- 1Department of Medical Oncology, the First Affiliated Hospital of Xi’an Jiaotong University, Xi’an, China
- 2Department of Breast Surgery, the First Affiliated Hospital of Xi’an Jiaotong University, Xi’an, China
- 3Department of Gastroenterology, the First Affiliated Hospital of Xi’an Jiaotong University, Xi’an, China
- 4Department of Clinical Laboratory, the 940th Hospital of Joint Logistics Support Force of Chinese People’s Liberation Army, Key Laboratory of Stem Cells and Gene Drug in Gansu Province, Lanzhou, China
- 5Center for Translational Medicine, the First Affiliated Hospital of Xi’an Jiaotong University, Xi’an, China
- 6Key Laboratory for Tumor Precision Medicine of Shaanxi Province, Xi’an, China
Background: The therapeutic effect of immune checkpoint inhibitors on tumors is not only related to CD8+ effector T cells but also sufficiently related to CD4+ helper T (TH) cells. The immune characteristics of breast cancer, including gene characteristics and tumor-infiltrating lymphocytes, have become significant biomarkers for predicting prognosis and immunotherapy response in recent years.
Methods: Breast cancer samples from The Cancer Genome Atlas (TCGA) database and triple-negative breast cancer (TNBC) samples from GSE31519 in the Gene Expression Omnibus (GEO) database were extracted and clustered based on gene sets representing TH cell signatures. CIBERSORT simulations of immune cell components in the tumor microenvironment and gene set enrichment analyses (GSEAs) were performed in the different clusters to verify the classification of the subtypes. The acquisition of differentially expressed genes (DEGs) in the different clusters was further used for Gene Ontology (GO) and Kyoto Encyclopedia of Genes and Genomes (KEGG) analyses. The clinical information from different clusters was used for survival analysis. Finally, the surgical tissues of TNBC samples were stained by immunofluorescence staining and Masson’s trichrome staining to explore the correlation of TH cell subtypes with extracellular matrix (ECM).
Results: The breast cancer samples from the datasets in TCGA database and GEO database were classified into TH-activated and TH-silenced clusters, which was verified by the immune cell components and enriched immune-related pathways. The DEGs of TH-activated and TH-silenced clusters were obtained. In addition to TH cells and other immune-related pathways, ECM-related pathways were found to be enriched by DEGs. Furthermore, the survival data of TCGA samples and GSE31519 samples showed that the 10-year overall survival (p-value < 0.001) and 10-year event-free survival (p-value = 0.162) of the TH-activated cluster were better, respectively. Fluorescent labeling of TH cell subtypes and staining of the collagen area of surgical specimens further illustrated the relationship between TH cell subtypes and ECM in breast cancer, among which high TH1 infiltration was related to low collagen content (p-value < 0.001), while high TH2 and Treg infiltration contained more abundant collagen (p-value < 0.05) in TNBC. With regard to the relationship of TH cell subtypes, TH2 was positively correlated with Treg (p-value < 0.05), while TH1 was negatively correlated with both of them.
Conclusions: The immune and ECM characteristics of breast cancer subtypes based on TH cell characteristics were revealed, and the relationship between different TH cell subsets and ECM and prognosis was explored in this study. The crosstalk between ECM and TH cell subtypes formed a balanced TME influencing the prognosis and treatment response in breast cancer, which suggests that the correlation between TH cells and ECM needs to be further emphasized in future breast cancer studies.
Introduction
The emergence of immunotherapy brought solid tumors to a new era (1), especially in breast cancer, which has the highest incidence (2). Currently, many clinical studies related to immune checkpoint inhibitors (ICIs) mainly focus on the regulation of CD8+ cytotoxic T cells (CTLs) on the tumor immune microenvironment (TIME) and the possible mechanism of immune-related targets, for example, IMpassion 130 (3), KEYNOTE-119 (4), and the FUTURE trial (5) for advanced breast cancer and KEYNOTE-173 (6) and IMpassion 031 (7) for early breast cancer. In reality, as the principal component of tumor-infiltrating lymphocytes (TILs), T lymphocytes play a key role in the occurrence and development of breast cancer, especially in triple-negative breast cancer (TNBC) (8, 9). Among TILs, not only CTLs but also CD4+ helper T (TH) cells directly or indirectly exert protumorigenic or/and antitumorigenic immune effects by affecting other immune cells, especially CTLs, through the inflammatory molecules secreted by different subtypes of TH cells and the accommodation of signal transduction (10, 11). It is precisely the various and interlaced immunoregulatory properties of TH cells that make the optimization of immunotherapy based on them more clinically significant. Based on previous studies (12–15), the conference on TH1 (T helper type 1), TH2 (T helper type 2), TH17 (T helper type 17), Tfh (T follicular helper), and Treg (CD4+ regulatory T) cells as a group of CD4+ TH cells that are independent and have a chain reaction with TILs is very meaningful to avoid tumor immune escape and improve the efficacy of immunotherapy for breast cancer.
Apart from immune cells, cancer-associated fibroblasts (CAFs) are also prominent components of the tumor microenvironment (TME) (16, 17), and the activation of cytokines such as interleukin-1 (IL-1) and IL-6 and immune-related pathways such as the Janus kinase-signal transducer and activator of transcription (JAK-STAT) and nuclear factor kappa-B (NF-κb) pathways plays an important role in the generation and recruitment of CAFs (18, 19). In fibrosis-related diseases, including tumors, each subtype of TH cells has different regulatory effects on fibroblast-induced collagen synthesis through inflammatory factors, including interferon-γ (IFN-γ) (20). In turn, CAFs also regulate the activation and function of TH cells by secreting cytokines and chemokines (16, 21). The extracellular matrix (ECM) structured through collagen produced by CAFs in the TME not only affects the differentiation of T cells but also affects the spatial distribution of T cells (22) to modulate antitumorigenic immunity by influencing the dialog between T cells and tumor cells.
Based on TIME-related characteristics as biomarkers for breast cancer prognosis and prediction of immunotherapy efficacy, many studies (23–26) have proposed prognostic models and detailed breast cancer typing in recent years. A total score of the immune microenvironment as indicators of the classification for the data samples was employed in most of them (27, 28). In contrast, this study will utilize the genetic traits of TH cells, such as heterogeneous and particular cells, and explore the characteristics of the TIME and ECM in breast cancer to put forward a reference for the breakthrough of immunotherapy in the field of TH cells.
Materials and Methods
Datasets and Clinical Samples
RNA-sequencing data of 1,097 breast cancer patients from The Cancer Genome Atlas (TCGA) database and the corresponding clinical data were extracted. Microarray gene expression data using the Affymetrix U133A array of 64 TNBC patients from GSE31519 were obtained from the National Center for Biotechnology Information (NCBI) in the Gene Expression Omnibus (GEO) Database, in which clinical survival data are available. The data from the above public databases were used for cluster analysis based on TH cell characteristics and for differential analysis and survival analysis among different clusters.
Formalin-fixed paraffin-embedded (FFPE) surgical tissue sections (4 µm thick) from 30 TNBC patients admitted to the Department of Medical Oncology, The First Affiliated Hospital of Xi’an Jiaotong University during 2016–2020 were used for immunofluorescent staining and Masson’s trichrome staining, which is for the analysis of different TH subtypes and collagen content in the TIME of TNBC patients. The detailed clinical information of patients is described in Supplementary Table S1, in which disease-free survival (DFS) was defined as the time from surgery to the occurrence of the first metastasis.
Identification of Subtypes Based on TH Cell Characteristics in Breast Cancer
Consensus cluster analysis was employed to identify breast cancer subtypes based on TH cell characteristics obtained from the single-sample gene set enrichment analysis (ssGSEA) (29, 30). The gene set representing five TH cell subtypes, including TH1, TH2, TH17, Tfh, and Treg cells, was used for clustering, as shown in Supplementary Table S2. The “ConsensusClusterPlus” package (http://www.bioconductor.org/) was used to divide the datasets with duplicate samples removed (1,090 in the TCGA dataset and 64 in the GSE31519 dataset, as shown in Supplementary Tables S3, S4, respectively) into k=2–9 subgroups followed by hierarchical agglomerative consensus, and optimal clustering was obtained by comparing the consensus matrix and cumulative distribution function (CDF) value.
Evaluation of TIME in Breast Cancer Samples From Datasets
The CIBERSORT deconvolution algorithm was used to estimate the fraction of 22 immune cell types in each sample to evaluate TIME in breast cancer (31), which was calculated via the online calculator (https://cibersort.stanford.edu/). The CIBERSORT results were filtered by p-value < 0.05 to obtain more accurate prediction results, and the samples upon the filter conditions were employed in subsequent differential analysis between different clusters (846 in TCGA dataset and 63 in GSE31519 dataset as shown in Supplementary Tables S5, S6, respectively). Principal component analysis (PCA) was used to distinguish immune cell components between different clusters through dimensionality reduction, pattern recognition, and exploratory visualization.
Gene Set Enrichment Analysis
GSEA was performed to compare the gene expression of different clusters by using GSEA version 4.1.0 provided by the Broad Institute (http://software.broadinstitute.org/gsea/index.jsp). Kyoto Encyclopedia of Genes and Genomes (KEGG) pathway enrichment analysis obtained through gene sets from the Broad Institute (http://ftp.broadinstitute.org://pub/gsea/gene_sets/c2.cp.kegg.v6.2.symbols.gmt) was used to determine the enriched pathways of clusters. In GSEA, the significance of each pathway was classified by a threshold of false discovery rate (FDR) q-value <0.05.
Extraction of Differentially Expressed Genes, Gene Ontology, and KEGG Pathway Enrichment Analysis of DEGs
TH-related differentially expressed genes (DEGs) were extracted and analyzed using the R packages “Limma,” “Impute,” and “EdgeR” through the gene expression profiles of different clusters. DEGs were filtered by logFCfilter=2 or 1 and fdrFilter=0.05. Gene Ontology (GO) analysis was conducted for the high-throughput annotation of biological functions (BPs), cellular components (CCs), and molecular function (MF) of the DEGs between different clusters, while KEGG analysis was conducted for the molecular and pathway levels of the DEGs. The R packages “DOSE,” “ClusterProfiler,” “Org.Hs.eg.db,” and “Enrichplot” were used in the GO and KEGG pathway enrichment analyses of DEGs (p-value < 0.05).
Tissue Immunofluorescent Staining and Masson’s Trichrome Staining
The FFPE tissue sections were deparaffinized to water using graded ethanol-dimethylbenzene, employed in ethylenediaminetetraacetic acid (EDTA) buffer (pH 8.0, ZHHC, PI001) for 15 min to recover the antigen, incubated in Triton X-100 (ZHHC, PI024) for 5 min to implement a permeable membrane, and sealed with bovine serum albumin (BSA). To label the TH1, TH2, and Treg subsets of the TIME in TNBC, sections were incubated overnight at 4 °C with primary antibodies against T-bet (Abcam, ab150440, 1:30), GATA-3 (Abcam, ab199428, 1:30), and CD25 (Abcam, ab231441, 1:50) and CD4 (Proteintech, 67786-1-Ig, 1:200). The sections were washed with phosphate-buffered saline (PBS) and incubated with the secondary antibody (Proteintech, SA00003-1 and SA00009-2, 1:50) at room temperature for 60 min. The nuclei were stained with 4,6-diamidino-2-phenylindole (DAPI) (ZHHC, CD110) for 3 min. The stained cells were observed, and images were acquired by a confocal laser scanning microscope (Leica Company) in at least 10 fields per section. For Masson’s trichrome staining, the deparaffinized sections were analyzed with a Masson’s trichrome stain kit (Solarbio, G1340) and were observed, and images were acquired by a Leica scanning optical microscope after dehydration, clearing, and mounting.
Survival Analysis
For plotting Kaplan–Meier (K–M) curves of TCGA and GSE31519 clusters based on TH cell characteristics, the R packages “survival” and “survminer” were used, while the log-rank test was employed to assess the significance of overall survival (OS) and event-free survival (EFS) differences. Similarly, K–M survival analysis and log-rank tests were conducted to compare the differences in DFS between the different groups of TH cell subtypes in 30 TNBC samples.
Statistical Analysis
The statistical analyses of data from the public database were performed using R software (http:///www.r-project.org/) and Bioconductor (http://bioconductor.org/). To compare any two groups of datasets in the study, the Wilcoxon test was conducted using R software. The R packages “pheatmap,” “ggplot2,” and “vioplot” were used to show the differential results in the study. The statistical analyses of 30 TNBC samples collected by us were performed using GraphPad Prism 8.0.0 (GraphPad Software Inc., San Diego, CA, USA). The non-parametric independent sample t-test was used for the difference in the different groupings of TH cell subtypes, and Pearson’s correlation was used for the correlation between different TH cell subtypes, in which the data were all normally distributed. All p-values were bilateral, and a p-value < 0.05 was considered statistically significant.
Results
Identification of Subtypes by TH Cells and the Corresponding TIME in Breast Cancer
The transcriptome and clinical data of 1,097 breast cancer samples from TCGA database were extracted, and the mRNA expression of 1,090 breast cancer samples after the removal of duplicate samples was analyzed. According to the expression of TH-cell-related gene characteristics, 1,090 samples were collected for cluster analysis. From the clustering results of k = 2–9, it can be found that when the samples were clustered into two clusters (k = 2, Cluster 1 included 654 samples and Cluster 2 included 436 samples, see Supplementary Table S3 for details), the consensus matrix had a relatively average distribution, less matrix overlap, and a smoothly decreasing CDF value (Figure 1A; Supplementary Figures S1A–D). In addition, we extracted the transcriptomic data of 64 TNBC samples from the GSE31519 dataset of the GEO database for the same cluster analysis as above (Figure 1B; Supplementary Figures S2A–D), which was still optimal when the samples were divided into two clusters (k=2, Cluster 1 included 38 samples and Cluster 2 included 26 samples; see Supplementary Table S4 for details).
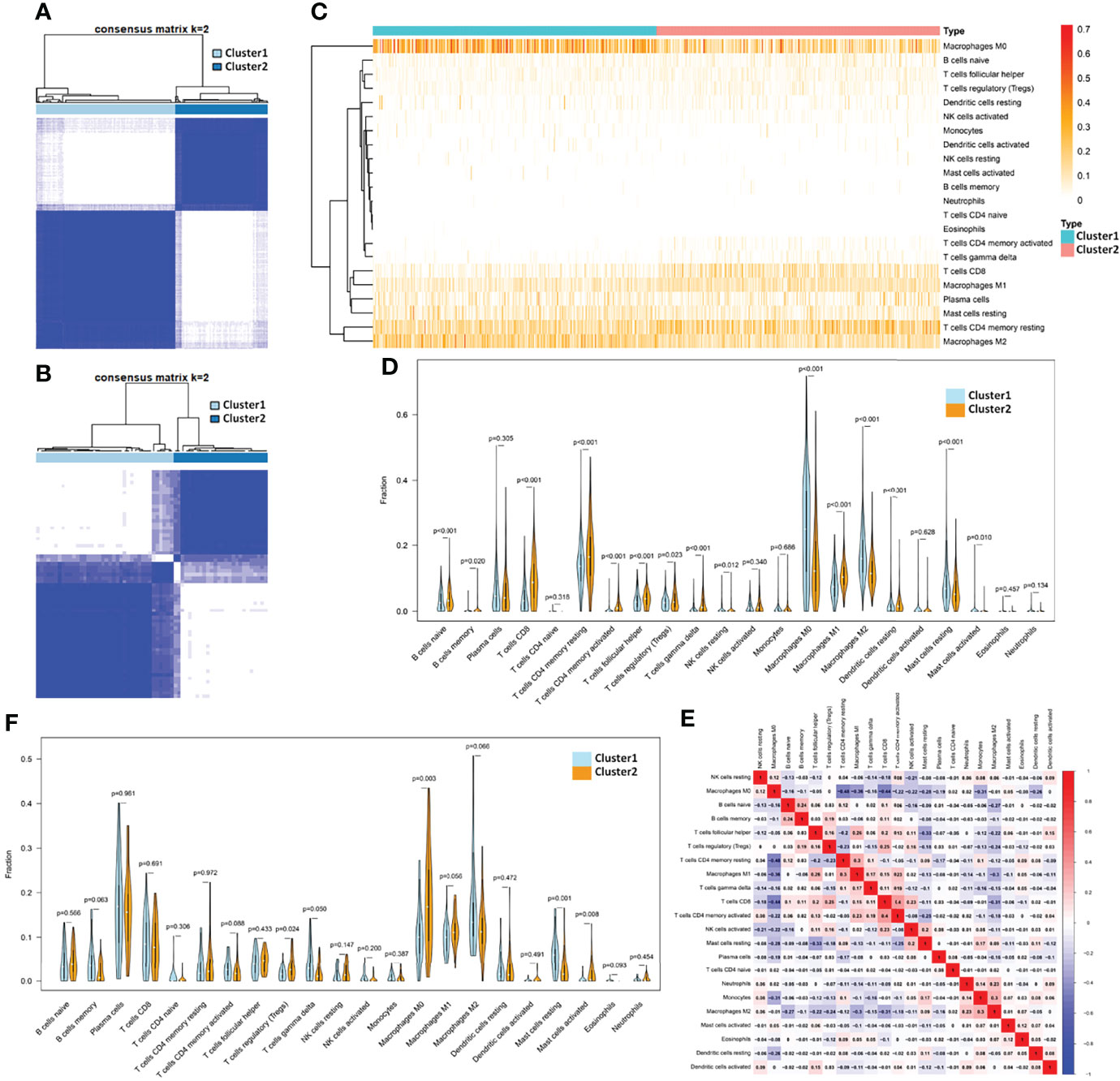
Figure 1 Identification of subtypes by TH cells and the corresponding TIME in breast cancer. (A) The consensus matrix when k =2 through cluster analysis based on the expression of TH cell-related gene characteristics in 1,090 samples from TCGA database and (B) 64 samples from GSE31519 dataset. Heatmap (C) and violinplot (D) of the fraction of 22 kinds of immune cells in the TIME of the two clusters obtained from the TCGA database. (E) Heatmap of the correlation of 22 immune cell components obtained from the TCGA database. (F) Violinplot of TIME in the two clusters from the GSE31519 dataset.
The deconvolution algorithm CIBERSORT was used to verify the immune microenvironment of the two clusters obtained from the TCGA dataset by simulating the fraction of 22 kinds of immune cells in the TIME of each sample (see Supplementary Table S5 for details). Differential analysis of immune cell components showed that Cluster 2 had more resting memory CD4 T cells, activated memory CD4 T cells, Tfh cells, gamma delta T cells (p-value < 0.001), and Treg (p value = 0.023) than Cluster 1. Therefore, Cluster 1 was defined as a TH-silenced cluster, and Cluster 2 was defined as a TH-activated cluster. Except for the above TH cells, the TH-activated cluster showed significantly more infiltration containing CD8 T cells, naive B cells, memory B cells, M1 macrophages, and resting dendritic cells and less infiltration of M0 macrophages, M2 macrophages, and resting mast cells (Figures 1C,D). In the TCGA samples, the correlation of 22 immune cell components can be seen in Figure 1E, in which CD8 T cells have a strong positive correlation with Treg cells and activated memory CD4 T cells, and M1 macrophages were positively correlated with Tfh cells and resting memory CD4 T cells, while M2 macrophages showed the opposite correlation. Similarly, CIBERSORT was used to verify the TIME of the samples in the GSE31519 dataset (see Supplementary Table S6 for details), from which we found that Tfh cells, Treg, and M1 macrophages were more abundant in Cluster 2 than in Cluster 1, while M2 macrophages and resting mast cells were less abundant, and the differences in other immune cells were not statistically significant (Figure 1F). Therefore, Cluster 1 in the GSE31519 dataset was still defined as the TH-silenced cluster, and Cluster 2 was defined as the TH-activated cluster.
Verification Towards the Characteristics of the Two Clusters Identified by TH Cells
A total of 1,090 samples classified into TH-silenced clusters and TH-activated clusters were evaluated for immune cell components using PCA. The two clusters were clearly separated (Figure 2A). The transcriptome data were prepared for the KEGG pathway-related GSEA, in which the pathways of normalized enrichment score (NES) top 15 in the two clusters were selected for demonstration. Activation of immune-related pathways was enriched in the TH-activated cluster, including the T cell, B-cell receptor signaling, and cytokine and chemokine signaling pathways (Figure 2B). This further verified the stronger immunogenicity and immune activity of the samples in the TH-activated cluster than in the TH-silenced cluster. The pathways enriched in the TH-silenced cluster included glucose metabolism-, amino acid metabolism- and fatty acid synthesis-related pathways (Figure 2C).
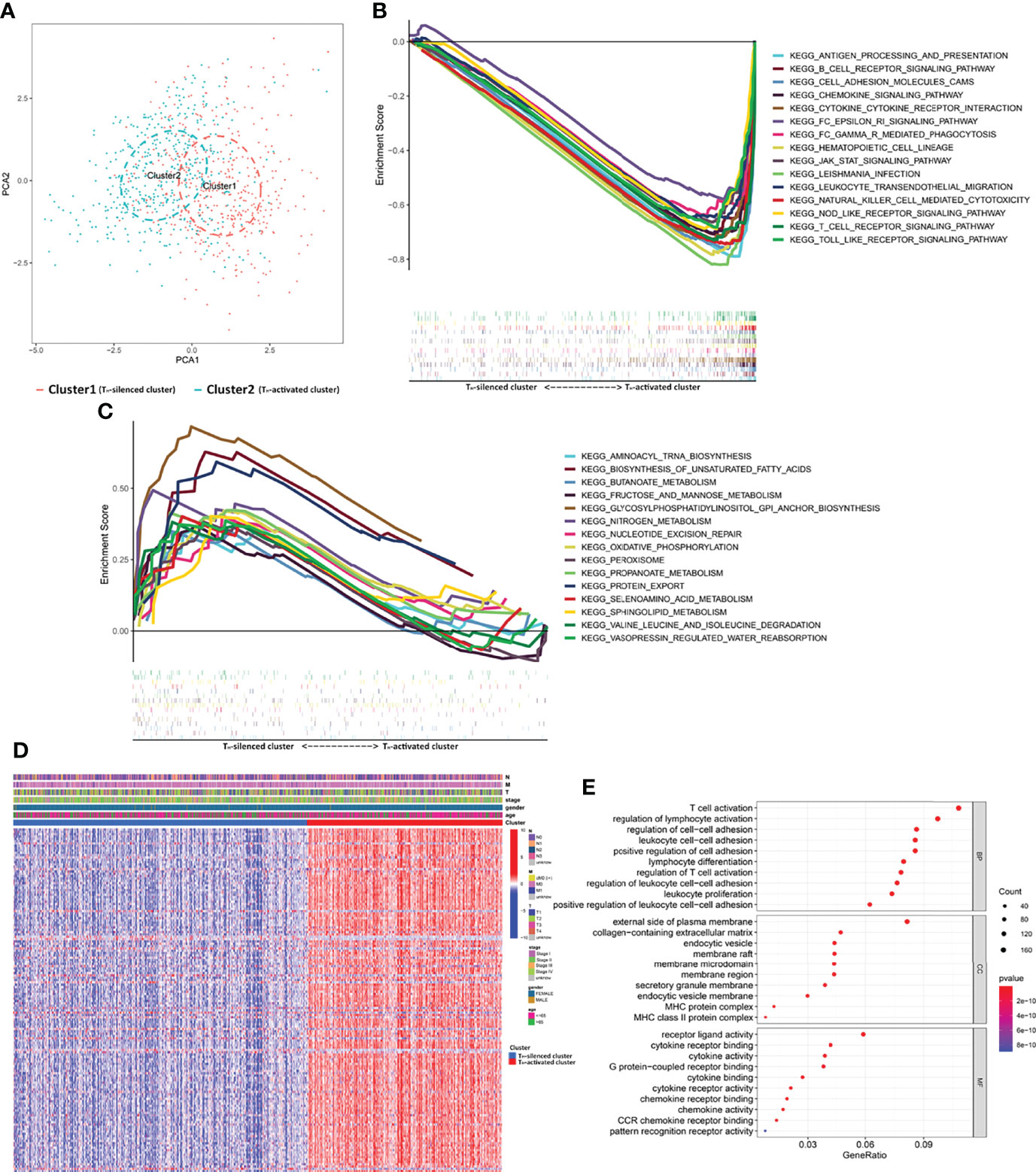
Figure 2 The gene profile characteristics of two clusters identified by TH cells from TCGA breast cancer samples cued the correlation between TH cells and ECM in the TIME. (A) PCA diagram of 1,090 samples classified into TH-silenced cluster and TH-activated cluster. (B) GSEA showed that the pathways of NES top 15 in the TH-activated cluster included abundant immune-related KEGG pathways, while (C) top 15 in the TH-silenced cluster included glucose metabolism- and fatty acid synthesis-related pathways. (D) The heatmap of differential expression of the DEGs in the two clusters (logFCfilter=2 and fdrFilter=0.05) and the distribution of clinical information. (E) GO enrichment analysis of the DEGs showed that collagen-containing ECM was enriched except for the immune-activated GO.
The Pathways Enriched by DEGs of Two Clusters Cued the Correlation Between TH Cells and ECM
Samples from the two clusters of TCGA database were used for gene expression differential analysis. When logFCfilter=2 and fdrFilter=0.05, 148 DEGs were obtained (see Supplementary Table S7 for details). The differential expression of these DEGs in the two clusters and the distribution of clinical information, including TNM stage, stage, age, and gender, are shown in Figure 2D. GO enrichment analysis (Figure 2E) showed that BP of the DEGs was most significantly related to the activation of T cells and differentiation and activation of lymphocytes, while the MF portion was also mostly cytokine related. In addition to the above manifestation that immune-activated GO is enriched with upregulation in TH-activated cluster versus TH-silenced cluster, collagen-containing ECM was enriched in the aspect of CC, indicating that synthesis of collagen and the construction of ECM may be correlated with TH cell activation.
A total of 310 DEGs were obtained between the transcriptional data of the samples in the two clusters from the GSE31519 dataset (see Supplementary Table S8, logFCfilter=1 and fdrFilter=0.05). The up- and downregulated expression of the 100 prominent DEGs between the two clusters is shown in the heatmap in Figure 3A. Furthermore, GO enrichment analysis of DEGs showed that ECM-related pathways were enriched in parts of BP, CC, and MF by comparing the TH-activated cluster versus the TH-silenced cluster, while cytokine activity was enriched in MF (Figures 3B, C). The possibility that ECM is associated with TH cells was reconfirmed by KEGG pathway enrichment analysis of these DEGs (Figures 3D, E), in which cytokine–cytokine receptor interaction and JAK-STAT signaling pathways related to immunity were enriched with upregulation, and ECM-receptor interaction was also enriched.
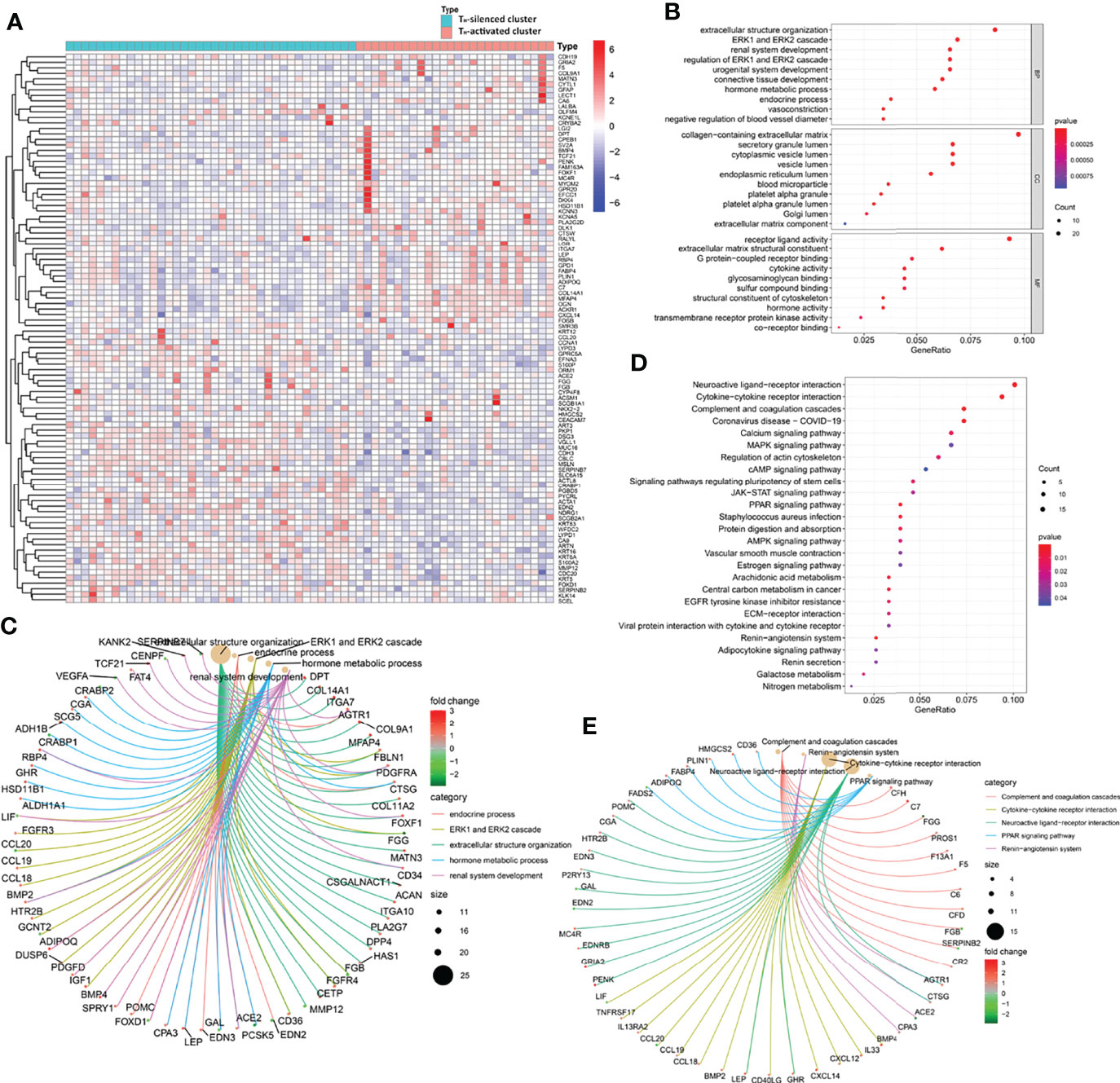
Figure 3 The gene profile characteristics of two clusters identified by TH cells from GSE31519 TNBC samples cued the correlation between TH cells and ECM in the TIME. (A) Heatmap of the differential expression of the 100 prominent DEGs between the two clusters from the GSE31519 dataset (logFCfilter=1 and fdrFilter=0.05). The bubble diagram (B) and circle diagram (C) showed that ECM-related pathways were enriched from GO enrichment analysis of the DEGs. The bubble diagram (D) and circle diagram (E) showed that cytokine–cytokine receptor interaction, JAK-STAT signaling pathway, and ECM–receptor interaction were enriched from KEGG enrichment analysis of the DEGs.
The Correlation Between Different TH Cell Subtypes and ECM
To further explore the content of tumor-infiltrating TH cell subtypes in breast cancer tissues, we performed immunofluorescence staining on paraffin tissue sections of primary foci from 30 TNBC patients, in which CD4+T-bet+ TH1, CD4+GATA3+ TH2, and CD4+CD25+ Treg cells were labeled (see Supplementary Table S1 for clinical information and corresponding TH cell content of these patients). According to the different contents of TH1, TH2, and Treg cells, 30 samples were divided into two groups for follow-up analysis (see Supplementary Figures S3A–C for the distribution after grouping of each TH cell subtype content). To explore the correlation between different subtypes of tumor-infiltrating TH cells and ECM in breast cancer, the collagen area in breast cancer tissues of grouping based on TH cell subtypes was analyzed for difference (the collagen area of each sample was the average value of three random fields after Masson’s trichrome staining, as shown in Supplementary Table S1). The breast cancer tissues with higher TH1 cell content had less collagen distribution; for example, a low tumor-infiltrating TH1 cell sample had abundant collagen, while a high tumor-infiltrating TH1 cell sample had the opposite distribution, as shown in Figure 4A. In contrast, as shown in Figures 4B, C, breast cancer tissues with higher tumor-infiltrating TH2 cells and Treg content were more collagen distributed. Furthermore, Pearson correlation analysis was conducted on the contents of the three subtypes of TH cells, and it was found that the content of TH2 was positively correlated with Treg (R2 = 0.3477, p-value < 0.001), while TH1 was negatively correlated with TH2 and Treg (p-value > 0.05), as shown in Figure 4D (the content of TH cell subtypes at each data point was the average value of three random fluorescence staining fields). Figures 4E–G are the t-test analyses of variance between the two groups of TH1 grouping (p-value < 0.001), TH2 grouping (p-value = 0.0246), and Treg grouping (p-value = 0.0326).
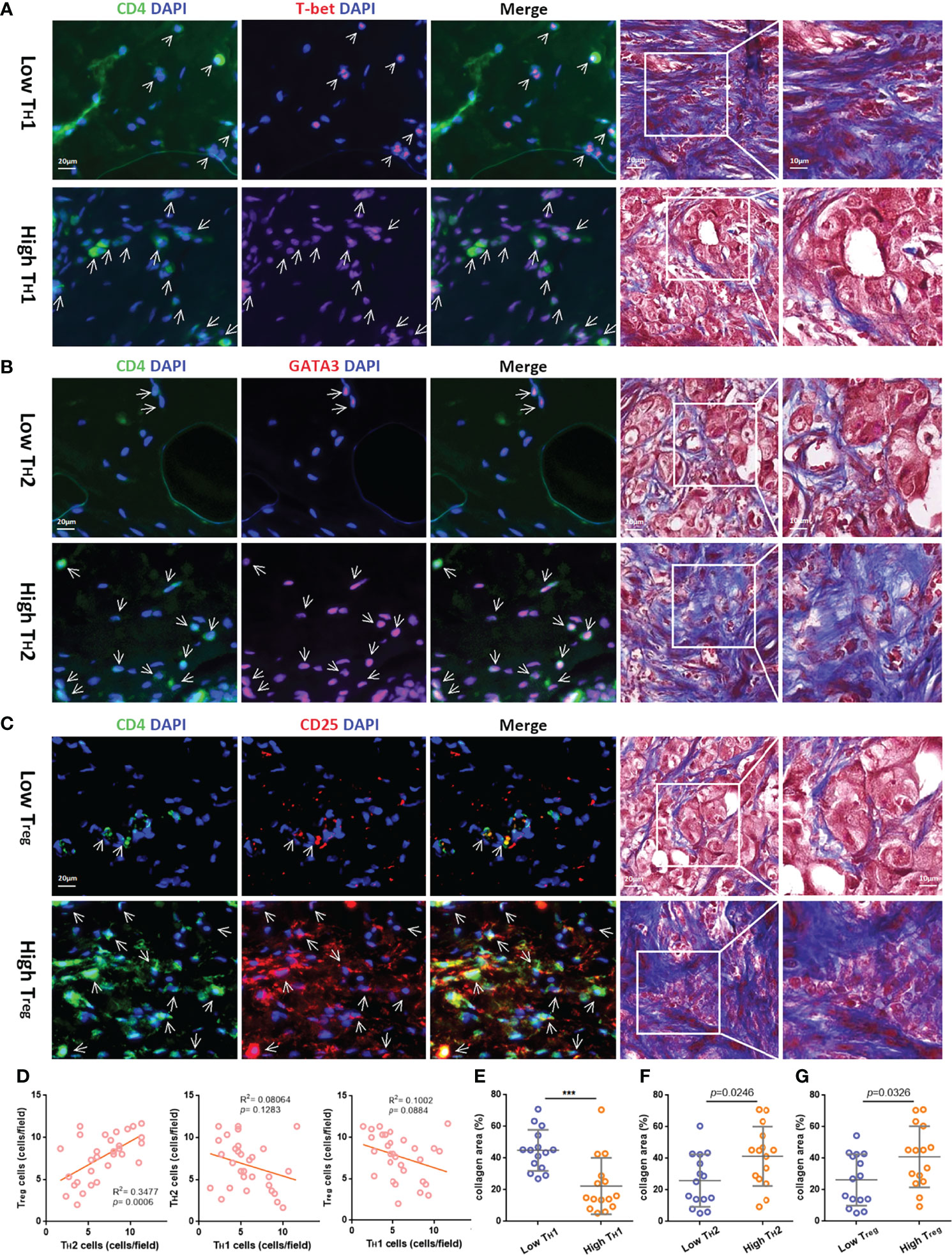
Figure 4 The relativity between different TH cell subtypes and the correlation of different TH cell subtypes and ECM in the TIME. Representative immunofluorescent staining for CD4 (green), T-bet, GATA3, or CD25 (red) and 4,6-diamidino-2-phenylindole (DAPI) (blue) (bar = 20 μm.) and corresponding Masson’s trichrome staining (bar = 10/20 μm.) in TNBC samples with low or high tumor-infiltrating TH1 (A), TH2 (B), and Treg cells (C), which are indicated by arrows. (D) Pearson correlation analysis of the three subtypes of TH cells. The t-test analysis of variance between the collagen area of two groups with low or high tumor-infiltrating TH1 (E), TH2 (F), and Treg cells (G). ***p value < 0.001.
The Prognosis Of Different Groupings Based on Total TH Cells and TH Cell Subtypes
To verify that the TH-activated cluster and TH-silenced cluster indicated significance for prognosis, K–M survival analysis was employed for samples from the public database. The 10-year OS of 1,090 samples from the TCGA database was compared in two clusters, of which the OS of the TH-activated cluster was longer (Figure 5A, p-value < 0.001). The 10-year EFS of 64 TNBC samples from the GSE31519 dataset was compared in two clusters, of which the EFS of the TH-activated cluster was longer (Figure 5B, p-value = 0.162).
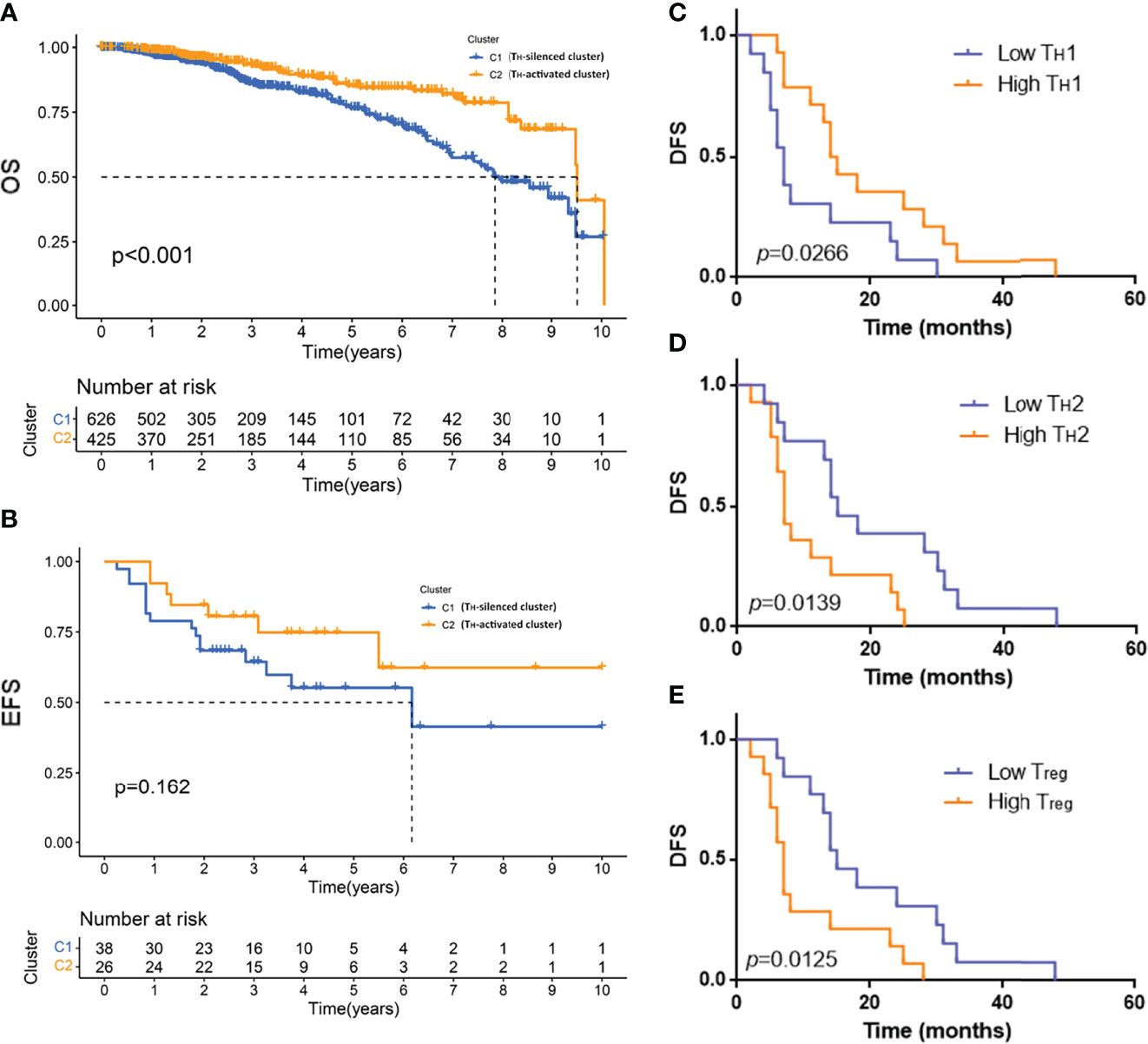
Figure 5 The prognosis of the TH-activated cluster and TH-silenced cluster in breast cancer and the prognosis of different groups based on TH cell subtypes. Kaplan–Meier (K–M) survival analysis was employed for 10-year overall survival (OS) of 1,090 samples from the TCGA database (A) and the 10-year event-free survival (EFS) of 64 TNBC samples from the GSE31519 dataset (B). K–M survival curve of disease-free survival (DFS) of 30 TNBC samples grouped by low or high tumor-infiltrating TH1 (C), TH2 (D), and Treg (E) cells. Two-tailed log-rank p-values are shown.
Based on the fluorescence staining results of TH cells of the 30 TNBC samples mentioned above, DFS of patients with different groupings of TH cell subtypes was employed to plot the K–M survival curve. Patients with high TH1 cell infiltration had a better prognosis with longer DFS, as shown in Figure 5C (p-value = 0.0266), while DFS was shorter in patients with high TH2 and high Treg cell infiltration, as shown in Figures 5D, E (TH2 grouping p-value = 0.0139, Treg grouping p-value = 0.0125).
Discussion
The predictive significance for treatment response and changes in tumor development of TILs as biomarkers have been an issue discussed in breast cancer as solid tumors. Many studies (32) have also classified breast cancer into high or low TIL subtypes to guide the treatment and prognosis of patients. In addition, a large number of studies (27, 33) have focused on the classification of breast cancer subtypes by immune score based on inflammatory factors and immune-cell-related genes in recent years. In the breast cancer subtype, TNBC was more widely treated with immunotherapy because of the emergence of effective biomarkers such as PD-L1 and well-founded classification of full inflamed (FI), stroma restricted (SR), margin restricted (MR), and immune desert (ID) subtypes (34). Therefore, in addition to all breast cancer samples of TCGA database, a TNBC dataset from GEO database was incorporated in this study, and the verification of TH cell subtypes was also conducted in TNBC samples. In other solid tumors, 65 combinations of T-cell markers have been used as indicators for determining the generation and early metastasis of colorectal cancer (35), while immune-related genes have also been used as gene sets for subtyping squamous cell carcinoma and lymphoma (36, 37). Regarding the role of subtyping and predicting the prognosis of breast cancer based on T cells, a study (38) showed that the gene score based on CD8+ T cells was associated with survival, especially in TNBC. In this study, a breast cancer dataset and a TNBC dataset were both divided into TH-silenced cluster and TH-activated cluster based on the gene characteristics of TH cells, among which two datasets had similar distributions of TH cell-related gene characteristics. The TH-activated cluster was characterized by upregulated immune-related gene characteristics, more activated immune-related pathways, and better prognosis, suggesting that the employment of TH cells as an independent biomarker for breast cancer could be achieved and have no less clinical significance than other prominent immune cell components, such as CTLs.
The role of TH cells in the TIME is related to the inflammatory factors secreted by them, while these inflammatory factors cannot only affect other types of immune cells but also interact with each other among different TH cell subtypes. As an example, IFN-γ secreted by TH1 can further induce the activation of STAT1 and STAT4 in T cells, thereby promoting TH1 differentiation with positive feedback and inhibiting TH2 and TH17 differentiation (39). Conversely, IL-4 secreted by TH2 can regulate TH2 differentiation with positive feedback while inhibiting TH1 differentiation (40), from which it can be found that the balance between IFN-γ and IL-4 is also the balance of TH1–TH2 in the TIME, corresponding to the balance of pro- and antitumorigenic immune effects. Tregs induced by transforming growth factor-β (TGF-β) in the TIME activate the STAT5 signaling pathway by binding IL-2 with high affinity through CD25 and exert opposite regulatory effects on TH1 and TH2 cells by secreting IL-10, IL-35 and TGF-β (41). In this study, correlation analysis of TH1, TH2 and Treg in TIME of TNBC also showed that TH2 was positively correlated with Treg, while TH1 was negatively correlated with both of them, which further confirmed that a relationship network containing promotion and restriction was formed between TH cell subtypes to achieve the balance of pro- and antitumorigenic immunity. In addition, a study (42) showed that different subtypes of peripheral blood Tregs in breast cancer patients have different effects on the secretion of intratumor TH1- and TH2-related inflammatory factors, which also suggests that it is necessary to pay attention to TH cells in peripheral blood.
In this study, ECM-related pathways were enriched in the TH-activated cluster, and the relationships between different TH cell subtypes and ECM were detected in clinical specimens. It was found that high TH1 infiltration was related to low collagen content, while TME with high TH2 and Treg infiltration contained more abundant collagen in TNBC. A study (43) has shown that IFN-γ can upregulate the expression of matrix metalloproteinases (MMPs) that degrade ECM components, such as MMP-2, MMP-7, MMP-9, and MMP-13. Therefore, IFN-γ secreted by TH1 cells may be the possible cause of ECM remodeling, and the antagonistic relationship between TH2 and TH1 cells may also lead to the opposite effect on ECM. Furthermore, IL-13 secreted by TH2 is also considered to be a factor positively related to ECM formation in many fibrotic diseases (44). The effects of Treg on fibrosis are not the same in different diseases, while studies (45) have shown that the decrease in IL-10 and TGF-β levels caused by Treg depletion may be the crucial procedure leading to the reduction in fibrosis. In addition, a study (46) reported that the expression level of fibrosis-related transcription factors in tissue-resident Treg cells was increased in renal fibrosis disease. In breast cancer, research on tissue-resident memory T (TRM) cells (47) as an emerging target in immunotherapy reveals that the combination of tissue-resident Treg cells and fibrosis-related biomarkers has outstanding clinical significance. On the other hand, in terms of the effect of ECM on TH cells in the TME, a study (48) found that the elimination of FAP+ CAFs in vivo can implement the polarization of TH2 cells to TH1 cells; moreover, in breast cancer, the CAF1-S1 subtype could achieve immunosuppression by recruiting and increasing the differentiation of CD4+CD25+ Treg (49). Therefore, the crosstalk between ECM and TH cell subtypes persistently accumulates, forming a balanced TME in breast cancer.
Similarly, a recent study (50) showing the association between clusters of CAFs and immunotherapy resistance by single-cell sequencing highlighted the positive feedback loop relationship between specific CAFs-S1 clusters and Treg and revealed the correlation between different CAF clusters and CD8+ and CD4+ T cells, which indicates that the identification of specific clusters instructs treatment and prognosis in cancer. In reality, different TH cell subtypes have different prognostic guidance for solid tumors, which has been proven by studies (51, 52) based on characteristic gene expression data from clinical samples and animal experiments. This study also found that DFS of 30 TNBC patients was longer under high TH1 infiltration, low TH2, and low Treg infiltration. Accordingly, studies of ECM characteristics identifying different prognoses in breast cancer gradually appeared in 10 years, in which a stiff TME with abundant ECM indicating a poor prognosis was generally approved (53). In addition, different subtypes of TNBC have different prognoses and treatment strategies according to the spatial heterogeneity of CD8+TILs (54), which makes consideration of whether the distribution of CD4+ TH cells in the TIME also has crucial clinical value. Correspondingly, the spatial distribution of TH cells and ECM-related studies (22) further reminded us of the significance of TH cell characteristics and ECM as a new combined biomarker for breast cancer. The intrinsic modulatory mechanism of cytokines secreted by CD4+ TH cells influencing ECM remodeling would be our study field in the future, which is a crucial dimension to explain the correlation of the TME with prognosis in breast cancer and find potential combined therapies in the clinic.
Conclusion
Overall, the immune and ECM characteristics of breast cancer subtypes based on TH cell characteristics were revealed in this study by analyzing the datasets, and the relationship between different TH cell subsets with ECM and prognosis were explored in clinical TNBC samples. The accumulation of crosstalk between ECM and TH cell subtypes formed a balanced TME in breast cancer, which suggests that the combination of TH cell characteristics and ECM as a new biomarker needs to be further emphasized in future breast cancer clinical studies.
Data Availability Statement
The datasets presented in this study can be found in online repositories. The names of the repository/repositories and accession number(s) can be found in the article/Supplementary Material.
Ethics Statement
The studies involving human participants were reviewed and approved by the Ethics Committee of the First Affiliated Hospital of Xi’an Jiaotong University. The patients/participants provided their written informed consent to participate in this study.
Author Contributions
YaZ, QT, and BW contributed to the study design and performed the experiments. HG and LZ contributed to data collection. YiZ, QT, CZ, and JY performed statistical analysis and interpretation. YaZ and BW drafted the manuscript. All authors contributed to the article and approved the submitted version.
Funding
This study was supported by the National Natural Science Foundation of China (No. 82002794), Key Research and Development Program of Shaanxi Province of China (No. 2015SF015 and 2019SF-147), and Institutional Foundation of the First Affiliated Hospital of Xi’an Jiaotong University (No. 2019ZYTS-13).
Conflict of Interest
The authors declare that the research was conducted in the absence of any commercial or financial relationships that could be construed as a potential conflict of interest.
Publisher’s Note
All claims expressed in this article are solely those of the authors and do not necessarily represent those of their affiliated organizations, or those of the publisher, the editors and the reviewers. Any product that may be evaluated in this article, or claim that may be made by its manufacturer, is not guaranteed or endorsed by the publisher.
Acknowledgments
We thank The Cancer Genome Atlas (TCGA) and Gene Expression Omnibus (GEO) for providing transcriptomics and clinicopathological data.
Supplementary Material
The Supplementary Material for this article can be found online at: https://www.frontiersin.org/articles/10.3389/fimmu.2022.859581/full#supplementary-material
Supplementary Figure 1 | Comparison of optimal cluster analysis for 1090 samples from TCGA database. The consensus matrix when k =3 (A) and k =4 (B) through cluster analysis based on the expression of TH cell-related gene characteristics in 1090 samples from TCGA database. The cumulative distribution function (CDF) value (C) and relative change in the area under the CDF curve (D) of k =2-9.
Supplementary Figure 2 | Comparison of optimal cluster analysis for 64 samples from the GSE31519 dataset. The consensus matrix when k =3 (A) and k =4 (B) through cluster analysis based on the expression of TH cell-related gene characteristics in 64 samples from the GSE31519 dataset. The cumulative distribution function (CDF) value (C) and relative change in the area under the CDF curve (D) of k =2-9.
Supplementary Figure 3 | The distribution of contents of TH cell subtypes after grouping in 30 TNBC samples. The distribution of contents of TH1 (A), TH2 (B) and Treg (C) cells after low or high infiltrating grouping in 30 TNBC samples.
References
1. Adams S, Gatti-Mays ME, Kalinsky K, Korde LA, Sharon E, Amiri-Kordestani L, et al. Current Landscape of Immunotherapy in Breast Cancer: A Review. JAMA Oncol (2019) 5(8):1205–14. doi: 10.1001/jamaoncol.2018.7147
2. Albrengues J, Bertero T, Grasset E, Bonan S, Maiel M, Bourget I, et al. Epigenetic Switch Drives the Conversion of Fibroblasts Into Proinvasive Cancer-Associated Fibroblasts. Nat Commun (2015) 6:10204. doi: 10.1038/ncomms10204
3. Althobiti M, Aleskandarany MA, Joseph C, Toss M, Mongan N, Diez-Rodriguez M, et al. Heterogeneity of Tumour-Infiltrating Lymphocytes in Breast Cancer and its Prognostic Significance. Histopathology (2018) 73(6):887–96. doi: 10.1111/his.13695
4. Autio M, Leivonen SK, Brück O, Mustjoki S, Mészáros Jørgensen J, Karjalainen-Lindsberg ML, et al. Immune Cell Constitution in the Tumor Microenvironment Predicts the Outcome in Diffuse Large B-Cell Lymphoma. Haematologica (2021) 106(3):718–29. doi: 10.3324/haematol.2019.243626
5. Bareche Y, Buisseret L, Gruosso T, Girard E, Venet D, Dupont F, et al. Unraveling Triple-Negative Breast Cancer Tumor Microenvironment Heterogeneity: Towards an Optimized Treatment Approach. J Natl Cancer Inst (2020) 112(7):708–19. doi: 10.1093/jnci/djz208
6. Basu A, Ramamoorthi G, Albert G, Gallen C, Beyer A, Snyder C, et al. Differentiation and Regulation of T(H) Cells: A Balancing Act for Cancer Immunotherapy. Front Immunol (2021) 12:669474. doi: 10.3389/fimmu.2021.669474
7. Borst J, Ahrends T, Bąbała N, Melief CJM, Kastenmüller W. CD4(+) T Cell Help in Cancer Immunology and Immunotherapy. Nat Rev Immunol (2018) 18(10):635–47. doi: 10.1038/s41577-018-0044-0
8. Byrne A, Savas P, Sant S, Li R, Virassamy B, Luen SJ, et al. Tissue-Resident Memory T Cells in Breast Cancer Control and Immunotherapy Responses. Nat Rev Clin Oncol (2020) 17(6):341–8. doi: 10.1038/s41571-020-0333-y
9. Chinen T, Kannan AK, Levine AG, Fan X, Klein U, Zheng Y, et al. An Essential Role for the IL-2 Receptor in T(reg) Cell Function. Nat Immunol (2016) 17(11):1322–33. doi: 10.1038/ni.3540
10. Costa A, Kieffer Y, Scholer-Dahirel A, Pelon F, Bourachot B, Cardon M, et al. Fibroblast Heterogeneity and Immunosuppressive Environment in Human Breast Cancer. Cancer Cell (2018) 33(3):463–79.e410. doi: 10.1016/j.ccell.2018.01.011
11. Criscitiello C, Esposito A, Trapani D, Curigliano G. Prognostic and Predictive Value of Tumor Infiltrating Lymphocytes in Early Breast Cancer. Cancer Treat Rev (2016) 50:205–7. doi: 10.1016/j.ctrv.2016.09.019
12. do Valle Duraes F, Lafont A, Beibel M, Martin K, Darribat K, Cuttat R, et al. Immune Cell Landscaping Reveals a Protective Role for Regulatory T Cells During Kidney Injury and Fibrosis. JCI Insight (2020) 5(3):e130651. doi: 10.1172/jci.insight.130651
13. Dobrzanski MJ. Expanding Roles for CD4 T Cells and Their Subpopulations in Tumor Immunity and Therapy. Front Oncol (2013) 3:63. doi: 10.3389/fonc.2013.00063
14. Emens LA, Molinero L, Loi S, Rugo HS, Schneeweiss A, Diéras V, et al. Atezolizumab and Nab-Paclitaxel in Advanced Triple-Negative Breast Cancer: Biomarker Evaluation of the IMpassion130 Study. J Natl Cancer Inst (2021) 113(8):1005–16. doi: 10.1093/jnci/djab004
15. Erez N, Truitt M, Olson P, Arron ST, Hanahan D. Cancer-Associated Fibroblasts Are Activated in Incipient Neoplasia to Orchestrate Tumor-Promoting Inflammation in an NF-kappaB-Dependent Manner. Cancer Cell (2010) 17(2):135–47. doi: 10.1016/j.ccr.2009.12.041
16. Gieseck RL 3rd, Wilson MS, Wynn TA. Type 2 Immunity in Tissue Repair and Fibrosis. Nat Rev Immunol (2018) 18(1):62–76. doi: 10.1038/nri.2017.90
17. Gruosso T, Gigoux M, Manem VSK, Bertos N, Zuo D, Perlitch I, et al. Spatially Distinct Tumor Immune Microenvironments Stratify Triple-Negative Breast Cancers. J Clin Invest (2019) 129(4):1785–800. doi: 10.1172/jci96313
18. Gu-Trantien C, Loi S, Garaud S, Equeter C, Libin M, de Wind A, et al. CD4⁺ Follicular Helper T Cell Infiltration Predicts Breast Cancer Survival. J Clin Invest (2013) 123(7):2873–92. doi: 10.1172/jci67428
19. Hänzelmann S, Castelo R, Guinney J. GSVA: Gene Set Variation Analysis for Microarray and RNA-Seq Data. BMC Bioinf (2013) 14:7. doi: 10.1186/1471-2105-14-7
20. Harper J, Sainson RC. Regulation of the Anti-Tumour Immune Response by Cancer-Associated Fibroblasts. Semin Cancer Biol (2014) 25:69–77. doi: 10.1016/j.semcancer.2013.12.005
21. Jiang YZ, Liu Y, Xiao Y, Hu X, Jiang L, Zuo WJ, et al. Molecular Subtyping and Genomic Profiling Expand Precision Medicine in Refractory Metastatic Triple-Negative Breast Cancer: The FUTURE Trial. Cell Res (2021) 31(2):178–86. doi: 10.1038/s41422-020-0375-9
22. Kalluri R. The Biology and Function of Fibroblasts in Cancer. Nat Rev Cancer (2016) 16(9):582–98. doi: 10.1038/nrc.2016.73
23. Kieffer Y, Hocine HR, Gentric G, Pelon F, Bernard C, Bourachot B, et al. Single-Cell Analysis Reveals Fibroblast Clusters Linked to Immunotherapy Resistance in Cancer. Cancer Discovery (2020) 10(9):1330–51. doi: 10.1158/2159-8290.Cd-19-1384
24. Li B, Cui Y, Nambiar DK, Sunwoo JB, Li R. The Immune Subtypes and Landscape of Squamous Cell Carcinoma. Clin Cancer Res (2019) 25(12):3528–37. doi: 10.1158/1078-0432.Ccr-18-4085
25. Liao D, Luo Y, Markowitz D, Xiang R, Reisfeld RA. Cancer Associated Fibroblasts Promote Tumor Growth and Metastasis by Modulating the Tumor Immune Microenvironment in a 4T1 Murine Breast Cancer Model. PLos One (2009) 4(11):e7965. doi: 10.1371/journal.pone.0007965
26. Liu F, Liu J, Weng D, Chen Y, Song L, He Q, et al. CD4+CD25+Foxp3+ Regulatory T Cells Depletion may Attenuate the Development of Silica-Induced Lung Fibrosis in Mice. PLos One (2010) 5(11):e15404. doi: 10.1371/journal.pone.0015404
27. Liu Q, Nie R, Li M, Li L, Zhou H, Lu H, et al. Identification of Subtypes Correlated With Tumor Immunity and Immunotherapy in Cutaneous Melanoma. Comput Struct Biotechnol J (2021) 19:4472–85. doi: 10.1016/j.csbj.2021.08.005
28. Mittendorf EA, Zhang H, Barrios CH, Saji S, Jung KH, Hegg R, et al. Neoadjuvant Atezolizumab in Combination With Sequential Nab-Paclitaxel and Anthracycline-Based Chemotherapy Versus Placebo and Chemotherapy in Patients With Early-Stage Triple-Negative Breast Cancer (IMpassion031): A Randomised, Double-Blind, Phase 3 Trial. Lancet (2020) 396(10257):1090–100. doi: 10.1016/s0140-6736(20)31953-x
29. Newman AM, Liu CL, Green MR, Gentles AJ, Feng W, Xu Y, et al. Robust Enumeration of Cell Subsets From Tissue Expression Profiles. Nat Methods (2015) 12(5):453–7. doi: 10.1038/nmeth.3337
30. Oshi M, Asaoka M, Tokumaru Y, Yan L, Matsuyama R, Ishikawa T, et al. CD8 T Cell Score as a Prognostic Biomarker for Triple Negative Breast Cancer. Int J Mol Sci (2020) 21(18):6968. doi: 10.3390/ijms21186968
31. Pagès F, Berger A, Camus M, Sanchez-Cabo F, Costes A, Molidor R, et al. Effector Memory T Cells, Early Metastasis, and Survival in Colorectal Cancer. N Engl J Med (2005) 353(25):2654–66. doi: 10.1056/NEJMoa051424
32. Pérez-Pena J, Tibor Fekete J, Páez R, Baliu-Piqué M, García-Saenz J, García-Barberán V, et al. A Transcriptomic Immunologic Signature Predicts Favorable Outcome in Neoadjuvant Chemotherapy Treated Triple Negative Breast Tumors. Front Immunol (2019) 10:2802. doi: 10.3389/fimmu.2019.02802
33. Plitas G, Konopacki C, Wu K, Bos PD, Morrow M, Putintseva EV, et al. Regulatory T Cells Exhibit Distinct Features in Human Breast Cancer. Immunity (2016) 45(5):1122–34. doi: 10.1016/j.immuni.2016.10.032
34. Ravelli A, Roviello G, Cretella D, Cavazzoni A, Biondi A, Cappelletti MR, et al. Tumor-Infiltrating Lymphocytes and Breast Cancer: Beyond the Prognostic and Predictive Utility. Tumour Biol (2017) 39(4):1010428317695023. doi: 10.1177/1010428317695023
35. Roderfeld M, Rath T, Pasupuleti S, Zimmermann M, Neumann C, Churin Y, et al. Bone Marrow Transplantation Improves Hepatic Fibrosis in Abcb4-/- Mice via Th1 Response and Matrix Metalloproteinase Activity. Gut (2012) 61(6):907–16. doi: 10.1136/gutjnl-2011-300608
36. Sahai E, Astsaturov I, Cukierman E, DeNardo DG, Egeblad M, Evans RM, et al. A Framework for Advancing Our Understanding of Cancer-Associated Fibroblasts. Nat Rev Cancer (2020) 20(3):174–86. doi: 10.1038/s41568-019-0238-1
37. Salmon H, Franciszkiewicz K, Damotte D, Dieu-Nosjean MC, Validire P, Trautmann A, et al. Matrix Architecture Defines the Preferential Localization and Migration of T Cells Into the Stroma of Human Lung Tumors. J Clin Invest (2012) 122(3):899–910. doi: 10.1172/jci45817
38. Saravia J, Chapman NM, Chi H. Helper T Cell Differentiation. Cell Mol Immunol (2019) 16(7):634–43. doi: 10.1038/s41423-019-0220-6
39. Savas P, Salgado R, Denkert C, Sotiriou C, Darcy PK, Smyth MJ, et al. Clinical Relevance of Host Immunity in Breast Cancer: From TILs to the Clinic. Nat Rev Clin Oncol (2016) 13(4):228–41. doi: 10.1038/nrclinonc.2015.215
40. Schmid P, Salgado R, Park YH, Muñoz-Couselo E, Kim SB, Sohn J, et al. Pembrolizumab Plus Chemotherapy as Neoadjuvant Treatment of High-Risk, Early-Stage Triple-Negative Breast Cancer: Results From the Phase 1b Open-Label, Multicohort KEYNOTE-173 Study. Ann Oncol (2020) 31(5):569–81. doi: 10.1016/j.annonc.2020.01.072
41. Shen Y, Peng X, Shen C. Identification and Validation of Immune-Related lncRNA Prognostic Signature for Breast Cancer. Genomics (2020) 112(3):2640–6. doi: 10.1016/j.ygeno.2020.02.015
42. Siegel RL, Miller KD, Fuchs HE, Jemal A. Cancer Statistics, 2021. CA Cancer J Clin (2021) 71(1):7–33. doi: 10.3322/caac.21654
43. Thibaudin M, Chaix M, Boidot R, Végran F, Derangère V, Limagne E, et al. Human Ectonucleotidase-Expressing CD25(high) Th17 Cells Accumulate in Breast Cancer Tumors and Exert Immunosuppressive Functions. Oncoimmunology (2016) 5(1):e1055444. doi: 10.1080/2162402x.2015.1055444
44. Tiriveedhi V, Ivy MT, Myles EL, Zent R, Rathmell JC, Titze J. Ex Vivo High Salt Activated Tumor-Primed Cd4+T Lymphocytes Exert a Potent Anti-Cancer Response. Cancers (Basel) (2021) 13(7):1690. doi: 10.3390/cancers13071690
45. Tosolini M, Kirilovsky A, Mlecnik B, Fredriksen T, Mauger S, Bindea G, et al. Clinical Impact of Different Classes of Infiltrating T Cytotoxic and Helper Cells (Th1, Th2, Treg, Th17) in Patients With Colorectal Cancer. Cancer Res (2011) 71(4):1263–71. doi: 10.1158/0008-5472.Can-10-2907
46. Varn FS, Mullins DW, Arias-Pulido H, Fiering S, Cheng C. Adaptive Immunity Programmes in Breast Cancer. Immunology (2017) 150(1):25–34. doi: 10.1111/imm.12664
47. Wang L, Simons DL, Lu X, Tu TY, Solomon S, Wang R, et al. Connecting Blood and Intratumoral T(reg) Cell Activity in Predicting Future Relapse in Breast Cancer. Nat Immunol (2019) 20(9):1220–30. doi: 10.1038/s41590-019-0429-7
48. Wang S, Zhang Q, Yu C, Cao Y, Zuo Y, Yang L. Immune Cell Infiltration-Based Signature for Prognosis and Immunogenomic Analysis in Breast Cancer. Brief Bioinform (2021) 22(2):2020–31. doi: 10.1093/bib/bbaa026
49. Winer EP, Lipatov O, Im SA, Goncalves A, Muñoz-Couselo E, Lee KS, et al. Pembrolizumab Versus Investigator-Choice Chemotherapy for Metastatic Triple-Negative Breast Cancer (KEYNOTE-119): A Randomised, Open-Label, Phase 3 Trial. Lancet Oncol (2021) 22(4):499–511. doi: 10.1016/s1470-2045(20)30754-3
50. Xu Q, Chen S, Hu Y, Huang W. Landscape of Immune Microenvironment Under Immune Cell Infiltration Pattern in Breast Cancer. Front Immunol (2021) 12:711433. doi: 10.3389/fimmu.2021.711433
51. Yang B, Chou J, Tao Y, Wu D, Wu X, Li X, et al. An Assessment of Prognostic Immunity Markers in Breast Cancer. NPJ Breast Cancer (2018) 4:35. doi: 10.1038/s41523-018-0088-0
52. Zhang M, Zhang S. T Cells in Fibrosis and Fibrotic Diseases. Front Immunol (2020) 11:1142. doi: 10.3389/fimmu.2020.01142
53. Bergamaschi A, Tagliabue E, Sørlie T, Naume B, Triulzi T, Orlandi R, et al. Extracellular Matrix Signature Identifies Breast Cancer Subgroups With Different Clinical Outcome. J Pathol (2008) 214(3):357–67. doi: 10.1002/path.2278
Keywords: breast cancer, T helper cell (Th), tumor microenvironment, extracellular matrix, tumor immunity
Citation: Zhou Y, Tian Q, Gao H, Zhu L, Zhang Y, Zhang C, Yang J and Wang B (2022) Immunity and Extracellular Matrix Characteristics of Breast Cancer Subtypes Based on Identification by T Helper Cells Profiling. Front. Immunol. 13:859581. doi: 10.3389/fimmu.2022.859581
Received: 21 January 2022; Accepted: 19 May 2022;
Published: 20 June 2022.
Edited by:
Weikun Hou, Xi’an Honghui Hospital, ChinaReviewed by:
Wentao Wang, Sichuan University, ChinaYing Zhong, Peking Union Medical College Hospital (CAMS), China
Copyright © 2022 Zhou, Tian, Gao, Zhu, Zhang, Zhang, Yang and Wang. This is an open-access article distributed under the terms of the Creative Commons Attribution License (CC BY). The use, distribution or reproduction in other forums is permitted, provided the original author(s) and the copyright owner(s) are credited and that the original publication in this journal is cited, in accordance with accepted academic practice. No use, distribution or reproduction is permitted which does not comply with these terms.
*Correspondence: Jiao Yang, eWFveml3ZWltZW5nQDEyNi5jb20=; Bo Wang, cmVhbHdib0B4anR1LmVkdS5jbg==; orcid.org/0000-0001-7633-4435