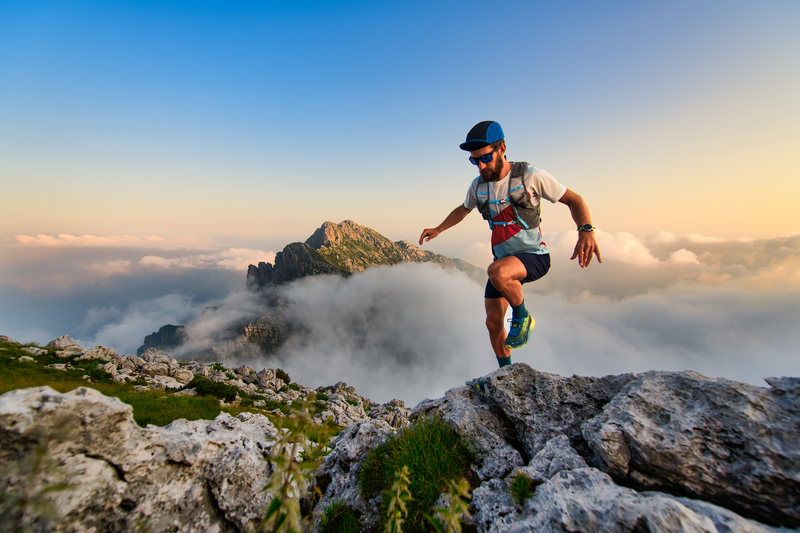
95% of researchers rate our articles as excellent or good
Learn more about the work of our research integrity team to safeguard the quality of each article we publish.
Find out more
OPINION article
Front. Immunol. , 03 June 2022
Sec. Cancer Immunity and Immunotherapy
Volume 13 - 2022 | https://doi.org/10.3389/fimmu.2022.853300
This article is part of the Research Topic Insights in Cancer Immunity and Immunotherapy: 2021 View all 9 articles
Over the past decade, immunotherapy has revolutionized cancer treatment by inducing durable remission and prolonging patient survival in diverse types of cancer (1). In the landscape of cancer immunotherapies, therapeutically targeting immune inhibitory checkpoints through the blockade of cytotoxic T-lymphocyte-associated protein 4 (CTLA-4), programmed cell death protein-1 (PD-1), and programmed death ligand-1 (PD-L1) are most broadly effective (2, 3). Based on its unprecedented success in multiple types of cancer (4), immune checkpoint blockade (ICB) treatment is now FDA approved for cancer patients with tumor mutation burden (TMB) >10 mutations/megabase (mut/Mb) (5). However, high TMB (>10 mut/Mb) is not associated with improved survival of ICB-treated patients in all types of cancer (6, 7). It is not unexpected that the arbitrary TMB cut-off of 10 mut/Mb fails to predict ICB response, especially considering that TMB levels can widely range from 0.01 mut/Mb to more than 400 mut/Mb (8). Recently, Dr. Yarchoan et al. have reported a positive correlation between TMB and improved overall survival (OS) following ICB treatment in cancer types with higher TMB, but the correlation did not meet statistical significance (9). Hence, although the preliminary finding by Dr. Yarchoan et al. is promisingly positive, there is still a scarcity of data regarding TMB as a continuous prognostic factor that the effect of increasing TMB levels on improved ICB response remains to be elucidated.
Previously, we have developed an analytical framework for modeling the non-linear relationship of prognostic data with the continuous spectrum of biomarker (10). This analytical framework introduced flexibility into Cox proportional hazards regression model based on the spline-based smoothing method (11, 12). A statistical approach described as smoothHR (13) was applied to construct the natural cubic regression spline curve of hazard ratio (HR) with 95% confidence intervals (CIs) (14). To the best of our knowledge, this method allowed us to investigate the non-linear relationship between TMB gradient and ICB response for the first time (Supplementary Figure 1).
The non-linear HR analysis was conducted using the Morris’s cohort of 1,662 patients with cancer, who had received ICB treatment through the blockades of PD1, PD-L1, and CTLA4 (Supplementary Figure 2). The patients’ TMB values were measured by next-generation sequencing (NGS) using the MSK-IMPACT panel previously, and the detailed clinical information, including cancer types, sex, age, ICI drug class, year of ICB start, and OS, were retained from Morris’s study (15). Here, we presented the data modeling the TMB gradient as a continuous risk predictor of the OS following ICB treatment. The HRs were calculated by univariate and multivariate analyses of Cox proportional hazard regression taking TMB = 0 mut/Mb as a reference, and the smoothing estimate of HR was calculated across the spectrum of TMB values. Multivariate analysis was conducted using covariates of cancer types, sex, age, ICI drug class, and year of ICB start. In order to rule out the outliers at the distal end of the log-HR curve, truncation was performed to remove TMB values above the 95th percentile. Next, the log-HR curve with 95% CIs was plotted with a straightforward interpretation that the 95% CIs above or below 0 is equivalent to a significance of two-sided p < 0.05.
In both univariate and multivariate analyses of total patients, the smoothing log-HR curves showed that TMB was significantly associated with an improved OS following ICB treatment in a dose-dependent manner (Figure 1A). The log-HR curves revealed similar trends in univariate and multivariate analyses, indicating TMB as an independent prognostic factor of ICB response. In agreement with the above findings, Kaplan-Meier analyses showed a significant difference in OS between patients with high-TMB (TMB-H) versus low-TMB (TMB-L) tumors (p < 0.0001; Figure 1B). TMB-H and TMB-L patients were defined using the FDA-approved TMB cutoff — 10 mut/Mb; and, TMB-H patients were further divided equally into low, moderate, and high TMB-H subgroups. For each decrease in TMB levels, there was a stepwise reduction in OS. The TMB-L patients had the poorest survival with a median OS time of 1.25 years (95% CIs: 1.08 to 1.42). By contrast, the low, moderate, and high TMB-H subgroups had median OS times of 1.42 years (95% CIs; 0.92 to NR [not reached]), 3.67 years (95% CIs: 2.00 to NR), and 3.92 years (95% CIs: 3.42 to NR), respectively.
Figure 1 The dose-dependent association between tumor mutation burden (TMB) and overall survival (OS) in cancer patients following immune checkpoint blockade (ICB) treatment. (A) The smoothing estimate of hazard ratios (HRs) with 95% confidence intervals (CIs) across the spectrum of TMB gradient. The continuous log-HR curves (solid line) with 95% CIs (shading) show the association of TMB with the OS following ICB treatment in total patients from the Morris’ ICB cohort. The HRs were fitted by univariate and multivariate Cox proportional hazards regressions. Multivariate analysis was conducted using covariates of cancer type, sex, age, ICI drug class, and year of ICB start. To rule out the outliers at the distal end of log-HR curve, truncation was performed to remove TMB values above the 95th percentile. The x-axis shows TMB values (mut/Mb), and the y-axis shows HR, taking the patients with TMB = 0 mut/Mb as the reference. (B) The Kaplan-Meier curves show the OS of total cancer patients following ICB treatment according to TMB. High-TMB (TMB-H) and low-TMB (TMB-L) Patients were defined using the FDA-approved TMB cutoff of 10 mut/Mb; next, TMB-H patients were divided equally into low, moderate, and high TMB-H subgroups. The significance was measured by the log-rank test. The bottom panel shows the number of patients at risk every one year. (C) The pie plot shows the percentage of patients by cancer type and category. Category I cancers were cancer types where CD8+ TIL-T-cell levels positively correlated with neoantigen loads, while no such correlation was observed in category II cancers. Category I cancers: non-small cell lung cancer, melanoma, bladder cancer, and colorectal cancer; category II cancers: renal cell carcinoma, head and neck cancer, oesophagogastric cancer, glioma, cancer of unknown primary, and breast cancer. (D) The continuous log-HR curves (solid line) with 95% CIs (shading) show the association of TMB with the OS following ICB treatment in cancer patients of category II cancer types. (E) The Kaplan-Meier curves show the OS following ICB treatment according to TMB in cancer patients of category II cancer types. The bottom panel shows the number of patients at risk every one year. The significance was measured by the log-rank test. (F) The continuous log-HR curves (solid line) with 95% CIs (shading) show the association of TMB with the OS following ICB treatment in cancer patients of category I cancer types. (G) The Kaplan-Meier curves show the OS following ICB treatment according to TMB in cancer patients of category I cancer types. The significance was measured by the log-rank test.
A previous study has reported that the assessment of ICB response is related to the context of cancer categories defined by whether CD8 T-cell infiltration is positively correlated with neoantigen load (7). As previously reported, we divided the total patients into category I and II cancers (Figure 1C). Category I cancers include non-small cell lung cancer, melanoma, bladder cancer, and colorectal cancer, where the levels of CD8+ tumor-infiltrating T cells were positively correlated with the neoantigen loads of tumor, while no such correlation was observed in the rest cancers of category II (7). As shown in Figure 1D, the log-HR curves for category II cancers were generally constant and slightly dropped at the distant end where the TMB level was above 15 mut/Mb, and the range of 95% CIs of HR was relatively broad, showing a generally insignificant association. Consistently, the Kaplan-Meier plot showed no significant difference in OS between TMB-L and TMB-H patients with category II cancers (Figure 1E).
Comparatively, in category I cancers, HRs significantly decreased with increasing TMB values (Figure 1F). A consistent finding was observed in the Kaplan-Meier analyses. As shown in Figure 1G, the TMB-L patients with category I cancers had the poorest survival with median OS times of 1.25 years (95% CIs: 1.08 to 1.50), and the low, moderate, and high TMB-H subgroups had median OS times of 1.25 years (95% CIs: 0.83 to NR), 3.67 years (95% CIs: 2.67 to NR), and 3.92 years (95% CIs: 3.41 to NR), respectively. Thus, the benefit of ICB treatment is increased with elevated TMB levels, but it mainly depends on the context of cancer types.
ICB treatment was initially demonstrated efficacy and FDA approved for patients with melanoma, and later expanded to other types of cancer (16). The 2020 FDA approval of pembrolizumab (anti-PD-1) in TMB-H patients represents a transformative event of ICB therapies (5). Since then, ICB therapies have been extended to a remarkably skyrocketing number of patients in a broad range of cancer types. However, as we look to the foreseeably inspiring future of ICB-based immunotherapy, many aspects of clinical practice and biological mechanisms underlying ICB remain to be elucidated. At present, one of the major factors affecting ICB response is the absolute level of TMB (4). To the best of our knowledge, this is the first study showing that the increasing level of TMB has a significant effect on the improved prognosis following ICB treatment in a dose-dependent manner. The dose-dependent relationship strongly implies the causal role of TMB in ICB response, with important clinical and therapeutic implications for ICB-based immunotherapy.
In this study, we found that TMB-H tumors were substantially more responsive to ICB treatment. ICB treatment inhibits tumor progression by boosting the anti-tumor immunity of cytotoxic T cells (CTLs) (17). Based on blocking the co-inhibitory pathways, anti-CTLA-4 enhances the co-stimulation signaling and lowers the activation threshold of T cell receptor (TCR) (18, 19), while anti-PD-1/PD-L1 reduces the requirement for TCR signaling and reinvigorates exhausted CTLs (20). As indicated by the above-stated mechanisms, ICB treatment works better in tumors recognizable by CTLs. T-cell recognition of tumor cells is based on the interaction of TCRs with major histocompatibility complex (MHC)-presented neoantigens (21, 22). Indeed, neoantigens are mutated proteins derived from tumor somatic mutations. Before this study, TMB has been typically considered as a logical surrogate of neoantigen load. Therefore, it seems reasonable to assume that TMB-H tumors, harboring a greater immunogenicity of higher abundance and variety of neoantigens, are statistically more likely to be recognized and killed by CTLs.
Previous studies have exhaustively tested the robustness of TMB-H as a predictive biomarker for improved ICB response (6, 7). Consistent with previous studies, the finding in this study also suggests that the higher the TMB level, the better the ICB response. However, these exciting results were observed only in category I cancers, such as non-small cell lung cancer, melanoma, bladder cancer, and colorectal cancer. These cancers are much more likely to be exposed to mutagens such as chemical carcinogens and ultraviolet radiation that induce somatic mutations. Actually, category I cancers have relatively higher TMB levels (6), where immunotherapy was initially discovered to be successful (23).
Unlike category I cancers, most category II cancers have relatively lower TMB levels (6), poorer inherently immunogenicity, and less T cell infiltration (7). In category II cancers, such as breast cancer and glioma, TMB-H was not associated with a favorable prognosis but even related to an unfavorable ICB response (6). Moreover, according to this study, the dose-dependent relationship between TMB and ICB response is most significant in category I cancers but generally insignificant in category II cancers. In conclusion, the favorable ICB responsiveness is increased with increasing TMB levels, but also depends on the context of cancer types. Consequently, we should be cautious about generalizing the present success of ICB treatment to other cancer types directly. Future studies should focus on the cancer type specificity of ICB response in patients with TMB-H tumors.
MZ conceived the project, developed the method, conducted data analysis, and wrote the manuscript. MZ supervised this project and is responsible for the overall content.
This project was supported by the National Natural Science Foundation of China (32100739) to MZ.
The author declares that the research was conducted in the absence of any commercial or financial relationships that could be construed as a potential conflict of interest.
All claims expressed in this article are solely those of the authors and do not necessarily represent those of their affiliated organizations, or those of the publisher, the editors and the reviewers. Any product that may be evaluated in this article, or claim that may be made by its manufacturer, is not guaranteed or endorsed by the publisher.
We thank Dr. Luc G. T. Morris and his colleagues for the TMB and clinical data of ICB-treated cancer patients from their previously published study.
The Supplementary Material for this article can be found online at: https://www.frontiersin.org/articles/10.3389/fimmu.2022.853300/full#supplementary-material
Supplementary Figure 1 | The analytical framework is based on non-linear model of hazard ratio (HR) across the continuous spectrum of biomarker. Based on Cox proportional hazard regression, the pointwise hazard ratio (HR) was calculated for each value of tumor mutation burden (TMB; mut/Mb), taking TMB = 0 mut/Mb as the reference. The association between TMB and patient survival was assessed by both univariate and multivariate Cox regression.
Supplementary Figure 2 | (A) The pie plot shows the percentage of patients by ICI drug class and cancer type. ICI drug class: anti-PD-1 or PD-L1; anti-CTLA-4; and a combination of anti-CTLA-4 and anti-PD-1/PD-L1 therapies. Category I cancers were cancer types where CD8+ TIL-T cell levels positively correlated with neoantigen loads, while no such correlation was observed in category II cancers. Category I cancers: non-small cell lung cancer, melanoma, bladder cancer, and colorectal cancer; category II cancers: renal cell carcinoma, head and neck cancer, oesophagogastric cancer, glioma, cancer of unknown primary, and breast cancer.
1. Esfahani K, Roudaia L, Buhlaiga N, Del Rincon SV, Papneja N. Miller WH, Jr. A Review of Cancer Immunotherapy: From the Past, to the Present, to the Future. Curr Oncol (2020) 27(Suppl 2):S87–97. doi: 10.3747/co.27.5223
2. Postow MA, Callahan MK, Wolchok JD. Immune Checkpoint Blockade in Cancer Therapy. J Clin Oncol (2015) 33(17):1974–82. doi: 10.1200/JCO.2014.59.4358
3. Hargadon KM, Johnson CE, Williams CJ. Immune Checkpoint Blockade Therapy for Cancer: An Overview of FDA-Approved Immune Checkpoint Inhibitors. Int Immunopharmacol (2018) 62:29–39. doi: 10.1016/j.intimp.2018.06.001
4. Marabelle A, Fakih M, Lopez J, Shah M, Shapira-Frommer R, Nakagawa K, et al. Association of Tumour Mutational Burden With Outcomes in Patients With Advanced Solid Tumours Treated With Pembrolizumab: Prospective Biomarker Analysis of the Multicohort, Open-Label, Phase 2 KEYNOTE-158 Study. Lancet Oncol (2020) 21(10):1353–65. doi: 10.1016/S1470-2045(20)30445-9
5. FDA. FDA Approves Pembrolizumab for Adults and Children With TMB-H Solid Tumors (2020). Available at: https://www.fda.gov/drugs/drug-approvals-and-databases/fda-approves-pembrolizumab-adults-and-children-tmb-h-solid-tumors.
6. Zheng M. Tumor Mutation Burden for Predicting Immune Checkpoint Blockade Response: The More, the Better. J ImmunoTher Cancer (2022) 10(1):e003087. doi: 10.1136/jitc-2021-003087
7. McGrail DJ, Pilie PG, Rashid NU, Voorwerk L, Slagter M, Kok M, et al. High Tumor Mutation Burden Fails to Predict Immune Checkpoint Blockade Response Across All Cancer Types. Ann Oncol (2021) 32(5): 661–72. doi: 10.1016/j.annonc.2021.02.006
8. Prasad V, Addeo A. The FDA Approval of Pembrolizumab for Patients With TMB >10 Mut/Mb: Was It a Wise Decision? No. Ann Oncol (2020) 31(9):1112–4. doi: 10.1016/j.annonc.2020.07.001
9. Osipov A, Lim SJ, Popovic A, Azad NS, Laheru DA, Zheng L, et al. Tumor Mutational Burden, Toxicity, and Response of Immune Checkpoint Inhibitors Targeting PD(L)1, CTLA-4, and Combination: A Meta-Regression Analysis. Clin Cancer Res (2020) 26(18):4842–51. doi: 10.1158/1078-0432.CCR-20-0458
10. Zheng M. The Gradient of Immune/Inflammatory Response and COVID-19 Prognosis With Therapeutic Implications. Front Immunol (2021) 12:739482(4365). doi: 10.3389/fimmu.2021.739482
11. De Boor C, De Boor C. A Practical Guide to Splines: Springer-Verlag New York. (1978) 27: 325. doi: 10.1007/978-1-4612-6333-3
12. Eilers PH, Marx BD. Flexible Smoothing With B-Splines and Penalties. Stat Sci (1996) 11(2):89–121. doi: 10.1214/ss/1038425655
13. Meira-Machado L, Cadarso-Suarez C, Gude F, Araujo A. smoothHR: An R Package for Pointwise Nonparametric Estimation of Hazard Ratio Curves of Continuous Predictors. Comput Math Methods Med (2013) 2013:745742. doi: 10.1155/2013/745742
14. Cadarso-Suárez C, Meira-Machado L, Kneib T, Gude F. Flexible Hazard Ratio Curves for Continuous Predictors in Multi-State Models: An Application to Breast Cancer Data. Stat Modelling (2010) 10(3):291–314. doi: 10.1177/1471082X0801000303
15. Samstein RM, Lee CH, Shoushtari AN, Hellmann MD, Shen R, Janjigian YY, et al. Tumor Mutational Load Predicts Survival After Immunotherapy Across Multiple Cancer Types. Nat Genet (2019) 51(2):202–6. doi: 10.1038/s41588-018-0312-8
16. Sondak VK, Smalley KS, Kudchadkar R, Grippon S, Kirkpatrick P. Ipilimumab. Nat Rev Drug Discov (2011) 10(6):411–3. doi: 10.1038/nrd3463
17. Wieder T, Eigentler T, Brenner E, Rocken M. Immune Checkpoint Blockade Therapy. J Allergy Clin Immunol (2018) 142(5):1403–14. doi: 10.1016/j.jaci.2018.02.042
18. Alegre ML, Frauwirth KA, Thompson CB. T-Cell Regulation by CD28 and CTLA-4. Nat Rev Immunol (2001) 1(3):220–8. doi: 10.1038/35105024
19. Egen JG, Kuhns MS, Allison JP. CTLA-4: New Insights Into Its Biological Function and Use in Tumor Immunotherapy. Nat Immunol (2002) 3(7):611–8. doi: 10.1038/ni0702-611
20. Tumeh PC, Harview CL, Yearley JH, Shintaku IP, Taylor EJ, Robert L, et al. PD-1 Blockade Induces Responses by Inhibiting Adaptive Immune Resistance. Nature (2014) 515(7528):568–71. doi: 10.1038/nature13954
21. Chan TA, Yarchoan M, Jaffee E, Swanton C, Quezada SA, Stenzinger A, et al. Development of Tumor Mutation Burden as an Immunotherapy Biomarker: Utility for the Oncology Clinic. Ann Oncol (2019) 30(1):44–56. doi: 10.1093/annonc/mdy495
22. Reuben A, Zhang J, Chiou SH, Gittelman RM, Li J, Lee WC, et al. Comprehensive T Cell Repertoire Characterization of Non-Small Cell Lung Cancer. Nat Commun (2020) 11(1):603. doi: 10.1038/s41467-019-14273-0
Keywords: immune checkpoint blockade (ICB), tumor mutation burden (TMB), hazard ratio (HR), human cancer, overall survival (OS), cytotoxic T-lymphocyte-associated protein 4 (CTLA-4), programmed cell death protein-1 (PD-1), programmed death ligand-1 (PD-L1)
Citation: Zheng M (2022) Dose-Dependent Effect of Tumor Mutation Burden on Cancer Prognosis Following Immune Checkpoint Blockade: Causal Implications. Front. Immunol. 13:853300. doi: 10.3389/fimmu.2022.853300
Received: 12 January 2022; Accepted: 27 April 2022;
Published: 03 June 2022.
Edited by:
Catherine Sautes-Fridman, INSERM U1138 Centre de Recherche des Cordeliers (CRC), FranceReviewed by:
Shihai Liu, The Affiliated Hospital of Qingdao University, ChinaCopyright © 2022 Zheng. This is an open-access article distributed under the terms of the Creative Commons Attribution License (CC BY). The use, distribution or reproduction in other forums is permitted, provided the original author(s) and the copyright owner(s) are credited and that the original publication in this journal is cited, in accordance with accepted academic practice. No use, distribution or reproduction is permitted which does not comply with these terms.
*Correspondence: Ming Zheng, bW16aGVuZ0BmbW11LmVkdS5jbg==; emhlbmdtaW5nX2NoaW5hQDE2My5jb20=; orcid.org/0000-0002-3651-7701
Disclaimer: All claims expressed in this article are solely those of the authors and do not necessarily represent those of their affiliated organizations, or those of the publisher, the editors and the reviewers. Any product that may be evaluated in this article or claim that may be made by its manufacturer is not guaranteed or endorsed by the publisher.
Research integrity at Frontiers
Learn more about the work of our research integrity team to safeguard the quality of each article we publish.