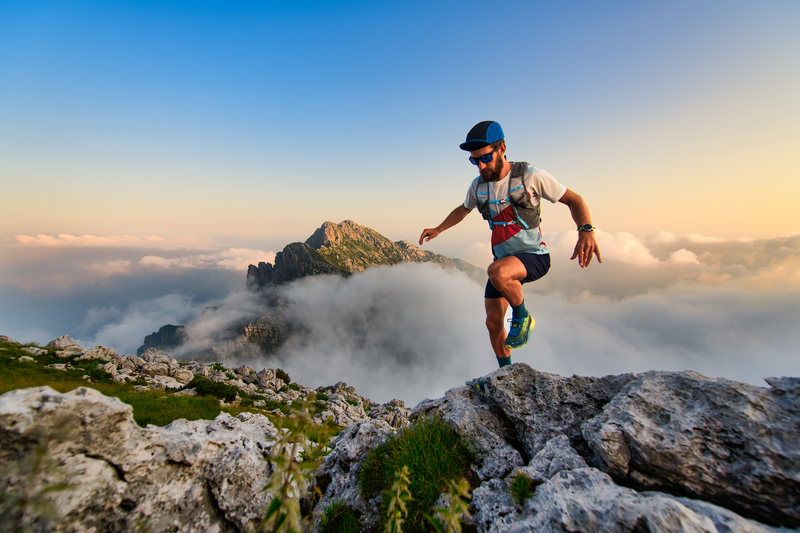
95% of researchers rate our articles as excellent or good
Learn more about the work of our research integrity team to safeguard the quality of each article we publish.
Find out more
ORIGINAL RESEARCH article
Front. Immunol. , 27 April 2022
Sec. Autoimmune and Autoinflammatory Disorders
Volume 13 - 2022 | https://doi.org/10.3389/fimmu.2022.843576
This article is part of the Research Topic New Biomarkers for the Diagnosis and Treatment of Systemic Lupus Erythematosus View all 16 articles
Introduction: Lupus nephritis (LN) is a major risk factor of morbidity and mortality. Glomerular injury is associated with different pathogeneses and clinical presentations in LN patients. However, the molecular mechanisms involved are not well understood. This study aimed to explore the molecular characteristics and mechanisms of this disease using bioinformatics analysis.
Methods: To characterize glomeruli in LN, microarray datasets GSE113342 and GSE32591 were downloaded from the Gene Expression Omnibus database and analyzed to determine the differentially expressed genes (DEGs) between LN glomeruli and normal glomeruli. Functional enrichment analyses and protein–protein interaction network analyses were then performed. Module analysis was performed using the Search Tool for the Retrieval of Interacting Genes/Proteins and Cytoscape software. Immunofluorescence staining was performed to identify the glomerular expression of S100A8 in various International Society of Nephrology/Renal Pathology Society (ISN/RPS) class LN patients. The image of each glomerulus was acquired using a digital imaging system, and the green fluorescence intensity was quantified using Image-Pro Plus software.
Results: A total of 13 DEGs, consisting of 12 downregulated genes and one upregulated gene (S100A8), were identified in the microarray datasets. The functions and pathways associated with the DEGs mainly include inflammatory response, innate immune response, neutrophil chemotaxis, leukocyte migration, cell adhesion, cell–cell signaling, and infection. We also found that monocytes and activated natural killer cells were upregulated in both GSE113342 and GSE32591. Glomerular S100A8 staining was significantly enhanced compared to that in the controls, especially in class IV.
Conclusions: The DEGs identified in the present study help us understand the underlying molecular mechanisms of LN. Our results show that glomerular S100A8 expression varies in different pathological types; however, further research is required to confirm the role of S100A8 in LN.
We use a bioinformatics method to obtain the DEGs between LN glomerulus and normal glomerulus and performed immunofluorescence staining to identify the expression of S100A8 in various ISN/RPS class LN patients.
➣Monocytes and activated NK cells were upregulated in LN glomerulus.
➣Glomerular S100A8 is different in different pathological types.
➣The glomerular S100A8 staining was obviously enhanced compared with the controls, especially in class IV.
Systemic lupus erythematosus (SLE) is an autoimmune disease characterized by immune inflammation, which can affect multiple organs. It is known to affect the kidneys in approximately 50% of patients (1). Lupus nephritis (LN) is a major risk factor of morbidity and mortality. It was found that glomerular injury, including that of mesangial cells, endothelial cells, and podocytes, is associated with different pathogeneses and clinical presentations in LN patients (2). Intense efforts have been made to elucidate the pathogenesis and molecular mechanisms of LN; however, they are still not well understood (3). Therefore, it is necessary to further explore the molecular characteristics and mechanisms of the disease. To characterize glomeruli in LN using bioinformatics analysis, microarray datasets GSE113342 and GSE32591 were downloaded from the Gene Expression Omnibus (GEO) database and analyzed to determine the differentially expressed genes (DEGs) between the LN glomerulus and normal glomerulus. Functional enrichment analyses and protein–protein interaction (PPI) network analyses were then performed. Module analysis was performed using the Search Tool for the Retrieval of Interacting Genes/Proteins (STRING) and Cytoscape software.
S100A8 was identified as one of the 13 DEGs. However, its mRNA expression was different in the abovementioned two microarray datasets. S100A8 (also known as MRP8) is a calcium-binding protein belonging to the S100 family (4). It is mainly expressed in granulocytes and mononuclear blood cells, such as neutrophils and macrophages (5). S100A8 is expressed in various autoimmune diseases, such as systemic sclerosis, psoriasis, dermatomyositis, and Sjogren’s syndrome (6). Some studies have also found that serum and urine S100A8 levels are elevated in patients with LN (7, 8). To our knowledge, the glomerular expression of S100A8 in various ISN/RPS class LN patients is unknown. In this study, immunofluorescence staining and semiquantitative analysis were performed, and the relationship between glomerular expression of S100A8 and clinical data, such as disease activity or urinary protein measurements, was explored.
NCBI GEO (http://www.ncbi.nlm.nih.gov/geo) (9) is a public functional genomics data repository with high-throughput gene expression data, chips, and microarrays. We used “lupus nephritis” (keywords) to search, and there were 335 results in the GEO Database. Then, we selected Homo sapiens, and 301 results were left. We browsed 301 links in detail. In this study, we want to explore the DEGs between LN glomerulus and normal glomerulus. In the first step, we selected LN kidney samples. And only 5 datasets were left. They were GSE112943, GSE127797, GSE113342, GSE32591, and GSE69438. Then, only glomeruli and tubulointerstitium separated samples were what we needed, and GSE113342 and GSE32591 were selected. The two gene expression datasets GSE113342 (10) and GSE32591 (11) were downloaded. The GSE113342 dataset contained 14 LN glomerulus biopsy samples and six normal glomerulus biopsy samples. GSE32591 contained 32 LN glomerulus biopsy samples and 14 normal glomerulus biopsy samples.
The GEO2R online tool was used to identify DEGs between LN and normal glomerular biopsy samples. Log fold change (FC) >1 and P-value <0.01 were considered statistically significant. The raw data were checked online using Venn software to detect common DEGs. DEGs with log FC <0 were considered downregulated, whereas DEGs with log FC >0 were considered upregulated.
The Database for Annotation, Visualization, and Integrated Discovery (DAVID; http://david.ncifcrf.gov) (12) is an online biological information database. The Kyoto Encyclopedia of Genes and Genomes (KEGG) is a database resource for understanding high-level functions and biological systems from large-scale molecular datasets generated using high-throughput experimental technologies (13). Gene Ontology (GO) is a major bioinformatics tool for annotating genes and analyzing their biological processes (14). DAVID 6.8 Bioinformatics Resources was used for pathway annotations. Statistical significance was set at P < 0.05. A PPI network was established using the STRING (version 10.0) (15) online search tool, and an interaction with a combined score >0.4 was considered statistically significant. The PPI network was visualized using Cytoscape (version 3.7.2).
To assess the expression changes in immune cells and obtain the proportion of various types of immune cells from the glomerulus, we used the online CIBERSORT algorithm (https://cibersort.stanford.edu/). The GSE113342 and GSE32591 series matrix txt format files were downloaded from the NCBI GEO website, and the glomerular expression data were selected (GSM3103966, GSM3103968, GSM3103970, GSM3103972, GSM3103974, GSM3103976, GSM3103978, GSM3103980, GSM3103982, GSM3103984, GSM3103986, GSM3103988, GSM3103990, GSM3103992, GSM3103994-99, GSM807889-7934). Differences in 22 immune cells between normal and lupus glomeruli were analyzed.
All protocols were approved by the ethics committee of the Fujian Provincial Hospital. Overall, 30 LN patients with a mean age of 32.70 ± 12.17 years were included. Six types of pathological classifications (Classes II, III, IV, V, III+V, and IV+V) were used, with five patients in each pathological classification. Normal renal tissues from 5 patients with a mean age of 55.47 ± 8.82 years who underwent nephrectomy due to renal tumors were used as normal controls.
Renal biopsy specimens were embedded in an OCT mixture (Sakura, Hayward, CA, USA) and sliced into 5-μm frozen sections (16). The mouse anti-S100A8 antibody (Proteintech Group, Inc., Chicago, IL, USA) was used. Rabbit anti-synaptopodin antibody (Proteintech Group, Inc., Chicago, IL, USA) was used as a podocyte marker for double immunofluorescence staining. Goat anti-rabbit IgG/Alexa Fluor 594 antibody and goat anti-mouse IgG/Alexa Fluor 488 antibody (BIOGOT, Nanjing, China) were used to visualize the different proteins. 4',6-diamidino-2-phenylindole (DAPI) was used to stain the nucleus. Images were captured using a fluorescence microscope (Nikon Eclipse C1, Japan). Integral optical density (IOD) and the area ratio (AR) of the positively stained area to the glomerular area were used as semiquantitative values of the expression of S100A8. We used Image-Pro Plus software (17).
The clinical data are from the cohort used for fluorescence staining of S100A8 in our study. Thirty LN patients were used for this correlation analysis. Six types of pathological classifications (Classes II, III, IV, V, III+V, and IV+V) were used, with five patients in each pathological classification.
SPSS (version 21.0; SPSS Inc., Chicago, IL, USA) was used to compare the differences between the two groups using the t-test or Mann–Whitney U test. The ratio was calculated using the χ2 test. Spearman correlation analysis was performed between clinical data and glomerular S100A8 expression levels. Statistical significance was set at P < 0.05.
From the GSE113342 dataset, 93 DEGs were successfully identified, including 53 upregulated and 40 downregulated genes. From the GSE32591 dataset, 345 DEGs, involving 97 upregulated and 248 downregulated genes, were observed. Out of all the DEGs, 13 were common between the two datasets, as shown in the Venn diagram (Figure 1A). These 13 DEGs discovered between the LN glomerulus and the normal glomerulus based on the two microarray datasets consisted of 12 downregulated genes and one upregulated gene (S100A8). The 13 DEGs are plotted in Figure 1B, where the red and green dots represent upregulated and downregulated genes, respectively.
Figure 1 Identification of common DEGs from GSE32591 and GSE113342 datasets. (A) Venn diagram of DEGs based on the two GEO datasets. (B) Volcano plot of the 13 DEGs. Red, upregulation; green, downregulation. DEGs, differentially expressed genes; GEO, Gene Expression Omnibus.
To gain deeper insight into the biological roles of these 13 DEGs, functional and pathway enrichment analyses were performed using DAVID. The enriched GO terms and KEGG pathways are shown in Table 1 and Figure 2. KEGG pathway analysis revealed that the DEGs were mainly associated with infections and the Toll-like receptor (TLR) signaling pathway. GO biological process analysis indicated that the 13 DEGs were significantly associated with inflammatory response, innate immune response, neutrophil chemotaxis, leukocyte migration, cell adhesion, and cell–cell signaling. The top 3 significantly enriched terms regarding changes in cell component of DEGs were plasma membrane, extracellular exosome, and integral component of the plasma membrane. Changes in molecular function are primarily associated with protein binding.
Figure 2 Distribution of integrated DEGs in LN glomerulus for different enriched functions. The DEG enrichment of BP, MF, CC, and KEGG pathways (P < 0.05).
A total of 13 DEGs were imported into the PPI network complex, comprising 13 nodes and 57 edges, including 12 downregulated genes and the upregulated gene S100A8 (Figure 3). We then applied Cytotype MCODE for further analysis, and the results are shown in Figure 3B.
Figure 3 PPI network and the significant module of DEGs. (A) The PPI network of DEGs. (B) The significant module was obtained from PPI network constructed using Cytoscape with 13 nodes and 57 edges. S100A8 is marked in yellow, and downregulated genes are marked in green.
We can conclude that monocytes (GSE32591) and eosinophils (GSE113342) accounted for the majority of all infiltrating cells in the glomerulus, especially in patients with LN. The differential expression proportion of immune-infiltrating cells in the LN and normal groups is shown in Figures 4, 5. Based on analyses of the GSE32591 database, we found that memory B cells, follicular helper T cells, regulatory T cells (Tregs), and resting natural killer (NK) cells were downregulated in LN glomeruli. In addition, monocytes and activated NK cells were upregulated. Analyses of the GSE113342 database revealed that naive B cells, plasma cells, and resting mast cells were downregulated, and monocytes and activated NK cells were upregulated, similar to the observations from GSE32591. In addition, activated mast cells and eosinophils were also upregulated.
Figure 4 The differences of 22 immune cells between normal and lupus glomeruli. Monocytes and activated NK cells were upregulated in GSE32591 and GSE113342. *P < 0.05; **P < 0.01; ***P < 0.001; ****P < 0.0001.
Figure 5 Stacked bar charts of 22 immune cell proportions in the glomeruli. In GSE32591, monocytes accounted for the majority of all infiltrating cells in the glomeruli. While in GSE113342, eosinophils are the majority.
No significant age- or sex-dependent differences were found among the renal biopsies of different LN groups. There were some differences in Systemic Lupus Erythematosus Disease Activity Index (SLEDAI), Creatinine (Cr), Blood Urea Nitrogen (BUN), C3, C4, and Albumin (Alb) among various ISN/RPS class LN patients. In patients with LN, no significant difference in 24-h urinary protein measurements was found. The details are presented in Table 2.
Using immunofluorescence microscopy, the glomerular staining of S100A8 in the controls was found to be weak, and the staining in patients with classes II and V was similar to that of the controls. Glomerular staining was markedly enhanced in Class IV. We found the S100A8 proteins to be distributed throughout the glomerulus, and S100A8 did not colocalize with the podocyte marker synaptopodin (Figure 6).
We conducted a semiquantitative analysis and found a significant increase in IOD and AR in LN compared with that of the controls. However, no significant differences were found in the glomerular expression of S100A8 between the control and class II groups or control and class V groups (Tables 2, 3).
The IOD of S100A8 positively correlated with the AR of S100A8. However, the IOD and AR of S100A8 did not correlate with clinical and laboratory data. Correlations were observed among anti-ds DNA, SLEDAI, Cr, BUN, C3, C4, Alb, and 24-h urinary total protein (UTP) in various ISN/RPS class LN patients. The details are presented in Figure 7.
Figure 7 The correlation of glomerular expression of S100A8 with clinical and laboratory data. *P < 0.05; **P < 0.01;***P < 0.001.
In this study, we used bioinformatics analysis to identify 13 DEGs (CYBB, C1QA, C1QB, ITGB2, ITGAM, IL10RA, TLR1, MYD88, CCL4, CD44, CCR1, FCER1G, and S100A8) that were common between LN and normal glomeruli based on gene expression profiles obtained from the GSE113342 and GSE32591 datasets.
Proteins expressed by these genes are distributed in a variety of inflammatory cells. They are also chemokine receptors for inflammatory cells (18–28). Through enrichment analysis, we found that these genes were mainly related to the inflammatory response, innate immune response, neutrophil chemotaxis, leukocyte migration, cell adhesion, and cell–cell signaling. These factors are closely related to the pathogenesis of LN.
We also observed differential expression of immune cells, including T cells, B cells, NK cells, and macrophages, in the LN and normal groups. Studies have reported that humoral and cellular immunity is involved in the pathogenesis of LN (29). A variety of autoantibodies that form immune complexes are deposited in the glomerulus, causing kidney tissue damage (30). Various immune cells can infiltrate kidney tissues. B-cell infiltration can produce many antibodies, causing kidney tissue damage and aggravating local inflammation. Activated T cells infiltrate kidney tissue and secrete cytokines, causing kidney damage. Macrophages activate a variety of signaling pathways and promote inflammation (31). They can cause glomerular mesangial matrix proliferation, innate cells, and damage to the structure or function of the kidney tissue (32). Macrophages can also release a large number of chemical and inflammatory mediators that aggravate kidney damage (33).
In this study, we found that S100A8 was differentially regulated in the above two microarray datasets between LN and normal glomeruli. Using immunofluorescence staining, we found that S100A8 levels in the controls were weak. Glomerular staining was markedly enhanced compared to that in the controls, especially in class IV. Protein S100A8 belongs to the calcium-binding S100 protein family and has gained considerable interest as a critical modulator of inflammatory response after its cellular release (34). Basic and clinical studies have suggested a potential link between S100A8 and LN (7, 8). Consistent with our research, Frosch et al. (35) reported that the expression pattern of S100A8 markedly differed between the glomeruli and interstitium in LN. S100A8 expression was significantly increased in the interstitium, paralleling the findings in glomeruli. Intrarenal S100A8 expression is increased in refractory patients with ISN/RPS class III/IV LN (36). Davies et al. (37) found that serum and urine S100A8 levels were elevated in patients with SLE, and the urine/serum ratios were elevated in patients with active LN. Tantivitayakul et al. (38) detected S100A8 in infiltrating cells of glomeruli and peritubular capillaries.
Macrophage infiltration is associated with the severity of the inflammatory response, and macrophages express a large amount of S100A8, which participates in the pathogenesis of LN. Staining of S100A8 in patients with classes II and V was similar to that of the controls and was enhanced in classes III, IV, III+V, and IV+V. Therefore, we speculate that the pathogenesis of S100A8 varies in different pathological types. Unfortunately, we did not find a relationship between S100A8 levels and clinical or laboratory data. However, the exact mechanisms of pathogenesis remain unclear. Further research is required to confirm the role of S100A8 in LN. One study reported that S100A8 could be a promising therapeutic target for myocardial ischemia–reperfusion injury (39). This is an important question that needs to be explored in future research.
We used bioinformatics to determine the DEGs between the LN glomerulus and normal glomerulus. Immunofluorescence staining was used to identify the expression level of S100A8 in various ISN/RPS classes of LN. We found that the number of monocytes and activated NK cells were upregulated in the LN glomeruli, and the glomerular S100A8 level differed in different pathological types. Glomerular S100A8 staining was markedly increased in LN glomeruli compared to that in the controls, especially in class IV. Our results indicate that S100A8 participates in the pathogenesis of LN, and the precise mechanisms of this process need to be explored in our follow-up research.
The datasets presented in this study can be found in online repositories. The names of the repository/repositories and accession number(s) can be found in the article/Supplementary Material.
The studies involving human participants were reviewed and approved by the ethics committee of Fujian Provincial Hospital. The patients/participants provided their written informed consent to participate in this study.
WQ collected and analyzed clinical data and drafted the article. PM did immunofluorescence staining. YQ and GF helped WQ to collect and interpret data for the work. CZ and WZ designed this topic and approved the final version of manuscript. LH revised the manuscript carefully.
This work was supported by Natural Science Foundation of Fujian province (Grant No.2019J01184, 2021J05065) and joint funds for the innovation of science and technology of Fujian province (Grant No.2020Y9027).
The authors declare that the research was conducted in the absence of any commercial or financial relationships that could be construed as a potential conflict of interest.
All claims expressed in this article are solely those of the authors and do not necessarily represent those of their affiliated organizations, or those of the publisher, the editors and the reviewers. Any product that may be evaluated in this article, or claim that may be made by its manufacturer, is not guaranteed or endorsed by the publisher.
The Supplementary Material for this article can be found online at: https://www.frontiersin.org/articles/10.3389/fimmu.2022.843576/full#supplementary-material
1. Almaani S, Meara A, Rovin BH. Update on Lupus Nephritis. Clin J Am Soc Nephrol (2017) 12(5):825–35. doi: 10.2215/CJN.05780616
2. Wang Y, Yu F, Song D, Wang SX, Zhao MH. Podocyte Involvement in Lupus Nephritis Based on the 2003 ISN/RPS System: A Large Cohort Study From a Single Center. Rheumatology (2014) 53:1235–44. doi: 10.1093/rheumatology/ket491
3. Yung S, Yap DY, Chan TM. A Review of Advances in the Understanding of Lupus Nephritis Pathogenesis as a Basis for Emerging Therapies. F1000Res (2020) 9:F1000 Faculty Rev–905. doi: 10.12688/f1000research.22438.1
4. Wang S, Song R, Wang Z, Jing Z, Wang S, Ma J. S100A8/A9 in Inflammation. Front Immunol (2018) 9:1298. doi: 10.3389/fimmu.2018.01298
5. Pruenster M, Vogl T, Roth J, Sperandio M. S100A8/A9: From Basic Science to Clinical Application. Pharmacol Ther (2016) 167:120–31. doi: 10.1016/j.pharmthera.2016.07.015
6. Liya L, Xiaoxia Z, Yizhi X, Di L, Hui L, Honglin Z. Neutrophil-Derived Exosome From Systemic Sclerosis Inhibits the Proliferation and Migration of Endothelial Cells. Biochem Biophys Res Commun (2020) 526(2):334–40. doi: 10.1016/j.bbrc.2020.03.088
7. Donohue SJ, Midgley A, Davies JC, Wright RD, Bruce I, Beresford MW, et al. Differential Analysis of Serum and Urine S100 Proteins in Juvenile-Onset Systemic Lupus Erythematosus (jSLE). Clin Immunol (2020) 214:108375. doi: 10.1016/j.clim.2020.108375
8. Turnier JL, Fall N, Thornton S, Witte D, Bennett MR, Appenzeller S, et al. Urine S100 Proteins as Potential Biomarkers of Lupus Nephritis Activity. Arthritis Res Ther (2017) 19(1):242. doi: 10.1186/s13075-017-1444-4
9. Barrett T, Troup DB, Wilhite SE, Ledoux P, Rudnev D, Evangelista C, et al. NCBI GEO: Mining Tens of Millions of Expression Profiles–Database and Tools Update. Nucleic Acids Res (2007) 35(Database issue):D760–5. doi: 10.1093/nar/gkl887
10. Available at: https://www.ncbi.nlm.nih.gov/geo/query/acc.cgi?acc=GSE113342.
11. Available at: https://www.ncbi.nlm.nih.gov/geo/query/acc.cgi?acc=GSE32591.
12. Dennis G Jr, Sherman BT, Hosack DA, Yang J, Gao W, Lane HC, et al. DAVID: Database for Annotation, Visualization, and Integrated Discovery. Genome Biol (2003) 4(5):P3. doi: 10.1186/gb-2003-4-5-p3
13. Ogata H, Goto S, Sato K, Fujibuchi W, Bono H, Kanehisa M, et al. KEGG: Kyoto Encyclopedia of Genes and Genomes. Nucleic Acids Res (1999) 27(1):29–34. doi: 10.1093/nar/27.1.29
14. Available at: http://geneontology.org/page/download-ontology.
15. Available at: http://string-db.org.
16. QiJiao W, Han X, Na G, Yali R, Xiaoya L, Guohong W, et al. Overproduction of Mitochondrial Fission Proteins in Membranous Nephropathy in Children. Kidney Blood Press Res (2018) 43:1927–34. doi: 10.1159/000496006
17. QiJiao W, Xiaoya L, Guohong W, Hairong W, Sainan Z, Na G, et al. Mitochondrial Fission Proteins Are Involved in Proteinuria in Adriamycin-Induced Rat Nephropathy. J Nephrol Dialy Transplant (2017) 26(1):37–43. doi: 10.3969/cndt.j.issn.1006—298X.2017.01.007
18. Klocke J, Kopetschke K, Grießbach AS, Langhans V, Humrich JY, Biesen R, et al. Mapping Urinary Chemokines in Human Lupus Nephritis: Potentially Redundant Pathways Recruit CD4+ and CD8+ T Cells and Macrophages. Eur J Immunol (2017) 47(1):180–92. doi: 10.1002/eji.201646387
19. Yung S, Chan TM. The Role of Hyaluronan and CD44 in the Pathogenesis of Lupus Nephritis. Autoimmune Dis (2012) 2012:207190. doi: 10.1155/2012/207190
20. Yang L, Lian Z, Zhang B, Liz, Zen GL, Li W, et al. Effect of Ligustrazine Nanoparticles on Th1/Th2 Balance by TLR4/MyD88/NF-κb Pathway in Rats With Postoperative Peritoneal Adhesion. BMC Surg (2021) 21(1):211. doi: 10.1159/000496006
21. Zhou XJ, Klionsky DJ, Zhang H. Podocytes and Autophagy: A Potential Therapeutic Target in Lupus Nephritis. Autophagy (2019) 15(5):908–12. doi: 10.1080/15548627.2019.1580512
22. Lee YH, Bae SC. Association Between the Functional ITGAM Rs1143679 G/A Polymorphism and Systemic Lupus Erythematosus/Lupus Nephritis or Rheumatoid Arthritis: An Update Meta-Analysis. Rheumatol Int (2015) 35(5):815–23. doi: 10.1007/s00296-014-3156-2
23. Ghafouri-Fard S, Shahir M, Taheri M, Salimi A. A Review on the Role of Chemokines in the Pathogenesis of Systemic Lupus Erythematosus. Cytokine (2021) 146:155640. doi: 10.1016/j.cyto.2021.155640
24. Yavuz S, Bianchi M, Kozyrev S, Bolin K, Leonard D, Pucholt P, et al. Toll-Like Receptors Revisited; a Possible Role for TLR1 in Lupus Nephritis. Ann Rheum Dis (2020) 80(3):404–6. doi: 10.1136/annrheumdis-2020-218373
25. Chen Z, Lan R, Ye K, Chen H, Chen C, Xu Y. Prioritization of Diagnostic and Prognostic Biomarkers for Lupus Nephritis Based on Integrated Bioinformatics Analyses. Front Bioeng Biotechnol (2021) 9:717234. doi: 10.3389/fbioe.2021.717234
26. Liu T, Yang M, Xia Y, Jiang C, Li C, Jiang Z, et al. Microarray-Based Analysis of Renal Complement Components Reveals a Therapeutic Target for Lupus Nephritis. Arthritis Res Ther (2021) 23(1):223. doi: 10.1186/s13075-021-02605-9
27. Liao DJ, Cheng XP, Li N, Liang KL, Fan H, Zhang SY, et al. A Comparative Study on the Incidence, Aggravation, and Remission of Lupus Nephritis Based on iTRAQ Technology. Comb Chem High Throughput Screen (2020) 23(7):649–57. doi: 10.2174/1386207323666200416151836
28. Cao Y, Tang W, Tang W. Immune Cell Infiltration Characteristics and Related Core Genes in Lupus Nephritis: Results From Bioinformatic Analysis. BMC Immunol (2019) 20(1):37. doi: 10.1186/s12865-019-0316-x
29. Chang A, Clark MR, Ko K. Cellular Aspects of the Pathogenesis of Lupus Nephritis. Curr Opin Rheumatol (2021) 33(2):197–204. doi: 10.1097/BOR.0000000000000777
30. Onishi S, Adnan E, Ishizaki J, Miyazaki T, Tanaka Y, Matsumoto T, et al. Novel Autoantigens Associated With Lupus Nephritis. PloS One (2015) 10(6):e0126564. doi: 10.1371/journal.pone.0126564
31. Kuroiwa T, Lee EG. Cellular Interactions in the Pathogenesis of Lupus Nephritis: The Role of T Cells and Macrophages in the Amplification of the Inflammatory Process in the Kidney. Lupus (1998) 7(9):597–603. doi: 10.1191/096120398678920712
32. Chen PM, Wilson PC, Shyer JA, Veselits M, Steach HR, Cui C, et al. Kidney Tissue Hypoxia Dictates T Cell-Mediated Injury in Murine Lupus Nephritis. Sci Transl Med (2020) 12(538):eaay1620. doi: 10.1126/scitranslmed.aay1620
33. Kim J, Jeong JH, Jung J, Jeon H, Lee S, Lim JS, et al. Immunological Characteristics and Possible Pathogenic Role of Urinary CD11c+ Macrophages in Lupus Nephritis. Rheumatol (Oxford) (2020) 59(8):2135–45. doi: 10.1093/rheumatology/keaa053
34. Foell D, Wittkowski H, Vogl T, Roth J. S100 Proteins Expressed in Phagocytes: A Novel Group of Damage-Associated Molecular Pattern Molecules. J Leukoc Biol (2007) 81:28–37. doi: 10.1189/jlb.0306170
35. Frosch M, Vogl T, Waldherr R, Sorg C, Sunderkötter C, Roth J. Expression of MRP8 and MRP14 by Macrophages Is a Marker for Severe Forms of Glomerulonephritis. J Leukoc Biol (2004) 75(2):198–206. doi: 10.1189/jlb.0203076
36. Benjachat T, Tongyoo P, Tantivitayakul P, Somparn P, Hirankarn N, Prom-On S, et al. Biomarkers for Refractory Lupus Nephritis: A Microarray Study of Kidney Tissue. Int J Mol Sci (2015) 16(6):14276–90. doi: 10.3390/ijms160614276
37. Davies JC, Midgley A, Carlsson E, Donohue S, Bruce IN, Beresford MW, et al. Urine and Serum S100A8/A9 and S100A12 Associate With Active Lupus Nephritis and may Predict Response to Rituximab Treatment. RMD Open (2020) 6(2):e001257. doi: 10.1136/rmdopen-2020-001257
38. Tantivitayakul P, Benjachat T, Somparn P, Leelahavanichkul A, Kittikovit V, Hirankarn N, et al. Elevated Expressions of Myeloid-Related Proteins-8 and -14 are Danger Biomarkers for Lupus Nephritis. Lupus (2016) 25(1):38–45. doi: 10.1177/0961203315598015
Keywords: lupus nephritis, differentially expressed genes, microarray, immunofluorescence, S100A8
Citation: Qijiao W, Zhihan C, Makota P, Qing Y, Fei G, Zhihong W and He L (2022) Glomerular Expression of S100A8 in Lupus Nephritis: An Integrated Bioinformatics Analysis. Front. Immunol. 13:843576. doi: 10.3389/fimmu.2022.843576
Received: 26 December 2021; Accepted: 28 March 2022;
Published: 27 April 2022.
Edited by:
Nancy Agmon-Levin, Sheba Medical Center, IsraelReviewed by:
Arif Ali, Shanghai Jiao Tong University, ChinaCopyright © 2022 Qijiao, Zhihan, Makota, Qing, Fei, Zhihong and He. This is an open-access article distributed under the terms of the Creative Commons Attribution License (CC BY). The use, distribution or reproduction in other forums is permitted, provided the original author(s) and the copyright owner(s) are credited and that the original publication in this journal is cited, in accordance with accepted academic practice. No use, distribution or reproduction is permitted which does not comply with these terms.
*Correspondence: Chen Zhihan, MTM3NzAzNzAxMEBxcS5jb20=; Lin He, bGluaGUwMDg2QDE2My5jb20=; Wang Zhihong, NTYxMzcxNzBAcXEuY29t
Disclaimer: All claims expressed in this article are solely those of the authors and do not necessarily represent those of their affiliated organizations, or those of the publisher, the editors and the reviewers. Any product that may be evaluated in this article or claim that may be made by its manufacturer is not guaranteed or endorsed by the publisher.
Research integrity at Frontiers
Learn more about the work of our research integrity team to safeguard the quality of each article we publish.