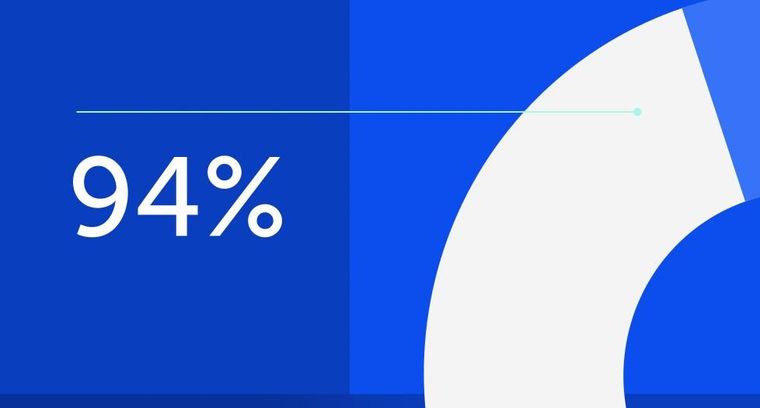
94% of researchers rate our articles as excellent or good
Learn more about the work of our research integrity team to safeguard the quality of each article we publish.
Find out more
ORIGINAL RESEARCH article
Front. Immunol., 25 March 2022
Sec. Viral Immunology
Volume 13 - 2022 | https://doi.org/10.3389/fimmu.2022.848886
Long-COVID is a new emerging syndrome worldwide that is characterized by the persistence of unresolved signs and symptoms of COVID-19 more than 4 weeks after the infection and even after more than 12 weeks. The underlying mechanisms for Long-COVID are still undefined, but a sustained inflammatory response caused by the persistence of SARS-CoV-2 in organ and tissue sanctuaries or resemblance with an autoimmune disease are within the most considered hypotheses. In this study, we analyzed the usefulness of several demographic, clinical, and immunological parameters as diagnostic biomarkers of Long-COVID in one cohort of Spanish individuals who presented signs and symptoms of this syndrome after 49 weeks post-infection, in comparison with individuals who recovered completely in the first 12 weeks after the infection. We determined that individuals with Long-COVID showed significantly increased levels of functional memory cells with high antiviral cytotoxic activity such as CD8+ TEMRA cells, CD8±TCRγδ+ cells, and NK cells with CD56+CD57+NKG2C+ phenotype. The persistence of these long-lasting cytotoxic populations was supported by enhanced levels of CD4+ Tregs and the expression of the exhaustion marker PD-1 on the surface of CD3+ T lymphocytes. With the use of these immune parameters and significant clinical features such as lethargy, pleuritic chest pain, and dermatological injuries, as well as demographic factors such as female gender and O+ blood type, a Random Forest algorithm predicted the assignment of the participants in the Long-COVID group with 100% accuracy. The definition of the most accurate diagnostic biomarkers could be helpful to detect the development of Long-COVID and to improve the clinical management of these patients.
The emergent virus named severe acute respiratory syndrome coronavirus 2 (SARS-CoV-2) is responsible for the coronavirus disease 2019 pandemic (COVID-19), which has caused 281 million infections worldwide and more than 5.4 million deaths to date (1). These numbers are rapidly increasing due to new emergent variants such as Omicron (2).
The clinical outcome of COVID-19 is highly variable, ranging from asymptomatic or mild to a fatal disease. This variability is mostly dependent on how the immune system reacts during the primary infection, due to an exacerbated systemic inflammatory response that has been described in the most severe and critical forms of the disease that may lead to multiple organ dysfunction syndromes (3–5). Those patients who develop severe or critical COVID-19 usually present bilateral pneumonia, respiratory failure with low blood oxygenation, and acute respiratory distress syndrome (ARDS) (6). Other organs may also be compromised, leading to nervous and cardiac system injuries that cause severe complications such as ataxia, acute cerebrovascular disease, olfactory dysfunction, blood hypercoagulability, or cardiomyopathy (7). The median hospital length of stay for patients with severe forms of COVID-19 ranges from less than 1 week to nearly 2 months, whereas the median stay at the intensive care unit (ICU) for those patients with critical COVID-19 varies from 1 to 3 weeks, being shorter for those patients who die from the disease (8). Those hospitalized patients who recover from the most severe forms of the disease may suffer permanent sequelae (9, 10).
The duration of the clinical signs and symptoms may also be very variable among those patients who do not require hospitalization due to asymptomatic or mild COVID-19. In the case of short or acute COVID-19, all signs and symptoms usually disappear from 10 days to 4 weeks after diagnosis (11). However, there is an increasing proportion of patients with mild COVID-19 in which the symptoms do not resolve completely after 4 weeks and may last up to 12 weeks after the clinical onset, then termed Ongoing Symptomatic COVID-19, or even more than 12 weeks, then termed Post-COVID-19 Syndrome (12). These new forms of the disease have been grouped under the term Long-COVID (13, 14), which describes those cases in which the signs and symptoms that continue or develop after acute COVID−19 last from 4 to more than 12 weeks (12). Long-COVID may be developed by 1 in 10 patients who passed COVID-19 (15), and it includes both general and neuropsychiatric persistent symptoms such as cough, fatigue, muscle, and joint pains, insomnia, breathlessness, myalgia, or diarrhea. Other relevant symptoms may compromise the cardiopulmonary system, such as dyspnea, pericarditis, or heart failure (13, 16, 17). Long-COVID may be developed not only by patients who were admitted at the ICU due to critical COVID-19 and now present long-term respiratory sequelae but also by patients with mild COVID-19 who did not require hospitalization.
The causes of Long-COVID are still uncertain, and in most cases, no clear evidence of organ damage is usually found (17). However, some host factors such as gender and age have been related to a higher susceptibility to develop this prolonged syndrome. Women under 50 years of age seem to be more susceptible to present unresolved COVID-19 (18), which indicates a potential role of sex hormones in Long-COVID (19). Another plausible hypothesis could be viral persistence. Although the SARS-CoV-2 viral cycle is different, viral persistence is known to occur in other RNA viruses such as the human immunodeficiency virus (HIV) or hepatitis C virus (HCV), which may produce chronic infection with sustained activation of the immune system (20). Accordingly, prolonged viral shedding has been found in feces from patients with acute COVID-19 after negative PCR in respiratory specimens, suggesting that not only RNA of SARS-CoV-2 but also virions may persist in cells from the gastrointestinal tract (21, 22), likely in enterocytes and small vessels, as was described for the first SARS-CoV (23). The presence of SARS-CoV-2 in the intestinal mucosa could cause intestinal damage that, along with systemic inflammation, may increase bacterial translocation, which would play an essential role in the sustained immune activation and cytokine release syndrome characteristic of COVID-19 (24). On the other hand, several symptoms described in individuals with Long-COVID are quite similar to those developed during autoimmune diseases such as fibromyalgia or chronic fatigue syndrome, which are also linked to persistent inflammation and exacerbated immune responses (25). Individuals with COVID-19 may develop autoantibodies against tissue-associated antigens and immunomodulatory proteins, both soluble such as interferon (IFN) type I or bound to the surface of the immune cells, which would also promote the persistence of the symptoms (26, 27).
In summary, the recovery from COVID-19 seems to be beyond hospital discharge or testing negative for SARS-CoV-2 (28), but the causes for the subsequent perpetuation of the symptoms are still undetermined. To define the clinical guidelines for the prevention, diagnosis, follow-up, and rehabilitation of these individuals, it is necessary to gain a better understanding of the underlying mechanisms of Long-COVID. Our group previously determined that an inefficient cytotoxic response is a reliable biomarker for COVID-19 severity (4). Consequently, in this study, we evaluated the relative importance of this impaired cellular immune response, along with several demographic and clinical features, to develop the persistence of the symptoms and the evolution to Long-COVID by using a Random Forest algorithm.
Blood samples from 50 individuals who had passed mild, symptomatic COVID-19 during the first pandemic peak in Madrid (Spain) (March–April 2020) were collected in the Primary Healthcare Center Doctor Pedro Laín Entralgo (Madrid, Spain). The inclusion criteria were as follows: over 18 years old, have a positive RT-qPCR assay for SARS-CoV-2 in nasopharyngeal smear or positive titers of virus-specific IgG, and did not require hospitalization while with COVID-19. The subjects were subsequently classified into Long-COVID (n = 30) and Recovered (n = 20). The Long-COVID group included those individuals who had passed mild COVID-19 and referred the presence of at least 8 clinical signs and symptoms compatible with Ongoing Symptomatic COVID-19 or Post-COVID-19 Syndrome according to the National Institute for Health and Care Excellence (NICE) guideline (12) for at least 4 to 12 weeks or more than 12 weeks after the clinical diagnosis of COVID-19. Individuals with Long-COVID were recruited in collaboration with the non-profit Spanish Association of Patients with Long-COVID (Long-COVID-ACTS, Madrid, Spain). The Recovered group included those individuals who had passed acute COVID-19, were homebound until medical discharge, and had the resolution of all signs and symptoms 2 to 4 weeks after the clinical diagnosis. Re-infection or vaccination previous to sampling was not reported by any participant. The most relevant clinical data of all participants with Long-COVID or completely recovered are described in Supplementary Tables 1, 2, respectively.
The individuals participating in this study were recruited at the Primary Healthcare Center Doctor Pedro Lain Entralgo (Alcorcón, Madrid, Spain). All participants gave informed written consent to participate, and their anonymity was ensured by the current Spanish and European Data Protection Law. This study was conducted following the Declaration of Helsinki, and it was approved by the Ethical Committee of Instituto de Salud Carlos III (IRB IORG0006384) (CEI PI 07_2021) and the Central Research Commission from the Health Counseling (Comunidad de Madrid, Spain) (favorable report 20210008).
Peripheral blood lymphocytes (peripheral blood mononuclear cells (PBMCs)) and plasma were isolated from blood samples by centrifugation through Ficoll–Hypaque gradient (Pharmacia Corporation, North Peapack, NJ, USA) and cryopreserved until the moment of analysis. Due to the low quantity of samples, not all the parameters could be determined in all participants. K562 cell line (ECACC 89121407) was kindly provided by Dr Cristina Eguizabal (Basque Centre Transfusions and Human Tissue, Álava, Spain), and Vero E6 (African green monkey kidney) cell line (ECACC 85020206) was kindly provided by Dr. Antonio Alcami (CBM Severo Ochoa, Madrid).
Conjugated antibodies CD3-APC, CD4-PercP, CD8-APC-H7, CD8-PercP-Cy5.5, CD16-PercP-Cy5.5, CD25-PE-Cy5, CD56-FITC, CD57-PE, CD107a-PE-Cy7, CD127-FITC, CD158f-BV421, NKG2D-PECy7, NKp44-BUV395, and NKp46-BV650 were purchased from BD Biosciences (San Jose, CA, USA). PD-1-BV650, NKG2A-PE, and NKG2C-AlexaFluor700 were obtained from R&D Systems (Minneapolis, MN, USA). TCRγδ-PE was obtained from BioLegend (San Diego, CA, USA). CD4+ and CD8+ T-cell memory subpopulations were quantified after staining with CCR7-FITC and CD45RA-PE-Cy7 (BD Biosciences) as follows: naïve (CD45RA+CCR7+), central memory (TCM) (CD45RA−CCR7+), effector memory (TEM) (CD45RA−CCR7−), and terminally differentiated effector memory (TEMRA) (CD45RA+CCR7−) cells. Data acquisition was performed in a BD LSRFortessa X-20 flow cytometer using FACS Diva software (BD Biosciences). Data analysis was performed with FlowJo_V10 software (TreeStar, Ashland, OR, USA).
For intracellular staining of IFNγ, TNFα and granzyme B (GZB) in CD3+CD8+ T cells, PBMCs were treated for 4 h at 37°C with PepMix™ SARS-CoV-2 (NCAP) (JPT Peptide Technologies, Berlin, Germany), which contains 102 peptides derived from the Nucleoprotein of SARS-CoV-2, to stimulate the cytotoxic activity of CD8+ T cells, in the presence of brefeldin A (BD Biosciences). Cells were then stained with anti-CD3-APC and anti-CD8-PercP. After fixation and permeabilization with IntraPrep Permeabilization Reagent (Beckman Coulter, Brea, CA, USA), cells were stained with antibodies against IFNγ-PE, TNFα-PE, and GZB-FITC (BD Biosciences) and then acquired and analyzed in a BD LSRFortessa X-20 flow cytometer (BD Biosciences) using FACS Diva (BD Biosciences) and FlowJo_V10 software (TreeStar).
Viral RNA was extracted from the blood samples of all participants of the Long-COVID group using the QIAamp MinElute Virus Kit (Qiagen Iberia, Madrid, Spain). The presence of RNA of SARS-CoV-2 was determined in the blood of all participants in this study following the protocol previously described (29), which is part of the Interim Guidance of the WHO for the diagnostic testing of SARS-CoV-2 (30).
One-cycle pseudotyped virus encoding SARS-CoV-2 S glycoprotein and the reporter gene renilla within an HIV-1 Δenv genome (pNL4-3ΔenvRen) (31) was used to perform the infection assay in Vero E6 cells. As previously described, cDNA encoding G614 SARS-CoV-2 S glycoprotein (QHU36824.1) without the last 19 amino acids (32) was cloned into pcDNA3.1 expression vector (4). A mutant clone introducing D614G change was generated by site-directed mutagenesis due to D614 viruses being the majority of the earliest variants detected in Spain within clade 19B (33). A monolayer of Vero E6 was infected with identical amounts of both D614 and G614 pseudoviruses (100 ng p24/Gag per p48 well). After 48 h of incubation, Vero cells were cocultured for 1 h with PBMCs isolated from the blood of individuals from our cohort (1:2). Caspase-3 activity was measured in the monolayer as an indicator of cellular cytotoxicity by using the Caspase-Glo 3/7 Assay system (Promega, Madison, WI, USA).
K562 cells that do not express the Major Histocompatibility Complex (MHC) class I molecules on the cell surface (missing self) were used as classical targets of NK cells to evaluate their cytotoxic activity as previously described (4). Briefly, K562 cells were stained with PKH Red Fluorescence Cell Linker kit (Sigma-Aldrich Merck, Darmstadt, Germany) and then cocultured with PBMCs (1:2). After 1 h, they were collected and stained with Annexin V conjugated with fluorescein isothiocyanate (FITC) (Thermo Fisher, Waltham, MA, USA) to quantify early apoptosis by measuring the expression of phosphatidylserine on the cell surface by flow cytometry. BD LSRFortessa X-20 flow cytometer and FACS Diva software were used for data acquisition, and FlowJo_V10 software was used for data analysis.
A Random Forest algorithm (34) was applied to evaluate the accuracy of those demographic, clinical, and immunological parameters that showed significant differences (p < 0.05) in the comparison with the Recovered group to predict the predisposition to develop Long-COVID. The selected parameters were as follows: demographic factors: female gender and O+ blood type; clinical factors: lethargy, pleuritic chest pain, dermatological injuries, mean body temperature, dyspnea, diarrhea, conjunctivitis, previous autoimmune diseases, and treatments during COVID-19 such as corticosteroids, antibiotics, and/or vitamin D; immune response factors: total NK cells (CD56+), CD3−CD56+CD16+, CD56+NKG2A−NKG2C+, and CD56+CD57+NKG2C+ subpopulations; CD3+PD-1+; total CD8+ T cells, CD8+ TEMRA, and CD8±TCRγδ+ subpopulations; CD4+ Tregs; and cytotoxic activity against NK target cells K562 and/or SARS-CoV-2 infected Vero E6 cells. A nested 5-fold cross-validation procedure for each competing algorithm was performed in order to avoid bias in the selection of training, testing, and validation sets, as previously described (35, 36). The relative importance for each feature in the categorization of patients was calculated by using the Gini Variable Importance Measure (VIM) method (37).
Statistical analysis was performed using Graph Pad Prism 8.0 (Graph Pad Software Inc., San Diego, CA, USA). Quantitative variables were represented as the mean and standard error of the mean (SEM), and significance was analyzed using the unpaired, non-parametric Mann–Whitney test to compare between both Long-COVID and Recovered groups. Qualitative variables were represented as percentages, and significance was analyzed using Fisher’s exact test. p-Values (p) <0.05 were considered statistically significant in all comparisons.
Fifty individuals who passed natural infection by SARS-CoV-2 previous to vaccination were recruited for this study. They were assigned to the Long-COVID group if they showed persistent clinical signs and symptoms from 4 to more than 12 weeks after diagnosis (n = 30), or to the Recovered group if they had resolved all signs and symptoms of COVID-19 in the first 4 weeks after diagnosis. The main demographic and clinical characteristics of all participants are summarized in Tables 1, 2 and detailed in Supplementary Tables 1, 2. Most individuals in the Long-COVID group (86.6%) were female, whereas 55% of the participants in the Recovered group were female (p = 0.0205). Median age at SARS-CoV-2 acute infection in the Long-COVID and Recovered groups was 42 (interquartile range (IQR) 37–46) and 45 years (IQR 28–57), respectively. Median time from the clinical onset to sampling was 49.7 weeks or 348 days (IQR 150–369 days) in the Long-COVID group versus 11.8 weeks or 83 days (IQR 73–99 days) in the Recovered group. Median length with signs and symptoms compatible with Long-COVID at sampling was 49.7 weeks or 348 days (IQR 150–369 days) and 1.8 weeks or 13 days (IQR 0–49 days), respectively. The most frequent Rh blood group was A+ in both cohorts, whereas O+ was present in 36.6% of the individuals from the Long-COVID-19 group and 5% from the Recovered group (p = 0.0160). During COVID-19, individuals from the Long-COVID group reported 38.2°C (± 0.66) of recurrent peaks of fever, in comparison with 37.7°C (± 0.18) of peak fever during the first 10 days post-infection in participants from the Recovered group (p = 0.0156). Dyspnea was developed by the majority of participants from the Long-COVID group (86.6%) and 35% of individuals from the Recovered group (p = 0.0002), whereas pleuritic chest pain was present in 76.6% and 25% of the individuals, respectively (p = 0.0005). Additional signs and symptoms with statistical significance reported by individuals from the Long-COVID group, in comparison with individuals from the Recovered group, were lethargy (80%, p < 0.0001), diarrhea (73.3%, p = 0.0037), dermatological injuries (63.3%, p = 0.0003), and conjunctivitis (30%, p = 0.0073). Most participants from the Recovered group did not receive any drug for the treatment of COVID-19, whereas individuals from the Long-COVID group mostly received antibiotics (70%, p = 0.0012), corticosteroids (50%, p < 0.0001), and/or cholecalciferol/vitamin D (40%, p < 0.0001). The most prevalent comorbidities in the group of Long-COVID were autoimmune diseases (30%, p = 0.0366), including psoriasis, rheumatoid arthritis, Hashimoto’s thyroiditis, mixed connective tissue disease (MCTD), and Raynaud’s disease, versus one participant from the Recovered group (5%) who reported both Sjögren’s syndrome and antiphospholipid syndrome prior to SARS-CoV-2 infection.
Table 1 Demographic and clinical data of all participants from the Long-COVID group and the Recovered group that were recruited for this study.
Table 2 Clinical signs and symptoms reported by the participants from the Long-COVID group recruited for this study.
We did not find differences in the levels of total peripheral CD4+ T cells (Figure 1A) or the composition of CD4+ memory subpopulations between both Long-COVID and Recovered groups (Figure 1B). The total level of lymphocytes (CD3+) expressing the immune exhaustion marker PD-1 on the cell surface was increased 1.5-fold in the Long-COVID group, and this difference nearly reached statistical significance (p = 0.0506) (Figure 1C). The level of regulatory T cells (Tregs) (CD4+CD25+CD127low) was significantly increased 2.5-fold in the individuals from the Long-COVID group (p = 0.0007), in comparison with the Recovered group (Figure 1D). The flow cytometry gating strategy is shown in Supplementary Figure 1.
Figure 1 Analysis of CD3+ and CD4+ T-cell populations in PBMCs from the Long-COVID and Recovered groups. Total levels of CD4+ T cells (A) and CD4+ T-cell memory subpopulations (B) were analyzed by flow cytometry. Individual data are shown in a dot plot, and mean data are shown in the pie charts. (C) The expression of the exhaustion marker PD-1 was analyzed in CD3+ T cells. (D) The levels of CD4+ Tregs were also quantified by flow cytometry in both groups of individuals. Each dot in the graphs corresponds to one sample, and lines represent mean ± standard error of the mean (SEM). Statistical significance was calculated using non-parametric Mann–Whitney test. PBMCs, peripheral blood mononuclear cells.
Total levels of CD8+ T cells were increased 1.5-fold in the Long-COVID group, in comparison with the Recovered group (p = 0.0005) (Figure 2A). We also observed changes in the distribution of CD8+ T-cell memory subpopulations, as the effector CD8+ TEMRA cells were increased 1.4-fold in the Long-COVID group (p = 0.00487), whereas the naïve CD8 T cells were reduced 1.4-fold, in comparison with the Recovered group (Figure 2B). Regarding the highly cytotoxic CD3+ T cells with TCRγδ, both CD8+TCRγδ+ and CD8−TCRγδ+ populations were increased 2.0-fold (p = 0.049) and 2.2-fold (p = 0.005), respectively, in the Long-COVID group in comparison with the Recovered group (Figure 2C). Stimulation of PBMCs with a pool of nucleoprotein peptides from SARS-CoV-2 showed no significant differences in the capacity of CD8+ T cells to release pro-inflammatory cytokines such as IFNγ and TNFα, as well as the serine protease GZB, between individuals with Long-COVID and the Recovered participants (Figure 2D). The flow cytometry gating strategy is shown in Supplementary Figure 2.
Figure 2 Analysis of CD8+ T-cell populations in PBMCs from the Long-COVID and Recovered groups. Total levels of CD8+ T cells (A) and CD8+ T-cell memory subpopulations (B) were analyzed by flow cytometry. Individual data are shown in a dot plot, and mean data are shown in the pie charts. (C) The levels of CD8 ± TCRγδ+ were also determined by flow cytometry in both groups of individuals. (D) Quantification of the release of pro-inflammatory cytokines IFNg and TNFa, as well as the serine protease GZB from PBMCs from individuals with Long COVID and the Recovered participants after stimulation with a pool of nucleoprotein peptides from SARS-CoV-2. Each dot in the graphs corresponds to one sample, and lines represent mean ± SEM. Statistical significance was calculated using non-parametric Mann–Whitney test. PBMCs, peripheral blood mononuclear cells.
The total levels of NK cells, characterized by the expression of the activation marker CD56, were increased 1.7-fold (p = 0.0005) in the Long-COVID group, in comparison with the Recovered group (Figure 3A). No significant differences were found in the expression of the immune exhaustion marker PD-1 in NK cells between both groups (Figure 3B). The population of NK cells expressing CD16 marker on the surface (CD3−CD56+CD16+) was increased 1.7-fold (p = 0.032) in the Long-COVID group (Figure 3C, left graph), but no significant differences were found regarding the expression of the degranulation marker CD107a+ in this population (Figure 3C, right graph). There were no statistically significant differences either between both groups in NK cells not expressing CD16 marker (Figure 3D) or in NKT cell populations (Figures 3E, F). The flow cytometry gating strategy is shown in Supplementary Figure 3.
Figure 3 Analysis of NK and NKT cell subpopulations in PBMCs from the Long-COVID and Recovered groups. Total levels of CD56+ NK/NKT cells were analyzed by flow cytometry (A), as well as the expression of the exhaustion marker PD-1 in CD56+ T cells (B). Total levels of NK cell subpopulation CD56+CD16+ over CD3− population or with the degranulation marker CD107a over this population CD3−CD56+CD16+ (C) and total levels of NK cell subpopulation CD56+CD16− over CD3− population or with the degranulation marker CD107a over this population CD3−CD56+CD16− (D) were also analyzed by flow cytometry. Analysis by flow cytometry of the total levels of NKT cell subpopulation CD56+CD16+ over CD3+ population or with the degranulation marker CD107a over this population CD3+CD56+CD16+ (E) and total levels of NKT cell subpopulation CD56+CD16− over CD3+ population or with the degranulation marker CD107a over this population CD3+CD56+CD16− (F). Each dot in the graphs corresponds to one sample, and lines represent mean ± SEM. Statistical significance was calculated using non-parametric Mann–Whitney test. PBMCs, peripheral blood mononuclear cells.
NK cell subpopulation expressing the activation marker NKG2C (CD56+NKG2A−NKG2C+) was increased 1.4-fold (p = 0.0149) in the Long-COVID group, in comparison with the Recovered group (Figure 4A, left graph), whereas no significant differences were found between both groups in the NK subpopulation with the inhibitory markers NKG2A (CD56+NKG2A+NKG2C−) (Figure 4A, middle graph) or KIR2DL5/CD158f (Figure 4A, right graph). There were no differences in the total levels of NK cells with the memory marker CD57 between both groups (Figure 4B, left graph), but the population of activated memory NK cells (CD56+CD57+NKG2C+) was increased 1.5-fold (p = 0.044) in the Long-COVID group, in comparison with the Recovered group (Figure 4B, right graph). The flow cytometry gating strategy is shown in Supplementary Figure 4.
Figure 4 Analysis of the expression of NK cell markers in PBMCs from the Long-COVID and Recovered groups. (A) Analysis by flow cytometry of the expression of the activating receptor NKG2C and the inhibitory receptors NKG2A and KIR2DL5/CD158f on the surface of total CD56+ cells. (B) Analysis by flow cytometry of the expression of the activating receptor NKG2C and the memory marker CD57 on the surface of total CD56+ cells. Each dot in the graphs corresponds to one sample, and lines represent mean ± SEM. Statistical significance was calculated using non-parametric Mann–Whitney test. PBMCs, peripheral blood mononuclear cells.
The cytotoxic activity of PBMCs from the individuals of the Long-COVID group against the classical target of NK cells K562 was increased 2.3-fold (p < 0.0001), in comparison with the Recovered group (Figure 5A). The cytotoxic activity of PBMCs was also tested against Vero E6 cells infected with pseudotyped SARS-CoV-2. The activation of caspase-3 was increased 1.7-fold (p = 0.0092) in the monolayer when they were cocultured with PBMCs from individuals of the Long-COVID group, in comparison with the Recovered group (Figure 5B).
Figure 5 Measurement of cytotoxic activity of PBMCs from the Long-COVID and Recovered groups. (A) Diagram (left) and dot plot graph (right) of the assay for the quantification by flow cytometry of Annexin V binding to K562 cells cocultured with PBMCs (1:2) from the Long-COVID and Recovered groups for 1 h (B) Diagram (left) and dot plot graph (right) of the assay for the quantification by chemiluminescence of caspase-3 activation in a monolayer of SARS-CoV-2-infected Vero E6 cells cocultured with PBMCs (1:2) from the Long-COVID and Recovered groups for 1 h Each dot in the graphs corresponds to one sample, and lines represent mean ± SEM. Statistical significance was calculated using non-parametric Mann–Whitney test. PBMCs, peripheral blood mononuclear cells.
All individuals from the Long-COVID group showed an absence of detection of RNA from SAR-CoV-2 in blood, except for one individual (Patient ID 26; see Supplementary Table 1) (3.33%) who showed amplification of RNA in blood at cycle threshold (CT) 32.97 (data not shown).
An accuracy of 94% ± 4.90% was obtained for the 5 iterations of the outer loop of the nested K-fold cross validation for each competing algorithm (Figure 6A). As a result, all 30 patients (100%) in the Long-COVID group were correctly assigned to this group, whereas 17 individuals of 20 (85%) were correctly assigned to the Recovered group (Figure 6B). The Gini VIM method determined that clinical parameters such as lethargy, pleuritic chest pain, dermatological injuries, treatment with corticosteroids, immune factors (such as enhanced total levels of NK cells, CD8+ T lymphocytes, and Tregs), and increased cytotoxic activity were the most important variables to assign the individuals to the Long-COVID group (Figure 6C), whereas demographic parameters such as female gender and O+ blood type, or previous history of autoimmune disease, were within the variables with less importance.
Figure 6 Application of Random Forest algorithm and Gini VIM method for the evaluation of the importance of demographic, clinical, and immunological parameters with statistical significance as diagnostic biomarkers for Long-COVID. (A) Calculation of the accuracy for 5 iterations of the outer loop of the nested K-fold cross validation. (B) Confusion matrix confronting the conditions predicted by the algorithm and the true conditions for the correct assignment of the participants to the Long-COVID group or the Recovered group. (C) Classification of the demographic, clinical, and immunological features with statistical significance according to their importance to predict the correct classification of the individuals to the Long-COVID group or the Recovered group. VIM, Variable Importance Measure.
Long-COVID is emerging as a new relevant syndrome worldwide that is characterized by the maintenance of long-term signs and symptoms of COVID-19 that are not resolved in the first 4 weeks after the infection (12). Several host and viral factors may be involved in the development of Long-COVID, although the underlying basic mechanisms are still unknown. To determine the most important host factors that could be used as diagnostic parameters to evaluate the development of Long-COVID, we collected and analyzed essential demographic, clinical, and immunological characteristics in a Spanish cohort of individuals with signs and symptoms compatible with Long-COVID in comparison with individuals who recovered completely before 4 weeks after the infection. A Random Forest algorithm was applied to identify the most important variables to predict the susceptibility to develop Long-COVID.
Regarding the host factors that might be related to Long-COVID, some demographic characteristics such as age and gender have been appointed as contributing host factors for an increased susceptibility (11, 19, 38). Before the general vaccination against COVID-19, most patients admitted to the ICU due to a critical form of the disease were usually men over 65 years old (39). In contrast, most participants in our cohort of Long-COVID were women under 45 years old, which is in accordance with previous studies (18, 40). Rh blood O+ type was also significantly more frequent in the individuals of the Long-COVID group, but we cannot rule out that most Spanish populations present this blood type (41). Although it has been reported that individuals with Rh− blood type may be at lower risk of SARS-CoV-2 infection and development of severe illness or death by COVID-19 (42, 43), other studies did not find a significant impact of Rh on COVID-19 severity (44, 45). In our study, according to the Random Forest analysis, both female gender and Rh blood type were within the less important possible diagnostic factors for Long-COVID. However, clinical signs and symptoms such as persistent lethargy, pleuritic chest pain, and recurrent dermatological injuries showed more relative importance for the prediction of higher susceptibility to Long-COVID.
Considering the potential viral factors that might be involved in the development of Long-COVID, one hypothesis that should be taken into account is that SARS-CoV-2 might find mechanisms to persist in the organism after the acute infection. In this case, Long-COVID syndrome would be a consequence of an unresolved SARS-CoV-2 infection (46). A prolonged SARS-CoV-2 viral shedding in feces has been described several months after the COVID-19 diagnosis (21, 47). This suggests that SARS-CoV-2 may persist in sanctuaries such as the epithelial cells of the gastrointestinal tract or the tissue macrophages in the gut-associated lymphoid tissue (GALT) (48, 49), which would explain not only the continuous viral shedding but also the gastrointestinal symptoms developed by individuals with Long-COVID (50). In fact, alterations in the gut microbiota have been described in individuals with Long-COVID even 6 months after the infection (51). We cannot rule out this possibility in our cohort, as at least 70% of individuals with Long-COVID had been treated with broad-spectrum antibiotics. However, SARS-CoV-2 could also persist in other anatomical sanctuary organs with immune privilege such as the central nervous system (CNS) (52) or in other organs and cells in which ACE2 receptor is highly expressed such as the intestine, kidneys, cardiac tissue, reproductive system, thyroid, gallbladder, and nasal mucosa (53, 54), which would also explicate the highly variable signs and symptoms that are associated with Long-COVID (55). The persistence of SARS-CoV-2 in the organism would also correlate with the recurrent peaks of fever reported by the participants from the Long-COVID group, which was significantly increased at 0.5°C in comparison with the individuals from the Recovered group, in which the fever stopped once the infection was resolved. Although more studies will be necessary to determine whether the virus may be found in the feces of these individuals, low SARS-CoV-2 viremia was detected in one participant from the Long-COVID group (Patient ID 26). This participant was a 44-year-old woman, A+, with previous history of asthma and chronic obstructive pulmonary disease (COPD) who was treated with azithromycin during the first days after COVID-19 diagnosis, had signs and symptoms compatible with Long-COVID at the time of sampling (21.4 weeks post-infection), and did not report a subsequent re-infection after the first exposure to SARS-CoV-2. She informed about persistent neurological symptoms and diarrhea, which would support the possibility of a viral persistence in the CNS or in the GALT, with occasional viremia.
The persistence of SARS-CoV-2 or its viral products in the organism would then contribute to the increased antiviral, cytotoxic activity detected in individuals from the Long-COVID group, along with the associated gastrointestinal symptoms, which were likely also due to the presence of activated immune cells in the GALT (56). Persistent epithelial damage due to a chronic inflammatory response has been appointed as a possible cause of Long-COVID (57). It is noteworthy that patients with critical COVID-19 who are admitted to the ICU usually present CD4+ cytopenia (4, 58), which makes it difficult to develop a complete, effective immune response against SARS-CoV-2. However, the individuals from the Long-COVID group in our study showed not only normal levels of CD4+ T cells in peripheral blood but also CD8+ lymphocytosis with an increased proportion of TEMRA cells, which are highly differentiated effector cells essential for CD8+ function (59). This enhanced terminal differentiation of memory CD8+ T cells pointed at the presence of a potent antiviral immune response that was supported by the presence of increased levels of highly cytotoxic populations of CD8± T lymphocytes expressing TCRγδ. These cells have been related to a potent antiviral and antineoplastic response, and therefore, they are currently the target of different strategies to reprogram their cytotoxic potential against specific targets such as viruses or cancerous cells (60, 61). Although the capacity of CD8+ T cells to produce pro-inflammatory cytokines and GZB was not significantly different between individuals with Long-COVID and those who completely recovered, the sustained presence of these cytotoxic cell populations from the adaptive immune system indicated that the participants from the Long-COVID group had developed a potent memory response against SARS-CoV-2 that was still fully active after more than 49 weeks post-infection, whereas this response had waned in the Recovered group once the infection was cleared. The increased expression of exhaustion markers such as PD-1 in CD3+ T lymphocytes from these individuals of the Long-COVID group could be a consequence of the continuous activation of the immune system. The PD-1/PD-L1 axis is upregulated during acute viral infection and after infection with persistent RNA viruses such as HIV and HCV, and it has been related to viral evasion of the immune system and prolonged inflammatory responses (62). Moreover, the significant increase of Tregs observed in PBMCs from individuals of the Long-COVID group may indicate the failed attempt of the immune system to control this persistent immune response.
The long-term activation of the immune system in response to triggers such as pathogens, vaccines, drugs, or chemicals has been previously related to the development of autoimmune diseases due to the loss of tolerance (63). The link between the development of autoimmunity and a previous infection is not always clear, as occurs with common autoimmune diseases such as lupus erythematosus, multiple sclerosis, type 1 diabetes mellitus, and rheumatoid arthritis. However, the discovery of autoantibodies in individuals with Long-COVID may link this syndrome not only to a persistent viral infection but also to an immune dysregulation that would lead to a sustained targeting of own cells (26, 64). The detection of high levels of functional cytotoxic memory cells in peripheral blood of the participants from the Long-COVID group may explain why several signs and symptoms of this syndrome resembled those of an autoimmune disease. Some previous predisposition may be then contributing since the most prevalent comorbidities showed by these individuals were autoimmune diseases, although they were not considered within the most accurate diagnostic biomarkers for Long-COVID in our Random Forest model. However, the hypothesis that a persistent memory cytotoxic response against SARS-CoV-2 could be the cause for the development of Long-COVID was supported in our cohort by the presence not only of high levels of CD8+ TEMRA cells but also of NK cells expressing both memory (CD57) and activation (NKG2C) markers that did not waste and wane after SARS-CoV-2 infection was cleared, despite the presence of high levels of Tregs. Moreover, these immunological factors were considered the most important variables for the prediction of a higher susceptibility to develop Long-COVID in our Random Forest model.
The difference in the median time from the clinical onset to sampling in the Long-COVID group versus the Recovered group could be considered a potential limitation of our study. However, all participants were infected with SARS-CoV-2 during the same period of time that was the first pandemic peak in Spain (March to April 2020), and whereas all signs and symptoms of COVID-19 were completely resolved at the time of sampling (12 weeks post-infection) in all participants of the Recovered group, the individuals of the Long-COVID group still presented recurrent signs and symptoms of the disease more than 49 weeks post-infection. Therefore, all inclusion criteria were met in both groups.
In conclusion, Long-COVID syndrome could be the consequence of a long-lasting memory cytotoxic immune response that had been triggered by SARS-CoV-2, or its viral products are hidden in some anatomical sanctuaries such as the CNS or tissue macrophages in the GALT, where it would be protected from clearance by the immune system. However, we also have to consider that Long-COVID shows characteristics of autoimmune disease and that infected individuals may be more susceptible to developing this syndrome due to the activation of an exacerbated memory immune response that cannot be adequately controlled by homeostatic mechanisms once the infection was cleared. Having a better understanding of the underlying mechanisms of Long-COVID could be helpful to prevent the development of this syndrome and to improve the clinical management of these patients. According to our Random Forest model, 100% of the participants included in the Long-COVID group were correctly assigned to this group using parameters related to long-lasting cytotoxicity, which proved their validity as biomarkers to diagnose the development of this syndrome.
The original contributions presented in the study are included in the article/Supplementary Material. Further inquiries can be directed to the corresponding authors.
The studies involving human participants were reviewed and approved by the Ethical Committee of Instituto de Salud Carlos III (IRB IORG0006384) (CEI PI 07_2021) and the Central Research Commission from the Health Counseling (Comunidad de Madrid, Spain) (favorable report 20210008). The patients/participants provided their written informed consent to participate in this study.
Esther Alonso Herrador1, Pablo Amich Alemany1, Victoria Bosch Martos1, Lorena Cordova-Castaño1, Aurora Expósito Mora1, Javier García-Pérez2,3, María Mercedes Gea Martinez1, Alberto Gomez Bonilla1, María Victoria Leon Gomez1, Gema Lora Rey1, Maria Luisa Muñoz Balsa1, Javier Pérez Gonzalez1, Sandra Pérez-Santos1, Jose Sanchez Hernández1, Andrea Vinssac Rayado1
1Centro de Salud Doctor Pedro Laín Entralgo, Alcorcón, Spain.
2AIDS Immunopathology Unit, National Center of Microbiology, Instituto de Salud Carlos III, Majadahonda, Madrid, Spain.
3Biomedical Research Center Network in Infectious Diseases (CIBERINFEC), Spain
MC and ML-H conceptualized the project. MG, LV, MC, and ML-H wrote the manuscript. LV, SR-M, FR-M, MT, GC, and EM processed and stored all blood samples. MM-A, SD-M, and MT identified, selected, and recruited the patients. MG, LV, GC, MT, EM, and SR-M performed the analytical experiments. LV and MG collected and analyzed the clinical data and laboratory results. DF and VP performed the Random Forest analysis. All co-authors read and approved the final version of the manuscript.
This work was supported by the Coordinated Research Activities at the National Center of Microbiology (CNM, Instituto de Salud Carlos III) (COV20_00679) to promote an integrated response against SARS-CoV-2 in Spain (Spanish Ministry of Science and Innovation), which is coordinated by Dr Inmaculada Casas (WHO National Influenza Center of the CNM); a generous donation provided by Chiesi España, S.A.U. (Barcelona, Spain); the Spanish Ministry of Science and Innovation (PID2019-110275RB-I00); and the Spanish AIDS Research Network RD16CIII/0002/0001 that is included in Acción Estratégica en Salud, Plan Nacional de Investigación Científica, Desarrollo e Innovación Tecnológica 2016-2020, Instituto de Salud Carlos III, European Region Development Fund (ERDF). The work of ML-H and SR-M is financed by NIH grant R01AI143567. The work of MT is supported by Instituto de Salud Carlos III (COV20_00679). The work of LV is supported by a pre-doctoral grant from Instituto de Salud Carlos III (FIS PI16CIII/00034-ISCIII-FEDER). The work of FR-M is financed by the Spanish Ministry of Science and Innovation (PID2019-110275RB-I00).
The authors declare that the research was conducted in the absence of any commercial or financial relationships that could be construed as a potential conflict of interest.
All claims expressed in this article are solely those of the authors and do not necessarily represent those of their affiliated organizations, or those of the publisher, the editors and the reviewers. Any product that may be evaluated in this article, or claim that may be made by its manufacturer, is not guaranteed or endorsed by the publisher.
We greatly appreciate all the participants for their contribution to this study. We also thank the excellent secretarial assistance of Mrs Olga Palao.
The Supplementary Material for this article can be found online at: https://www.frontiersin.org/articles/10.3389/fimmu.2022.848886/full#supplementary-material
1. WHO. Coronavirus (COVID-19) Dashboard. Available at: https://covid19.who.int/.
2. Kandeel M, Mohamed MEM, Abd El-Lateef HM, Venugopala KN, El-Beltagi HS. Omicron Variant Genome Evolution and Phylogenetics. J Med Virol (2021) 94(4):1627–32. doi: 10.1002/jmv.27515
3. Chen N, Zhou M, Dong X, Qu J, Gong F, Han Y, et al. Epidemiological and Clinical Characteristics of 99 Cases of 2019 Novel Coronavirus Pneumonia in Wuhan, China: A Descriptive Study. Lancet (2020) 395:507–13. doi: 10.1016/S0140-6736(20)30211-7
4. Vigón L, Fuertes D, García-Pérez J, Torres M, Rodríguez-Mora S, Mateos E, et al. Impaired Cytotoxic Response in PBMCs From Patients With COVID-19 Admitted to the ICU: Biomarkers to Predict Disease Severity. Front Immunol (2021) 12:665329:665329. doi: 10.3389/fimmu.2021.665329
5. Oronsky B, Larson C, Hammond TC, Oronsky A, Kesari S, Lybeck M. Reid TR. A Review of Persistent Post-COVID Syndrome (PPCS). Clin Rev Allergy Immunol (2021) 20:1–9. doi: 10.1007/s12016-021-08848-3
6. Ruan Q, Yang K, Wang W, Jiang L, Song J. Clinical Predictors of Mortality Due to COVID-19 Based on an Analysis of Data of 150 Patients From Wuhan, China. Intensive Care Med (2020) 46:846–8. doi: 10.1007/s00134-020-05991-x
7. SeyedAlinaghi S, Afsahi AM, MohsseniPour M, Behnezhad F, Salehi MA, Barzegary A, et al. Late Complications of COVID-19; a Systematic Review of Current Evidence. Arch Acad Emerg Med (2021) 9:e14. doi: 10.22037/aaem.v9i1.1058
8. Rees EM, Nightingale ES, Jafari Y, Waterlow NR, Clifford S, B. Pearson CA, et al. COVID-19 Length of Hospital Stay: A Systematic Review and Data Synthesis. BMC Med (2020) 18:270. doi: 10.1186/s12916-020-01726-3
9. Lopez-Leon S, Wegman-Ostrosky T, Perelman C, Sepulveda R, Rebolledo PA, Cuapio A, et al. More Than 50 Long-Term Effects of COVID-19: A Systematic Review and Meta-Analysis. Sci Rep (2021) 11:16144. doi: 10.1038/s41598-021-95565-8
10. Yang K, Wen G, Wang J, Zhou S, Da W, Meng Y, et al. Complication and Sequelae of COVID-19: What Should We Pay Attention to in the Post-Epidemic Era. Front Immunol (2021) 12:711741. doi: 10.3389/fimmu.2021.711741
11. Sudre CH, Murray B, Varsavsky T, Graham MS, Penfold RS, Bowyer RC, et al. Attributes and Predictors of Long COVID. Nat Med (2021) 27:626–31. doi: 10.1038/s41591-021-01292-y
12. Excellence NI for H and C. NICE. COVID-19 Rapid Guideline: Managing the Long-Term Effects of COVID-19 (2021). Available at: https://www.nice.org.uk/guidance/ng188/resources/covid19-rapid-guideline-managing-the-longterm-effects-of-covid19-pdf-51035515742.
13. Greenhalgh T, Knight M, A’Court C, Buxton M, Husain L. Management of Post-Acute Covid-19 in Primary Care. BMJ (2020) 370:m3026. doi: 10.1136/bmj.m3026
14. Mahase E. Covid-19: What do We Know About “Long Covid”? BMJ (2020) 370:m2815. doi: 10.1136/bmj.m2815
15. World Health Organization RO for E. New Policy Brief Calls on Decision-Makers to Support Patients as 1 in 10 Report Symptoms of “Long COVID.” (2021). Available at: https://www.euro.who.int/en/health-topics/health-emergencies/coronavirus-covid-19/news/news/2021/2/new-policy-brief-calls-on-decision-makers-to-support-patients-as-1-in-10-report-symptoms-of-long-covid.
16. Mitrani RD, Dabas N, Goldberger JJ. COVID-19 Cardiac Injury: Implications for Long-Term Surveillance and Outcomes in Survivors. Hear Rhythm (2020) 17:1984–90. doi: 10.1016/j.hrthm.2020.06.026
17. Ladds E, Rushforth A, Wieringa S, Taylor S, Rayner C, Husain L, et al. Persistent Symptoms After Covid-19: Qualitative Study of 114 “Long Covid” Patients and Draft Quality Principles for Services. BMC Health Serv Res (2020) 20:1144. doi: 10.1186/s12913-020-06001-y
18. Sigfrid L, Drake TM, Pauley E, Jesudason EC, Olliaro P, Lim WS, et al. Long Covid in Adults Discharged From UK Hospitals After Covid-19: A Prospective, Multicentre Cohort Study Using the ISARIC WHO Clinical Characterisation Protocol. Lancet Reg Heal Eur (2021) 8:100186. doi: 10.1016/j.lanepe.2021.100186
19. Stewart S, Newson L, Briggs TA, Grammatopoulos D, Young L, Gill P. Long COVID Risk - a Signal to Address Sex Hormones and Women’s Health. Lancet Reg Heal - Eur (2021) 11:100242. doi: 10.1016/j.lanepe.2021.100242
20. Zhou Y, Zhang Y, Moorman JP, Yao ZQ, Jia ZS. Viral (Hepatitis C Virus, Hepatitis B Virus, HIV) Persistence and Immune Homeostasis. Immunology (2014) 143:319–30. doi: 10.1111/imm.12349
21. Xing Y-H, Ni W, Wu Q, Li W-J, Li G-J, Wang W-D, et al. Prolonged Viral Shedding in Feces of Pediatric Patients With Coronavirus Disease 2019. J Microbiol Immunol Infect (2020) 53:473–80. doi: 10.1016/j.jmii.2020.03.021
22. Arostegui D, Castro K, Schwarz S, Vaidy K, Rabinowitz S, Wallach T. Persistent SARS-CoV-2 Nucleocapsid Protein Presence in the Intestinal Epithelium of a Pediatric Patient 3 Months After Acute Infection. JPGN Rep (2022) 3:e152. doi: 10.1097/PG9.0000000000000152
23. To K, Tong JH, Chan PK, Au FW, Chim SS, Allen Chan K, et al. Tissue and Cellular Tropism of the Coronavirus Associated With Severe Acute Respiratory Syndrome: An in-Situ Hybridization Study of Fatal Cases. J Pathol (2004) 202:157–63. doi: 10.1002/path.1510
24. Cardinale V, Capurso G, Ianiro G, Gasbarrini A, Arcidiacono PG, Alvaro D. Intestinal Permeability Changes With Bacterial Translocation as Key Events Modulating Systemic Host Immune Response to SARS-CoV-2: A Working Hypothesis. Dig Liver Dis (2020) 52:1383–9. doi: 10.1016/j.dld.2020.09.009
25. Chen L, Deng H, Cui H, Fang J, Zuo Z, Deng J, et al. Inflammatory Responses and Inflammation-Associated Diseases in Organs. Oncotarget (2018) 9:7204–18. doi: 10.18632/oncotarget.23208
26. Wang EY, Mao T, Klein J, Dai Y, Huck JD, Jaycox JR, et al. Diverse Functional Autoantibodies in Patients With COVID-19. Nature (2021) 595:283–8. doi: 10.1038/s41586-021-03631-y
27. Bastard P, Rosen LB, Zhang Q, Michailidis E, Hoffmann H-H, Zhang Y, et al. Autoantibodies Against Type I IFNs in Patients With Life-Threatening COVID-19. Sci (80- ) (2020) 370:6515. doi: 10.1126/science.abd4585
28. Alwan NA. A Negative COVID-19 Test Does Not Mean Recovery. Nature (2020) 584:170–0. doi: 10.1038/d41586-020-02335-z
29. Corman VM, Landt O, Kaiser M, Molenkamp R, Meijer A, Chu DK, et al. Detection of 2019 Novel Coronavirus (2019-Ncov) by Real-Time RT-PCR. Eurosurveillance (2020) 25:2000045. doi: 10.2807/1560-7917.ES.2020.25.3.2000045
30. WHO/2019-nCoV/laboratory/2020.6. Emergencies Preparedness, WHO Headquarters (HQ). Diagnostic Testing for SARS-CoV-2. Interim Guidance 2020. Geneva: World Health Organization (WHO) (2020).
31. Garcia-Perez J, Sanchez-Palomino S, Perez-Olmeda M, Fernandez B, Alcami J. A New Strategy Based on Recombinant Viruses as a Tool for Assessing Drug Susceptibility of Human Immunodeficiency Virus Type 1. J Med Virol (2007) 79:127–37. doi: 10.1002/jmv.20770
32. Ou X, Liu Y, Lei X, Li P, Mi D, Ren L, et al. Characterization of Spike Glycoprotein of SARS-CoV-2 on Virus Entry and its Immune Cross-Reactivity With SARS-CoV. Nat Commun (2020) 11:1620. doi: 10.1038/s41467-020-15562-9
33. Díez-Fuertes F, Iglesias-Caballero M, García-Pérez J, Monzón S, Jiménez P, Varona S, et al. A Founder Effect Led Early SARS-CoV-2 Transmission in Spain. J Virol (2021) 95(3):e01583–20. doi: 10.1128/JVI.01583-20
35. Meyer HV, Birney E. PhenotypeSimulator: A Comprehensive Framework for Simulating Multi-Trait, Multi-Locus Genotype to Phenotype Relationships. Bioinformatics (2018) 34:2951–6. doi: 10.1093/bioinformatics/bty197
36. Sarica A, Cerasa A, Quattrone A. Random Forest Algorithm for the Classification of Neuroimaging Data in Alzheimer’s Disease: A Systematic Review. Front Aging Neurosci (2017) 9:329. doi: 10.3389/fnagi.2017.00329
37. Nembrini S, König IR, Wright MN. The Revival of the Gini Importance? Bioinformatics (2018) 34:3711–8. doi: 10.1093/bioinformatics/bty373
38. Bai F, Tomasoni D, Falcinella C, Barbanotti D, Castoldi R, Mulè G, et al. Female Gender is Associated With Long COVID Syndrome: A Prospective Cohort Study. Clin Microbiol Infect (2021) S1198:00629–7. doi: 10.1016/j.cmi.2021.11.002
39. Mallapaty S. The Coronavirus is Most Deadly If You are Older and Male — New Data Reveal the Risks. Nature (2020) 585:16–7. doi: 10.1038/d41586-020-02483-2
40. Taquet M, Dercon Q, Luciano S, Geddes JR, Husain M, Harrison PJ. Incidence, Co-Occurrence, and Evolution of Long-COVID Features: A 6-Month Retrospective Cohort Study of 273,618 Survivors of COVID-19. PloS Med (2021) 18:e1003773. doi: 10.1371/journal.pmed.1003773
41. Campillo FL, Gallardo LE, Senra A. ABO and Rh Blood Groups in Spain. Rev Sanid Hig Publica (Madr) (1971) 45:1057–64.
42. Ray JG, Schull MJ, Vermeulen MJ, Park AL. Association Between ABO and Rh Blood Groups and SARS-CoV-2 Infection or Severe COVID-19 Illness. Ann Intern Med (2021) 174:308–15. doi: 10.7326/M20-4511
43. Zietz M, Zucker J, Tatonetti NP. Associations Between Blood Type and COVID-19 Infection, Intubation, and Death. Nat Commun (2020) 11:5761. doi: 10.1038/s41467-020-19623-x
44. Leaf RK, Al-Samkari H, Brenner SK, Gupta S, Leaf DE. ABO Phenotype and Death in Critically Ill Patients With COVID-19. Br J Haematol (2020) 190:e204–8. doi: 10.1111/bjh.16984
45. Kim Y, Latz CA, DeCarlo CS, Lee S, Png CYM, Kibrik P, et al. Relationship Between Blood Type and Outcomes Following COVID-19 Infection. Semin Vasc Surg (2021) 34:125–31. doi: 10.1053/j.semvascsurg.2021.05.005
46. Proal AD, VanElzakker MB. Long COVID or Post-Acute Sequelae of COVID-19 (PASC): An Overview of Biological Factors That May Contribute to Persistent Symptoms. Front Microbiol (2021) 12:698169:698169. doi: 10.3389/fmicb.2021.698169
47. Liu J, Xiao Y, Shen Y, Shi C, Chen Y, Shi P, et al. Detection of SARS-CoV-2 by RT-PCR in Anal From Patients Who Have Recovered From Coronavirus Disease 2019. J Med Virol (2020) 92:1769–71. doi: 10.1002/jmv.25875
48. Song X, Hu W, Yu H, Zhao L, Zhao Y, Zhao X, et al. Little to No Expression of Angiotensin-Converting Enzyme-2 on Most Human Peripheral Blood Immune Cells But Highly Expressed on Tissue Macrophages. Cytom Part A (2020):cyto.a.24285. doi: 10.1002/cyto.a.24285
49. Lamers MM, Beumer J, van der Vaart J, Knoops K, Puschhof J, Breugem TI, et al. SARS-CoV-2 Productively Infects Human Gut Enterocytes. Science (2020) 369:50–4. doi: 10.1126/science.abc1669
50. Yusuf F, Fahriani M, Mamada SS, Frediansyah A, Abubakar A, Maghfirah D, et al. Global Prevalence of Prolonged Gastrointestinal Symptoms in COVID-19 Survivors and Potential Pathogenesis: A Systematic Review and Meta-Analysis. F1000Research (2021) 10:301. doi: 10.12688/f1000research.52216.1
51. Liu Q, Mak JWY, Su Q, Yeoh YK, Lui GC-Y, Ng SSS, et al. Gut Microbiota Dynamics in a Prospective Cohort of Patients With Post-Acute COVID-19 Syndrome. Gut (2022) 71:544–52. doi: 10.1136/gutjnl-2021-325989
52. Meinhardt J, Radke J, Dittmayer C, Franz J, Thomas C, Mothes R, et al. Olfactory Transmucosal SARS-CoV-2 Invasion as a Port of Central Nervous System Entry in Individuals With COVID-19. Nat Neurosci (2021) 24:168–75. doi: 10.1038/s41593-020-00758-5
53. Hamming I, Timens W, Bulthuis M, Lely A, Navis G, van Goor H. Tissue Distribution of ACE2 Protein, the Functional Receptor for SARS Coronavirus. A First Step in Understanding SARS Pathogenesis. J Pathol (2004) 203:631–7. doi: 10.1002/path.1570
54. Salamanna F, Maglio M, Landini MP, Fini M. Body Localization of ACE-2: On the Trail of the Keyhole of SARS-CoV-2. Front Med (2020) 7:594495:594495. doi: 10.3389/fmed.2020.594495
55. Cabrera Martimbianco AL, Pacheco RL, Bagattini ÂM, Riera R. Frequency, Signs and Symptoms, and Criteria Adopted for Long COVID-19: A Systematic Review. Int J Clin Pract (2021) 75:e14357. doi: 10.1111/ijcp.14357
56. Lehmann M, Allers K, Heldt C, Meinhardt J, Schmidt F, Rodriguez-Sillke Y, et al. Human Small Intestinal Infection by SARS-CoV-2 is Characterized by a Mucosal Infiltration With Activated CD8+ T Cells. Mucosal Immunol (2021) 14:1381–92. doi: 10.1038/s41385-021-00437-z
57. Raveendran AV, Jayadevan R, Sashidharan S. Long COVID: An Overview. Diabetes Metab Syndr Clin Res Rev (2021) 15:869–75. doi: 10.1016/j.dsx.2021.04.007
58. Wang F, Nie J, Wang H, Zhao Q, Xiong Y, Deng L, et al. Characteristics of Peripheral Lymphocyte Subset Alteration in COVID-19 Pneumonia. J Infect Dis (2020) 221:1762–9. doi: 10.1093/infdis/jiaa150
59. Jacquemont L, Tilly G, Yap M, Doan-Ngoc T-M, Danger R, Guérif P, et al. Terminally Differentiated Effector Memory CD8 + T Cells Identify Kidney Transplant Recipients at High Risk of Graft Failure. J Am Soc Nephrol (2020) 31:876–91. doi: 10.1681/ASN.2019080847
60. Raverdeau M, Cunningham SP, Harmon C, Lynch L. γδ T Cells in Cancer: A Small Population of Lymphocytes With Big Implications. Clin Transl Immunol (2019) 8:e01080. doi: 10.1002/cti2.1080
61. Sabbaghi A, Miri SM, Keshavarz M, Mahooti M, Zebardast A, Ghaemi A. Role of γδ T Cells in Controlling Viral Infections With a Focus on Influenza Virus: Implications for Designing Novel Therapeutic Approaches. Virol J (2020) 17:174. doi: 10.1186/s12985-020-01449-0
62. Schönrich G, Raftery MJ. The PD-1/PD-L1 Axis and Virus Infections: A Delicate Balance. Front Cell Infect Microbiol (2019) 9:207. doi: 10.3389/fcimb.2019.00207
63. Selmi C, Gao B, Gershwin ME. The Long and Latent Road to Autoimmunity. Cell Mol Immunol (2018) 15:543–6. doi: 10.1038/s41423-018-0018-y
Keywords: Long-COVID, cytotoxic immune response, immune exhaustion, CD8+ T cells, NK cells, Random Forest algorithm
Citation: Galán M, Vigón L, Fuertes D, Murciano-Antón MA, Casado-Fernández G, Domínguez-Mateos S, Mateos E, Ramos-Martín F, Planelles V, Torres M, Rodríguez-Mora S, López-Huertas MR and Coiras M (2022) Persistent Overactive Cytotoxic Immune Response in a Spanish Cohort of Individuals With Long-COVID: Identification of Diagnostic Biomarkers. Front. Immunol. 13:848886. doi: 10.3389/fimmu.2022.848886
Received: 05 January 2022; Accepted: 25 February 2022;
Published: 25 March 2022.
Edited by:
Lucia Lopalco, San Raffaele Hospital (IRCCS), ItalyReviewed by:
Ester Gea-Mallorquí, University of Oxford, United KingdomCopyright © 2022 Galán, Vigón, Fuertes, Murciano-Antón, Casado-Fernández, Domínguez-Mateos, Mateos, Ramos-Martín, Planelles, Torres, Rodríguez-Mora, López-Huertas and Coiras. This is an open-access article distributed under the terms of the Creative Commons Attribution License (CC BY). The use, distribution or reproduction in other forums is permitted, provided the original author(s) and the copyright owner(s) are credited and that the original publication in this journal is cited, in accordance with accepted academic practice. No use, distribution or reproduction is permitted which does not comply with these terms.
*Correspondence: Mayte Coiras, bWNvaXJhc0Bpc2NpaWkuZXM=; María Rosa López-Huertas, bXJsaHVlcnRhc0Bpc2NpaWkuZXM=
†These authors have contributed equally to this work and share first authorship
‡These authors have contributed equally to this work and share last authorship
Disclaimer: All claims expressed in this article are solely those of the authors and do not necessarily represent those of their affiliated organizations, or those of the publisher, the editors and the reviewers. Any product that may be evaluated in this article or claim that may be made by its manufacturer is not guaranteed or endorsed by the publisher.
Research integrity at Frontiers
Learn more about the work of our research integrity team to safeguard the quality of each article we publish.