- 1Clinical Research Center, Beijing Children’s Hospital, Capital Medical University, National Center for Children’s Health, Beijing, China
- 2School of Pharmacy, Capital Medical University, Beijing, China
- 3Department of Gastroenterology, Beijing Children’s Hospital, Capital Medical University, National Center for Children’s Health, Beijing, China
Information on comparative drug efficacy is of great importance for drug development as well as clinical practice. Up to now, the relative efficacy of biologics and small targeted molecules for Crohn’s disease (CD) remains unclear. The objective of this study was to quantify the relative efficacy of investigational and approved biological treatments for CD measured in Crohn’s Disease Activity Index (CDAI), Inflammatory Bowel Disease Questionnaire (IBDQ), and C-reactive protein (CRP). The analysis dataset was composed of summary-level data from 46 trials, containing 12,846 patients, with treatment of 24 drugs. Six mathematical models with non-parametric placebo estimations were developed to describe the time course and dose–response of six efficacy measures. The effects of covariate were further evaluated. Time–response relationships were found in outcomes measured in CDAI. The patients’ age, disease duration, baseline CDAI, and CRP showed an impact on the efficacy. Model simulations were performed to compare the efficacies across different drugs. The most achievement in clinical remission (defined as CDAI less than 150) and clinical response (defined as the reduction in CDAI for 100 or 70) was observed in the simulation for PF-04236921 and infliximab, respectively. The most improvement in IBDQ was shown in tofacitinib. In general, tumor necrosis factor (TNF)-α inhibitors were the most effective biologics, and the highest efficacy of small targeted molecules was observed in janus kinase (JAK) inhibitors. These findings have important implications for clinical practice in CD.
Introduction
Crohn’s disease (CD) is a chronic inflammatory disease of the gastrointestinal tract, with symptoms like chronic abdominal pain, diarrhea, obstruction, and perianal lesions (1–4). Worldwide, the estimated incidence of CD ranges from 0.58 to 20.2 cases per 100,000 person-years, while the prevalence amount to 50–322 per 100,000 persons (3, 5). Medical therapy used to treat CD includes the categories of 5-aminosalicylates (5-ASA), antibiotics, corticosteroids, immunomodulators, and biologics (6). Biologics are by far the most potent treatment for CD (3) and are strongly recommended for patients with moderate-to-severe CD who failed to respond to conventional therapy (6, 7). Six biologic agents have been approved for the treatment of CD, and a number of biologics and small targeted molecules are under investigation. However, no specific drug is preferred in the guidelines (6–8), and physicians often choose therapies on the basis of personal experience due to the deficiency of head-to-head comparison (3).
Several meta-analyses and network meta-analyses have been conducted for the potential difference between treatments for CD (2, 9–11). However, these researches focused on the absolute efficacy, without considering the placebo effect, and the relative clinical efficacy remains unknown. Besides, in most studies, the assessments of drug efficacy were only based on the end-of-study results without considering the time course. Furthermore, the efficacies of different doses were pooled as summary-level data, which led to the inadequate utilization of available data. The influence of baseline characteristics on efficacy has been researched in only several drugs by previous studies (12–14), and the influence on most drugs still has not been measured.
Model-based meta-analysis (MBMA) is an extension of traditional meta-analysis (15), representing a framework for assessing the magnitude of the treatment response and its time course (16). The introduction of dose–response and time-course models, as well as the influence of baseline characteristics, makes it possible to incorporate all studies and treatments into the analysis and to utilize the totality of the information learned from trials (17). In addition, predictions can be made for all regimens of interest in an identical study design for a more valid comparison between treatments (15, 17). Therefore, it could offer a more informative view of the data in contrast to the traditional meta-analysis (15).
The main objective of this study is to use an MBMA approach to accurately quantify the relative efficacy and onset across different biologics and small targeted molecules, including those approved and undergoing investigation. The efficacy is measured by six outcomes reported in the clinical trials of CD: absolute Crohn’s Disease Activity Index (CDAI) score of less than 150 (CDAI150), reduction of at least 70 points in the CDAI score (CDAI-70), reduction of at least 100 points in the CDAI score (CDAI-100), change from baseline in CDAI (18), C-reactive protein (CRP) (19), and Inflammatory Bowel Disease Questionnaire (IBDQ) (20).
Methods
Data Development
The Cochrane Preferred Reporting Items for Systematic Reviews and Meta-Analyses (PRISMA) was used to collate data and report results (21). An electronic literature search was performed in MEDLINE (via PubMed), CENTRAL, EMBASE, and ClinicalTrials.gov website from inception to March 14, 2020. Keywords included were as follows: infliximab, etanercept, certolizumab, adalimumab, natalizumab, onercept, vedolizumab, ustekinumab, risankizumab, tofacitinib, filgotinib, fontolizumab, biologic, small targeted molecule, CD, and randomized controlled trial. Comprehensively, generic, code, and trade names of each drug were searched simultaneously. Abstracts from the United European Gastroenterology Week (UEGW), the American College of Gastroenterology (ACG), Digestive Disease Week (DDW), and the Congress of European Crohn’s and Colitis Organisation (ECCO) were searched until 2019. Reference lists of previous reviews were also searched for possible articles. Specific inclusion criteria were listed as follows:
1. Double-blinded randomized clinical trials reported with control treatment.
2. Included patients were at least 18 years old with moderate-to-severe active CD. CD was confirmed by radiologic, endoscopic, or histologic criteria.
3. Patients were treated with biologics or small targeted molecules. Concomitant medications, such as 5-ASA, oral steroids, and immunomodulators (azathioprine, 6-mercaptopurine, or methotrexate), were allowed. History of tumor necrosis factor (TNF) inhibitor was allowed.
4. Trials reported one of the following outcomes: CDAI score, ΔCDAI (change from baseline in CDAI), CDAI150, CDAI-100, CDAI-70, CRP, ΔCRP (change from baseline in CRP), IBDQ, and ΔIBDQ (change from baseline in IBDQ).
Search results were screened, and data were extracted by two reviewers (BY and SJ) independently. Disagreements between two reviewers were resolved by discussion and consensus with a third reviewer (LZ). Only data from trials of the induction period were included. Exclusion criteria included the following: trials in patients having surgery for CD within 3 months, trials without available baseline characteristics, and trials with a combination of anti-TNF and other biologics or small targeted molecules. Data extracted from citations included but were not limited to the following: publication year, title, author, trial name, trial design, and primary outcome. Patient demographics were captured, as well as treatment information of each arm, such as dose, frequency, and administration routes.
Efficacy outcomes were extracted from text, tables, and figures, including CDAI150, CDAI-100, CDAI-70, CDAI, IBDQ, and CRP. Different dose regimens were normalized by daily dose; for example, upadacitinib 12 mg twice daily was standardized to upadacitinib 24 mg daily. Dose regimens that need to be calculated by weight were normalized by 70 kg per patient. The CRP, which was reported in mg/dL or mg/L, was standardized into mg/L. Changes from baseline in continuous outcomes were extracted from articles directly or calculated by subtracting postbaseline values from baseline values. The relative effect was extracted for our analysis to be able to reduce the bias of estimation (15).
In the development of the analytical dataset, intent-to-treat populations were used whenever available. When multiple statistic values were available, the mean value was chosen over the median value. For the trial arms that were stratified by the baseline level, only the outcome values characterizing the overall level of the trial arms were included. The missing values of SDs were imputed by exploring the fixed-effect, linear, log, exponential, and maximum effect (Emax) models. The model-predicted SD values, combined with given SD values, were then used for derivation of weights during the model development. For missing covariates, if the missing values were ≤40%, the median value of the database was used for interpolation, and if the missing values were >40%, the baseline characteristic was not incorporated into the final models.
Risk of Bias Assessment
The risk of bias was assessed by two investigators independently using the Cochrane risk of bias tool. The evaluation items included random sequence generation, allocation concealment, blinding of participants and personnel, blinding in the outcome assessment, incomplete outcome data, selective reporting, and other biases (22). Disagreements were resolved through discussion with a third investigator.
Model Development
Data of all the dose regimens were utilized to explore the potential dose–response relationship. However, only data from multiple-dose trials as well as data from the single-dose trial whose time point within the minimum dosing interval of multiple-dose trials were included in the modeling. The longitudinal profiles of efficacy outcomes were characterized using a hierarchical regression model with the maximum likelihood estimation method. To avoid misestimation of placebo effects, a non-parametric method was implemented to estimate placebo effects in each trial and at each time point. The model could be generally described as
Eijt represents the efficacy in the jth treatment arm of the ith trial at t time, which is the sum of E0it (the placebo effects of the ith trial at t time) and Edrug (the drug effects in the jth treatment arm of the ith trial at t time). For outcomes measured as probability, a logit translation was performed to limit the probability to a range of 0–1. Edrug is a function dependent on the type of drug, dose, regimen, time, fixed-effect model parameters θ, and covariates X.
At first, the drug effects were set not to change over time. Then, during model development, if model fit improved, a time variable was added to create a non-linear model to describe the time-varying drug effects. The formula was listed as follows:
where represents the maximum efficacy of each treatment and k represents the rate constant describing the onset of drug effect.
In the process of the model development, the maximum efficacy of each drug was initially incorporated to be constant over different dosages and described by a scaling factor, Emax. Then, the parameter Emax was separated into several parameters matching different dose regimens. For drugs with dose range, a dose–response relationship was estimated by Emax or sigmoid Emax model. For drugs with poor dose levels, it was hard to estimate a clear dose–response relationship with Emax model, so a simple fixed-effect or linear dose–response model was used.
Weight was introduced according to the standard error of fitted values for CDAI150, CDAI-100, and CDAI-70 models, and the standard error of observed values for CDAI, CRP, and IBDQ models (Equations 4 and 5). The number of subjects for each trial arm within each trial (N) ensured that more influence on estimating the parameters was imposed by the larger studies.
A more technical exposition is available in the model development section of Supplementary Materials.
Covariate
Baseline characteristics, including age, percentage of male, disease duration, smoking status, CDAI, CRP, and IBDQ, were set as the covariates in the model. Covariates were investigated for their possible impact on the treatment efficacies with the following equation, where θ was the parameter quantifying the covariate effect.
Different correlation forms were tested as the within-arm autocorrelation structure, such as AR1, AR2, compound symmetry, and autoregressive moving average structure. Model development and iteration were based on the data and guided by successful convergence of the minimization routine. Model selection was based on the Akaike information criterion and the log-likelihood ratio at an acceptance p-value of 0.05.
Model Evaluation and Simulation
The model fits across trials were evaluated by model-fitted time-course plots and diagnostic plots. The parameters from the final models were used to sample a total of 10,000 model parameters for predicting the treatment efficacies at hypothetical time points.
All data exploration and model development, evaluation, and simulation were carried out with the R software version 3.6.3 [R Core Team (2020)] and the “gnls” function in the “nlme” package version 3.1–145. Literature quality assessment was performed using the Review Manager (RevMan), version 5.4.1, The Cochrane Collaboration, 2020.
Results
Available Data
A total of 3,223 citations were retrieved from the initial search. After the review of the abstracts and full articles, 46 trials containing 146 treatment arms and 12,846 patients were included in the analysis (12–14, 23–64). The complete process of literature searching and screening is shown in the flow diagram (Figure 1). Among the 46 included trials, the overall quality was assessed as high with a low risk of bias; detailed information on the assessment of literature quality is shown in Supplementary Figures 1 and 2.
Seventeen biologics and 7 small targeted molecules were involved, including TNF-α inhibitors, integrin inhibitors, interleukin (IL) inhibitors, matrix metalloproteinase-9 (MMP-9) inhibitor, janus kinase (JAK) inhibitors, T-cell activation inhibitors, mucosal addressing cell adhesion molecule (MAdCAM) inhibitor, interferon (IFN)-γ inhibitor, and C-C chemokine receptor-9 (CCR-9) antagonist. The drug classification and overview of included trials as well as prespecified baseline characteristics are displayed in Table 1.
Most reported outcomes were CDAI150, CDAI-100, CDAI-70, ΔCDAI, ΔCRP, and ΔIBDQ, which were evaluated in 38, 27, 24, 21, 26, and 20 trials, respectively. These six outcomes were selected for modeling. Among them, CDAI150 was defined as clinical remission, while CDAI-100 or CDAI-70 were defined as the clinical response. The detailed information about reported time points of each outcome in included trials was shown in Supplementary Table 1.
Before modeling, all the prespecified covariates were screened, and missing values of age, percentage of male, disease duration, smoking status baseline CDAI, CRP, and IBDQ were found in 0%–38% of the trials. To further develop the database, missing values were imputed with the median values of given baseline characteristics. The database used for the MBMA can be found in Supplementary Materials.
Final Models
The time course and dose–response relationship were adequately described by the longitudinal models, which were shown as follows:
The model fitted time-course plots of representative trials for six models are shown in Figures 2 and 3, and additional plots can be found in Supplementary Materials. The time-varying drug efficacy was found in the CDAI150 and ΔCDAI models, as the exponential function shown in Equation 7, where represents the maximum efficacy, and k represents the rate constant describing the onset of drugs. Based on the exponential model (Figure 2), the time to reach 50% of the maximum effect (ET50) of JAK inhibitors was estimated to be about 6.3 weeks, and the time to reach 90% of the maximum effect (ET90) was estimated to be 20.9 weeks. Moreover, kgeneral (the rate constant for all treatments) was estimated for the ΔCDAI model. Based on the estimated values, ET50 and ET90 were assumed to be 3.2 and 10.5 weeks in the ΔCDAI model. Dose–response relationship was estimated for each drug with Emax model. For drugs without enough information available in the database to estimate a clear dose–response relationship, a simple fixed-effect or linear model was used.
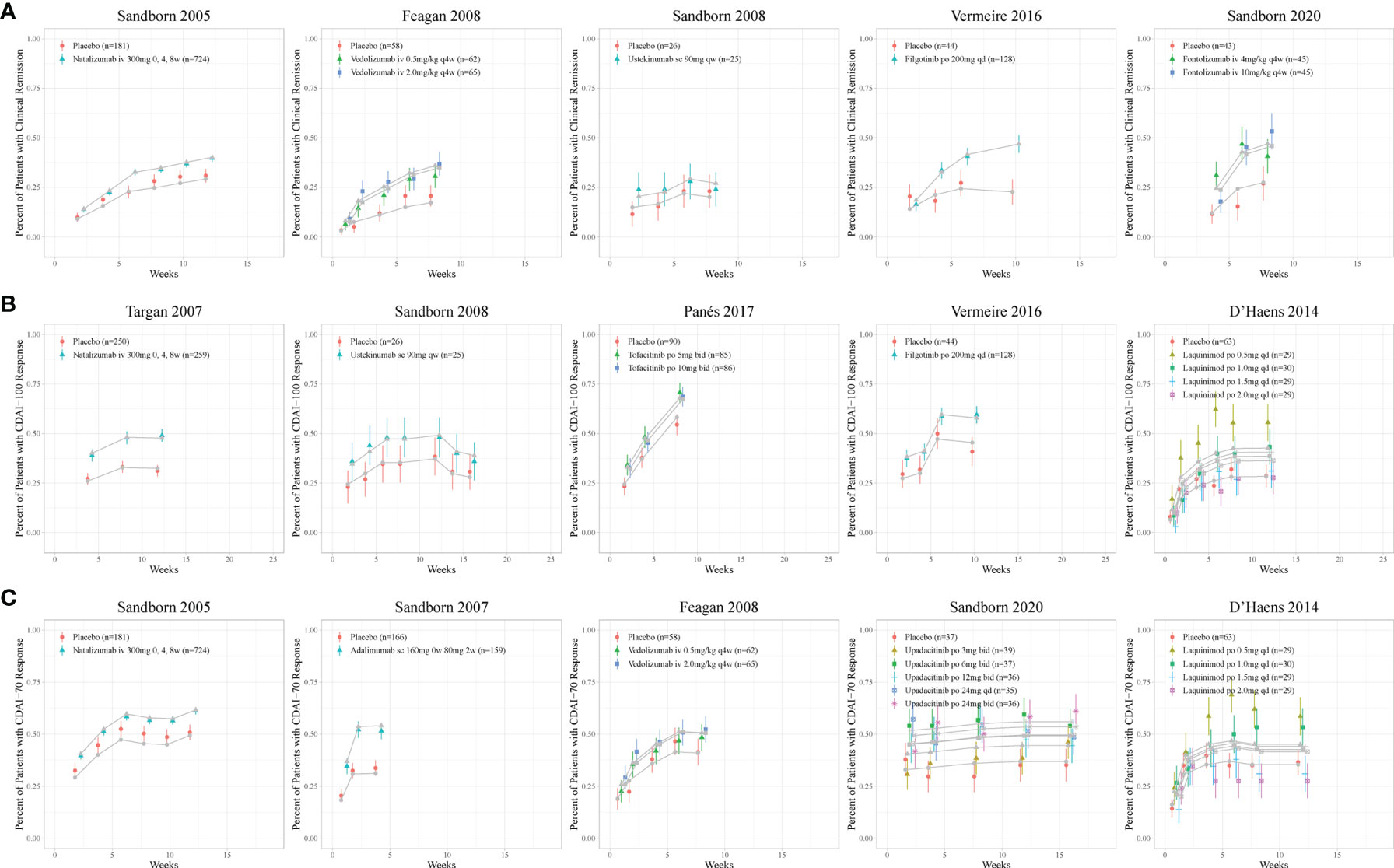
Figure 2 Model fitted time-course plots of response rate for (A) CDAI150, (B) CDAI-100, and (C) CDAI-70 for representative trials. Color symbols and vertical bars are observed mean and calculated weight of time points; gray symbols and lines are the model predictions. CDAI, Crohn’s Disease Activity Index; CDAI150, an absolute CDAI score of less than 150; CDAI-100, reduction of at least 100 points in the CDAI score; CDAI-70, reduction of at least 70 points in the CDAI score; qd, once daily; bid, twice daily; qw, once weekly; q4w, once every 4 weeks.
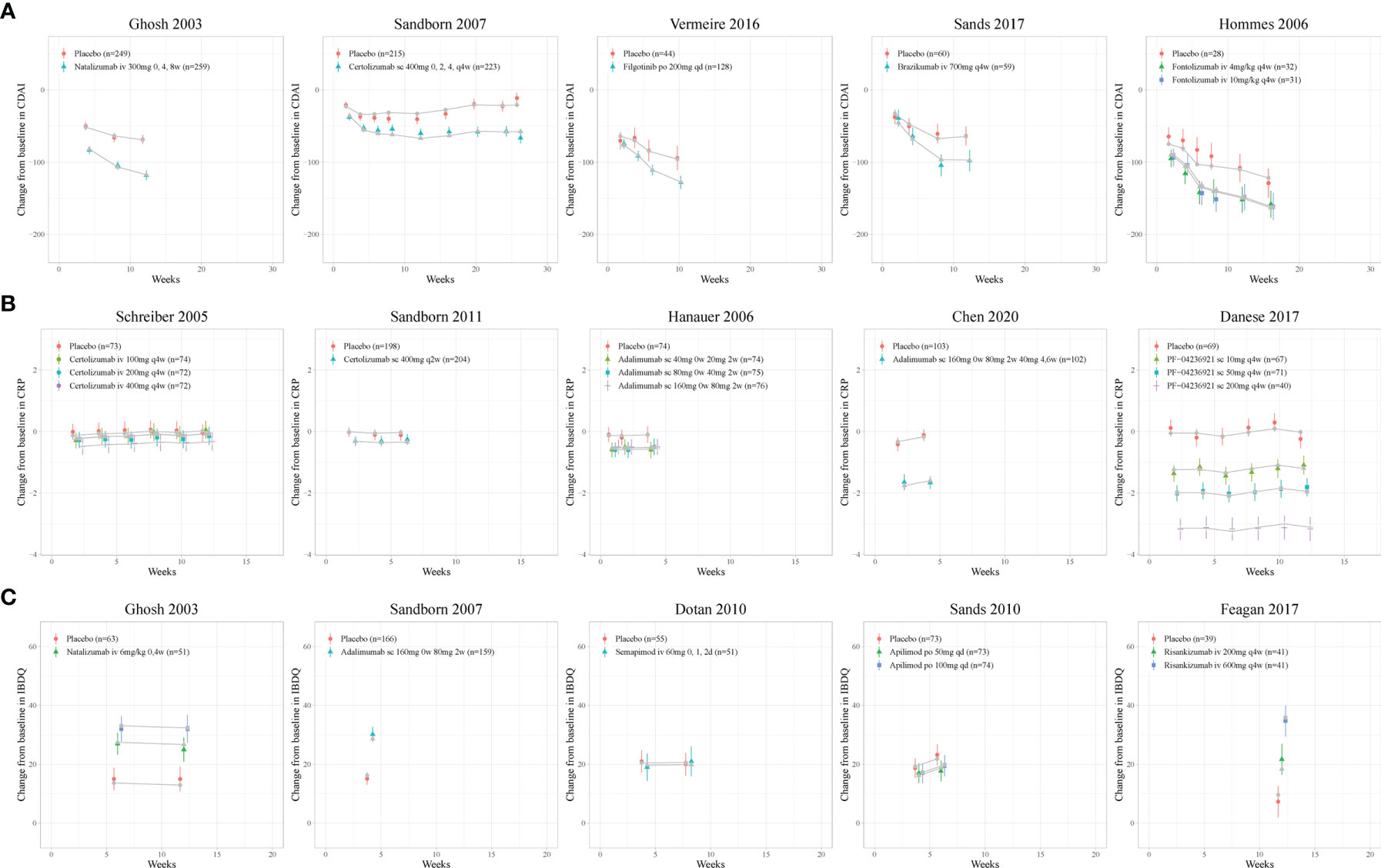
Figure 3 Model fitted time-course plots of (A) CDAI, (B) CRP, and (C) IBDQ change from baseline for representative trials. Color symbols and vertical bars are observed mean and calculated weight of time points; gray symbols and lines are the model predictions. CDAI, Crohn’s Disease Activity Index; CRP, C-reactive protein; IBDQ, Inflammatory Bowel Disease Questionnaire; qd, once every day; q2w, once every 2 weeks; q4w, once every 4 weeks.
Covariates
Seven prespecified covariates (percentage of men, age, disease duration, smoking status, CDAI, CRP, and IBDQ) were tested for their association with the drug efficacies. Age, disease duration, baseline CDAI, and CRP were included in the final models. For CDAI150, CDAI-100, CDAI-70, and ΔCDAI model, the estimated covariate parameters of negative value for baseline CDAI (Table 2) indicated that the patients with lower baseline CDAI were expected to get greater efficacy. The parameters for age (−7.69 [95% CI: −11.11 to −4.26]) in the CRP model means that younger patients were assumed to get more decrease in CRP. Baseline CRP was also estimated as a covariate in the CDAI150, CDAI-100, CDAI-70, and ΔCRP model (Table 2), which means that patients with higher baseline CRP are assumed to get more improvement measured in CDAI and less decrease in CRP. The covariate parameters for disease duration were estimated as 4.95 (95% CI: 3.60 to 6.29) for ΔCRP and −8.98 (95% CI: −10.60 to −7.36) for ΔIBDQ, indicating a better decrease in CRP and less improvement in IBDQ among patients with longer CD duration.
More detailed results and code were available in the final model section of Supplementary Materials.
Model Simulation
To compare all treatments, drug effect at week 12, the most common duration of the induction period, as well as the most common time point of primary outcome among included trials, was simulated with final models. A typical trial indicating the common characteristic of included trials was assumed for the simulation with a hypothesis population with 46.09% men, 9.52 years of disease duration, baseline CDAI of 306.75, and baseline CRP of 1.67. To generate the simulation, a longitudinal placebo model was developed for each outcome.
Figures 4 and 5 show the ranking of median placebo-corrected treatment effects at 12 weeks for each outcome. Among the result of the simulation for the six outcomes, the consistent highest efficacy was provided by the TNF-α inhibitor, IL-23 inhibitor, and integrin-a4 inhibitor with a narrow 95% CI. The model simulation of the CDAI150, with a placebo effect estimated as 21.26%, is shown in Figure 4A. It reveals that PF-04236921 200 mg had the best response in CDAI150 (median: 56.12%, 95% CI: 32.55% to 78.83%) with a large 95% CI, followed by risankizumab 600 mg (median: 53.55%, 95% CI: 31.09% to 75.39%) and infliximab (median: 44.44%, 95% CI: 37.39% to 51.63%). For CDAI-100 (Figure 4B), with a placebo effect estimated as 31.28%, infliximab was predicted to have the highest efficacy as 56.75% (95% CI: 51.12% to 62.37%), followed by upadacitinib (median: 56.29%, 95% CI: 35.41% to 76.68%) and brazikumab (median: 54.13%, 95% CI: 35.39% to 72.86%) with a large 95% CI. For the response rate of CDAI-70 (Figure 4C), the placebo effect was estimated as 39.48%. Infliximab (median: 67.49%, 95% CI: 62.33% to 72.63%) and adalimumab (median: 60.34%, 95% CI: 54.02% to 66.65%) were predicted to have the highest drug efficacy. As presented in Figure 5A, the most placebo-corrected decrease in CDAI was simulated for risankizumab (median: −133.40, 95% CI: −169.53 to −97.35) and adalimumab 160 mg (median −124.75, 95% CI: −177.58 to −60.98) with large 95% CI. The placebo effect was estimated as −58.67. For CRP, the placebo effect was simulated as 0.016 with a longitudinal placebo model. PF-04236921 200 mg (median: −5.52, 95% CI: −7.83 to −2.63) was shown to be the most effective regimen with a large 95% CI. Natalizumab (median: −0.95, 95% CI: −1.21 to −0.70) also showed great efficacy. Ranking of the treatments by predicted IBDQ improvement (Figure 5C) showed that tofacitinib (median: 70.01, 95% CI: 56.56 to 83.48) was predicted to be most effective. The placebo effect was simulated as 17.36 with a longitudinal model, shown as the dashed line in Figure 5C.
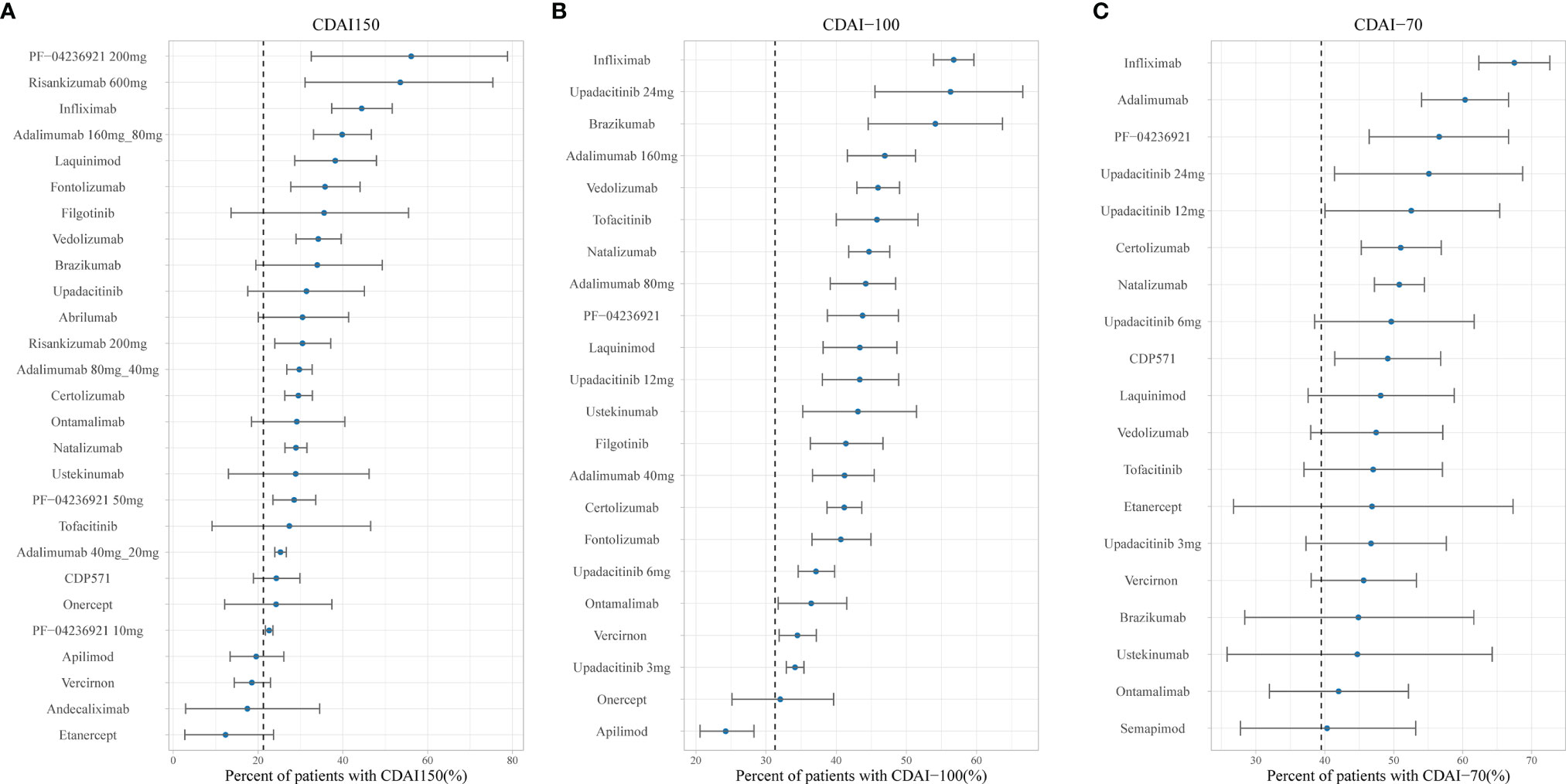
Figure 4 Ranking of the treatments by predicted placebo-corrected median percent of patients with (A) CDAI150, (B) CDAI-100, and (C) CDAI-70 at week 12 (from high to low). Point estimates and 95% CIs were predicted from a model simulation of N = 10,000. Dashed lines represent simulated placebo efficacy. For treatments with multiple dosage regimens, only regimens with different efficacy at week 12 were listed separately. CDAI, Crohn’s Disease Activity Index; CDAI150, an absolute CDAI score of less than 150; CDAI-100, reduction of at least 100 points in the CDAI score; CDAI-70, reduction of at least 70 points in the CDAI score; bid, twice daily.
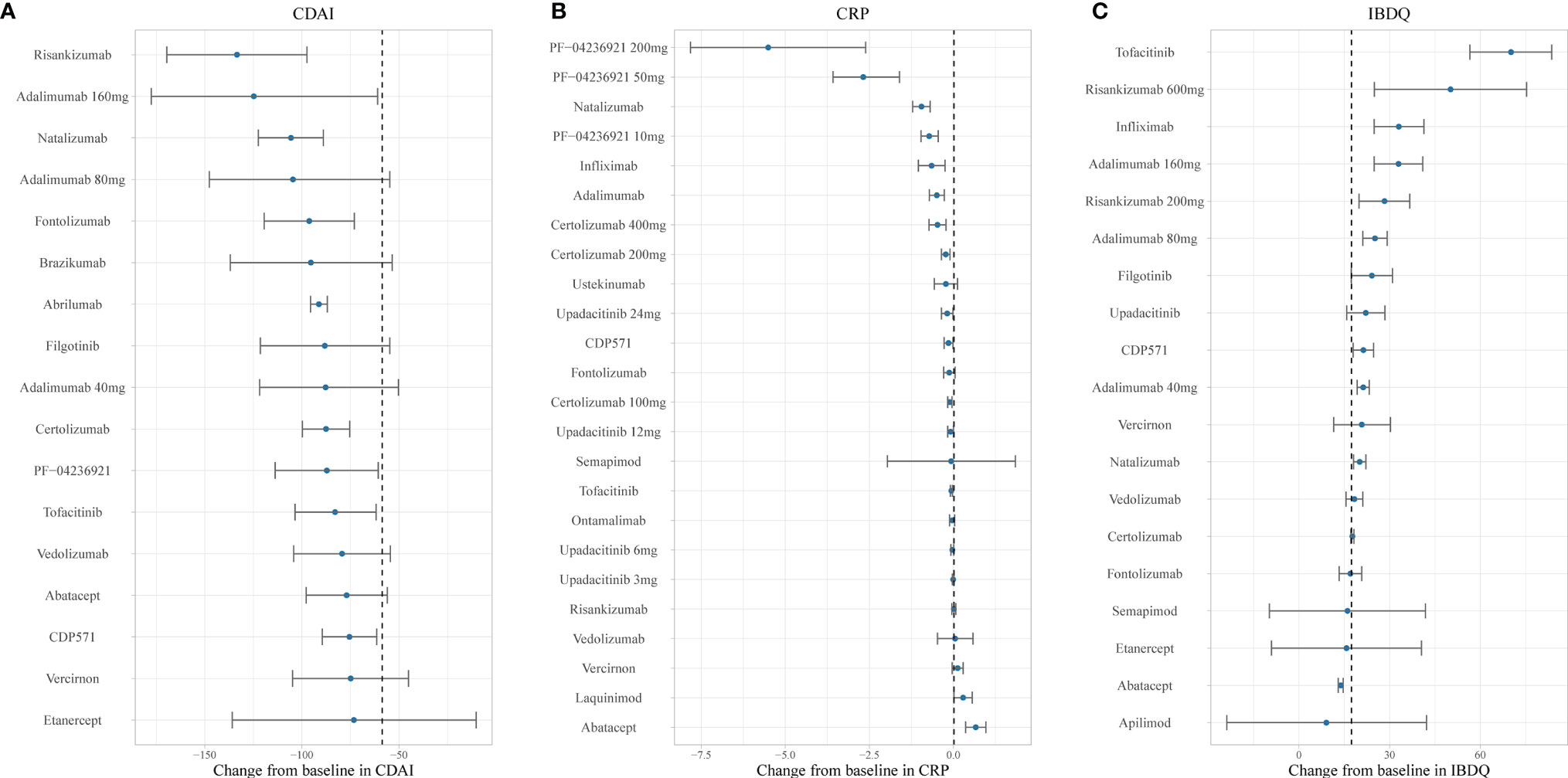
Figure 5 Ranking of the treatments by predicted placebo-corrected median change in (A) CDAI, (B) CRP, and (C) IBDQ at week 12 (from high to low). Point estimates and 95% CIs were predicted from a model simulation of N = 10,000. Dashed lines represent simulated placebo efficacy. For treatments with multiple dosage regimens, only regimens with different efficacy at week 12 were listed separately. CDAI, Crohn’s Disease Activity Index; CRP, C-reactive protein; IBDQ, Inflammatory Bowel Disease Questionnaire.
Residual Correlation
After comparison of the model fit, the AR1 model was used to account for residual correlation for the CDAI-70, ΔCDAI, and ΔIBDQ models; AR2 model was used for CDAI150 and ΔCRP models; and compound symmetry structure was used for the CDAI-100 model.
Discussion
Our MBMA quantitatively compared the efficacy of 24 drugs in six outcomes. To normalize the comparison, the analysis was adjusted by the percentage of male patients, duration of disease, age, smoking status, baseline CDAI, baseline CRP, baseline IBDQ, dose regimen, and administration route. The estimated model was used to predict and compare drug effects, which could help physicians make appropriate treatment strategies. For example, Figure 4 demonstrates that infliximab shows the best efficacy in clinical response. These results were known from trials (65, 66) or meta-analyses (2, 10, 11, 67–69); however, most of those researches focused on only one or several specific classes of biologics. Our study is the first to simultaneously evaluate and report all the biologics and small targeted molecules with a quantitative method, which quantified the efficacy of each drug as well as the influence of dosage, time, and covariates.
A total of six outcomes, including continuous outcomes (ΔCDAI, ΔCRP, and ΔIBDQ) and binary outcomes (CDAI150, CDAI-100, and CDAI-70), were evaluated. They mainly assessed the improvement in disease activity and life quality, as well as change of biomarker. To provide a comprehensive understanding, drug efficacies were evaluated based on these three aspects. CDAI was the most commonly used scoring system in clinical practice. However, some clear limitations were observed in it (e.g., interobserver variability) (8, 70), so objective laboratory test data (CRP) and scoring system for life quality (IBDQ) (19, 70) were also included in our analysis. Although CDAI150, CDAI-100, and CDAI-70 are determined by the same CDAI score, they were reported in different trials. To catch the whole picture of targeted treatment in CD, all these outcomes were included in the analysis. Mucosal healing is considered a therapeutic goal of CD (4, 71); however, most of the included trials did not report consistent endoscopic outcomes, and the efficacy of achieving endoscopic remission was not examined in our analysis. Some of the outcomes and covariates were not reported in all included trials (46); however, as the missing value is considered random, the result would be considered unbiased (72).
In general, the efficacy trends of drugs measured in six outcomes were similar. TNF-α inhibitors were the most effective biologics, followed by integrin inhibitors and IL-12/23 inhibitors. For small targeted molecules, the highest efficacy was observed in JAK inhibitors. These results were supported by several previous meta-analyses (2, 10, 11, 67–69). However, there were still some differences in the rank order across six outcomes between our results and previous research, which was attributed to the different covariate effects estimated for each outcome, and the limit in sample size. The results should be interpreted with caution.
The onset speed of drug effects in CD is of great importance for physicians to adjust treatment plans in time. In our longitudinal MBMA, the time-course models could quantitatively estimate the onset of drugs. However, time-response relationships were only found in CDAI150 and ΔCDAI, which was reported by most trials. Although it is generally accepted that continuous outcomes were more sensitive to changes (73), there may not be enough data to estimate a time-course model for CRP and IBDQ. Because of the long T1/2 of monoclonal antibodies, which is reported to be 15–51 days or more (25, 74), it may be unable for some drugs to reach steady-state during the induction period of remission, which may also be the reason for the poor result in the estimation of time-response relationships. Therefore, caution is needed in interpreting the results.
The impact of dose regimen on the treatment efficacy was also tested in our analysis. The dose–response relationships of adalimumab, upadacitinib, certolizumab pegol, risankizumab, and PF-04236921 were identified by Emax or linear model, indicating that a higher dose could improve the drug efficacy. A common Emax parameter was tried to be estimated during the modeling. However, due to the poor sample size or lack of dose range, the dose-varying efficacy was only successfully estimated in several drugs. It may also result from short research duration (15), as more visible dose–response relationships were observed in longer-term efficacy in trials (14, 26).
A benefit of MBMA is that the impact of covariates on the treatment efficacy can be quantitatively described (75), and five covariates were included in our final models. Patients with lower baseline CDAI were shown to have greater improvement in CDAI150, CDAI-100, CDAI-70, ΔCDAI, and ΔCRP, which was consistent with previous studies (75). In addition, a lower CRP level was also considered as a predictor of more decrease in CRP (76). Our analysis suggested that younger patients were shown to get better efficacy in ΔCRP models. In the ΔIBDQ model, patients with shorter disease duration showed more improvement, while more response in the ΔCRP model was shown in patients with longer disease duration. In other studies, early intervention with biologics was considered to show more benefits (77). The reason for the difference may be that early intervention was defined as 8 weeks to 2 years in other studies (77), but in our analysis, the mean disease duration of included trials was 9.5 years. The study of CD’s natural history suggested that a longer course of the disease may lead to more serious and complex conditions (78), so there may be a correlation between the duration and severity of the disease. Moreover, our identification of covariates was based on the aggregation of trial-level data, which contains less information than patient-level data (79, 80). Besides, for models with few baseline characteristics available, there may not be enough power to detect the impact of covariates on drug efficacy.
There are several advantages of our MBMA. First, our analysis included the largest number of trials, drugs, and patients. Second, longitudinal models and different dose–response models were used to describe the drug effect. Thus, data of drugs in different dose regimens at all the time points were able to be utilized in the analysis. Third, our inclusion criteria limited the studies to randomized controlled trials (RCTs), reducing unnecessary biases between treatment arms. Besides, the placebo effect for each trial was estimated respectively, because of the non-negligible between-trial variability among placebo effects of trials for CD (81). Thus, we were able to quantify the relative drug effect in an unbiased way. Fourth, the framework can be adapted and reused in other drugs for CD, and the model can be easily updated with more data of clinical efficacy available.
There are still some limitations in our analysis. First, it should be noticed that heterogeneity in the population was observed in several trials. For example, trials of some drugs included patients who had no response to previous treatment (46). This may lead to lower efficacy for these drugs in our analysis. However, previous studies indicated that prior exposure to both anti-TNF and other treatments did not impact the result of comparison between biologics significantly (2, 10, 69, 82). Moreover, the combined efficacy of anti-TNF-naive and anti-TNF-exposed patients was reported in several trials, so it is difficult to estimate the impact of anti-TNF experience separately. Second, the generalizability of our results is limited to the population enrolled in the included trials. For example, the inclusion criteria limited patients in moderate-to-severe CD, and thus, our interpretations do not necessarily relate to patients with mild disease activity. Third, mucosal healing, which is considered an important therapeutic endpoint in the management of CD, was not included in our meta-analysis due to the deficiency in data. Fourth, efficacy data were still inadequate for some drugs, which may lead to imprecise and unreliable estimates (15). Thus, caution is needed in interpreting these results.
In conclusion, our analysis provided an MBMA framework that combined evidence from 46 RCTs, allowing the estimate and prediction of efficacy for multiple agents across time course and a range of doses. In general, TNF-α inhibitors were the most effective biologics, and the highest efficacy of small targeted molecules was observed in JAK inhibitors. Besides, the patients’ age, disease duration, baseline CDAI, and CRP were identified as the covariates that show the impact on drug efficacy. We hope that our results will enable physicians and patients to understand better the differences and similarities across 17 biologics and 7 small targeted molecules in CD for 6 important outcomes.
Data Availability Statements
The original contributions presented in the study are included in the article/Supplementary Material. Further inquiries can be directed to the corresponding authors.
Author Contributions
XW, JZ, BY, LZ, SJ, and HH wrote the manuscript. XW, JZ, BY, and LZ designed the research. BY, LZ, SJ, and HH performed the research. BY, LZ, SJ, and HH analyzed the data. All authors listed have made a substantial, direct, and intellectual contribution to the work and approved it for publication.
Funding
This work was supported by the National Science and Technology Major Project [Grant number 2018ZX09721003] and Beijing Municipal Natural Science Foundation [Grant number L202042].
Conflict of Interest
The authors declare that the research was conducted in the absence of any commercial or financial relationships that could be construed as a potential conflict of interest.
Publisher’s Note
All claims expressed in this article are solely those of the authors and do not necessarily represent those of their affiliated organizations, or those of the publisher, the editors and the reviewers. Any product that may be evaluated in this article, or claim that may be made by its manufacturer, is not guaranteed or endorsed by the publisher.
Acknowledgments
The authors acknowledge Xiaoli Xie, Xiaohui Xie, and Xiaoxia Peng for their valuable suggestions during the design of the research. The authors also express their gratitude to Jiaqi Han and Jiamin Xu for the fruitful discussions during the preparation of the manuscript and Yunjiao Wu for the technological support.
Supplementary Material
The Supplementary Material for this article can be found online at: https://www.frontiersin.org/articles/10.3389/fimmu.2022.828219/full#supplementary-material
References
1. Moćko P, Kawalec P, Pilc A. Safety Profile of Biologic Drugs in the Therapy of Crohn Disease: A Systematic Review and Network Meta-Analysis. Pharmacol Rep (2016) 68:1237–43. doi: 10.1016/j.pharep.2016.07.013
2. Hazlewood GS, Rezaie A, Borman M, Panaccione R, Ghosh S, Seow CH, et al. Comparative Effectiveness of Immunosuppressants and Biologics for Inducing and Maintaining Remission in Crohn's Disease: A Network Meta-Analysis. Gastroenterology (2015) 148:344–54.e345; quiz e314-345. doi: 10.1053/j.gastro.2014.10.011
3. Roda G, Chien Ng S, Kotze PG, Argollo M, Panaccione R, Spinelli A, et al. Crohn's Disease. Nat Rev Dis Primers (2020) 6:22. doi: 10.1038/s41572-020-0156-2
4. Torres J, Mehandru S, Colombel JF, Peyrin-Biroulet L. Crohn's Disease. Lancet (2017) 389:1741–55. doi: 10.1016/s0140-6736(16)31711-1
5. Ng SC, Shi HY, Hamidi N, Underwood FE, Tang W, Benchimol EI, et al. Worldwide Incidence and Prevalence of Inflammatory Bowel Disease in the 21st Century: A Systematic Review of Population-Based Studies. Lancet (2018) 390:2769–78. doi: 10.1016/s0140-6736(17)32448-0
6. Lichtenstein GR, Loftus EV, Isaacs KL, Regueiro MD, Gerson LB, Sands BE. ACG Clinical Guideline: Management of Crohn's Disease in Adults. Am J Gastroenterol (2018) 113:481–517. doi: 10.1038/ajg.2018.27
7. Torres J, Bonovas S, Doherty G, Kucharzik T, Gisbert JP, Raine T, et al. ECCO Guidelines on Therapeutics in Crohn's Disease: Medical Treatment. J Crohns Colitis (2020) 14:4–22. doi: 10.1093/ecco-jcc/jjz180
8. Gomollón F, Dignass A, Annese V, Tilg H, Van Assche G, Lindsay JO, et al. 3rd European Evidence-Based Consensus on the Diagnosis and Management of Crohn's Disease 2016: Part 1: Diagnosis and Medical Management. J Crohns Colitis (2017) 11:3–25. doi: 10.1093/ecco-jcc/jjw168
9. Chandar AK, Singh S, Murad MH, Peyrin-Biroulet L, Loftus EV Jr. Efficacy and Safety of Natalizumab and Vedolizumab for the Management of Crohn's Disease: A Systematic Review and Meta-Analysis. Inflammation Bowel Dis (2015) 21:1695–708. doi: 10.1097/mib.0000000000000373
10. Singh S, Fumery M, Sandborn WJ, Murad MH. Systematic Review and Network Meta-Analysis: First- and Second-Line Biologic Therapies for Moderate-Severe Crohn's Disease. Aliment Pharmacol Ther (2018) 48:394–409. doi: 10.1111/apt.14852
11. Singh S, Garg SK, Pardi DS, Wang Z, Murad MH, Loftus EV Jr. Comparative Efficacy of Biologic Therapy in Biologic-Naïve Patients With Crohn Disease: A Systematic Review and Network Meta-Analysis. Mayo Clin Proc (2014) 89:1621–35. doi: 10.1016/j.mayocp.2014.08.019
12. Sandborn WJ, Schreiber S, Feagan BG, Rutgeerts P, Younes ZH, Bloomfield R, et al. Certolizumab Pegol for Active Crohn's Disease: A Placebo-Controlled, Randomized Trial. Clin Gastroenterol Hepatol (2011) 9:670–8.e673. doi: 10.1016/j.cgh.2011.04.031
13. Schreiber S, Rutgeerts P, Fedorak RN, Khaliq-Kareemi M, Kamm MA, Boivin M, et al. A Randomized, Placebo-Controlled Trial of Certolizumab Pegol (CDP870) for Treatment of Crohn's Disease. Gastroenterology (2005) 129:807–18. doi: 10.1053/j.gastro.2005.06.064
14. Sandborn WJ, Lee SD, Tarabar D, Louis E, Klopocka M, Klaus J, et al. Phase II Evaluation of Anti-MAdCAM Antibody PF-00547659 in the Treatment of Crohn's Disease: Report of the OPERA Study. Gut (2018) 67:1824–35. doi: 10.1136/gutjnl-2016-313457
15. Wu Y, Feng X, Li J, Wang X, Yang C, Zhao L. Model-Based Meta-Analysis in Ankylosing Spondylitis: A Quantitative Comparison of Biologics and Small Targeted Molecules. Clin Pharmacol Ther (2019) 105:1244–55. doi: 10.1002/cpt.1305
16. Demin I, Hamrén B, Luttringer O, Pillai G, Jung T. Longitudinal Model-Based Meta-Analysis in Rheumatoid Arthritis: An Application Toward Model-Based Drug Development. Clin Pharmacol Ther (2012) 92:352–9. doi: 10.1038/clpt.2012.69
17. Maloney A, Rosenstock J, Fonseca V. A Model-Based Meta-Analysis of 24 Antihyperglycemic Drugs for Type 2 Diabetes: Comparison of Treatment Effects at Therapeutic Doses. Clin Pharmacol Ther (2019) 105:1213–23. doi: 10.1002/cpt.1307
18. Best WR, Becktel JM, Singleton JW, Kern F Jr. Development of a Crohn's Disease Activity Index. National Cooperative Crohn's Disease Study. Gastroenterology (1976) 70:439–44. doi: 10.1016/S0016-5085(76)80163-1
19. Sachar DB. Role of Biomarkers in the Study and Management of Inflammatory Bowel Disease: A "Nonsystematic" Review. Inflamm Bowel Dis (2014) 20:2511–8. doi: 10.1097/mib.0000000000000135
20. Irvine EJ, Feagan B, Rochon J, Archambault A, Fedorak RN, Groll A, et al. Quality of Life: A Valid and Reliable Measure of Therapeutic Efficacy in the Treatment of Inflammatory Bowel Disease. Canadian Crohn's Relapse Prevention Trial Study Group. Gastroenterology (1994) 106:287–96. doi: 10.1016/0016-5085(94)90585-1
21. Page MJ, Mckenzie JE, Bossuyt PM, Boutron I, Hoffmann TC, Mulrow CD, et al. The PRISMA 2020 Statement: An Updated Guideline for Reporting Systematic Reviews. BMJ (Clin Res ed.) (2021) 372:n71. doi: 10.1136/bmj.n71
22. Cochrane Handbook for Systematic Reviews of Interventions. Version 6.2 (2021). Available at: http://training.cochrane.org/handbook (Accessed 6 February 2022).
23. Chen B, Gao X, Zhong J, Ren J, Zhu X, Liu Z, et al. Efficacy and Safety of Adalimumab in Chinese Patients With Moderately to Severely Active Crohn's Disease: Results From a Randomized Trial. Therap Adv Gastroenterol (2020) 13:1756284820938960. doi: 10.1177/1756284820938960
24. Colombel JF, Sandborn WJ, Reinisch W, Mantzaris GJ, Kornbluth A, Rachmilewitz D, et al. Infliximab, Azathioprine, or Combination Therapy for Crohn's Disease. N Engl J Med (2010) 362:1383–95. doi: 10.1056/NEJMoa090449
25. Danese S, Vermeire S, Hellstern P, Panaccione R, Rogler G, Fraser G, et al. Randomised Trial and Open-Label Extension Study of an Anti-Interleukin-6 Antibody in Crohn's Disease (ANDANTE I and II). Gut (2019) 68:40–8. doi: 10.1136/gutjnl-2017-314562
26. D'haens G, Sandborn WJ, Colombel JF, Rutgeerts P, Brown K, Barkay H, et al. A Phase II Study of Laquinimod in Crohn's Disease. Gut (2015) 64:1227–35. doi: 10.1136/gutjnl-2014-307118
27. D'haens G, Van Deventer S, Van Hogezand R, Chalmers D, Kothe C, Baert F, et al. Endoscopic and Histological Healing With Infliximab Anti-Tumor Necrosis Factor Antibodies in Crohn's Disease: A European Multicenter Trial. Gastroenterology (1999) 116:1029–34. doi: 10.1016/s0016-5085(99)70005-3
28. Dotan I, Rachmilewitz D, Schreiber S, Eliakim R, van der Woude CJ, Kornbluth A, et al. A Randomised Placebo-Controlled Multicentre Trial of Intravenous Semapimod HCl for Moderate to Severe Crohn's Disease. Gut (2010) 59:760–6. doi: 10.1136/gut.2009.179994
29. Feagan BG, Greenberg GR, Wild G, Fedorak RN, Paré P, Mcdonald JW, et al. Treatment of Active Crohn's Disease With MLN0002, a Humanized Antibody to the Alpha4beta7 Integrin. Clin Gastroenterol Hepatol (2008) 6:1370–7. doi: 10.1016/j.cgh.2008.06.007
30. Feagan BG, Sandborn WJ, D'haens G, Lee SD, Allez M, Fedorak RN, et al. Randomised Clinical Trial: Vercirnon, an Oral CCR9 Antagonist, vs. Placebo as Induction Therapy in Active Crohn's Disease. Aliment Pharmacol Ther (2015) 42:1170–81. doi: 10.1111/apt.13398
31. Feagan BG, Sandborn WJ, D'haens G, Panés J, Kaser A, Ferrante M, et al. Induction Therapy With the Selective Interleukin-23 Inhibitor Risankizumab in Patients With Moderate-to-Severe Crohn's Disease: A Randomised, Double-Blind, Placebo-Controlled Phase 2 Study. Lancet (2017) 389:1699–709. doi: 10.1016/s0140-6736(17)30570-6
32. Feagan BG, Sandborn WJ, Gasink C, Jacobstein D, Lang Y, Friedman JR, et al. Ustekinumab as Induction and Maintenance Therapy for Crohn's Disease. N Engl J Med (2016) 375:1946–60. doi: 10.1056/NEJMoa1602773
33. Ghosh S, Goldin E, Gordon FH, Malchow HA, Rask-Madsen J, Rutgeerts P, et al. Natalizumab for Active Crohn's Disease. N Engl J Med (2003) 348:24–32. doi: 10.1056/NEJMoa020732
34. Gordon FH, Lai CW, Hamilton MI, Allison MC, Srivastava ED, Fouweather MG, et al. A Randomized Placebo-Controlled Trial of a Humanized Monoclonal Antibody to Alpha4 Integrin in Active Crohn's Disease. Gastroenterology (2001) 121:268–74. doi: 10.1053/gast.2001.26260
35. Hanauer SB, Sandborn WJ, Rutgeerts P, Fedorak RN, Lukas M, Macintosh D, et al. Human Anti-Tumor Necrosis Factor Monoclonal Antibody (Adalimumab) in Crohn's Disease: The CLASSIC-I Trial. Gastroenterology (2006) 130:323–33; quiz 591. doi: 10.1053/j.gastro.2005.11.030
36. Hommes DW, Mikhajlova TL, Stoinov S, Stimac D, Vucelic B, Lonovics J, et al. Fontolizumab, a Humanised Anti-Interferon Gamma Antibody, Demonstrates Safety and Clinical Activity in Patients With Moderate to Severe Crohn's Disease. Gut (2006) 55:1131–7. doi: 10.1136/gut.2005.079392
37. Keshav S, Vaňásek T, Niv Y, Petryka R, Howaldt S, Bafutto M, et al. A Randomized Controlled Trial of the Efficacy and Safety of CCX282-B, an Orally-Administered Blocker of Chemokine Receptor CCR9, for Patients With Crohn's Disease. PloS One (2013) 8:e60094. doi: 10.1371/journal.pone.0060094
38. Nct. Clinical Study of CDP870/Certolizumab Pegol in Patients With Active Crohn's Disease (2006). Available at: https://clinicaltrials.gov/show/NCT00291668.
39. Nct. AMG 181 in Subjects With Moderate to Severe Crohn's Disease (2012). Available at: https://clinicaltrials.gov/show/NCT01696396.
40. Panés J, Sandborn WJ, Schreiber S, Sands BE, Vermeire S, D'haens G, et al. Tofacitinib for Induction and Maintenance Therapy of Crohn's Disease: Results of Two Phase IIb Randomised Placebo-Controlled Trials. Gut (2017) 66:1049–59. doi: 10.1136/gutjnl-2016-312735
41. Reinisch W, De Villiers W, Bene L, Simon L, Rácz I, Katz S, et al. Fontolizumab in Moderate to Severe Crohn's Disease: A Phase 2, Randomized, Double-Blind, Placebo-Controlled, Multiple-Dose Study. Inflamm Bowel Dis (2010) 16:233–42. doi: 10.1002/ibd.21038
42. Rutgeerts P, Sandborn WJ, Fedorak RN, Rachmilewitz D, Tarabar D, Gibson P, et al. Onercept for Moderate-to-Severe Crohn's Disease: A Randomized, Double-Blind, Placebo-Controlled Trial. Clin Gastroenterol Hepatol (2006) 4:888–93. doi: 10.1016/j.cgh.2006.04.022
43. Sandborn WJ, Colombel JF, Enns R, Feagan BG, Hanauer SB, Lawrance IC, et al. Natalizumab Induction and Maintenance Therapy for Crohn's Disease. N Engl J Med (2005) 353:1912–25. doi: 10.1056/NEJMoa043335
44. Sandborn WJ, Colombel JF, Sands BE, Rutgeerts P, Targan SR, Panaccione R, et al. Abatacept for Crohn's Disease and Ulcerative Colitis. Gastroenterology (2012) 143:62–69.e64. doi: 10.1053/j.gastro.2012.04.010
45. Sandborn WJ, Feagan BG, Fedorak RN, Scherl E, Fleisher MR, Katz S, et al. A Randomized Trial of Ustekinumab, a Human Interleukin-12/23 Monoclonal Antibody, in Patients With Moderate-to-Severe Crohn's Disease. Gastroenterology (2008) 135:1130–41. doi: 10.1053/j.gastro.2008.07.014
46. Sandborn WJ, Feagan BG, Loftus EV Jr., Peyrin-Biroulet L, Van Assche G, D'haens G, et al. Efficacy and Safety of Upadacitinib in a Randomized Trial of Patients With Crohn's Disease. Gastroenterology (2020) 158:2123–38.e2128. doi: 10.1053/j.gastro.2020.01.047
47. Sandborn WJ, Feagan BG, Radford-Smith G, Kovacs A, Enns R, Innes A, et al. CDP571, a Humanised Monoclonal Antibody to Tumour Necrosis Factor Alpha, for Moderate to Severe Crohn's Disease: A Randomised, Double Blind, Placebo Controlled Trial. Gut (2004) 53:1485–93. doi: 10.1136/gut.2003.035253
48. Sandborn WJ, Feagan BG, Rutgeerts P, Hanauer S, Colombel JF, Sands BE, et al. Vedolizumab as Induction and Maintenance Therapy for Crohn's Disease. N Engl J Med (2013) 369:711–21. doi: 10.1056/NEJMoa1215739
49. Sandborn WJ, Feagan BG, Stoinov S, Honiball PJ, Rutgeerts P, Mason D, et al. Certolizumab Pegol for the Treatment of Crohn's Disease. N Engl J Med (2007) 357:228–38. doi: 10.1056/NEJMoa067594
50. Sandborn WJ, Gasink C, Gao LL, Blank MA, Johanns J, Guzzo C, et al. Ustekinumab Induction and Maintenance Therapy in Refractory Crohn's Disease. N Engl J Med (2012) 367:1519–28. doi: 10.1056/NEJMoa1203572
51. Sandborn WJ, Ghosh S, Panes J, Vranic I, Wang W, Niezychowski W. A Phase 2 Study of Tofacitinib, an Oral Janus Kinase Inhibitor, in Patients With Crohn's Disease. Clin Gastroenterol Hepatol (2014) 12:1485–93.e1482. doi: 10.1016/j.cgh.2014.01.029
52. Sandborn WJ, Hanauer SB, Katz S, Safdi M, Wolf DG, Baerg RD, et al. Etanercept for Active Crohn's Disease: A Randomized, Double-Blind, Placebo-Controlled Trial. Gastroenterology (2001) 121:1088–94. doi: 10.1053/gast.2001.28674
53. Sandborn WJ, Rutgeerts P, Enns R, Hanauer SB, Colombel JF, Panaccione R, et al. Adalimumab Induction Therapy for Crohn Disease Previously Treated With Infliximab: A Randomized Trial. Ann Intern Med (2007) 146:829–38. doi: 10.7326/0003-4819-146-12-200706190-00159
54. Sands BE, Chen J, Feagan BG, Penney M, Rees WA, Danese S, et al. Efficacy and Safety of MEDI2070, an Antibody Against Interleukin 23, in Patients With Moderate to Severe Crohn's Disease: A Phase 2a Study. Gastroenterology (2017) 153:77–86.e76. doi: 10.1053/j.gastro.2017.03.049
55. Sands BE, Feagan BG, Rutgeerts P, Colombel JF, Sandborn WJ, Sy R, et al. Effects of Vedolizumab Induction Therapy for Patients With Crohn's Disease in Whom Tumor Necrosis Factor Antagonist Treatment Failed. Gastroenterology (2014) 147:618–27.e613. doi: 10.1053/j.gastro.2014.05.008
56. Sands BE, Jacobson EW, Sylwestrowicz T, Younes Z, Dryden G, Fedorak R, et al. Randomized, Double-Blind, Placebo-Controlled Trial of the Oral Interleukin-12/23 Inhibitor Apilimod Mesylate for Treatment of Active Crohn's Disease. Inflamm Bowel Dis (2010) 16:1209–18. doi: 10.1002/ibd.21159
57. Schreiber S, Siegel CA, Friedenberg KA, Younes ZH, Seidler U, Bhandari BR, et al. A Phase 2, Randomized, Placebo-Controlled Study Evaluating Matrix Metalloproteinase-9 Inhibitor, Andecaliximab, in Patients With Moderately to Severely Active Crohn's Disease. J Crohns Colitis (2018) 12:1014–20. doi: 10.1093/ecco-jcc/jjy070
58. Stack WA, Mann SD, Roy AJ, Heath P, Sopwith M, Freeman J, et al. Randomised Controlled Trial of CDP571 Antibody to Tumour Necrosis Factor-Alpha in Crohn's Disease. Lancet (1997) 349:521–4. doi: 10.1016/s0140-6736(97)80083-9
59. Targan SR, Feagan BG, Fedorak RN, Lashner BA, Panaccione R, Present DH, et al. Natalizumab for the Treatment of Active Crohn's Disease: Results of the ENCORE Trial. Gastroenterology (2007) 132:1672–83. doi: 10.1053/j.gastro.2007.03.024
60. Targan SR, Hanauer SB, Van Deventer SJ, Mayer L, Present DH, Braakman T, et al. A Short-Term Study of Chimeric Monoclonal Antibody Ca2 to Tumor Necrosis Factor Alpha for Crohn's Disease. Crohn's Disease Ca2 Study Group. N Engl J Med (1997) 337:1029–35. doi: 10.1056/nejm199710093371502
61. Vermeire S, Schreiber S, Petryka R, Kuehbacher T, Hebuterne X, Roblin X, et al. Clinical Remission in Patients With Moderate-to-Severe Crohn's Disease Treated With Filgotinib (the FITZROY Study): Results From a Phase 2, Double-Blind, Randomised, Placebo-Controlled Trial. Lancet (2017) 389:266–75. doi: 10.1016/s0140-6736(16)32537-5
62. Watanabe K, Motoya S, Ogata H, Kanai T, Matsui T, Suzuki Y, et al. Effects of Vedolizumab in Japanese Patients With Crohn's Disease: A Prospective, Multicenter, Randomized, Placebo-Controlled Phase 3 Trial With Exploratory Analyses. J Gastroenterol (2020) 55:291–306. doi: 10.1007/s00535-019-01647-w
63. Watanabe M, Hibi T, Lomax KG, Paulson SK, Chao J, Alam MS, et al. Adalimumab for the Induction and Maintenance of Clinical Remission in Japanese Patients With Crohn's Disease. J Crohns Colitis (2012) 6:160–73. doi: 10.1016/j.crohns.2011.07.013
64. Winter TA, Wright J, Ghosh S, Jahnsen J, Innes A, Round P. Intravenous CDP870, a PEGylated Fab' Fragment of a Humanized Antitumour Necrosis Factor Antibody, in Patients With Moderate-to-Severe Crohn's Disease: An Exploratory Study. Aliment Pharmacol Ther (2004) 20:1337–46. doi: 10.1111/j.1365-2036.2004.02285.x
65. Riis A, Martinsen TC, Waldum HL, Fossmark R. Clinical Experience With Infliximab and Adalimumab in a Single-Center Cohort of Patients With Crohn's Disease. Scand J Gastroenterol (2012) 47:649–57. doi: 10.3109/00365521.2012.672591
66. Van Assche G, Vermeire S, Ballet V, Gabriels F, Noman M, D'haens G, et al. Switch to Adalimumab in Patients With Crohn's Disease Controlled by Maintenance Infliximab: Prospective Randomised SWITCH Trial. Gut (2012) 61:229–34. doi: 10.1136/gutjnl-2011-300755
67. Miligkos M, Papamichael K, Vande Casteele N, Mantzaris GJ, Gils A, Levesque BG, et al. Efficacy and Safety Profile of Anti-Tumor Necrosis Factor-α Versus Anti-Integrin Agents for the Treatment of Crohn's Disease: A Network Meta-Analysis of Indirect Comparisons. Clin Ther (2016) 38:1342–58.e1346. doi: 10.1016/j.clinthera.2016.03.018
68. Stidham RW, Lee TC, Higgins PD, Deshpande AR, Sussman DA, Singal AG, et al. Systematic Review With Network Meta-Analysis: The Efficacy of Anti-TNF Agents for the Treatment of Crohn's Disease. Aliment Pharmacol Ther (2014) 39:1349–62. doi: 10.1111/apt.12749
69. Varu A, Wilson FR, Dyrda P, Hazel M, Hutton B, Cameron C. Treatment Sequence Network Meta-Analysis in Crohn's Disease: A Methodological Case Study. Curr Med Res Opin (2019) 35:733–56. doi: 10.1080/03007995.2019.1580094
70. Sturm A, Maaser C, Calabrese E, Annese V, Fiorino G, Kucharzik T, et al. ECCO-ESGAR Guideline for Diagnostic Assessment in IBD Part 2: IBD Scores and General Principles and Technical Aspects. J Crohns Colitis (2019) 13:273–84. doi: 10.1093/ecco-jcc/jjy114
71. Cholapranee A, Hazlewood GS, Kaplan GG, Peyrin-Biroulet L, Ananthakrishnan AN. Systematic Review With Meta-Analysis: Comparative Efficacy of Biologics for Induction and Maintenance of Mucosal Healing in Crohn's Disease and Ulcerative Colitis Controlled Trials. Aliment Pharmacol Ther (2017) 45:1291–302. doi: 10.1111/apt.14030
72. Austin PC, White IR, Lee DS, Van Buuren S. Missing Data in Clinical Research: A Tutorial on Multiple Imputation. Can J Cardiol (2020) 1322–31. doi: 10.1016/j.cjca.2020.11.010
73. Schmitz S, Adams R, Walsh CD, Barry M, Fitzgerald O. A Mixed Treatment Comparison of the Efficacy of Anti-TNF Agents in Rheumatoid Arthritis for Methotrexate Non-Responders Demonstrates Differences Between Treatments: A Bayesian Approach. Ann Rheum Dis (2012) 71:225–30. doi: 10.1136/annrheumdis-2011-200228
74. Mitrev N, Vande Casteele N, Seow CH, Andrews JM, Connor SJ, Moore GT, et al. Review Article: Consensus Statements on Therapeutic Drug Monitoring of Anti-Tumour Necrosis Factor Therapy in Inflammatory Bowel Diseases. Aliment Pharmacol Ther (2017) 46:1037–53. doi: 10.1111/apt.14368
75. Mandema JW, Gibbs M, Boyd RA, Wada DR, Pfister M. Model-Based Meta-Analysis for Comparative Efficacy and Safety: Application in Drug Development and Beyond. Clin Pharmacol Ther (2011) 90:766–9. doi: 10.1038/clpt.2011.242
76. Kiss LS, Szamosi T, Molnar T, Miheller P, Lakatos L, Vincze A, et al. Early Clinical Remission and Normalisation of CRP Are the Strongest Predictors of Efficacy, Mucosal Healing and Dose Escalation During the First Year of Adalimumab Therapy in Crohn's Disease. Aliment Pharmacol Ther (2011) 34:911–22. doi: 10.1111/j.1365-2036.2011.04827.x
77. Danese S, Fiorino G, Peyrin-Biroulet L. Early Intervention in Crohn's Disease: Towards Disease Modification Trials. Gut (2017) 66:2179–87. doi: 10.1136/gutjnl-2017-314519
78. Peyrin-Biroulet L, Loftus EV Jr, Colombel JF, Sandborn WJ. The Natural History of Adult Crohn's Disease in Population-Based Cohorts. Am J Gastroenterol (2010) 105:289–97. doi: 10.1038/ajg.2009.579
79. Higgins JP, Thompson SG. Controlling the Risk of Spurious Findings From Meta-Regression. Stat Med (2004) 23:1663–82. doi: 10.1002/sim.175
80. Lambert PC, Sutton AJ, Abrams KR, Jones DR. A Comparison of Summary Patient-Level Covariates in Meta-Regression With Individual Patient Data Meta-Analysis. J Clin Epidemiol (2002) 55:86–94. doi: 10.1016/s0895-4356(01)00414-0
81. Su C, Lichtenstein GR, Krok K, Brensinger CM, Lewis JD. A Meta-Analysis of the Placebo Rates of Remission and Response in Clinical Trials of Active Crohn's Disease. Gastroenterology (2004) 126:1257–69. doi: 10.1053/j.gastro.2004.01.024
Keywords: model-based meta-analysis, Crohn’s disease, biologics, small targeted molecules, relative efficacy
Citation: Yu B, Zhao L, Jin S, He H, Zhang J and Wang X (2022) Model-Based Meta-Analysis on the Efficacy of Biologics and Small Targeted Molecules for Crohn’s Disease. Front. Immunol. 13:828219. doi: 10.3389/fimmu.2022.828219
Received: 03 December 2021; Accepted: 14 February 2022;
Published: 17 March 2022.
Edited by:
Bruno Bonaz, Centre Hospitalier Universitaire de Grenoble, FranceReviewed by:
Javier P. Gisbert, Princess University Hospital, SpainChengliang Zhang, Huazhong University of Science and Technology, China
Fernando Gomollón, University of Zaragoza, Spain
Copyright © 2022 Yu, Zhao, Jin, He, Zhang and Wang. This is an open-access article distributed under the terms of the Creative Commons Attribution License (CC BY). The use, distribution or reproduction in other forums is permitted, provided the original author(s) and the copyright owner(s) are credited and that the original publication in this journal is cited, in accordance with accepted academic practice. No use, distribution or reproduction is permitted which does not comply with these terms.
*Correspondence: Jing Zhang, zhangjing@bch.com.cn; Xiaoling Wang, wangxiaoling@bch.com.cn
†These authors have contributed equally to this work and share first authorship