- 1DSI-NRF Centre of Excellence for Biomedical Tuberculosis Research, South African Medical Research Council Centre for Tuberculosis Research, Division of Molecular Biology and Human Genetics, Faculty of Medicine and Health Sciences, Stellenbosch University, Cape Town, South Africa
- 2South African Tuberculosis Vaccine Initiative, Division of Immunology, Department of Pathology, Institute of Infectious Disease and Molecular Medicine, University of Cape Town, Cape Town, South Africa
- 3Division of Pulmonology, Department of Medicine, Stellenbosch University and Tygerberg Academic Hospital, Cape Town, South Africa
Myeloid-derived suppressor cells (MDSC) have been identified in the peripheral blood and granulomas of patients with active TB disease, but their phenotype-, function-, and immunosuppressive mechanism- spectrum remains unclear. Importantly, the frequency and signaling pathways of MDSC at the site of disease is unknown with no indication how this compares to MDSC identified in peripheral blood or to those of related myeloid counterparts such as alveolar macrophages and monocytes. Most phenotypic and functional markers have been described in oncological studies but have not yet been validated in TB. Using a panel of 43 genes selected from pathways previously shown to contribute to tumor-derived MDSC, we set out to evaluate if the expression of these additional functional markers and properties may also be relevant to TB-derived MDSC. Differential expression was investigated between MDSC, alveolar macrophages and monocytes enriched from bronchoalveolar lavage fluid and peripheral blood of patients with active TB, patients with other lung diseases (OLD). Results demonstrated that anatomical compartments may drive compartment-specific immunological responses and subsequent MDSC immunosuppressive functions, demonstrated by the observation that MDSC and/or monocytes from PB alone can discriminate, via hierarchical clustering, between patients with active TB disease and OLD. Our data show that the gene expression patterns of MDSC in peripheral blood and bronchoalveolar lavage fluid do not cluster according to disease states (TB vs OLD). This suggests that MDSC from TB patients may display similar gene expression profiles to those found for MDSC in cancer, but this needs to be validated in a larger cohort. These are important observations for TB research and may provide direction for future studies aimed at repurposing and validating cancer immunotherapies for use in TB.
Introduction
Tuberculosis (TB), one of the most prominent communicable diseases in existence, is caused by Mycobacterium tuberculosis (M.tb) infection (1). While some people remain asymptomatically infected, others develop active disease. A major aim of TB research efforts is to characterize host immune components contributing to TB disease susceptibility and resistance (2). Host immune modulators are currently explored as a popular approach to enhance immune responses through novel vaccines and drugs thereby reducing susceptibility to infection, delaying disease progression and accelerating cure (2–4).
Myeloid derived suppressor cells (MDSC) are a heterogenous population of immature myeloid cells, with potent immune suppressive functions (5). MDSC are further grouped into subsets, such as immature MDSC progenitor cells known as early-stage MDSC (e-MDSC), polymorphonuclear MDSC (PMN-MDSC) and monocytic MDSC (M-MDSC) (6–8). The most recently described subset of eosinophilic MDSC (Eo-MDSC) expand our understanding of the suppressive granulocyte lineage (9). A multitude of immunosuppressive mechanisms have been reported for tumor-derived MDSC, presumably reflective of the heterogeneity and functional redundancy of this cell population. While these mechanisms are designed to suppress excess inflammation, they are frequently demonstrated to rather inactivate pivotal immune responses required for protection against tumor cells and pathogenic infections alike (10, 11).
As a major immunotherapeutic target in the field of oncology, studies of tumor-derived MDSC suggest that the factors involved in their differentiation, expansion and activation can be characterized in two classes. Firstly, factors stimulating myelopoiesis and inhibiting differentiation from immature myeloid cells (IMC) into mature myeloid cells (MMC) (5, 12, 13) enhance the accumulation of IMC in lymphoid organs and the tumor microenvironment (cancer). Such factors include interleukin (IL)-6 and vascular endothelial growth factor alpha (VEGFA) which activate the signal transducer and activator of transcription factor 3 (STAT3) signaling pathway (14–17). Secondly, factors such as such as the transcription factors c-myc and C/EBP homologous protein (CHOP), reactive oxygen species (ROS), inducible nitric oxide synthases (iNOS), arginase, IFN-y, TNF-α, IL-13, S100A8/9, transforming growth factor beta (TGF-β), IL-10, and prostaglandin E2 (PGE2) are involved in the pathological expansion and activation of hematopoietic stem cells (HPC) into IMC and eventually MDSC (5, 16). In cancer, these factors are secreted by activated T cells and tumor stromal cells (5, 14–16).
Suppressive myeloid cells have long been known to be expanded in cancer (18, 19), but the identification of their expansion in other inflammatory conditions including infectious diseases such as active TB disease, are more juvenile. Increased MDSC frequencies have been measured in the peripheral blood of patients with active pulmonary TB disease (20–22), however, little is known about the true phenotype and immunosuppressive mechanisms of these cells in TB. Importantly, the frequency and signaling pathways of MDSC at the site of TB disease (the lung) is also unknown with no indication how this compares to those identified in the blood or to those of related myeloid counterparts such as alveolar macrophages, monocytes, dendritic cells, or neutrophils. In recent studies it was shown that MDSC exhibit monocytic and granulocytic morphologies, although M-MDSC appear more relevant in the context of M.tb-infected participation, due to its phagocytic potentials (20, 23, 24).
In the field of immuno-oncology, the minimally accepted set of surface markers for phenotypic classification of human MDSC is HLA-DR, CD33, CD11b and CD14 (M-MDSC) or CD15 (PMN-MDSC) (25). These markers have also been adopted in the human TB MDSC research field, specifically to characterize blood-derived MDSC. Many additional phenotypic and functional markers have, however, recently been described in oncological studies, relating to the function and ontology of MDSC, the most promising being LOX-1 (PMN-MDSC) and S100A9 (M-MDSC) (26–28). Here we set out to evaluate if the expression of these and other functional markers and functional properties associated with MDSC in cancer, may also be relevant to TB-derived MDSC. Additionally, we hypothesize that MDSC from the blood of TB patients could display similar characteristics to lung-derived MDSC but would differ to those of other control myeloid subsets. We make use of myeloid cells from peripheral blood cells and bronchoalveolar lavage cells from of individuals with active TB or with non-TB MDSC-inducing lung diseases, other than TB, to assess expression of genes previously shown to be linked to MDSC in lung malignancies. Specifically, we compare expression between MDSC, alveolar macrophages (AM) and monocytes, to determine how these compare between myeloid subsets.
Materials and Methods
Ethics Approval and Statement
Ethical approval was obtained from the Health Research Ethics Committee of Stellenbosch University as part of ongoing clinical studies, namely TANDEM: Concurrent Tuberculosis and Diabetes Mellitus - Unravelling the causal link and improving care (N13/05/064); ICIDR: Biology and Biosignatures of Anti-Tuberculosis Treatment Response (NIH/U01/AI115619); Screen TB study: Evaluation of host biomarker-based point-of-care tests for targeted screening for active TB (N16/05/070 and N16/04/050); The effect of cigarette smoking on host and M.tb responses (N10/08/276). The study was conducted in accordance with the Declaration of Helsinki and International Conference on Harmonisation guidelines.
Participant Recruitment and Sample Collection
Participants were enrolled from a sub-district in Cape Town, South Africa and written informed consent was obtained. Participants were grouped as healthy household contacts (HHC), other lung diseases (OLD) or active TB (Figure 1). The criteria for inclusion in the HHC or OLD groups were no clinical, radiological, or microbiological signs of active TB with a negative sputum GeneXpert. The inclusion criteria for active TB were clinical signs of TB (symptoms such as, persistent cough, fever, night sweats, weight loss, loss of appetite) with a chest x-ray screen showing signs of TB, or a positive sputum culture test (culture negative TB included if positive for GeneXpert). Participants were excluded if they had a history of previous TB, tested positive for diabetes, or tested positive for HIV. Peripheral blood (PB) was obtained by venepuncture into NaHep Vacutainers®. broncho-alveolar lavage fluid (BALF) was collected by bronchoscopy conducted by an experienced pulmonologist. Samples were processed within 2 hours of collection.
Immune Cell Enrichment
Peripheral blood mononuclear cells (PBMC) were isolated from PB using the standard ficoll density medium separation method (GE Healthcare, Piscataway, New Jersey, United States of America). BALF was filtered using a 70 µm cell strainer and the cell pellet was washed using RPMI supplemented with 5% FBS. MDSC were enriched from PBMC and BALF cells using magnetic-activated cell sorting (MACS) through a series of negative and positive selection steps, to obtain the CD3-/HLA-DR-/CD33+ cell fraction enriched for MDSC (MiltenyiBiotec, Germany). In parallel, for each participant, monocytes (from PBMC) and AM (from BAL) were enriched from the HLA-DR+ fraction through plastic adherence in an overnight culture. Cells were counted and viability assessed through Trypan Blue staining. Samples displayed viability >95% and the quality and efficacy of the isolation was assessed by flow cytometric assessment of peripheral monocytes (CD33+HLA-DR+-PE) and MDSC (HLA-DR-; CD33+ BV510) (BD FACS Canto II). Data was analysed using FlowJo (version 10). Enriched fractions were stored in RLT Plus® Buffer (Qiagen, Germany) at -80°C for batch analysis.
RNA Extraction, cDNA Synthesis and Quantitative Real-Time PCR (qRT-PCR)
Total RNA was isolated from enriched cell fractions using the RNEasy® Micro Kit (Qiagen, Germany) according to the manufacturer’s instructions and stored at -80°C. The RNA purity, quality and quantity were assessed using the Agilent 2100 BioAnalyzer (Agilent Technologies Inc.) An RNA integrity number (RIN) score above 7 was considered sufficient for downstream processing. Isolated RNA was thawed on ice and cDNA synthesized using the Fluidigm Reverse Transcription Master Mix (Fluidigm PN 100-6297) and a conventional thermal cycler (Life Technologies, United States of America). Aliquots of the cDNA were further subjected to either 16 or 20 pre-amplification cycles using the Fluidigm One Tube PreAmp Master Mix (Fluidigm PN 100-5580) and DNA was removed by exonuclease-I treatment (PN M0293S/L; 20 U/µl).
Multiplexed Gene Expression Analysis
A customised 48-gene panel (Delta Gene Assay, Fluidigm, California, USA) was designed, based on phenotypic and functional markers related to MDSC as reported in the literature from in vitro or in vivo studies of various diseases in humans or animal models (Table 1). Included in these were 5 candidate reference genes: ACTB (NM_001101.N), G6PD (NM_000402.N), GAPDH (NM_001289745), RPS12 (NM_001016.N), and RPS18 (NM_022551.N). The multiplex qRT-PCR was conducted according to manufacturer’s instructions (Fluidigm BioMark HD-HX platform, California, United States of America). All genes were assayed in duplicate.
Controls included no-template controls, no-reverse-transcription controls, and no-pre-amplification controls. Upon successful completion of the Fluidigm assay the Ct-values (the fractional number of cycles required for the signal to cross the detection threshold), proportional to the inverse gene expression levels, were exported for further analysis.
Data Processing and Statistical Analysis
Data processing and analysis was performed using R [“R Core Team (29)] along with the BioConductor packages readqPCR and normqPCR. R packages tidyr, ggplot2 and ComplexHeatmap were used for processing and visualization of the data. Bayesian statistical analysis was done using Stan (30) via the brms package (31). Analysis of differences in expression levels (Ct-values) was done using Bayesian multilevel models with either cell-type or sample-type as the fixed-effect modelled using a student t-distribution and subject and pre-amplification cycle as random intercept effects with a default flat prior on the effect estimate. Sampling was set at 5000 iterations, including a warmup of 1500, using 4 chains and an adapt_delta value of 0.9. Correlation analysis was done using the Spearman rank method.
Results
Participant Characteristics, Sample Size and Immune Cell Enrichment
Peripheral blood (n=8) and BALF (n=9) samples were collected from participants upon diagnosis of active pulmonary TB disease prior to initiation of standard antibiotic treatment and controls with lung disease other than TB, residing in the same community (n=4) (Table 2). Of the OLD patients, the OLD included pulmonary complications associated with leukaemia, a pulmonary fungal infection, lung cancer, and sarcoidosis.
MDSC (CD3-/HLA-DR-/CD33+) were enriched from PBMC and BALF, while AMs and monocytes (CD3-/HLA-DR+/CD33+) were enriched from BALF and PBMC, respectively, using MACS isolation technology, as compartmental control populations. Purity checks were run on the enriched MDSC and monocyte populations by flow cytometry and were demonstrated to have an average purity of 73% and 100%, respectively. Because of the lower purity of the MDSC population, this is a population enriched for MDSC, rather than a pure population. Purity checks could not be performed on the AMs owing to the known issues with autofluorescence in these cells because of the high level of carbon loading within the macrophage population of participants from the Western Cape province of South Africa (32). Literature shows that between 85-96% of cells from the BALF of the human lung are alveolar macrophages (33, 34). Therefore, while not 100% pure, the cells obtained from the lung in this study are enriched for AMs.
Reference Gene Selection
To compare gene expression levels between cell or sample types, the absolute expression levels were first normalized using the Ct values for a set of reference genes. A panel of five candidate reference genes was selected as endogenous controls based on the criterion of constitutive expression, regardless of cell, tissue, or sample type. These were: ACTB, G6PD, GAPDH, RPS12 and RPS18. We selected an optimal subset of these candidate reference genes based on an assessment of gene expression stability as determined by the method of Vandersmpele et al. using the geNorm package (35). We found good average stability (M-values, higher values denote lower stability) of the reference sets, improving with the progressive exclusion each least stable gene. The pairwise ratios of the M-values showed nominal changes in stability with each additional reference gene, although we observed a notable increase with the addition of a fifth gene in some sample-types. The implication being that there is little benefit to be gained from using more than the recommended three reference genes. As ACTB and RPS18 were the least stable references in the 16- and 20-times pre-amplification assays respectively, we chose to exclude both. Normalised delta-Ct values were therefore calculated based on the geometric mean expression of the G6PD, GAPDH and RPS12 genes.
Hierarchical Clustering and Statistical Analysis of Normalized Expression Data
Gene Expression Profiles of MDSC and/or Monocytes in the Blood Discriminate Between Patients With TB Versus Those With OLD
It remains unclear how the phenotype and function of MDSC differ in various chronic inflammatory conditions. This information will be critical in selecting and designing targeted host-directed therapeutic (HDT) strategies and inform repurposing of compounds being tested in immune-oncology. We measured the expression of 43 target genes, previously shown to have relevance in MDSC phenotype and function in immune-oncology, in peripheral blood-derived MDSC and monocytes from TB patients and those with OLD, (Figure 2A). We observed no obvious distinction between the expression patterns of either peripheral MDSC or monocytes between patients with TB versus those with OLD. We also investigated changes in gene expression of BAL-derived MDSC and monocytes between patients with active TB and OLD. Hierarchical cluster analysis showed no obvious distinction between the expression patterns of either BAL-derived MDSC or AM between TB and OLD (Figure 2B).
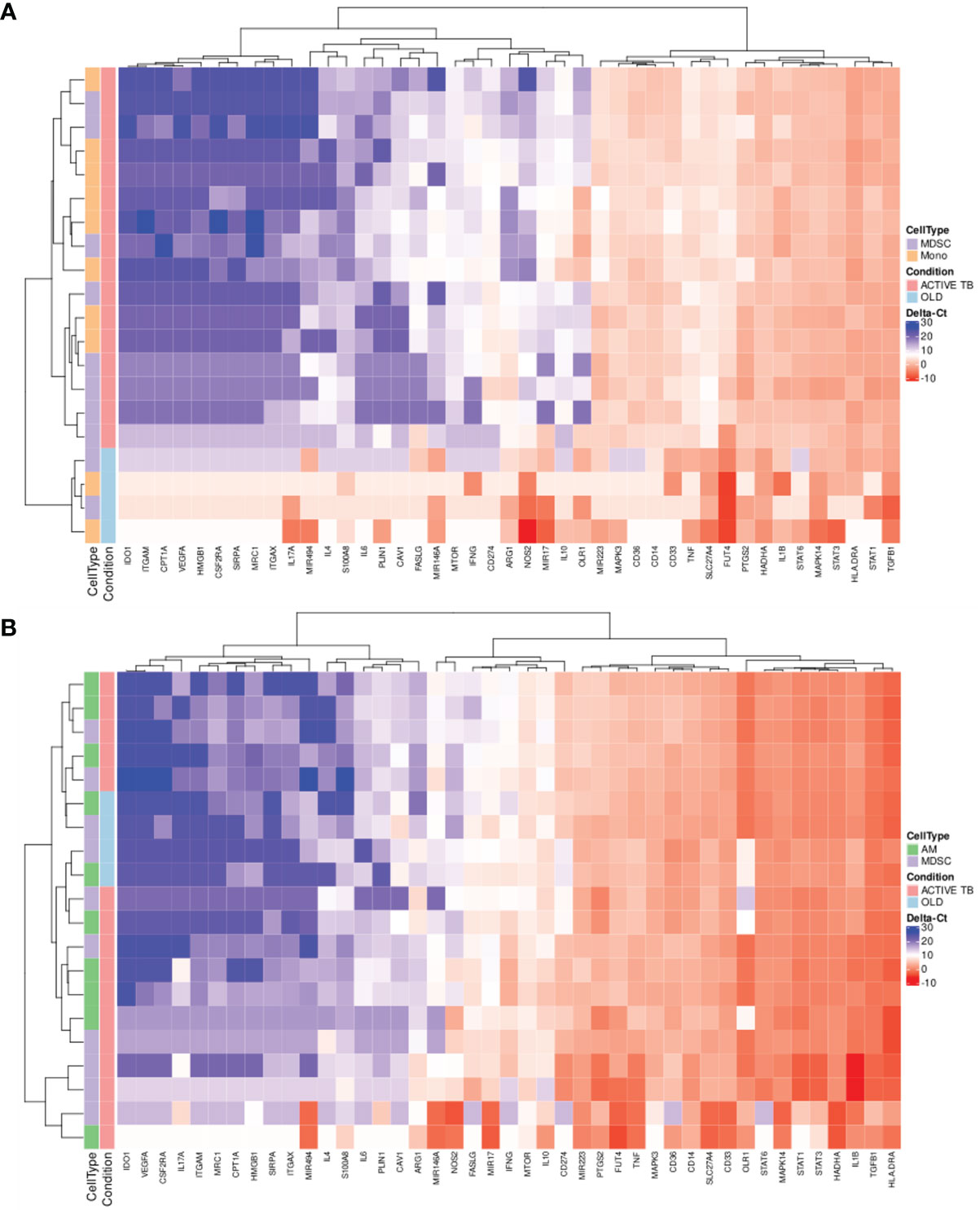
Figure 2 Scaled gene expression patterns of (A) MDSC and monocytes in PB (16x pre-amplification), and (B) MDSC and AM in BALF (16x pre-amplification). Lower expression (higher ΔCt-values) is indicated by blue. Hierarchical clustering of similar expression patterns across subject and genes is indicated by the dendrograms.
Gene expression can also be employed for the discovery of discriminatory gene or multigene signatures in disease. Interestingly, hierarchical cluster analysis of gene expression levels in both MDSC and monocytes in PB displayed clear differences between patients with active TB and those with OLD, resulting in complete separation of the two conditions (Figure 2A).
Blood-Derived MDSC and Monocytes From TB Patients Display Differential Enrichment in Selected Gene Transcriptional Levels
Hypotheses as to the source of circulating MDSC in TB patients include the export of immature cells into the blood stream through emergency myelopoiesis or the reprogramming of exported peripheral monocytes (18, 36, 37). Some have shown that these reprogrammed monocytes also have the same phenotype and function as M-MDSC in cancer patients (26). We therefore assessed the transcriptional differences of the 43 genes, previously shown to demonstrate differential abundance between monocytes and MDSC in cancer, in TB patient-derived MDSC and TB patient-derived monocytes. Statistical analysis of gene expression between MDSC and monocytes in PB of active TB patients yielded differences in ΔCt-values in 12 genes at the 95% Bayesian highest posterior density interval (HDI) level (Table 3, starred values). A further 9 genes (Table 3, not starred) showed possible differential expression at the 90% HDI level and require further investigation. Of these 21 genes, seven showed a ΔΔCt of at least 2, equivalent to a difference in expression of a factor of at least 4. Of these, ARG1, CPT1, ITGAM, IL4 and IL17A showed higher expression in MDSC than in monocytes, whereas IFNG and FASLG expression was lower in MDSC. The changes in all 43 genes’ ΔCt-values can be found in Supplementary Figure 1.
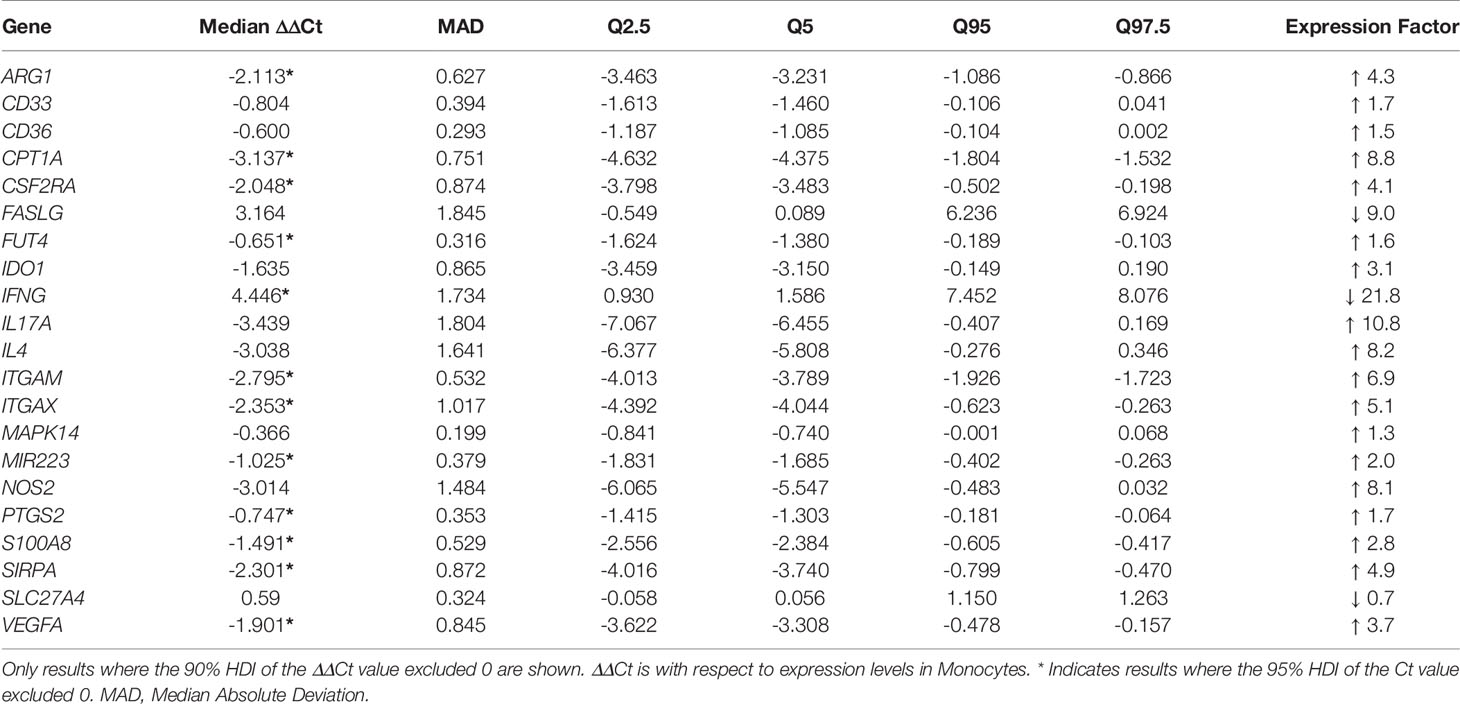
Table 3 Differential gene expression levels between MDSC and Monocytes from PB, with respect to expression levels in Monocytes.
Lung-Derived MDSC and Macrophages From TB Patients Display Differential Enrichment in Selected Gene Transcriptional Levels
The mechanism(s) employed by MDSC to suppress T-cell responses in TB is yet unknown and investigation into these will improve selection of precision strategies to specifically target MDSC. We included in our gene panel a selection of genes known to mediate the suppressive function of tumor-derived MDSC, to evaluate if these are enriched also in TB-derived MDSC. Importantly, the suppressive function of MDSC is likely to feature at the inflammation site, which in cancer is at the tumor site, or in the case of TB, the lung infection site. MDSC and AM, isolated from lung-derived BALF of TB patients, yielded gene expression differences in ΔCt-values in 6 genes at the 95% HDI level (Table 4, starred values). Four of these: CAV1, IL10, IL6 and MIR146A, showed a difference in ΔCt of at least 4. In all four, expression was lower in MDSC than in AM. Only IL1B expression was higher in MDSC versus AM. The changes in all 43 genes’ ΔCt-values can be found in Supplementary Figure 2.
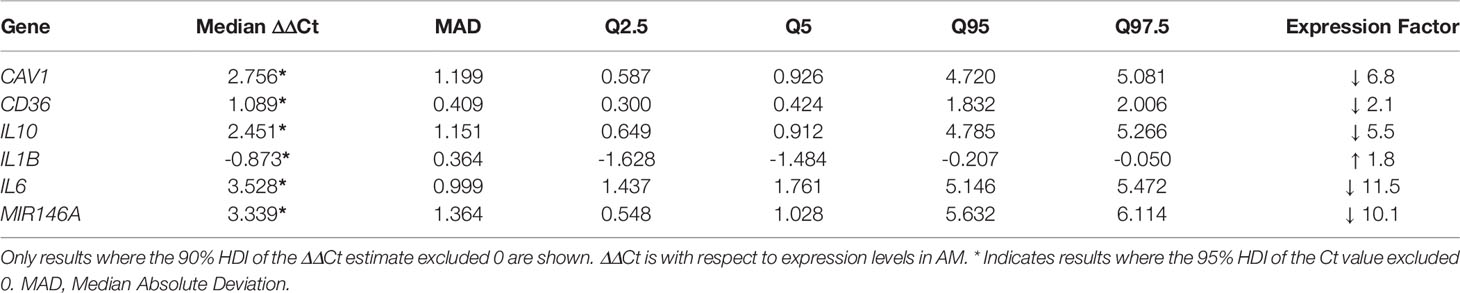
Table 4 Differential gene expression levels between MDSC and AM in BALF, with respect to expression levels in AM.
Gene Expression Profiling Suggests That MDSC Phenotype and Function Differ Depending on Anatomical Compartments in TB Patients
The fate of circulating MDSC in pulmonary TB patients has not been determined, but the theory is that MDSC released from the bone marrow migrate via the blood stream to the site of infection in the lung. How the transcriptional profile of circulating MDSC compares to MDSC entering the disease site, remains to be determined. Statistical analysis of gene expression between MDSC in PB and BALF of active TB patients yielded differences in ΔCt-values in 7 genes at the 95% HDI level (Table 5, starred values). Five of these: IFNG, IL1B, OLR1, SLC27A4 and TNF, showed a difference in ΔCt of at least 2 in expression. In all five, expression was lower in PB MDSC than in BALF MDSC. Of note is OLR1 which was lower by a factor of 180.9 in MDSC in PB compared to BALF. A further 3 genes showed possible differential expression at the 90% HDI level (Table 5, not starred). Hierarchical cluster analysis of gene expression levels in MDSC in PB and BALF did not discriminate patients with active TB from those with OLD (Figure 3). We also observed no obvious distinction between the expression patterns of the two cell-types. As PB is a much more accessible sample-type that BALF, we did a correlation analysis of the gene expression levels between the two compartments. The results showed moderate to no correlation (Supplementary Table 1). The changes in all 43 genes’ ΔCt-values can be found in Supplementary Figure 3.
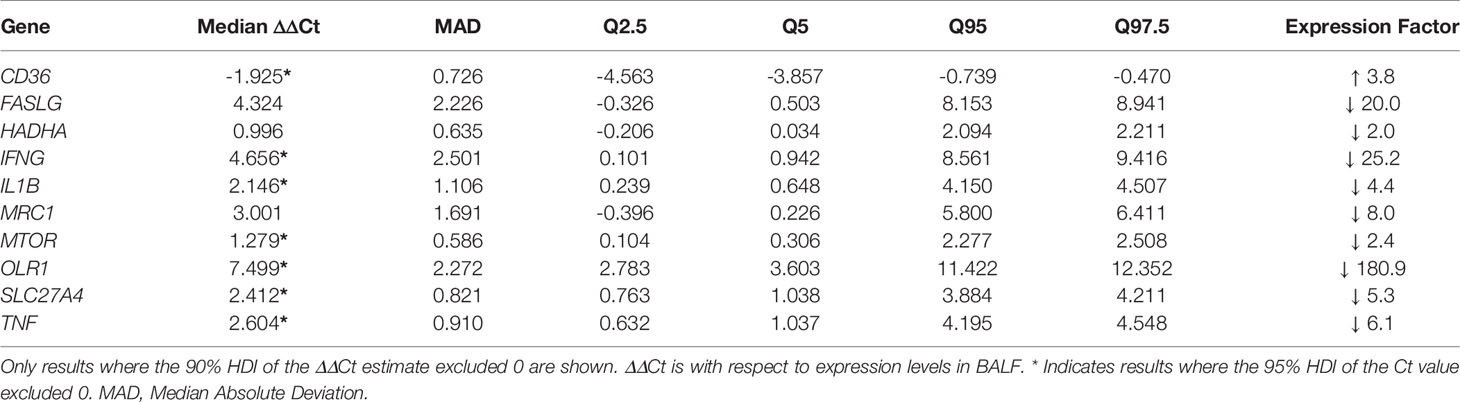
Table 5 Differential gene expression levels between MDSC in BALF and PB, with respect to expression levels in BALF.
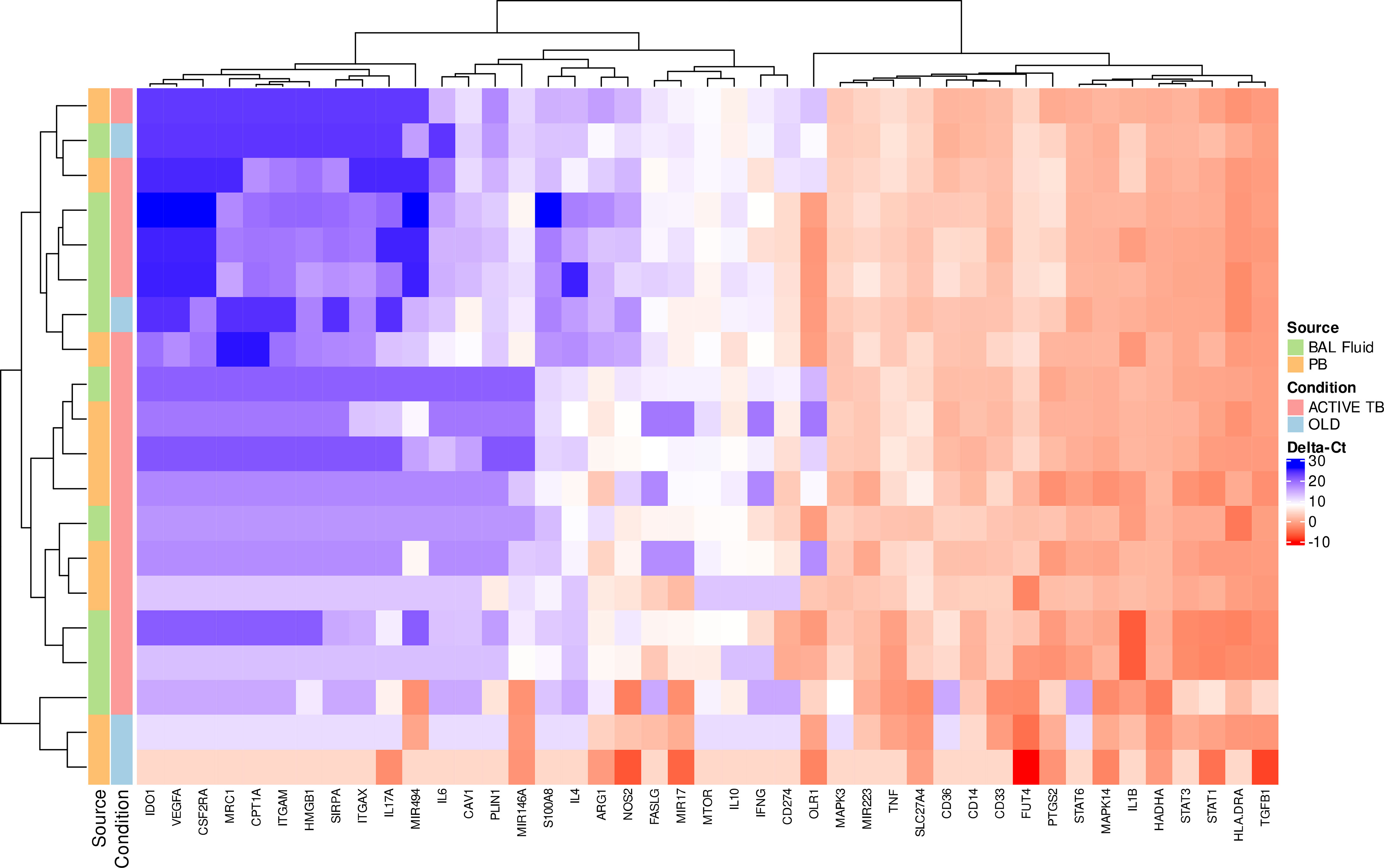
Figure 3 Scaled gene expression patterns of MDSC in BALF and PB (16x pre-amplification). Lower expression (higher ΔCt-values) is indicated by blue. Hierarchical clustering of similar expression patterns across subject and genes is indicated by the dendrograms.
Discussion
The phenotype and function of MDSC in the context of TB remains incompletely defined. Most importantly, PB blood has long been used for measurement of soluble mediators and immune cell profile during TB disease, as proxy for the immunological profile at the site-of-disease (38). Previous studies have focussed on profiling actively expressed receptors on the surface of MDSC in the periphery during active TB and their soluble mediators, but few have investigated the gene expression profiles of MDSC isolated from different immunological compartments (20, 24, 39, 40). The immune phenotype of MDSC in relation to other related immune cell types have also not been compared between the periphery and the lungs during active TB disease. To address these shortfalls, we performed a pilot, observational study in which we assembled a panel of 43 genes from pathways previously shown to contribute to MDSC phenotype, activation, and function in tumor biology. Since MDSC are actively targeted in immune-oncology, owing to the comprehensive knowledge available on the role of these cells in failed host immunity to cancer cells (41), we aimed to identify which MDSC-related pathways also applies to TB. We measured the expression of these genes in MDSC, AM and monocytes from BALF and PB of patients with TB, and those of patients with lung disease other than TB, previously described to show an upregulation in MDSC. This information is important to inform the selection of HDT strategies in TB, specifically those that may be repurposed from the immune-oncology field should the gene expression profiles of MDSC in TB and cancer (OLD) be similar.
In this study, we measured the expression of 43 target genes previously shown to have relevance in MDSC phenotype and function in immune-oncology, to determine their relevance in TB-derived MDSC. We compared these MDSC expression signatures to those observed in control monocyte and AM populations owing to the majority of MDSC in the blood being of the monocyte lineage. Studies have demonstrated that M-MDSC are the predominant subset in the periphery (20, 21, 24), and data from immune-oncology studies have demonstrated that M-MDSC entering the tumor are capable of differentiating into tumor-associated macrophages (TAMs), supporting the use of alveolar macrophages as a control population from the lung (42).
In this study we did not observe any significant differences in gene expression in blood-derived MDSC between active TB patients and those with OLD. We also did not measure any significant changes in gene expression of BAL-derived MDSC between active TB patients and those with OLD. The same was also observed for blood- and BAL-derived monocytes between TB patients and OLD. Although there were only two OLD patients in the dataset, we observed a clustering capable of distinguishing between the two groups of patients using PB. This was true for both MDSC and monocytes which suggests that it may be worthwhile investigating the possibility of a PB, cell type-specific RNA diagnostic signature for TB in a larger study. Interestingly, no such discriminating pattern was observed for either MDSC or AM isolated from BALF. These results suggest that there may still be value in investigating PB-specific RNA biomarkers, specifically for the discrimination of OLD from TB disease, and that perhaps previous studies looking at secreted soluble mediators should rather investigate at a gene expression level.
Monocytes and MDSC derived from the PB of TB patients recruited in this study demonstrated 21 differentially expressed genes at both the 90% and 95% Bayesian HDI level. It is interesting to note that of the 21 differentially expressed genes between MDSC and monocytes in PB, many of the genes were associated with the PMN-MDSC subset specifically. These included FUT4, IFNG, ITGAM, MIR223, PTGS2, VEGFA and MAPK14 to name a few. Conventional studies using PB rely on the cryopreservation of PBMC for batched downstream analyses which is known to result in the loss of the majority, if not the entire, PMN-MDSC subset (43). Because this study made use of PBMC immediately stored in an RNA-preserving buffer, we were able to preserve PMN-MDSC-specific RNA for differential expression analyses. It is clear from these results that the PMN-MDSC subset is severely under-reported in the context of active TB disease, and its functional role has subsequently not been accounted for. It would be prudent for future studies to identify realistic PMN-MDSC proportions in the PB of patients with active TB disease and focus on their immunosuppressive role.
Among the differentially expressed genes were also IFNG and IL17A, which were 21.8 times lower and 10.8 times higher in MDSC than in monocytes, respectively. The former finding was not unexpected. Current knowledge suggests that, during active TB disease, ARG1 and NOS2 play a pivotal role in the suppression of T cell proliferation and IFN-γ production, thereby preventing the efficient killing of intracellular pathogens in macrophages and impairing T cell activation (21, 44–46). The 10-fold upregulation of IL17A alludes to the role of IL-17-producing CD4+ T cells (Th17 cells) during tumor development, which drive tumorigenesis (47). In contrast, IL-17A is known to be protective against mycobacterial infection in the host, and a lack thereof increases TB susceptibility (48). Given that the main producing cell type of IL-17A remains CD4+ and CD8+ T cells, evidence suggests that TAMs (derived from M-MDSC) are capable of producing IL-17A and may therefore be present in the PB of active TB patients (49). Other differentially expressed genes of note were FASLG (9 times lower in MDSC than monocytes), and CPT1A (8.8 times higher in MDSC than monocytes). The expression of FASLG on the surface of MDSC is responsible for inducing the apoptosis of T cells by binding with T cell-expressed Fas (50, 51). Data from FASLG deficient mice demonstrate that the lack thereof results in a significant reduction of MDSC in the tumor microenvironment, but more so of the PMN-MDSC subset, suggesting that the lack of FASLG skews the remaining MDSC population to the M-MDSC, and therefore more immunosuppressive, phenotype (52). Considering that this 9 times reduction in MDSC compared to monocytes occurred within the PB suggests that circulating MDSC lack the FasL-Fas immunosuppressive mechanism, which was supported by our data in Table 5. This demonstrated that the expression of FASLG on MDSC was 20 times lower in PB compared to BALF. CPT1A is a mitochondrial enzyme involved in fatty acid metabolism, the lipid metabolic state preferred by MDSC to which their immunosuppressive potentials can be attributed (53, 54). The upregulation of this gene was not unexpected as it is in line with immune-oncology findings, but it is promising that these findings are similar between cancer and TB.
In contrast to the abundance of differentially expressed genes between MDSC and monocytes from PB, we identified only 6 genes that were differentially expressed between MDSC and AM isolated from the BALF of active TB patients. These included CAV1, CD36, IL10, IL1B, IL6, and MIR146A. Physiologically, the suppressive function of MDSC is likely to feature most notably at the inflammation site, which in cancer is at the tumor site, or in the case of TB, the lung. We have demonstrated that lung-derived MDSC and macrophages from active TB patients do indeed display differential enrichment in selected gene transcripts, which could inform the immunosuppressive mechanisms of lung-derived MDSC during active TB disease. IL-6, IL-10 and IL1-β are well known cytokines involved in the induction/expansion of MDSC, with IL-10 also playing a role in their effector functions at the site of disease (44, 55–58). Of interest is the observed downregulation of CAV1 and CD36, both of which are involved in lipid metabolism and metabolic reprogramming of MDSC, and mycobacterial infections, and are associated with poor clinical outcomes in malignant tumors (59, 60). Previous mycobacterium-specific research demonstrated that vesicular TLR2/Cav-1 signalling, while dispensable for Mycobacterium bovis internalization specifically, is required for T cell suppressive functions within the MDSC milieu (61). Five out of the six genes were less differentially expressed in the MDSC population compared to the AM, suggesting that AM are responsible for the expression of these genes, including IL-10, possibly to induce the expansion of MDSC at the site of disease, while utilising CAV1/CD36-driven lipid metabolism pathways to create a niche for mycobacterial survival (62, 63), thereby exacerbating disease progression.
The fate of circulating MDSC in active TB patients has not been determined, but the theory is that MDSC released from the bone marrow migrate via the blood stream to the site of infection in the lung. How the transcriptional profile of circulating MDSC compares to MDSC entering the disease site, therefore remains to be determined and is of importance to future HDT strategies. Our comparison of gene expression levels in MDSC from PB vs. BALF showed that, at least for the 10 genes listed in Table 5, the gene expression pattern observed in PB does not reflect levels seen in the lung. This was confirmed by the observation that there was little to no correlation between the expression levels in MDSC from the two sites for all genes (Supplementary Table 1), as well as the high expression factor differences between the two compartments. It is clear from this data that there may be some phenotype and functional profile differences depending on the anatomical compartment from which MDSC were derived. Unfortunately, though, hierarchical cluster analysis demonstrated that, at least during this study, the gene expression profiles of MDSC in PB compared to BALF could not discriminate patients with active TB from those with OLD. Of note from this comparison is the observation of OLR1 being lower by a factor of 180.9 in MDSC in PB compared to BALF. OLR1 codes for the lectin-type oxidized low-density lipoprotein receptor 1 (also known as LOX-1), and is highly expressed in PMN-MDSC (27). With this in mind, previous studies suggesting M-MDSC are the dominant subset of MDSC within the PB compartment are supported, further suggesting the PMN-MDSC subset may dominate the lung compartment, but this needs to be validated.
A significant limitation of this study is that it was unfortunately not possible to obtain paired BALF and PB samples from the same patients which would have enhanced the power of this study. Working with clinical samples is known to be challenging in the TB field; this is especially true for the collection of BALF samples as the bronchoscopy procedure is more invasive and uncomfortable than venepuncture. Often, paired blood specimens cannot be collected in tandem, and additionally, it is likely that the inability to discriminate gene expression profiles of MDSC between other cells or anatomical compartments, is as a result of the small sample size available during this study. A follow-up study is currently being conducted by our research group making use of paired samples from a larger number of participants to validate the findings observed during this study. In addition, our sample size for the comparative OLD group was incredibly small and each individual presented with a distinct diagnosis. This is problematic for representative sampling and should be considered when interpreting the results obtained during this study. Future studies may do well to focus on obtaining patient samples specifically from those with lung cancer to draw stronger parallels between the TB and OLD group. Lastly, this study would benefit from additional validation studies with a particular need being the use of a larger cohort with paired samples.
The results achieved from this study support the hypothesis that anatomical compartments may drive compartment-specific immunological responses, which have considerable effects on the MDSC population and their immunosuppressive functions specifically. The most interesting findings are that the gene expression profiles of MDSC and/or monocytes from PB are able to discriminate between patients with active TB disease and OLD, and that differential gene expression in MDSC vs monocytes is highly variable compared to differential gene expression in MDSC vs AM, with the lung compartment demonstrating a preferential trend to upregulate genes involved in MDSC expansion and lipid metabolism. Considering that the gene expression profiles of MDSC in PB and BALF are not capable of clustering according to disease groups (TB or OLD), it is feasible to speculate that MDSC from TB share similar gene expression profiles with those from cancer but needs to be validated in a larger study. These are important observations for the TB field, possibly lending support to repurpose and validate cancer immunotherapies as HDT for active TB disease. This study provides a backbone upon which future studies into MDSC-related gene expression during active TB disease should be based, as a guide for both improvement and targeted investigations.
Data Availability Statement
The data presented in the study are deposited in the GEO repository, accession number GSE197113 (https://www.ncbi.nlm.nih.gov/geo/query/acc.cgi?acc=GSE197113).
Ethics Statement
The studies involving human participants were reviewed and approved by Health Research Ethics Committee of Stellenbosch University. The patients/participants provided their written informed consent to participate in this study.
Author Contributions
This study was conceived by NP and designed with support from GS and GW. BL, MM, and ME conducted the experiments and analysed the data with support from AP-N, GS, and TS. LK, GS, and NP wrote the manuscript with comments from GW. SA and STM were responsible for participant recruitment, while BA and CK were responsible for the bronchoscopies performed to acquire the BAL fluid. All authors contributed to the article and approved the submitted version.
Funding
This work was supported by the National Institute of Health (NIH) International Collaborations in Infectious Disease Research (ICIDR): Biology and Biosignatures of anti-TB Treatment Response (U01 AI115619), European & Developing Countries Clinical Trials Partnership (EDCTP; CDF1546), and South African Research Chair Initiative (SARChI): Biomarkers for TB (SANRF86535).
Conflict of Interest
The authors declare that the research was conducted in the absence of any commercial or financial relationships that could be construed as a potential conflict of interest.
Publisher’s Note
All claims expressed in this article are solely those of the authors and do not necessarily represent those of their affiliated organizations, or those of the publisher, the editors and the reviewers. Any product that may be evaluated in this article, or claim that may be made by its manufacturer, is not guaranteed or endorsed by the publisher.
Acknowledgments
The authors would like to acknowledge the participants who gave consent for their specimens to be used in this study and greatly acknowledge the clinicians, nurses and staff at the clinicians, nurses and staff at the Molecular Biology Clinical Research Unit at Stellenbosch University, the Division of Pulmonology, Department of Medicine, Stellenbosch University, and Tygerberg Academic Hospital for their contribution to this study.
Supplementary Material
The Supplementary Material for this article can be found online at: https://www.frontiersin.org/articles/10.3389/fimmu.2022.839747/full#supplementary-material
Supplementary Figure 1 | ΔCt-values for all 43-genes assessed for differential expression between MDSC and monocytes in the PB of active TB patients. Results are given for the 16X (pink) and 20X (blue) pre-amplification cycles.
Supplementary Figure 2 | ΔCt-values for all 43-genes assessed for differential expression between MDSC and AM in the BALF of active TB patients. Results are given for the 16X (pink) and 20X (blue) pre-amplification cycles.
Supplementary Figure 3 | ΔCt-values for all 43-genes assessed for differential expression between MDSC in the PB and BALF of active TB patients. Results are given for the 16X (pink) and 20X (blue) pre-amplification cycles.
References
1. World Health Organization. Global Tuberculosis Report 2021. Geneva: World Health Organization (2021). Available at: https://apps.who.int/iris/handle/10665/346387.
2. Darboe F, Mbandi SK, Thompson EG, Fisher M, Rodo M, van Rooyen M, et al. Diagnostic Performance of an Optimized Transcriptomic Signature of Risk of Tuberculosis in Cryopreserved Peripheral Blood Mononuclear Cells. Tuberculosis (Edinburgh Scotland) (2018) 108:124–26. doi: 10.1016/j.tube.2017.11.001
3. Flynn JL, Chan J. Immunology of Tuberculosis. Annu Rev Immunol (2001) 19:93–129. doi: 10.1146/annurev.immunol.19.1.93
4. North RJ, Jung Y-J. Immunity to Tuberculosis. Annu Rev Immunol (2004) 22(1):599–6235. doi: 10.1146/annurev.immunol.22.012703.104635
5. Medina E, Hartl D. Myeloid-Derived Suppressor Cells in Infection: A General Overview. J Innate Immun (2018) 10(5–6):407–13. doi: 10.1159/000489830
6. Peranzoni E, Zilio S, Marigo I, Dolcetti L, Zanovello P, Mandruzzato S, et al. Myeloid-Derived Suppressor Cell Heterogeneity and Subset Definition. Curr Opin Immunol (2010) 22(2):238–445. doi: 10.1016/j.coi.2010.01.021
7. Gabrilovich DI, Ostrand-Rosenberg S, Bronte V. Coordinated Regulation of Myeloid Cells by Tumours. Nat Rev Immunol (2012) 12(4):253–685. doi: 10.1038/nri3175
8. Kim HR, Park S-M, Seo S-U, Jung I, Yoon HI, Gabrilovich DI, et al. The Ratio of Peripheral Regulatory T Cells to Lox-1+ Polymorphonuclear Myeloid-Derived Suppressor Cells Predicts the Early Response to Anti-PD-1 Therapy in Patients With Non-Small Cell Lung Cancer. Am J Respir Crit Care Med (2019) 199(2):243–465. doi: 10.1164/rccm.201808-1502LE
9. Goldmann O, Beineke A, Medina E. Identification of a Novel Subset of Myeloid-Derived Suppressor Cells During Chronic Staphylococcal Infection That Resembles Immature Eosinophils. J Infect Dis (2017) 216(11):1444–515. doi: 10.1093/infdis/jix494
10. Srivastava MK, Sinha P, Clements VK, Rodriguez P, Ostrand-Rosenberg S. Myeloid-Derived Suppressor Cells Inhibit T-Cell Activation by Depleting Cystine and Cysteine. Cancer Res (2010) 70(1):68–775. doi: 10.1158/0008-5472.CAN-09-2587
11. Gabrilovich DI. Myeloid-Derived Suppressor Cells. Cancer Immunol Res (2017) 5(1):3–8. doi: 10.1158/2326-6066.CIR-16-0297
12. Uhel F, Azzaoui I, Grégoire M, Pangault C, Dulong J, Tadié J-M, et al. Early Expansion of Circulating Granulocytic Myeloid-Derived Suppressor Cells Predicts Development of Nosocomial Infections in Patients With Sepsis. Am J Respir Crit Care Med (2017) 196(3):315–27. doi: 10.1164/rccm.201606-1143OC
13. Groth C, Hu X, Weber R, Fleming V, Altevogt P, Utikal J, et al. Immunosuppression Mediated by Myeloid-Derived Suppressor Cells (MDSCs) During Tumour Progression. Br J Cancer (2019) 120(1):16–255. doi: 10.1038/s41416-018-0333-1
14. Talmadge JE. Pathways Mediating the Expansion and Immunosuppressive Activity of Myeloid-Derived Suppressor Cells and Their Relevance to Cancer Therapy. Clin Cancer Res (2007) 13(18 Pt 1):5243–48. doi: 10.1158/1078-0432.CCR-07-0182
15. Marigo I, Dolcetti L, Serafini P, Zanovello P, Bronte V. Tumor-Induced Tolerance and Immune Suppression by Myeloid Derived Suppressor Cells. Immunol Rev (2008) 222(1):162–795. doi: 10.1111/j.1600-065X.2008.00602.x
16. Murdoch C, Muthana M, Coffelt SB, Lewis CE. The Role of Myeloid Cells in the Promotion of Tumour Angiogenesis. Nat Rev Cancer (2008) 8(8):618–315. doi: 10.1038/nrc2444
17. Wang P-F, Song S-Y, Wang T-J, Ji W-J, Li S-W, Liu N, et al. Prognostic Role of Pretreatment Circulating MDSCs in Patients With Solid Malignancies: A Meta-Analysis of 40 Studies. Oncoimmunology (2018) 7(10):e14941135. doi: 10.1080/2162402X.2018.1494113
18. Gabrilovich DI, Nagaraj S. Myeloid-Derived Suppressor Cells as Regulators of the Immune System. Nat Rev Immunol (2009) 9(3):162–745. doi: 10.1038/nri2506
19. Young M, Rita I, Wright MA, Lozano Y, Matthews JP, Benefield J, et al. Mechanisms of Immune Suppression in Patients With Head and Neck Cancer: Influence on the Immune Infiltrate of the Cancer. Int J Cancer (1996) 67(3):333–85. doi: 10.1002/(SICI)1097-0215(19960729)67:3<333::AID-IJC5>3.0.CO;2-S
20. Du Plessis N, Loebenberg L, Kriel M, von Groote-Bidlingmaier F, Ribechini E, Loxton AG, et al. Increased Frequency of Myeloid-Derived Suppressor Cells During Active Tuberculosis and After Recent Mycobacterium Tuberculosis Infection Suppresses T-Cell Function. Am J Respir Crit Care Med (2013) 188(6):724–325. doi: 10.1164/rccm.201302-0249OC
21. El Daker S, Sacchi A, Tempestilli M, Carducci C, Goletti D, Vanini V, et al. Granulocytic Myeloid Derived Suppressor Cells Expansion During Active Pulmonary Tuberculosis Is Associated With High Nitric Oxide Plasma Level. PLoS One (2015) 10(4):e01237725. doi: 10.1371/journal.pone.0123772
22. Davids M, Pooran A, Smith L, Tomasicchio M, Dheda K. The Frequency and Effect of Granulocytic Myeloid-Derived Suppressor Cells on Mycobacterial Survival in Patients With Tuberculosis: A Preliminary Report. Front Immunol (2021) 12:676679. doi: 10.3389/fimmu.2021.676679
23. Dorhoi A, Du Plessis N. Monocytic Myeloid-Derived Suppressor Cells in Chronic Infections. Front Immunol (2017) 8:1895. doi: 10.3389/fimmu.2017.01895
24. Kotze LA, Leukes VN, Fang Z, Lutz MB, Fitzgerald BL, Belisle J, et al. Evaluation of Autophagy Mediators in Myeloid-Derived Suppressor Cells During Human Tuberculosis. Cell Immunol (2021) 369:104426. doi: 10.1016/j.cellimm.2021.104426
25. Bronte V, Brandau S, Chen S-H, Colombo MP, Frey AB, Greten TF, et al. Recommendations for Myeloid-Derived Suppressor Cell Nomenclature and Characterization Standards. Nat Commun (2016) 7:12150. doi: 10.1038/ncomms12150
26. Bergenfelz C, Larsson A-M, von Stedingk K, Gruvberger-Saal S, Aaltonen K, Jansson S, et al. Systemic Monocytic-MDSCs Are Generated From Monocytes and Correlate With Disease Progression in Breast Cancer Patients. PloS One (2015) 10(5):e0127028. doi: 10.1371/journal.pone.0127028
27. Condamine T, Dominguez GA, Youn J-I, Kossenkov AV, Mony S, Alicea-Torres K, et al. Lectin-Type Oxidized LDL Receptor-1 Distinguishes Population of Human Polymorphonuclear Myeloid-Derived Suppressor Cells in Cancer Patients. Sci Immunol (2016) 1(2):aaf8943. doi: 10.1126/sciimmunol.aaf8943
28. Hegde S, Leader AM, Merad M. MDSC: Markers, Development, States, and Unaddressed Complexity. Immunity (2021) 54(5):875–45. doi: 10.1016/j.immuni.2021.04.004
29. R Core Team (2020). Methodology Reference. 2020 (2020). Available at: https://www.r-project.org/.
30. Carpenter B, Gelman A, Hoffman MD, Lee D, Goodrich B, Betancourt M, et al. Stan: A Probabilistic Programming Language. J Stat Softw (2017) 76(January):1–32. doi: 10.18637/jss.v076.i01
31. Bürkner P-C. Advanced Bayesian Multilevel Modeling With the R Package Brms. R J (2018) 10(1):395. doi: 10.32614/RJ-2018-017
32. Young C, Ahlers P, Hiemstra AM, Loxton AG, Gutschmidt A, Malherbe ST, et al. Performance and Immune Characteristics of Bronchoalveolar Lavage by Research Bronchoscopy in Pulmonary Tuberculosis and Other Lung Diseases in the Western Cape, South Africa. Trans Med Commun (2019) 4(1):7. doi: 10.1186/s41231-019-0039-2
33. Heron M, Grutters JC, ten Dam-Molenkamp KM, Hijdra D, van Heugten-Roeling A, Claessen AME, et al. Bronchoalveolar Lavage Cell Pattern From Healthy Human Lung. Clin Exp Immunol (2012) 167(3):523–31. doi: 10.1111/j.1365-2249.2011.04529.x
34. Morales-Nebreda L, Misharin AV, Perlman H, Budinger GRS. The Heterogeneity of Lung Macrophages in the Susceptibility to Disease. Eur Respir Rev (2015) 24(137):505–95. doi: 10.1183/16000617.0031-2015
35. Vandesompele J, De Preter K, Pattyn F, Poppe B, Van Roy N, De Paepe A, et al. Accurate Normalization of Real-Time Quantitative RT-PCR Data by Geometric Averaging of Multiple Internal Control Genes. Genome Biol (2002) 3(7):research0034.1. doi: 10.1186/gb-2002-3-7-research0034
36. Beury DW, Carter KA, Nelson C, Sinha P, Hanson E, Nyandjo M, et al. Myeloid-Derived Suppressor Cell Survival and Function Are Regulated by the Transcription Factor Nrf2. J Immunol (Baltimore Md: 1950) (2016) 196(8):3470–785. doi: 10.4049/jimmunol.1501785
37. Veglia F, Sanseviero E, Gabrilovich DI. Myeloid-Derived Suppressor Cells in the Era of Increasing Myeloid Cell Diversity. Nat Rev Immunol (2021) 21(8):485–985. doi: 10.1038/s41577-020-00490-y
38. Silver RF, Myers AJ, Jarvela J, Flynn J, Rutledge T, Bonfield T, et al. Diversity of Human and Macaque Airway Immune Cells at Baseline and During Tuberculosis Infection. Am J Respir Cell Mol Biol (2016) 55(6):899–9085. doi: 10.1165/rcmb.2016-0122OC
39. Du Plessis N, Jacobs R, Gutschmidt A, Fang Z, van Helden PD, Lutz MB, et al. Phenotypically Resembling Myeloid Derived Suppressor Cells Are Increased in Children With HIV and Exposed/Infected With Mycobacterium Tuberculosis. Eur J Immunol (2017) 47(1):107–85. doi: 10.1002/eji.201646658
40. Leukes VN, Dorhoi A, Malherbe ST, Maasdorp E, Khoury J, McAnda S, et al. Targeting of Myeloid-Derived Suppressor Cells by All-Trans Retinoic Acid as Host-Directed Therapy for Human Tuberculosis. Cell Immunol (2021) 364(June):104359. doi: 10.1016/j.cellimm.2021.104359
41. Law AMK, Valdes-Mora F, Gallego-Ortega D. Myeloid-Derived Suppressor Cells as a Therapeutic Target for Cancer. Cells (2020) 9(3):5615. doi: 10.3390/cells9030561
42. Ugel S, De Sanctis F, Mandruzzato S, Bronte V. Tumor-Induced Myeloid Deviation: When Myeloid-Derived Suppressor Cells Meet Tumor-Associated Macrophages. J Clin Invest (2015) 125(9):3365–765. doi: 10.1172/JCI80006
43. Trellakis S, Bruderek K, Hütte J, Elian M, Hoffmann TK, Lang S, et al. Granulocytic Myeloid-Derived Suppressor Cells Are Cryosensitive and Their Frequency Does Not Correlate With Serum Concentrations of Colony-Stimulating Factors in Head and Neck Cancer. Innate Immun (2013) 19(3):328–65. doi: 10.1177/1753425912463618
44. Knaul JK, Jörg S, Oberbeck-Mueller D, Heinemann E, Scheuermann L, Brinkmann V, et al. Lung-Residing Myeloid-Derived Suppressors Display Dual Functionality in Murine Pulmonary Tuberculosis. Am J Respir Crit Care Med (2014) 190(9):1053–665. doi: 10.1164/rccm.201405-0828OC
45. Ribechini E, Eckert I, Beilhack A, Du Plessis N, Walzl G, Schleicher U, et al. Heat-Killed Mycobacterium Tuberculosis Prime-Boost Vaccination Induces Myeloid-Derived Suppressor Cells With Spleen Dendritic Cell-Killing Capability. JCI Insight (2019) 5(June):128664. doi: 10.1172/jci.insight.128664
46. Rodriguez PC, Ochoa AC, Al-Khami AA. Arginine Metabolism in Myeloid Cells Shapes Innate and Adaptive Immunity. Front Immunol (2017) 8:93. doi: 10.3389/fimmu.2017.00093
47. Ortiz ML, Kumar V, Martner A, Mony S, Donthireddy L, Condamine T, et al. Immature Myeloid Cells Directly Contribute to Skin Tumor Development by Recruiting IL-17–Producing CD4+ T Cells. J Exp Med (2015) 212(3):351–67. doi: 10.1084/jem.20140835
48. Segueni N, Tritto E, Bourigault M-L, Rose S, Erard F, Le Bert M, et al. Controlled Mycobacterium Tuberculosis Infection in Mice Under Treatment With Anti-IL-17A or IL-17f Antibodies, in Contrast to TNFα Neutralization. Sci Rep (2016) 6(November):36923. doi: 10.1038/srep36923
49. Zhu X, Mulcahy LA, Mohammed RAA, Lee AHS, Franks HA, Kilpatrick L, et al. IL-17 Expression by Breast-Cancer-Associated Macrophages: IL-17 Promotes Invasiveness of Breast Cancer Cell Lines. Breast Cancer Res (2008) 10(6):R95. doi: 10.1186/bcr2195
50. Mohammadpour H, MacDonald CR, Qiao G, Chen M, Dong B, Hylander BL, et al. B2 Adrenergic Receptor–Mediated Signaling Regulates the Immunosuppressive Potential of Myeloid-Derived Suppressor Cells. J Clin Invest (2019) 129(12):5537–525. doi: 10.1172/JCI129502
51. Zhu J, Powis de Tenbossche CG, Cané S, Colau D, van Baren N, Lurquin C, et al. Resistance to Cancer Immunotherapy Mediated by Apoptosis of Tumor-Infiltrating Lymphocytes. Nat Commun (2017) 8(1):14045. doi: 10.1038/s41467-017-00784-1
52. Peyvandi S, Buart S, Samah B, Vétizou M, Zhang Y, Durrieu L, et al. Fas Ligand Deficiency Impairs Tumor Immunity by Promoting an Accumulation of Monocytic Myeloid-Derived Suppressor Cells. Cancer Res (2015) 75(20):4292–301. doi: 10.1158/0008-5472.CAN-14-1848
53. Wu C, Tan X, Hu X, Zhou M, Yan J, Ding C. Tumor Microenvironment Following Gemcitabine Treatment Favors Differentiation of Immunosuppressive Ly6Chigh Myeloid Cells. J Immunol (2020) 204(1):212–35. doi: 10.4049/jimmunol.1900930
54. Yan D, Adeshakin AO, Xu M, Afolabi LO, Zhang G, Chen YH, et al. Lipid Metabolic Pathways Confer the Immunosuppressive Function of Myeloid-Derived Suppressor Cells in Tumor. Front Immunol (2019) 10:1399(June):1399. doi: 10.3389/fimmu.2019.01399
55. Agrawal N, Streata I, Pei G, Weiner J, Kotze L, Bandermann S, et al. Human Monocytic Suppressive Cells Promote Replication of Mycobacterium Tuberculosis and Alter Stability of In Vitro Generated Granulomas. Front Immunol (2018) 9:2417(October). doi: 10.3389/fimmu.2018.02417
56. Shipp C, Speigl L, Janssen N, Martens A, Pawelec G. A Clinical and Biological Perspective of Human Myeloid-Derived Suppressor Cells in Cancer. Cell Mol Life Sci (2016) 73(21):4043–615. doi: 10.1007/s00018-016-2278-y
57. Mayer-Barber KD, Andrade BB, Barber DL, Hieny S, Feng CG, Caspar P, et al. Innate and Adaptive Interferons Suppress IL-1α and IL-1β Production by Distinct Pulmonary Myeloid Subsets During Mycobacterium Tuberculosis Infection. Immunity (2011) 35(6):1023–345. doi: 10.1016/j.immuni.2011.12.002
58. Sumida K, Wakita D, Narita Y, Masuko K, Terada S, Watanabe K, et al. Anti-IL-6 Receptor MAb Eliminates Myeloid-Derived Suppressor Cells and Inhibits Tumor Growth by Enhancing T-Cell Responses. Eur J Immunol (2012) 42(8):2060–725. doi: 10.1002/eji.201142335
59. Zaas DW, Duncan M, Rae Wright J, Abraham SN. The Role of Lipid Rafts in the Pathogenesis of Bacterial Infections. Biochim Biophys Acta (BBA) - Mol Cell Res (2005) 1746(3):305–13. doi: 10.1016/j.bbamcr.2005.10.003. Lipid Rafts: From Model Membranes to Cells.
60. Martinez-Outschoorn UE, Sotgia F, Lisanti MP. Caveolae and Signalling in Cancer. Nat Rev Cancer (2015) 15(4):225–375. doi: 10.1038/nrc3915
61. John V, Kotze LA, Ribechini E, Walzl G, Du Plessis N, Lutz MB. Caveolin-1 Controls Vesicular TLR2 Expression, P38 Signaling and T Cell Suppression in BCG Infected Murine Monocytic Myeloid-Derived Suppressor Cells. Front Immunol (2019) 10:2826. doi: 10.3389/fimmu.2019.02826
62. Kotzé LA, Young C, Leukes VN, John V, Fang Z, Walzl G, et al. Mycobacterium Tuberculosis and Myeloid-Derived Suppressor Cells: Insights Into Caveolin Rich Lipid Rafts. EBioMedicine (2020) 53(March):102670. doi: 10.1016/j.ebiom.2020.102670
Keywords: myeloid derived suppressor cells, alveolar macrophages, monocytes, tuberculosis, lung disease, bronchoalveolar lavage
Citation: Kotze LA, van der Spuy G, Leonard B, Penn-Nicholson A, Musvosvi M, McAnda S, Malherbe ST, Erasmus M, Scriba T, Koegelenberg CFN, Allwood BW, Walzl G and du Plessis N (2022) Targeted Gene Expression Profiling of Human Myeloid Cells From Blood and Lung Compartments of Patients With Tuberculosis and Other Lung Diseases. Front. Immunol. 13:839747. doi: 10.3389/fimmu.2022.839747
Received: 20 December 2021; Accepted: 08 February 2022;
Published: 08 March 2022.
Edited by:
Maziar Divangahi, McGill University, CanadaReviewed by:
Andrew J. Olive, Michigan State University, United StatesBabak Javid, University of California, San Francisco, United States
Copyright © 2022 Kotze, van der Spuy, Leonard, Penn-Nicholson, Musvosvi, McAnda, Malherbe, Erasmus, Scriba, Koegelenberg, Allwood, Walzl and du Plessis. This is an open-access article distributed under the terms of the Creative Commons Attribution License (CC BY). The use, distribution or reproduction in other forums is permitted, provided the original author(s) and the copyright owner(s) are credited and that the original publication in this journal is cited, in accordance with accepted academic practice. No use, distribution or reproduction is permitted which does not comply with these terms.
*Correspondence: Nelita du Plessis, bmVsaXRhQHN1bi5hYy56YQ==
†These authors have contributed equally to this work and share first authorship