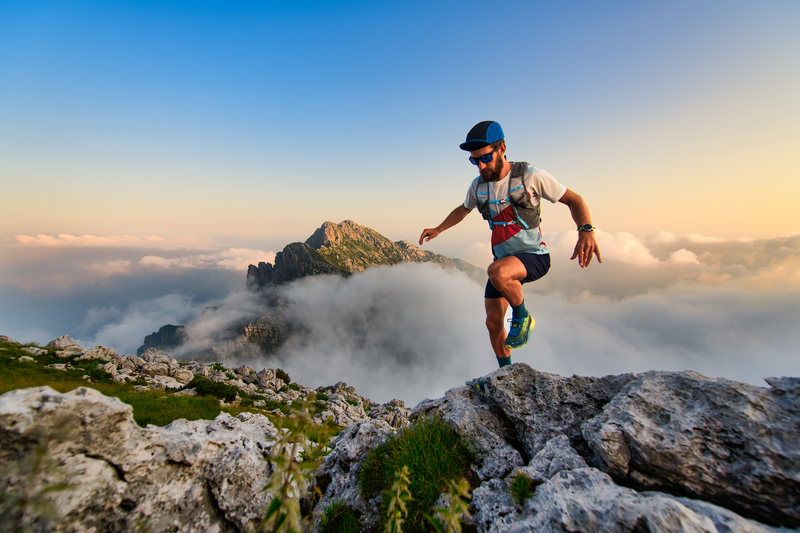
94% of researchers rate our articles as excellent or good
Learn more about the work of our research integrity team to safeguard the quality of each article we publish.
Find out more
ORIGINAL RESEARCH article
Front. Immunol. , 25 February 2022
Sec. Inflammation
Volume 13 - 2022 | https://doi.org/10.3389/fimmu.2022.840399
This article is part of the Research Topic Epigenetics of the Immune Component of Inflammation View all 43 articles
Glaesserella parasuis (G. parasuis) can elicit serious inflammatory responses and cause meningitis in piglets. Previous epigenetic studies have indicated that alterations in host DNA methylation may modify the inflammatory response to bacterial infection. However, to date, genome-wide analysis of the DNA methylome during meningitis caused by G. parasuis infection is still lacking. In this study, we employed an unbiased approach using deep sequencing to profile the DNA methylome and transcriptome from G. parasuis infected porcine brain (cerebrum) and integrated the data to identify key differential methylation regions/sites involved in the regulation of the inflammatory response. Results showed that DNA methylation patterns and gene expression profiles from porcine brain were changed after G. parasuis infection. The majority of the altered DNA methylation regions were found in the intergenic regions and introns and not associated with CpG islands, with only a low percentage occurring at promoter or exon regions. Integrated analysis of the DNA methylome and transcriptome identified a number of inversely and positively correlated genes between DNA methylation and gene expression, following the criteria of |log2FC| > 0.5, |diffMethy| > 0.1, and P < 0.05. Differential expression and methylation of two significant genes, semaphoring 4D (SEMA4D) and von Willebrand factor A domain containing 1 (VWA1), were validated by qRT-PCR and bisulfite sequencing. Gene Ontology (GO) and Kyoto Encyclopedia of Genes and Genomes (KEGG) enrichment analyses demonstrated that DNA methylation inversely correlated genes in G. parasuis infected porcine brains were mainly involved with cell adhesion molecules (CAMs), bacterial invasion of epithelial cells, RIG-1-like receptor signaling pathways, and hematopoietic cell lineage signaling pathways. In addition, a protein-protein interaction network of differentially methylated genes found potential candidate molecular interactions relevant to the pathology of G. parasuis infection. To the best of our knowledge, this is the first attempt to integrate the DNA methylome and transcriptome data from G. parasuis infected porcine brains. Our findings will help understanding the contribution of genome-wide DNA methylation to the pathogenesis of meningitis in pigs and developing epigenetic biomarkers and therapeutic targets for the treatment of G. parasuis induced meningitis.
Glaesserella parasuis (G. parasuis), is a commensal pathogen found in the porcine upper respiratory tract, and can invade the bloodstream and cause polyserositis, arthritis, meningitis, and frequently, pneumonia like symptoms, and is the etiological agent of Glässer’s disease (1). To date, 15 serovars have been identified on the basis of immunodiffusion, using heat-stable antigen extracts, and numerous serovars do not induce protective cross-immunity (2). Epidemiological studies have shown that serovar 5 is the most prevalent form associated with this disease in China, followed by serovars 4, 7, 13, and 14 (3). Because of the high morbidity and mortality caused by G. parasuis infection, it has become a major threat to the swine industry throughout the world, and its control and prevention are urgently needed (4).
Meningitis, a common symptom of G. parasuis infection, is defined as inflammation of the meninges, and an abnormal number of white blood cells in the cerebrospinal fluid with specific clinical symptoms (5). Aseptic and bacterial meningitis vary significantly (6, 7), with most cases of aseptic meningitis being associated with viral infection and generally self-limited with a good prognosis. However, bacterial meningitis requires prompt diagnosis and medical treatment but even then mortality still occurs regardless of its acute or chronic onset (8, 9). Streptococcus pneumoniae, Haemophilus influenzae and Neisseria are the main bacterial pathogens responsible for acute bacterial meningitis (9, 10) and it is reported that the host genetic factors are the major determinants of susceptibility for bacterial meningitis (7). Recently, zoonotic meningitis has been occasionally linked to pig farmers who have developed acute bacterial meningitis after handling raw swine meat (11). At present, there remains an incomplete understanding of the pathogenesis and the best methods for the prevention and treatment of bacterial meningitis and further research looking at the pathogenesis of bacterial meningitis is urgently needed. Thus, research on the pathology of bacterial meningitis caused by G. parasuis using an infected porcine model appears to be meaningful for both the porcine industry and human public health.
DNA methylation is an epigenetic modification of DNA involving the addition of a methyl group onto the 5-carbon of cytosine to form 5-mehylcytosine (5mC), and represents a major regulator of gene expression, depending on the genomic context such as islands, transcriptional start sites, gene bodies, regulatory elements and repeat sequences (12, 13). Genome-wide DNA methylation patterns have been shown to be associated with gene transcription, cancer, mammalian embryo development, and a variety of diseases, including autoimmune and inflammatory disorders (14, 15). In a recent study of multiple sclerosis, and autoimmune disease, DNA methylation was reported to be involved in the process blood-brain barrier breakdown, inflammatory response, failure of myelination and neurodegeneration (16). This epigenetic modification has also been implicated in the pathogenesis of chronic inflammatory responses by regulating differentiation, proliferation, apoptosis, and activation of immune cells (17). Furthermore, the host DNA methylation profile can be altered upon exposure of cells to environmental pathogens, and these changes induced by the action of viral and bacterial infection may suppress the production of anti-inflammatory factors and activate the pro-inflammatory cells, as well as modify the immune response to bacterial infection (17, 18). Although lots of research has focused on the pathogenic mechanism of G. parasuis, little is known about alterations in host DNA methylation or the corresponding effects on the regulation of the transcriptome in meningitis under pathogenic conditions.
In the present study, we aimed to identify key differentially methylated regions/sites that may potentially regulate the function of gene expression during the process of G. parasuis infection, and these data may provide useful information for the development of epigenetic biomarkers and/or therapeutic targets for the treatment of G. parasuis induced meningitis. Thus, we examined the DNA methylation profile at a single base-pair resolution using whole-genome bisulfite sequencing and integrated the methylome information with transcriptome data from RNA-seq in normal and G. parasuis infected porcine brains.
A highly virulent strain of serovar 5 (G. parasuis SH0165 strain), was isolated from the lungs of a commercial pig showing signs of arthritis, fibrinous polyserositis, hemorrhagic pneumonia, and meningitis (19). The SH0165 strain was cultured in tryptic soy broth (Difco Laboratories, Franklin Lakes, NJ, USA) or tryptic soy agar (Difco Laboratories) at 37°C, supplemented with 10 µg/mL of nicotinamide adenine dinucleotide (NAD) (Sigma-Aldrich Corporation, St. Louis, MO, USA) and 10% fetal bovine serum (Gibco, Carlsbad, CA, USA).
This study was conducted in strict accordance with the recommendations of the Chinese Regulations for the Administration of Affairs Concerning Experimental Animals 1988 and the Hubei Regulations for the Administration of Affairs Concerning Experimental Animals 2005. The protocol was ratified by the Hubei Province Science and Technology Department of China (permit number SYXK[ER] 2010-0029). Animal studies were approved by the Animal Care and Use Committee of Wuhan Polytechnical University, Hubei Province, China (EM947, 5th November 2020). All experimental animals were euthanized at the end of the experiment.
Six 28-day-old naturally farrowed early weaned piglets (Duroc × Landrace × Large white), weighing 8-10 kg, were obtained from Wuhan Wannianqing Animal Husbandry Co., Ltd (Wuhan, China) for in vivo experimentation. All experimental piglets were negative for antibodies against G. parasuis when tested using INgezim Haemophilus 11.HPS.K.1 (INgezim, Spain).
The six piglets were randomly divided into two groups including the negative control group and infected group. The infected group was challenged intraperitoneally with 2 × 109 CFU of the G. parasuis SH0165 strain in 1 mL of normal saline. The negative control group was injected intraperitoneally with an equivalent volume of saline alone. All the piglets were monitored for 7 days after challenge, and the cerebrum tissues from the infected and control groups were collected for whole-genome bisulfite sequencing and RNA-seq.
Genomic DNA from porcine brain tissue was extracted with Allprep DNA/RNA/Protein Mini Kit (Qiagen, Hilden, Germany) according to the manufacturer’s protocol. Then extracted DNA (1 µg) was spiked with 1 ng of fragmented unmethylated phage λ DNA (Promega, Madison, WI, USA, average size: 300 bp), which was end-repaired, A tailed, and adapter-ligated using Truseq DNA sample prep kit (Illumina Inc., USA) according to the manufacturer’s protocol. Adapter-ligated DNA was gel isolated and recovered using a QIAquick gel extraction kit (Qiagen, Hilden, Germany). After a cleanup with AMPure XP beads (Beckman Coulter Inc., Fullerton, CA, USA), bisulfite conversion was performed using EZ-DNA Methylation-Gold Kit (Zymo Research, Orange, CA, USA) according to the manufacturer’s protocol. Then, the bisulfite converted DNA was amplified with PfuTurbo Cx Hotstart DNA Polymerase (Agilent Technologies, Inc., Santa Clara, CA, USA) using the following thermal cycles: 95°C 5 min, 98°C 30 s, 12 cycles of (98°C 10 s, 65°C 30 s, 72°C 30 s), 72°C 5 min. Libraries were cleaned with AMpure XP beads and fragment length determined using an Agilent 2100 (Agilent Technologies, Inc., Santa Clara, CA, USA). Quantified libraries (concentration > 2 nM) were then sequenced using the Illumina HiSeq2500 platform.
Total RNA was extracted using the miRNeasy Mini Kit (Qiagen, Hilden, Germany) in accordance with the manufacturer’s instructions and RIN number assess for RNA integrity using an Agilent Bioanalyzer 2100 (Agilent Technologies, Inc., Santa Clara, CA, USA). Quantified total RNA was further purified using an RNAClean XP Kit (Beckman Coulter Inc., Fullerton, CA, USA) and RNase-Free DNase (Qiagen, Hilden, Germany). Only RNA with a RIN of ≥ 7.0 and an 28S/18S ratio of ≥ 0.7 were selected for deep sequencing. Libraries were generated using the VAHTS Total RNA-seq Library PrepKit for Illumina (Vazyme Biotech Co., Ltd., Nanjing, China) and were subsequently sequenced using the Illumina HiSeq X-Ten platform.
General quality control was performed using FastQC v 0.11.5 (http://www.bioinformatics.babraham.ac.uk/projects/fastqc/). Low quality reads (error ratio > 0.01 or read length < 70 bp or unpaired-reads) and adaptors were removed using Trimgalore v0.4.1 (http://www.bioinformatics.babraham.ac.uk/projects/trim_galore/) and Cutadapt v1.9.1 (20). Filtered datasets were aligned to the reference genome, Sus-Scrofa.Sscrofa 10.2, using Bismark v 0.15.0 (21), and using Bowtie2 v 2.2.6 (22) as the underlying alignment tool. Mapping for all datasets generated from the same library were merged, and duplicates removed via the Bismark deduplication tool. Mapped reads were then separated by genome (Sscrofa 10.2 or phage λ) and by source strand (plus or minus). The first four and last one base of each read2 in all read pairs was clipped due to positional methylation bias, and any redundant mapped bases due to overlapping mates from the same read pair were trimmed to avoid bias in the quantification of methylation status. Read pairs mapped to phage λ were used as a QC assessment to confirm that the observed bisulfite conversion rate was >99%. Read pairs mapped to the Sscrofa 10.2 reference genome were used for downstream analysis.
The R package DSS (Dispersion shinkage for sequencing data, DSS) was used to identify DML (Differential methylation loci, DML) with the callDML function, and DMR (Differential methylation region, DMR) with the callDMR function. DMRs that contain at least three validated CpG sites and a minimum length of 50 bp were retained for downstream analysis. DMLs and DMRs with a threshold p value < 0.05 and mean methylation difference > 0.1 were considered to be significantly differentially methylated.
The genomic context of the identified DMLs and DMRs with respect to known genes and gene features was analyzed by using the R package goldmine (http://jeffbhasin.github.io/goldmine). The function getCpgFeatures, getFeatures, and getGenes were used to obtain CpG islands, ENCODE supertracks, and RefSeq gene models from the database of the UCSC Genome Browser (23, 24).
The raw reads were filtered by using the Seqtk sequence processing tool (https://github.com/lh3/seqtk) and mapped to the reference genome (Sus-scrofa.Sscrofa10.2.dna.toplevel.fa) by using the Hisat2 alignment program (25) and StringTie was used to count the gene fragments (26, 27). Package edgeR (28) was used to identify differentially expressed genes with a threshold fold change > 2 and adjusted p-value < 0.05, which was considered as significantly differentially expressed.
To reveal the correlation between DNA methylation status and gene expression, we integrated DNA methylation and gene expression data. First, genes that were significantly differentially methylated in the promoter regions were selected with a threshold of mean methylation difference > 0.1 and p value < 0.05. Second, genes that were significantly differentially expressed were selected with a threshold of |log2FC| >0.5 and p value < 0.05. For these two selected gene sets, they were firstly merged by the merge function in R. Then, the correlation for the methylation difference and expression difference were determined by “cor. test” in R using Pearson method. Significantly negative correlations were considered if the correlation coefficient was negative and the p value was < 0.05, and significantly positive correlations were considered if the correlation coefficient was positive, and the p value was < 0.05. Differentially expressed genes that were negatively correlated with methylation were subjected to further studies.
To discover the potential biological function underlying the identified genes, we conducted GO and KEGG enrichment analysis on the differentially expressed or differentially methylated genes. Genes were mapped to each term within the GO database. GO terms with adjusted p values < 0.05 were considered as significantly enriched. Pathway annotation of the entire genome was conducted by the KEGG automatic annotation server. Pathways with p values ≤ 0.05 were deemed to be significantly enriched.
STRING database (ELIXIR, Hinxton, Cambridge shire, UK) were utilized for the protein-protein interaction (PPI) network construction, based on the methylation and expression of inversely correlated genes. Network Analyzer (http://www.mpi-inf.mpg.de/) was used to determine the topological properties of the biological networks. Cytoscape software (Institute for Systems Biology, Seattle, WA, USA) was used for network visualization.
Bisulfite sequencing was performed to validate the DMRs detected by the whole-genome DNA methylation analysis. DNA (330 ng) was isolated using Quick-DNA Miniprep Kit (Zymo Research, Orange, CA, USA) and DNA bisulfite conversion was conducted by using the EZ DNA Methylation Gold Kit (Zymo Research, Orange, CA, USA). Bisulfite-converted DNA (1 µg) was amplified by PCR with EpiTaq polymerase (for bisulfite-treated DNA) (TaKaRa Biotechnology Co., Ltd., Dalian, China), and primers (Supplementary File 1) were manually designed using Primer Premier 5. Before primer design, DNA sequences need to be methylation converted in the MethPrimer website (http://www.urogene.org/methprimer/). PCR products were cloned using the Topo TA cloning kit (Invitrogen Corporation, Carlsbad, CA, USA) and after verification of successful cloning, at least ten different clones for each sample were sent for sequencing at TSINGKE Biological Technology. The DNA methylation profile of individual CpG sites were analyzed and presented using BiQ Analyzer (https://biq-analyzer.bioinf.mpi-inf.mpg.de/).
Total RNA was extracted from porcine brain tissues using RNeasy Mini Kit (Qiagen, Hilden, Germany), and reverse transcribed into cDNA using reverse transcriptase (TaKaRa Biotechnology Co., Ltd., Dalian, China). cDNA was further quantified using the SYBR Green PCR Kit (TaKaRa Biotechnology Co., Ltd., Dalian, China) according to the manufacturer’s protocol. Individual samples underwent three technical repeats, with β-actin as the reference gene. The Primer Premier 5.0 software was utilized to design the PCR primers (Supplementary File 2).
Experimental data were analyzed using the two-tailed Student’s t-test and presented as the mean ± standard deviation and differences with P < 0.05 were considered to be significant (*P < 0.05; **P < 0.01).
Whole-genome bisulfite sequencing was performed with the Illumina HiSeq 2500 to explore the global DNA methylation changes associated with the pathology of meningitis induced by G. parasuis. A total of 626.50 ± 47.40 million and 627.76 ± 36.65 million raw reads were obtained from the infected and negative control groups, respectively. After filtration and quality control, the clean reads ratio ranged from 96.1% to 99.0% (Supplementary File 3). All the multi-mapped reads duplicated reads and adaptors were removed and aligned to the Susscrofa genome, with the unique mapping ratio ranging from 54.3% to 60.7% (Supplementary File 3). Unmethylated phage λ DNA was added to evaluate the bisulfite conversion ratio, which ranged from 99.1% to 99.4% (Supplementary File 3).
Cytosine methylation levels at a single base-pair resolution were explored using callDML function in the R package DSS. Results showed that 316,621 CpG sites were differentially methylated in G. parasuis infected porcine brain, with 132,557 CpG sites hypermethylated and 184,064 CpG sites hypomethylated (P < 0.05) (Supplementary File 4). We also identified 12,145 (DMRs) that were statistically significant between the two groups, with 4,649 DMRs hypermethylated and 7,496 DMRs hypomethylated in G. parasuis infected porcine brain (P < 0.05) (Supplementary File 5).
The genomic and CpG island context of the DMRs were investigated in G. parasuis infected porcine brain and results showed that the differentially methylated regions were distributed throughout the whole genome (Figure 1). DMRs were most common in intergenic regions (64.7%), and less common in introns (26.1%). Only a minor percentage of DMRs occurred in promoters (3.9%), followed by exons (2.8%), transcriptional termination sites (TTS, 1.6%), 3’ untranslated regions (3’ UTR, 0.6%) and 5’ untranslated region (5’ UTR, 0.4%) (Figure 1A). The genomic annotation profile exhibited similarities when we focused on hyper- and hypo- methylated DMRs separately. The majority of DMRs were annotated in intergenic regions (64.4% and 64.9% for hypermethylated and hypomethylated DMRs, respectively), and a minor percentage of DMRs were annotated in promoters (4.7% and 3.3% respectively) (Figure 1C). Furthermore, the CGI (CpG island) annotation showed that most DMRs were found outside CGIs and most of these were in the open sea regions (greater than 4 kb from CpG islands) (Figure 1B). For hypermethylated and hypomethylated DMRs, 14.7% and 10.0% were annotated in CGIs, 12% and 11.2% were in shores (2000 bp flanking CpG islands), and 5.4% and 5.7% were in shelves (2000 bp flanking shore regions) (Figure 1D).
Figure 1 Genome wide distribution of differentially methylated regions (DMRs). (A) The proportion of DMRs that overlap with 5’ UTR, exons, TTS, 3’ UTR, promoter-TSS, intron, and intergenic regions. (B) The proportion of DMRs with respect to CpG islands versus CpG shores (2000 bp flanking CpG islands), shelves (2000 bp flanking shore), and open sea regions (greater than 4 kb from CpG islands). (C) The distribution of hyper and hypomethylated regions with respect to their genetic location to genes. (D) The distribution of hyper and hypomethylated regions with respect to their distance to CGIs.
RNA-seq analysis was performed to explore changes in the global transcriptome occurring in meningitis induced by G. parasuis infection. Unsupervised hierarchical clustering identified two unique clusters that had distinct signatures (Figure 2A). A total of 1,126 genes were significantly differentially expressed in G. parasuis infected porcine brain, including 606 up-regulated and 520 down-regulated genes (fold change > 2, adjusted p < 0.05) (Figure 2B and Supplementary File 6).
Figure 2 Transcriptome analysis comparing G. parasuis-infected porcine brain vs. control porcine brain. (A) Hierarchical clustering heatmap of transcript abundance from RNA-seq. Each row represents a transcript, H.1, H.2, H.3 represent G. parasuis-infected porcine brain, and C.1, C.2, C.3 represent negative controls. (B) Volcano plot showing differentially expressed genes. The vertical axis shows the -log10 (q-value), and the horizontal axis shows log2 (Fold-change). (C) GO functional category analysis of differentially expressed genes. The vertical axis shows the number of genes in each term, and the horizontal axis shows biological process, cellular component, and molecular function terms. (D) KEGG pathway enrichment of differentially expressed genes. Top 30 KEGG pathways are shown in the vertical axis and the horizontal axis shows the rich factor.
GO and KEGG enrichment were performed to reveal the potential function of the affected genes involved in the pathogenesis of meningitis induced by G. parasuis. GO enrichment showed that cellular processes, single-organism processes, and metabolic processes were the most abundant terms in the biological process category. In the cellular component category, cell, cell part and membrane were the top three terms, while binding, catalytic activity, and molecular transducer activity were the top terms in the molecular function category (Figure 2C). KEGG analysis revealed that cytokine-cytokine receptor interaction, cell adhesion molecules (CAMs), and phagosome were the most significantly abundant pathways involved in the pathogenesis of G. parasuis induced meningitis (Figure 2D).
DNA methylation and gene transcription are normally causal related, where hypermethylation might be associated with gene down-regulation and hypomethylation might be related to gene up-regulation, especially when methylated sites are located in the promoter region of genes. The hierarchical clustering of the top-10000 significantly differentially methylation sites, considering different genomic context (5’UTR, 3’UTR, exon, intergenic, intron, promoter-TSS, and TSS), showed a striking difference between the control and G. parasuis infected samples (Figure 3A and Supplementary Figure 1). A volcano plot showed the distribution of different methylation sites under different methylation thresholds (Supplementary Figure 2). To further identify the significance of DNA methylation in the regulation of gene expression, we integrated the CpGs methylation patterns with the gene expression profiles obtained from the same sample. The differentially methylated CpG sites in the promoter region and the differentially expressed mRNA transcripts were aligned to chromosomes throughout the whole genome (Figure 4). The integrated analysis showed that 301 differentially expressed genes corresponding to 1068 differentially methylated CpGs in their promoter regions exhibited an inverse correlation (|log2FC| > 0.5, |diffMethy| > 0.1, and P < 0.05) (Figure 3B and Supplementary File 7). Furthermore, 141 genes (28%) were significantly hypermethylated and down-regulated, while 160 genes (29%) were significantly hypomethylated and up-regulated (|log2FC| > 0.5, |diffMethy| > 0.1, and P < 0.05) (Figure 3B). The inversely correlated methylation sites were detected throughout the genome on all chromosomes except chromosome 19, 20 and chromosome Y (Figure 3C).
Figure 3 Integrated analysis of DNA methylation and mRNA expression when comparing G. parasuis-infected porcine brain vs. control porcine brain. (A) Hierarchical clustering heatmap of the top-1000 differentially methylated CpG sites from whole-genome bisulfite sequencing. Each row represents a methylated CpG site, H1, H2, H3 represent G. parasuis-infected porcine brain, and C1, C2, C3 represent negative controls. (B) Scatter plot integrating CpGs methylation and mRNA expression. The vertical axis shows differences in gene expression and the horizontal axis shows differences in DNA methylation for the CpG sites. 1, 3-quadrants represent positive correlated genes, in which gene up-regulation correlates with hypermethylation and gene down-regulation correlates with hypomethylation. The 2, 4-quadrants represent inversely correlated genes, in which gene up-regulation correlates with hypomethylation and gene down-regulation correlates with hypermethylation. (C) Karyogram showing genome-wide coverage of differentially methylated sites that inversely correlate to gene expression (2, 4-quadrants in B). Hypermethylated sites correlated with gene down-regulation are shown in red (HyperLoss). Hypomethylated sites correlated with gene up-regulation are shown in blue (HypoGain). (D) Scatter plot integrating DMRs and mRNA expression. The vertical axis shows differences in gene expression and the horizontal axis shows differences in DNA methylation of DMRs. The 1, 3-quadrants represent positively correlated genes and the 2, 4-quadrants represent inversely correlated genes.
Figure 4 Circos plot showing DNA methylation and mRNA transcription on pig chromosomes. The outermost circle displays the pig chromosomes; The second layer from the outside represents the genome-wide distribution of differentially methylated CpGs in promoter regions; The innermost layer represents the differentially expressed mRNAs identified by RNA-seq. Red bars indicate hypermethylation or up-expression, and blue/green bars indicate hypomethylation or down-expression.
The same integrated analysis was performed on the DMRs and gene expression profiles. Forty DEGs corresponding to 40 DMRs in the promoter regions were inversely associated, and 33 DEGs were positively correlated with their promoter DMRs (|log2FC| > 0.5, |diffMethy| > 0.1, and P < 0.05) (Figure 3D and Supplementary Files 8, 9). Table 1 shows the top 30 significant DEGs with an inverse correlation between DMR and gene expression.
Table 1 Top 30 genes with an inverse correlation between DMR and gene expression in G. parasuis-infected porcine brain.
In addition to the inversely correlated genes, we also observed an equivalent number of positively correlated genes, which were hypermethylated but up-regulated or hypomethylated but down-regulated (Figures 3B, D). Thus, both negative and positive correlations between DNA methylation and mRNA expression were observed in our G. parasuis infected porcine brain samples, suggesting that there may be two different mechanisms involving DNA methylation-dependent gene regulation for G. parasuis induced meningitis.
GO and KEGG enrichment were performed to reveal the biological dysfunctions caused by the DNA methylation and the expressed inversely correlated genes in G. parasuis infected samples. GO enrichment showed that cellular processes, single-organism processes, and metabolic processes were the most abundant terms in the biological process category. In the cellular component category, cell, cell part, and organelle were the top three terms, while binding, and catalytic activity were the top terms in the molecular function category (Figure 5A). When compared to the KEGG pathways enriched by all the differential genes (Figure 2D), cell adhesion CAMs, and hematopoietic cell lineages were common between the two enrichments, and bacterial invasion of epithelial cells, and RIG-1-like receptor signaling pathways were particularly enriched by the inversely correlated genes in G. parasuis infected porcine brain (Figure 5B).
Figure 5 GO functional category analysis and KEGG pathway enrichment of the inversely correlated genes in G. parasuis-infected porcine brain. (A) GO functional category analysis of inversely correlated genes. The vertical axis shows the number of genes in each term, and the horizontal axis shows biological processes, cellular component, and molecular function terms. (B) KEGG pathway enrichment of inversely correlated genes. Top 30 KEGG pathways are shown in the vertical axis and the horizontal axis shows the rich factor.
To determine the reliability of the sequencing data, mRNA expression and DNA methylation at base-pair resolutions were examined on two inversely correlated genes, semaphoring 4D (SEMA4D) and von Willebrand factor A domain containing 1 (VWA1), according to the significance criteria. Bisulfite sequencing showed that the methylation rate in the DMR of SEMA4D decreased from 65.4% to 39.1% after G. parasuis stimulation, while the methylation rate in the DMR of VWA1 decreased from 45.8% to 21.5% after G. parasuis stimulation (Figures 6A, B). Validation with RT-PCR showed that the mRNA expression of SEMA4D and VWA1 were significantly up-regulated after G. parasuis stimulation (P < 0.01), consistent with the hypomethylation in the promoter regions of each gene (Figure 6C).
Figure 6 Bisulfite sequencing and qRT-PCR validation of SEMA4D and VWA1 in G. parasuis-infected vs. control porcine brain. (A) Bisulfite sequencing validation of SEMA4D. On the left, bisulfite sequencing depth shows the SEMA4D DMR and adjacent flaking regions in three G. parasuis-infected and three negative control samples. On the right, targeted bisulfite sequencing validation of a region overlapping the SEMA4D DMR as indicated by the rectangle over the sequencing tracks. Each row represents a single clone. Dark circles represent methylated and open circles represent unmethylated cytosines. (B) Bisulfite sequencing validation of VWA1. Similarly, bisulfite sequencing depth for VWA1 DMR and adjacent flaking regions is shown on the left, and bisulfite sequencing validation is shown on the right. (C) qRT-PCR validation of SEMA4D and VWA1. The vertical axis indicates the relative expression levels of SEMA4D or VWA1 in reference to β-actin, and the horizontal axis indicates the G. parasuis-infected vs. negative control samples. **P < 0.01.
To reveal the potential associations within the differentially methylated genes, we created a protein-protein interaction network which consisted of 193 protein nodes and 243 interactions based on the differential genes (|log2FC| > 0.5, |diffMethy| > 0.1, and P < 0.05) (Figure 7A), with CCL5 and CXCL10 as the core genes. The crucial roles of genes were determined by the node degree and the top 40 genes with the highest connection degrees were selected and subsequently used for KEGG pathway enrichment (Figure 7B). The KEGG result showed that the top 40 genes mainly participated in chemokine signaling pathways, cytosolic DNA-sensing pathways, RIG-1-like receptor signaling pathway, Toll-like receptor signaling pathway, CAMs signaling pathways, and TNF signaling pathway (Figure 7C).
Figure 7 PPI network. (A) PPI network constructed from significantly differentially expressed and methylated genes in G. parasuis-infected porcine brain. Nodes represent genes and edges represent interactions. The node size represents the node degree. (B) The top 40 hub genes with the highest node degrees corresponding to the network. The vertical axis shows gene name, and the horizontal axis shows the node degree. (C) KEGG pathway enrichment of the top 40 hub genes. Top 30 KEGG pathways are shown in the vertical axis and the horizontal axis shows the rich factor.
Genome-wide DNA methylation has been shown to be associated with a variety of diseases, and the host DNA methylation profile can be altered upon exposure of cells to environmental pathogens (14, 18). Intensive studies have been performed looking at the pathogenic mechanism of G. parasuis infection, but the genome-wide DNA methylation pattern as well as the relationship between DNA methylation and gene expression during the process of infection is still unknown. To our knowledge, this study represents the first attempt to profile a genome-wide DNA methylation pattern and integrate both DNA methylome and transcriptome data from porcine brain to identify key genes and pathways that are possibly regulated by DNA methylation during the process of G. parasuis infection. This work may provide information for the development of epigenetic biomarkers and potential therapeutic target for G. parasuis induced meningitis.
Genomic location of DNA methylation has a major effect to the type of function produced by this epigenetic modification (12, 14). Thus, we investigated the genomic and CpG island contexts of the DMRs in G. parasuis infected porcine brain. Our results found that DMRs are most frequent in intergenic regions and introns, with only a comparatively low percentage of DMRs occurring in promoters and CpG islands, which is consistent with other report of the distribution patterns of DNA methylation in other diseases (29, 30). Intergenic regions contain cis factors that participate in the regulation of gene transcription during pathogen infection and inflammatory responses (12, 14). Published data indicate that DNA methylation within introns may participate in alternative exon splicing (31). Whereas DNA methylation outside of CGIs in shores/shelves may regulate expression by creating alternative transcriptional start sites (32). Research on human dendritic cells reported that bacterial infection-induced demethylation rarely occurs at promoter regions and instead localizes to distal enhancer elements, some of which regulate the activation of immune transcription factors (33). Therefore, DMRs in distal regulatory elements may also have meaningful functions in gene regulation. The large amount and genome-wide distribution of differential DNA methylation observed in our data indicate that this epigenetic modification may play an important role during the process of G. parasuis infection.
Whether de novo DNA methylation is the reason for gene silencing or a result of gene regulation is still debated but increasing evidence has indicated that there are causal associations between DNA methylation changes and phenotypes (34, 35). It has been reported that methylated CpGs can prevent the binding of some transcription factors (12), and DNA methylation can induce a more compact and rigid nucleosome structure and regulate alternative polyadenylation of mRNA during transcription (36, 37). Therefore, it is well accepted that DNA methylation and gene transcription is inversely correlated, with hypermethylation associated with gene down regulation and that hypomethylation is associated with gene up regulation. However, in our integrated analysis, the amount of inversely correlated genes and positively correlated genes were similar, suggesting that the relationship between DNA methylation and gene transcription may be more complicated than our previous expectation. The regulatory mechanism involved in DNA methylation and gene expression may vary depending on the genomic location of the methylated sequence or site (12, 14).
To explore the potential functions of these differentially expressed genes and provide new perspectives on the function of DNA methylation, we performed KEGG pathway enrichment analysis on all DEGs and DNA methylation-mRNA expression inversely correlated genes. After comparing the results, we found CAMs, and hematopoietic cell lineages were common between the two enrichments, with cytokine-cytokine receptor interaction specifically appearing in the pathways enriched by all DEGs and bacterial invasion of epithelial cells, and RIG-1-like receptor signaling pathways specifically identified in the results from inversely correlated genes. During the pathology of bacterial meningitis, which is an inflammatory disease of the CNS, bacterial epithelial cell adhesion and invasion is an important step followed by invasion and break down of the blood-brain barrier (BBB) (38, 39). The first step in the interaction of blood-born bacteria with the brain endothelium is adherence/attachment to brain microvascular endothelial cells, in which CAMs and receptors bind to multiple ligands from the bacterial surface and act as a bridges (40). Thus, we infer those changes in DNA methylation that are inversely associated with the regulation of gene expression may participate in the process of bacterial invasion of epithelial cells during G. parasuis infection and finally result in meningitis. Further experiments however are needed in porcine brain microvascular endothelial cells.
We also constructed a protein-protein interaction network among differentially methylated and expressed genes (|log2FC| > 0.5, |diffMethy| > 0.1, and P < 0.05), in which CCL5 and CXCL10 were identified as the core genes of the network. CCL5 (chemokine ligand 5) and CXCL10 (CXC motif chemokine ligand 10) are chemokines responsible for facilitating inflammatory responses, including the adhesion and migration of different T cell subsets in immune responses, and are involved in various pathological processes including inflammation, chronic diseases, and cancers as well as the infection of COVID-19 (41, 42). Interestingly, as key genes in the cytokine-cytokine receptor interaction signaling pathway, CCL5 and CXCL10 were also identified as core genes in our previous study in porcine aortic vascular endothelial cells infected with G. parasuis (19), and this prompted us to infer that these two chemokines may play a very important role in the immune response during G. parasuis infection and further study is necessary to confirm this.
For the inversely correlated genes, we validated SEMA4D and VWA1, for which hypomethylation in the promoter region of both genes was associated with gene up regulation in G. parasuis infected porcine brain. SEMA4D is a classic member of the semaphorin family that is widely represented in the immune system and plays an important role in many physiological and pathological processes, including immunoregulation, angiogenesis, neurogenesis, and tumor progression (43, 44). It is reported that SEMA4D contributes to the formation of cellular, humoral, and innate immune responses, such as inflammation, immune cell migration, and immunological synapse formation (45). It has been demonstrated that SEMA4D plays an important role in angiogenesis by promoting chemotaxis of endothelial cells (46). Although the effect of SEMA4D in the pathogenesis of meningitis has not been reported, since the expression of SEMA4D is up regulated with hypomethylation in the promoter region after G. parasuis infection, we speculate that SEMA4D may play an important role in immunoregulation and inflammation during this process. However, further study is needed to validate this hypothesis. VWA1 encodes the von Willebrand factor A domain containing 1 protein, which is an extracellular matrix protein expressed in muscle and peripheral nerves but whose function is poorly understood (47, 48). Lack of VWA1 is known to compromise peripheral nerve structure and function in a VWA1 knock-out mouse model (49). Exome sequencing identified bi-allelic loss of function variants in VWA1 as the molecular basis of neuromyopathy, a neuromuscular disorder (50). Hypermethylation of a non-coding region 1.1 kb upstream from the VWA1 gene has been reported to be associated with clear-cell ovarian cancer (51). These results demonstrating that hypomethylation in the promoter region of VWA1 and its gene up-regulation in G. parasuis infected porcine brain provides a new understanding of the potential function of VWA1.
Our study is the first attempt to integrate DNA methylome and transcriptome data from G. parasuis infected porcine brains and has found that G. parasuis can induce changes to whole-genome DNA methylation and gene expression profiles in porcine brain. The network and pathways identified by the DNA methylation-gene expression correlated genes suggest that key sites of differential DNA methylation could lead to molecular aberrations underlying meningitis and provide potential targets for further pathological study. Our data also provides a basis for defining the contribution of genome-wide DNA methylation to the pathogenesis of meningitis in pigs and provide information for the development of epigenetic biomarkers and potential therapeutic target for G. parasuis induced meningitis.
The data used in this study was deposited in the NCBI Sequence Read Archive (SRA) repository, accession number PRJNA800576.
The animal study was reviewed and approved by Animal Care and Use Committee of Wuhan Polytechnical University, Hubei Province, China (EM947, 5th November 2020); Hubei Province Science and Technology Department of China (permit number SYXK[ER] 2010-0029).
YQ and LG conceived and designed the experiments. LG, HXC, SF, JL, and YZ performed the experiments. LG, HXC, SF, and HBC analyzed the data. LG, SF, and YQ wrote the paper. All authors contributed to the article and approved the submitted version.
This work was supported by the National Natural Science Foundation of China (grant no. 32072917), science and technology research program of Hubei Provincial Department of Education (No. D20211606), Key Laboratory of Animal Embryo Engineering and Molecular Breeding of Hubei Province (KLAEMB-2019-03), and key laboratory of prevention and control agents for animal bacteriosis (Ministry of Agriculture and Rural Affairs) (KLPCAAB-2020-01).
The authors declare that the research was conducted in the absence of any commercial or financial relationships that could be construed as a potential conflict of interest.
All claims expressed in this article are solely those of the authors and do not necessarily represent those of their affiliated organizations, or those of the publisher, the editors and the reviewers. Any product that may be evaluated in this article, or claim that may be made by its manufacturer, is not guaranteed or endorsed by the publisher.
The Supplementary Material for this article can be found online at: https://www.frontiersin.org/articles/10.3389/fimmu.2022.840399/full#supplementary-material
Supplementary Figure 1 | Hierarchical clustering heatmaps of differentially methylated CpG sites considering genomic context. Hierarchical clustering heatmap of differentially methylated CpG sites in 3’UTR (A), 5’UTR (B), exon (C), intergenic (D), intron (E), promoter-TSS (F), TTS (G). Each row represents a methylated CpG site, H1, H2, H3 represent G. parasuis-infected porcine brain, and C1, C2, C3 represent negative controls.
Supplementary Figure 2 | Volcano plot showing differentially methylated CpGs at different DNA methylation difference thresholds. The vertical axis shows the -log10 (p-value), and the horizontal axis shows DNA methylation difference. Blue dots shows the hypo-methylated CpGs when DNA methylation difference threshold is 0.1, red dots shows the hyper-methylated CpGs when DNA methylation difference threshold is 0.1
1. Macedo N, Rovira A, Torremorell M. Haemophilus Parasuis: Infection, Immunity and Enrofloxacin. Vet Res (2015) 46:128. doi: 10.1186/s13567-015-0263-3
2. Kielstein P, Rapp-Gabrielson VJ. Designation of 15 Serovars of Haemophilus Parasuis on the Basis of Immunodiffusion Using Heat-Stable Antigen Extracts. J Clin Microbiol (1992) 30(4):862–5. doi: 10.1128/jcm.30.4.862-865.1992
3. Zhao Y, Wang Q, Li J, Lin X, Huang X, Fang B. Epidemiology of Haemophilus Parasuis Isolates From Pigs in China Using Serotyping, Antimicrobial Susceptibility, Biofilm Formation and ERIC-PCR Genotyping. PeerJ (2018) 6:e5040. doi: 10.7717/peerj.5040
4. Fu S, Zhang M, Xu J, Ou J, Wang Y, Liu H, et al. Immunogenicity and Protective Efficacy of Recombinant Haemophilus Parasuis SH0165 Putative Outer Membrane Proteins. Vaccine (2013) 31(2):347–53. doi: 10.1016/j.vaccine.2012.11.003
5. Putz K, Hayani K, Zar FA. Meningitis. Prim Care (2013) 40(3):707–26. doi: 10.1016/j.pop.2013.06.001
6. Kohil A, Jemmieh S, Smatti MK, Yassine HM. Viral Meningitis: AnOoverview. Arch Virol (2021) 166(2):335–45. doi: 10.1007/s00705-020-04891-1
7. Heckenberg SG, Brouwer MC, van de Beek D. Bacterial Meningitis. Handb Clin Neurol (2014) 121:1361–75. doi: 10.1016/B978-0-7020-4088-7.00093-6
8. Mount HR, Boyle SD. Aseptic and Bacterial Meningitis: Evaluation, Treatment, and Prevention. Am Fam Physician (2017) 96(5):314–22.
9. Wall EC, Chan JM, Gil E, Heyderman RS. Acute Bacterial Meningitis. Curr Opin Neurol (2021) 34(3):386–95. doi: 10.1097/WCO.0000000000000934
10. Kim KS. Acute Bacterial Meningitis in Infants and Children. Lancet Infect Dis (2010) 10(1):32–42. doi: 10.1016/S1473-3099(09)70306-8
11. Moraga J, Fica A, Navarrete B, Henriquez R, Navarrete-Asenjo C. [Meningitis and Hypoacusia Due to Swine Meat Handling]. Rev Chil Infectol (2018) 35(6):731–2. doi: 10.4067/S0716-10182018000600731
12. Jones PA. Functions of DNA Methylation: Islands, Start Sites, Gene Bodies and Beyond. Nat Rev Genet (2012) 13(7):484–92. doi: 10.1038/nrg3230
13. Moore LD, Le T, Fan G. DNA Methylation and its Basic Function. Neuropsychopharmacology (2013) 38(1):23–38. doi: 10.1038/npp.2012.112
14. Schübeler D. Function and Information Content of DNA Methylation. Nature (2015) 517(7534):321–6. doi: 10.1038/nature14192
15. Koch A, Joosten SC, Feng Z, de Ruijter TC, Draht MX, Melotte V, et al. Analysis of DNA Methylation in Cancer: Location Revisited. Nat Rev Clin Oncol (2018) 15(7):459–66. doi: 10.1038/s41571-018-0004-4
16. Celarain N, Tomas-Roig J. Aberrant DNA Methylation Profile Exacerbates Inflammation and Neurodegeneration in Multiple Sclerosis Patients. J Neuroinflamm (2020) 17(1):21. doi: 10.1186/s12974-019-1667-1
17. Perez-Novo CA, Bachert C. DNA Methylation, Bacteria and Airway Inflammation: Latest Insights. Curr Opin Allergy Clin Immunol (2015) 15(1):27–32. doi: 10.1097/ACI.0000000000000130
18. Qin W, Scicluna BP, van der Poll T. The Role of Host Cell DNA Methylation in the Immune Response to Bacterial Infection. Front Immunol (2021) 12:696280. doi: 10.3389/fimmu.2021.696280
19. Guo L, Liu J, Zhang Y, Fu S, Qiu Y, Ye C, et al. The Effect of Baicalin on the Expression Profiles of Long Non-Coding RNAs and mRNAs in Porcine Aortic Vascular Endothelial Cells Infected With Haemophilus Parasuis. DNA Cell Biol (2020) 39(5):801–15. doi: 10.1089/dna.2019.5340
20. Martin M. Cutadapt Removes Adapter Sequences From High-Throughput Sequencing Reads. EMBnet J (2011) 17(1):10–2. doi: 10.14806/ej.17.1.200
21. Krueger F, Andrews SR. Bismark: A Flexible Aligner and Methylation Caller for Bisulfite-Seq Applications. Bioinformatics (2011) 27(11):1571–2. doi: 10.1093/bioinformatics/btr167
22. Langmead B, Salzberg SL. Fast Gapped-Read Alignment With Bowtie 2. Nat Methods (2012) 9(4):357–9. doi: 10.1038/nmeth.1923
23. Haeussler M, Zweig AS, Tyner C, Speir ML, Rosenbloom KR, Raney BJ, et al. The UCSC Genome Browser Database: 2019 Update. Nucleic Acids Res (2019) 47(D1):D853–8. doi: 10.1093/nar/gky1095
24. Rosenbloom KR, Sloan CA, Malladi VS, Dreszer TR, Learned K, Kirkup VM, et al. ENCODE Data in the UCSC Genome Browser: Year 5 Update. Nucleic Acids Res (2013) 41(Database issue):D56–63. doi: 10.1093/nar/gks1172
25. Kim D, Langmead B, Salzberg SL. HISAT: A Fast Spliced Aligner With Low Memory Requirements. Nat Methods (2015) 12(4):357–60. doi: 10.1038/nmeth.3317
26. Pertea M, Kim D, Pertea GM, Leek JT, Salzberg SL. Transcript-Level Expression Analysis of RNA-Seq Experiments With HISAT, StringTie and Ballgown. Nat Protoc (2016) 11(9):1650–67. doi: 10.1038/nprot.2016.095
27. Pertea M, Pertea GM, Antonescu CM, Chang TC, Mendell JT, Salzberg SL. StringTie Enables Improved Reconstruction of a Transcriptome From RNA-Seq Reads. Nat Biotechnol (2015) 33(3):290–5. doi: 10.1038/nbt.3122
28. Robinson MD, McCarthy DJ, Smyth GK. Edger: A Bioconductor Package for Differential Expression Analysis of Digital Gene Expression Data. Bioinformatics (2010) 26(1):139–40. doi: 10.1093/bioinformatics/btp616
29. Sadler T, Bhasin JM, Xu Y, Barnholz-Sloan J, Chen Y, Ting AH, et al. Genome-Wide Analysis of DNA Methylation and Gene Expression Defines Molecular Characteristics of Crohn’s Disease-Associated Fibrosis. Clin Epigenet (2016) 8:30. doi: 10.1186/s13148-016-0193-6
30. Li Z, Chen S, Yang Y, Zhuang X, Tzeng CM. Novel Biomarker ZCCHC13 Revealed by Integrating DNA Methylation and mRNA Expression Data in Non-Obstructive Azoospermia. Cell Death Discovery (2018) 4:36. doi: 10.1038/s41420-018-0033-x
31. Maunakea AK, Nagarajan RP, Bilenky M, Ballinger TJ, D’Souza C, Fouse SD, et al. Conserved Role of Intragenic DNA Methylation in Regulating Alternative Promoters. Nature (2010) 466(7303):253–7. doi: 10.1038/nature09165
32. Irizarry RA, Ladd-Acosta C, Wen B, Wu Z, Montano C, Onyango P, et al. The Human Colon Cancer Methylome Shows Similar Hypo- and Hypermethylation at Conserved Tissue-Specific CpG Island Shores. Nat Genet (2009) 41(2):178–86. doi: 10.1038/ng.298
33. Pacis A, Tailleux L, Morin AM, Lambourne J, MacIsaac JL, Yotova V, et al. Bacterial Infection Remodels the DNA Methylation Landscape of Human Dendritic Cells. Genome Res (2015) 25(12):1801–11. doi: 10.1101/gr.192005.115
34. Yamazaki T, Hatano Y, Taniguchi R, Kobayashi N, Yamagata K. Editing DNA Methylation in Mammalian Embryos. Int J Mol Sci (2020) 21(2):637. doi: 10.3390/ijms21020637
35. Chen Z, Zhang Y. Role of Mammalian DNA Methyltransferases in Development. Annu Rev Biochem (2020) 89:135–58. doi: 10.1146/annurev-biochem-103019-102815
36. Choy JS, Wei S, Lee JY, Tan S, Chu S, Lee TH. DNA Methylation Increases Nucleosome Compaction and Rigidity. J Am Chem Soc (2010) 132(6):1782–3. doi: 10.1021/ja910264z
37. Wood AJ, Schulz R, Woodfine K, Koltowska K, Beechey CV, Peters J, et al. Regulation of Alternative Polyadenylation by Genomic Imprinting. Genes Dev (2008) 22(9):1141–6. doi: 10.1101/gad.473408
38. Yau B, Hunt NH, Mitchell AJ, Too LK. BloodBrain Barrier Pathology and CNS Outcomes in Streptococcus Pneumoniae Meningitis. Int J Mol Sci (2018) 19(11):3555. doi: 10.3390/ijms19113555
39. Jin T, Guan N, Du Y, Zhang X, Li J, Xia X. Cronobacter Sakazakii ATCC 29544 Translocated Human Brain Microvascular Endothelial Cells via Endocytosis, Apoptosis Induction, and Disruption of Tight Junction. Front Microbiol (2021) 12:675020:675020. doi: 10.3389/fmicb.2021.675020
40. Anil A, Banerjee A. Pneumococcal Encounter With the Blood-Brain Barrier Endothelium. Front Cell Infect Microbiol (2020) 10:590682:590682. doi: 10.3389/fcimb.2020.590682
41. Zeng Z, Lan T, Wei Y, Wei X. CCL5/CCR5 Axis in Human Diseases and Related Treatments. Genes Dis (2022) . 9(1):12–27. doi: 10.1016/j.gendis.2021.08.004
42. Karimabad MN, Kounis NG, Hassanshahi G, Hassanshahi F, Mplani V, Koniari I, et al. The Involvement of CXC Motif Chemokine Ligand 10 (CXCL10) and Its Related Chemokines in the Pathogenesis of Coronary Artery Disease and in the COVID-19 Vaccination: A Narrative Review. Vaccines (Basel) (2021) 9(11):1224. doi: 10.3390/vaccines9111224
43. Kuklina E. Semaphorin 4D as a Guidance Molecule in the Immune System. Int Rev Immunol (2021) 40(4):268–73. doi: 10.1080/08830185.2021.1905807
44. Kuklina EM. Receptor Functions of Semaphorin 4d. Biochem (Mosc) (2019) 84(9):1021–7. doi: 10.1134/S0006297919090049
45. Rajabinejad M, Asadi G, Ranjbar S, Afshar Hezarkhani L, Salari F, Gorgin Karaji A, et al. Semaphorin 4A, 4C, and 4D: Function Comparison in the Autoimmunity, Allergy, and Cancer. Gene (2020) 746:144637. doi: 10.1016/j.gene.2020.144637
46. Zhou H, Yang YH, Basile JR. Characterization of the Effects of Semaphorin 4d Signaling on Angiogenesis. Methods Mol Biol (2017) 1493:429–41. doi: 10.1007/978-1-4939-6448-2_31
47. Ashrafi M, Sebastian A, Shih B, Greaves N, Alonso-Rasgado T, Baguneid M, et al. Whole Genome Microarray Data of Chronic Wound Debridement Prior to Application of Dermal Skin Substitutes. Wound Repair Regener (2016) 24(5):870–5. doi: 10.1111/wrr.12460
48. Fitzgerald J. WARP: A Unique Extracellular Matrix Component of Cartilage, Muscle, and Endothelial Cell Basement Membranes. Anat Rec (Hoboken) (2020) 303(6):1619–23. doi: 10.1002/ar.24087
49. Pagnamenta AT, Kaiyrzhanov R, Zou Y, Da’as SI, Maroofian R, Donkervoort S, et al. An Ancestral 10-Bp Repeat Expansion in VWA1 Causes Recessive Hereditary Motor Neuropathy. Brain (2021) 144(2):584–600. doi: 10.1093/brain/awaa420
50. Deschauer M, Hengel H, Rupprich K, Kreiss M, Schlotter-Weigel B, Grimmel M, et al. Bi-Allelic Truncating Mutations in VWA1 Cause Neuromyopathy. Brain (2021) 144(2):574–83. doi: 10.1093/brain/awaa418
Keywords: Glaesserella parasuis, meningitis, inflammation, Whole-genome DNA methylation, transcriptome
Citation: Guo L, Cheng H, Fu S, Liu J, Zhang Y, Qiu Y and Chen H (2022) Methylome and Transcriptome-Based Integration Analysis Identified Molecular Signatures Associated With Meningitis Induced by Glaesserella parasuis. Front. Immunol. 13:840399. doi: 10.3389/fimmu.2022.840399
Received: 21 December 2021; Accepted: 07 February 2022;
Published: 25 February 2022.
Edited by:
Cheong-Meng Chong, University of Macau, ChinaReviewed by:
Michael Jay Corley, Cornell University, United StatesCopyright © 2022 Guo, Cheng, Fu, Liu, Zhang, Qiu and Chen. This is an open-access article distributed under the terms of the Creative Commons Attribution License (CC BY). The use, distribution or reproduction in other forums is permitted, provided the original author(s) and the copyright owner(s) are credited and that the original publication in this journal is cited, in accordance with accepted academic practice. No use, distribution or reproduction is permitted which does not comply with these terms.
*Correspondence: Yinsheng Qiu, cWl1eWluc2hlbmc2NDA1QGFsaXl1bi5jb20=
†These authors have contributed equally to this work
Disclaimer: All claims expressed in this article are solely those of the authors and do not necessarily represent those of their affiliated organizations, or those of the publisher, the editors and the reviewers. Any product that may be evaluated in this article or claim that may be made by its manufacturer is not guaranteed or endorsed by the publisher.
Research integrity at Frontiers
Learn more about the work of our research integrity team to safeguard the quality of each article we publish.