnz-Rozyk K, et al. Immunosuppressive Properties of Human Pd-1 + , Pdl-1 + and Cd80 + Dendritic Cells From Lymph Nodes Aspirates of Lung Cancer Patients. Cancer Immunol Immunother (2022)13:2996. doi: 10.1007/s00262-022-03178-5
56. Hu G, Wang S. Tumor-Infiltrating Cd45ro(+) Memory T Lymphocytes Predict Favorable Clinical Outcome in Solid Tumors. Sci Rep (2017) 7(1):10376. doi: 10.1038/s41598-017-11122-2
57. Principe N, Kidman J, Goh S, Tilsed CM, Fisher SA, Fear VS, et al. Tumor Infiltrating Effector Memory Antigen-Specific Cd8(+) T Cells Predict Response to Immune Checkpoint Therapy. Front Immunol (2020) 11:584423. doi: 10.3389/fimmu.2020.584423
58. Manjarrez-Orduno N, Menard LC, Kansal S, Fischer P, Kakrecha B, Jiang C, et al. Circulating T Cell Subpopulations Correlate With Immune Responses at the Tumor Site and Clinical Response to Pd1 Inhibition in Non-Small Cell Lung Cancer. Front Immunol (2018) 9:1613. doi: 10.3389/fimmu.2018.01613
59. Li X, Wenes M, Romero P, Huang SC, Fendt SM, Ho PC. Navigating Metabolic Pathways to Enhance Antitumour Immunity and Immunotherapy. Nat Rev Clin Oncol (2019) 16(7):425–41. doi: 10.1038/s41571-019-0203-7
60. Ohue Y, Nishikawa H. Regulatory T (Treg) Cells in Cancer: Can Treg Cells Be a New Therapeutic Target? Cancer Sci (2019) 110(7):2080–9. doi: 10.1111/cas.14069
61. Amarillo D, Brugnini A, Trias N, Rodríguez M, Rodríguez V, Salisbury S, et al. Fp12.08 the Survival Impact of Circulating T Regulatory Cells Subsets in Patients With Untreated Lung Cancer. Int Assoc Study Lung Cancer (2021) 16(3):S220. doi: 10.1016/j.jtho.2021.01.134
62. Wang W, Hodkinson P, McLaren F, MacKinnon A, Wallace W, Howie S, et al. Small Cell Lung Cancer Tumour Cells Induce Regulatory T Lymphocytes, and Patient Survival Correlates Negatively With Foxp3+ Cells in Tumour Infiltrate. Int J Cancer (2012) 131(6):E928–37. doi: 10.1002/ijc.27613
63. Miyara M, Yoshioka Y, Kitoh A, Shima T, Wing K, Niwa A, et al. Functional Delineation and Differentiation Dynamics of Human Cd4+ T Cells Expressing the Foxp3 Transcription Factor. Immunity (2009) 30(6):899–911. doi: 10.1016/j.immuni.2009.03.019
64. Booth NJ, McQuaid AJ, Sobande T, Kissane S, Agius E, Jackson SE, et al. Different Proliferative Potential and Migratory Characteristics of Human Cd4+ Regulatory T Cells That Express Either Cd45ra or Cd45ro. J Immunol (2010) 184(8):4317–26. doi: 10.4049/jimmunol.0903781
65. Hirota K, Martin B, Veldhoen M. Development, Regulation and Functional Capacities of Th17 Cells. Semin Immunopathol (2010) 32(1):3–16. doi: 10.1007/s00281-009-0187-y
66. Zambrano-Zaragoza JF, Romo-Martinez EJ, Duran-Avelar Mde J, Garcia-Magallanes N, Vibanco-Perez N. Th17 Cells in Autoimmune and Infectious Diseases. Int J Inflamm (2014) 2014:651503. doi: 10.1155/2014/651503
67. Carmi Y, Rinott G, Dotan S, Elkabets M, Rider P, Voronov E, et al. Microenvironment-Derived Il-1 and Il-17 Interact in the Control of Lung Metastasis. J Immunol (2011) 186(6):3462–71. doi: 10.4049/jimmunol.1002901
68. Li Q, Han Y, Fei G, Guo Z, Ren T, Liu Z. Il-17 Promoted Metastasis of Non-Small-Cell Lung Cancer Cells. Immunol Lett (2012) 148(2):144–50. doi: 10.1016/j.imlet.2012.10.011
69. Chen X, Wan J, Liu J, Xie W, Diao X, Xu J, et al. Increased Il-17-Producing Cells Correlate With Poor Survival and Lymphangiogenesis in Nsclc Patients. Lung Cancer (2010) 69(3):348–54. doi: 10.1016/j.lungcan.2009.11.013
70. Wilke CM, Kryczek I, Wei S, Zhao E, Wu K, Wang G, et al. Th17 Cells in Cancer: Help or Hindrance? Carcinogenesis (2011) 32(5):643–9. doi: 10.1093/carcin/bgr019
71. Chang SH. T Helper 17 (Th17) Cells and Interleukin-17 (Il-17) in Cancer. Arch Pharmacal Res (2019) 42(7):549–59. doi: 10.1007/s12272-019-01146-9
72. Li Y, Cao ZY, Sun B, Wang GY, Fu Z, Liu YM, et al. Effects of Il-17a on the Occurrence of Lung Adenocarcinoma. Cancer Biol Ther (2011) 12(7):610–6. doi: 10.4161/cbt.12.7.16302
73. Xu C, Hao K, Yu L, Zhang X. Serum Interleukin-17 as a Diagnostic and Prognostic Marker for Non-Small Cell Lung Cancer. Biomarkers Biochem Indic Exposure Response Susceptibility to Chemicals (2014) 19(4):287–90. doi: 10.3109/1354750X.2014.908954
74. Kwiecien I, Rutkowska E, Raniszewska A, Rzepecki P, Domagala-Kulawik J. Modulation of the Immune Response by Heterogeneous Monocytes and Dendritic Cells in Lung Cancer. World J Clin Oncol (2021) 12(11):966–82. doi: 10.5306/wjco.v12.i11.966
75. Ogino S, Galon J, Fuchs CS, Dranoff G. Cancer Immunology–Analysis of Host and Tumor Factors for Personalized Medicine. Nat Rev Clin Oncol (2011) 8(12):711–9. doi: 10.1038/nrclinonc.2011.122
76. Domagala-Kulawik J, Kwiecien I, Pankowski J, Pasieka-Lis M, Wolosz D, Zielinski M. Elevated Foxp3/Cd8 Ratio in Lung Adenocarcinoma Metastatic Lymph Nodes Resected by Transcervical Extended Mediastinal Lymphadenectomy. BioMed Res Int (2017) 2017:5185034. doi: 10.1155/2017/5185034
77. Ochsenbein AF, Klenerman P, Karrer U, Ludewig B, Pericin M, Hengartner H, et al. Immune Surveillance Against a Solid Tumor Fails Because of Immunological Ignorance. Proc Natl Acad Sci USA (1999) 96(5):2233–8. doi: 10.1073/pnas.96.5.2233
Keywords: lymph nodes, CD4+ cells, CD8+ cells, EBUS-TBNA, regulatory T cells, recent thymic emigrants cells, effector memory T cells, Th17- related cells
Citation: Kwiecień I, Rutkowska E, Sokołowski R, Bednarek J, Raniszewska A, Jahnz-Różyk K, Rzepecki P and Domagała-Kulawik J (2022) Effector Memory T Cells and CD45RO+ Regulatory T Cells in Metastatic vs. Non-Metastatic Lymph Nodes in Lung Cancer Patients. Front. Immunol. 13:864497. doi: 10.3389/fimmu.2022.864497
Received: 28 January 2022; Accepted: 07 April 2022;
Published: 02 May 2022.
Edited by:
Eyad Elkord, University of Salford, United KingdomReviewed by:
Mehrnoosh Doroudchi, Shiraz University of Medical Sciences, IranNirupama Darshan Verma, University of New South Wales, Australia
Copyright © 2022 Kwiecień, Rutkowska, Sokołowski, Bednarek, Raniszewska, Jahnz-Różyk, Rzepecki and Domagała-Kulawik. This is an open-access article distributed under the terms of the Creative Commons Attribution License (CC BY). The use, distribution or reproduction in other forums is permitted, provided the original author(s) and the copyright owner(s) are credited and that the original publication in this journal is cited, in accordance with accepted academic practice. No use, distribution or reproduction is permitted which does not comply with these terms.
*Correspondence: Iwona Kwiecień, ikwiecien@wim.mil.pl; kwiecieniwi@gmail.com