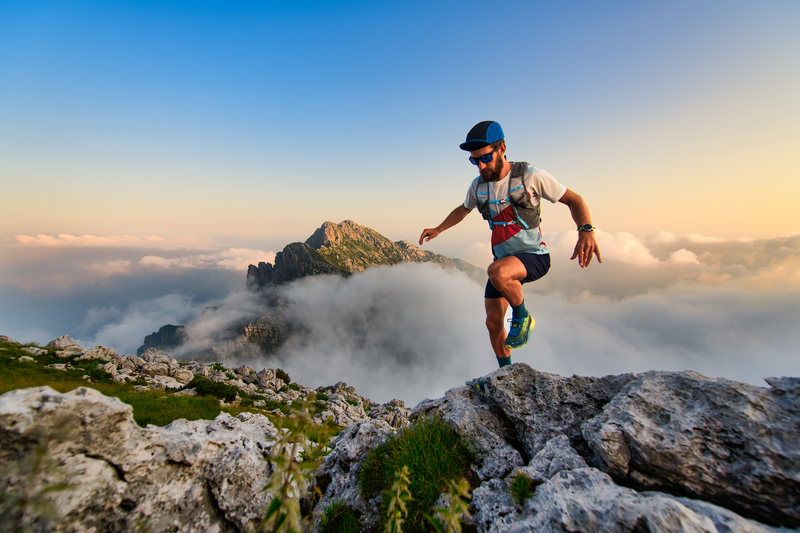
95% of researchers rate our articles as excellent or good
Learn more about the work of our research integrity team to safeguard the quality of each article we publish.
Find out more
ORIGINAL RESEARCH article
Front. Immunol. , 10 May 2022
Sec. Autoimmune and Autoinflammatory Disorders
Volume 13 - 2022 | https://doi.org/10.3389/fimmu.2022.814303
This study aimed to identify susceptibility genes and pathways associated with ankylosing spondylitis (AS) by integrating whole transcriptome-wide association study (TWAS) analysis and mRNA expression profiling data. AS genome-wide association study (GWAS) summary data from the large GWAS database were used. This included data of 1265 AS patients and 452264 controls. A TWAS of AS was conducted using these data. The analysis software used was FUSION, and Epstein-Barr virus–transformed lymphocytes, transformed fibroblasts, peripheral blood, and whole blood were used as gene expression references. Gene ontology (GO) and Kyoto Encyclopedia of Genes and Genomes (KEGG) enrichment analyses were performed for the important genes identified via TWAS. Protein-protein interaction (PPI) network analysis based on the STRING database was also performed to detect genes shared by TWAS and mRNA expression profiles in AS. TWAS identified 920 genes (P <0.05) and analyzed mRNA expression profiles to obtain 1183 differential genes. Following comparison of the TWAS results and mRNA expression characteristics, we obtained 70 overlapping genes and performed GO and KEGG enrichment analyses of these genes to obtain 16 pathways. Via PPI network analysis, we obtained the protein interaction network and performed MCODE analysis to acquire the HUB genes. Similarly, we performed GO and KEGG analyses on the genes identified by TWAS, obtained 98 pathways after screening, and analyzed protein interactions via the PPI network. Through the integration of TWAS and mRNA expression analysis, genes related to AS and GO and KEGG terms were determined, providing new evidence and revealing the pathogenesis of AS. Our AS TWAS work identified novel genes associated with AS, as well as suggested potential tissues and pathways of action for these TWAS AS genes, providing a new direction for research into the pathogenesis of AS.
Ankylosing spondylitis (AS) is a chronic inflammatory disease that primarily affects the axial skeleton (1) and is a common rheumatic disease characterized by chronic lower back pain and spinal ankylosing (2). AS usually causes chronic back pain and morning stiffness in patients, resulting in restricted spinal cord mobility (3), which adversely affects the quality of life of patients. Studies have shown that AS is a prevalent and potentially incapacitating chronic inflammatory arthritis, affecting 0.5% to 1.5% of the western population (4).
With the development of genetics, increasingly more researchers are focusing on the genetic basis of AS. Genome-wide association studies (GWAS) are a powerful method to identify disease and trait-related variants of AS (5). Thus far, GWAS have determined that 113 single nucleotide polymorphisms (SNPs) affect the risk of AS, and ongoing GWAS research may identify more than 100 new risk sites (6). However, the reliability of GWAS in assessing the risk of complex diseases is limited as most SNPs recognized by GWAS are located in the non-coding region of the disease genome (7). Therefore, the resulting SNPs indisputably lack the explanatory power for the disease. To alleviate problems associated with statistical power and interpretability, a recent trend in large-scale association studies is the transcriptome-wide association study (TWAS) (8). Some studies have proposed that the whole TWAS that integrates GWAS and expression quantitative trait loci (eQTL) data are relatively effective at unraveling gene-trait associations (9). Recently, increasingly more researchers have used TWAS to identify susceptibility sites associated with complex diseases. For example, the study uses eQTL data for TWAS to improve the current largest-scale schizophrenia GWAS summary statistics (10). Recently, researchers performed a large TWAS of prostate cancer (PrCa), in which multiple associations between genetically predicted gene expression and PrCa risk were identified (11). A study using self-brain, blood, and adipose tissue as a gene expression reference for schizophrenia was performed, and following co-localization with mRNA expression profiles, 157 schizophrenia-associated genes were identified, 35 of which were novel (12).
In this study, for the first time, a large-scale AS GWAS data set was used to identify genetic sites that may be associated with AS by TWAS, and perform enrichment analysis and PPI network analysis on the identified genes to identify AS-related genes and biological pathways. Our research has discovered new genetic loci related to AS and provided more research directions for AS research.
The GWAS summary data for AS were derived from UK Biobank samples (UK Biobank fields: 20002_1313). Briefly, the British Biobank gene data set contains the genome-wide genotype data of 452264 participants, including 1265 AS patients. DNA was extracted from stored blood samples of individuals, and genotyped using the UK Biobank Axiom array (13). There are 62,394 genotype variants that have passed quality control through the Applied Biosystems British Biobank Axiom Array. This data set contains 9,113,133 calculation variants after filtering (13). The IMPUTE4 program is used to perform responsibilities (http://jmarchini.org/software/). Detailed information regarding subjects, genotypes, blame, and quality control can be found in published studies (14). We retrieved the results of previous GWAS studies on ankylosing spondylitis presented in Table 1.
In this study, FUSION software was used to analyze the GWAS aggregated data of TWAS to AS (http://gusevlab.org/projects/fusion/). FUSION is a set of tools used to evaluate the association between gene expression and target disease/phenotype based on pre-calculated gene expression weights and GWAS summary data (19). Briefly, this study used the predictive model in FUSION to calculate gene expression. Thereafter, the calculated tissue-related expression weight was combined with summary-level GWAS results, and transcriptional regulation was used as an intermediary between genetic variation and phenotype, and the association between a single genetic variation and phenotype was converted into genes/transcripts and phenotypes ‘S association. In previous studies, Epstein-Barr virus–transformed lymphocytes (EL), transformed fibroblasts (TF), peripheral blood (NBL), and whole blood (YBL) were used for rheumatoid arthritis (RA) TWAS analysis (20). In the pathogenesis of AS, various types of cells and tissues are attacked, including bones, cartilage, muscles, ligaments, synovium, fibroblasts, immune cells, etc. Therefore, for AS, which is also an autoimmune disease, the use of four gene expression weights were selected as a reference in this study. The gene expression weight panel for pre-calculation was downloaded from the FUSION website (http://gusevlab.org/projects/fusion/).
We downloaded the mRNA expression profile of AS from the Gene Expression Gene Expression Omnibus DataSets (https://www.ncbi.nlm.nih.gov/geo/accession number: GSE11886). The platform of the GSE11886 chip is GPL570, Affymetrix Human Genome U133 Plus 2.0 Array expression beads, and tested samples are from eight AS patients (median course of disease 13 years [range <1–43 years]) and nine healthy control subjects 7 d old peripheral blood. We selected eight peripheral blood samples from AS patients and nine normal peripheral blood samples from the control group for differential gene analysis. The R language limma package was used for differential gene expression analysis. We screened genes with a folding change (FC) >2 or <0.5 (|log2FC |> 1) and an adjusted P value <0.05 as differentially expressed genes.
For genes identified using TWAS and mRNA expression profiles, Kyoto Encyclopedia of Genes and Genomes (KEGG) and gene ontology (GO) were used to identify and confirm related biological processes. The enrichment of KEGG and GO was done using R package’s “org.Hs.eg.db,” “clusterProfiler”.
PPI network analysis was performed in this study using the STRING v11.5 database (STRING, https://string-db/org) to complete, the interactive network is generated based on the experiment with the required 0.15 confidence score and “active interactive source” (21).
In this study, the TWAS identified 920 genes, which included 448, 209, 159, and 310 genes of EL, TF, NBL, and YBL, respectively. All genes are shown in Figure 1. To find the specific and the most representative genes of each tissue, we performed overlap analysis on the genes identified by the TWAS in different tissues/cells. The overlap result is shown in the Venn diagram (Figure 2). For example, in TF, 332 genes were identified by the TWAS that are related to AS; there are 44 important genes in EL and TF; 14 in EL, TF, and YBL, and seven identified in the four joint identifications. The seven TWAS significant AS susceptibility genes jointly identified in the four tissues/cells are LIPT1, LARS, MICA, MICB, BTN3A2, HLA-A, and ACTA2. The detailed information of these seven overlapping genes are listed in Table 2, including the tissue/cell name, gene name (Gene), CHR and the most important GWAS Snp rsid (BEST.GWAS.ID), TWAS Z score (Zc), and TWAS P value (P).
Figure 1 Venn diagram of genes obtained from TWAS identification in four: Gray, YBL; orange, NBL; blue, EL; pink, TF.
Figure 2 Manhattan plot of AS-associated genes identified by TWAS (colored dots). Each dot represents a gene, the x-axis is the physical location (chromosomal localization) and the y-axis is the -log10 (p-value) of the gene’s association with AS. Significant genes in different tissues/cells are highlighted in different colors (A, TF; B, EL; C, YBL; D, NBL).
We further compared genes recognized by the TWAS with the differential genes recognized by AS mRNA expression profile analysis. We detected 70 common genes shared by TWAS and mRNA expression analysis, such as RETSAT(P value=0.0243), EOMES(P value=0.00368), CXCR6(P value=0.0284), PPIC(P value=0.00496), and TCF19(P value=0.0481). The 10 genes commonly shared by the TWAS, and mRNA expression analysis are shown in Table 3.
We performed GO and KEGG pathway enrichment analysis on 920 genes identified using TWAS. Seventy-two GO terms, with p.adjust <0.05 and 26 KEGG terms with p.adjust <0.05, such as antigen processing and presentation were detected in the GO enrichment pathway. The results for endogenous peptide antigen (p.adjust = 3.31342E-05), interferon-γ-mediated signaling pathway (p.adjust = 0.000207229), antigen processing and presentation through MHC class Ib (p.adjust = 0.000363369), allograft rejection (p.adjust = 1.28509E-13), RA in the KEGG pathway (p.adjust = 5.07762E-05)), systemic lupus erythematosus (p.adjust = 0.010482717), etc. are shown in Figure 3. Similarly, we also performed GO and KEGG pathway enrichment analysis on common genes identified by the TWAS in the mRNA expression profile. Thirty-two GO terms with p.adjust <0.05 and 5 KEGG terms with p.adjust <0.05 were detected. The results for T cell–mediated cytotoxicity (p.adjust = 0.015712930095128), leukocyte-mediated positive immune regulation (p.adjust = 0.019575169), etc. are shown in Figure 4. Further comparison of TWAS and common gene enrichment analysis results detected 16 common pathways with p.adjust <0.05, such as antigen processing and presentation of endogenous peptide antigens, MHC class I antigen processing and presentation of endogenous peptide antigens, etc. (Figure 5).
Figure 3 Venn diagram of TWAS versus common genes identified by mRNA expression profiling. Gray, TWAS; Orange, DEGs.
Figure 4 Network diagram of GO term analysis for TWAS-identified genes, where each circular point in the network represents a term whose size is proportional to the number of input genes for that term. (A) KEGG; (B) BP; (C) CC; (D) MF.
Figure 5 GO term analysis network diagram for TWAS-identified genes, where each circular dot in the network represents a term whose size is proportional to the number of input genes for that term. (A) Common pathway; (B) Common genes enrichment pathways.
The PPI network was constructed based on 920 genes identified by the TWAS. We further analyzed the constructed PPI network using MCODE. The important modules from the PPI network formed six MCODE groups with a class of genes (Figure 6).
Recently, TWAS has been proposed as a principled approach to integrate eQTL analyses with GWAS to identify genes whose genetically regulated expression is associated to disease risk (22). The TWAS has garnered substantial interest and become increasingly popular within the human genetics research community (23). Effect sizes of cis-eQTLs were utilized to impute gene expression from genotyped samples in the TWAS (24). It captures heterogeneous signals better than individual SNPs or cis-eQTLs and focuses prediction on the genetic component of expression that avoids confounding factors from environmental differences caused by the trait that may influence expression (20). In this study, the TWAS is the first in the field of AS susceptibility gene research.
Ankylosing spondylitis (AS) is a chronic inflammatory disease of unknown etiology and, unlike other systemic autoimmune diseases, the innate immune system has a dominant role in AS (25). It is an important chronic inflammatory disease with an incidence between 0.5% and 1.0% (26). In AS, inflammation most likely originates at the tendon–bone interface (an example of enthesitis), leading to erosion and bone proliferation via mechanisms that are poorly understood (27). AS is a common chronic immune mediator disease characterized by inflammation of the axial bones, and there are extra-articular manifestations such as the co-occurrence of psoriasis and inflammatory bowel disease, with uveitis being the most common (27). In the pathogenesis of AS, various types of cells and tissues are attacked, including bones, cartilage, muscles, ligaments, synovium, fibroblasts, immune cells, etc. The greatest contributor to AS is HLA-B27 (Human leukocyte antigen); more than 90% of AS patients are HLA-B27 positive, thus the HLA-B27 factor in the blood is primarily used to diagnose AS (28). Therefore, in this study, we used four tissues/cells as a reference for gene expression, and conducted a TWAS on AS.
Among the genes identified by the TWAS, together with mRNA expression profiles, we found multiple susceptibility genes for AS, which can be studied further. Eomesodermin (EOMES) are T-box transcription factors that drive differentiation and function of cytotoxic lymphocytes, such as NK cells (29). It has been shown that although several transcription factors are important for NK cell maturation and function, T-box-related T-BET (encoded by TBX21) and EOMES transcription factors are essential for terminal NK cell maturation and function as mice lacking Tbx21 and EOMES lack mature NK cells (30). The chemokine receptor, CXCR6, is an important receptor for CXCL16 (31). It has been shown that Fc fusion proteins reduce the RANCL/OPG ratio by inhibiting the CXCL16/CXCR6 pathway, thereby suppressing inflammation and bone destruction in AS (32). Heme 4D (Sema4D) is expressed on T cells and osteoblasts and regulates T cell proliferation and skeletal remodeling, and findings suggest that Sema4D, a potent activator of T cells in the immune response, contributes to inflammation in AS by inducing imbalances in Th17 and Treg cell populations in an AhR-dependent manner, indicating that it is a key player in the pathogenesis of AS (33).
In this study, we used four tissues/cells as a reference for gene expression and conducted a TWAS on AS. We used the TWAS to identify 920 statistically significant genes in TF, EL, YBL, and NBL. Among them, seven genes LIPT1, LARS, MICA, MICB, BTN3A2, HLA-A, and ACTA2 were jointly identified using the four tissues/cells. Seventy genes were jointly identified using the TWAS and mRNA expression profiles, among which MICA and HLA-A were also identified using the four tissues/cells.
HLA-B27 and AS are a paradigmatic example of association between the HLA and an immuno-mediated disease (34). HLA molecules are highly polymorphic, HLA -A, HLA -B, and HLA -DRB1 belong to the same family of molecules and are considered closely related to allogeneic rejection and autoimmune reactions (35). MICA interacts with NKG2D on immune cells to regulate the host immune response, which is highly related to the autoimmune response (36). MICA is a member of the non-classical MHC class I family and is highly polymorphic. The promoter SNP rs4349859 of MICA, previously used as an HLA-B27 tag SNP, has the strongest association with European AS (37). This evidence supports that the application of the TWAS in AS is relatively effective. It can be seen that the genes identified in this study can elucidate the mechanism of AS.
Studies using one or more anti-rheumatic drugs (methometamine, hydroxychloroquine, or sulfonamide) on 46 patients with suspected RA, followed by a genome-wide methylation analysis of baseline T lymphocyte DNA found that the BTN3A2 gene is Two CpG sites are closely related to a treatment response (38). AS and RA are both autoimmune diseases; the CpG locus of the BTN3A2 gene may be related to AS, which provides a new research direction for the pathogenesis of AS. Some studies have shown that three human mitochondrial diseases that directly affect lipoic acid metabolism result from heterozygous missense and nonsense mutations in LIAS, LIPT1, and LIPT2 genes (39). It has been shown that the oxidative serum environment of AS promotes mesenchymal stem cell aging by inducing mitochondrial dysfunction and excessive ROS production (40), which suggests that the pathogenesis of AS is closely related to mitochondrial dysfunction, further suggesting that LIPT1 may be related to the pathogenesis of AS. Moreover, aberrant regulation of autophagy has been implicated in an increasing number of autoimmune disorders such as systemic lupus erythematous, RA, AS, multiple sclerosis, Crohn’s disease, and vitiligo (41). It has been shown that autophagy in fibroblasts correlates with mRNA levels of ACTA2, TGF-1, FGF2, and PDGFRA (42). This suggests that ACTA2 may cause AS via induction of fibroblast autophagy.
GO enrichment analysis detected several GO terms such as T cell–mediated cytotoxicity, positive regulation of T cell–mediated cytotoxicity, lymphocyte mediated immune regulation, cell killing regulation, Th17 cell differentiation, antigen processing and presentation via MHC class I for endogenous peptide antigens, antigen processing and presentation via MHC class Ib, autoimmune thyroid disease, etc. Some researchers have detected cd4t cells with cytotoxic potential in RA and AS and are able to mediate graft rejection through a perforin-dependent mechanism (43). It has also been suggested that local HLA-dependent activation of peptide-specific cytotoxic CD8+ T cells in chondrocytes may play a role in the inflammatory process of AS (44). It has been suggested that genes associated with AS (HLA-B27, ERAP-1, and KIR) may affect NK cell function. There are some functional changes in a subset of NK cells that suggest an NK cell response similar to the Th1 pathway, and some changes in NK cell phenotype/function can be used to predict treatment response in patients with AS (45). IL-17 is a cytokine implicated in the pathophysiology of AS, and researchers have used anti-IL-17 monoclonal antibodies for the treatment of AS and found significant efficacy (46). AS is closely associated with the MHC class I allele HLA-B27, which strongly suggests that our use of the TWAS to identify the resulting gene is highly effective (47). These identified biological pathways have been shown to have some association with AS, providing us with additional areas of research interest.
In this study, we used TWAS and mRNA expression profiling together to identify susceptibility genes for AS. The TWAS can detect genes associated with AS at the DNA level, mRNA expression profiling can explain the pathogenesis of AS at the protein expression level, and the combination of the two can more accurately identify susceptibility genes for AS. Our research has identified new genes and biological pathways associated with AS, providing additional research directions for the study of AS.
However, the study has some limitations. First, the GWAS pooled data are from the UK Biobank, and study subjects are predominantly from European populations. Therefore, the results of this study should be used with caution when studying AS in other populations. Second, some susceptibility genes of AS obtained from the analysis have not been verified via molecular biology experiments, which should be performed in future studies.
The datasets presented in this study can be found in online repositories. The names of the repository/repositories and accession number(s) can be found in the article/Supplementary Material.
RF, ML and PX designed the study. All authors contributed to the article and approved the submitted version.
This work was supported by the National Natural Scientific Foundation of China (82072432, 81772410).
The authors declare that the research was conducted in the absence of any commercial or financial relationships that could be construed as a potential conflict of interest.
All claims expressed in this article are solely those of the authors and do not necessarily represent those of their affiliated organizations, or those of the publisher, the editors and the reviewers. Any product that may be evaluated in this article, or claim that may be made by its manufacturer, is not guaranteed or endorsed by the publisher.
We are indebted to all the individuals who participated in, or helped with, our research.
The Supplementary Material for this article can be found online at: https://www.frontiersin.org/articles/10.3389/fimmu.2022.814303/full#supplementary-material
Supplementary Table 1 | TWAS result of AS.
Supplementary Table 2 | AS TWAS detects candidate genes GO and KEGG enrichment analysis results.
Supplementary Table 3 | The intersection result of the pathway enrichment analysis result of the common gene and the AS TWAS enrichment pathway.
Supplementary Table 4 | Common genes.
AS, Ankylosing spondylitis; RA, Rheumatoid arthritis; EL, EBV-transformed lymphocytes; TF, transformed fibroblasts; NBL, peripheral blood; YBL, whole blood; GWAS, Genome-wide association study; TWAS, Transcriptome-wide association studies; eQTL, expression quantitative trait Loci; DEGs, Differentially expressed genes; GTEx, Genotype-Tissue Expression; PPI, protein-protein interaction; GO, Gene ontology; KEGG, Kyoto Encyclopedia of Genes and Genomes; HLA, Human leukocyte antigen; MHC, Major histocompatibility complex; MICA, Major Histocompatibility Complex Type I Chain Related Gene A.
1. Arends S, Spoorenberg A, Bruyn GA, Houtman PM, Leijsma MK, Kallenberg CG, et al. The Relation Between Bone Mineral Density, Bone Turnover Markers, and Vitamin D Status in Ankylosing Spondylitis Patients With Active Disease: A Cross-Sectional Analysis. Osteoporosis Int J Established As Result Cooperation Between Eur Foundation Osteoporosis Natl Osteoporosis Foundation USA (2011) 22(5):1431–9. doi: 10.1007/s00198-010-1338-7
2. Qian H, Chen R, Wang B, Yuan X, Chen S, Liu Y, et al. Associations of Platelet Count With Inflammation and Response to Anti-TNF-α Therapy in Patients With Ankylosing Spondylitis. Front Pharmacol (2020) 11:559593–3. doi: 10.3389/fphar.2020.559593
3. Sieper J, Poddubnyy D. Axial Spondyloarthritis. Lancet (London England) (2017) 390(10089):73–84. doi: 10.1016/S0140-6736(16)31591-4
4. de Winter JJ, van Mens LJ, van der Heijde D, Landewé R, Baeten DL. Prevalence of Peripheral and Extra-Articular Disease in Ankylosing Spondylitis Versus Non-Radiographic Axial Spondyloarthritis: A Meta-Analysis. Arthritis Res Ther (2016) 18(1):196–6. doi: 10.1186/s13075-016-1093-z
5. Canver MC, Lessard S, Pinello L, Wu Y, Ilboudo Y, Stern EN, et al. Variant-Aware Saturating Mutagenesis Using Multiple Cas9 Nucleases Identifies Regulatory Elements at Trait-Associated Loci. Nat Genet (2017) 49(4):625–34. doi: 10.1038/ng.3793
6. Nancy Z, Yan L, Hui S, Paul B, Liye C. From the Genetics of Ankylosing Spondylitis to New Biology and Drug Target Discovery. Front Immunol (2021) 12:624632–2. doi: 10.3389/fimmu.2021.624632
7. Xu J, Zeng Y, Si H, Liu Y, Li M, Zeng J, et al. Integrating Transcriptome-Wide Association Study and mRNA Expression Profile Identified Candidate Genes Related to Hand Osteoarthritis. Arthritis Res Ther (2021) 23(1):81–1. doi: 10.1186/s13075-021-02458-2
8. Bhattacharya A, García-Closas M, Olshan AF, Perou CM, Troester MA, Love MI. A Framework for Transcriptome-Wide Association Studies in Breast Cancer in Diverse Study Populations. Genome Biol (2020) 21(1):42–2. doi: 10.1186/s13059-020-1942-6
9. Porcu E, Rüeger S, Lepik K, e QC, Consortium B, Santoni FA, et al. Mendelian Randomization Integrating GWAS and eQTL Data Reveals Genetic Determinants of Complex and Clinical Traits. Nat Commun (2019) 10(1):3300–0. doi: 10.1038/s41467-019-10936-0
10. Collado-Torres L, Burke EE, Peterson A, Shin J, Straub RE, Rajpurohit A, et al. Regional Heterogeneity in Gene Expression, Regulation, and Coherence in the Frontal Cortex and Hippocampus Across Development and Schizophrenia. Neuron (2019) 103(2):203–216.e208. doi: 10.1016/j.neuron.2019.05.013
11. Wu L, Yang Y, Guo X, Shu X-O, Cai Q, Shu X, et al. An Integrative Multi-Omics Analysis to Identify Candidate DNA Methylation Biomarkers Related to Prostate Cancer Risk. Nat Commun (2020) 11(1):3905–5. doi: 10.1038/s41467-020-17673-9
12. Gusev A, Mancuso N, Won H, Kousi M, Finucane HK, Reshef Y, et al. Transcriptome-Wide Association Study of Schizophrenia and Chromatin Activity Yields Mechanistic Disease Insights. Nat Genet (2018) 50(4):538–48. doi: 10.1038/s41588-018-0092-1
13. Ma M, Li P, Liu L, Cheng S, Cheng B, Liang CJ, et al. Integrating Transcriptome-Wide Association Study and mRNA Expression Profiling Identifies Novel Genes Associated With Osteonecrosis of the Femoral Head. Front Genet (2021) 12:663080–0. doi: 10.3389/fgene.2021.663080
14. Canela-Xandri O, Rawlik K, Tenesa A. An Atlas of Genetic Associations in UK Biobank. Nat Genet (2018) 50(11):1593–9. doi: 10.1038/s41588-018-0248-z
15. Lin Z, Bei JX, Shen M, Li Q, Liao Z, Zhang Y, et al. A Genome-Wide Association Study in Han Chinese Identifies New Susceptibility Loci for Ankylosing Spondylitis. Nat Genet (2011) 44(1):73–7. doi: 10.1038/ng.1005
16. Australo-Anglo-American Spondyloarthritis Consortium (TASC), Reveille JD, Sims AM, Danoy P, Evans DM, Leo P, et al. Genome-wide Association Study of Ankylosing Spondylitis Identifies Non-MHC Susceptibility Loci. Nat Genet (2010) 42(2):123–7. doi: 10.1038/ng.513
17. Evans DM, Spencer CC, Pointon JJ, Su Z, Harvey D, Kochan G, et al. Interaction Between ERAP1 and HLA-B27 in Ankylosing Spondylitis Implicates Peptide Handling in the Mechanism for HLA-B27 in Disease Susceptibility. Nat Genet (2011) 43(8):761–7. doi: 10.1038/ng.873
18. Li Z, Akar S, Yarkan H, Lee SK, Çetin P, Can G, et al. Genome-Wide Association Study in Turkish and Iranian Populations Identify Rare Familial Mediterranean Fever Gene (MEFV) Polymorphisms Associated With Ankylosing Spondylitis. PLoS Genet (2019) 15(4):e1008038. doi: 10.1371/journal.pgen.1008038
19. Gusev A, Ko A, Shi H, Bhatia G, Chung W, Penninx BWJH, et al. Integrative Approaches for Large-Scale Transcriptome-Wide Association Studies. Nat Genet (2016) 48(3):245–52. doi: 10.1038/ng.3506
20. Wu C, Tan S, Liu L, Cheng S, Li P, Li W, et al. Transcriptome-Wide Association Study Identifies Susceptibility Genes for Rheumatoid Arthritis. Arthritis Res Ther (2021) 23(1):38–8. doi: 10.1186/s13075-021-02419-9
21. Jensen LJ, Kuhn M, Stark M, Chaffron S, Creevey C, Muller J, et al. STRING 8–a Global View on Proteins and Their Functional Interactions in 630 Organisms. Nucleic Acids Res (2009) 37:D412–416. doi: 10.1093/nar/gkn760
22. Gusev A, Lawrenson K, Lin X, Lyra PC Jr., Kar S, Vavra KC, et al. A Transcriptome-Wide Association Study of High-Grade Serous Epithelial Ovarian Cancer Identifies New Susceptibility Genes and Splice Variants. Nat Genet (2019) 51(5):815–23. doi: 10.1038/s41588-019-0395-x
23. Wu C, Pan W. A Powerful Fine-Mapping Method for Transcriptome-Wide Association Studies. Hum Genet (2020) 139(2):199–213. doi: 10.1007/s00439-019-02098-2
24. Sun B, Chen L. Quantile Regression for Challenging Cases of eQTL Mapping. Brief Bioinform (2020) 21(5):1756–65. doi: 10.1093/bib/bbz097
25. Li L, Fu J, Xu C, Guan H, Ni M, Chai W, et al. Factors Associated With Blood Loss in Ankylosing Spondylitis Patients With Hip Involvement Undergoing Primary Total Hip Arthroplasty: A Cross-Sectional Retrospective Study of 243 Patients. J Orthop Surg Res (2020) 15(1):541–1. doi: 10.1186/s13018-020-02064-z
26. Lee S-H, Lee Y-A, Woo D-H, Song R, Park E-K, Ryu M-H, et al. Association of the Programmed Cell Death 1 (PDCD1) Gene Polymorphism With Ankylosing Spondylitis in the Korean Population. Arthritis Res Ther (2006) 8(6):R163–3. doi: 10.1186/ar2071
27. Simone D, Al Mossawi MH, Bowness P. Progress in Our Understanding of the Pathogenesis of Ankylosing Spondylitis. Rheumatol (Oxford) (2018) 57(suppl_6):vi4–9. doi: 10.1093/rheumatology/key001
28. Zhai Y, Lin P, Feng Z, Lu H, Han Q, Chen J, et al. TNFAIP3-DEPTOR Complex Regulates Inflammasome Secretion Through Autophagy in Ankylosing Spondylitis Monocytes. Autophagy (2018) 14(9):1629–43. doi: 10.1080/15548627.2018.1458804
29. Zhang J, Marotel M, Fauteux-Daniel S, Mathieu AL, Viel S, Marçais A, et al. T-Bet and Eomes Govern Differentiation and Function of Mouse and Human NK Cells and ILC1. Eur J Immunol (2018) 48(5):738–50. doi: 10.1002/eji.201747299
30. Scoville SD, Mundy-Bosse BL, Zhang MH, Chen L, Zhang X, Keller KA, et al. A Progenitor Cell Expressing Transcription Factor Rorγt Generates All Human Innate Lymphoid Cell Subsets. Immunity (2016) 44(5):1140–50. doi: 10.1016/j.immuni.2016.04.007
31. Guo L, Cui Z-M, Zhang J, Huang Y. Chemokine Axes CXCL12/CXCR4 and CXCL16/CXCR6 Correlate With Lymph Node Metastasis in Epithelial Ovarian Carcinoma. Chin J Cancer (2011) 30(5):336–43. doi: 10.5732/cjc.010.10490
32. Zhang P, Zhou S, Chen Z, Tian Y, Wang Q, Li H, et al. TNF Receptor: Fc Fusion Protein Downregulates RANKL/OPG Ratio by Inhibiting CXCL16/CXCR6 in Active Ankylosing Spondylitis. Curr Pharm Biotechnol (2021) 22(2):305–16. doi: 10.2174/1389201021666200302104418
33. Xie J, Wang Z, Wang W. Semaphorin 4d Induces an Imbalance of Th17/Treg Cells by Activating the Aryl Hydrocarbon Receptor in Ankylosing Spondylitis. Front Immunol (2020) 11:2151. doi: 10.3389/fimmu.2020.02151
34. Paladini F, Fiorillo MT, Tedeschi V, Cauli A, Mathieu A, Sorrentino R. Ankylosing Spondylitis: A Trade Off of HLA-B27, ERAP, and Pathogen Interconnections? Focus on Sardinia. Front Immunol (2019) 10:35–5. doi: 10.3389/fimmu.2019.00035
35. Ma H, Wang M, Zhou Y, Yang J-J, Wang L-Y, Yang R-H, et al. Noncoding RNA 886 Alleviates Tumor Cellular Immunological Rejection in Host C57BL/C Mice. Cancer Med (2020) 9(14):5258–71. doi: 10.1002/cam4.3148
36. Wang C-M, Tan K-P, Jan Wu Y-J, Lin J-C, Zheng J-W, Yu AL, et al. MICA*019 Allele and Soluble MICA as Biomarkers for Ankylosing Spondylitis in Taiwanese. J Pers Med (2021) 11(6):564. doi: 10.3390/jpm11060564
37. Evans DM, Spencer CCA, Pointon JJ, Su Z, Harvey D, Kochan G, et al. Interaction Between ERAP1 and HLA-B27 in Ankylosing Spondylitis Implicates Peptide Handling in the Mechanism for HLA-B27 in Disease Susceptibility. Nat Genet (2011) 43(8):761–7. doi: 10.1038/ng.873
38. Glossop JR, Nixon NB, Emes RD, Sim J, Packham JC, Mattey DL, et al. DNA Methylation at Diagnosis Is Associated With Response to Disease-Modifying Drugs in Early Rheumatoid Arthritis. Epigenomics (2017) 9(4):419–28. doi: 10.2217/epi-2016-0042
39. Cronan JE. Progress in the Enzymology of the Mitochondrial Diseases of Lipoic Acid Requiring Enzymes. Front Genet (2020) 11:510–0. doi: 10.3389/fgene.2020.00510
40. Ye G, Xie Z, Zeng H, Wang P, Li J, Zheng G, et al. Oxidative Stress-Mediated Mitochondrial Dysfunction Facilitates Mesenchymal Stem Cell Senescence in Ankylosing Spondylitis. Cell Death Dis (2020) 11(9):775–5. doi: 10.1038/s41419-020-02993-x
41. Alessandri C, Ciccia F, Priori R, Astorri E, Guggino G, Alessandro R, et al. CD4 T Lymphocyte Autophagy Is Upregulated in the Salivary Glands of Primary Sjögren's Syndrome Patients and Correlates With Focus Score and Disease Activity. Arthritis Res Ther (2017) 19(1):178–8. doi: 10.1186/s13075-017-1385-y
42. Inoue T, Hayashi Y, Tsujii Y, Yoshii S, Sakatani A, Kimura K, et al. Suppression of Autophagy Promotes Fibroblast Activation in P53-Deficient Colorectal Cancer Cells. Sci Rep (2021) 11(1):19524–4. doi: 10.1038/s41598-021-98865-1
43. Hua L, Yao S, Pham D, Jiang L, Wright J, Sawant D, et al. Cytokine-Dependent Induction of CD4+ T Cells With Cytotoxic Potential During Influenza Virus Infection. J Virol (2013) 87(21):11884–93. doi: 10.1128/JVI.01461-13
44. Kuhne M, Erben U, Schulze-Tanzil G, Köhler D, Wu P, Richter FJ, et al. HLA-B27-Restricted Antigen Presentation by Human Chondrocytes to CD8+ T Cells: Potential Contribution to Local Immunopathologic Processes in Ankylosing Spondylitis. Arthritis Rheum (2009) 60(6):1635–46. doi: 10.1002/art.24549
45. Kucuksezer UC, Akas Cetin E, Esen F, Tahrali I, Akdeniz N, Gelmez MY, et al. The Role of Natural Killer Cells in Autoimmune Diseases. Front Immunol (2021) 12:622306–6. doi: 10.3389/fimmu.2021.622306
46. Wendling D, Verhoeven F, Prati C. Anti-IL-17 Monoclonal Antibodies for the Treatment of Ankylosing Spondylitis. Expert Opin Biol Ther (2019) 19(1):55–64. doi: 10.1080/14712598.2019.1554053
Keywords: ankylosing spondylitis, genome-wide association study (GWAS), transcriptome-wide association study (TWAS), mRNA expression profile, susceptibility genes
Citation: Feng R, Lu M, Liu L, Xu K and Xu P (2022) Transcriptome-Wide Association Studies and Integration Analysis of mRNA Expression Profiles Identify Candidate Genes and Pathways Associated With Ankylosing Spondylitis. Front. Immunol. 13:814303. doi: 10.3389/fimmu.2022.814303
Received: 13 November 2021; Accepted: 29 March 2022;
Published: 10 May 2022.
Edited by:
Mi-La Cho, Catholic University of Korea, South KoreaReviewed by:
Rajeev Kumar Pandey, Johns Hopkins Medicine, United StatesCopyright © 2022 Feng, Lu, Liu, Xu and Xu. This is an open-access article distributed under the terms of the Creative Commons Attribution License (CC BY). The use, distribution or reproduction in other forums is permitted, provided the original author(s) and the copyright owner(s) are credited and that the original publication in this journal is cited, in accordance with accepted academic practice. No use, distribution or reproduction is permitted which does not comply with these terms.
*Correspondence: Peng Xu, c291c291MzY5QDE2My5jb20=
†These authors have contributed equally to this work
Disclaimer: All claims expressed in this article are solely those of the authors and do not necessarily represent those of their affiliated organizations, or those of the publisher, the editors and the reviewers. Any product that may be evaluated in this article or claim that may be made by its manufacturer is not guaranteed or endorsed by the publisher.
Research integrity at Frontiers
Learn more about the work of our research integrity team to safeguard the quality of each article we publish.