- 1Nephrology Department, First Teaching Hospital of Tianjin University of Traditional Chinese Medicine, Tianjin University of Traditional Chinese Medicine, Tianjin, China
- 2Graduate School, Tianjin University of Traditional Chinese Medicine, Tianjin, China
- 3Institute of Traditional Chinese Medicine, Tianjin University of Traditional Chinese Medicine, Tianjin, China
- 4Nephrology Department, The First Affiliated Hospital of Henan University of Chinese Medicine, Henan, China
Objective: To identify potential diagnostic markers of lupus nephritis (LN) based on bioinformatics and machine learning and to explore the significance of immune cell infiltration in this pathology.
Methods: Seven LN gene expression datasets were downloaded from the GEO database, and the larger sample size was used as the training group to obtain differential genes (DEGs) between LN and healthy controls, and to perform gene function, disease ontology (DO), and gene set enrichment analyses (GSEA). Two machine learning algorithms, least absolute shrinkage and selection operator (LASSO) and support vector machine-recursive feature elimination (SVM-RFE), were applied to identify candidate biomarkers. The diagnostic value of LN diagnostic gene biomarkers was further evaluated in the area under the ROC curve observed in the validation dataset. CIBERSORT was used to analyze 22 immune cell fractions from LN patients and to analyze their correlation with diagnostic markers.
Results: Thirty and twenty-one DEGs were screened in kidney tissue and peripheral blood, respectively. Both of which covered macrophages and interferons. The disease enrichment analysis of DEGs in kidney tissues showed that they were mainly involved in immune and renal diseases, and in peripheral blood it was mainly enriched in cardiovascular system, bone marrow, and oral cavity. The machine learning algorithm combined with external dataset validation revealed that C1QA(AUC = 0.741), C1QB(AUC = 0.758), MX1(AUC = 0.865), RORC(AUC = 0.911), CD177(AUC = 0.855), DEFA4(AUC= 0.843)and HERC5(AUC = 0.880) had high diagnostic value and could be used as diagnostic biomarkers of LN. Compared to controls, pathways such as cell adhesion molecule cam, and systemic lupus erythematosus were activated in kidney tissues; cell cycle, cytoplasmic DNA sensing pathways, NOD-like receptor signaling pathways, proteasome, and RIG-1-like receptors were activated in peripheral blood. Immune cell infiltration analysis showed that diagnostic markers in kidney tissue were associated with T cells CD8 and Dendritic cells resting, and in blood were associated with T cells CD4 memory resting, suggesting that CD4 T cells, CD8 T cells and dendritic cells are closely related to the development and progression of LN.
Conclusion: C1QA, C1QB, MX1, RORC, CD177, DEFA4 and HERC5 could be used as new candidate molecular markers for LN. It may provide new insights into the diagnosis and molecular treatment of LN in the future.
1. Introduction
Lupus nephritis (LN) is one of the most common and serious complications of systemic lupus erythematosus (SLE) (1), with a high morbidity and mortality rate. According to statistics, the annual incidence of SLE worldwide is 1/100,000 to 8.7/100,000, and 40% to 60% of SLE patients have LN at onset (1). About one-third of patients with severe LN develop end-stage renal disease (ESRD) after 10 years. The mortality rate is increased 8-fold compared to the general population (2, 3). Given LN’s high morbidity and mortality, early diagnosis and intervention can significantly reduce CKD and ESRD (4). Kidney biopsy remains the gold standard for diagnosing LN but is somewhat invasive. It is also considered that SLE is a systemic autoimmune disease with multi-organ involvement and multiple autoantibody positivity as the primary clinical features, while peripheral blood is prevalent throughout the body and can reflect systemic activity (5, 6). In addition, peripheral blood contains a variety of LN-associated immune cells, easy accessibility, low cost of the examination, and accurate results, making gene expression analysis of peripheral blood cells an ideal source of LN biomarkers (7). For treatment, LN is usually treated with immunosuppressive therapy such as mycophenolate mofetil or cyclophosphamide and glucocorticoids. On the one hand, they are not specific drugs for the disease and are closely associated with a wide range of adverse effects (8). On the other hand, difficulty adhering to treatment due to potential adverse effects may lead to treatment failure and progression to refractory LN (9, 10). Therefore, it is imperative to develop new candidate biomarkers and find potential therapeutic targets to improve the prognosis of LN patients.
With the development of gene microarray technology and high-throughput technology, the use of bioinformatics methods to mine gene microarray data can quickly and effectively screen differential genes(DEGs). In recent years, it has been widely used in the elucidation of the pathogenic mechanism of diseases and screening of drug therapeutic targets (11–13). Both SLE and LN are largely influenced by genes (14). Therefore, in this study, we used bioinformatics methods to obtain RNA gene matrices from peripheral blood and kidney tissues of LN, respectively, from the GEO database, and performed DEGs enrichment analysis between LN and healthy samples. Two machine learning algorithms were used to screen biomarkers associated with LN, and candidate genes closely related to immune infiltration were further validated in another validation cohort. In this study, CIBERSORT was used for the first time to quantify the proportion of immune cells in LN and normal tissue samples based on gene expression profiles. In addition, we explored the relationship between the identified biomarkers and infiltrating immune cells to provide new ideas for the further prevention and treatment of LNs.
2. Materials and methods
2.1. Microarray data
In the Gene Expression Omnibus (GEO) database (https://www.ncbi.nlm.nih.gov/geo/), use “Lupus nephritis” as the search term, limit entry type to series, and detection method/data type to expression profiling by array, the organization source is homo sapiens, and all gene expression data related to LNs were retrieved as of October 3rd, 2022. The data sets were differentiated for tissue origin: peripheral blood and kidney tissue. Three eligible gene expression datasets were screened in the renal group: GSE32591 (64 LN, 29 healthy controls), GSE113342 (56 LN, 16 healthy controls), and GSE200306 (79 LN, 19 healthy controls), enrolling a total of 199 patients with LN and 64 healthy controls. Three eligible gene expression data sets were screened in the peripheral blood group: (GSE72798 (30 LN, 17 healthy controls), GSE99967 (29 LN, 17 controls), and GSE81622 (14 LN, 24 controls)’s, which included a total of 73 LN patients and 58 healthy controls.
2.2. Data processing and DEG screening
Firstly, convert the probe matrix into a gene matrix according to the probe annotation file. For multiple probes corresponding to the same gene, the average value of the probes is calculated as the final expression value of the gene. Secondly, the two data sets with larger sample size were pooled and used as training groups, GSE99967, GSE72798 and GSE32591, GSE113342 for peripheral blood and kidney tissues respectively, and GSE81622 and GSE200306 for the validation group. The sva package was used to process the batch effect, the limma package was used to analyze theDEGs in the matrix, and the DEGs were selected with the gene expression | log2 fold change, logFC | > 1 and the adjusted P value <0.05 as the screening conditions. Finally, ggplot was used to visualize the DEGs and plot the corresponding volcanoe map.
2.3. Functional enrichment analysis of DEGs
The “clusterProfiler” and “DOSE” packages of the R package were used to perform disease ontology (DO) enrichment analysis of DEGs. And gene set enrichment analysis (GSEA) was also used to identify significant pathways between the LN and control groups. The “c2.cp.kegg.v7.4.symbols.gmt” from the Molecular Signatures Database (MSigDB) was used as the reference gene set. If p. adjust < 0.05, the pathways were considered significantly enriched.
2.4. Screening of diagnostic biomarkers
Machine learning is a novel algorithm analysis tool, and in this study, the least absolute shrinkage and selection operator (LASSO) combined with support vector machine-recursive feature elimination (SVM-RFE) was applied to the screening of LN biomarkers to predict the status of the disease. LASSO is a regularized regression algorithm using the “glmnet” package in R (15). SVM-RFE is a supervised machine learning technique that can rank features based on recursion to avoid overfitting (16, 17). The genes screened by the two algorithms are intersected and the overlapping genes are the candidate bio-diagnostic markers.
2.5. Diagnostic value of biodiagnostic markers in LN
In order to test the accuracy of the biodiagnostic markers screened by machine learning, we generated ROC curves using the “diff gene xp” between the LN group and the normal sample group. The greater the area under curve (AUC), the higher the accuracy of the gene as a diagnostic marker in LN. In the same method, its effectiveness was further verified in the validation group GSE81622/GSE200306.
2.6. Identification of immune cell subsets
The CIBERSORT algorithm is a reliable machine learning method based on linear support vector regression (SVR), which is widely used to assess the relative content and dynamic regulatory process of 22 immune cells and is superior to other methods for identifying human immune cell phenotypes in terms of noise and unknown mixed content (18, 19). In order to understand the relative proportions of different immune cells in the LN sample, this study used the R language program and linked CIBERSORT to simulate and calculate the transcription feature matrix of 22 immune cells, and the number of calculations was set to 1,000. Based on the P value<0.05, use the “corrplot” package to draw correlation heat maps to visualize the correlation between 22 infiltrating immune cells; use “vioplot” to draw a violin chart to visualize the differences between the immune cells of the LN group and the control group.
2.7. Correlation analysis between bioiagnostic markers and infiltrating immune cells
Correlation analysis between genes and immune cells was performed using Spearman correlation coefficient. For those with p < 0.05, the results were visualized using the “ggplot2” package.
2.8. Correlation of bioiagnostic markers with clinicopathological features
To explore the correlation of candidate gene markers with different pathological types, relapse frequency, renal tubulointerstitial inflammation, complex immune accumulation, and renal function in patients with lupus nephritis based on current relevant literature and the Nephroseq V5 tool (https://nephroseq.org/).
2.9. Statistical analysis
For continuous variables between two groups, t-test was applied if they conformed to normal distribution; Mann-Whitney U-test was used for non-normal distribution. ANOVA was applied for continuous variables between three groups. Pearson’s analysis was used to analyze the correlation between gene expression and immune cell fraction. ROC curve analysis was used to determine the diagnostic performance of the diagnostic indicators identified in the study. All statistical analyses were performed using R software (version 4.1.2) and SPSS (version 27.0) software. All statistical analyses were two-sided with P < 0.05 were regarded statistically significant.
3. Results
3.1. Identification of DEGs in LN
According to the screening criteria adjusted P value <0. 05 and |log fold change(FC)| >1, a total of 30 DEGs were screened in the LN kidney group, of which 25 were significantly up-regulated, including C1QB, MX1, C1QA, IL32, etc.; 5 were significantly down-regulated, including ZBTB16, FKBP5, DEFB1, RORC, EGR1. Volcano map of DEGs (Figure 1A). 21 DEGs were obtained from LN peripheral blood, all significantly up-regulated, volcano map drawn for DEGs (Figure 1B).
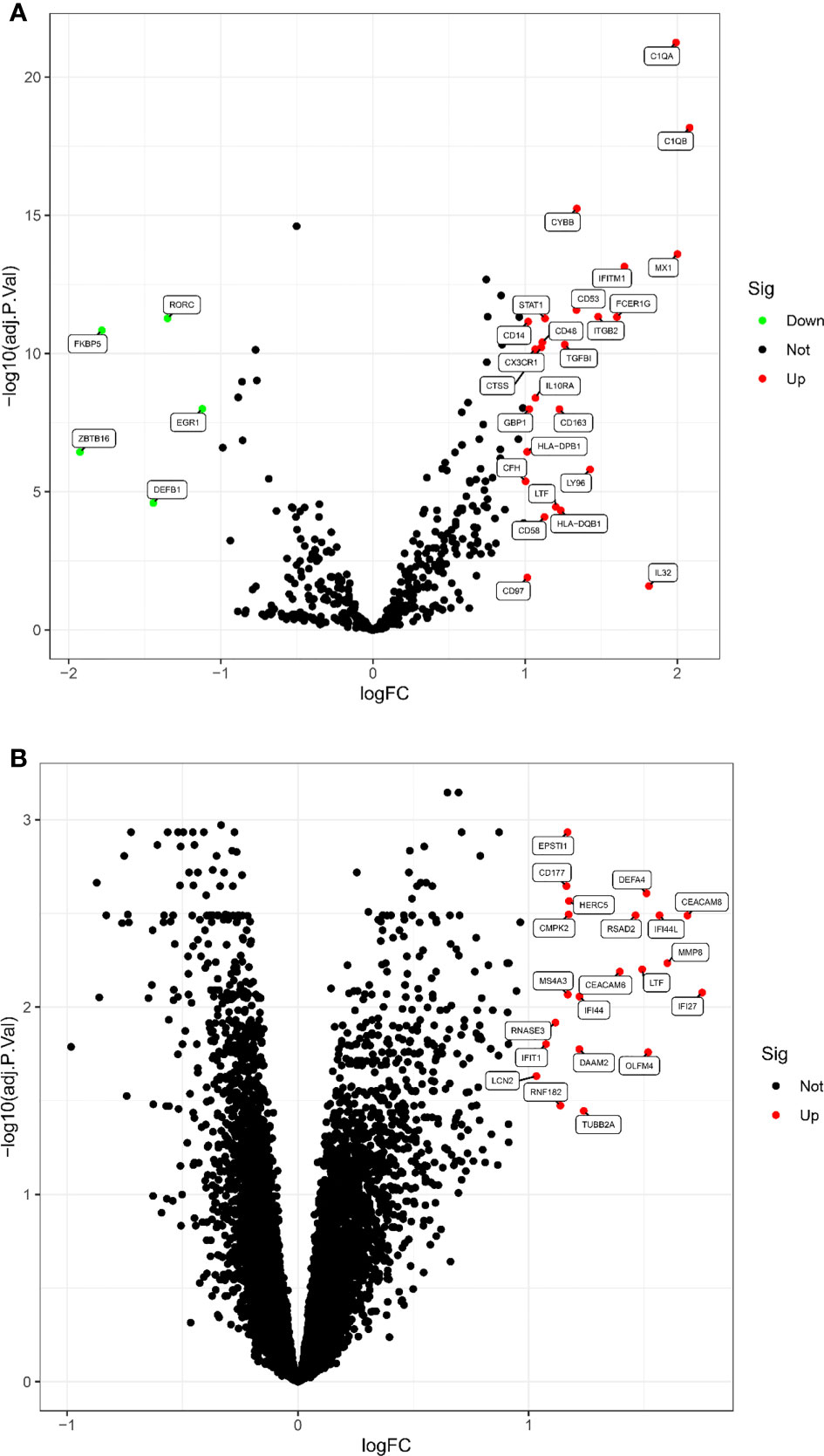
Figure 1 The volcano map of DEGs between LN and healthy samples. (A) Kidney tissue; (B) peripheral blood. Each dot represents a gene. Red dots indicate up-regulated gene expression; green dots indicate down-regulated gene expression; black dots indicate no significant difference between these genes in LN. The horizontal axis indicates the fold difference in gene expression in LN compared to healthy controls, and the vertical axis indicates the significance of the difference (adj. P. Value).
3.2. Functional correlation analysis
3.2.1. Disease enrichment analysis of DEGs
As shown in Figure 2A, the gene ontology disease enrichment (DO) results showed that DEGs in the LN kidney group were mainly enriched in immune class, inflammatory, renal, and cardiovascular diseases with “p values< 0.01”. Examples include primary immunodeficiency disease, vasculitis, and renal failure. DEGs in the LN peripheral blood were mainly enriched in diseases such as thrombocytosis, erythrocytosis, bone marrow diseases, and oral diseases. See Figure 2C.
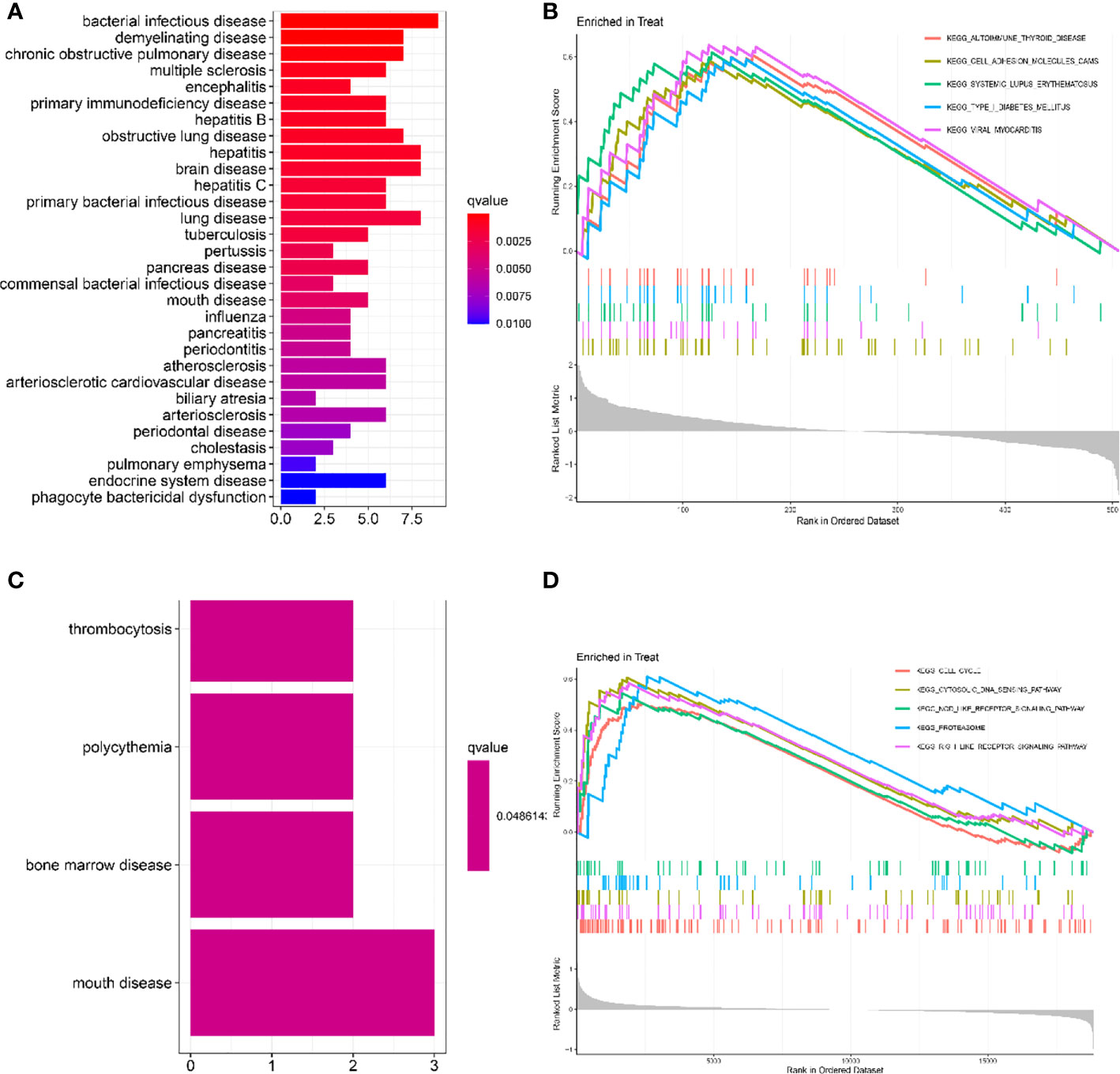
Figure 2 Functional enrichment analysis to identify potential biological processes by DO and GSEA. (A, C) Disease ontology (DO) enrichment analysis of DEGs. The vertical coordinate represents the name of the disease, the horizontal coordinate represents the number of genes enriched on the disease, and the color represents the significance of the enrichment. The redder the color, the more significant DEGs enrichment on the disease. (B, D) Gene set enrichment analysis (GSEA) is used to see which pathways were active in the healthy control or LN group. It consists of three main parts: The first part is the enrichment score line graph: it shows the trend of the cumulative enrichemnt score (ES) value when the analysis calculates the ES value of each gene in the gene set along the ranked list (gene list). The score at the highest peak is the ES value for that gene set. The main focus is on the peaks, with peaks at the top and ES > 0 indicating that they are active in the set; peaks at the bottom and ES < 0 indicating that they are inactive in the set. The five colors represent each of the five pathways (the top 5 in order of corrected p-value). The second part, with lines marking where members of the gene set appear in the gene sorting list, the pathways to which the different colors belong. The third part: the signal-to-noise ratio for each gene is shown as a gray frame plot. The horizontal coordinate represents the ranking in the ordered data set, and the vertical coordinate represents the Running Enrichment Score. (A, B) Kidney tissue; (C, D) Peripheral blood.
3.2.2. Significant gene enrichment pathways active in the test and control groups
In renal tissue, the top 5 significantly enriched pathways in LN compared to healthy controls were: autoimmune thyroid disease, cell adhesion molecule cam, and systemic lupus erythematosus were activated (Figure 2B). In peripheral blood, cell cycle, cytosolic DNA sensing pathway, NOD-like receptor signaling pathway, proteasome, and RIG-1-like receptor signaling pathway were activated in the test group compared to the control group (Figure 2D).
3.3. Diagnostic biomarker identification and validation
A total of two machine learning algorithms, LASSO regression and SVM-RFE, were used to screen biomarkers in this study. In the renal group, LASSO regression identified 16 biomarkers (Figure 3A), SVM-RFE algorithm established 6 biomarkers (Figure 3B), and the overlapping genes C1QA, C1QB, MX1, RORC of both algorithms were used as diagnostic biomarkers (Figure 3C). To further validate the accuracy of the diagnostic biomarkers, their expression levels were further verified in the validation set GSE200306. C1QA, C1QB, MX1, and RORC were expressed at significantly higher levels in LN than in controls (p < 0.05) (Figure 4A–D). In the peripheral blood group, LASSO regression identified 7 biomarkers (Figure 3D) and SVM-RFE algorithm established 4 biomarkers (Figure 3E). Eventually, three overlapping genes CD177, DEFA4, and HERC5 from both algorithms were taken as diagnostic biomarkers (Figure 3F). To further validate the accuracy of diagnostic biomarkers, they were further validated for differences in the validation set GSE81622. All three diagnostic genes had significantly higher expression levels in LN than in controls (p < 0.05) (Figure 5A-C)
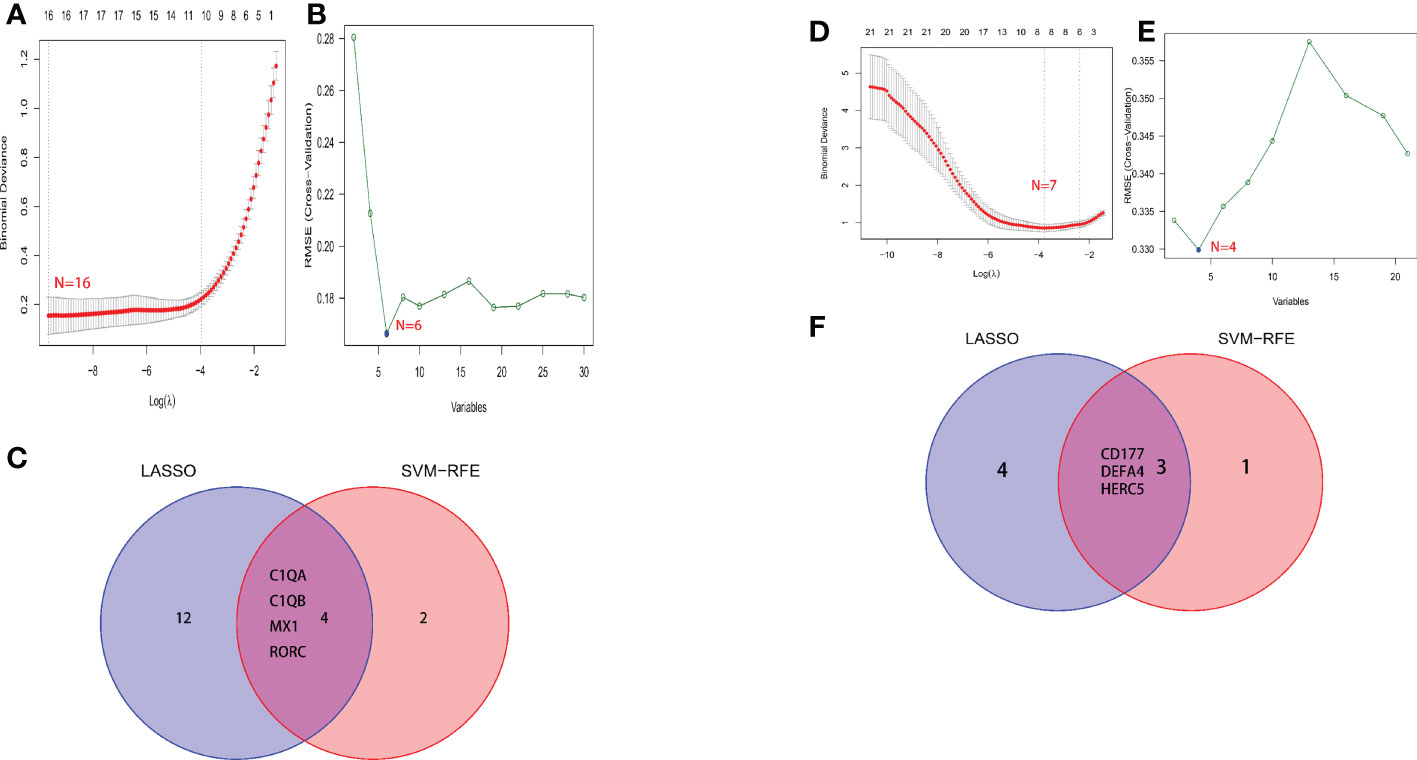
Figure 3 Screening process for diagnostic biomarkers of LN. (A, D) The LASSO model. (B, E) The SVM-RFE model. (C, F) LASSO and SVM-RFE overlap biomarkers. (A-C) Kidney tissue; (D-F) Peripheral blood.
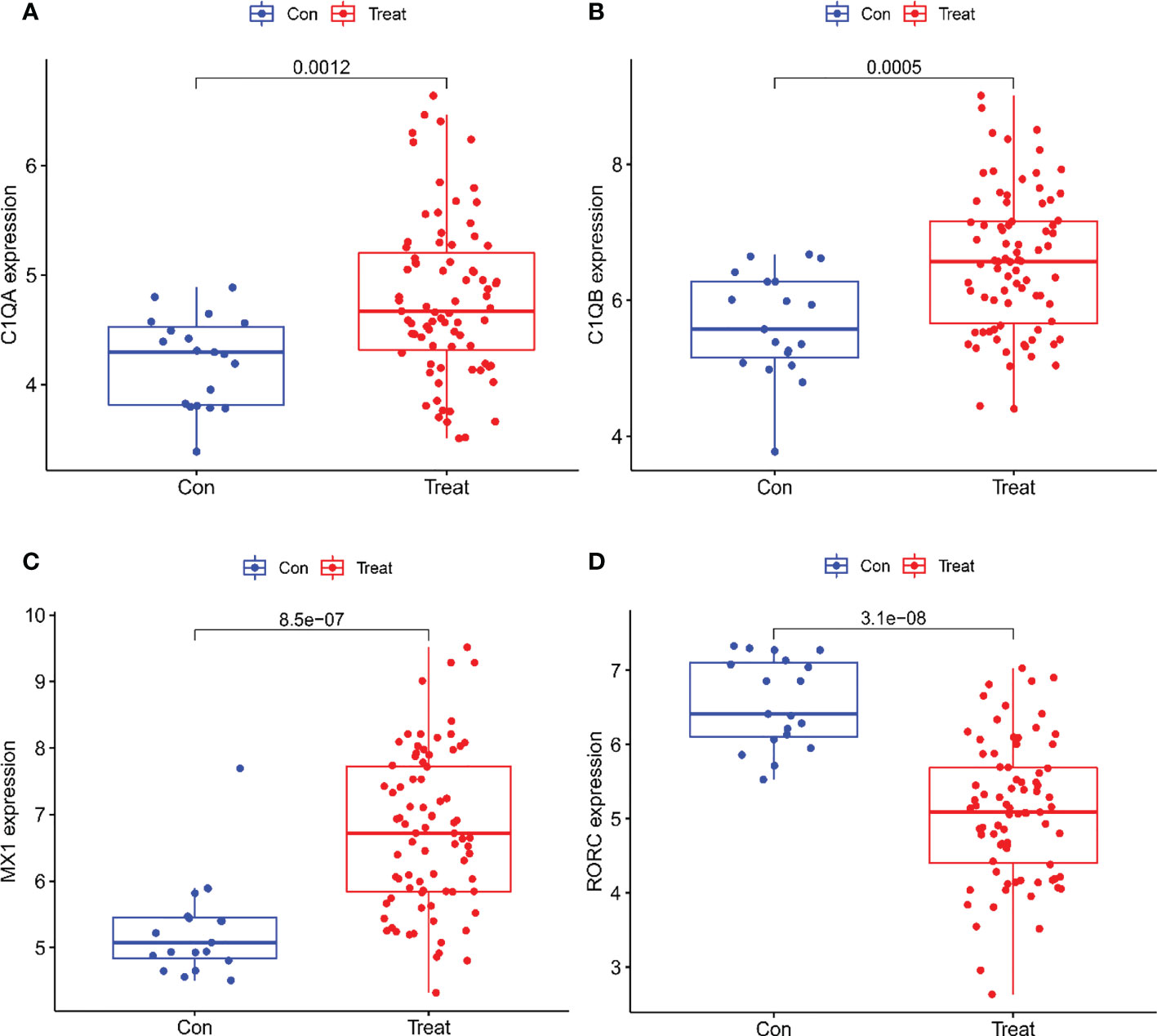
Figure 4 Expression of the Four biomarkers in the Validation group GSE200306 dataset. (A) C1QA; (B) C1QB; (C) MX1; (D) RORC.
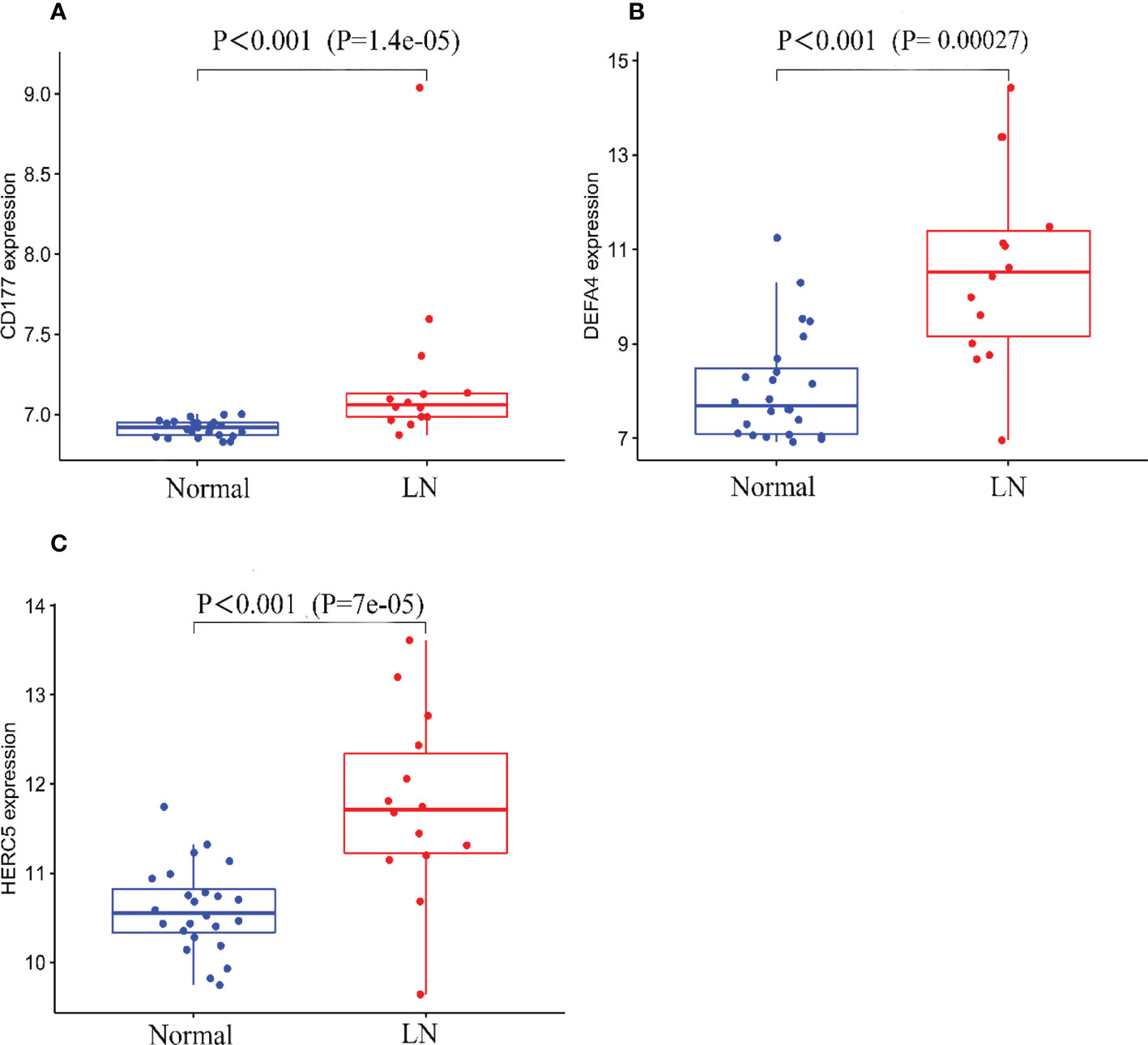
Figure 5 Expression of the three biomarkers in the validation group GSE81622 dataset. (A) CD177; (B) DEFA4; (C) HERC5.
3.4. Diagnostic value of characteristic biomarkers in LN
As shown in Figure 6, all six biomarkers screened had a high diagnostic value in LN. In the kidney tissue training group, the AUCs of the selected biomarkers were: C1QA (0.948, 95% CI: 0.909−0.978), C1QB (0.926, 95% CI: 0.885 − 0.962), MX1 (0.881, 95% CI: 0.823 − 0.925), and RORC (0.819, 95% CI: 0.740 − 0.889), as shown in Figure 6A; in the validation group, the AUCs of the biomarkers were C1QA(0.741, 95% CI: 0.634−0.836), C1QB(0.758, 95% CI: 0.646−0.856), MX1(0.865, 95% CI: 0.775−0.944), and RORC(0.911, 95% CI: 0.846−0.962), as shown in Figure 6B. The AUCs of the selected biomarkers in peripheral blood in the training group were: CD177 (0.791, 95% CI: 0.685 − 0.878), DEFA4 (0.812, 95% CI: 0.707 − 0.905), and HERC5 (0.775, 95% CI: 0.654 − 0.884), respectively (Figure 6C); the AUCs of the selected biomarkers in the validation group were: CD177 (0.885, 95% CI: 0.752 − 0.979), DEFA4 (0.843, 95% CI: 0.685 − 0.965), and HERC5 (0.880, 95% CI: 0.725 − 0.987), respectively (Figure 6D). The biomarkers showed a high diagnostic value in both the LN training and validation groups, with AUCs largely ≥0.8.
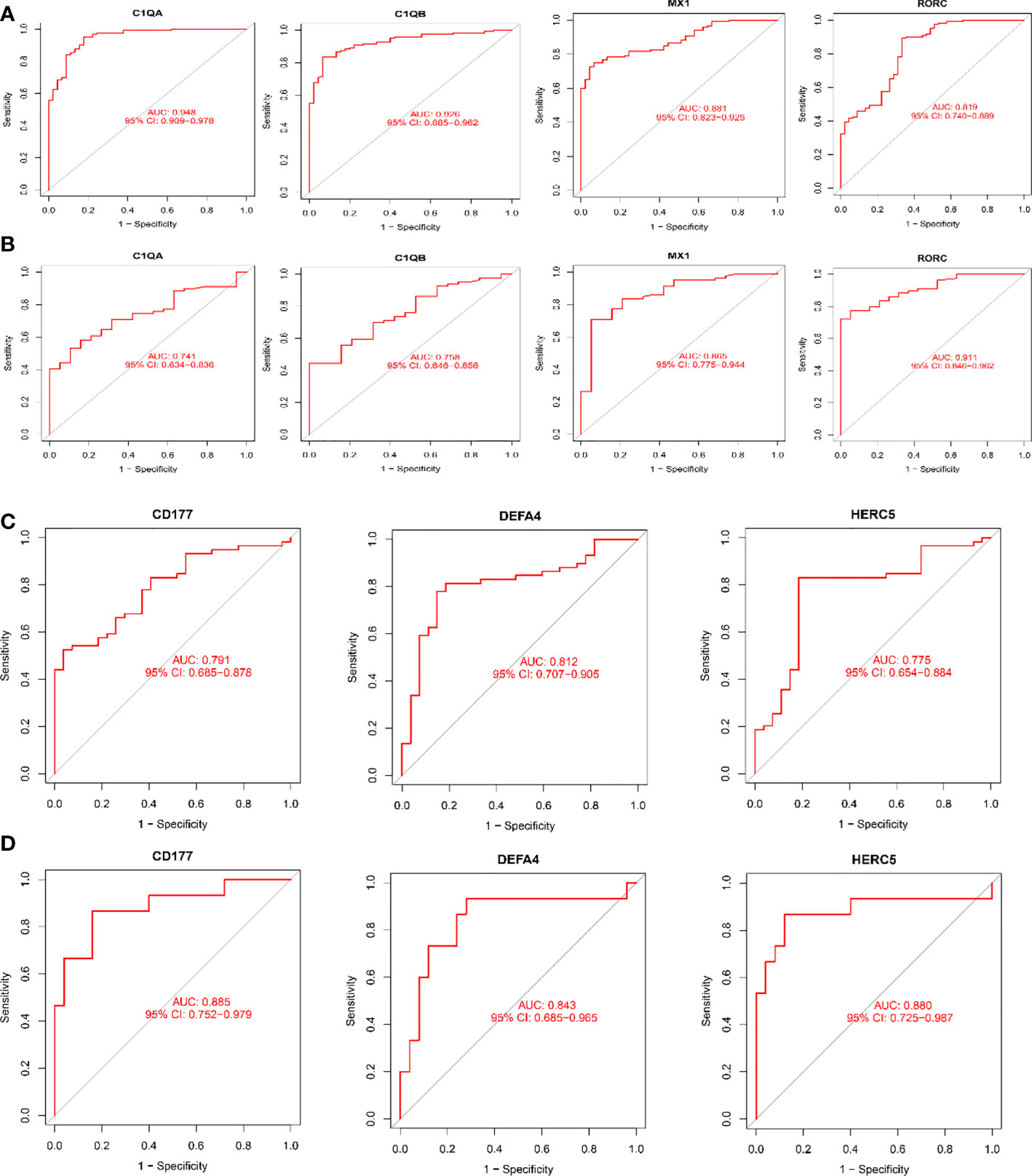
Figure 6 Receiver operating characteristic (ROC) curves for diagnostic validity of LN biodiagnostic markers. (A) ROC curves after fitting one variable for C1QA, C1QB, MX1, and RORC in the original data cohort; (B) ROC curves after fitting one variable for C1QB, MX1, and RORC in the GSE200306 data set. (C) ROC curve of CD177, DEFA4 and HERC5 after fitting one variable in the original data cohort; (D) ROC curve of CD177, DEFA4 and HERC5 after fitting one variable in the GSE81622 data set curve. (A, B) Kidney tissue; (C, D) Peripheral blood.
3.5. Analysis of immune cell infiltration
Differential analysis of immune cells in LN and normal samples showed that T cells regulatory (Tregs) (p = 0.017) were significantly lower and NK cells resting (p = 0.019) were significantly higher in LN kidney tissue than in the normal group (see Figure 7A). In LN blood tissues, B cells naive (p<0.001), B cells memory (p<0.001), T cells CD4 memory resting (p=0.007), Monocytes (p=0.006), Macrophages M1 (p=0.011), Macrophages M2 (p=0.039), Dendritic cells activated (p<0.001) were significantly higher than the control group (Figure 7C).
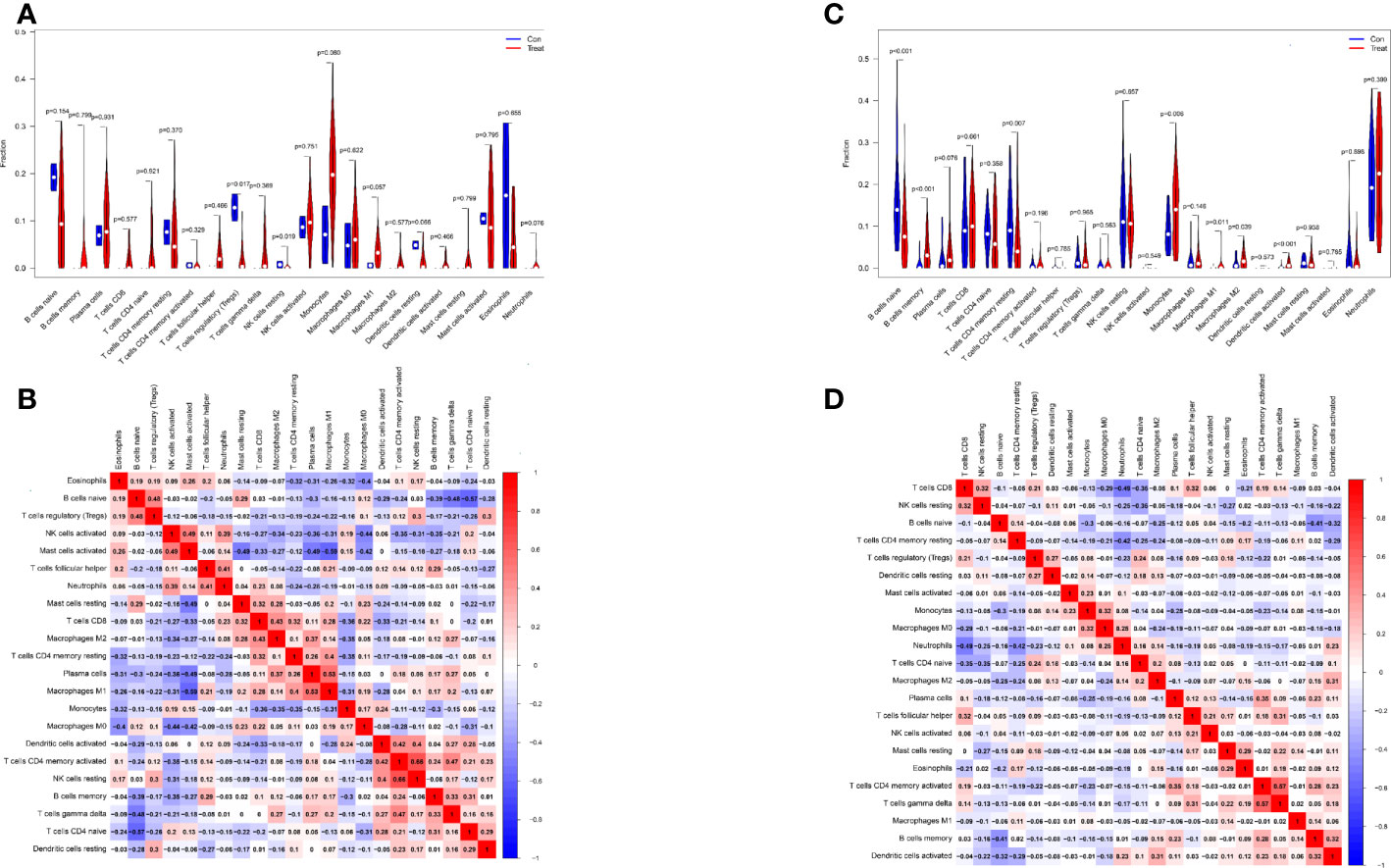
Figure 7 Distribution and visualization of immune cell infiltration. (A, C) Comparison of 22 immune cell subtypes in LN and normal tissues. (A, C) LN compared with 22 immune cell subtypes in normal tissue. Blue represents control group, red represents LN kidney group ((A) LN, (C) peripheral blood group). (B, D) Heatmap of correlations between 22 immune cell subtypes. Both horizontal and vertical axes show immune cell subtypes, and the values inside represent the correlation coefficients of immune cells. Red represents positive correlation and blue represents negative correlation. The darkest red lattice represents the highest positive correlation between the two genes, and the darkest blue lattice represents the most significant negative correlation between the two genes.
In addition, the correlation of 22 immune cells in all samples was analyzed (Figure 7B, D). There was a significant positive correlation between NK cells resting and T cells CD4 memory activated (r = 0.66) and a significant negative correlation between Mast cells activated and MacrophagesM1 (r = − 0.59) in LN kidney tissue and normal group samples (Figure 7B). In LN peripheral blood and normal group samples, T cells CD8 showed a significant negative correlation with Neutrophils (r=-0.49); T cells CD4 memory activated showed a significant positive correlation with T cells gamma delta (r=0.57) (Figure 7D).
3.6. Correlation of biomarkers with infiltrating immune cells
In kidney tissue, C1QA was positively correlated with T cells CD8 (R= 0.56, P= 7.94E-05)、Macrophages M1(R= 0.47, P= 0.0016) and Mast cells resting(R= 0.32, P= 0.032); and negatively correlated with Mast cells activated (R= -0.40, P= 0.0087), T cells CD4 naïve (R= -0.39, P= 0.0088) and NK cells activated(R= -0.32, P= 0.033) (Figure 8A). C1QB was positively correlated with MacrophagesM1 (R = 0.39, p = 0.0099), Neutrophils (R = 0.34, p= 0.024), and TcellsCD8 (R = 0.44, p = 0.0029); and negatively correlated with Dendritic cells resting (R = − 0.36, p= 0.017) and Mast cells activated (R= − 0.39, p= 0.01) (Figure 8B). MX1 was positively correlated with Eosinophils (R = 0.52, p = 0.00037), T cells CD8 (R = 0.35, p = 0.023), T cells follicular helper (R = 0.45, p = 0.0023); and negatively correlated with Dendritic cells resting (R = − 0.33, p = 0.032), TcellsCD4naive (R= − 0.53, p = 0.00026) (Figure 8C). RORC was positively correlated with Dendritic cells resting (R = 0.49, p = 0.00084); negatively correlated with B cells naive (R= − 0.42, p= 0.0056) (Figure 8D), and C1QB, MX1, and RORC were all correlated with Dendritic cells resting.
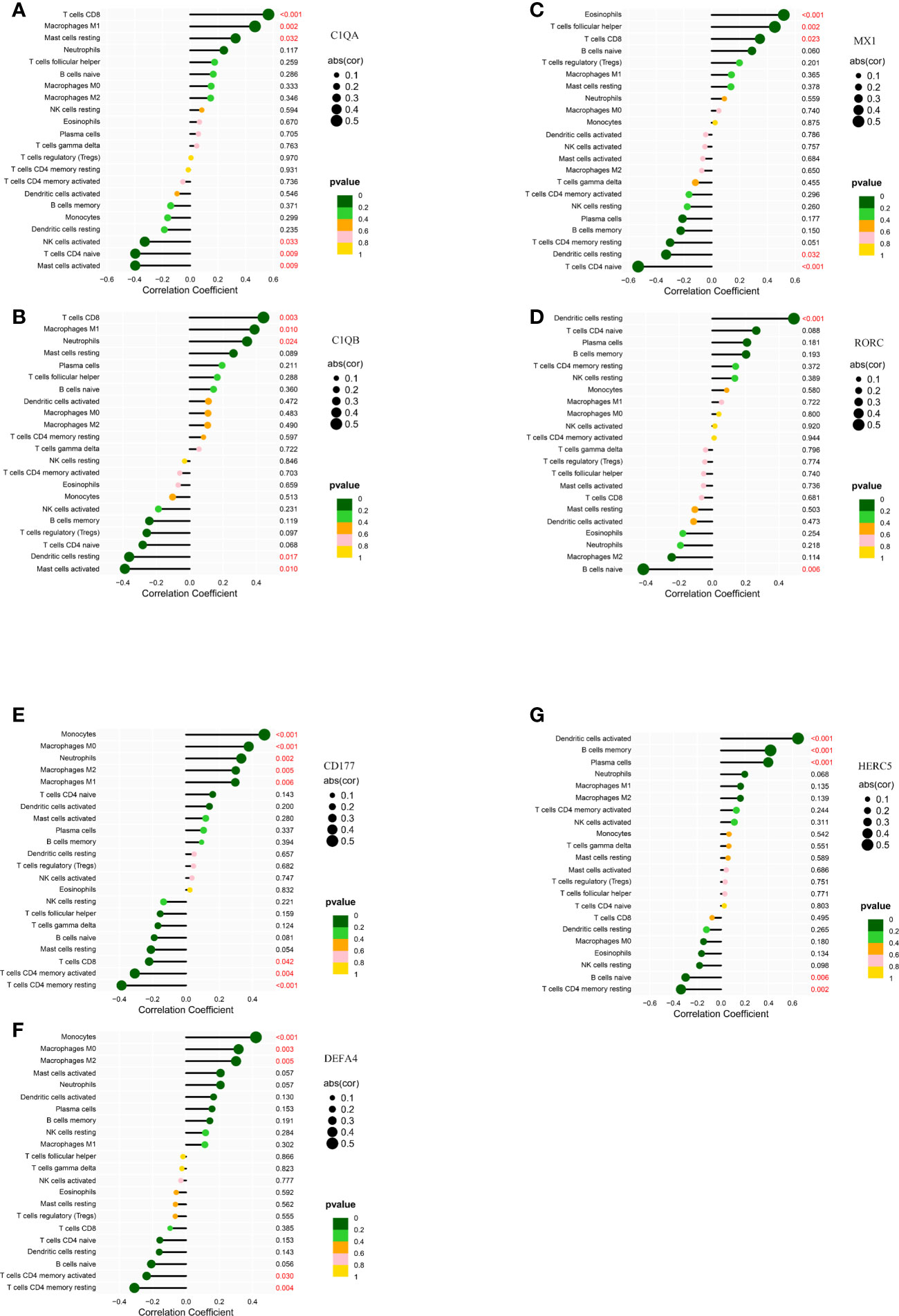
Figure 8 Correlation of LN kidney tissue biomarkers C1QA (A), C1QB (B), MX1 (C), RORC (D) and LN peripheral blood biomarkers CD177 (E), DEFA4 (F), HERC5 (G) with infiltrating immune cells. The vertical ordinate represents the name of the immune cell and the abscissa represents the correlation coefficient. The circle size represents the absolute value size of the correlation coefficient, and the color of the circle represents the P value of the correlation test. If p value < 0.05, p values are indicated in red.
In peripheral blood tissues, CD177 was positively correlated with Macrophages M0 (R = 0.38, p = 0.00037), Macrophages M1 (R = 0.3, p = 0.0059), Macrophages M2 (R = 0.3, p = 0.0054), Monocytes (R = 0.47, p = 6.8e − 06), Neutrophils (R = 0.33, p = 0.002), and negatively correlated with T cells CD4 memory activated (R= − 0.31, p = 0.0042), T cells CD4 memory activated (R = − 0.39, p = 0.00026), and T cells CD8 (R = − 0.22, p = 0.042) (Figure 8E). DEFA4 was positively correlated with MacrophagesM0 (R = 0.32, p = 0.0032), Macrophages M2 (R = 0.3, p = 0.0051), Monocytes (R = 0.42, p = 6.1e − 05), and negatively correlated with T cells CD4 memory activated (R = − 0.24, p = 0.03), T cells CD4 memoryresting (R = − 0.31, p = 0.0039) (Figure 8F). HERC5 was positively correlated with B cells memory (R= 0.42, p=7.6e-05), Dendritic cells activated (R = 0.65, p = 2.2e − 11), Plasma cells (R = 0.4, p = 0.00017), and negatively correlated with B cells naive (R= − 0.3, p = 0.0056), T cell CD4 memoryresting (R = − 0.34, p = 0.0016) (Figure 8G). CD177, DEFA4, HERC5 were all associated with T cells CD4 memory resting.
3.7. Clinicopathological correlation analysis with target immune markers
To determine the clinical applicability of these markers, we consulted the Nephroseq database and related literature to obtain correlations between seven immunomarkers and clinicopathology. The summary is shown in Table 1 and is confirmed below.
3.7.1. Correlation with different pathological types of LN
According to the Nephroseq database and related literature, seven candidate gene expressions correlated with the WHO lupus nephritis class. The expression of C1QA and C1QB was greater in class III than in class II, as shown in Figure 9. Compared to peripheral blood, peripheral blood C1Q antibodies have been studied more frequently, because human kidneys are more challenging to obtain. Because the expression of renal C1Q was positively correlated with peripheral blood C1Q antibodies, studies related to C1Q antibodies may have some implications. Zhu Chen et. found that in terms of quantitative C1q antibody levels, serum resistance was highest in class IV patients, followed by class III (20). Class III levels were also higher than class II but were not statistically significant. These data are also consistent with Fang et al. (21) and Cai et al. (20, 22). In summary, the expression of C1QA and C1QB was class IV > class III > class II.
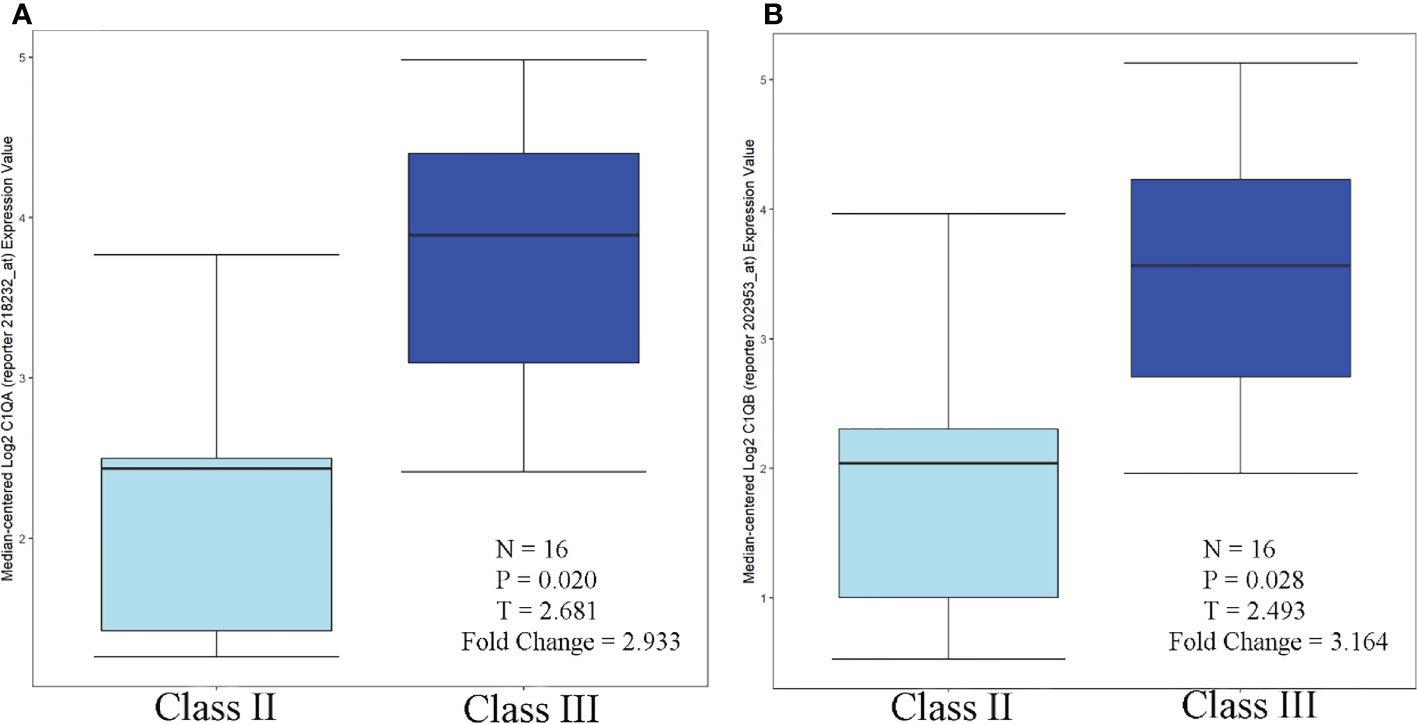
Figure 9 C1QA/C1QB over-expression in WHO Class III vs. WHO Class II (Lupus Nephritis Samples. (A) C1QA, (B) C1QB.
3.7.2. Analysis of infiltration of diagnostic genetic markers in the renal
C1QA, C1QB, MX1 and RORC were expressed in both glomeruli and tubules, with greater tubular than glomerular expression. Based on available literature and the Nephroseq database. Tao Liu et al. performed immunohistochemical analysis of kidneys from LN patients and healthy controls, and C1q was expressed in both glomeruli and tubules. Moreover, C1q protein levels were higher in the renal tubules (23), consistent with Desiree Tampe et al. Desiree Tampe observed a significant induction of glomerular, tubulointerstitial compartments, C1QA, and C1QB expression levels in patients with LN compared to healthy controls (24). MX1 is one of the IFN-I-inducible genes. Increased expression of the MX1 gene was detected in renal cells, PBMC, and renal tubules of patients with LN (25–28). Based on the Nephroseq database, it is known that RORC was distributed in both glomeruli and tubules, and the expression in tubules was greater than that in glomeruli (Figure 10).
3.7.3. Correlation with the activity and recurrence frequency of LN
Based on the current study, the relevant literature directly or indirectly confirms that the expression of C1QA/B (29–33), MX1 (25, 27), RORC (34), CD177 (35, 36), DEFA4 (37, 38), and HERC5 (39, 40) is strongly correlated with SLE/LN activity in a broad sense. However, there are a few studies with different results. In terms of LN activity-related and relapse rates, N Marto et al. found a higher prevalence of anti-C1q antibodies in patients with active LN than in those without the renal disease (74% V 32%, p<0.0001) (41). GabriellaMoroniMD et al. showed that the onset or recurrence of LN was associated with elevated anti-C1q antibody titers in the previous six months (42). High levels of C1q antibodies appear two to six months before the onset of LN and decrease or become undetectable after successful treatment (41, 43–49). In addition, RORC also showed significant downregulation in patients with LN in remission (50). In summary, C1QA, C1QB, MX1, RORC, CD177, DEFA4, and HERC5 can somewhat predict the prognosis of LN (37, 38, 40, 41, 51–53).
3.7.4. Relationship with immune complexes
LN begins with the in situ formation and deposition of immune complexes. Subsequently, immune complexes deposition triggers a complex series of events involving in situ activation of complement (C1q A, C1QB), Fc receptors, and adhesion molecules, leading to the recruitment and activation of inflammatory cells and ultimately kidney injury (54, 55). Therefore, we hypothesized that the gene expression of seven candidate genes, except RORC, is positively correlated with immune complexes. The deposition of immune complexes activates their expression. The available literature confirms the following.
① C1q is crucial in clearing immune complexes and apoptotic cells (56–58). This clearance may be impaired by low C1q levels of apoptotic cells and cellular debris, which are sources of autoantigens (59, 60). The immune response induced by the inefficient clearance of apoptotic cells and cellular debris gives rise to autoantibodies.
② MX1 and HERC5 are interferon-inducible genes positively associated with immune complexes. The immune complexes are endogenous IFN inducers (61). Precipitates of immune complexes in the kidney activate a series of inflammatory responses and the production of type I interferon (62–64). Type I interferon binds to the type I interferon receptor (IFNαR), which induces interferon-stimulated genes (ISG) (including MX1, HERC5) (65).
③ The relationship between RORC, CD177, DEFA4 and immune complexes has not been reported for the time being.
3.7.5. Relationship with renal function
Correlations between six immunomarkers and renal function (glomerular filtration rate, GFR) were obtained from the Nephroseq database. Scatter plots showed that all were negatively correlated with GFR except for RORC (Figure 11), consistent with relevant contemporary research by Zhu Chen et al. (20). Anti-C1q was positively correlated with renal activity indices, including intra-capillary hypercellularity, glomerular leukocyte infiltration, and nuclear rupture/fibrin-like necrosis. It was negatively correlated with chronic indices, such as glomerulosclerosis and interstitial fibrosis.
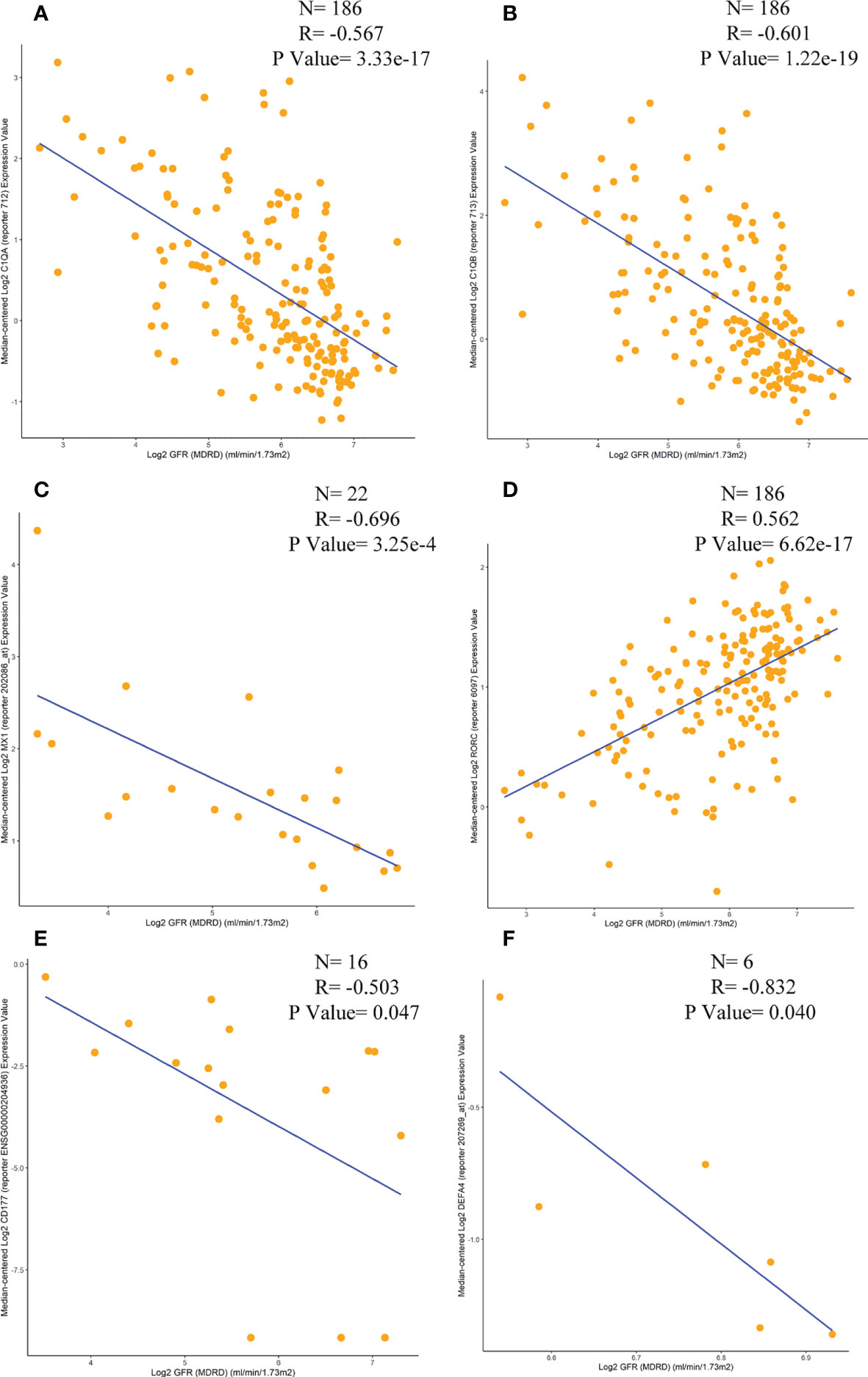
Figure 11 Correlation analysis of the expression levels of C1QA, C1QB, MX1, RORC, CD177 and DEFA4 with GFR. (A) C1QA, (B) C1QB, (C) MX1, (D) RORC, (E) CD177, (F) DEFA4.
3.7.6. Correlation with traditional prognostic markers (e.g., ANA, anti-dsDNA titers, C3, and C4)
There are few articles on the association of peripheral blood genetic markers CD177, DEFA4, and HERC5 with traditional prognostic markers (e.g., ANA, anti-dsDNA titers, C3, C4), although there are relevant theoretical underpinnings. Future studies could be conducted in this direction to clarify the correlation between the two further. The C1Q antibody is currently relatively well-studied. Rosalie M. Sterner et al. found that the bound C1 complex could cleave C4 and C2 to form the C3 convertase complex, which could cleave C3 into C3a and C3b, with C3b contributing to phagocytosis of regulatory substances and clearance of immune complexes (4, 66). Tao Liu et al. further found that the levels of C1q and C4 were positively correlated with the levels of C3 in the blood (41). In addition, N Marto et al. found that peripheral blood anti-C1q titers were negatively correlated with C3 and C4 levels and positively correlated with anti-dsDNA (22, 41, 67). C1Q in serum was negatively correlated with anti-C1Q antibodies. In summary, C1q expression was positively correlated with the levels of C3 and C4. MX1 was negatively correlated with C3 and C4 and positively correlated with Anti-DNA antibodies (25).
3.7.7. Applications
The applications of the genetic biomarkers screened in this study are broadly divided into two major blocks: one for diagnosing LN, assessing disease status, and predicting the prognosis of LN; and the other for guiding clinical drug use and precise treatment.
3.7.7.1. Diagnosis and assessment of the activity
① Anti-C1q antibodies can be used to predict the onset of nephritis (21, 41, 68–70). A previous meta-analysis showed that anti-C1q antibodies had good sensitivity and specificity in the diagnosis of LN (0.58 and 0.75, respectively) (71). In addition, high titers of anti-C1q autoantibodies were associated with active LN with a sensitivity of 97% and a specificity of 92% (41, 42, 68, 69, 72). On the other hand, the C1q gene can be used for early screening. A quantitative or functional defect in C1q in pure congeners has been reported to be a strong disease susceptibility factor, with more than 90% of patients presenting with SLE and SLE-like symptoms (57, 73–75).
② Upregulation of ISG in peripheral blood is a hallmark of SLE and has been associated with SLE disease activity, playing an important role in the pathogenesis of SLE (27, 38, 51, 76, 77). MX1 is of great importance as a biomarker for SLE (78).
③ CD177 and DEFA4 are neutrophil subsets that correlate with the onset, severity and prognosis of SLE/LN (79–81). In renal biopsies, neutrophils were found to be a marker of glomerular activity in SLE, while detection of neutrophil-derived proteins in urine is an alternative marker of disease activity (35). An increase in circulating LDG population was also observed in adolescent SLE patients, which correlated with dsDNA antibody concentrations and disease activity scores. This suggests that the neutrophil subpopulation (CD177, DEFA4) may be a useful biomarker (36).
④ Kennedy et al. reported that the HERC5 gene could be used as an IFN biomarker in SLE patients (82). A recent study also concluded that IFN characteristics may be a better biomarker of overall disease severity than disease activity, and that IFN-High patients tend to have a more severe disease course (51, 83).
3.7.7.2. Treatment
① C1Q: SLE patients with C1q deficiency were successfully treated by fresh frozen plasma, which transiently restored complement activity and reduced circulating immune complexes (84–86).
② MX1: Clinically, a number of biologic agents targeting type I interferon have emerged (87). In addition, Mx1 gene expression in peripheral blood cells has been used as a sensitive biomarker for LN treatment, identifying patients who have responded well to anti-I interferon therapy (27, 88).
③ RORC: RORγt is a core transcription factor for Th17 cell differentiation. Many studies suggest that RORγt may be a potential therapeutic target for Th17-mediated autoimmune diseases (89–93). Currently, several pharmaceutical companies are developing RORgt blockers and more than 200 small molecule compounds have been patented. They can inhibit RORγt activity, block Th17 differentiation and improve autoimmune disease manifestations (90, 94). Several Phase I and Phase II studies are already underway (95, 96).
④ CD177, DEF4: NETosis intervention improves the performance of experimental SLE (97, 98). Neutropenia has been reported in 20-40% of SLE patients and SLE patients treated with immunosuppressive drugs may also be at risk of developing neutropenia (99, 100). Breaking this pathway may lead to the development of new drugs. Anti-CD20 therapy has been shown to improve SLE in humans (101).
⑤ HERC5: Several drugs targeting the type I interferon pathway in SLE and LN are currently being investigated. Several drugs targeting the type I interferon pathway in SLE and LN are being investigated, including anti-interferon monoclonal antibodies (sifalimumab, rontaziumab), and monoclonal antibodies targeting the type I interferon receptor (anifolumab) (102–104). Also, many clinical trials have included the measurement of IFN characteristics in their studies. Because the underlying disease mechanisms may differ between SLE patients with IFN-High and IFN-Neg, interferon scores have been applied to stratify patients for treatment.
4. Discussion
Due to the invasive nature of renal biopsy and the non-specific nature of LN treatment methods, LN cannot be treated precisely, and there is a steady trend of LN patients progressing to ESRD worldwide (105). How to identify LN early, intervene in time, and reduce LN patients into chronic renal failure is significant. The traditional biomarkers of LN, C3, C4, ds-DNA and anti-Sm, have some significance in the evaluation of LN disease, but they all have some limitations (106–110). Therefore, it is urgent to develop new biomarkers to predict the renal pathology of LN patients. The pathogenesis of LN is not clear in modern medicine, but it is generally believed that renal infiltrating immune cells play an important role in the pathogenesis of LN (111–113). Significant accumulation of various types of immune cells, including T cells, B cells, plasma cells, NK cells, and macrophages, has been observed in the kidneys of active LN and lupus-prone mice (114–116). Epidemiological studies have shown significant familial aggregation of LN, suggesting that genetic factors play an important role in the development of LN and disease progression (117). Up to now, a large number of related genes that may be involved in the pathogenesis of LN have been reported, but few studies have focused on abnormally expressed gene biomarkers associated with immune infiltration between LN and normal tissues. Therefore, we aimed to identify candidate diagnostic biomarkers for LN and investigate the role of immune cell infiltration in LN.
4.1. Correlation of DEGs with LN
In this study, DEGs in kidney tissue and peripheral blood of LN were analyzed by combined GEO database multi-chip. A total of 30 DGEs were screened in kidney tissues, 25 of which were upregulated, mainly CD163, CFH, CX3CR1, HLA-DPB1, HLA-DQB1, IFITM1, IL10RA, etc.; 5 were downregulated, mainly RORC, etc. A total of 21 DGEs were screened in LN peripheral blood, all of which were significantly upregulated. The main ones were IFI44L, LCN2, CD177, etc. DEGs were all closely related to LN.
Professor Xueqing Yu of Sun Yat-sen University found that several independent susceptibility loci such as HLA-DPB1 and HLA- DQB1 are closely associated with the development of LN (118). Also, HLA- DQB1 is a susceptibility gene for SLE (119). Differentiated antigen cluster 163 (CD163) is expressed in monocytes/macrophages, a marker of macrophage activation, and has the ability to mediate the clearance of free hemoglobin by macrophages, thereby reducing kidney damage from the reaction of heme iron with endogenous hydrogen peroxide to generate free radicals (120, 121). It has the ability to modulate immunity, scavenge tumor necrosis factor-like weak apoptosis-inducing factors, activate inflammatory responses, and induce cell proliferation and apoptosis (122, 123). Several studies have shown that CD163+ macrophages infiltrate significantly in patients with LN (124, 125), and the number of CD163+ macrophage infiltration is closely related to the activity of LN (125). Studies on other macrophages are relatively scarce and need to be further explored in the future. CX3CR1 is widely expressed in the renal tissue of patients with LN and can be used as one of the alternatives to renal biopsy (126). CX3CR1 is involved in the pathogenesis of human type IV LN (127). Administration of an analogue of fractalkine as an antagonist in MRL/lpr lupus mice significantly inhibited the development and progression of their LN (128). An investigation by Professor Wang Fengmei of Peking University showed that serum CFH levels were closely related to LN disease activity and that CFH deficiency accelerated the development of LN (129). CFH-related genes are also associated with SLE susceptibility (130). IFN is a major pathogenic factor in systemic lupus erythematosus (SLE) and LN (131). More than 20 cross-sectional case-control studies have shown that the expressions of IFI27, IFI44, IFI44L, IFIT1, PRKR and RSAD2 are proportionally clustered in LN (131). IFI44L promoter methylation can be used as a blood biomarker for LN (132–134). The protein encoded by IL10RA is a receptor for interleukin 10 (IL-10) (135). Overexpression of IL-10 in LN renal tissue and peripheral blood is highly correlated with the onset and activity of LN (136, 137). FKBP5 and EGR1 are closely associated with SLE, but the direct correlation with LN needs to be further confirmed (138, 139).
The interferon-inducible transmembrane protein (IFITM) gene family includes IFITM1, which plays a role in a number of biological activities such as interferon-homotypic cell adhesion function and anti-proliferative activity of cells (140–143). Lipocalin-2 (LCN2) is an indicator of the severity of LN and plays a key role in the immune response, which promotes Th1 cell differentiation exacerbating LN in an autocrine or paracrine manner, mainly through the IL-12/STAT4 pathway (144). Urinary LCN2 can be used as an early biomarker of LN (145, 146). RSAD2 is an interferon-inducible iron-sulfur cluster-binding antiviral protein. In a study of genome-wide DNA methylation in LN, methylation of the CpG site of the RSAD2 gene was shown to be highly correlated with LN (147). TGFB1 and MMP8 may be involved in LN through a mediated matrix pathway (148). The relationship between diagnostic biomarker genes and LN is detailed in the biomarkers section.
4.2. Functional correlation analysis
The disease enrichment analysis of LN kidney tissue DGEs was mainly enriched in immune class, inflammatory, renal and cardiovascular diseases, such as: primary immunodeficiency disease, vasculitis, renal failure, renal cancer, and renal disease. This fits in previous studies. In terms of the pathogenesis of SLE, multiple factors such as multiple genes and environmental factors are involved (149), and viral infections are often used as environmental triggers of SLE (150). In other words, LN is essentially an immune-mediated inflammatory response. In terms of the symptoms and outcomes of LN, there is a significant correlation between LN and cardiovascular disease (151), and 20.8% of deaths in LN patients are caused by cardiovascular disease (152). Many LN patients end up with chronic kidney disease (CKD) and ESRD despite receiving potent anti-inflammatory and immunosuppressive therapy.
Differential genetic disease enrichment in peripheral blood is mainly associated with hematologic disorders, bone marrow. is mainly associated with hematologic disorders, bone marrow. For example, erythrocytosis, thrombocytosis, bone marrow disorders, oral diseases, etc. SLE often involves the blood system, and hematological abnormalities are a common manifestation of SLE (153). Hypercoagulation is often accompanied in LN, which may be related to its immune complexes which can promote platelet aggregation, form thrombus, and cause microcirculation disorders. One of the more controversial points is that thrombocytosis may be an indicator of disease activity and responsiveness. Because about a quarter of SLE patients usually show thrombocytopenia, thrombocytosis is an unusual finding. LN can also be accompanied by oral ulcers. For example, a retrospective study conducted by a tertiary medical center in Assam, India, showed that 176 patients with LN had a 31.8% probability of having a concomitant oral ulcer (154). SLE, especially patients with LN, is often accompanied by osteoporosis, which may be related to the long-term use of prednisone (155). Ramsey et al., 1994, in the US National Health Survey, found that the incidence of fractures in patients with SLE was five times higher than in the normal population (156).
GSEA results indicate that the immune response plays a crucial role in LN. GSEA enrichment of kidney tissue mainly involved immunity with inflammation. For example, the five pathways of autoimmune thyroid disease, cell adhesion molecules cams, systemic lupus erythematosus, type 1 diabetes mellitus, and viral myocarditis were activated. Cell adhesion molecules (CAM) play a key role in inflammation, immune response, and thrombosis (157). Intercellular adhesion molecule-1 (ICAM-1) and vascular cell adhesion molecule-1 (VCAM-l) are two CAMs that are currently receiving the most attention. In the autoimmune MRL/lpr model in LN mice, it was found that the severity of renal histopathology was correlated with the expression of VCAM-1, and ICAM-1 was also used as a major marker of renal inflammatory activity (158). Blocking this pathway may inhibit the pathological infiltration of LN inflammatory cells (159). In clinical trials, the expression of ICAM-1 and VCAM-1 in glomerular endothelial cells and cells, serum and urine of LN patients was significantly increased, which was positively correlated with LN activity and played an important role in the development of LN (160–162). At present, anti-adhesion molecule antibodies have achieved a certain effect in rheumatism (163), vascular remodeling, heart and cerebral ischemia-reperfusion (164, 165) and other aspects, and the field of nephropathy urgently needs to be developed. The results of LN peripheral blood GSEA enrichment analysis showed that the pathways were mainly involved in immune, cell cycle. For example, cell cycle, cytosolic DNA sensing pathway, NOD-like receptor signaling pathway, proteasome, and RIG-1-like receptor signaling pathway were activated. Inflammatory response or cellular injury triggers abnormal proliferation of LN tethered cells, activates the cell cycle and ultimately leads to glomerulosclerosis and end-stage renal failure. Interference with the cell cycle can prevent cell proliferation or promote apoptosis (166). For example, tacrolimus and motilmic acidophilus, commonly used drugs in LN, inhibit the proliferation of thylakoid cells by acting on different phases of the cell cycle of thylakoid cells and thus slow down the progression of glomerular disease (167, 168). The transmission mechanism of cytoplasmic DNA is the molecular basis for the immune system to produce an immune response. Aberrant self-DNA accumulation and cellular type I interferon (IFN-I) secretion are the two main parts in the pathogenesis of LN (169, 170), while cellular sensors play an important role in the process of extracellular DNA triggering IFN-I (171). Various DNA sensors have been reported so far, but specific cytoplasmic DNA sensors involved in LN’s own DNA-induced autoimmune response are yet to be further investigated. Nucleotide-binding oligomerization domain NLR and RIG-1 like receptors are involved in the pathogenesis of SLE/LN, which mainly stimulate cytokine production by activating innate immunity through the uptake of immune complexes (ICs) between antinuclear antibodies and nucleic acids into cells (172, 173). In addition, the NLR family also drives key molecules of the inflammatory response through the formation of “inflammasomes”, resulting in transcriptional induction of type 1 IFNs and other inflammatory cytokines (150). This information is essential to improve therapeutic strategies for LN, such as the production of drugs that target interferon or interferon receptors. Proteasome inhibition is a new therapy for LN and plays an important role in the treatment of LN, such as cyclophosphamide, rituximab, and bortezomib (174).
4.3. Biodiagnostic markers screened by machine learning
Based on two machine learning algorithms, LASSO and SVM-RFE, four biomarkers with diagnostic value were screened in kidney tissues: C1QA, C1QB, MX1, and RORC. C1q (C1qA, C1qB and C1qC) is the first component of the classical pathway of complement activation and plays an essential role in SLE. In recent years, domestic and international studies have shown a significant correlation between C1q deficiency and abnormal C1qAb and the onset, disease activity and pathological type transformation of SLE, especially LN (42, 175, 176). Genetic defects in C1q are susceptibility factors for SLE, and close association of LN with C1q deficiency has been demonstrated in both human SLE and C1q-deficient animal models (4, 175, 175, 177–179). Based on a systematic review of reported cases, approximately 75-93% of patients with C1q deficiency have SLE or SLE-like disorders (74, 180). In 1998, in a review of patients with C1q deficiency, Walport et al. found that 38 of 41 subjects (93%) exhibited a clinical syndrome closely related to SLE (57). In 2011, Schejbel et al. found that 88% of the 63 patients reported with C1q deficiency had SLE/SLE-like disease (181). In 2014, Mihaela Stegert et al. reviewed the overall prevalence of SLE/SLE-like syndrome in all 71 patients with C1q deficiency reported as 77.5% (182). In the C1q-deficient SLE mouse model (MRL/Mp-+/+), C1q deficiency in mice can also lead to autoimmune diseases such as SLE (177). On the one hand, it is due to the impaired phagocytic clearance of apoptotic cells, and on the other hand, C1q deficiency significantly enhances the production of anti-C1q antibodies, leading to the development of proliferative immune complex glomerulonephritis (183, 184). It explains the lack of association of C1q with SLE/LN (177). The association between C1QA and C1QB in the renal biomarker screen may further confirm the importance of the complement cascade in LNs. According to related studies complement cascade activation in SLE patients leads to hypocomplementemia and deposition of complement components at sites of tissue damage (4, 184). Human mucovirus resistance protein 1 (Mx1), one of the type I interferon (IFN)-inducible genes (27), is a type I IFN-dependent transcript that plays a major role in apoptosis and cytokine-mediated cell signaling. In LN, MX1 is used as a potential marker for diagnosing peripheral blood LN activity (25, 28) and is also considered as a susceptibility gene for SLE (25, 28). RORC is a key factor in coordinating the transcription of genes encoding IL17 and plays a key role in the regulation of inflammatory response (185). Studies have confirmed that it plays an important role in the dysregulated immune response associated with SLE (50).
Three biomarkers with diagnostic value were screened in peripheral blood: CD177, DEFA4, HERC5. CD177 is an important neutrophil gene encoding neutrophil membrane glycoprotein, but its function in neutrophils is not fully understood. Neutrophils play an active role in driving autoimmune response and tissue damage in SLE. We speculate that CD177 is mainly involved in the pathogenesis of SLE/LN by regulating NET and/or neutrophils (186). Current studies of CD177 are mainly focused on anti-neutrophil cytoplasmic antibody (ANCA)-related systemic vasculitis (187). In the future, we should pay attention to related research to fill the gap in LN. DEFA4 is expressed at a higher level in patients with active SLE (188), and is involved in immune-mediated tissue damage caused by SLE autoantibody deposition (189). In addition, DEFA4 is related to early granulocyte production and plays a role in the maturation of neutrophils and the formation of neutrophil extracellular traps (NETs). In other words, DEFA4 may be involved in the pathogenesis of LN by affecting neutrophils (37). HERC5 is an interferon-inducible gene (190–193). CoitP et al. found that HERC5 is hypomethylated in lupus patients with renal involvement (194), and Lingling Shen et al. further validated the crucial role of HERC5 in the pathogenesis of LN by PCR (qRT-PCR) and enzyme-linked immunosorbent assay (ELISA) (134).
4.4. Types of immune cell infiltration
Analysis of LN samples and normal samples using CIBERSORT revealed that a variety of immune cell subtypes were closely related to important biological processes in LN. In kidney tissue, T cells regulatory (Tregs) infiltration was reduced; NK cells resting infiltration was increased; in blood samples, B cells memory, T cells CD4 memory resting, Monocytes, Macrophages M1 and Macrophages M2 Dendritic cells activated infiltration was increased; B cells naive infiltration was decreased. The screened biomarkers were correlated with infiltrating immune cells, and the biomarkers C1QB, MX1, and RORC were correlated with Dendritic cells resting in kidney tissues. Peripheral blood tissue CD177, DEFA4, HERC5 were correlated with T cells CD4 memory resting. The specific mechanism can be traced back to the fact that LN is often triggered by abnormal immune system, involving a variety of immune cells, cytokines and related pathways (195). T cells e.g., CD4+, CD8+) play a central role in the pathogenesis of LN (196). In animal experiments, CD4 was shown to induce LN when infused into lupus-prone mice with CD4+ T cell lines (197). In the clinic, Masutani et al. found that CD4+ cells were the predominant cell type in grade I, IV and V renal infiltrates in patients with lupus nephritis (198, 199). CD4+ T is involved in LN pathogenesis and has been demonstrated to mediate LN inflammation (200), which exerts its function mainly by secreting cytokines (e.g., IFN-γ, IL-17 and IL-10, etc.) upon antigen activation and by transmitting tissue inflammation (201). In addition, it was reported that CD8+ T lymphocyte infiltration was predominant around the glomeruli of patients with LN, and the infiltrating interstitial cells were mainly CD4+ve T cells or CD8+ve (199, 202–205). Nataly Manjarrez-Orduño et al. found that a subpopulation of LN exhibited enhanced CD8+ terminal differentiation, suggesting a pathological role for CD8+ T cells in a specific subpopulation of LN and laying the groundwork for CD8-targeted therapy (206).
At the same time, relevant studies have shown that RNA sensing by traditional dendritic cells (DC) is central to LN development (207). Dendritic cells are the most potent antigen presenting cells known so far and are essential in the induction and maintenance of immune tolerance (208). DC have two subpopulations of myeloid dendritic cells (mDCs) and plasmacytoid dendritic cells (pDCs) (209). Regardless of which subpopulation is dysregulated, it will lead to tolerance disruption and self-attack, resulting in the development of LN (210). Both peripheral DC subpopulations are expected to be alternative diagnostic tools for invasive biopsy. Current SLE therapies, both conventional and biological, target specific subsets of adaptive immune cells, primarily B cells, T cells, and B and T cells are still considered to be the culprits of immune dysregulation in SLE (211), but DCs are paramount in establishing and maintaining peripheral tolerance (212, 213). DCs have a superior ability to acquire and process antigen for delivery to T cells and express high levels of co-stimulatory or co-inhibitory molecules that determine immune activation or non-response. This further highlights the importance of developing DCs in LN therapy, such as the administration of DC vaccines that may bring new hope for the treatment of LN (214).
Although this study used a large GEO dataset of LN kidney tissue and peripheral blood to explore immune cells and immune-related genes in LN and identified six diagnostic gene biomarkers with diagnostic and predictive value in patients with LN, there are still some limitations to be addressed. First, the study was retrospective and did not allow for first-hand, clinically important information. Second, the CIBERSORT algorithm has limitations in distinguishing Th17 from other CD4+ T cell subpopulations and cannot further analyze the correlation between the CD4+ T subpopulation Th17 and LN biomarkers. Third, bioinformatic determination of LN biomarkers and the function of immune cell infiltration requires further confirmation in a larger sample size prospectively.
5. Conclusion
C1QA, C1QB, MX1 and RORC (kidney); CD177, DEFA4 and HERC5 (peripheral blood) can be used as new candidate molecular markers for LN. Dendritic cells resting and T cells CD4 memory resting may be involved in LN development. These immune cells have the potential to be new targets for immunotherapy in LN patients.
Data availability statement
The datasets presented in this study can be found in online repositories. The names of the repository/repositories and accession number(s) can be found in the article/Supplementary Material.
Author contributions
HTY, KY directed the research and revised the manuscript; LW, ZY, HXY performed the research and wrote the paper; WL modified the tables and figures and revised the manuscript; RW was involved in finding and organizing the literature during the rework. All authors contributed to the article and approved the submitted version.
Funding
This work is supported by the National Natural Science Foundation of China (81873263).
Acknowledgments
The authors are thankful to Tianjin University of Traditional Chinese Medicine for the help in conducting this study.
Conflict of interest
The authors declare that the research was conducted in the absence of any commercial or financial relationships that could be construed as a potential conflict of interest.
Publisher’s note
All claims expressed in this article are solely those of the authors and do not necessarily represent those of their affiliated organizations, or those of the publisher, the editors and the reviewers. Any product that may be evaluated in this article, or claim that may be made by its manufacturer, is not guaranteed or endorsed by the publisher.
Supplementary material
The Supplementary Material for this article can be found online at: https://www.frontiersin.org/articles/10.3389/fimmu.2022.839197/full#supplementary-material
Abbreviations
ESRD, End-stage renal disease; LN, Lupus nephritis; DEGs, differential express genes; LASSO, least absolute shrinkage and selection operator; SVM-RFE, support vector machine-recursive feature elimination; AUC, area under curve; DO, gene ontology disease enrichment; GSEA, gene set enrichment analysis; NLR, NOD-like receptor.
References
1. Anders H-J, Saxena R, Zhao M-h, Parodis I, Salmon JE. Mohan CJNrDp.Lupus Nephritis. Nat Rev Dis Primers (2020) 6(1):1–25. doi: 10.1038/s41572-019-0141-9
2. Mok CC, Wong RW-S, Lau CS. Lupus nephritis in southern Chinese patients: Clinicopathologic findings and long-term outcome. Am J Kidney Dis (1999) 34(2):315–23. doi: 10.1016/S0272-6386(99)70361-6
3. Mok CC, Ying KY, Ng WL, Lee KW, To CH, Lau CS, et al. Long-term outcome of diffuse proliferative lupus glomerulonephritis treated with cyclophosphamide. Am J Med (2006) 119(4):e325–355.e333. doi: 10.1016/j.amjmed.2005.08.045
4. Cook HT, Botto M. Mechanisms of disease: The complement system and the pathogenesis of systemic lupus erythematosus. Nat Clin Pract Rheumatol (2006) 2(6):330–7. doi: 10.1038/ncprheum0191
5. Tsokos GC. Systemic lupus erythematosus. Ann Internal Med (2011) 365(22):2110–21. doi: 10.1056/NEJMra1100359
6. Durcan L, O'Dwyer T, Petri M. Management strategies and future directions for systemic lupus erythematosus in adults. Lancet (2019) 393(10188):2332–43. doi: 10.1016/S0140-6736(19)30237-5
7. Miteva LD, Manolova IM, Ivanova MG, Stoilov RM, Stanilova SA. High interleukin-18 and low Foxp3 mrnas in peripheral blood of women with severe systemic lupus erythematosus: A cross-sectional study. Rheumatol Int (2020) 40(5):727–35. doi: 10.1007/s00296-020-04542-3
8. Tunnicliffe DJ, Palmer SC, Henderson L, Masson P, Craig JC, Tong A, et al. Immunosuppressive treatment for proliferative lupus nephritis. Cochrane Database Syst Rev (2018) 6:1465–58. doi: 10.1002/14651858.CD002922.pub4
9. Lightstone L, Doria A, Wilson H, Ward FL, Larosa M, Bargman JM. Can we manage lupus nephritis without chronic corticosteroids administration? Autoimmun Rev (2018) 17(1):4–10. doi: 10.1016/j.autrev.2017.11.002
10. Bruce IN, Gladman DD, Urowitz MBJAC. Factors associated with refractory renal disease in patients with systemic lupus erythematosus: The role of patient nonadherence. Arthritis Care Res (2000) 13(6):406–8. doi: 10.1002/1529-0131(200012)13:6<406::AID-ART11>3.0.CO;2-2
11. Yang W, Shi J, Zhou Y, Liu T, Zhan F, Zhang K, et al. Integrating proteomics and transcriptomics for the identification of potential targets in early colorectal cancer. Int J Oncol (2019) 55(2):439–50. doi: 10.3892/ijo.2019.4833
12. Li K, Du Y, Li L, Wei D-Q. Bioinformatics approaches for anti-cancer drug discovery. Curr Drug Targets (2020) 21(1):3–17. doi: 10.2174/1389450120666190923162203
13. Baselli GA, Dongiovanni P, Rametta R, Meroni M, Pelusi S, Maggioni M, et al. Liver transcriptomics highlights interleukin-32 as novel nafld-related cytokine and candidate biomarker. Gut (2020) 69(10):1855–66. doi: 10.1136/gutjnl-2019-319226
14. Lisnevskaia L, Murphy GJ, Isenberg D. Systemic lupus erythematosus. Lancet (2014) 384(9957):1878–88. doi: 10.1016/S0140-6736(14)60128-8
15. Cheung-Lee WL, Link AJ. Genome mining for lasso peptides: Past, present, and future. J Ind Microbiol Biotechnol (2019) 46(9-10):1371–9. doi: 10.1007/s10295-019-02197-z
16. Zhao E, Xie H, Zhang YJ. Predicting diagnostic gene biomarkers associated with immune infiltration in patients with acute myocardial infarction. Front Cardiovasc Med (2020) 7. doi: 10.3389/fcvm.2020.586871
17. Bhavani C, Govardhan AJ. Supervised algorithms of machine learning in the prediction of cervical cancer: A comparative analysis. Ann Romanian Soc Cell Biol (2021) 25(5):1380–93. doi: 10.31661/jbpe.v0i0.1912-1027
18. Newman AM, Liu CL, Green MR, Gentles AJ, Feng W, Xu Y, et al. Robust enumeration of cell subsets from tissue expression profiles. Nat Methods (2015) 12(5):453–7. doi: 10.1038/nmeth.3337
19. Lu G, Chen L, Wu S, Feng Y, Lin TJ. Comprehensive analysis of tumor-infiltrating immune cells and relevant therapeutic strategy in esophageal cancer. Dis Markers (2020) 2020:1–12. doi: 10.1155/2020/8974793
20. Chen Z, Wang GS, Wang GH, Li XP. Anti-C1q antibody is a valuable biological marker for prediction of renal pathological characteristics in lupus nephritis. Clin Rheumatol (2012) 31(9):1323–9. doi: 10.1007/s10067-012-2017-1
21. Fang QY, Yu F, Tan Y, Xu LX, Wu LH, Liu G, et al. Anti-C1q antibodies and igg subclass distribution in sera from Chinese patients with lupus nephritis. Nephrol Dial Transplant (2009) 24(1):172–8. doi: 10.1093/ndt/gfn453
22. Cai X, Yang X, Lian F, Lin X, Liang M, Li J, et al. Correlation between serum anti-C1q antibody levels and renal pathological characteristics and prognostic significance of anti-C1q antibody in lupus nephritis. J Rheumatol (2010) 37(4):759–65. doi: 10.3899/jrheum.090779
23. Liu T, Yang M, Xia Y, Jiang C, Li C, Jiang Z, et al. Microarray-based analysis of renal complement components reveals a therapeutic target for lupus nephritis. Arthritis Res Ther (2021) 23(1):223. doi: 10.1186/s13075-021-02605-9
24. Tampe D, Hakroush S, Tampe B. Dissecting signalling pathways associated with intrarenal synthesis of complement components in lupus nephritis. RMD Open (2022) 8(2):e002517. doi: 10.1136/rmdopen-2022-002517
25. Shimizu Y, Yasuda S, Kimura T, Nishio S, Kono M, Ohmura K, et al. Interferon-inducible Mx1 protein is highly expressed in renal tissues from treatment-naïve lupus nephritis, but not in those under immunosuppressive treatment. Mod Rheumatol (2018) 28(4):661–9. doi: 10.1080/14397595.2017.1404711
26. Floege J, Burg M, Al Masri AN, Gröne HJ, von Wussow P. Expression of interferon-inducible mx-proteins in patients with iga nephropathy or henoch-schönlein purpura. Am J Kidney Dis (1999) 33(3):434–40. doi: 10.1016/S0272-6386(99)70179-4
27. Feng X, Wu H, Grossman JM, Hanvivadhanakul P, FitzGerald JD, Park GS, et al. Association of increased interferon-inducible gene expression with disease activity and lupus nephritis in patients with systemic lupus erythematosus. Arthritis Rheum (2006) 54(9):2951–62. doi: 10.1002/art.22044
28. Watanabe S, Imaizumi T, Tsuruga K, Aizawa T, Ito T, Matsumiya T, et al. Glomerular expression of myxovirus resistance protein 1 in human mesangial cells: Possible activation of innate immunity in the pathogenesis of lupus nephritis. Nephrol (Carlton) (2013) 18(12):833–7. doi: 10.1111/nep.12155
29. Potlukova E, Kralikova P. Complement component C1q and anti-C1q antibodies in theory and in clinical practice. Scand J Immunol (2008) 67(5):423–30. doi: 10.1111/j.1365-3083.2008.02089.x
30. Moroni G, Radice A, Giammarresi G, Quaglini S, Gallelli B, Leoni A, et al. Are laboratory tests useful for monitoring the activity of lupus nephritis? a 6-year prospective study in a cohort of 228 patients with lupus nephritis. Ann Rheum Dis (2009) 68(2):234–7. doi: 10.1136/ard.2008.094508
31. Akhter E, Burlingame RW, Seaman AL, Magder L, Petri M. Anti-C1q antibodies have higher correlation with flares of lupus nephritis than other serum markers. Lupus (2011) 20(12):1267–74. doi: 10.1177/0961203311411597
32. Matrat A, Veysseyre-Balter C, Trolliet P, Villar E, Dijoud F, Bienvenu J, et al. Simultaneous detection of anti-C1q and anti-double stranded DNA autoantibodies in lupus nephritis: Predictive value for renal flares. Lupus (2011) 20(1):28–34. doi: 10.1177/0961203310379871
33. Pang Y, Tan Y, Li Y, Zhang J, Guo Y, Guo Z, et al. Serum A08 C1q antibodies are associated with disease activity and prognosis in Chinese patients with lupus nephritis. Kidney Int (2016) 90(6):1357–67. doi: 10.1016/j.kint.2016.08.010
34. Pan HF, Leng RX, Feng CC, Li XP, Chen GM, Li BZ, et al. Expression profiles of Th17 pathway related genes in human systemic lupus erythematosus. Mol Biol Rep (2013) 40(1):391–9. doi: 10.1007/s11033-012-2073-2
35. Brunner HI, Mueller M, Rutherford C, Passo MH, Witte D, Grom A, et al. Urinary neutrophil gelatinase-associated lipocalin as a biomarker of nephritis in childhood-onset systemic lupus erythematosus. Arthritis Rheum (2006) 54(8):2577–84. doi: 10.1002/art.22008
36. Midgley A, Beresford MW. Increased expression of low density granulocytes in juvenile-onset systemic lupus erythematosus patients correlates with disease activity. Lupus (2016) 25(4):407–11. doi: 10.1177/0961203315608959
37. Jourde-Chiche N, Whalen E, Gondouin B, Speake C, Gersuk V, Dussol B, et al. Modular transcriptional repertoire analyses identify a blood neutrophil signature as a candidate biomarker for lupus nephritis. Rheumatol (Oxford) (2017) 56(3):477–87. doi: 10.1093/rheumatology/kew439
38. Bennett L, Palucka AK, Arce E, Cantrell V, Borvak J, Banchereau J, et al. Interferon and granulopoiesis signatures in systemic lupus erythematosus blood. J Exp Med (2003) 197(6):711–23. doi: 10.1084/jem.20021553
39. Banchereau J, Pascual V. Type I interferon in systemic lupus erythematosus and other autoimmune diseases. Immunity (2006) 25(3):383–92. doi: 10.1016/j.immuni.2006.08.010
40. Kirou KA, Lee C, George S, Louca K, Peterson MG, Crow MK. Activation of the interferon-alpha pathway identifies a subgroup of systemic lupus erythematosus patients with distinct serologic features and active disease. Arthritis Rheum (2005) 52(5):1491–503. doi: 10.1002/art.21031
41. Marto N, Bertolaccini ML, Calabuig E, Hughes GR, Khamashta MA. Anti-C1q antibodies in nephritis: Correlation between titres and renal disease activity and positive predictive value in systemic lupus erythematosus. Ann Rheum Dis (2005) 64(3):444–8. doi: 10.1136/ard.2004.024943
42. Moroni G, Trendelenburg M, Del Papa N, Quaglini S, Raschi E, Panzeri P, et al. Anti-C1q antibodies may help in diagnosing a renal flare in lupus nephritis. Am J Kidney Dis (2001) 37(3):490–8. doi: 10.1053/ajkd.2001.22071
43. Siegert CE, Daha MR, Halma C, van der Voort EA, Breedveld FC. Igg and iga autoantibodies to C1q in systemic and renal diseases. Clin Exp Rheumatol (1992) 10(1):19–23.
44. Trendelenburg M, Marfurt J, Gerber I, Tyndall A, Schifferli JA. Lack of occurrence of severe lupus nephritis among anti-C1q autoantibody-negative patients. Arthritis Rheum (1999) 42(1):187–8. doi: 10.1002/1529-0131(199901)42:1<187::AID-ANR24>3.0.CO;2-U
45. Ravelli A, Wisnieski JJ, Ramenghi B, Ballardini G, Zonta L, Martini A. Igg autoantibodies to complement C1q in pediatric-onset systemic lupus erythematosus. Clin Exp Rheumatol (1997) 15(2):215–9. doi: 10.1002/art.1780400335
46. Mahler M, van Schaarenburg RA, Trouw LA. Anti-C1q autoantibodies, novel tests, and clinical consequences. Front Immunol (2013) 4:117. doi: 10.3389/fimmu.2013.00117
47. Horák P, Hermanová Z, Zadrazil J, Ciferská H, Ordeltová M, Kusá L, et al. C1q complement component and -antibodies reflect sle activity and kidney involvement. Clin Rheumatol (2006) 25(4):532–6. doi: 10.1007/s10067-005-0110-4
48. Siegert CE, Daha MR, Tseng CM, Coremans IE, van Es LA, Breedveld FC. Predictive value of igg autoantibodies against C1q for nephritis in systemic lupus erythematosus. Ann Rheum Dis (1993) 52(12):851–6. doi: 10.1136/ard.52.12.851
49. Coremans IE, Spronk PE, Bootsma H, Daha MR, van der Voort EA, Kater L, et al. Changes in antibodies to C1q predict renal relapses in systemic lupus erythematosus. Am J Kidney Dis (1995) 26(4):595–601. doi: 10.1016/0272-6386(95)90595-2
50. El-Karaksy SM, Raafat HA, Abadir MN, Hanna MO. Down-regulation of expression of retinoid acid-related orphan receptor c (Rorc) in systemic lupus erythematosus. J Receptor Signal Transduct Res (2016) 36(2):207–12. doi: 10.3109/10799893.2015.1075042
51. Baechler EC, Batliwalla FM, Karypis G, Gaffney PM, Ortmann WA, Espe KJ, et al. Interferon-inducible gene expression signature in peripheral blood cells of patients with severe lupus. Proc Natl Acad Sci USA (2003) 100(5):2610–5. doi: 10.1073/pnas.0337679100
52. Crow MK. Interferon pathway activation in systemic lupus erythematosus. Curr Rheumatol Rep (2005) 7(6):463–8. doi: 10.1007/s11926-005-0053-4
53. Rönnblom L, Alm GV. Systemic lupus erythematosus and the type I interferon system. Arthritis Res Ther (2003) 5(2):68–75. doi: 10.1186/ar625
54. Nangaku M, Couser WG. Mechanisms of immune-deposit formation and the mediation of immune renal injury. Clin Exp Nephrol (2005) 9(3):183–91. doi: 10.1007/s10157-005-0357-8
55. Tsirogianni A, Pipi E, Soufleros K. Relevance of anti-C1q autoantibodies to lupus nephritis. Ann New York Acad Sci (2009) 1173:243–51. doi: 10.1111/j.1749-6632.2009.04750.x
56. Botto M, Walport MJ. C1q, autoimmunity and apoptosis. Immunobiology (2002) 205(4-5):395–406. doi: 10.1078/0171-2985-00141
57. Walport MJ, Davies KA, Botto M. C1q and systemic lupus erythematosus. Immunobiology (1998) 199(2):265–85. doi: 10.1016/S0171-2985(98)80032-6
58. Walport MJ. Complement. second of two parts. New Engl J Med (2001) 344(15):1140–4. doi: 10.1056/NEJM200104123441506
59. Pang Y, Yang XW, Song Y, Yu F, Zhao MH. Anti-C1q autoantibodies from active lupus nephritis patients could inhibit the clearance of apoptotic cells and complement classical pathway activation mediated by C1q in vitro. Immunobiology (2014) 219(12):980–9. doi: 10.1016/j.imbio.2014.07.004
60. Kishore U, Gaboriaud C, Waters P, Shrive AK, Greenhough TJ, Reid KB, et al. C1q and tumor necrosis factor superfamily: Modularity and versatility. Trends Immunol (2004) 25(10):551–61. doi: 10.1016/j.it.2004.08.006
61. Rönnblom L, Alm GV, Eloranta ML. The type I interferon system in the development of lupus. Semin Immunol (2011) 23(2):113–21. doi: 10.1016/j.smim.2011.01.009
62. Rönnblom L, Eloranta ML, Alm GV. The type I interferon system in systemic lupus erythematosus. Arthritis Rheum (2006) 54(2):408–20. doi: 10.1002/art.21571
63. Tang Y, Luo X, Cui H, Ni X, Yuan M, Guo Y, et al. Microrna-146a contributes to abnormal activation of the type I interferon pathway in human lupus by targeting the key signaling proteins. Arthritis Rheum (2009) 60(4):1065–75. doi: 10.1002/art.24436
64. Baechler EC, Gregersen PK, Behrens TW. The emerging role of interferon in human systemic lupus erythematosus. Curr Opin Immunol (2004) 16(6):801–7. doi: 10.1016/j.coi.2004.09.014
65. Woods MW, Kelly JN, Hattlmann CJ, Tong JG, Xu LS, Coleman MD, et al. Human Herc5 restricts an early stage of hiv-1 assembly by a mechanism correlating with the isgylation of gag. Retrovirology (2011) 8(1):1–18. doi: 10.1186/1742-4690-8-95
66. Kościelska-Kasprzak K, Bartoszek D, Myszka M, Zabińska M, Klinger M. The complement cascade and renal disease. Archivum Immunol Ther Experimentalis (Warsz) (2014) 62(1):47–57. doi: 10.1007/s00005-013-0254-x
67. Emad G, Al-Barshomy SM. Anti-C1q antibodies in lupus nephritis and their correlation with the disease activity. Saudi J Kidney Kiseases Transplant (2020) 31(2):342–52. doi: 10.4103/1319-2442.284008
68. Sinico RA, Radice A, Ikehata M, Giammarresi G, Corace C, Arrigo G, et al. Anti-C1q autoantibodies in lupus nephritis: Prevalence and clinical significance. Ann New York Acad Sci (2005) 1050:193–200. doi: 10.1196/annals.1313.020
69. Trendelenburg M, Lopez-Trascasa M, Potlukova E, Moll S, Regenass S, Frémeaux-Bacchi V, et al. High prevalence of anti-C1q antibodies in biopsy-proven active lupus nephritis. Nephrol Dial Transplant (2006) 21(11):3115–21. doi: 10.1093/ndt/gfl436
70. Yang XW, Tan Y, Yu F, Zhao MH. Combination of anti-C1q and anti-dsdna antibodies is associated with higher renal disease activity and predicts renal prognosis of patients with lupus nephritis. Nephrol Dial Transplant (2012) 27(9):3552–9. doi: 10.1093/ndt/gfs179
71. Toomayan GA, Chen LE, Jiang HX, Qi WN, Seaber AV, Frank MM, et al. C1-esterase inhibitor and a novel peptide inhibitor improve contractile function in reperfused skeletal muscle. Microsurgery (2003) 23(6):561–7. doi: 10.1002/micr.10210
72. Flierman R, Daha MR. Pathogenic role of anti-C1q autoantibodies in the development of lupus nephritis–a hypothesis. Mol Immunol (2007) 44(1-3):133–8. doi: 10.1016/j.molimm.2006.06.010
73. Pettigrew HD, Teuber SS, Gershwin ME. Clinical significance of complement deficiencies. Ann New York Acad Sci (2009) 1173:108–23. doi: 10.1111/j.1749-6632.2009.04633.x
74. Pickering MC, Botto M, Taylor PR, Lachmann PJ, Walport MJ. Systemic lupus erythematosus, complement deficiency, and apoptosis. Adv Immunol (2000) 76:227–324. doi: 10.1016/S0065-2776(01)76021-X
75. Roumenina LT, Sène D, Radanova M, Blouin J, Halbwachs-Mecarelli L, Dragon-Durey MA, et al. Functional complement C1q abnormality leads to impaired immune complexes and apoptotic cell clearance. J Immunol (2011) 187(8):4369–73. doi: 10.4049/jimmunol.1101749
76. Yap DY, Lai KN. The role of cytokines in the pathogenesis of systemic lupus erythematosus - from bench to bedside. Nephrol (Carlton) (2013) 18(4):243–55. doi: 10.1111/nep.12047
77. Feng X, Huang J, Liu Y, Xiao L, Wang D, Hua B, et al. Identification of interferon-inducible genes as diagnostic biomarker for systemic lupus erythematosus. Clin Rheumatol (2015) 34(1):71–9. doi: 10.1007/s10067-014-2799-4
78. Nielsen CT, Lood C, Ostergaard O, Iversen LV, Voss A, Bengtsson A, et al. Plasma levels of galectin-3-Binding protein reflect type I interferon activity and are increased in patients with systemic lupus erythematosus. Lupus Sci Med (2014) 1(1):e000026. doi: 10.1136/lupus-2014-000026
79. Huang YH, Lo MH, Cai XY, Liu SF, Kuo HC. Increase expression of Cd177 in Kawasaki disease. Pediatr Rheumatol Online J (2019) 17(1):13. doi: 10.1186/s12969-019-0315-8
80. Hu N, Westra J, Huitema MG, Bijl M, Brouwer E, Stegeman CA, et al. Coexpression of Cd177 and membrane proteinase 3 on neutrophils in antineutrophil cytoplasmic autoantibody-associated systemic vasculitis: Anti-proteinase 3-mediated neutrophil activation is independent of the role of Cd177-expressing neutrophils. Arthritis Rheum (2009) 60(5):1548–57. doi: 10.1002/art.24442
81. Lalezari P, Murphy GB, Allen FH Jr. Nb1, a new neutrophil-specific antigen involved in the pathogenesis of neonatal neutropenia. J Clin Invest (1971) 50(5):1108–15. doi: 10.1172/JCI106582
82. Kennedy WP, Maciuca R, Wolslegel K, Tew W, Abbas AR, Chaivorapol C, et al. Association of the interferon signature metric with serological disease manifestations but not global activity scores in multiple cohorts of patients with sle. Lupus Sci Med (2015) 2(1):e000080. doi: 10.1136/lupus-2014-000080
83. Siddiqi KZ, Zinglersen AH, Iversen KK, Rasmussen NS, Nielsen CT, Jacobsen S. A cluster of type ii interferon-regulated genes associates with disease activity in patients with systemic lupus erythematosus. J Autoimmun (2022) 132:102869. doi: 10.1016/j.jaut.2022.102869
84. van Schaarenburg RA, Magro-Checa C, Bakker JA, Teng YK, Bajema IM, Huizinga TW, et al. C1q deficiency and neuropsychiatric systemic lupus erythematosus. Front Immunol (2016) 7:647. doi: 10.3389/fimmu.2016.00647
85. Mehta P, Norsworthy PJ, Hall AE, Kelly SJ, Walport MJ, Botto M, et al. Sle with C1q deficiency treated with fresh frozen plasma: A 10-year experience. Rheumatol (Oxford) (2010) 49(4):823–4. doi: 10.1093/rheumatology/kep387
86. Ekinci Z, Ozturk K. Systemic lupus erythematosus with C1q deficiency: Treatment with fresh frozen plasma. Lupus (2018) 27(1):134–8. doi: 10.1177/0961203317741565
87. Borba HH, Wiens A, de Souza TT, Correr CJ, Pontarolo R. Efficacy and safety of biologic therapies for systemic lupus erythematosus treatment: Systematic review and meta-analysis. Biodrugs (2014) 28(2):211–28. doi: 10.1007/s40259-013-0074-x
88. Yao Y, Higgs BW, Richman L, White B, Jallal B. Use of type I interferon-inducible mrnas as pharmacodynamic markers and potential diagnostic markers in trials with sifalimumab, an anti-ifnα antibody, in systemic lupus erythematosus. Arthritis Res Ther (2010) 12(1):1–7. doi: 10.1186/ar2887
89. Huh JR, Leung MW, Huang P, Ryan DA, Krout MR, Malapaka RR, et al. Digoxin and its derivatives suppress Th17 cell differentiation by antagonizing rorγt activity. Nature (2011) 472(7344):486–90. doi: 10.1038/nature09978
90. Solt LA, Kumar N, Nuhant P, Wang Y, Lauer JL, Liu J, et al. Suppression of Th17 differentiation and autoimmunity by a synthetic ror ligand. Nature (2011) 472(7344):491–4. doi: 10.1038/nature10075
91. Xiao S, Yosef N, Yang J, Wang Y, Zhou L, Zhu C, et al. Small-molecule rorγt antagonists inhibit T helper 17 cell transcriptional network by divergent mechanisms. Immunity (2014) 40(4):477–89. doi: 10.1016/j.immuni.2014.04.004
92. Huang Z, Xie H, Wang R, Sun Z. Retinoid-related orphan receptor gamma T is a potential therapeutic target for controlling inflammatory autoimmunity. Expert Opin TherapeuticTargets (2007) 11(6):737–43. doi: 10.1517/14728222.11.6.737
93. Jetten AM, Ueda E. Retinoid-related orphan receptors (Rors): Roles in cell survival, differentiation and disease. Cell Death Differ (2002) 9(11):1167–71. doi: 10.1038/sj.cdd.4401085
94. Bronner SM, Zbieg JR, Crawford JJ. Rorγ antagonists and inverse agonists: A patent review. Expert Opin Ther Patents (2017) 27(1):101–12. doi: 10.1080/13543776.2017.1236918
95. Huh JR, Englund EE, Wang H, Huang R, Huang P, Rastinejad F, et al. Identification of potent and selective diphenylpropanamide rorγ inhibitors. ACS Med Chem Lett (2013) 4(1):79–84. doi: 10.1021/ml300286h
96. Kojetin DJ, Burris TP. Rev-erb and ror nuclear receptors as drug targets. Nat Rev Drug Discovery (2014) 13(3):197–216. doi: 10.1038/nrd4100
97. Knight JS, Zhao W, Luo W, Subramanian V, O'Dell AA, Yalavarthi S, et al. Peptidylarginine deiminase inhibition is immunomodulatory and vasculoprotective in murine lupus. J Clin Invest (2013) 123(7):2981–93. doi: 10.1172/JCI67390
98. Knight JS, Subramanian V, O'Dell AA, Yalavarthi S, Zhao W, Smith CK, et al. Peptidylarginine deiminase inhibition disrupts net formation and protects against kidney, skin and vascular disease in lupus-prone Mrl/Lpr mice. Ann Rheum Dis (2015) 74(12):2199–206. doi: 10.1136/annrheumdis-2014-205365
99. Keeling DM, Isenberg DA. Haematological manifestations of systemic lupus erythematosus. Blood Rev (1993) 7(4):199–207. doi: 10.1016/0268-960X(93)90006-P
100. Carli L, Tani C, Vagnani S, Signorini V, Mosca M. Leukopenia, lymphopenia, and neutropenia in systemic lupus erythematosus: Prevalence and clinical impact–a systematic literature review. Semin Arthritis Rheum (2015) 45(2):190–4. doi: 10.1016/j.semarthrit.2015.05.009
101. Anolik JH, Barnard J, Cappione A, Pugh-Bernard AE, Felgar RE, Looney RJ, et al. Rituximab improves peripheral b cell abnormalities in human systemic lupus erythematosus. Arthritis Rheum (2004) 50(11):3580–90. doi: 10.1002/art.20592
102. Furie R, Khamashta M, Merrill JT, Werth VP, Kalunian K, Brohawn P, et al. Anifrolumab, an anti-Interferon-A receptor monoclonal antibody, in moderate-to-Severe systemic lupus erythematosus. Arthritis Rheumatol (2017) 69(2):376–86. doi: 10.1002/art.39962
103. Khamashta M, Merrill JT, Werth VP, Furie R, Kalunian K, Illei GG, et al. Sifalimumab, an anti-Interferon-A monoclonal antibody, in moderate to severe systemic lupus erythematosus: A randomised, double-blind, placebo-controlled study. Ann Rheum Dis (2016) 75(11):1909–16. doi: 10.1136/annrheumdis-2015-208562
104. Kalunian KC, Merrill JT, Maciuca R, McBride JM, Townsend MJ, Wei X, et al. A phase ii study of the efficacy and safety of rontalizumab (Rhumab interferon-A) in patients with systemic lupus erythematosus (Rose). Ann Rheum Dis (2016) 75(1):196–202. doi: 10.1136/annrheumdis-2014-206090
105. Tektonidou MG, Dasgupta A, Ward MM. Risk of end-stage renal disease in patients with lupus nephritis, 1971–2015: A systematic review and Bayesian meta-analysis. Arthritis Rheumatol (2016) 68(6):1432–41. doi: 10.1002/art.39594
106. Arriens C, Wren JD, Munroe ME, Mohan C. Systemic lupus erythematosus biomarkers: The challenging quest. Rheumatol (Oxford England) (2017) 56(suppl_1):i32–45. doi: 10.1093/rheumatology/kew407
107. Song D, Guo W-y, Wang F-M, Li Y-Z, Song Y, Yu F, et al. Complement alternative Pathway' s activation in patients with lupus nephritis. Am J Med Sci (2017) 353(3):247–57. doi: 10.1016/j.amjms.2017.01.005
108. Ritchie RF, Palomaki GE, Neveux LM, Navolotskaia O, Ledue TB, Craig WY. Reference distributions for complement proteins C3 and C4: A practical, simple and clinically relevant approach in a Large cohort. J Clin Lab Anal (2004) 18(1):1–8. doi: 10.1002/jcla.10100
109. Bigler C, Lopez-Trascasa M, Potlukova E, Moll S, Danner D, Schaller M, et al. Antinucleosome antibodies as a marker of active proliferative lupus nephritis. Am J Kidney Dis (2008) 51(4):624–9. doi: 10.1053/j.ajkd.2007.10.041
110. Ho A, Magder LS, Barr SG, Petri MJ. Decreases in anti–Double-Stranded DNA levels are associated with concurrent flares in patients with systemic lupus erythematosus. Arthritis Rheum (2001) 44(10):2342–9. doi: 10.1002/1529-0131(200110)44:10<2342::AID-ART397>3.0.CO;2-8
111. Ramanujam M, Steffgen J, Visvanathan S, Mohan C, Fine JS, Putterman C. Phoenix from the flames: Rediscovering the role of the Cd40–Cd40l pathway in systemic lupus erythematosus and lupus nephritis. Autoimmun Rev (2020) 19(11):102668. doi: 10.1016/j.autrev.2020.102668
112. Ding X, Ren Y, He XJ. Ifn-I mediates lupus nephritis from the beginning to renal fibrosis. Front Immunol (2021) 12:1359. doi: 10.3389/fimmu.2021.676082
113. Frangou E, Georgakis S, Bertsias G. Update on the cellular and molecular aspects of lupus nephritis. Clin Immunol (2020) 216:108445. doi: 10.1016/j.clim.2020.108445
114. Sekine H, Watanabe H, Gilkeson GS. Enrichment of anti-glomerular antigen antibody-producing cells in the kidneys of Mrl/Mpj-faslpr mice. J Immunol (2004) 172(6):3913–21. doi: 10.4049/jimmunol.172.6.3913
115. Foster MH. T Cells and b cells in lupus nephritis. Semin Nephrol (2007) 27(1):47–58. doi: 10.1016/j.semnephrol.2006.09.007
116. Spada R, Rojas JM, Perez-Yague S, Mulens V, Cannata-Ortiz P, Bragado R, et al. Nkg2d ligand overexpression in lupus nephritis correlates with increased nk cell activity and differentiation in kidneys but not in the periphery. J Leukocyte Biol (2015) 97(3):583–98. doi: 10.1189/jlb.4A0714-326R
117. Mohan C, Putterman C. Genetics and pathogenesis of systemic lupus erythematosus and lupus nephritis. Nat Rev Nephrol (2015) 11(6):329–41. doi: 10.1038/nrneph.2015.33
118. Xu R, Li Q, Liu R, Shen J, Li M, Zhao M, et al. Association analysis of the mhc in lupus nephritis. J Am Soc Nephrol (2017) 28(11):3383–94. doi: 10.1681/ASN.2016121331
119. Arnett F, Olsen M, Anderson K, Reveille JJ. Molecular analysis of major histocompatibility complex alleles associated with the lupus anticoagulant. J Clin Invest (1991) 87(5):1490–5. doi: 10.1172/JCI115158
120. Fabriek BO, van Bruggen R, Deng DM, Ligtenberg AJ, Nazmi K, Schornagel K, et al. The macrophage scavenger receptor Cd163 functions as an innate immune sensor for bacteria. Blood (2009) 113(4):887–92. doi: 10.1182/blood-2008-07-167064
121. Van Gorp H, Delputte PL, Nauwynck HJ. Scavenger receptor CD163, a Jack-of-all-trades and potential target for cell-directed therapy. Mol Immunol (2010) 47(7-8):1650–60. doi: 10.1016/j.molimm.2010.02.008
122. Onofre G, Kolácková M, Jankovicova K, Krejsek J. Scavenger receptor Cd163 and its biological functions. Acta Med (Hradec Kralove) (2009) 52(2):57–61. doi: 10.14712/18059694.2016.105
123. Pengbo X, Liguo Y, Hongsheng S. Research progress on the relationship between Cd163 and systemic lupus erythematosus. Chin J Clin Res (2019) 32(12):1724–6. doi: 10.13429/j.cnki.cjcr.2019.12.030
124. Olmes G, Büttner-Herold M, Ferrazzi F, Distel L, Amann K, Daniel C, et al. CD163+ M2c-like macrophages predominate in renal biopsies from patients with lupus nephritis. Arthritis Res Ther (2016) 18(1):1–16. doi: 10.1186/s13075-016-0989-y
125. Endo N, Tsuboi N, Furuhashi K, Shi Y, Du Q, Abe T, et al. Urinary soluble Cd163 level reflects glomerular inflammation in human lupus nephritis. J Clin Invest (2016) 31(12):2023–33. doi: 10.1093/ndt/gfw214
126. Arazi A, Rao DA, Berthier CC, Davidson A, Liu Y, Hoover PJ, et al. The immune cell landscape in kidneys of patients with lupus nephritis. Nat Immunol (2019) 20(7):902–14. doi: 10.1038/s41590-019-0398-x
127. Kejing T. Fractalkine/Cx3cr1 in the pathogenesis of lupus nephritis. Guangdong: Sun Yat-Sen University (2004).
128. Inoue A, Hasegawa H, Kohno M, Ito MR, Terada M, Imai T, et al. Antagonist of fractalkine (Cx3cl1) delays the initiation and ameliorates the progression of lupus nephritis in Mrl/Lpr mice. Arthritis Rheum (2005) 52(5):1522–33. doi: 10.1002/art.21007
129. Bao L, Haas M, Quigg RJ. Complement factor h deficiency accelerates development of lupus nephritis. J Am Soc Nephrol (2011) 22(2):285–95. doi: 10.1681/ASN.2010060647
130. Zhao J, Wu H, Khosravi M, Cui H, Qian X, Kelly JA, et al. Association of genetic variants in complement factor h and factor h-related genes with systemic lupus erythematosus susceptibility. PloS Genet (2011) 7(5):e1002079. doi: 10.1371/journal.pgen.1002079
131. Siddiqi KZ, Wilhelm TR, Ulff-Møller CJ, Jacobsen S. Cluster of highly expressed interferon-stimulated genes associate more with African ancestry than disease activity in patients with systemic lupus erythematosus. a systematic review of cross-sectional studies. Trans Res (2021) 238:63–75. doi: 10.1016/j.trsl.2021.07.006
132. Zhao M, Zhou Y, Zhu B, Wan M, Jiang T, Tan Q, et al. IFI44L promoter methylation as a blood biomarker for systemic lupus erythematosus. Ann Rheum Dis (2016) 75(11):1998–2006. doi: 10.1136/annrheumdis-2015-208410
133. Mei X, Jin H, Zhao M, Lu Q. Association of immune-related genetic and epigenetic alterations with lupus nephritis. Kidney Dis (2022) 8(4):286–96. doi: 10.1159/000524937
134. Shen L, Lan L, Zhu T, Chen H, Gu H, Wang C, et al. Identification and validation of Ifi44 as key biomarker in lupus nephritis. Front Med (2021) 8:1937. doi: 10.3389/fmed.2021.762848
135. Ding Y, Li H, Xu L, Wang Y, Yang HJ. Identification and validation of prognostic biomarkers specifically expressed in macrophage in iga nephropathy patients based on integrated bioinformatics analyses. Front Mol Biosci (2022) 9. doi: 10.3389/fmolb.2022.884588
136. Saraiva M, Vieira P, O’garra AJ. Biology and therapeutic potential of interleukin-10. J Exp Med (2020) 217(1):e20190418. doi: 10.1084/jem.20190418
137. Xiaolan C ed. Expression and significance of il-10 in peripheral blood mononuclear cells and renal tissue of patients with lupus nephritis. In: "2004 annual meeting of nephrology branch of Chinese medical association" and "The second national academic conference on nephrology for middle-aged and young people". (Hangzhou, China: Medical and Health Sciences).
138. Lou Q-Y, Li Z, Teng Y, Xie Q-M, Zhang M, Huang S-W, et al. Associations of FKBP4 and FKBP5 gene polymorphisms with disease susceptibility, glucocorticoid efficacy, anxiety, depression, and health-related quality of life in systemic lupus erythematosus patients. Clin Rheumatol (2021) 40(1):167–79. doi: 10.1007/s10067-020-05195-0
139. Scharer CD, Blalock EL, Mi T, Barwick BG, Jenks SA, Deguchi T, et al. Epigenetic programming underpins b cell dysfunction in human sle. Nat Immunol (2019) 20(8):1071–82. doi: 10.1038/s41590-019-0419-9
140. Lange U, Saitou M, Western P, Barton S, Surani MJ. The fragilis interferon-inducible gene family of transmembrane proteins is associated with germ cell specification in mice. BMC Dev Biol (2003) 3(1):1–11. doi: 10.1186/1471-213x-3-1
141. Lewin AR, Reid LE, McMahon M, Stark GR, Kerr IMJ. Molecular analysis of a human interferon-inducible gene family. Eur J Biochem (1991) 199(2):417–23. doi: 10.1111/j.1432-1033.1991.tb16139.x
142. Evans SS, Collea RP, Appenheimer MM, Gollnick SO. Interferon-alpha induces the expression of the l-selectin homing receptor in human b lymphoid cells. J Cell Biol (1993) 123(6):1889–98. doi: 10.1083/jcb.123.6.1889
143. Andreu P, Colnot S, Godard C, Laurent-Puig P, Lamarque D, Kahn A, et al. Identification of the ifitm family as a new molecular marker in human colorectal tumors. Cancer Res (2006) 66(4):1949–55. doi: 10.1158/0008-5472.CAN-05-2731
144. Chen W, Li W, Zhang Z, Tang X, Wu S, Yao G, et al. Lipocalin-2 exacerbates lupus nephritis by promoting Th1 cell differentiation. J Am Soc Nephrol (2020) 31(10):2263–77. doi: 10.1681/ASN.2019090937
145. Schwartz N, Michaelson JS, Putterman C. Lipocalin-2, tweak, and other cytokines as urinary biomarkers for lupus nephritis. Ann New York Acad Sci (2007) 1109(1):265–74. doi: 10.1196/annals.1398.032
146. Pitashny M, Schwartz N, Qing X, Hojaili B, Aranow C, Mackay M, et al. Urinary lipocalin-2 is associated with renal disease activity in human lupus nephritis. Arthritis Rheum (2007) 56(6):1894–903. doi: 10.1002/art.22594
147. Mok A, Solomon O, Nayak RR, Coit P, Quach HL, Nititham J, et al. Genome-wide profiling identifies associations between lupus nephritis and differential methylation of genes regulating tissue hypoxia and type 1 interferon responses. Lupus Sci Med (2016) 3(1):e000183. doi: 10.1136/lupus-2016-000183
148. Grande JP. Mechanisms of progression of renal damage in lupus nephritis: Pathogenesis of renal scarring. Lupus (1998) 7(9):604–10. doi: 10.1191/096120398678920721
149. Arbuckle MR, McClain MT, Rubertone MV, Scofield RH, Dennis GJ, James JA, et al. Development of autoantibodies before the clinical onset of systemic lupus erythematosus. New Engl J Med (2003) 349(16):1526–33. doi: 10.1056/NEJMoa021933
150. Shrivastav M, Niewold TB. Nucleic acid sensors and type I interferon production in systemic lupus erythematosus. Front Immunol (2013) 4:319. doi: 10.3389/fimmu.2013.00319
151. Liu Y, Kaplan MJ. Cardiovascular disease in systemic lupus erythematosus: An update. Curr Opin Rheumatol (2018) 30(5):441–8. doi: 10.1097/BOR.0000000000000528
152. Yap DY, Tang CS, Ma MK, Lam MF, Chan TM. Survival analysis and causes of mortality in patients with lupus nephritis. Nephrol Dialysis Transplant (2012) 27(8):3248–54. doi: 10.1093/ndt/gfs073
153. Beyan E, Beyan C, Turan MJH. Hematological presentation in systemic lupus erythematosus and its relationship with disease activity. Hematology (2007) 12(3):257–61. doi: 10.1080/10245330701214145
154. Sharma M, Das HJ, Doley PK, Mahanta PJ. Clinical and histopathological profile of lupus nephritis and response to treatment with cyclophosphamide: A single center study. Saudi J Kidney Dis Transplant (2019) 30(2):501. doi: 10.4103/1319-2442.256857
155. Wen L, li H, Qiu Z, Yi-Bo S, Ming-Gong Y. Lupus nephritis bone mineral density after prednisone treatment and changes in bone metabolism indicators. Chin J Rheumatol (2002) 06):420–3.
156. Ramsey-Goldman R, Dunn JE, Huang CF, Dunlop D, Rairie JE, Fitzgerald S, et al. Frequency of fractures in women with systemic lupus erythematosus: Comparison with united states population data. Arthritis Rheum (1999) 42(5):882–90. doi: 10.1002/1529-0131(199905)42:5<882::AID-ANR6>3.0.CO;2-C
157. Sari R, Taysi S, Erdem F, Yilmaz Ö, Keleş S, Kiziltunç A, et al. Correlation of serum levels of soluble intercellular adhesion molecule-1 with disease activity in systemic lupus erythematosus. Rheumatol Int (2002) 21(4):149–52. doi: 10.1007/s00296-001-0159-6
158. Nakatani K, Fujii H, Hasegawa H, Terada M, Arita N, Ito MR, et al. Endothelial adhesion molecules in glomerular lesions: Association with their severity and diversity in lupus models. Kidney Int (2004) 65(4):1290–300. doi: 10.1111/j.1523-1755.2004.00537.x
159. Wuthrich RP, Snyder TL. Vascular cell adhesion molecule-1 (Vcam-1) expression in murine lupus nephritis. Kidney Int (1992) 42(4):903–14. doi: 10.1038/ki.1992.367
160. Parodis I, Gokaraju S, Zickert A, Vanarsa K, Zhang T, Habazi D, et al. Alcam and vcam-1 as urine biomarkers of activity and long-term renal outcome in systemic lupus erythematosus. Rheumatol (Oxford England) (2020) 59(9):2237–49. doi: 10.1093/rheumatology/kez528
161. Sabry A, Sheashaa H, El-Husseini A, El-Dahshan K, Abdel-Rahim M, Elbasyouni SR. Intercellular adhesion molecules in systemic lupus erythematosus patients with lupus nephritis. Clin Rheumatol (2007) 26(11):1819–23. doi: 10.1007/s10067-007-0580-7
162. Xiang-Mei C, Qi-He X, Li T, Suo-Zhu S, Li-Fang Y. Expression of intercellular adhesion molecule-1 and vascular adhesion molecule-1 in kidney of patients with lupus nephritis and membrano-proliferative glomerulonephritis. Chin J Pathol (1995) 03):149–51.
163. Breedveld F, van der Lubbe PJ. Monoclonal antibody therapy of inflammatory rheumatic diseases. Br Med Bull (1995) 51(2):493–502. doi: 10.1093/oxfordjournals.bmb.a072975
164. Yamazaki T, Seko Y, Tamatani T, Miyasaka M, Yagita H, Okumura K, et al. Expression of intercellular adhesion molecule-1 in rat heart with Ischemia/Reperfusion and limitation of infarct size by treatment with antibodies against cell adhesion molecules. Am J Pathol (1993) 143(2):410. doi: 10.1007/BF02915112
165. Zhao ZQ, Lefer DJ, Sato H, Hart K, Jefforda RR, Vinten-Johansen JJ. Monoclonal antibody to icam-1 preserves postischemic blood flow and reduces infarct size after ischemia-reperfusion in rabbit. J Leukocyte Biol (1997) 62(3):292–300. doi: 10.1002/jlb.62.3.292
166. Pastukhov O, Schwalm S, Römer I, Zangemeister-Wittke U, Pfeilschifter J, Huwiler A, et al. Ceramide kinase contributes to proliferation but not to prostaglandin E2 formation in renal mesangial cells and fibroblasts. Cell Physiol Biochem (2014) 34(1):119–33. doi: 10.1159/000362989
167. Zhou X, Workeneh B, Hu Z, Li RJ. Effect of immunosuppression on the human mesangial cell cycle. Mol Med Rep (2015) 11(2):910–6. doi: 10.3892/mmr.2014.2861
168. Liu C, Zhou L, Cheng Q, Jiang S, Sheng J, Sun J, et al. Effect of bradykinin on renal mesangial cell proliferation and extracellular matrix secretion. Genet Mol Res (2014) 13(1):490–8. doi: 10.4238/2014.January.21.18
169. Hsieh S-C, Tsai C-Y, Yu C-L. Potential serum and urine biomarkers in patients with lupus nephritis and the unsolved problems. Open Access Rheumatol (2016) 8:81. doi: 10.2147/OARRR.S112829
171. Zheng L, Zhang H, Tang Y. In lupus nephritis, how do extracellular dnas trigger type I interferon secretion: Under the assistance of Hmgb1-cgas? Med Hypotheses (2018) 121:51–3. doi: 10.1016/j.mehy.2018.09.015
172. Richez C, Blanco P, Rifkin I, Moreau J-F, Schaeverbeke T. Role for toll-like receptors in autoimmune disease: The example of systemic lupus erythematosus. Joint Bone Spine (2011) 78(2):124–30. doi: 10.1016/j.jbspin.2010.09.005
173. Pisetsky DS. The central role of nucleic acids in the pathogenesis of systemic lupus erythematosus. F1000Research (2019) 8 F1000 Faculty Rev-368. doi: 10.12688/f1000research.17959.1
174. Walhelm T, Gunnarsson I, Heijke R, Leonard D, Trysberg E, Eriksson P, et al. Clinical experience of proteasome inhibitor bortezomib regarding efficacy and safety in severe systemic lupus erythematosus: A nationwide study. Front Immunol (2021) 12. doi: 10.3389/fimmu.2021.756941
175. Bowness P, Davies K, Norsworthy PJ, Athanassiou P, Taylor-Wiedeman J, Borysiewicz LK, et al. Hereditary C1q deficiency and systemic lupus erythematosus. QJM: Int J Med (1994) 87(8):455–64. doi: 10.1093/oxfordjournals.qjmed.a068955
176. Gunnarsson I, Sundelin B, Heimbürger M, Forslid J, van Vollenhoven R, Lundberg I, et al. Repeated renal biopsy in proliferative lupus nephritis–predictive role of serum C1q and albuminuria. J Rheumatol (2002) 29(4):693–9. doi: 10.1097/00124743-200204000-00014
177. Botto M. C1q knock-out mice for the study of complement deficiency in autoimmune disease. Exp Clin Immunogenet (1998) 15(4):231–4. doi: 10.1159/000019076
178. Flodrops H. Contribution of rare and predicted pathogenic gene variants to childhood-onset lupus: A Large, genetic panel analysis of British and French cohorts. Lancet Rheumatol (2020) 2(2):e99–e109. doi: 10.1016/S2665-9913(19)30142-0
179. Morgan BP, Walport MJ. Complement deficiency and disease. Immunol Today (1991) 12(9):301–6. doi: 10.1016/0167-5699(91)90003-C
180. Taylor PR, Carugati A, Fadok VA, Cook HT, Andrews M, Carroll MC, et al. A hierarchical role for classical pathway complement proteins in the clearance of apoptotic cells in vivo. J Exp Med (2000) 192(3):359–66. doi: 10.1084/jem.192.3.359
181. Schejbel L, Skattum L, Hagelberg S, Åhlin A, Schiller B, Berg S, et al. Molecular basis of hereditary C1q deficiency–revisited: Identification of several novel disease-causing mutations. Genes Immun (2011) 12(8):626–34. doi: 10.1038/gene.2011.39
182. Stegert M, Bock M, Trendelenburg M. Clinical presentation of human C1q deficiency: How much of a lupus? Mol Immunol (2015) 67(1):3–11. doi: 10.1016/j.molimm.2015.03.007
183. Mitchell DA, Pickering MC, Warren J, Fossati-Jimack L, Cortes-Hernandez J, Cook HT, et al. C1q deficiency and autoimmunity: The effects of genetic background on disease expression. J Immunol (2002) 168(5):2538–43. doi: 10.4049/jimmunol.168.5.2538
184. Botto M, Dell'Agnola C, Bygrave AE, Thompson EM, Cook HT, Petry F, et al. Homozygous C1q deficiency causes glomerulonephritis associated with multiple apoptotic bodies. Nat Genet (1998) 19(1):56–9. doi: 10.1038/ng0598-56
185. AlFadhli S, AlFailakawi AA, Ghanem AAM. Th-17 related regulatory network in the pathogenesis of Arab patients with systemic lupus erythematosus and lupus nephritis. Int J Rheum Dis (2016) 19(5):512–20. doi: 10.1111/1756-185X.12393
186. Thieblemont N, Wright HL, Edwards SW, Witko-Sarsat V. Human neutrophils in auto-immunity. Semin Immunol (2016) 28(2):159–73. doi: 10.1016/j.smim.2016.03.004
187. AbdGawad M, Gunnarsson L, Bengtsson A, Geborek P, Nilsson L, Segelmark M, et al. Elevated neutrophil membrane expression of proteinase 3 is dependent upon Cd177 expression. Clin Exp Immunol (2010) 161(1):89–97. doi: 10.1111/j.1365-2249.2010.04154.x
188. Sthoeger ZM, Bezalel S, Chapnik N, Asher I, Froy O. High A-defensin levels in patients with systemic lupus erythematosus. Immunology (2009) 127(1):116–22. doi: 10.1111/j.1365-2567.2008.02997.x
189. Villanueva E, Yalavarthi S, Berthier CC, Hodgin JB, Khandpur R, Lin AM, et al. Netting neutrophils induce endothelial damage, infiltrate tissues, and expose immunostimulatory molecules in systemic lupus erythematosus. J Immunol (2011) 187(1):538–52. doi: 10.4049/jimmunol.1100450
190. Giugliano S, Kriss M, Golden-Mason L, Dobrinskikh E, Stone AE, Soto-Gutierrez A, et al. Hepatitis c virus infection induces autocrine interferon signaling by human liver endothelial cells and release of exosomes, which inhibits viral replication. Gastroenterology (2015) 148(2):392–402.e313. doi: 10.1053/j.gastro.2014.10.040
191. Bernheim A, Millman A, Ofir G, Meitav G, Avraham C, Shomar H, et al. Prokaryotic viperins produce diverse antiviral molecules. Nature (2021) 589(7840):120–4. doi: 10.1038/s41586-020-2762-2
192. Mathieu NA, Paparisto E, Barr SD, Spratt DE. HERC5 and the isgylation pathway: Critical modulators of the antiviral immune response. Viruses (2021) 13(6):1102. doi: 10.3390/v13061102
193. Oshiumi H, Kouwaki T, Seya T. Accessory factors of cytoplasmic viral rna sensors required for antiviral innate immune response. Front Immunol (2016) 7:200. doi: 10.3389/fimmu.2016.00200
194. Coit P, Renauer P, Jeffries MA, Merrill JT, McCune WJ, Maksimowicz-McKinnon K, et al. Renal involvement in lupus is characterized by unique DNA methylation changes in naïve Cd4+ T cells. J Autoimmun (2015) 61:29–35. doi: 10.1016/j.jaut.2015.05.003
195. Song K, Liu L, Zhang X, Chen X. An update on genetic susceptibility in lupus nephritis. Clin Immunol (Orlando Fla.) (2020) 210:108272. doi: 10.1016/j.clim.2019.108272
196. Edelbauer M, Kshirsagar S, Riedl M, Billing H, Tönshoff B, Haffner D, et al. Activity of childhood lupus nephritis is linked to altered T cell and cytokine homeostasis. J Clin Immunol (2012) 32(3):477–87. doi: 10.1007/s10875-011-9637-0
197. Okamoto A, Fujio K, Tsuno NH, Takahashi K, Yamamoto K. Kidney-infiltrating Cd4+ T-cell clones promote nephritis in lupus-prone mice. Kidney Int (2012) 82(9):969–79. doi: 10.1038/ki.2012.242
198. Masutani K, Akahoshi M, Tsuruya K, Tokumoto M, Ninomiya T, Kohsaka T, et al. Predominance of Th1 immune response in diffuse proliferative lupus nephritis. Arthritis Rheum (2001) 44(9):2097–106. doi: 10.1002/1529-0131(200109)44:9<2097::AID-ART360>3.0.CO;2-6
199. Couzi L, Merville P, Deminière C, Moreau JF, Combe C, Pellegrin JL, et al. Predominance of Cd8+ T lymphocytes among periglomerular infiltrating cells and link to the prognosis of class iii and class iv lupus nephritis. Arthritis Rheum (2007) 56(7):2362–70. doi: 10.1002/art.22654
200. Abdirama D, Tesch S, Grießbach A-S, von Spee-Mayer C, Humrich JY, Stervbo U, et al. Nuclear antigen–reactive Cd4+ T cells expand in active systemic lupus erythematosus, produce effector cytokines, and invade the kidneys. Kidney Int (2021) 99(1):238–46. doi: 10.1016/j.kint.2020.05.051
201. Lipocalin O, Key I. Op084-Lb6 the comparative study of split-dose of polyethylene glycol (Peg) between low volume peg plus ascorbic acid focusing on the bowel cleansing efficacy, patients’affinity to preparation solution and mucosal injury: A prospective randomized Trial.30. UEG week. In: 2014 oral presentations (Vienna, Austria: United European Gastroenterology Journal) (2014) 30.
202. Alexopoulos E, Seron D, Hartley RB, Cameron JS. Lupus nephritis: Correlation of interstitial cells with glomerular function. Kidney Int (1990) 37(1):100–9. doi: 10.1038/ki.1990.14
203. Caligaris-Cappio F, Bergui L, Tesio L, Ziano R, Camussi G. Hla-dr+ T cells of the leu 3 (Helper) type infiltrate the kidneys of patients with systemic lupus erythematosus. Clin Exp Immunol (1985) 59(1):185–9.
204. D'Agati VD, Appel GB, Estes D, Knowles DM 2nd, Pirani CL. Monoclonal antibody identification of infiltrating mononuclear leukocytes in lupus nephritis. Kidney Int (1986) 30(4):573–81. doi: 10.1038/ki.1986.223
205. Castiglione A, Bucci A, Fellin G, d'Amico G, Atkins RC. The relationship of infiltrating renal leucocytes to disease activity in lupus and cryoglobulinaemic glomerulonephritis. Nephron (1988) 50(1):14–23. doi: 10.1159/000185110
206. Manjarrez-Orduño N, Menard LC, Carman JA, Suchard SJ, Casano F, Lee D, et al. A systemic lupus erythematosus endophenotype characterized by increased Cd8 cytotoxic signature associates with renal involvement. ImmunoHorizons (2017) 1(7):124–32. doi: 10.4049/immunohorizons.1700028
207. Celhar T, Hopkins R, Thornhill SI, De Magalhaes R, Hwang S-H, Lee H-Y, et al. Rna sensing by conventional dendritic cells is central to the development of lupus nephritis. Proc Natl Acad Sci United States America (2015) 112(45):E6195–204. doi: 10.1073/pnas.1507052112
208. Devi KSP, Anandasabapathy N. The origin of dcs and capacity for immunologic tolerance in central and peripheral tissues. Semin Immunopathol (2017) 39(2):137–52. doi: 10.1007/s00281-016-0602-0
209. Collin M, Bigley V. Human dendritic cell subsets: An update. Immunology (2018) 154(1):3–20. doi: 10.1111/imm.12888
210. Waisman A, Lukas D, Clausen BE, Yogev N. Dendritic cells as gatekeepers of tolerance. Semin Immunopathol (2017) 39(2):153–63. doi: 10.1007/s00281-016-0583-z
211. Herrada AA, Escobedo N, Iruretagoyena M, Valenzuela RA, Burgos PI, Cuitino L, et al. Innate immune cells' contribution to systemic lupus erythematosus. Front Immunol (2019) 10:772. doi: 10.3389/fimmu.2019.00772
212. Honarpisheh M, Köhler P, von Rauchhaupt E, Lech M. The involvement of micrornas in modulation of innate and adaptive immunity in systemic lupus erythematosus and lupus nephritis. J Immunol Res (2018) 2018:4126106. doi: 10.1155/2018/4126106
213. Lorenz G, Anders H-J. Neutrophils, dendritic cells, toll-like receptors, and interferon-A in lupus nephritis. Semin Nephrol (2015) 35(5):410–26. doi: 10.1016/j.semnephrol.2015.08.003
Keywords: lupus nephritis, immune infiltration, comprehensive bioinformatics, biomarkers, CIBERSORT
Citation: Wang L, Yang Z, Yu H, Lin W, Wu R, Yang H and Yang K (2022) Predicting diagnostic gene expression profiles associated with immune infiltration in patients with lupus nephritis. Front. Immunol. 13:839197. doi: 10.3389/fimmu.2022.839197
Received: 19 December 2021; Accepted: 09 November 2022;
Published: 02 December 2022.
Edited by:
Tianfu Wu, University of Houston, United StatesReviewed by:
Ranjit Kumar Sahu, University of Virginia, United StatesScott E. Wenderfer, British Columbia Children’s Hospital, Canada
Copyright © 2022 Wang, Yang, Yu, Lin, Wu, Yang and Yang. This is an open-access article distributed under the terms of the Creative Commons Attribution License (CC BY). The use, distribution or reproduction in other forums is permitted, provided the original author(s) and the copyright owner(s) are credited and that the original publication in this journal is cited, in accordance with accepted academic practice. No use, distribution or reproduction is permitted which does not comply with these terms.
*Correspondence: Hongtao Yang, dGp0Y21odEAxMjYuY29t; Kang Yang, dGp6eXlrQDEyNi5jb20=
†These authors have contributed equally to this work and share first authorship