- Laboratory of Immunoregulation, Systems Biology and Immunology Department, Sidra Medicine, Doha, Qatar
Asthma is ranked among the most common chronic conditions and has become a significant public health issue due to the recent and rapid increase in its prevalence. Investigations into the underlying genetic factors predict a heritable component for its incidence, estimated between 35% and 90% of causation. Despite the application of large-scale genome-wide association studies (GWAS) and admixture mapping approaches, the proportion of variants identified accounts for less than 15% of the observed heritability of the disease. The discrepancy between the predicted heritable component of disease and the proportion of heritability mapped to the currently identified susceptibility loci has been termed the ‘missing heritability problem.’ Here, we examine recent studies involving both the analysis of genetically encoded features that contribute to asthma and also the role of non-encoded heritable characteristics, including epigenetic, environmental, and developmental aspects of disease. The importance of vertical maternal microbiome transfer and the influence of maternal immune factors on fetal conditioning in the inheritance of disease are also discussed. In order to highlight the broad array of biological inputs that contribute to the sum of heritable risk factors associated with allergic disease incidence that, together, contribute to the induction of a pro-atopic state. Currently, there is a need to develop in-depth models of asthma risk factors to overcome the limitations encountered in the interpretation of GWAS results in isolation, which have resulted in the missing heritability problem. Hence, multiomics analyses need to be established considering genetic, epigenetic, and functional data to create a true systems biology-based approach for analyzing the regulatory pathways that underlie the inheritance of asthma and to develop accurate risk profiles for disease.
Introduction
To address the epidemic of asthma currently faced by society, it is necessary to gain a deeper understanding of the interwoven elements that lead to the initiation of the disease state. Why a significant proportion of the population suffers from asthma, while the rest remain healthy, has yet to be adequately determined. Asthma is a serious worldwide health issue and is now counted among the most common of chronic conditions in industrialized nations with over 300 million people affected worldwide and approximately 250,000 deaths recorded on a yearly basis (1). While the initial sensitization to environmental allergens that is responsible for the occurrence of atopic asthma typically occurs during childhood, we postulate that the predisposition to develop atopy occurs very early in life. Mediated through the inheritance of genetic risk factors which act in combination with multiple non-genetically encoded factors that are passed on either prior to birth or in the immediate post-natal period leading to the establishment of a predisposition for atopic sensitization (2) and the development of asthma.
The incidence of asthma has rapidly increased by 3-5 fold over the last 40 years (3, 4). During this time, a large number of genetic contributors to asthma have been identified (5), indicating a significant genetic component to the disease. However, the rapid rise in the rate of disease, coupled with the strong familial association, indicates that this dramatic increase is also the result of alterations in non-genetically encoded heritable factors (6, 7). As such, asthma can be characterized as a highly complex syndrome with genetic, epigenetic, and environmental risk factors that contribute to the establishment of the disease. Multiple genetic components have been identified as either protective or contributory to disease susceptibility; with interactions between genetic, epigenetic, and non-genetic factors then setting a threshold of susceptibility for an individual’s environmental exposure to sensitizing allergens governing the onset of disease. Additionally, alterations in lifestyle associated with modern industrialized living conditions may be responsible for the increased risk, ultimately leading to the induction of disease in a growing proportion of the population (8). Interestingly, the major risk factor for developing asthma has been shown to be a familial history of disease (9), with maternal rates of inheritance contributing significantly more than paternal transmission rates (5-fold greater) (10). Estimations from GWAS indicate that the single nucleotide polymorphisms (SNPs) currently identified are only able to account for 2.5%-14% of asthma heritability (5). Whereas twin studies have shown asthma heritability rates in the adult population of up to 55%, with even higher estimates reported among young children (11, 12). The low rates of currently identified variants accounting for the heritability of asthma points to the importance of additional non-genetically encoded factors playing a role in the susceptibility to asthma and atopic sensitization. These observations have led to the development of ‘the missing heritability problem’, as a theory to help explain the limitation of GWAS and other genetic studies to elucidate the variance in heritability of disease (13).
Missing Heritability Theory
The advent of GWAS techniques along with the ability to sequence and identify variants that contribute to complex human diseases and traits has allowed the study of inherited predisposing factors, far beyond the scope provided through family linkage studies; with the latter previously proving to be powerful in the identification of Mendelian disorders caused by single gene variants (14).
Next generation sequencing (NGS) has driven the development of new tools for assaying the relative impact of rare variants that have much more modest effects in contributing to the phenotypic outcome (13). Inclusion of these rare variants into multiple variant analysis to account for the development of specific conditions has proved to be highly informative, where the variants have a relatively large effect on disease development (>two-fold increase).
In the classical example of macular degeneration (15), five discrete loci account for 50% of the genetic heritability observed. Conversely, early studies into the variants responsible for human height could only account for approximately 5% of the genetic heritability observed through twin studies (estimated at 50%-80%), with over 50 variants associated with human height (16). Whereas, more recent studies have been able to account for up to 45% of the genetic association between SNPs and height, by adapting the analysis of individual SNPs that contribute to the variance of a characteristic through the use of models that are able to simultaneously consider multiple SNP associations (16). These early studies identified a still unresolved problem in using GWAS to analyze inherited characteristics now known as the ‘missing heritability problem’ (13). Part of the issue in using such analysis lies in the fact that many of the identified SNPs have been shown to only confer a minor increase in the disease odds ratio (1.1-1.4), combined with the trend for these variants to be present in the population at low to rare minor allele frequencies. Asthma, similar to other complex traits, likely has significant degrees of heritability stemming from multiple non-genetically encoded factors, which may account for much of the ‘missing heritability problem’ observed when trying to calculate risk scores from GWAS results. This is evident when considering both the degree of heritability and the dramatic rise in the rate of asthma incidence in developed countries that have been observed over the past 40 years. Together, these data indicate that a significant interplay exists between the inheritance of predisposing genetic factors, with the inheritance of more malleable and adaptive components, including those epigenetic factors that work in concert with developmental and environmental cues (Figure 1). All of which need to be considered in order to fully understand the heritable risk factors associated with asthma inheritance (17).
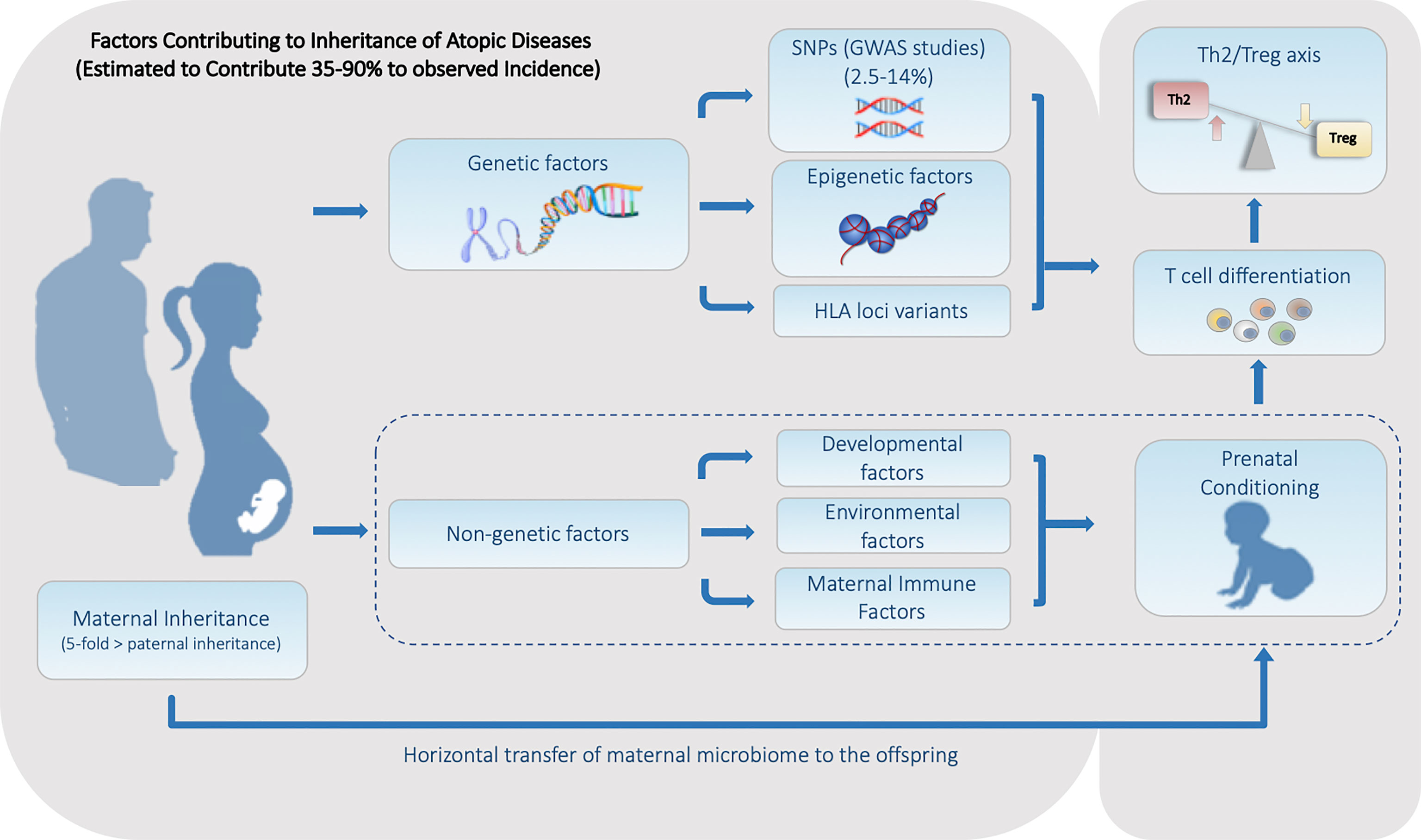
Figure 1 Summary of the Heritable Genetic and Non-Genetic Factors that Contribute to the Predisposition for Atopic Diseases. Factors can be divided into two major categories. (1) Genetic factors including genetically encoded features such as SNPs and HLA haplotype, along with alterable non-genetically encoded epigenetic features including DNA methylation and histone acetylation status. (2) Non-Genetic factors consisting of developmental, environmental, and maternal influences including the maternal microbiome is all transmitted during fetal and/or early neonatal development.
GWAS Discovery of Asthma Associated Genetic Linkages
The first GWAS of asthma was published in 2007 by Moffatt et al. who focused on mapping the effects of SNPs associated with childhood asthma and revealed the association of the ORMDL3 gene with susceptibility to asthma (18). Over the past 15 years, GWAS have identified tens of thousands of additional SNP variants associated with asthma-susceptibility loci mapped to both coding and non-coding regions of the genome (5, 19, 20). In a previous review by Kim et al. (20), the authors summarized the results from GWAS of asthma from the years 2008 to 2018 to show the importance of merging the GWAS findings with deep learning approaches to understand the genetic architecture of the asthma. To summarize the up-to-date results of GWAS of asthma, here we searched for “asthma” using the GWAS catalog database. A total of 29 asthma GWAS studies were conducted from July 2018 to November 2021 and an estimated 2000 SNPs were identified with 580 SNPs being replicated in at least two GWAS studies. (see Table 1: Summary of replicated SNPs in more than 4 GWAS.)
Several of the largest studies have been performed in the UK using data from the Trans-National Asthma Genetic Consortium (23,000 asthma cases versus 118,000 controls) (31) and the UK Biobank (64,000 cases versus 329,000 controls) (28). A meta-analysis of these two studies revealed 167 significantly associated loci of which 66 were novel loci; further accounting for an additional 1.5% of the reported asthma heritability, bringing the total estimate of heritability to approximately 8-9% (19). Together, these findings underscore the difficulty of determining the genetic basis of complex disorders, even when using extremely large cohorts and indicate that non-genetically encoded inherited features may be key to developing a comprehensive understanding of the inherited incidence of asthma.
A large percentage of the SNPs identified via GWAS are non-coding variants, mapping to either intergenic or intronic regions and are currently lacking biological evidence for their functional relevance (5). Studies of GWAS data combined with functional annotations and quantitative trait loci (QTL) have sought to strengthen their context-specific relevance. In addition to replicating previously known loci, new candidate genes including DGKQ, SLC26A1, and IDUA genes, as well as variants of genes encoding transcription factors like NF-KB, cJUN, STAT family, ERG1, ELF1, EBF1, and the GATA family have been identified (32–35). Atopy-associated GWAS have identified multiple relevant biological pathways, including autoimmune and inflammatory response pathways, thereby providing insights into the underlying heritable risk (31, 36). Interestingly, asthma-risk variants identified in several studies have been mapped to 17q12-21 locus, including ORMDL3, GSDML, and CDHR3 genes (35, 37–41) and have yielded several functional validations (42–44). In addition, variants of the TSLP gene, a cytokine secreted by epithelial cells that initiates allergic inflammation (45) have been replicated in several studies suggesting that this could be a potentially critical target for drug development (5, 35, 46, 47).
Many of the identified SNPs are known to be associated with pathways involved in the development and differentiation that regulate either the induction of inflammatory Th2 cells, which promotes the onset and progression of atopic asthma or are associated with maintenance of the Treg subset that is required to mediate immune homeostasis and tolerance to both self and innocuous environmental antigens (Figure 2A). Th2-associated genes identified include the master regulator of differentiation GATA3 and several genes associated with its upstream signaling pathways, including STAT6, IL4R, TSLP, and IL-33R. Further, multiple genes associated with Treg differentiation have also been implicated, including FOXP1 (53) and FOXP3 (54). Defects in FOXP3 can lead to an inability to generate Treg cells (55), leading to the spontaneous induction of allergic disease, further demonstrating the essential role of Treg cells in controlling spontaneous reactions to common environmental stimuli and the suppression of Th2 mediated diseases (56). GWAS have also detected multiple variants affecting Treg-related differentiation-promoting genes that are also associated with an increased risk of asthma, such as BACH2, IL2Rb, and SMAD3 (5) (Figure 2B).
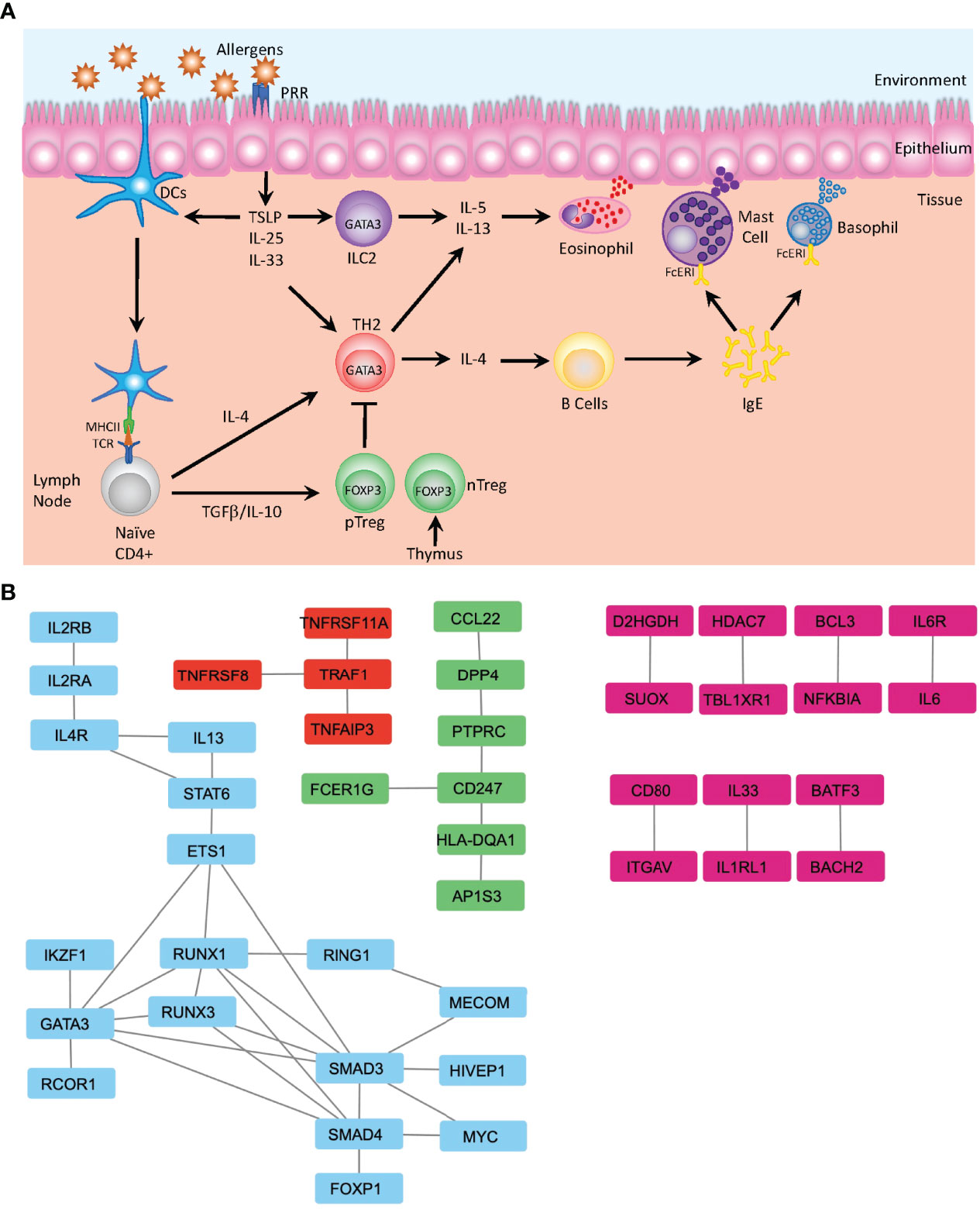
Figure 2 Mechanisms of Allergic Disease Development. (A) Representative image depicting the first stage of an allergic response driven by the recognition of environmental allergens as inflammatory mediators by pattern recognition receptors (PRR) present on the surface of dendritic cells (DC) and epithelial cells in barrier tissues. Epithelial cells produce defensive cytokines including TSLP, IL-25 and IL-33 that modulate activated DC and direct naïve T cells towards the TH2 phenotype. In the absence of inflammatory stimuli, naïve T cells may be activated in a tolerogenic manner and differentiate to become peripherally induced Treg cells (pTreg), which in concert with thymically produced T regs (nTreg), mediate the dampening of inflammatory responses. Activated TH2 cells produce IL-4 and mediate B cell class switching and production of IgE. Together with ILC2, Th2 cells additionally produce IL-5 and IL-13 that regulate the activation of the innate immune system and induces migration of eosinophils, mast cells and basophils to the sites of inflammation, where IgE bound to the FcERI receptors on mast cells and basophils recognizes the cognate allergen. This induces degranulation and release of further inflammatory mediators including histamine, leukotrienes, and heparin. (B) Analysis of protein-protein interactions of the 216 genes identified by GWAS from Han et al. (19) using the STRING database (48). Identification of several clusters of highly relevant pathways that overlay with the known functional pathways previously associated with asthma and allergic diseases, including the major cytokines signals associated with TH2 differentiation (IL-2, IL-4 and IL-13) along with the downstream mediators of differentiation—STAT6 and GATA3—and transcription factors associated with Treg differentiation—FOXP1 and SMAD—which are involved in the TGFβ signaling (blue). Additionally, the TRAF1 signaling nexus is identified, where TRAF1 has previously been identified as a key factor in allergic inflammation and the regulation of IL-4 production (red) (49). FcERI signaling and interactions between TCR and MHCII (HLA-DQA1) are identified (green) and multiple pairs of protein-protein interactions integral for development of allergic inflammation are also identified (pink)—notably IL-33-IL1RL1 (50) and BATF3-BACH2 (51). Data displayed is developed using Cytoscape v.3.9 (52).
It is important to note that genome-wide findings have some limitations in terms of the quality and quantity of samples gathered and methodological biases which may lead to significant false-positive associations. Additionally, the highly heterogeneous nature and multiple endotypes of complex diseases such as asthma (57) need to be adequately controlled. The lack of adequate controls potentially lead to studies being conducted that have been hampered by poor design, such as a lack of specificity in disease characteristics being used in order to gain a sufficiently large population size. Also, ignoring the effects of environmental interactions on disease subtypes potentially could lower the number of asthma-risk variants identified to date (17). Multiple asthma endotypes have now become well recognized, with atopic asthma being the most common form, affecting from 70%–90% of childhood and approximately 50% of adult patients (1). Patients with this phenotype typically have high levels of Th2 cytokines, significant eosinophilia, and high FeNO. In addition to atopic asthma, several other endotypes can be confirmed by differential diagnosis including: allergic bronchopulmonary aspergillosis, allergic bronchopulmonary mycosis, aspirin-exacerbated respiratory disease, late onset eosinophilic, paucigranulocytic, neutrophilic, and smoking related asthma (57, 58). In addition to recruitment of asthmatics for GWAS studies being complicated with multiple distinct endotypes and phenotypes, studies into rates of asthma misdiagnosis consistently report rates as high as 30% (59, 60). As the minimum criteria for inclusion in asthma related GWAS may only require the self-reporting of asthma or a previous medical diagnosis of asthma, this infers that a non-significant proportion of asthmatics included in large scale GWAS may not actually suffer from the disease, further confounding outcomes. On the contrary, a classification of asthma based on distinct phenotypes and endotypes allows for a more accurate clinical definition of the exact nature of the inflammatory response underlying disease and ultimately allows for the design of an optimal recruitment strategy. Where the phenotype reflects the observable characteristics of disease in a patient and endotype refers to the underlying biological processes that give rise to the observed properties of the phenotype (61). As such, specific biomarkers for disease endotypes as measurable characteristics of asthma subtype are becoming increasingly more essential for clinicians and researchers to complement a clinical diagnosis of phenotype and to identify the specific phenotypes and endotypes of asthma. Current biomarkers for endotype include such factors as IgE, allergen specific IgE (sIgE), eosinophil and neutrophil counts in blood and sputum, fraction of exhaled nitric oxide (FeNO), urinary Leukotriene E4, and an increasing array of SNPs (57). This approach is already suggesting entirely novel pathways to disease—e.g., alternative macrophage specification, steroid refractory innate immunity, the interleukin-17–regulatory T-cell axis, epidermal growth factor receptor co-amplification, and Th2-mimicking but non-T-cell, interleukins 18 and 33 dependent processes that can offer unexpected therapeutic opportunities for specific patient endotypes (62).
The use of GWAS analysis in combination with other genetic analysis tools, including expression quantitative trait loci (eQTL), admixture mapping, and polygenic risk scores (PRS), in addition to the study of the underlying biological pathways identified, may help to better understand the etiology of the disease. eQTL analysis is an effective tool used to identify the effects of genetic variations on gene expression analysis through direct testing of association between genetic variations and gene expression levels. In a recent eQTL analysis study, the authors generated a list of 154 asthma-associated genes and observed that the top 30 polymorphisms were linked to multiple gene transcripts, indicating that a single SNP may be responsible for regulating the function of multiple genes (63). Conversely, eQTL analysis has also been used to show a strong relationship between ORMDL3 transcription levels and multiple asthma-associated SNPs (18). Conversely, admixture mapping is a tool used to study genetic landscapes and assess the differential risks associated with the disease, allowing for the ancestry specific genomic risk association of a trait identified by GWAS to be compared against other ancestries and to look for alterations in the odds ratio associated with inheritance. The prevalence of asthma in African American and Latino/Hispanic populations are notable examples of where admixture mapping has yielded abundant data. Studies have identified genes responsible for both increases in the prevalence of asthma and a reduction in the response to common asthma medications in African American admixture populations when compared to those with European American ancestry (64). The genetic contribution to complex traits such as asthma, likely results from the accumulation of multiple SNPs with relatively minor effects. As such, the calculation of PRS, which has previously been widely used in animal and plant breeding (65) is a relatively new approach to better understand an individual’s overall inherited risk of disease. PRS values are obtained by weighting the effect size of the risk allele from the GWAS to the sum of the genomic risk alleles of an individual. Calculation of PRS is being rapidly applied in the public health sector to accelerate personalized preventive, diagnostic, and therapeutic strategies (66–68). A recent Canadian study, utilizing both GWAS loci previously identified in the literature and those found in two Canadian cohorts, explained that 37% of the variance in disease incidence was seen in a Canadian population (69). Allowing PRS to then be used to identify individuals who may benefit the most from timely interventions, such as the use of emollient moisturizers (70) or early introduction of potential food allergens as seen in the LEAP and EAT studies (71, 72). It was shown that the introduction of common allergens including peanuts (LEAP) and milk, peanut, sesame, fish, egg, and wheat (EAT) during the first year of life could prevent allergy in infants identified as either high-risk, sensitized, or non-sensitized. Despite these significant advances in identifying the underlying causes of allergic disease provided through GWAS and other forms of genetic analysis, there are still significant gaps in our current models of the disease development that can likely be filled through analysis of other, non-genetically-encoded forms of inheritance.
Epigenetic Inheritance of Asthma
Relatively recently, the field of asthma epigenetics has been greatly advanced with the introduction of epigenome-wide association studies (EWAS), in which changes in DNA methylation across the whole epigenome, of either particular cells or tissues, can be investigated to draw associations to asthma incidence. An excellent example of what can be accomplished using EWAS type analysis was recently provided following the CpG methylation meta-analysis from the Pregnancy and Childhood Epigenetics (PACE) consortium, in which over 3,000 sites were identified in newborns as being associated with maternal smoking, including several linked to an enhanced risk of asthma (73). Additionally, the application of EWAS has helped in the identification of differentially methylated regions in asthma-related genes including IL-4, IL-13, and RUNX3 (74). EWAS was also able to detect hypomethylation in genes related to eosinophils and cytotoxic T cell activation in whole blood of childhood asthmatic patients (75) and EWAS of sputum samples revealed the hypermethylation of the PCDH2 gene. This is an important factor in the maintenance of airway epithelial connectivity which has also been associated with asthma (76) and may play an integral role in barrier defense, similar to filaggrin in atopic dermatitis (77).
Further, a distinct CpG methylation profile associated with asthma was also found to be present following an EWAS meta-analysis of the cord blood collected from 8 cohorts of newborns (78). EWAS meta-analysis has also been applied to investigate cytokine interaction pathways related to asthma in blood of children with atopic asthma, identifying 35 hypermethylated and 95 hypomethylated genes associated to asthma development (79). In adults, DNA methylation associated with asthma has been applied to PBMCs, including eosinophils, neutrophils, and monocytes identifying 9 common hypermethylated genes. It also highlighted common pathways and gene expression networks associated with asthma, including 3 pathways in eosinophilic asthma and a novel network related to the Wnt signaling pathway in neutrophilic asthma (80). Moreover, several differentially methylated genes associated with asthma have been identified from nasal brushings of childhood atopic asthma patients (nasal methylome) (81), with several studies indicating that asthma associated epigenomic profiles obtained by EWAS can be tissue-specific (82).
With the emergence of NGS technologies, it is increasingly easier to study the developmental origins of asthma and to assess the epigenetic factors that contribute to the induction of disease (83). In particular, it is now possible to assess how fetal conditioning plays a role in setting thresholds for disease development by controlling genetic-epigenetic-environmental interactions. Previously, traditional immunological studies, GWAS, and studies of monogenic diseases with associated atopy have determined that CD4+ T cells are a crucial component in the establishment of asthma and other atopic diseases (84). Studies on the epigenetic regulation in these populations have revealed that both Th2 and Treg cell populations can be influenced in a heritable manner, with epigenetic changes at the IL4 promoter being associated with a predisposition to develop asthma (85, 86). In Tregs, the permissiveness of histone methylation at conserved non-coding sequences and the relative abundance of histone acetylases in comparison to histone deacetylases have emerged as key epigenetic factors linking Treg development with disease susceptibility (87). Additionally, alterations in the DNA methylation patterns in DC2 dendritic cells that prime Th2 differentiation have been observed (88). Human birth cohort studies have shown distinct methylation signatures of IL-4R and GATA3 genes being present at birth in children who developed asthma (89). Methylation of SMAD3 at birth, a locus previously identified by GWAS and known to regulate FOXP3 induction during Treg development (90) was also found to be strongly associated with the development of childhood asthma, potentially through a dysregulation of IL-1b signaling (91). Studies in both animal models and humans (85, 86) have shown evidence for the epigenetic heritability of allergic disease across generations (88). This leads to the theory that disease incidence can be passed to the next generation, both by shared environmental factors and by “induced epigenetic transmission” through both histone modifications and DNA methylation patterns, via the formation of metastable epialleles (92). Additionally, evidence of epigenetic transmission has been gained from studies on the impact of maternal smoking during pregnancy that showed increases for the risk of asthma in children (93, 94). Also, induction of differential DNA methylation in genes involved in the fundamental developmental processes of offspring of mothers who smoked (95) and, as mentioned above, a meta-analysis of 13 cohorts by the PACE consortium which identified nearly 3,000 CpG sites corresponding to differentially methylated genes in response to maternal smoking, including the ESR-1, ASRT and IL-32 genes were previously implicated in genetic studies of asthma (96). Furthermore, exposure to diesel particulate matter in mice during pregnancy was found not only to increase the incidence of asthma sensitivity in the F1 generation but also increase the sensitivity in the F2 and F3 generations. This finding correlates distinct and persistent changes in the methylation status of multiple genes with various chromatin modification pathways (97) and raises the possibility of the enhanced risk of asthma being transferred epigenetically through multiple generations via trans-generational epigenetic inheritance (98).
Together, these studies highlight the profound effects of epigenetic landscape alterations on the inheritance of asthma and the need to include an analysis of the impact of differential epigenetic modifications on gene expression when assessing potential asthma risk factors.
Asthma and the Transfer of Maternal Immune Factors
Previous studies report that maternal asthma is a greater risk factor than paternal asthma for the inheritance of the disease (5- fold greater) (9) and a lack of maternal asthma control during pregnancy imparts a greater risk of asthma/recurrent wheeze in the offspring when compared to a controlled maternal asthma status throughout pregnancy (9). During in utero development, the fetus is constantly exposed to an environment in which it is surrounded by the maternal metabolic and immune milieu (99). A growing number of studies indicate that the in utero environment of asthmatic mothers is distinct from that of non-asthmatic mothers, where the presence of those cytokines associated with asthma may cause developmental alterations (100), with reports indicating that both asthma and atopy have their origins during in utero development (93, 100). Maternal atopy has been found to be associated with differential methylation patterns in the cord blood of neonates (99). A study of 36 patients enrolled in the Infant Immune Study looking for DNA methylation signatures predictive of childhood asthma identified that cord blood mononuclear cells (CBMCs) harbored 589 differentially methylated regions (DMRs) associated with childhood asthma; further analysis of which showed a significant enrichment of DMRs in genes controlled by immunoregulatory TGF-β1 and pro-inflammatory IL-1β (101). Moreover, CBMCs of asthmatic children from asthmatic mothers secreted more of IL-1β and exhibited high SMAD3 methylation as compared to non-asthmatic children from asthmatic mothers (101). Additional cord blood studies show that the association of increased methylation of a functional CpG site in the IL-2 promoter increased the likelihood of severe asthma exacerbations, as well as hospital admissions for asthma/wheeze in children between 2 and 8 years of age (102). Moreover, Barton et al. showed that higher methylation of GATA3 CpGs-2211/-2209 at birth was associated with a reduced risk of asthma at 3 years of age (103). A very recent EWAS of cord-blood samples in a cohort of 96 mother-child pairs, recruited as part of the ongoing ELMA- Epigenetic Hallmark of Maternal Atopy and Diet study, indicated that maternal atopy was associated with specific epigenetic signatures in the offspring, identifying 83 CpG islands mapping to 50 genes associated with maternal atopy (99). The genes C20orf166, STAC, SYT8, KCNJ15, SCD, LINCOO669, PLEKHA2, ITM2C, NT5C3A and NPEPL1 had the most significant differentially methylated sites of which SCD, ITM2C, NT5C3A and NPEPL1 genes are known to impact the immune system, allergy, and asthma; thereby suggesting that maternal atopy constitutes a unique intrauterine environment which is associated with the induction of distinct methylation patterns associated with the inheritance of a pro-atopic state (99).
There is also evidence to suggest that the transmission of maternally derived immune factors during fetal development may be a significant source of inherited immunological conditioning. As in utero exposure to stress response factors (104) and inflammatory cytokines (105, 106) have both been found to be important risk factors for the development of asthma. During development, fetal and neonatal immune responses are considered immature and present significant differences to those in adults, displaying a significant skewing towards the development of type 2 inflammatory responses, as part of a mechanism that protects against fetal rejection by the maternal immune system (107). Maternal cytokine production levels have been shown to influence the development of the immune system, with higher maternal IFN-γ:IL-13 and IFN-γ:IL-4 ratios during pregnancy being associated with decreased risk for childhood asthma at the age of 5 (100) and a decreased IFN-γ:IL-4 ratio during the first trimester of pregnancy being associated with an increased risk of atopy (108). This coincides with an increased rate of Th2 cells and a significant decrease in Treg: Th2 cells ratio present at birth in the cord blood of children born to atopic mothers (109). Together, these data suggest that the bias towards generating a type 2 inflammatory response during fetal development may carry over into neonatal life, where exposure to otherwise innocuous environmental antigens could induce an inflammatory response, as opposed to a tolerogenic response.
Among other maternal factors thought to play a potentially significant role in the development of asthma are the presence of maternal cells in fetal lymph nodes, which occurs through a process known as maternal microchimerism (MMc) (110). While the presence of MMc has been found to be associated with chronic inflammation and autoimmune diseases, it has also been shown to be protective against the development of asthma (111). The transplacental migration of maternal cells leads to their localization in fetal lymph nodes, where fetal CD4+ Tregs have been shown to preferentially develop following exposure to maternally associated non-inherited antigens, creating an early tolerogenic environment (112). These initial fetal clonotypes have then been observed to exert a lasting influence on the development of the T cell repertoire which can be observed in the persistence of clonotypes into adulthood (113).
Breastfeeding provides a further potential avenue for the vertical transmission of familial traits. As the complement of maternal immunoglobulins, cytokines, and immune cells contained in breastmilk provide an early form of defense during the initial conditioning of the neonate to the external environment (114) and may play a key role in setting immune tolerance thresholds to potential allergens; an aspect of which is especially important during the first few weeks of life when the infant gut remains highly permeable to both macromolecules and cellular components (115). Currently, no conclusive mechanistic evidence exists for how the transfer of maternal factors is protective against asthma, but multiple meta-analyses have shown protective effects of breast feeding against the induction of asthma and atopic disease [reviewed extensively in (116)]. However, a recent study by Ramanan et al. (117) demonstrated that the transmission of maternal Ig via breast milk was able to induce a multigenerational setpoint that governs the generation of gut resident Tregs responsible for induction of tolerance. Establishment of a protective population of RORg+ Treg was shown to be dependent on the maternal transmission of IgA and was reliant on a double negative feedback pathway whereby RORg+ Treg and IgA+ B cells co-regulate each other. Transmission of maternal IgA depressed the induction of RORg+ Tregs, allowing the production of commensal-specific B cell production of IgA, which could in turn be passed on to the next generation. Notably, these effects were found to be non-genetic, non-epigenetic, and non-microbial and were carried over multiple generations, even when animals were continuously backcrossed into their original genetic strain. In human subjects, patients with food allergies have been shown to have reduced numbers of RORg+ Tregs, and tolerance induction mediated by commensal bacteria has been shown to be dependent on RORg+ Tregs (118). Together, these data, built on previous findings, indicate dysbiosis in IgA recognition patterns in children with allergies who are readily observable at one month of age, a time when the IgA antibodies present are predominantly of maternal origin in breast-fed children (119). The establishment of RORg+ Treg levels by maternal Ig-based factors could then provide an initial setpoint in determining the baseline atopic state for an individual, leading to a predisposition towards food allergies and then progressing through the atopic march and asthma in later life (120, 121).
Environmental and Microbial Factors in the Development of Atopic Disease
Apart from genetic susceptibilities, the incidence and progression of asthma are greatly influenced by early environmental exposures, with the likelihood of asthma development beginning with in utero exposures (93). Factors including maternal diet, environmental pollutants, maternal microbiome, and maternal consumption of antibiotics during pregnancy have all been identified as contributing risk factors (122). Maternal dietary patterns significantly influence childhood immune development and function and a balanced healthy diet during pregnancy has been shown to confer protection against childhood asthma (93). Specifically, the regular dietary intake of foods high in antioxidants, vitamin D, and omega-3 polyunsaturated fatty acids during pregnancy has been shown to have protective effects against the development of asthma and other atopic diseases (123, 124). A meta-analysis of epidemiological studies based on the maternal dietary profile during the gestational period and documentation of asthma in their offspring revealed that an appropriate dietary intake of vitamin D, vitamin E, and zinc reduces the risk of childhood wheezing (125). Additionally, a birth cohort study of mother-child pairs revealed that high maternal dietary intake of potential food allergens, including peanut, milk, and wheat, in early pregnancy could reduce the risk of mid-childhood allergy and asthma (126).
Considerable effects of early life environmental exposures have been found to play a critical role in governing the rates of childhood asthma. Multiple studies, including the PARSIFAL and GABRIEL cohorts, have shown that children born in rural farming families are more resistant to atopic diseases, including asthma, than children raised in more urban environments. This is potentially due to an exposure, during early life, to a wider range of microbes present in farming environments (127–132). Additionally, maternal exposure to a microbial environment associated with livestock has been shown to be important in shaping fetal immune responses (132). A recent study found that chronic exposure to either low-dose bacterial endotoxin (lipopolysaccharide) or farm dust conferred protection against house dust mite (HDM)-induced asthma (129). The study also revealed that levels of a ubiquitin-modifying enzyme, A20 (TNFAIP3 protein), present in the lung epithelium, were responsible for providing protection against asthma in children growing up on dairy farms. Consistent with these findings, a loss-of-function SNP present in the TNFAIP3 gene confers an increased risk of asthma in these children (129), highlighting the strong role of gene-environment interplay in the development of asthma.
A study of mothers living in farming environments that raise livestock showed increased rates of consumption of microbial-rich unpasteurized milk, which is associated with diverse maternal gut microbiomes and, in turn, confer protective effects against asthma development in their children (93). The maternal gut microbiome composition and the microbial metabolites produced, including short-chain fatty acids (SCFAs), have a direct impact on the development of the fetal immune system (133). The effects of SCFAs on atopy were further characterized in pregnant mice fed either on a high-fiber diet or given SCFAs in drinking water, both of which conferred protection against asthma in offspring, mediated via the induction of enhanced Treg cell numbers and function (130). Murine studies on the transmission of mammalian gut microbiota have also shown that vertical inheritance from mother to offspring is predominant over the horizontal transmission of microbiota, although this is most likely associated with pathogenicity (128). A paired longitudinal metagenomic study of mother and child stool samples in a Finnish birth cohort identified that although the mother’s dominant gut bacterial strains were often vertically transmitted to the offspring, non-dominant strain transmissions also occurred in the offspring (131). The maternal gut microbiome has been shown to be vertically inherited from mother to child both prenatally and based on the mode of delivery (127, 131), as well as postnatally through breastfeeding (134) where the infant gut microbiome co-develops along with the intestinal immune system following birth. Vaginally delivered babies develop gut microbial communities similar to maternal vaginal microbial composition, whereas, in case of caesarean sections, babies acquire gut microbial communities similar to the maternal skin microbiome profile (135). Inevitably, the composition of the maternal microbiome during pregnancy has a significant impact on fetal immune development and influences the predisposition of the offspring to develop asthma (133). Furthermore, exposure of the fetus to probiotic bacterial species Lactobacillus and Bifidobacteria in the pregnant mother confers increased resistance to atopic diseases in these children, and this was shown to be achieved through enhanced recruitment of Tregs to the fetus through cord blood (136, 137).
Conversely, maternal exposure to antibiotics during pregnancy can increase the risk of asthma in children (138). Maternal use of antibiotics induces perturbations in the composition of the indigenous gut microbiome, resulting in microbial dysbiosis. Maternal microbial dysbiosis can, in turn, negatively affect the inheritance of a healthy maternal microbiome in the fetus. Human cohort studies have shown that prenatal and perinatal exposure to antibiotics can result in an increased risk of childhood asthma (139). Findings from the Copenhagen Prospective Study on Asthma in Childhood identified an increased risk of asthma with maternal use of antibiotics in the third trimester of pregnancy, and the Danish National Birth Cohort study showed an increased risk of asthma in children in response to maternal use of antibiotics irrespective of the pregnancy stage (140). Administration of intrapartum antibiotics, such as Group B Streptococcus antigen, can disturb the vaginal microbiome composition (141), leading to disruption of maternal microbiome inheritance in the offspring. As found in the Canadian Healthy Infant Longitudinal Development (CHILD) study, children of mothers who received intrapartum antibiotics exhibited microbial dysbiosis at three months of age, which was characterized by low levels of Bacteroides and high levels of Enterococcus and Clostridium (142). Administration of longer-term intrapartum antibiotics has been shown to increase the risk of atopic dermatitis in children by up to 28.9% at the age of two (143). Together, these studies identify the importance of both developmental and environmental factors in modulating the asthma risk profile in offspring and demonstrate additional mechanisms for the inheritance of non-genetically encoded factors that have a direct influence on the incidence of disease.
Metabolomics and Proteomics Profiling in Asthmatics
In-depth metabolomic and proteomic analyses of samples from asthma patients have been assessed in a broad range of tissues including blood, urine, solid tissue biopsies, exhaled breath condensate (EBC), bronchoalveolar lavage fluid (BALF), sputum, and stool, showing distinct differential associations of proteins and metabolites with disease states (Table 2: Significant targets resulting from Metabolic and Proteomic studies in Asthmatic Patients). At a high-level view, the associations observed to correlate with asthma reflect those of general stress response mechanisms and involve both immune and inflammatory functions (144, 145), with many of the biomarkers identified, reflecting diverse inflammatory pathologies, which can be used to stratify asthma phenotypes and endotypes (146).
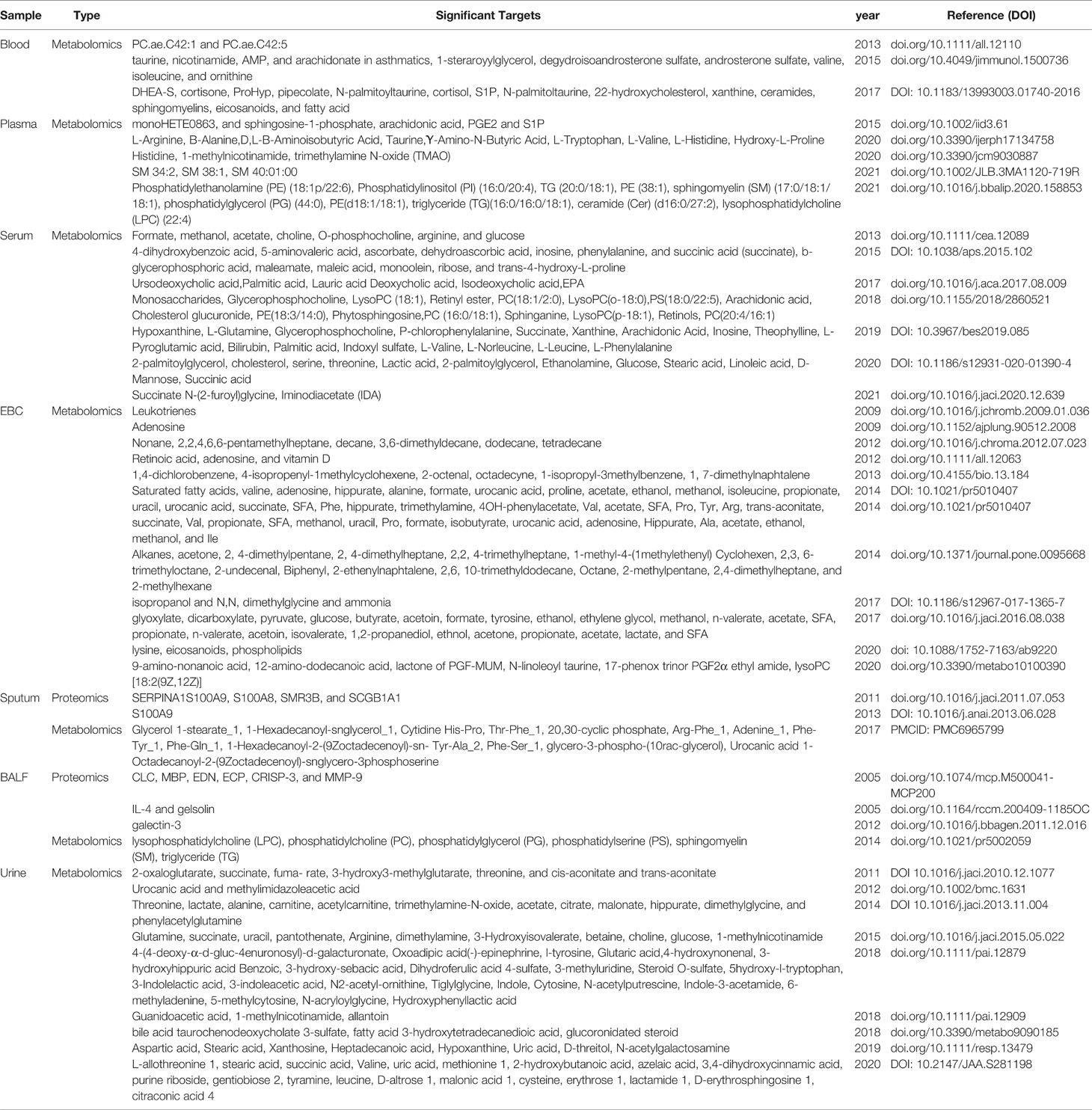
Table 2 Significant Targets Resulting from Metabolomic and Proteomic Studies of Samples Derived from Asthmatic Patients.
EBC is a very practical non-invasive method to collect samples, although it is still currently limited in the range of metabolites that can be detected. EBC methodology has been successfully applied, whereby differential metabolic profiles between healthy subjects and the severity of asthma subtypes could be readily distinguished (147–149). In comparison, proteomic analyses of asthmatic EBC and sputum show more fine-grained results, indicating alterations in the inflammatory state including levels of α1 antitrypsin, α2 macroglobulin, SERPINs, S100-family proteins, apolipoproteins, complement proteins, and Th2-related cytokines (150, 151). Whereas BALF and induced sputum samples have the potential to reflect the local pathophysiological state in a more direct and accurate manner, they are highly invasive and difficult to obtain. Interestingly, an analysis of a lipid repertoire present following metabolomic analysis of asthma patients noted that increases observed in asthmatic BALF samples were similar to those observed in the sputum and urine samples and mainly involved those lipids from glycerophospholipid and fatty acid metabolism associated pathways (152–154). Plasma metabolomics and proteomics patterns have also been used to indicate the presence of multiple and different asthma disease states within the broader population of asthmatic patients. Here, molecules involved in inflammation were found to include chaperone proteins, interferon response elements and leukocyte migration factors which were identified alongside those of allergy associated Th2 cytokines and were found to be differentially expressed between sub-phenotypes (155, 156). Additionally, studies focused on pediatric asthmatic populations have identified tryptophan, tyrosine, and biliary acids metabolism associated metabolites in the urine and plasma as markers that were predictive of the development and severity progression of the disease (157).
As such both protein and metabolite biomarkers are beginning to provide powerful new means to develop personalized diagnoses, treatments, and prognoses for asthma patients. Additionally, the use of these biomarkers should prove to be a highly useful tool for the accurate stratification of asthma endotypes/phenotypes when recruiting patients for GWAS. However, a better understanding of the impact and crosstalk between discovered biomarkers with the immune system is still needed not only to better define the variety of phenotype and endotypes associated with asthma, but also to evaluate the risk factors associated with developing asthma. Recently, the microbial metabolome has emerged as a new player that also requires consideration for both asthma predisposition and severity (158), with data indicating that microbial metabolites such as the short chain fatty acids butyrate (159) and propionate (160) are highly important for inducing the T regulatory subsets associated with homeostatic maintenance. Whereas other metabolites including 12,13-diHOME, may be responsible for induction of disease through induction of Th2 differentiation (2, 161) and recruitment of type 2 inflammatory cells including ILC2’s. Hence, future studies to form an in-depth understanding of the proteomic and metabolomic profiles present during pregnancy, stemming from both maternal and microbial sources, will be required to formally investigate how these factors may contribute to a pro-atopic state.
Current Application of Multiomics Analysis to Asthma Studies
While relatively few truly multiomics analyses of asthma have been generated to date, the breadth of data acquired so far in terms of epigenomic, genomic, metabolomic, microbiomic, proteomic and transcriptomic is quite staggering. This holds great promise for both application and incorporation of existing datasets for metanalytical studies and for use in complementing de novo studies. A recent methodology for utilization of omics data generated from multiple sources describes a promising pipeline for generating multiomics analyses from data collected in multiple studies, known as a Genome Wide Cross-Trait analysis Study (162). Here, multiple analyses including genetic correlation, cross-trait metanalysis, Mendelian randomization, PRS, and GWAS functional analysis are combined in an integrated manner to study genetic and functional effects on disease outcomes and associations. The application of this approach to data collected in the UK biobank, led to the identification of 38 genome-wide significant loci of which 7 were novel to the study and further analysis revealed that the shared loci were specifically enriched in immune/inflammatory systems and epithelial cells (163).
A similar strategy taken in Han et al. (19), began with the metanalysis of multiple GWAS datasets, analyzed enrichment of asthma associated loci at epigenetic markers, and incorporated EQTL data from GTEx Project and eQTLGen Consortium databases. This enabled the prioritization of loci for downstream verification, leading to the identification and functional validation of CD52 as a candidate causal gene for asthma and demonstrating the utility of integrating multiple omics datasets for target discovery. Additionally, multiomics approaches have been leveraged in order to better define asthmatic endotypes and to examine their longitudinal relation with asthma risk (164). Here, retroviral typing microbiome, cytokine, and metabolome data collected upon admission to hospital with bronchiolitis at <12 months of age were used in order to generate a model that identified biologically relevant bronchiolitis endotypes in infants who were at increased risk of developing asthma at 3 years of age.
Studies such as these highlight the importance and potential utility of applying multiomics based approaches to the study of asthma. However, significant difficulties still exist in comparing data generated on differing technologies due to both data heterogeneity and inherent biases in the systems used to generate these datasets. With the continued development of new methods and tools for bioinformatics analysis, including machine learning and deep learning techniques, these will undoubtedly help to ease the bottlenecks associated with the integration of high dimensional data generated from multiple sources in multiple modalities (165, 166).
Conclusion
The application of large-scale genome-wide association studies (GWAS) in asthma and atopic diseases over the last decade has led to the determination of a large number of gene variants linked to these conditions. Despite the power of genetic studies, the variants identified generally have small odds ratios and have only been able to partially explain the predicted heritability associated with the transfer of disease to the next generation. The rapid rise in the rates of these conditions over the past 50 years indicates that alterations in gene-environment and gene-developmental factors play a substantial role in the onset of disease, with multiple studies indicating that the identification of modifiable risk factors can exert a significant impact on the incidence of asthma. Furthermore, the observation that maternal incidence of asthma is significantly more predictive of childhood disease can now in part be explained by studies demonstrating that the transfer of immune factors during pre- and post-natal conditioning play a significant role in setting disease susceptibility thresholds. As the immune system first encounters the external environment and adapts to the subsequent colonization of the body by the microbiome, maternal factors play a key role during this time of life that is uniquely sensitive in terms of establishing a set point for a pro-atopic state. Future studies aimed at the integration of genomic risk factors via PRS in combination with multiomics analysis of cellular, epigenetic, and microbiome-influenced conditioning will allow the development of more accurate models, for both the prediction of disease, as well as for the application of targeted approaches aimed at reducing the disease prevalence.
Author Contributions
All authors listed have made a substantial, direct, and intellectual contribution to the work, and approved it for publication.
Conflict of Interest
The authors declare that the research was conducted in the absence of any commercial or financial relationships that could be construed as a potential conflict of interest.
Publisher’s Note
All claims expressed in this article are solely those of the authors and do not necessarily represent those of their affiliated organizations, or those of the publisher, the editors and the reviewers. Any product that may be evaluated in this article, or claim that may be made by its manufacturer, is not guaranteed or endorsed by the publisher.
Acknowledgments
We are grateful for the ongoing support of Sidra Medicine and the Qatar National Research Fund (QNRF grant award NPRP13S-0119-200102) for making this work possible. We would like to thank Editage (www.editage.com) for English language editing assistance.
References
1. Ali FR. Does This Patient Have Atopic Asthma? Clin Med (Lond) (2011) 11:376–80. doi: 10.7861/clinmedicine.11-4-376
2. Fujimura KE, Sitarik AR, Havstad S, Lin DL, Levan S, Fadrosh D, et al. Neonatal Gut Microbiota Associates With Childhood Multisensitized Atopy and T Cell Differentiation. Nat Med (2016) 22:1187–91. doi: 10.1038/nm.4176
3. Bach J-F. The Effect of Infections on Susceptibility to Autoimmune and Allergic Diseases. New Engl J Med (2002) 347:911–20. doi: 10.1056/NEJMra020100
4. Malik G, Tagiyeva N, Aucott L, McNeill G, Turner SW. Changing Trends in Asthma in 9-12 Year Olds Between 1964 and 2009. Arch Dis Child (2011) 96:227–31. doi: 10.1136/adc.2010.189175
5. Vicente CT, Revez JA, Ferreira MAR. Lessons From Ten Years of Genome-Wide Association Studies of Asthma. Clin Transl Immunol (2017) 6:e165. doi: 10.1038/cti.2017.54
6. Beasley R, Semprini A, Mitchell EA. Risk Factors for Asthma: Is Prevention Possible? Lancet (2015) 386:1075–85. doi: 10.1016/S0140-6736(15)00156-7
7. Thomsen SF. Exploring the Origins of Asthma: Lessons From Twin Studies. Eur Clin Respir J (2014) 2014:. doi: 10.3402/ecrj.v1.25535
8. Bijanzadeh M, Mahesh PA, Ramachandra NB. An Understanding of the Genetic Basis of Asthma. Indian J Med Res (2011) 134:149–61.
9. Mirzakhani H, Carey VJ, Zeiger R, Bacharier LB, O'Connor GT, Schatz MX, et al. Impact of Parental Asthma, Prenatal Maternal Asthma Control, and Vitamin D Status on Risk of Asthma and Recurrent Wheeze in 3-Year-Old Children. Clin Exp Allergy (2019) 49:419–29. doi: 10.1111/cea.13320
10. Litonjua AA, Carey VJ, Burge HA, Weiss ST, Gold DR. Parental History and the Risk for Childhood Asthma. Does Mother Confer More Risk Than Father? Am J Respir Crit Care Med (1998) 158:176–81. doi: 10.1164/ajrccm.158.1.9710014
11. Thomsen SF, Ulrik CS, Kyvik KO, Ferreira MA, Backer V. Multivariate Genetic Analysis of Atopy Phenotypes in a Selected Sample of Twins. Clin Exp Allergy (2006) 36:1382–90. doi: 10.1111/j.1365-2222.2006.02512.x
12. Thomsen SF, Ulrik CS, Kyvik KO, von Bornemann Hjelmborg J, Skadhauge LR, Steffensen I, et al. Genetic and Environmental Contributions to Hay Fever Among Young Adult Twins. Respir Med (2006) 100:2177–82. doi: 10.1016/j.rmed.2006.03.013
13. Manolio TA, Collins FS, Cox NJ, Goldstein DB, Hindorff LA, Hunter DJ, et al. Finding the Missing Heritability of Complex Diseases. Nature (2009) 461:747–53. doi: 10.1038/nature08494
14. Hardy J, Singleton A. Genomewide Association Studies and Human Disease. New Engl J Med (2009) 360:1759–68. doi: 10.1056/NEJMra0808700
15. Black JR, Clark SJ. Age-Related Macular Degeneration: Genome-Wide Association Studies to Translation. Genet Med (2016) 18:283–9. doi: 10.1038/gim.2015.70
16. Yang J, Benyamin B, McEvoy BP, Gordon S, Henders AK, Nyholt DR, et al. Common SNPs Explain a Large Proportion of the Heritability for Human Height. Nat Genet (2010) 42:565–9. doi: 10.1038/ng.608
17. Ober C. Asthma Genetics in the Post-GWAS Era. Ann Am Thorac Soc (2016) 13 Suppl 1:S85–90. doi: 10.1513/AnnalsATS.201507-459MG
18. Moffatt MF, Kabesch M, Liang L, Dixon AL, Strachan D, Heath S, et al. Genetic Variants Regulating ORMDL3 Expression Contribute to the Risk of Childhood Asthma. Nature (2007) 448:470–3. doi: 10.1038/nature06014
19. Han Y, Jia Q, Jahani PS, Hurrell BP, Pan C, Huang P, et al. Genome-Wide Analysis Highlights Contribution of Immune System Pathways to the Genetic Architecture of Asthma. Nat Commun (2020) 11:1776. doi: 10.1038/s41467-020-15649-3
20. Kim KW, Ober C. Lessons Learned From GWAS of Asthma. Allergy Asthma Immunol Res (2019) 11:170–87. doi: 10.4168/aair.2019.11.2.170
21. Ferreira MAR, Mathur R, Vonk JM, Szwajda A, Brumpton B, Granell R, et al. Genetic Architectures of Childhood- and Adult-Onset Asthma Are Partly Distinct. Am J Hum Genet (2019) 104:665–84. doi: 10.1016/j.ajhg.2019.02.022
22. Zhu Z, Guo Y, Shi H, Liu CL, Panganiban RA, Chung W, et al. Shared Genetic and Experimental Links Between Obesity-Related Traits and Asthma Subtypes in UK Biobank. J Allergy Clin Immunol (2020) 145:537–49. doi: 10.1016/j.jaci.2019.09.035
23. Shrine N, Portelli MA, John C, Soler Artigas M, Bennett N, Hall R, et al. Moderate-To-Severe Asthma in Individuals of European Ancestry: A Genome-Wide Association Study. Lancet Respir Med (2019) 7:20–34. doi: 10.1016/S2213-2600(18)30389-8
24. Pividori M, Schoettler N, Nicolae DL, Ober C, Im HK. Shared and Distinct Genetic Risk Factors for Childhood-Onset and Adult-Onset Asthma: Genome-Wide and Transcriptome-Wide Studies. Lancet Respir Med (2019) 7:509–22. doi: 10.1016/S2213-2600(19)30055-4
25. Olafsdottir TA, Theodors F, Bjarnadottir K, Bjornsdottir US, Agustsdottir AB, Stefansson OA, et al. Eighty-Eight Variants Highlight the Role of T Cell Regulation and Airway Remodeling in Asthma Pathogenesis. Nat Commun (2020) 11:393. doi: 10.1038/s41467-019-14144-8
26. Ishigaki K, Akiyama M, Kanai M, Takahashi A, Kawakami E, Sugishita H, et al. Large-Scale Genome-Wide Association Study in a Japanese Population Identifies Novel Susceptibility Loci Across Different Diseases. Nat Genet (2020) 52:669–79. doi: 10.1038/s41588-020-0640-3
27. Sakaue S, Kanai M, Tanigawa Y, Karjalainen J, Kurki M, Koshiba S, et al. A Cross-Population Atlas of Genetic Associations for 220 Human Phenotypes. Nat Genet (2021) 53:1415–24. doi: 10.1038/s41588-021-00931-x
28. Johansson A, Rask-Andersen M, Karlsson T, Ek WE. Genome-Wide Association Analysis of 350 000 Caucasians From the UK Biobank Identifies Novel Loci for Asthma, Hay Fever and Eczema. Hum Mol Genet (2019) 28:4022–41. doi: 10.1093/hmg/ddz175
29. Valette K, Li Z, Bon-Baret V, Chignon A, Berube JC, Eslami A, et al. Prioritization of Candidate Causal Genes for Asthma in Susceptibility Loci Derived From UK Biobank. Commun Biol (2021) 4:700. doi: 10.1038/s42003-021-02227-6
30. Daya M, Rafaels N, Brunetti TM, Chavan S, Levin AM, Shetty A, et al. Association Study in African-Admixed Populations Across the Americas Recapitulates Asthma Risk Loci in Non-African Populations. Nat Commun (2019) 10:880. doi: 10.1038/s41467-019-08469-7
31. Demenais F, Margaritte-Jeannin P, Barnes KC, Cookson WOC, Altmuller J, Ang W, et al. Multiancestry Association Study Identifies New Asthma Risk Loci That Colocalize With Immune-Cell Enhancer Marks. Nat Genet (2018) 50:42–53. doi: 10.1038/s41588-017-0014-7
32. Gao Y, Li J, Zhang Y, Zhang L. Replication Study of Susceptibility Variants Associated With Allergic Rhinitis and Allergy in Han Chinese. Allergy Asthma Clin Immunol (2020) 16:13. doi: 10.1186/s13223-020-0411-9
33. Hao K, Bosse Y, Nickle DC, Pare PD, Postma DS, Laviolette M, et al. Lung eQTLs to Help Reveal the Molecular Underpinnings of Asthma. PloS Genet (2012) 8:e1003029. doi: 10.1371/journal.pgen.1003029
34. Luo W, Obeidat M, Di Narzo AF, Chen R, Sin DD, Pare PD, et al. Airway Epithelial Expression Quantitative Trait Loci Reveal Genes Underlying Asthma and Other Airway Diseases. Am J Respir Cell Mol Biol (2016) 54:177–87. doi: 10.1165/rcmb.2014-0381OC
35. Gautam Y, Afanador Y, Ghandikota S, Mersha TB. Comprehensive Functional Annotation of Susceptibility Variants Associated With Asthma. Hum Genet (2020) 139(8):1037–58. doi: 10.1007/s00439-020-02151-5
36. Pickrell JK, Berisa T, Liu JZ, Segurel L, Tung JY, Hinds DA. Detection and Interpretation of Shared Genetic Influences on 42 Human Traits. Nat Genet (2016) 48:709–17. doi: 10.1038/ng.3570
37. Caliskan M, Bochkov YA, Kreiner-Moller E, Bonnelykke K, Stein MM, Du G, et al. Rhinovirus Wheezing Illness and Genetic Risk of Childhood-Onset Asthma. N Engl J Med (2013) 368:1398–407. doi: 10.1056/NEJMoa1211592
38. Bouzigon E, Corda E, Aschard H, Dizier MH, Boland A, Bousquet J, et al. Effect of 17q21 Variants and Smoking Exposure in Early-Onset Asthma. N Engl J Med (2008) 359:1985–94. doi: 10.1056/NEJMoa0806604
39. Bochkov YA, Watters K, Ashraf S, Griggs TF, Devries MK, Jackson DJ, et al. Cadherin-Related Family Member 3, A Childhood Asthma Susceptibility Gene Product, Mediates Rhinovirus C Binding and Replication. Proc Natl Acad Sci U.S.A. (2015) 112:5485–90. doi: 10.1073/pnas.1421178112
40. Bonnelykke K, Sleiman P, Nielsen K, Kreiner-Moller E, Mercader JM, Belgrave D, et al. A Genome-Wide Association Study Identifies CDHR3 as a Susceptibility Locus for Early Childhood Asthma With Severe Exacerbations. Nat Genet (2014) 46:51–5. doi: 10.1038/ng.2830
41. Wu H, Romieu I, Sienra-Monge JJ, Li H, del Rio-Navarro BE, London SJ. Genetic Variation in ORM1-Like 3 (ORMDL3) and Gasdermin-Like (GSDML) and Childhood Asthma. Allergy (2009) 64:629–35. doi: 10.1111/j.1398-9995.2008.01912.x
42. Loser S, Gregory LG, Zhang Y, Schaefer K, Walker SA, Buckley J, et al. Pulmonary ORMDL3 is Critical for Induction of Alternaria-Induced Allergic Airways Disease. J Allergy Clin Immunol (2017) 139:1496–1507 e3. doi: 10.1016/j.jaci.2016.07.033
43. Oyeniran C, Sturgill JL, Hait NC, Huang WC, Avni D, Maceyka M, et al. Aberrant ORM (Yeast)-Like Protein Isoform 3 (ORMDL3) Expression Dysregulates Ceramide Homeostasis in Cells and Ceramide Exacerbates Allergic Asthma in Mice. J Allergy Clin Immunol (2015) 136:1035–46 e6. doi: 10.1016/j.jaci.2015.02.031
44. Miller M, Broide DH. Why Is ORMDL3 on Chromosome 17q21 Highly Linked to Asthma? Am J Respir Crit Care Med (2019) 199:404–6. doi: 10.1164/rccm.201810-1941ED
45. Torgerson DG, Ampleford EJ, Chiu GY, Gauderman WJ, Gignoux CR, Graves PE, et al. Meta-Analysis of Genome-Wide Association Studies of Asthma in Ethnically Diverse North American Populations. Nat Genet (2011) 43:887–92. doi: 10.1038/ng.888
46. Watson B, Gauvreau GM. Thymic Stromal Lymphopoietin: A Central Regulator of Allergic Asthma. Expert Opin Ther Targets (2014) 18:771–85. doi: 10.1517/14728222.2014.915314
47. Gauvreau GM, O'Byrne PM, Boulet LP, Wang Y, Cockcroft D, Bigler J, et al. Effects of an Anti-TSLP Antibody on Allergen-Induced Asthmatic Responses. N Engl J Med (2014) 370:2102–10. doi: 10.1056/NEJMoa1402895
48. Szklarczyk D, Gable AL, Nastou KC, Lyon D, Kirsch R, Pyysalo S, et al. The STRING Database in 2021: Customizable Protein-Protein Networks, and Functional Characterization of User-Uploaded Gene/Measurement Sets. Nucleic Acids Res (2021) 49:D605–12. doi: 10.1093/nar/gkaa1074
49. Bryce PJ, Oyoshi MK, Kawamoto S, Oettgen HC, Tsitsikov EN. TRAF1 Regulates Th2 Differentiation, Allergic Inflammation and Nuclear Localization of the Th2 Transcription Factor, NIP45. Int Immunol (2006) 18:101–11. doi: 10.1093/intimm/dxh354
50. Molofsky AB, Van Gool F, Liang HE, Van Dyken SJ, Nussbaum JC, Lee J, et al. Interleukin-33 and Interferon-Gamma Counter-Regulate Group 2 Innate Lymphoid Cell Activation During Immune Perturbation. Immunity (2015) 43:161–74. doi: 10.1016/j.immuni.2015.05.019
51. Yamashita M, Kuwahara M. The Critical Role of Bach2 in Regulating Type 2 Chronic Airway Inflammation. Int Immunol (2018) 30:397–402. doi: 10.1093/intimm/dxy020
52. Shannon P, Markiel A, Ozier O, Baliga NS, Wang JT, Ramage D, et al. Cytoscape: A Software Environment for Integrated Models of Biomolecular Interaction Networks. Genome Res (2003) 13:2498–504. doi: 10.1101/gr.1239303
53. Ghosh S, Roy-Chowdhuri S, Kang K, Im SH, Rudra D. The Transcription Factor Foxp1 Preserves Integrity of an Active Foxp3 Locus in Extrathymic Treg Cells. Nat Commun (2018) 9:4473. doi: 10.1038/s41467-018-07018-y
54. Marques CR, Costa RS, Costa GNO, da Silva TM, Teixeira TO, de Andrade EMM, et al. Genetic and Epigenetic Studies of FOXP3 in Asthma and Allergy. Asthma Res Pract (2015) 1:10. doi: 10.1186/s40733-015-0012-4
55. Zhu J, Paul WE. Peripheral CD4+ T-Cell Differentiation Regulated by Networks of Cytokines and Transcription Factors. Immunol Rev (2010) 238:247–62. doi: 10.1111/j.1600-065X.2010.00951.x
56. Wan YY, Flavell RA. Regulatory T Cells, Transforming Growth Factor-Beta, and Immune Suppression. Proc Am Thorac Soc (2007) 4:271–6. doi: 10.1513/pats.200701-020AW
57. Carr TF, Kraft M. Use of Biomarkers to Identify Phenotypes and Endotypes of Severeasthma. Ann Allergy Asthma Immunol (2018) 121:414–20. doi: 10.1016/j.anai.2018.07.029
58. O'Neil SE, Lundback B, Lotvall J. Proteomics in Asthma and COPD Phenotypes and Endotypes for Biomarker Discovery and Improved Understanding of Disease Entities. J Proteomics (2011) 75:192–201. doi: 10.1016/j.jprot.2011.10.008
59. Saglani S, Menzie-Gow AN. Approaches to Asthma Diagnosis in Children and Adults. Front Pediatr (2019) 7:148. doi: 10.3389/fped.2019.00148
60. Kavanagh J, Jackson DJ, Kent BD. Over- and Under-Diagnosis in Asthma. Breathe (Sheff) (2019) 15:e20–7. doi: 10.1183/20734735.0362-2018
61. Lotvall J, Akdis CA, Bacharier LB, Bjermer L, Casale TB, Custovic A, et al. Asthma Endotypes: A New Approach to Classification of Disease Entities Within the Asthma Syndrome. J Allergy Clin Immunol (2011) 127:355–60. doi: 10.1016/j.jaci.2010.11.037
62. Asthma: Still More Questions Than Answers. Lancet (2008) 372(9643):1009. doi: 10.1016/S0140-6736(08)61414-2
63. El-Husseini ZW, Gosens R, Dekker F, Koppelman GH. The Genetics of Asthma and the Promise of Genomics-Guided Drug Target Discovery. Lancet Respir Med (2020) 8:1045–56. doi: 10.1016/S2213-2600(20)30363-5
64. Mersha TB. Mapping Asthma-Associated Variants in Admixed Populations. Front Genet (2015) 6:292. doi: 10.3389/fgene.2015.00292
65. Gianola D, Rosa GJ. One Hundred Years of Statistical Developments in Animal Breeding. Annu Rev Anim Biosci (2015) 3:19–56. doi: 10.1146/annurev-animal-022114-110733
66. Schork AJ, Schork MA, Schork NJ. Genetic Risks and Clinical Rewards. Nat Genet (2018) 50:1210–1. doi: 10.1038/s41588-018-0213-x
67. Khera AV, Chaffin M, Aragam KG, Haas ME, Roselli C, Choi SH, et al. Genome-Wide Polygenic Scores for Common Diseases Identify Individuals With Risk Equivalent to Monogenic Mutations. Nat Genet (2018) 50:1219–24. doi: 10.1038/s41588-018-0183-z
68. Torkamani A, Wineinger NE, Topol EJ. The Personal and Clinical Utility of Polygenic Risk Scores. Nat Rev Genet (2018) 19:581–90. doi: 10.1038/s41576-018-0018-x
69. Simard M, Madore AM, Girard S, Waserman S, Duan Q, Subbarao P, et al. Polygenic Risk Score for Atopic Dermatitis in the Canadian Population. J Allergy Clin Immunol (2021) 147:406–9. doi: 10.1016/j.jaci.2020.04.057
70. Horimukai K, Morita K, Narita M, Kondo M, Kitazawa H, Nozaki M, et al. Application of Moisturizer to Neonates Prevents Development of Atopic Dermatitis. J Allergy Clin Immunol (2014) 134:824–830 e6. doi: 10.1016/j.jaci.2014.07.060
71. Du Toit G, Roberts G, Sayre PH, Bahnson HT, Radulovic S, Santos AF, et al. Randomized Trial of Peanut Consumption in Infants at Risk for Peanut Allergy. New Engl J Med (2015) 372:803–13. doi: 10.1056/NEJMoa1414850
72. Perkin MR, Logan K, Marrs T, Radulovic S, Craven J, Flohr C, et al. Enquiring About Tolerance (EAT) Study: Feasibility of an Early Allergenic Food Introduction Regimen. J Allergy Clin Immunol (2016) 137:1477–1486 e8. doi: 10.1016/j.jaci.2015.12.1322
73. Joubert BR, Felix JF, Yousefi P, Bakulski KM, Just AC, Breton C, et al. DNA Methylation in Newborns and Maternal Smoking in Pregnancy: Genome-Wide Consortium Meta-Analysis. Am J Hum Genet (2016) 98:680–96. doi: 10.1016/j.ajhg.2016.02.019
74. Yang IV, Pedersen BS, Liu A, O'Connor GT, Teach SJ, Kattan M, et al. DNA Methylation and Childhood Asthma in the Inner City. J Allergy Clin Immunol (2015) 136:69–80. doi: 10.1016/j.jaci.2015.01.025
75. Xu CJ, Soderhall C, Bustamante M, Baiz N, Gruzieva O, Gehring U, et al. DNA Methylation in Childhood Asthma: An Epigenome-Wide Meta-Analysis. Lancet Respir Med (2018) 6:379–88. doi: 10.1016/S2213-2600(18)30052-3
76. Sood A, Petersen H, Blanchette CM, Meek P, Picchi MA, Belinsky SA, et al. Methylated Genes in Sputum Among Older Smokers With Asthma. Chest (2012) 142:425–31. doi: 10.1378/chest.11-2519
77. Drislane C, Irvine AD. The Role of Filaggrin in Atopic Dermatitis and Allergic Disease. Ann Allergy Asthma Immunol (2020) 124:36–43. doi: 10.1016/j.anai.2019.10.008
78. Reese SE, Xu CJ, den Dekker HT, Lee MK, Sikdar S, Ruiz-Arenas C, et al. Epigenome-Wide Meta-Analysis of DNA Methylation and Childhood Asthma. J Allergy Clin Immunol (2019) 143:2062–74. doi: 10.1016/j.jaci.2018.11.043
79. Chen R, Piao LZ, Liu L, Zhang XF. DNA Methylation and Gene Expression Profiles to Identify Childhood Atopic Asthma Associated Genes. BMC Pulm Med (2021) 21:292. doi: 10.1186/s12890-021-01655-8
80. Gunawardhana LP, Gibson PG, Simpson JL, Benton MC, Lea RA, Baines KJ. Characteristic DNA Methylation Profiles in Peripheral Blood Monocytes are Associated With Inflammatory Phenotypes of Asthma. Epigenetics (2014) 9:1302–16. doi: 10.4161/epi.33066
81. Yang IV, Pedersen BS, Liu AH, O'Connor GT, Pillai D, Kattan M, et al. The Nasal Methylome and Childhood Atopic Asthma. J Allergy Clin Immunol (2017) 139:1478–88. doi: 10.1016/j.jaci.2016.07.036
82. Cardenas A, Sordillo JE, Rifas-Shiman SL, Chung W, Liang L, Coull BA, et al. The Nasal Methylome as a Biomarker of Asthma and Airway Inflammation in Children. Nat Commun (2019) 10:3095. doi: 10.1038/s41467-019-11058-3
83. Long A, Bunning B, Sampath V, DeKruyff RH, Nadeau KC. Epigenetics and the Environment in Airway Disease: Asthma and Allergic Rhinitis. Adv Exp Med Biol (2020) 1253:153–81. doi: 10.1007/978-981-15-3449-2_6
84. Bonnelykke K, Sparks R, Waage J, Milner JD. Genetics of Allergy and Allergic Sensitization: Common Variants, Rare Mutations. Curr Opin Immunol (2015) 36:115–26. doi: 10.1016/j.coi.2015.08.002
85. Herz U, Joachim R, Ahrens B, Scheffold A, Radbruch A, Renz H. Prenatal Sensitization in a Mouse Model. Am J Respir Crit Care Med (2000) 162:S62–5. doi: 10.1164/ajrccm.162.supplement_2.ras-1
86. Song Y, Liu C, Hui Y, Srivastava K, Zhou Z, Chen J, et al. Maternal Allergy Increases Susceptibility to Offspring Allergy in Association With TH2-Biased Epigenetic Alterations in a Mouse Model of Peanut Allergy. J Allergy Clin Immunol (2014) 134:1339–1345 e7. doi: 10.1016/j.jaci.2014.08.034
87. Begin P, Nadeau KC. Epigenetic Regulation of Asthma and Allergic Disease. Allergy Asthma Clin Immunol (2014) 10:27. doi: 10.1186/1710-1492-10-27
88. Morkve Knudsen T, Rezwan FI, Jiang Y, Karmaus W, Svanes C, Holloway JW. Transgenerational and Intergenerational Epigenetic Inheritance in Allergic Diseases. J Allergy Clin Immunol (2018) 142:765–72. doi: 10.1016/j.jaci.2018.07.007
89. Barton SJ, Ngo S, Costello P, Garratt E, El-Heis S, Antoun E, et al. DNA Methylation of Th2 Lineage Determination Genes at Birth is Associated With Allergic Outcomes in Childhood. Clin Exp Allergy (2017) 47:1599–608. doi: 10.1111/cea.12988
90. Martinez GJ, Zhang Z, Chung Y, Reynolds JM, Lin X, Jetten AM, et al. Smad3 Differentially Regulates the Induction of Regulatory and Inflammatory T Cell Differentiation. J Biol Chem (2009) 284:35283–6. doi: 10.1074/jbc.C109.078238
91. DeVries A, Wlasiuk G, Miller SJ, Bosco A, Stern DA, Lohman IC, et al. Epigenome-Wide Analysis Links SMAD3 Methylation at Birth to Asthma in Children of Asthmatic Mothers. J Allergy Clin Immunol (2017) 140:534–42. doi: 10.1016/j.jaci.2016.10.041
92. Dolinoy DC, Das R, Weidman JR, Jirtle RL. Metastable Epialleles, Imprinting, and the Fetal Origins of Adult Diseases. Pediatr Res (2007) 61:30R–7R. doi: 10.1203/pdr.0b013e31804575f7
93. Grieger JA, Clifton VL, Tuck AR, Wooldridge AL, Robertson SA, Gatford KL. In Utero Programming of Allergic Susceptibility. Int Arch Allergy Immunol (2016) 169:80–92. doi: 10.1159/000443961
94. Silvestri M, Franchi S, Pistorio A, Petecchia L, Rusconi F. Smoke Exposure, Wheezing, and Asthma Development: A Systematic Review and Meta-Analysis in Unselected Birth Cohorts. Pediatr Pulm (2015) 50:353–62. doi: 10.1002/ppul.23037
95. Richmond RC, Simpkin AJ, Woodward G, Gaunt TR, Lyttleton O, McArdle WL, et al. Prenatal Exposure to Maternal Smoking and Offspring DNA Methylation Across the Lifecourse: Findings From the Avon Longitudinal Study of Parents and Children (ALSPAC). Hum Mol Genet (2015) 24:2201–17. doi: 10.1093/hmg/ddu739
96. Joubert BR, Felix JF, Yousefi P, Bakulski KM, Just AC, Breton C, et al. DNA Methylation in Newborns and Maternal Smoking in Pregnancy: Genome-Wide Consortium Meta-Analysis. Am J Hum Genet (2016) 98:680–96. doi: 10.1016/j.ajhg.2016.02.019
97. Gregory DJ, Kobzik L, Yang Z, McGuire CC, Fedulov AV. Transgenerational Transmission of Asthma Risk After Exposure to Environmental Particles During Pregnancy. Am J Physiol Lung Cell Mol Physiol (2017) 313:L395–405. doi: 10.1152/ajplung.00035.2017
98. Tuscher JJ, Day JJ. Multigenerational Epigenetic Inheritance: One Step Forward, Two Generations Back. Neurobiol Dis (2019) 132:104591. doi: 10.1016/j.nbd.2019.104591
99. Danielewicz H, Gurgul A, Dębińska A, Myszczyszyn G, Szmatoła T, Myszkal A, et al. Maternal Atopy and Offspring Epigenome-Wide Methylation Signature. Epigenetics (2021) 16:629–41. doi: 10.1080/15592294.2020.1814504
100. Rothers J, Stern DA, Lohman IC, Spangenberg A, Wright AL, DeVries A, et al. Maternal Cytokine Profiles During Pregnancy Predict Asthma in Children of Mothers Without Asthma. Am J Respir Cell Mol Biol (2018) 59:592–600. doi: 10.1165/rcmb.2017-0410OC
101. DeVries A, Wlasiuk G, Miller SJ, Bosco A, Stern DA, Nicodemus-Johnson J, et al. Neonatal Epigenetic Predictors of Childhood Asthma Map to Immunoregulatory and Pro-Inflammatory Pathways, B59. Asthma-Like Phenotype: Emergence (Epi)Genetics And Targeted Transgenesis Am Thorac Soc (2015) A3524–4. doi: 10.1164/ajrccm-conference.2015.191.1_MeetingAbstracts.A3524
102. Curtin JA, Simpson A, Belgrave D, Semic-Jusufagic A, Custovic A, Martinez FD. Methylation of IL-2 Promoter at Birth Alters the Risk of Asthma Exacerbations During Childhood. Clin Exp Allergy (2013) 43:304–11. doi: 10.1111/cea.12046
103. Barton SJ, Ngo S, Costello P, Garratt E, El-Heis S, Antoun E, et al. DNA Methylation of Th2 Lineage Determination Genes at Birth is Associated With Allergic Outcomes in Childhood. Clin Exp Allergy (2017) 47:1599–608. doi: 10.1111/cea.12988
104. Rosa MJ, Lee AG, Wright RJ. Evidence Establishing a Link Between Prenatal and Early-Life Stress and Asthma Development. Curr Opin Allergy Clin Immunol (2018) 18:148–58. doi: 10.1097/ACI.0000000000000421
105. McFadden JP, Thyssen JP, Basketter DA, Puangpet P, Kimber I. T Helper Cell 2 Immune Skewing in Pregnancy/Early Life: Chemical Exposure and the Development of Atopic Disease and Allergy. Br J Dermatol (2015) 172:584–91. doi: 10.1111/bjd.13497
106. Halonen M, Lohman IC, Stern DA, Ellis WL, Rothers J, Wright AL. Perinatal Tumor Necrosis Factor-Alpha Production, Influenced by Maternal Pregnancy Weight Gain, Predicts Childhood Asthma. Am J Respir Crit Care Med (2013) 188:35–41. doi: 10.1164/rccm.201207-1265OC
107. von Mutius E, Smits HH. Primary Prevention of Asthma: From Risk and Protective Factors to Targeted Strategies for Prevention. Lancet (2020) 396:854–66. doi: 10.1016/S0140-6736(20)31861-4
108. Kim JH, Kim KH, Woo HY, Shim JY. Maternal Cytokine Production During Pregnancy and the Development of Childhood Wheezing and Allergic Disease in Offspring Three Years of Age. J Asthma (2008) 45:948–52. doi: 10.1080/02770900802419676
109. Fu Y, Lou H, Wang C, Lou W, Wang Y, Zheng T, et al. T Cell Subsets in Cord Blood are Influenced by Maternal Allergy and Associated With Atopic Dermatitis. Pediatr Allergy Immunol (2013) 24:178–86. doi: 10.1111/pai.12050
110. Chougnet CA. Human Fetal Immune Cells Fight Back. Sci Transl Med (2018) 10(438):eaat3910. doi: 10.1126/scitranslmed.aat3910
111. Thompson EE, Myers RA, Du G, Aydelotte TM, Tisler CJ, Stern DA, et al. Maternal Microchimerism Protects Against the Development of Asthma. J Allergy Clin Immunol (2013) 132:39–44. doi: 10.1016/j.jaci.2012.12.1575
112. Mold JE, Michaelsson J, Burt TD, Muench MO, Beckerman KP, Busch MP, et al. Maternal Alloantigens Promote the Development of Tolerogenic Fetal Regulatory T Cells In Utero. Science (2008) 322:1562–5. doi: 10.1126/science.1164511
113. Pogorelyy MV, Elhanati Y, Marcou Q, Sycheva AL, Komech EA, Nazarov VI, et al. Persisting Fetal Clonotypes Influence the Structure and Overlap of Adult Human T Cell Receptor Repertoires. PloS Comput Biol (2017) 13:e1005572. doi: 10.1371/journal.pcbi.1005572
114. Moles JP, Tuaillon E, Kankasa C, Bedin AS, Nagot N, Marchant A, et al. Breastmilk Cell Trafficking Induces Microchimerism-Mediated Immune System Maturation in the Infant. Pediatr Allergy Immunol (2018) 29:133–43. doi: 10.1111/pai.12841
115. Reisinger KW, de Vaan L, Kramer BW, Wolfs TG, van Heurn LW, Derikx JP. Breast-Feeding Improves Gut Maturation Compared With Formula Feeding in Preterm Babies. J Pediatr Gastroenterol Nutr (2014) 59:720–4. doi: 10.1097/MPG.0000000000000523
116. Castro-Rodriguez JA, Forno E, Rodriguez-Martinez CE, Celedon JC. Risk and Protective Factors for Childhood Asthma: What Is the Evidence? J Allergy Clin Immunol Pract (2016) 4:1111–22. doi: 10.1016/j.jaip.2016.05.003
117. Ramanan D, Sefik E, Galvan-Pena S, Wu M, Yang L, Yang Z, et al. An Immunologic Mode of Multigenerational Transmission Governs a Gut Treg Setpoint. Cell (2020) 181:1276–1290 e13. doi: 10.1016/j.cell.2020.04.030
118. Abdel-Gadir A, Stephen-Victor E, Gerber GK, Noval Rivas M, Wang S, Harb H, et al. Microbiota Therapy Acts via a Regulatory T Cell MyD88/RORgammat Pathway to Suppress Food Allergy. Nat Med (2019) 25:1164–74. doi: 10.1038/s41591-019-0461-z
119. Dzidic M, Abrahamsson TR, Artacho A, Bjorksten B, Collado MC, Mira A, et al. Aberrant IgA Responses to the Gut Microbiota During Infancy Precede Asthma and Allergy Development. J Allergy Clin Immunol (2017) 139:1017–1025 e14. doi: 10.1016/j.jaci.2016.06.047
120. Alduraywish SA, Lodge CJ, Campbell B, Allen KJ, Erbas B, Lowe AJ, et al. The March From Early Life Food Sensitization to Allergic Disease: A Systematic Review and Meta-Analyses of Birth Cohort Studies. Allergy (2016) 71:77–89. doi: 10.1111/all.12784
121. Hill DA, Spergel JM. The Atopic March: Critical Evidence and Clinical Relevance. Ann Allergy Asthma Immunol (2018) 120:131–7. doi: 10.1016/j.anai.2017.10.037
122. Martino D, Prescott S. Epigenetics and Prenatal Influences on Asthma and Allergic Airways Disease. Chest (2011) 139:640–7. doi: 10.1378/chest.10-1800
123. Baïz N, Just J, Chastang J, Forhan A, de Lauzon-Guillain B, Magnier AM, et al. Maternal Diet Before and During Pregnancy and Risk of Asthma and Allergic Rhinitis in Children. Allergy Asthma Clin Immunol (2019) 15:40. doi: 10.1186/s13223-019-0353-2
124. Nwaru BI, Erkkola M, Lumia M, Kronberg-Kippilä C, Ahonen S, Kaila M, et al. Maternal Intake of Fatty Acids During Pregnancy and Allergies in the Offspring. Br J Nutr (2012) 108:720–32. doi: 10.1017/S0007114511005940
125. Beckhaus AA, Garcia-Marcos L, Forno E, Pacheco-Gonzalez RM, Celedón JC, Castro-Rodriguez JA. Maternal Nutrition During Pregnancy and Risk of Asthma, Wheeze, and Atopic Diseases During Childhood: A Systematic Review and Meta-Analysis. Allergy (2015) 70:1588–604. doi: 10.1111/all.12729
126. Bunyavanich S, Rifas-Shiman SL, Platts-Mills TA, Workman L, Sordillo JE, Camargo CA Jr., et al. Peanut, Milk, and Wheat Intake During Pregnancy is Associated With Reduced Allergy and Asthma in Children. J Allergy Clin Immunol (2014) 133:1373–82. doi: 10.1016/j.jaci.2013.11.040
127. Biasucci G, Rubini M, Riboni S, Morelli L, Bessi E, Retetangos C. Mode of Delivery Affects the Bacterial Community in the Newborn Gut. Early Hum Dev (2010) 86 Suppl 1:13–5. doi: 10.1016/j.earlhumdev.2010.01.004
128. Moeller AH, Suzuki TA, Phifer-Rixey M, Nachman MW. Transmission Modes of the Mammalian Gut Microbiota. Sci (New York NY) (2018) 362:453–7. doi: 10.1126/science.aat7164
129. Schuijs MJ, Willart MA, Vergote K, Gras D, Deswarte K, Ege MJ, et al. Farm Dust and Endotoxin Protect Against Allergy Through A20 Induction in Lung Epithelial Cells. Science (2015) 349:1106–10. doi: 10.1126/science.aac6623
130. Thorburn AN, McKenzie CI, Shen S, Stanley D, Macia L, Mason LJ, et al. Evidence That Asthma is a Developmental Origin Disease Influenced by Maternal Diet and Bacterial Metabolites. Nat Commun (2015) 6:7320. doi: 10.1038/ncomms8320
131. Yassour M, Vatanen T, Siljander H, Hämäläinen AM, Härkönen T, Ryhänen SJ, et al. Natural History of the Infant Gut Microbiome and Impact of Antibiotic Treatment on Bacterial Strain Diversity and Stability. Sci Trans Med (2016) 8:343ra81. doi: 10.1126/scitranslmed.aad0917
132. Ege MJ, Mayer M, Normand AC, Genuneit J, Cookson WO, Braun-Fahrländer C, et al. Exposure to Environmental Microorganisms and Childhood Asthma. New Engl J Med (2011) 364:701–9. doi: 10.1056/NEJMoa1007302
133. Vuillermin PJ, Macia L, Nanan R, Tang ML, Collier F, Brix S. The Maternal Microbiome During Pregnancy and Allergic Disease in the Offspring. Semin Immunopathol (2017) 39:669–75. doi: 10.1007/s00281-017-0652-y
134. Bäckhed F, Roswall J, Peng Y, Feng Q, Jia H, Kovatcheva-Datchary P, et al. Dynamics and Stabilization of the Human Gut Microbiome During the First Year of Life. Cell Host Microbe (2015) 17:690–703. doi: 10.1016/j.chom.2015.04.004
135. Knoop KA, Holtz LR, Newberry RD. Inherited Nongenetic Influences on the Gut Microbiome and Immune System. Birth Defects Res (2018) 110:1494–503. doi: 10.1002/bdr2.1436
136. Navarro S, Cossalter G, Chiavaroli C, Kanda A, Fleury S, Lazzari A, et al. The Oral Administration of Bacterial Extracts Prevents Asthma via the Recruitment of Regulatory T Cells to the Airways. Mucosal Immunol (2011) 4:53–65. doi: 10.1038/mi.2010.51
137. Schaub B, Liu J, Höppler S, Schleich I, Huehn J, Olek S, et al. Maternal Farm Exposure Modulates Neonatal Immune Mechanisms Through Regulatory T Cells. J Allergy Clin Immunol (2009) 123:774–82.e5. doi: 10.1016/j.jaci.2009.01.056
138. Shu SA, Yuen AWT, Woo E, Chu KH, Kwan HS, Yang GX, et al. Microbiota and Food Allergy. Clin Rev Allergy Immunol (2019) 57:83–97. doi: 10.1007/s12016-018-8723-y
139. Lapin B, Piorkowski J, Ownby D, Freels S, Chavez N, Hernandez E, et al. Relationship Between Prenatal Antibiotic Use and Asthma in at-Risk Children. Ann Allergy Asthma Immunol (2015) 114:203–7. doi: 10.1016/j.anai.2014.11.014
140. Stensballe LG, Simonsen J, Jensen SM, Bønnelykke K, Bisgaard H. Use of Antibiotics During Pregnancy Increases the Risk of Asthma in Early Childhood. J Pediatr (2013) 162:832–838.e3. doi: 10.1016/j.jpeds.2012.09.049
141. Cheng Q, Nelson D, Zhu S, Fischetti VA. Removal of Group B Streptococci Colonizing the Vagina and Oropharynx of Mice With a Bacteriophage Lytic Enzyme. Antimicrob Agents Chemother (2005) 49:111–7. doi: 10.1128/AAC.49.1.111-117.2005
142. Azad MB, Konya T, Persaud RR, Guttman DS, Chari RS, Field CJ, et al. Impact of Maternal Intrapartum Antibiotics, Method of Birth and Breastfeeding on Gut Microbiota During the First Year of Life: A Prospective Cohort Study. BJOG (2016) 123:983–93. doi: 10.1111/1471-0528.13601
143. Wohl DL, Curry WJ, Mauger D, Miller J, Tyrie K. Intrapartum Antibiotics and Childhood Atopic Dermatitis. J Am Board Family Med (2015) 28:82–9. doi: 10.3122/jabfm.2015.01.140017
144. Wang C, Jiang S, Zhang S, Ouyang Z, Wang G, Wang F. Research Progress of Metabolomics in Asthma. Metabolites (2021) 11(9). doi: 10.3390/metabo11090567
145. Kelly RS, Dahlin A, McGeachie MJ, Qiu W, Sordillo J, Wan ES, et al. Asthma Metabolomics and the Potential for Integrative Omics in Research and the Clinic. Chest (2017) 151:262–77. doi: 10.1016/j.chest.2016.10.008
146. Sim S, Choi Y, Park HS. Potential Metabolic Biomarkers in Adult Asthmatics. Metabolites (2021) 11(7). doi: 10.3390/metabo11070430
147. Sinha A, Desiraju K, Aggarwal K, Kutum R, Roy S, Lodha R, et al. Exhaled Breath Condensate Metabolome Clusters for Endotype Discovery in Asthma. J Transl Med (2017) 15:262. doi: 10.1186/s12967-017-1365-7
148. Chang-Chien J, Huang HY, Tsai HJ, Lo CJ, Lin WC, Tseng YL, et al. Metabolomic Differences of Exhaled Breath Condensate Among Children With and Without Asthma. Pediatr Allergy Immunol (2021) 32:264–72. doi: 10.1111/pai.13368
149. Ferraro VA, Carraro S, Pirillo P, Gucciardi A, Poloniato G, Stocchero M, et al. Breathomics in Asthmatic Children Treated With Inhaled Corticosteroids. Metabolites (2020) 10(10). doi: 10.3390/metabo10100390
150. Terracciano R, Pelaia G, Preiano M, Savino R. Asthma and COPD Proteomics: Current Approaches and Future Directions. Proteomics Clin Appl (2015) 9:203–20. doi: 10.1002/prca.201400099
151. Ferreira MA. Cytokine Expression in Allergic Inflammation: Systematic Review of In Vivo Challenge Studies. Mediators Inflammation (2003) 12:259–67. doi: 10.1080/09629350310001619717
152. Kang YP, Lee WJ, Hong JY, Lee SB, Park JH, Kim D, et al. Novel Approach for Analysis of Bronchoalveolar Lavage Fluid (BALF) Using HPLC-QTOF-MS-Based Lipidomics: Lipid Levels in Asthmatics and Corticosteroid-Treated Asthmatic Patients. J Proteome Res (2014) 13:3919–29. doi: 10.1021/pr5002059
153. Spahn JD. Asthma Biomarkers in Sputum. Immunol Allergy Clin North Am (2007) 27:607–22. doi: 10.1016/j.iac.2007.09.008
154. Jiang T, Dai L, Li P, Zhao J, Wang X, An L, et al. Lipid Metabolism and Identification of Biomarkers in Asthma by Lipidomic Analysis. Biochim Biophys Acta Mol Cell Biol Lipids (2021) 1866:158853. doi: 10.1016/j.bbalip.2020.158853
155. Crestani E, Harb H, Charbonnier LM, Leirer J, Motsinger-Reif A, Rachid R, et al. Untargeted Metabolomic Profiling Identifies Disease-Specific Signatures in Food Allergy and Asthma. J Allergy Clin Immunol (2020) 145:897–906. doi: 10.1016/j.jaci.2019.10.014
156. Ha T-W, Jung H-U, Kim DJ, Baek EJ, Lee WJ, Lim JE, et al. Association Between Environmental Factors and Asthma Using Mendelian Randomization: Increased Effect of Body Mass Index on Adult-Onset Moderate-To-Severe Asthma Subtypes. Front Genet (2021) 12. doi: 10.3389/fgene.2021.639905
157. Schjodt MS, Gurdeniz G, Chawes B. The Metabolomics of Childhood Atopic Diseases: A Comprehensive Pathway-Specific Review. Metabolites (2020) 10(10). doi: 10.3390/metabo10120511
158. Spertini F. Metabolomics and Allergy: Opening Pandora's Box. J Allergy Clin Immunol (2020) 145:782–4. doi: 10.1016/j.jaci.2020.01.012
159. Furusawa Y, Obata Y, Fukuda S, Endo TA, Nakato G, Takahashi D, et al. Commensal Microbe-Derived Butyrate Induces the Differentiation of Colonic Regulatory T Cells. Nature (2013) 504:446–50. doi: 10.1038/nature12721
160. Smith PM, Howitt MR, Panikov N, Michaud M, Gallini CA, Bohlooly YM, et al. The Microbial Metabolites, Short-Chain Fatty Acids, Regulate Colonic Treg Cell Homeostasis. Science (2013) 341:569–73. doi: 10.1126/science.1241165
161. Levan SR, Stamnes KA, Lin DL, Panzer AR, Fukui E, McCauley K, et al. Elevated Faecal 12,13-diHOME Concentration in Neonates at High Risk for Asthma is Produced by Gut Bacteria and Impedes Immune Tolerance. Nat Microbiol (2019) 4:1851–61. doi: 10.1038/s41564-019-0498-2
162. Zhu Z, Hasegawa K, Camargo CA Jr., Liang L. Investigating Asthma Heterogeneity Through Shared and Distinct Genetics: Insights From Genome-Wide Cross-Trait Analysis. J Allergy Clin Immunol (2021) 147:796–807. doi: 10.1016/j.jaci.2020.07.004
163. Zhu Z, Lee PH, Chaffin MD, Chung W, Loh PR, Lu Q, et al. A Genome-Wide Cross-Trait Analysis From UK Biobank Highlights the Shared Genetic Architecture of Asthma and Allergic Diseases. Nat Genet (2018) 50:857–64. doi: 10.1038/s41588-018-0121-0
164. Raita Y, Camargo CA Jr., Bochkov YA, Celedon JC, Gern JE, Mansbach JM, et al. Integrated-Omics Endotyping of Infants With Rhinovirus Bronchiolitis and Risk of Childhood Asthma. J Allergy Clin Immunol (2021) 147:2108–17. doi: 10.1016/j.jaci.2020.11.002
165. Gautam Y, Johansson E, Mersha TB. Multi-Omics Profiling Approach to Asthma: An Evolving Paradigm. J Pers Med (2022) 12(1). doi: 10.3390/jpm12010066
Keywords: asthma, GWAS - genome-wide association study, inheritability, epigenetics, microbiome and dysbiosis, maternal inheritance, atopic disease, multiomics approach
Citation: Augustine T, Al-Aghbar MA, Al-Kowari M, Espino-Guarch M and van Panhuys N (2022) Asthma and the Missing Heritability Problem: Necessity for Multiomics Approaches in Determining Accurate Risk Profiles. Front. Immunol. 13:822324. doi: 10.3389/fimmu.2022.822324
Received: 25 November 2021; Accepted: 25 April 2022;
Published: 25 May 2022.
Edited by:
Tobias R. Kollmann, University of Western Australia, AustraliaReviewed by:
Scott James Tebbutt, University of British Columbia, CanadaHanna Danielewicz, Wroclaw Medical University, Poland
Copyright © 2022 Augustine, Al-Aghbar, Al-Kowari, Espino-Guarch and van Panhuys. This is an open-access article distributed under the terms of the Creative Commons Attribution License (CC BY). The use, distribution or reproduction in other forums is permitted, provided the original author(s) and the copyright owner(s) are credited and that the original publication in this journal is cited, in accordance with accepted academic practice. No use, distribution or reproduction is permitted which does not comply with these terms.
*Correspondence: Nicholas van Panhuys, nvanpanhuys@sidra.org