- 1Tsinghua-Peking Center for Life Sciences, Beijing, China
- 2Department of Basic Medical Sciences, School of Medicine, Tsinghua University, Beijing, China
- 3Department of Infectious Diseases, Peking Union Medical College Hospital, Peking Union Medical College and Chinese Academy of Medical Sciences, Beijing, China
Purpose: The incomplete immune reconstitution is a complex phenomenon among human immunodeficiency virus (HIV)-infected patients despite the fact that they have achieved persistent viral suppression under the combined antiretroviral therapy. This study aims to screen and verify the immunological characteristics and underlying mechanisms of immunological non-responders (INRs).
Methods: The RNA-seq and the differentially expressed genes (DEGs) analysis were used to explore potential characteristics among INRs. Gene Ontology (GO) enrichment, ingenuity pathway analysis (IPA) analysis, Gene set enrichment analysis (GSEA) analysis, and the weighted gene co-expression network analysis (WGCNA) were used to explore the potential mechanism. The transcriptional meta-analysis was used to analyze the external efficiency.
Results: The RNA-seq identified 316 DEGs among INRs. The interferon signaling pathway was enriched via GO and IPA analysis among DEGs. The combined GSEA and WGCNA analysis confirmed that the IFN response was more correlated with INR. Furthermore, IFI27 (IFN-α Inducible Protein 27, also known as ISG12) was chosen based on combined DEG analysis, WGCNA analysis, and the transcriptional meta-analysis conducted on other published datasets about INRs. The expression of IFI27 was significantly negatively correlated with the CD4+ T-cell counts of PLWH, and the predictive efficiency of IFI27 level in distinguishing PLWH with poor immune recovery was also with significant power (AUC = 0.848).
Conclusion: The enhanced expression of IFI27 and the IFN response pathway are among the important immunological characteristics of INRs and exhibited promising efficiency as biomarkers for CD4+ T-cell recovery.
Introduction
The hallmark of the acquired immunodeficiency syndrome (AIDS), caused by the human immunodeficiency virus (HIV) infection, is accompanied by continued HIV replication and profound decline of circulating CD4+ T lymphocyte cells (1). Nevertheless, approximately 10%–40% of people living with HIV/AIDS (PLWH) are unable to achieve optimal CD4+ T-cell recovery despite virological suppression under the combined antiretroviral therapy (cART) (2). The U.S. Department of Health and Human Services (DHHS) considered that PLWH with CD4+ T cells less than 350–500 cells/μL after 4–7 years of suppressive cART therapy as immunological non-responders (INRs) (2, 3). INRs have increased risks of mortality and AIDS-related and non-AIDS-related events than the immunological responders (IRs), making it essential to explore the relevant mechanisms and develop therapeutic interventions (2, 4).
The precise number of peripheral CD4+ T cells results from the balance of production, destruction, and traffic between peripheral blood and lymphatic tissue. Based on the current knowledge, there are two primary causes: the declined production caused by thymic dysfunction and the enhanced destruction caused by immune activation (IA), which lead to the development of incomplete immune reconstitution (2, 5, 6). The persistence of chronic immune activation (IA) is considered as one of the best valued immunological features of INRs (7–9). The association of residual systemic inflammation with clinical outcomes among HIV/AIDS patients has been noted and continues to be evaluated, especially among INRs (10, 11). Given the lack of efficient thymic-improving therapy, along with the correlation between abnormal immune activation and adverse clinical outcomes, several clinical attempts had been made to reduce the chronic IA for years, however, with limited satisfied results.
To identify a relatively effective clinical intervention in treating INRs, a more profound understanding of underlying mechanisms for the persistent CD4 deficiency and chronic IA remains imperative (12). Several factors have been proposed, including the residual HIV replication, co-infection, microbial translocation, intestinal flora, and the imbalance of Treg cells, Th17 cells, etc. to explain the chronic IA among INRs (10, 13–22). These factors are considered to induce the activation of inflammatory pathways, such as interferon (IFN), NF-κB, caspase, and TLR signaling pathways, which would boost the enhanced secretion of downstream inflammatory factors, and eventually lead to the dysfunction and destruction of CD4+ T cells (2, 12, 20, 23–29). Among these various causes and immunological pathways, searching for the most prominent characteristic genes and patterns of chronic IA would help to understand the precise mechanisms of INRs.
Currently, the whole transcriptomic profiling is an unbiased approach to studying the gene expression patterns among tissues or cells, and it has been involved as the critical method of disease mechanisms discovery (30). To better understand the characteristics of gene expression in INRs and IRs, we conducted RNA-seq on INR patients and analyzed with comparative methods, including the ingenuity pathway analysis (IPA), the gene set enrichment analysis (GSEA), and the weighted gene co-expression network analysis (WGCNA) (31–33). To improve the external efficiency, we additionally conducted the meta-transcriptional analysis on published gene expression datasets of INRs and validated the identified genes (34, 35). The combined approaches have been involved in the mechanism discovery of several diseases, such as chronic obstructive pulmonary disease (36), multiple sclerosis (37), Alzheimer’s disease (38), and cancer (39). Up to now, our study is the first to use comparative transcriptional analysis to explore the gene signature of peripheral blood related to INR. These comprehensive bioinformatic analyses aim to provide underlying mechanisms of chronic IA in INR and information for subsequent research and personalized treatment.
Materials and Methods
Participants and Selection Criteria
The patients were recruited from the HIV/AIDS clinics of the Department of Infectious Disease in Peking Union Medical College Hospital (PUMCH). These patients received cART and were followed regularly. INR was defined by those patients who (1) were HIV seropositive (2); were treated with cART for more than 4 years (3); achieved virologic control (VL < 20 copies/mL) for more than 3.5 years; and (4) continued low CD4+ T-cell counts (<350 cells/μL). The IR definitions were the same as INR, except they achieved optimal CD4+ T-cell recovery (>500 cells/μL) in at least two consecutive measurements. The demographic information, immunological characteristics, co-infection, and treatment regimens were collected and compared.
Lymphocyte Subsets and HIV-1 Viral Measurement
EDTA-anticoagulated fresh whole blood of patients was collected and incubated with monoclonal fluorescence antibodies, and the percentage of immune cells within lymphocytes was analyzed by a flow cytometer (LSR Fortessa, BD Biosciences), including T cells (CD45+CD3+), B cells (CD45+CD3-CD19+), NK cells (CD45+CD3-CD16+CD56+), CD4+ T cells (CD45+CD3+CD4+), CD8+ T cells (CD45+CD3+CD8+), naïve CD4+ T cells (CD45+CD3+CD4+CD62L+CD45RA+), and RTE (recent thymic emigrant) CD4+ T cells (CD45+CD3+CD4+CD45RA+CD31+). The absolute immune cell number was converted from multiplying immune cell percentage and the lymphocyte counts obtained from routine blood tests. The plasma of patients was isolated from whole blood through centrifugation to measure the plasma HIV-1 RNA (plasma viral load) to monitor the efficiency of cART. According to the manufacturer’s recommendations, the viral load measurement was determined by the COBAS AmpliPrep/COBAS TaqMan V2.0 RT-PCR (Roche). The lower limit of HIV-1 viral load is 20 copies/mL.
Transcriptome Analysis With RNA-seq
The PBMC of patients was isolated from whole blood with density gradient centrifugation using separation medium (Ficoll-Paque PLUS, GM) and washed in PBS (Beijing Solarbio Science & Technology Co., China). Total RNA was extracted using TRIzol (Invitrogen Life Technologies, USA) and RNeasy Mini Kit (Qiagen company, GM). After extraction, the RNA purity was determined by a NanoPhotometer spectrophotometer (IMPLEN, CA, USA), the concentration was determined by a Qubit 2.0 Fluorometer (Life Technologies, CA, USA), and the integrity was determined by an RNA Nano 6000 Assay Kit in the Agilent Bioanalyzer 2100 system (Agilent Technologies, CA, USA) from the Biomark Technologies company (Qingdao, China).
The transcriptome sequencing on extracted total RNA was conducted in Hiseq 2500 platform (Illumina, San Diego, CA, USA) and generated with paired-end reads. The adaptor sequences and low-quality raw reads were removed as quality control and transformed into a clean read. After data processing, the clean reads were mapped to the reference genome using TopHat2 software, and mapped reads would be annotated and further analyzed as detectable genes (40).
Differential Expression and Enrichment Analysis
The RNA-seq data were analyzed by DESeq2 R package to identify differentially expressed genes (DEGs) between different disease conditions. The p-values were adjusted using Benjamini and Hochberg’s approach for controlling the false discovery rate. Genes with an adjusted p-value <0.05 and fold change (FC) value > |1.5| (equals log2FC > |0.584|) between groups were concluded as DEGs.
The canonical pathway enrichment analysis on the above DEGs was performed on IPA software (Ingenuity Systems; Qiagen China Co., Ltd.). Z-score was calculated to infer the bioactivated status of relative pathways, and those canonical pathways with p-value < 0.001 (equals -LogP > 3.0) and Z-score > |2.0| were considered as significantly activated (Z-score > 2.0) or significantly inhibited (Z-score < −2.0) pathway.
Gene Set Enrichment Analysis
The Gene Set Enrichment Analysis (GSEA) on whole-genome expression was performed on the GSEA software (v4.1.0). The hallmark gene set was downloaded from MSigDB Collections and considered the reference genome (41). The enrichment scores (ES) were calculated from weighted Kolmogorov–Smirnov-like statistics, and its magnitude reflected the correlation between a gene set and phenotype. The higher ES of the gene set means the higher possibility that this gene set was expected enriched in a particular phenotype.
Weighted Gene Co-Expression Network Analysis
The weighted gene co-expression network analysis (WGCNA) was performed on the SangerBox platform (http://sangerbox.com/Tool) with three steps, and default settings were used as thresholds. WGCNA can analyze the relationship between genes and divide them into multiple modules. The hub genes were defined as a high degree of connectivity (>0.90) inside different modules and significantly (adjusted p-value < 0.05) correlated to phenotype.
Transcriptional Meta-Analysis
For thorough searching of public datasets, the Preferred Reporting Items for Systematic Reviews and Meta-Analyses (PRISMA) workflow chart was made according to recommendations (42). The selected datasets were further input to ImaGEO to conduct the transcriptional meta-analysis (43). Since the divergence of included datasets, the effect size with the random-effect model was used as the estimation model. The meta-DEGs were defined as genes with adjusted p-value < 0.05, Qpval > 0.05, and tau2 = 0 in the output results.
HIV-1 Cell-Associated DNA and RNA Measurement
The total cellular DNA and RNA were extracted from isolated PBMC samples by QIAamp DNA Mini Kit (Qiagen, Valencia, California) and HiPure Total RNA Plus Mini Kit (Magen, Guangzhou, China), respectively, and then amplified and quantified with the HIV DNA and cell-associated RNA (ca-RNA) quantitative detection kits (SUPBIO, Guangzhou, China) on the Roche LightCycler 480 (LC480) real-time PCR platform. The quantification of HIV caDNA and caRNA was multiplied by the percentage of CD4+ T cells in PBMC and the quantification of DNA/RNA copies among 1× 106 cells.
Statistical Analyses
For continuous variables with normal distribution, data were presented as mean and standard deviations and were analyzed by Student’s t-test. For variables with non-normal distribution, data were expressed as median and range of IQR, and the p-values were determined by an unpaired, two-tailed Mann–Whitney test. The correlations were analyzed using the Spearman test. Correlations were analyzed using the Spearman test. The p-value < 0.05 was considered statistically significant. All statistical analyses were performed using SPSS (version 25.0, SPSS Inc., Chicago, IL, USA) and GraphPad Prism (v8.0.1, GraphPad Software, La Jolla, CA).
Results
Characteristics of the Patients
We identified 421 PLWH treated with cART for more than 4 years in the HIV/AIDS clinical center of PUMCH, and 58 patients (20 INRs and 38 IRs) were included eventually based on the criteria described in the methods. The median age of the included patients was 46 (IQR, 36–53) years old, and 89.7% were male. The INRs experienced poor immune reconstitution and had a significantly lower CD4+ T-cell count of median 252 (IQR, 211–290) cells/μL when compared to IRs [900 (IQR, 829–1,116) cells/μL, p < 0.0001], despite the similar duration of suppressed cART treatment (Table 1).
These included INRs showed lower naïve CD4+ T-cell counts (p < 0.001), lower RTE CD4+ T-cell counts (p< 0.001), and lower CD4/CD8 ratio (p < 0.001) when compared with IRs (Table 1). Meanwhile, the INRs exhibit elevated expression levels of HLA-DR, CD38, Ki-67, and PD-1 on CD4+ T cells and CD8+ T cells (Figure 1), showing the enhanced immune activation and exhaustion in the INRs. In line with our previous understanding, the insufficient thymic output and the elevated immune activation were composited as the salient immunological characteristics of INRs.
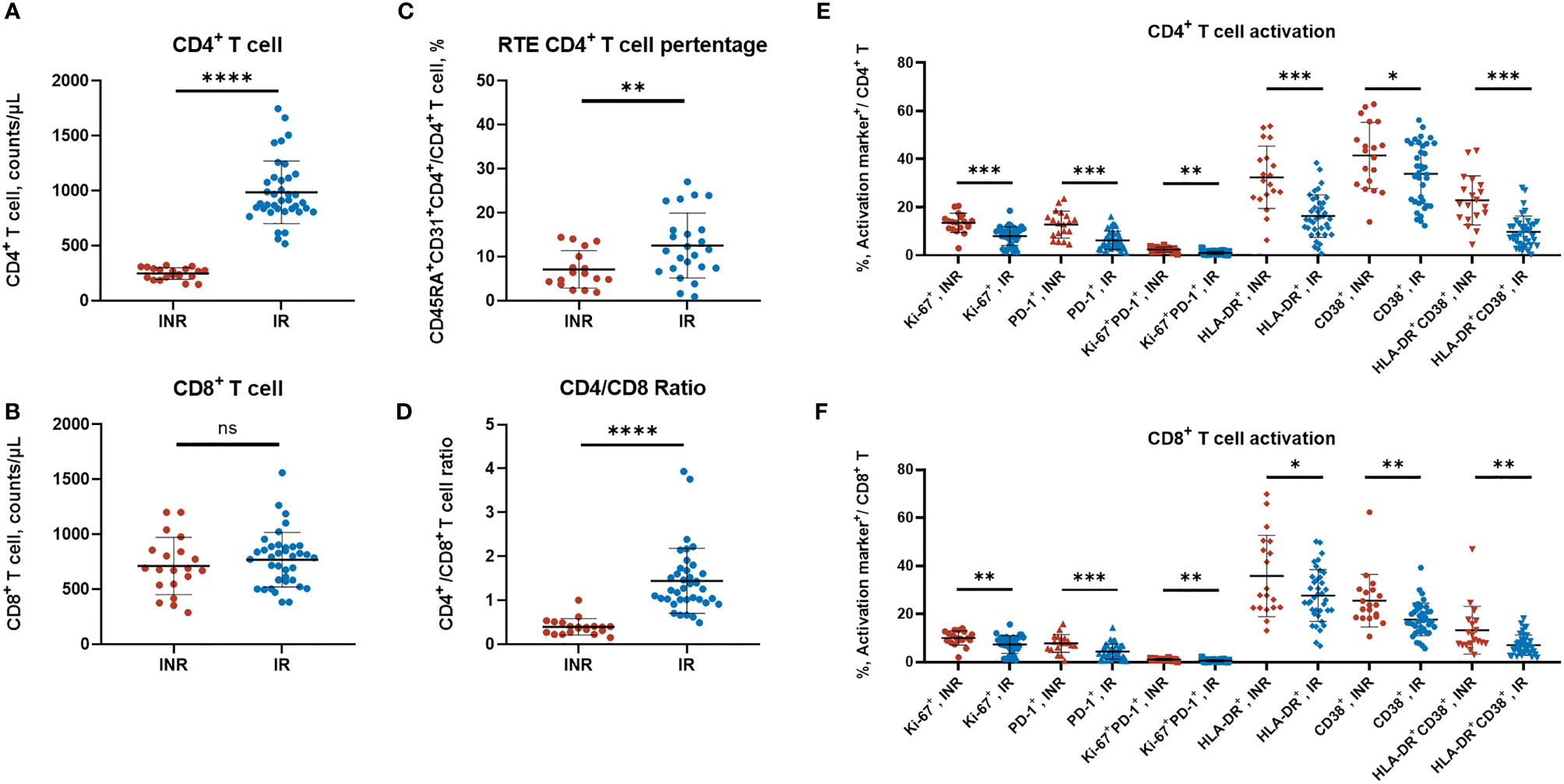
Figure 1 The T-cell subtypes and activation profiles of HIV/AIDS patients. (A–D) The CD4+ T-cell counts, CD8+ T-cell counts, RTE CD4+ T-cell percentage, and the CD4/CD8 ratio between INR and IR patients. Each dot represents one patient. (A, B, D) contained 20 INR and 38 IR samples, (C) contained 17 INR and 24 IR samples. (E, F) The CD4+ T and CD8+ T-cell activation profiles were measured by flow cytometry (surface markers including HLA-DR, CD38, PD-1, and intracellular marker Ki67). Data are mean ± SD, and the p-values were determined by a two-tailed Mann–Whitney test. *p < 0.05 **p < 0.01, ***p < 0.001, ****p < 0.0001; NS, not significant.
RNA-seq and DEGs Analysis
For a better understanding of the immune activation profiles of INR patients, we conducted the RNA-seq on 16 patients (8 INRs and 8 matched IRs) to evaluate the differential gene expression profiles. There was no significant statistical difference in sex (p= 1.000), age (p= 0.959), duration of cART (p= 0.853), and the nadir CD4+ T cells [INRs vs. IRs: 12 (IQR, 6–42) vs. 34 (IQR, 8–72) cells/μL (p= 0.505)] between the selected and matched patients, except the current CD4+ T cells [INRs vs. IRs: 223 (IQR, 166–250) vs. 756 (IQR, 658–921) cells/μL (p < 0.001)] (see Table S1).
The PBMC samples were collected and analyzed with RNA-seq. A total of 316 DEGs are identified, namely, 146 upregulated genes and the remaining downregulated 170 genes in the INRs compared to IRs (Figure 2A and Table S2). The Gene Ontology (GO) functional classification analysis identified 99 biological processes groups (BP), 18 cellular component groups (CC), and 11 molecular function groups (MF) among 146 up-DEGs. In contrast, no significant enriched group was identified among 170 down-DEGs (see Table S3). The defense response to virus (GO:0051607), the type I interferon signaling pathway (GO:0060337), and the mitotic cell cycle process (GO:1903047) were the most highly represented terms in BP (Figure 2B).
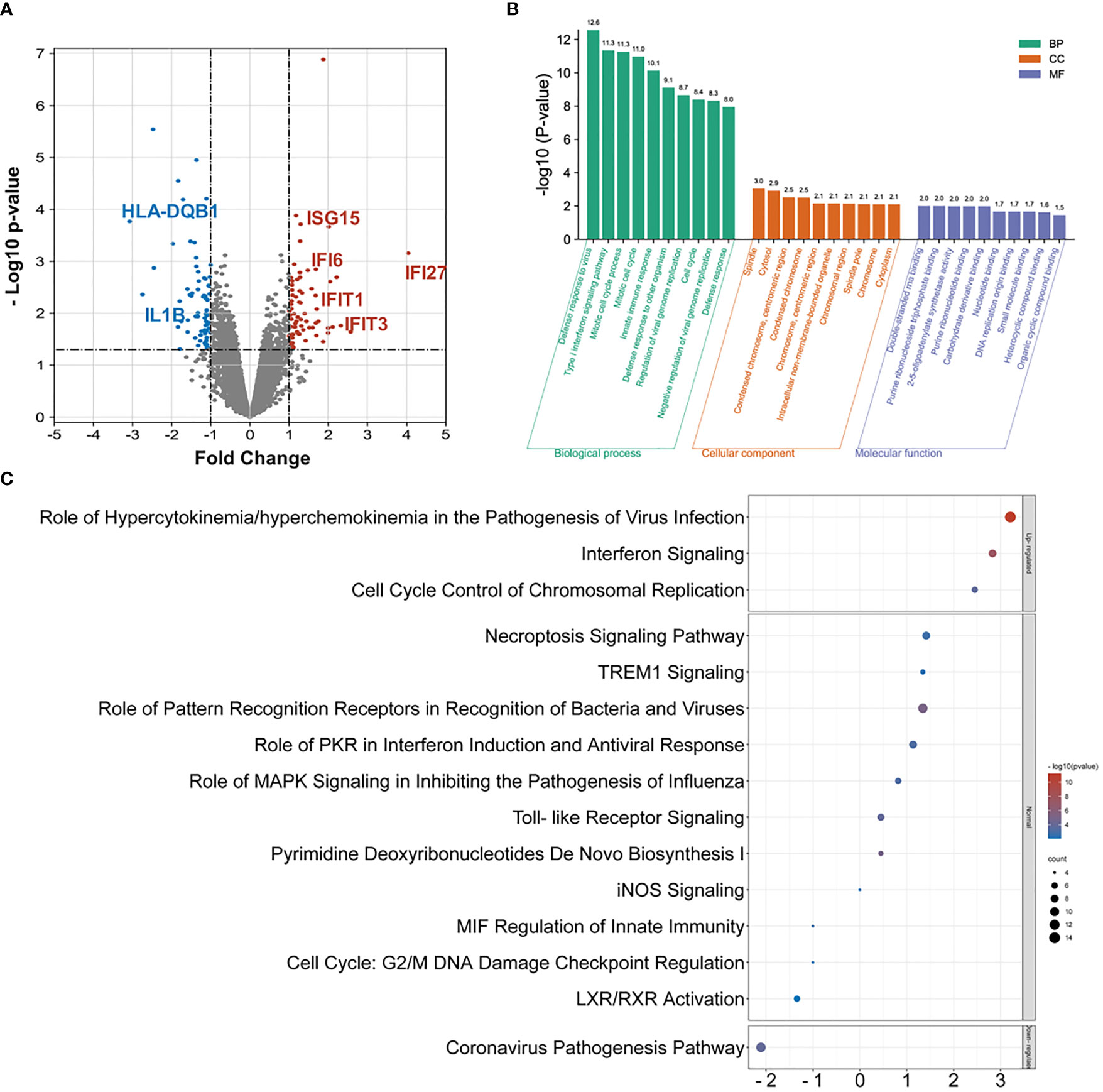
Figure 2 The RNA-seq and DEG analysis among INR and IR groups. (A) The volcano chart on differentially expressed genes (DEGs) identified by RNA-seq between INR and IR patients (DEG defined as p-value < 0.05 and FC > |1.5|). (B) The GO analysis on upregulated DEGs and only the top 10 enriched terms in each category were shown. (C) Top enriched canonical pathways following ingenuity pathway analysis (IPA). Canonical pathways with a p-value < 0.001 were labeled, and the x-axis represents the value of the Z-score.
The IPA was further performed to evaluate the function of the assembled up-DEGs and down-DEGs. A total of 54 pathways were enriched with predicted Z-scores, and 15 of them were considered statistically significant (see Table S4a). Among these, only the role of hypercytokinemia or hyperchemokinemia in the pathogenesis of virus infection pathway (ratio = 0.175), the interferon signaling pathway (ratio = 0.222), and the cell cycle control of chromosomal replication pathway (ratio = 0.107) were considered upregulated (Z-score > 2) and the coronavirus pathogenesis pathway (ratio = 0.057) was considered downregulated (Z-score < −2) (Figure 2C). Notably, the interferon signaling pathway exhibited the highest impacted ratio among the four regulated pathways. The upstream regulator analysis also identified a robust activation of the IFN-associated regulators among these DEGs (see Table S4b).
Gene Set Enrichment Analysis
The former GO and IPA analysis mainly focused on the identified 316 DEGs; we conducted the gene set enrichment analysis (GSEA) on whole-genome data to further illustrate the holonomic biological functions of the transcriptome. With 12,195 entries of mRNA expression data, the GSEA analysis identified 6,287 (51.6%) upregulated genes in INRs and 5,908 (49.7%) upregulated in IRs. The hallmark pathways mapping showed 43 enriched pathways in INRs and 6 enriched pathways in IRs (Figure 3A). Among these enriched pathways, the IFN-α response (ES = 0.62, signal%= 85%) and the IFN-γ response (ES = 0.59, signal%= 69%) were the top gene sets with the highest enrichment score in the INR group (Figure 3B). Forty-eight core genes were commonly enriched in both the IFN-α response and the IFN-γ response pathway, and 31 of 48 (64.6%) were not included in the former 316 DEGs, illustrating the additional functional role of non-DEGs (see Table S5).
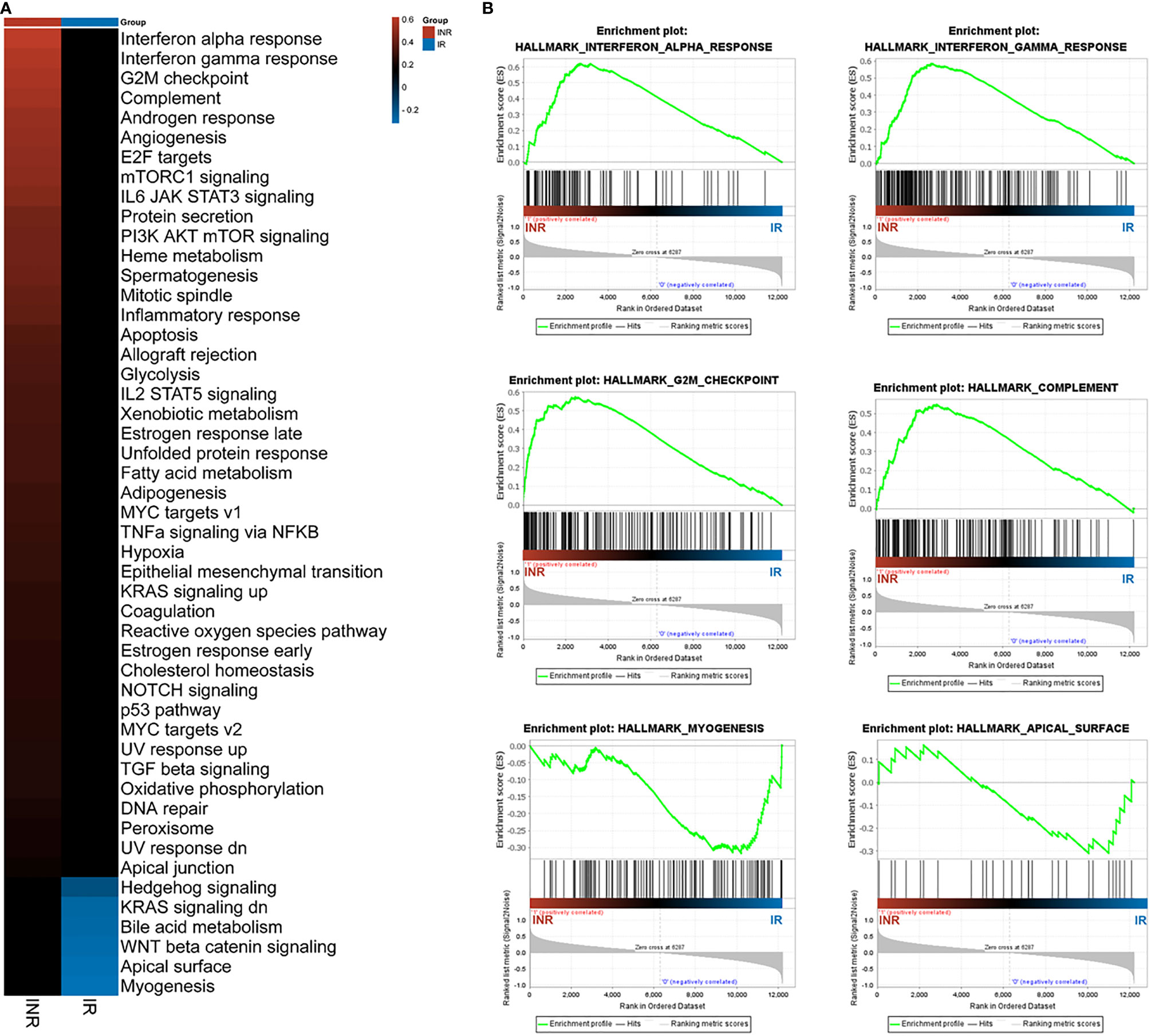
Figure 3 The GSEA analysis results. (A) The GSEA analysis results among patients. The colored patches were quantified with enrichment score (ES) from GSEA results. (B) The enriched top 4 pathways in INRs and the top 2 pathways in IRs. The y-axis represents ES, and the x-axis denotes genes (vertical black lines) represented in gene sets. The colored band at the bottom represents the degree of correlation of genes with the INR phenotype (red for positive and blue for negative correlation).
Weighted Gene Co-Expression Network Analysis
We additionally conducted the weighted gene co-expression network analysis (WGCNA) to explore the co-expression relationships among whole-genome data. With the correlation coefficient threshold selected as 0.85, the soft-thresholding power was set as 12 (Figure 4A). The WGCNA analysis identified 34 gene co-expression modules with internal relationships, and each of these was independent of other modules (Figure 4B). The brown module (n = 2007), the coral_1 module (n = 1366), the antique_white_4 module (n = 1272), the dark_olivegreen module (n = 1270), and the midnight_blue module (n = 1134) comprised the majority of 12,195 genes (Figure 4C). The weighted expression analysis showed good connectivity inside each gene module (Figure 4D). The module-trait correlation analysis showed that modules were related to INR, including the dark_violet module (r = 0.39), the skyblue_2 module (r = 0.38), the indian_red_4 module (r = 0.37), and the midnight_blue module (r = 0.35) (Figure 4E).
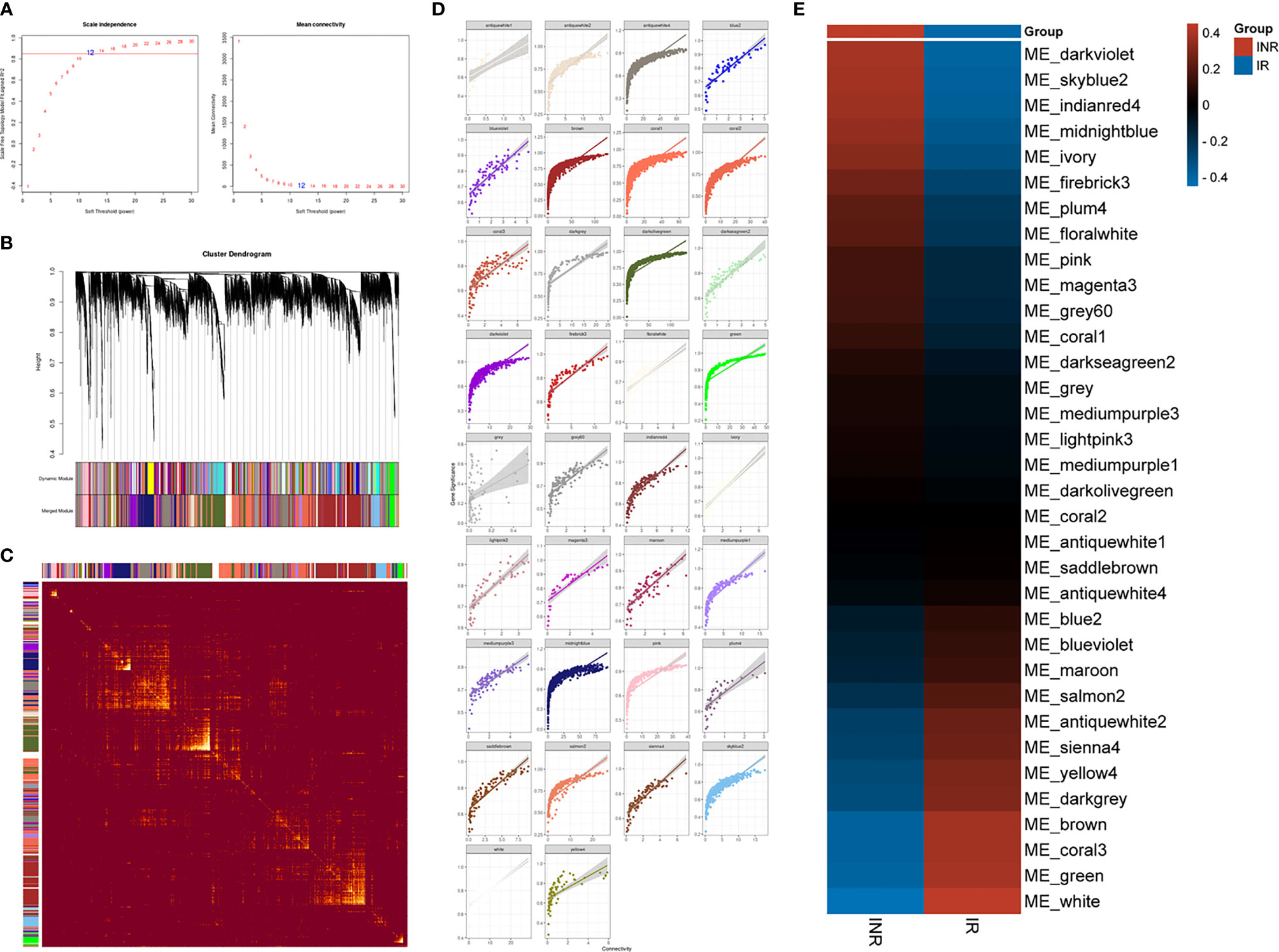
Figure 4 The WGCNA analysis results. (A) The analysis of the scale-free fit index (left) and the mean connectivity (right) to determine the soft-thresholding powers. (B) Cluster dendrogram of genes modules, with gene dissimilarity based on the topological overlap, together with assigned 34 module colors. (C) The network heatmap plot among 34 modules and the progressively saturated yellow colors indicated higher overlap between modules. (D) Scatterplot of the intramodular analysis (module membership versus gene significance) of genes in every separate module. (E) Module–trait relationship to the INR and IR phenotype. Each row responds to a colored module eigengene (ME) detected by WGCNA analysis and each column to a trait. The table is color-coded according to gene module and phenotype correlation coefficient, ranging from −1 to 1 (blue to red).
The midnight_blue module exhibited large gene counts (n = 1,134) and the higher correlation index (r = 0.35) with INR (see Table S6). The functional annotation showed that the type I interferon signaling pathway (GO:0060337) was the most significant progress (strength = 1.47, FDR = 7.12e-08) among 67 enriched biological progresses in the midnight_blue module (see Table S7). Several genes in the midnight_blue module such as IFI27 (R = 0.94), OAS1 (R = 0.93), STAT2 (R = 0.92), BST2 (R = 0.92), OAS2 (R = 0.92), XAF1 (R = 0.91), IFI35 (R = 0.91), MYD88 (R = 0.91), and SAMHD1 (R = 0.90) had high gene significance with INR and thus considered as hub genes. In total, there were 1,045 identified hub genes among 33 gene modules through WGCNA analysis that were considered related with INR and for further validation (see Table S8).
Transcriptional Meta-Analysis
To validate the external consistent of regulated genes, we further conducted the meta-analysis on published transcriptional data of INR patients. After screening 52 published records, we included three microarray datasets (GSE77939, GSE106792, and GSE143742) following the criteria of the PRISMA workflow (Figure 5).
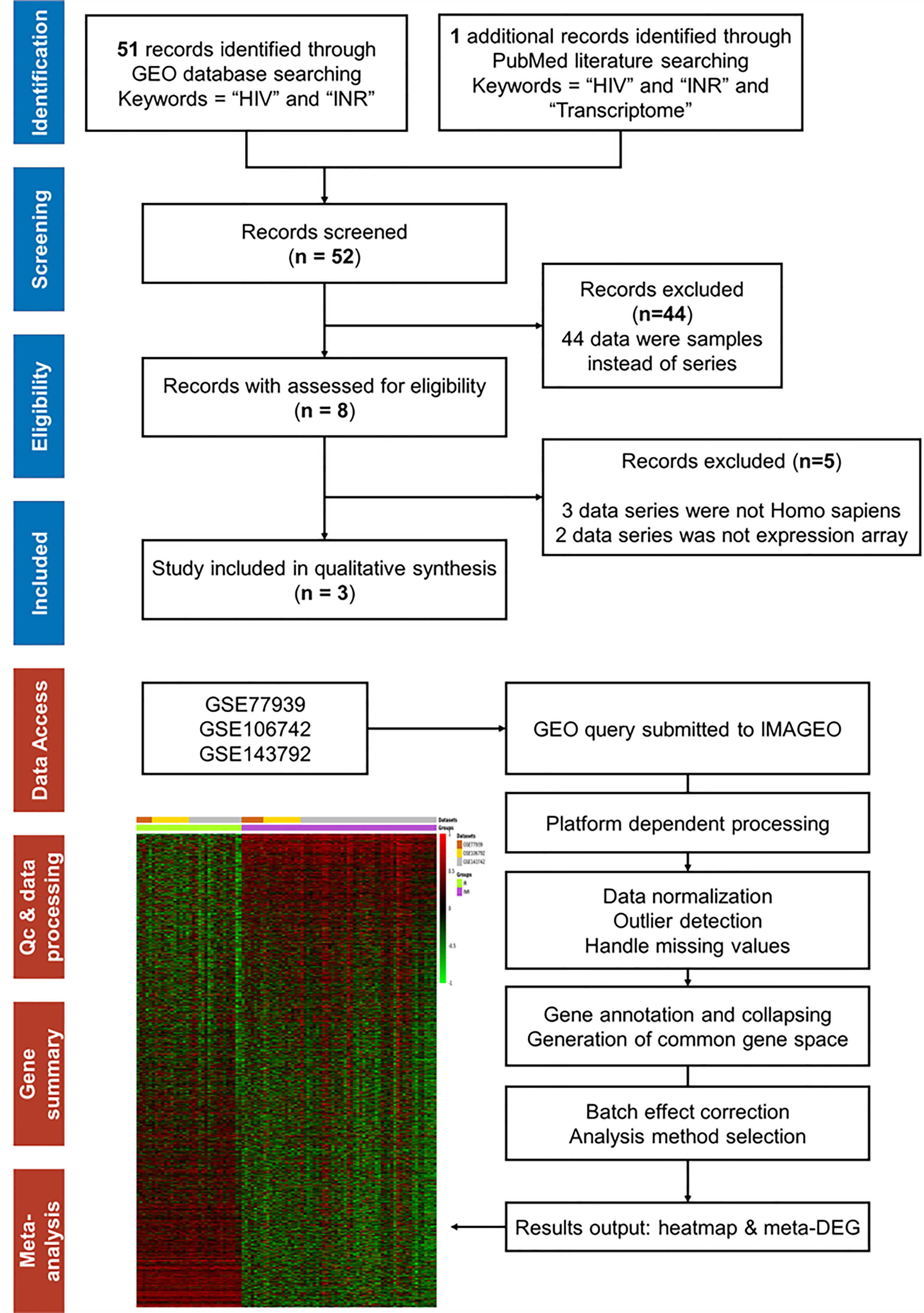
Figure 5 The PRISMA flow and search results for meta-analysis. The workflow diagram of database and literature search for mRNA expression array on INR patients and the selection process for inclusion in the meta-analysis. The workflow diagram of transcriptional meta-analysis on the IMAGEO website includes the following: First, input data were selected from public repositories. Secondly, the raw data were reprocessed to get gene expression matrices and quality controls were performed. Then, a common gene space for all the datasets was created where batch effect correction can be applied and, finally, the meta-analysis was performed. In our analysis, the Fisher p-value method [summary of −log(p-value) across studies] was selected as the analysis method. The allowed missing values (%) were defined as 10%, and the p-value < 0.05 was the adjusted p-value threshold.
In total, 63 INR and 34 IR samples from 3 datasets were included, and the dissimilarity in the immune recovery criteria, the microarray platform, and the cell types between different studies were undeniable (Table 2). Thus, the random effect test was chosen to reduce variance. After batching the expression baseline and examining the missing data, a total of 16,821 genes were included for further analysis, and the heatmap of whole-genome expression of 97 samples is shown in Figure 5. With the threshold of adjusted p-value < 0.05, Qpval > 0.05, and tau2 = 0 in the random effect test, 409 meta-DEGs (56 upregulated and 353 downregulated) were obtained (see Table S9).
Validation and Efficacy Evaluation of Identified Genes
Taken together, the IFI27 [IFN-α Inducible Protein 27, also known as IFN stimulated gene 12 (ISG12)] was the only gene that simultaneously identified as the DEG, the hub gene of WGCNA, and the meta-DEG (Figure 6A). The expression levels of IFI27 were consistently upregulated in INR patients across different datasets with different conditions, especially in the PBMC samples (Figure 6B). Furthermore, the expression of IFI27 in PBMC samples was significantly negatively correlated with the CD4+ T cells counts of PLWH, and the area under the curve (AUC) of the ROC curve was larger than 0.80 in dataset GSE44228 (Figure 6C). The sensitivity% and the specificity% were 94.7% and 67.9% as the relative expression levels of IFI27 to GAPDH > 0.60 in distinguishing PLWH with poor immune recovery (CD4+ T cells < 350 cells/μL) (see Table S10). These results supported the vital commonality of the enhanced ISG expression (especially IFI27) and the activated IFN signaling pathway in INRs.
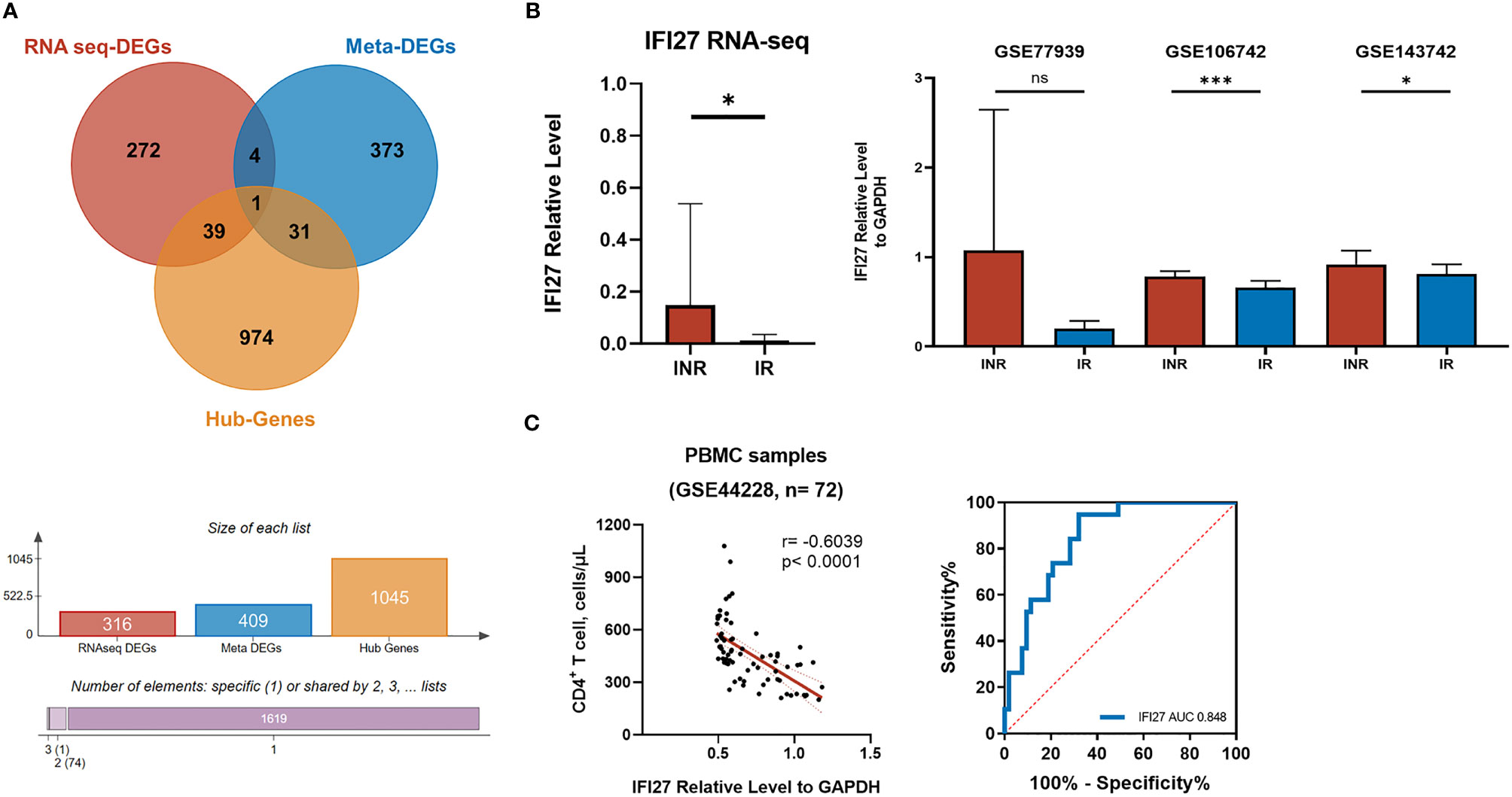
Figure 6 The screening of critical genes in the mechanism of INR. (A) The Venn diagram showing the overlapping of DEGs from our RNA-seq analysis (red), the meta DEGs identified from the transcriptional meta-analysis on published GEO data series (blue), and the hub genes from WGCNA analysis (yellow). (B) The relative expression level of IFI27 to GAPDH included transcriptome analysis. The probes of each GEO data series were transformed into gene symbols through R, and the geographical mean of the same gene was merged and calculated. The p-values of relative expression were determined by the random-effect model of IMAGEO. *p < 0.05, ***p < 0.001; NS, not significant. (C) The correlation between IFI27 expression and the CD4+ T-cell counts and the ROC curve.
IFN Response and the Viral Response
Although we identified the characteristic gene and patterns of INRs, questions remained to be answered as to what might be the driving factors of elevated IFI27 expression and the activated IFN response among these patients. The essential role of IFN signaling in responding to viral infection was well known. However, there was no significant difference in the rate of hepatitis B virus (HBV), cytomegalovirus (CMV) co-infection, or the level of CMV-specific IgG titer among our INR and IR patients (Figures 7A, C, D and Table 1). It was noted that the limited number of INR patients (2/20, 10%) were previously infected with hepatitis C virus (HCV), making it statistically different between two groups while unable to explain the extensive abnormal enhanced IFN response among INR patients (Figure 7B).
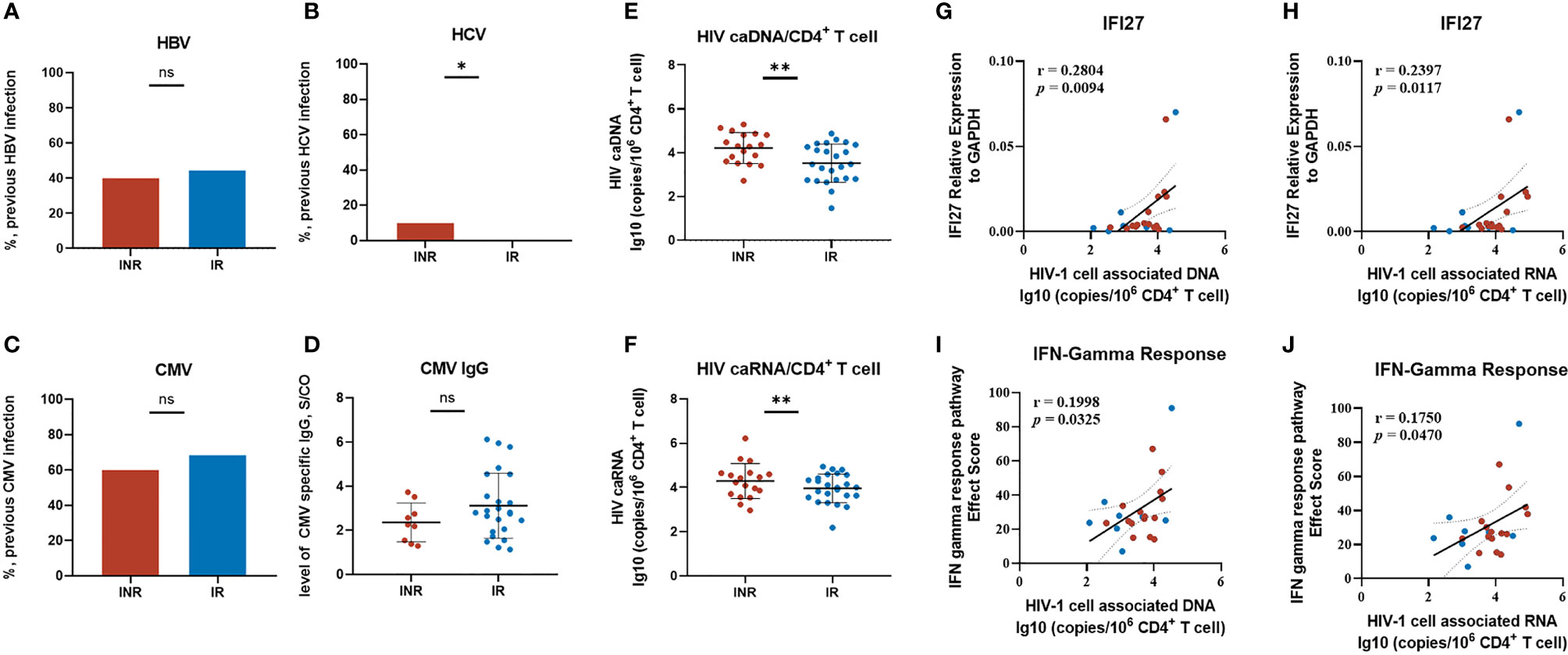
Figure 7 Exploring the driving factors of IFI27 expression and the IFN pathway. (A–D) The previously viral infection status of our INR and IR patients includes HBV, HCV, and CMV. (E, F) The level of HIV cell-associated DNA and RNA between groups. (G–J) The correlation of HIV caDNA/caRNA with IFI27 score and IFN-γ response score. The IFN response score was calculated by summing up the Effect Score (ES) in GSEA of every individual pathway-related gene. The p-values of infection rates among groups were determined by a two-tailed Mann–Whitney test. *p < 0.05, **p < 0.01; NS, not significant. The linear regression analysis was calculated by Spearman’s rank correlation coefficient when p-values < 0.05 were considered significant.
Attention came back to HIV. Despite the fact that all of our INR and IR patients had achieved viral control (HIV-1 viral load less than 20 copies/mL) for years, the HIV reservoirs persisted and could produce replication-competent viruses under cART. We measured the HIV-1 cell-associated DNA (caDNA) and cell-associated RNA (caRNA) among these patients. The level of HIV-1 total DNA/CD4+ T cells in INRs was higher than in the IRs (p < 0.001) (Figure 7E), and a similar result was also observed in HIV-1 caRNA/CD4+ T cells (p < 0.001) (Figure 7F). Further correlation analysis showed the expression of IFI27 and the IFN-γ response score were linearly dependent on the level of HIV-1 caDNA and HIV-1 caRNA (Figures 7G–J), indicating that the persistence and the transcription of HIV reservoirs might be in alliance with the activated IFN pathway in INR patients.
Discussion
The accessibility and activity of cART help to boost the rapid viral suppression by stalling various steps of the HIV-1 life cycle, and most HIV/AIDS patients can experience immune reconstitution after receiving cART (1). However, approximately 10%–40% of PLWH cannot achieve optimal CD4+ T-cell counts despite virological suppression and termed as INRs (2, 3). The suboptimal CD4+ T-cell recovery has been proved to be associated with a substantial increase in the risk of mortality, and AIDS-related and non-AIDS-related morbidity, calling for the urgency of investigating the underlying mechanisms of INR and developing relevant interventions (2, 4). Previously, studies focused on the gene expression profiles of the PBMC isolated from HIV-1 subtype-C infected INRs (44) and of the CD4+ T cells isolated from INRs (10, 45); these results provided valued but un-unified information due to the dissimilarity in sequencing platforms, cell types, and the selection criteria of included patients. In the present study, we conducted the RNA-seq on PBMC of INRs and matched IRs, and we constructed the consequent comparative transcriptional analysis and meta-analysis on other published datasets that helped to present the new insights into the molecular mechanisms of INR.
Our results agreed that the INRs exhibited persistent abnormal immune activation and insufficient thymic output as the important immunological characteristics. To reduce the bias, the further RNA-seq on INRs and matched IRs with similar nadir CD4+ T cell and other demographic profiles identified a total of 316 DEGs (146 up-DEGs and 170 down-DEGs) among groups. The GO and IPA analysis showed that the interferon signaling pathway was enriched among DEGs. Expanding to the whole genome expression data, the GSEA analysis confirmed that the IFN-α response (ES = 0.62) and the IFN-γ response (ES = 0.59) were the top gene sets with the highest ES in the INRs. The type I interferon signaling pathway (GO:0060337) was also the most significant progress in the midnight_blue module in the WGCNA analysis, which was identified with large gene counts (n = 1,134) and the higher correlation index (r = 0.35) with INR, showing the signature of enhanced IFN response among our INR patients.
Altogether, our main results revealed that the activated IFN response pathway contributed to the significant immunological characteristics of immune activation profiles among INRs. The binary connection between IFN signaling and HIV infection caused much interest and debate in the field (46). It has been reported that the enhancement of IFN signaling during acute infection would boost the anti-viral effects; however, the persisting activation of IFN signaling also results in immune depletion and reservoir maintenance during chronic infection (46). Studies showed that the administration of anti-IFNAR antibodies to ART-suppressed HIV-infected humanized mice reduced immune activation and reservoir size (47, 48). HIV/AIDS patients with higher circulating IFN-γ were associated with poor CD4+ T-cell recovery (49). In addition, clinical evidence had shown that applying the adjuvant IFN therapy to HIV/HBV co-infected patients resulted in the transient declines of CD4+ T-cell counts (50, 51). Taken together, we considered that limiting the contribution of the IFN response pathway in the maintenance of HIV reservoirs among INRs could be thought to be an important target for clinical improvement (52).
In addition, we conducted the meta-analysis on three published datasets and obtained 409 meta-DEGs (56 up-meta-DEGs and 353 down-meta-DEGs). The IFI27 from the IFN response pathway was the only gene that simultaneously identified as the DEG, the hub gene of WGCNA, and the meta-DEG. Notably, the association between HIV infection and ISG, especially IFI27, had been noticed years ago. Previous studies reported the relative higher IFI27 expression in the CD4+ T cells of PLHW with lower CD4+ T-cell counts (27), and also higher in the monocytes of PLWH with high HIV-1 viral load (53). Nevertheless, the expression of IFI27 in the CD8+ T cells was not significantly different from patients (27), and it was even downregulated in primary macrophages after HIV infection (54), while until now, the role and the driving factor of IFI27 expression in PLWH remained to be explored.
Through the analysis on RNA extracted from the whole PBMC, rather than specific cell types, and the research through unsupervised transcriptomic profiling on whole genome, instead of focusing on ISG as in previous studies, our results helped to provide relative unbiased insights of enhanced IFI27 expression among INR patients. We further examined the relationship of IFI27 with CD4+ T-cell recovery, and the efficiency of IFI27 was validated in another dataset. We found that the expression of IFI27 was significantly negatively correlated with the CD4+ T cells counts of PLWH, and the relative expression level of IFI27 to GAPDH > 0.60 was shown with significant power in distinguishing PLWH with poor immune recovery (CD4+ T cells < 350 cells/μL). The validated results supported the vital commonality of the enhanced ISG expression (especially IFI27) and the activated IFN signaling pathway in INRs.
Moreover, we found that the expression of IFI27 and the IFN-γ response score were linearly dependent on the level of HIV-1 caDNA and HIV-1 caRNA. We speculated that the persistence and transcription of HIV reservoirs might be the driving factor of enhanced IFI27 expression and IFN response among INRs. Previous studies had shown the relationship between HIV reservoirs and suboptimal CD4 recovery (55–57). Interestingly, a recent study showed that the increased homeostatic cytokine levels, especially IL-7 and IL-15, were also associated with HIV reservoirs in T-cell subsets and poor immune reconstitution during cART (58). The role of proinflammatory and homeostatic cytokines in the connection between viral reservoirs recovery and the immunopathogenesis of chronic IA had been reported (59, 60). Of note, the HIV-1 Vpr protein was reported as an essential factor in the HIV-1 gene expression progress of cytokine-treated resting CD4+ T cells (61). The HIV-1 Vpr could be produced and released from HIV-1 sequestered tissue reservoirs during cART treatment (62, 63), and Vpr has been shown to participate in the HIV-mediated immune dysfunction and CD4+ T-cell depletion (64–69). Besides, the persistence of Vpr was able to upregulate the expression of various ISGs, including IFI27, in monocyte-derived dendritic cells (70). Hence, we hypothesize that the persistence of HIV reservoirs among INRs may induce the IFN response by the production of Vpr. However, the specific mechanisms underlying the interaction between proinflammatory and homeostatic cytokines and the HIV viral reservoirs remain further explored.
Although the present study is the first to conduct a comparative transcriptional analysis to explore the characteristic genes and patterns of INRs, our study also has limitations. Firstly, our transcriptional data were restricted to the limited matched PLWH from PUMCH; these patients were relatively young, and their CD4+ T-cell counts were mainly below 100/μL at cART initiation. Even though we had used the external datasets to validate the efficiency of identified genes, whether these deficiencies would affect the results or the conclusions needed a larger sample size to validate. Secondly, we applied the PBMC samples to measure HIV reservoirs, considering the availability and convenience of acquiring samples. Despite the fact that HIV reservoirs would be mainly represented in lymphoid tissues and gut-associated lymphoid tissue (GALT) (71, 72), our previous studies exhibited that the measurement of HIV reservoirs through peripheral blood also provided valuable information (73–76). Finally, we did not further study the connection between IFN response and microbial translocation, which was reported as a significant cause of persistent immune activation among INRs (20, 77–79). Recent studies had shown that the gut ISG levels were correlated with microbial translocation and microbial metabolites (80–82), while we were not powered to investigate the IFN gene expression profiles of the gut district and the data of gut microbiota had not been undertaken. Thus, the associations between IFN response and CD4 recovery and viral reservoirs observed in this study should be interpreted with caution. Nevertheless, our findings and conclusions could inform the further exploration of immunological characteristics and underlying mechanisms of INRs.
In conclusion, the present study reports the immunological gene and pattern from the comprehensive bioinformatic analysis of INR patients. Of note, our study finds and validated the involvement of the expression of IFI27 and the IFN response pathway in the immunological characteristics of INRs. The enhanced ISG expression and IFN response may align with the persistence and transcription of HIV reservoirs. Our group has registered and initiated several randomized clinical trials in treating INRs, and the coming results will further build our knowledge of the connection between HIV reservoirs, the IFN response, and the CD4+ T-cell recovery.
Data Availability Statement
The original contributions presented in the study are deposited in the NCBI SRA repository, accession number PRJNA777889.
Ethics Statement
The studies involving human participants were reviewed and approved by the institutional ethics board of Peking Union Medical College Hospital. The patients/participants provided their written informed consent to participate in this study.
Author Contributions
XsL conducted the comparative transcriptional analysis, conducted the measurement of HIV reservoirs, and drafted the manuscript. LLi, LLu, XdL, XxL, YL, and XS provided regular clinic care on included patients. YH conducted the regular HIV-1 viral load measurement. ZQ conducted the regular cell cytometry measurement. WC reviewed the literature and revised the manuscript. TL advised the entire work. All authors reviewed the manuscript, provided feedback, and approved the manuscript in its final form.
Funding
The research was supported by the national key technologies R&D program for the 13th five-year plan (2017ZX10202101).
Conflict of Interest
The authors declare that the research was conducted in the absence of any commercial or financial relationships that could be construed as a potential conflict of interest.
Publisher’s Note
All claims expressed in this article are solely those of the authors and do not necessarily represent those of their affiliated organizations, or those of the publisher, the editors and the reviewers. Any product that may be evaluated in this article, or claim that may be made by its manufacturer, is not guaranteed or endorsed by the publisher.
Acknowledgments
We thank the IPA software service provided by Dr. Sun from the Institute of Basic Medical Sciences, Peking Union Medical College. We especially thank all the patients and participants in the AIDS research center, Peking Union Medical College Hospital.
Supplementary Material
The Supplementary Material for this article can be found online at: https://www.frontiersin.org/articles/10.3389/fimmu.2022.807890/full#supplementary-material
References
1. Moir S, Chun TW, Fauci AS. Pathogenic Mechanisms of HIV Disease. Annu Rev Pathol (2011) 6:223–48. doi: 10.1146/annurev-pathol-011110-130254
2. Yang X, Su B, Zhang X, Liu Y, Wu H, Zhang T. Incomplete Immune Reconstitution in HIV/AIDS Patients on Antiretroviral Therapy: Challenges of Immunological Non-Responders. J Leukoc Biol (2020) 107(4):597–612. doi: 10.1002/jlb.4mr1019-189r
3. Health UDo, Services H. Guidelines for the Use of Antiretroviral Agents in HIV-1-Infected Adults and Adolescents (2009). Available at: https://aidsinfonihgov/OrderPublication/OrderPubsBrowseSearchResultsTableaspx?ID=115.
4. Gaardbo JC, Hartling HJ, Gerstoft J, Nielsen SD. Incomplete Immune Recovery in HIV Infection: Mechanisms, Relevance for Clinical Care, and Possible Solutions. Clin Dev Immunol (2012) 2012:670957. doi: 10.1155/2012/670957
5. Okoye AA, Picker LJ. CD4(+) T-Cell Depletion in HIV Infection: Mechanisms of Immunological Failure. Immunol Rev (2013) 254(1):54–64. doi: 10.1111/imr.12066
6. Carvalho-Silva WHV, Andrade-Santos JL, Souto FO, Coelho AVC, Crovella S, Guimarães RL. Immunological Recovery Failure in cART-Treated HIV-Positive Patients Is Associated With Reduced Thymic Output and RTE CD4+ T Cell Death by Pyroptosis. J Leukoc Biol (2020) 107(1):85–94. doi: 10.1002/jlb.4a0919-235r
7. Sereti I, Altfeld M. Immune Activation and HIV: An Enduring Relationship. Curr Opin HIV AIDS (2016) 11(2):129–30. doi: 10.1097/coh.0000000000000244
8. d'Ettorre G, Paiardini M, Ceccarelli G, Silvestri G, Vullo V. HIV-Associated Immune Activation: From Bench to Bedside. AIDS Res Hum Retroviruses (2011) 27(4):355–64. doi: 10.1089/aid.2010.0342
9. Roider JM, Muenchhoff M, Goulder PJ. Immune Activation and Paediatric HIV-1 Disease Outcome. Curr Opin HIV AIDS (2016) 11(2):146–55. doi: 10.1097/coh.0000000000000231
10. Younes SA, Talla A, Pereira Ribeiro S, Saidakova EV, Korolevskaya LB, Shmagel KV, et al. Cycling CD4+ T Cells in HIV-Infected Immune Nonresponders Have Mitochondrial Dysfunction. J Clin Invest (2018) 128(11):5083–94. doi: 10.1172/jci120245
11. Saidakova EV, Shmagel KV, Korolevskaya LB, Shmage NG, Chereshnev VA. Lymphopenia-Induced Proliferation of CD4 T-Cells Is Associated With CD4 T-Lymphocyte Exhaustion in Treated HIV-Infected Patients. Indian J Med Res (2018) 147(4):376–83. doi: 10.4103/ijmr.IJMR_1801_15
12. Lv T, Cao W, Li T. HIV-Related Immune Activation and Inflammation: Current Understanding and Strategies. J Immunol Res (2021) 2021:7316456. doi: 10.1155/2021/7316456
13. Mavigner M, Delobel P, Cazabat M, Dubois M, L'Faqihi-Olive FE, Raymond S, et al. HIV-1 Residual Viremia Correlates With Persistent T-Cell Activation in Poor Immunological Responders to Combination Antiretroviral Therapy. PloS One (2009) 4(10):e7658. doi: 10.1371/journal.pone.0007658
14. Guihot A, Dentone C, Assoumou L, Parizot C, Calin R, Seang S, et al. Residual Immune Activation in Combined Antiretroviral Therapy-Treated Patients With Maximally Suppressed Viremia. Aids (2016) 30(2):327–30. doi: 10.1097/qad.0000000000000815
15. Maidji E, Somsouk M, Rivera JM, Hunt PW, Stoddart CA. Replication of CMV in the Gut of HIV-Infected Individuals and Epithelial Barrier Dysfunction. PloS Pathog (2017) 13(2):e1006202. doi: 10.1371/journal.ppat.1006202
16. Shmagel KV, Korolevskaya LB, Saidakova EV, Shmagel NG, Chereshnev VA, Margolis L, et al. HCV Coinfection of the HIV-Infected Patients With Discordant CD4(+) T-Cell Response to Antiretroviral Therapy Leads to Intense Systemic Inflammation. Dokl Biol Sci (2017) 477(1):244–7. doi: 10.1134/s0012496617060047
17. Singh KP, Crane M, Audsley J, Avihingsanon A, Sasadeusz J, Lewin SR. HIV-Hepatitis B Virus Coinfection: Epidemiology, Pathogenesis, and Treatment. Aids (2017) 31(15):2035–52. doi: 10.1097/qad.0000000000001574
18. Brenchley JM, Price DA, Schacker TW, Asher TE, Silvestri G, Rao S, et al. Microbial Translocation Is a Cause of Systemic Immune Activation in Chronic HIV Infection. Nat Med (2006) 12(12):1365–71. doi: 10.1038/nm1511
19. Mehraj V, Ramendra R, Isnard S, Dupuy FP, Ponte R, Chen J, et al. Circulating (1→3)-β-D-Glucan Is Associated With Immune Activation During Human Immunodeficiency Virus Infection. Clin Infect Dis (2020) 70(2):232–41. doi: 10.1093/cid/ciz212
20. Lu W, Feng Y, Jing F, Han Y, Lyu N, Liu F, et al. Association Between Gut Microbiota and CD4 Recovery in HIV-1 Infected Patients. Front Microbiol (2018) 9:1451. doi: 10.3389/fmicb.2018.01451
21. Marziali M, De Santis W, Carello R, Leti W, Esposito A, Isgrò A, et al. T-Cell Homeostasis Alteration in HIV-1 Infected Subjects With Low CD4 T-Cell Count Despite Undetectable Virus Load During HAART. Aids (2006) 20(16):2033–41. doi: 10.1097/01.aids.0000247588.69438.fd
22. Girard A, Vergnon-Miszczycha D, Depincé-Berger AE, Roblin X, Lutch F, Lambert C, et al. Brief Report: A High Rate of β7+ Gut-Homing Lymphocytes in HIV-Infected Immunological Nonresponders Is Associated With Poor CD4 T-Cell Recovery During Suppressive HAART. J Acquir Immune Defic Syndr (2016) 72(3):259–65. doi: 10.1097/qai.0000000000000943
23. Mogensen TH, Melchjorsen J, Larsen CS, Paludan SR. Innate Immune Recognition and Activation During HIV Infection. Retrovirology (2010) 7:54. doi: 10.1186/1742-4690-7-54
24. Oh H, Ghosh S. NF-κb: Roles and Regulation in Different CD4(+) T-Cell Subsets. Immunol Rev (2013) 252(1):41–51. doi: 10.1111/imr.12033
25. Kim E, Kim SH, Kim S, Kim TS. The Novel Cytokine P43 Induces IL-12 Production in Macrophages via NF-kappaB Activation, Leading to Enhanced IFN-Gamma Production in CD4+ T Cells. J Immunol (2006) 176(1):256–64. doi: 10.4049/jimmunol.176.1.256
26. Skelton JK, Ortega-Prieto AM, Kaye S, Jimenez-Guardeño JM, Turner J, Malim MH, et al. Kinetics of Early Innate Immune Activation During HIV-1 Infection of Humanized Mice. J Virol (2019) 93(11). doi: 10.1128/jvi.02123-18
27. Fernandez S, Tanaskovic S, Helbig K, Rajasuriar R, Kramski M, Murray JM, et al. CD4+ T-Cell Deficiency in HIV Patients Responding to Antiretroviral Therapy Is Associated With Increased Expression of Interferon-Stimulated Genes in CD4+ T Cells. J Infect Dis (2011) 204(12):1927–35. doi: 10.1093/infdis/jir659
28. Sivaraman V, Zhang L, Su L. Type I Interferon Contributes to CD4+ T Cell Depletion Induced by Infection With HIV-1 in the Human Thymus. J Virol (2011) 85(17):9243–6. doi: 10.1128/jvi.00457-11
29. Sedaghat AR, German J, Teslovich TM, Cofrancesco J Jr., Jie CC, Talbot CC Jr., et al. Chronic CD4+ T-Cell Activation and Depletion in Human Immunodeficiency Virus Type 1 Infection: Type I Interferon-Mediated Disruption of T-Cell Dynamics. J Virol (2008) 82(4):1870–83. doi: 10.1128/jvi.02228-07
30. Yang X, Kui L, Tang M, Li D, Wei K, Chen W, et al. High-Throughput Transcriptome Profiling in Drug and Biomarker Discovery. Front Genet (2020) 11:19. doi: 10.3389/fgene.2020.00019
31. Krämer A, Green J, Pollard J Jr., Tugendreich S. Causal Analysis Approaches in Ingenuity Pathway Analysis. Bioinformatics (2014) 30(4):523–30. doi: 10.1093/bioinformatics/btt703
32. Subramanian A, Tamayo P, Mootha VK, Mukherjee S, Ebert BL, Gillette MA, et al. Gene Set Enrichment Analysis: A Knowledge-Based Approach for Interpreting Genome-Wide Expression Profiles. Proc Natl Acad Sci USA (2005) 102(43):15545–50. doi: 10.1073/pnas.0506580102
33. Langfelder P, Horvath S. WGCNA: An R Package for Weighted Correlation Network Analysis. BMC Bioinf (2008) 9:559. doi: 10.1186/1471-2105-9-559
34. Han M, Zhu Y. Applications of Meta-Analysis in Multi-Omics. Sheng Wu Gong Cheng Xue Bao (2014) 30(7):1094–104.
35. Ritchie MD, Holzinger ER, Li R, Pendergrass SA, Kim D. Methods of Integrating Data to Uncover Genotype-Phenotype Interactions. Nat Rev Genet (2015) 16(2):85–97. doi: 10.1038/nrg3868
36. Deng M, Yin Y, Zhang Q, Zhou X, Hou G. Identification of Inflammation-Related Biomarker Lp-PLA2 for Patients With COPD by Comprehensive Analysis. Front Immunol (2021) 12:670971. doi: 10.3389/fimmu.2021.670971
37. Han Z, Hua J, Xue W, Zhu F. Integrating the Ribonucleic Acid Sequencing Data From Various Studies for Exploring the Multiple Sclerosis-Related Long Noncoding Ribonucleic Acids and Their Functions. Front Genet (2019) 10:1136. doi: 10.3389/fgene.2019.01136
38. Li QS, De Muynck L. Differentially Expressed Genes in Alzheimer's Disease Highlighting the Roles of Microglia Genes Including OLR1 and Astrocyte Gene CDK2AP1. Brain Behav Immun Health (2021) 13:100227. doi: 10.1016/j.bbih.2021.100227
39. Ma X, Tao R, Li L, Chen H, Liu Z, Bai J, et al. Identification of a 5−microRNA Signature and Hub miRNA−mRNA Interactions Associated With Pancreatic Cancer. Oncol Rep (2019) 41(1):292–300. doi: 10.3892/or.2018.6820
40. Kim D, Pertea G, Trapnell C, Pimentel H, Kelley R, Salzberg SL. TopHat2: Accurate Alignment of Transcriptomes in the Presence of Insertions, Deletions and Gene Fusions. Genome Biol (2013) 14(4):R36. doi: 10.1186/gb-2013-14-4-r36
41. Liberzon A, Birger C, Thorvaldsdóttir H, Ghandi M, Mesirov JP, Tamayo P. The Molecular Signatures Database (MSigDB) Hallmark Gene Set Collection. Cell Syst (2015) 1(6):417–25. doi: 10.1016/j.cels.2015.12.004
42. Shamseer L, Moher D, Clarke M, Ghersi D, Liberati A, Petticrew M, et al. Preferred Reporting Items for Systematic Review and Meta-Analysis Protocols (PRISMA-P) 2015: Elaboration and Explanation. BMJ (2015) 350:g7647. doi: 10.1136/bmj.g7647
43. Toro-Domínguez D, Martorell-Marugán J, López-Domínguez R, García-Moreno A, González-Rumayor V, Alarcón-Riquelme ME, et al. ImaGEO: Integrative Gene Expression Meta-Analysis From GEO Database. Bioinformatics (2019) 35(5):880–2. doi: 10.1093/bioinformatics/bty721
44. Singh S, Toor JS, Sharma A, Arora SK. Signature Genes Associated With Immunological Non-Responsiveness to Anti-Retroviral Therapy in HIV-1 Subtype-C Infection. PloS One (2020) 15(6):e0234270. doi: 10.1371/journal.pone.0234270
45. Ghneim K, Sharma AA, Ribeiro SP, Fourati S, Ahlers J, Kulpa D, et al. Microbiome and Metabolome Driven Differentiation of TGF-β Producing Tregs Leads to Senescence and HIV Latency. bioRxiv (2020). Available at: https://www.biorxiv.org/content/10.1101/2020.12.15.422949v22. (Accessed November 1, 2021)
46. Nganou-Makamdop K, Douek DC. Manipulating the Interferon Signaling Pathway: Implications for HIV Infection. Virol Sin (2019) 34(2):192–6. doi: 10.1007/s12250-019-00085-5
47. Cheng L, Ma J, Li J, Li D, Li G, Li F, et al. Blocking Type I Interferon Signaling Enhances T Cell Recovery and Reduces HIV-1 Reservoirs. J Clin Invest (2017) 127(1):269–79. doi: 10.1172/jci90745
48. Zhen A, Rezek V, Youn C, Lam B, Chang N, Rick J, et al. Targeting Type I Interferon-Mediated Activation Restores Immune Function in Chronic HIV Infection. J Clin Invest (2017) 127(1):260–8. doi: 10.1172/jci89488
49. Watanabe D, Uehira T, Suzuki S, Matsumoto E, Ueji T, Hirota K, et al. Clinical Characteristics of HIV-1-Infected Patients With High Levels of Plasma Interferon-γ: A Multicenter Observational Study. BMC Infect Dis (2019) 19(1):11. doi: 10.1186/s12879-018-3643-2
50. Miailhes P, Maynard-Muet M, Lebossé F, Carrat F, Bouix C, Lascoux-Combe C, et al. Role of a 48-Week Pegylated Interferon Therapy in Hepatitis B E Antigen Positive HIV-Co-Infected Patients on cART Including Tenofovir: EMVIPEG Study. J Hepatol (2014) 61(4):761–9. doi: 10.1016/j.jhep.2014.05.030
51. Ingiliz P, Valantin MA, Thibault V, Duvivier C, Dominguez S, Katlama C, et al. Efficacy and Safety of Adefovir Dipivoxil Plus Pegylated Interferon-Alpha2a for the Treatment of Lamivudine-Resistant Hepatitis B Virus Infection in HIV-Infected Patients. Antivir Ther (2008) 13(7):895–900.
52. Utay NS, Douek DC. Interferons and HIV Infection: The Good, the Bad, and the Ugly. Pathog Immun (2016) 1(1):107–16. doi: 10.20411/pai.v1i1.125
53. Patro SC, Pal S, Bi Y, Lynn K, Mounzer KC, Kostman JR, et al. Shift in Monocyte Apoptosis With Increasing Viral Load and Change in Apoptosis-Related ISG/Bcl2 Family Gene Expression in Chronically HIV-1-Infected Subjects. J Virol (2015) 89(1):799–810. doi: 10.1128/jvi.02382-14
54. Wie SH, Du P, Luong TQ, Rought SE, Beliakova-Bethell N, Lozach J, et al. HIV Downregulates Interferon-Stimulated Genes in Primary Macrophages. J Interferon Cytokine Res (2013) 33(2):90–5. doi: 10.1089/jir.2012.0052
55. Ostrowski SR, Katzenstein TL, Thim PT, Pedersen BK, Gerstoft J, Ullum H. Low-Level Viremia and Proviral DNA Impede Immune Reconstitution in HIV-1-Infected Patients Receiving Highly Active Antiretroviral Therapy. J Infect Dis (2005) 191(3):348–57. doi: 10.1086/427340
56. Marchetti G, Gori A, Casabianca A, Magnani M, Franzetti F, Clerici M, et al. Comparative Analysis of T-Cell Turnover and Homeostatic Parameters in HIV-Infected Patients With Discordant Immune-Virological Responses to HAART. Aids (2006) 20(13):1727–36. doi: 10.1097/01.aids.0000242819.72839.db
57. Zhang LX, Song JW, Zhang C, Fan X, Huang HH, Xu RN, et al. Dynamics of HIV Reservoir Decay and Naïve CD4 T-Cell Recovery Between Immune Non-Responders and Complete Responders on Long-Term Antiretroviral Treatment. Clin Immunol (2021) 229:108773. doi: 10.1016/j.clim.2021.108773
58. Pino M, Pereira Ribeiro S, Pagliuzza A, Ghneim K, Khan A, Ryan E, et al. Increased Homeostatic Cytokines and Stability of HIV-Infected Memory CD4 T-Cells Identify Individuals With Suboptimal CD4 T-Cell Recovery on-ART. PloS Pathog (2021) 17(8):e1009825. doi: 10.1371/journal.ppat.1009825
59. Catalfamo M, Le Saout C, Lane HC. The Role of Cytokines in the Pathogenesis and Treatment of HIV Infection. Cytokine Growth Factor Rev (2012) 23(4-5):207–14. doi: 10.1016/j.cytogfr.2012.05.007
60. Vidya Vijayan KK, Karthigeyan KP, Tripathi SP, Hanna LE. Pathophysiology of CD4+ T-Cell Depletion in HIV-1 and HIV-2 Infections. Front Immunol (2017) 8:580. doi: 10.3389/fimmu.2017.00580
61. Trinité B, Ohlson EC, Voznesensky I, Rana SP, Chan CN, Mahajan S, et al. An HIV-1 Replication Pathway Utilizing Reverse Transcription Products That Fail to Integrate. J Virol (2013) 87(23):12701–20. doi: 10.1128/jvi.01939-13
62. Poon B, Grovit-Ferbas K, Stewart SA, Chen IS. Cell Cycle Arrest by Vpr in HIV-1 Virions and Insensitivity to Antiretroviral Agents. Science (1998) 281(5374):266–9. doi: 10.1126/science.281.5374.266
63. Agarwal N, Iyer D, Patel SG, Sekhar RV, Phillips TM, Schubert U, et al. HIV-1 Vpr Induces Adipose Dysfunction In Vivo Through Reciprocal Effects on PPAR/GR Co-Regulation. Sci Transl Med (2013) 5(213):213ra164. doi: 10.1126/scitranslmed.3007148
64. Azad AA. Could Nef and Vpr Proteins Contribute to Disease Progression by Promoting Depletion of Bystander Cells and Prolonged Survival of HIV-Infected Cells? Biochem Biophys Res Commun (2000) 267(3):677–85. doi: 10.1006/bbrc.1999.1708
65. Groux H, Torpier G, Monté D, Mouton Y, Capron A, Ameisen JC. Activation-Induced Death by Apoptosis in CD4+ T Cells From Human Immunodeficiency Virus-Infected Asymptomatic Individuals. J Exp Med (1992) 175(2):331–40. doi: 10.1084/jem.175.2.331
66. Meyaard L, Schuitemaker H, Miedema F. T-Cell Dysfunction in HIV Infection: Anergy Due to Defective Antigen-Presenting Cell Function? Immunol Today (1993) 14(4):161–4. doi: 10.1016/0167-5699(93)90279-t
67. Ward J, Davis Z, DeHart J, Zimmerman E, Bosque A, Brunetta E, et al. HIV-1 Vpr Triggers Natural Killer Cell-Mediated Lysis of Infected Cells Through Activation of the ATR-Mediated DNA Damage Response. PloS Pathog (2009) 5(10):e1000613. doi: 10.1371/journal.ppat.1000613
68. Richard J, Sindhu S, Pham TN, Belzile JP, Cohen EA. HIV-1 Vpr Up-Regulates Expression of Ligands for the Activating NKG2D Receptor and Promotes NK Cell-Mediated Killing. Blood (2010) 115(7):1354–63. doi: 10.1182/blood-2009-08-237370
69. Kogan M, Rappaport J. HIV-1 Accessory Protein Vpr: Relevance in the Pathogenesis of HIV and Potential for Therapeutic Intervention. Retrovirology (2011) 8:25. doi: 10.1186/1742-4690-8-25
70. Zahoor MA, Xue G, Sato H, Aida Y. Genome-Wide Transcriptional Profiling Reveals That HIV-1 Vpr Differentially Regulates Interferon-Stimulated Genes in Human Monocyte-Derived Dendritic Cells. Virus Res (2015) 208:156–63. doi: 10.1016/j.virusres.2015.06.017
71. Guadalupe M, Reay E, Sankaran S, Prindiville T, Flamm J, McNeil A, et al. Severe CD4+ T-Cell Depletion in Gut Lymphoid Tissue During Primary Human Immunodeficiency Virus Type 1 Infection and Substantial Delay in Restoration Following Highly Active Antiretroviral Therapy. J Virol (2003) 77(21):11708–17. doi: 10.1128/jvi.77.21.11708-11717.2003
72. Mattapallil JJ, Douek DC, Hill B, Nishimura Y, Martin M, Roederer M. Massive Infection and Loss of Memory CD4+ T Cells in Multiple Tissues During Acute SIV Infection. Nature (2005) 434(7037):1093–7. doi: 10.1038/nature03501
73. Yue Y, Wang N, Han Y, Zhu T, Xie J, Qiu Z, et al. A Higher CD4/CD8 Ratio Correlates With an Ultralow Cell-Associated HIV-1 DNA Level in Chronically Infected Patients on Antiretroviral Therapy: A Case Control Study. BMC Infect Dis (2017) 17(1):771. doi: 10.1186/s12879-017-2866-y
74. Luo L, Wang N, Yue Y, Han Y, Lv W, Liu Z, et al. The Effects of Antiretroviral Therapy Initiation Time on HIV Reservoir Size in Chinese Chronically HIV Infected Patients: A Prospective, Multi-Site Cohort Study. BMC Infect Dis (2019) 19(1):257. doi: 10.1186/s12879-019-3847-0
75. Lyu T, Yue Y, Hsieh E, Han Y, Zhu T, Song X, et al. HIV-1 CRF01_AE Subtype and HIV-1 DNA Level Among Patients With Chronic HIV-1 Infection: A Correlation Study. BMC Infect Dis (2020) 20(1):66. doi: 10.1186/s12879-020-4785-6
76. Yue Y, Li Y, Cui Y, Wang N, Huang Y, Cao W, et al. Therapeutic Prediction of HIV-1 DNA Decay: A Multicenter Longitudinal Cohort Study. BMC Infect Dis (2021) 21(1):592. doi: 10.1186/s12879-021-06267-5
77. Paiardini M, Müller-Trutwin M. HIV-Associated Chronic Immune Activation. Immunol Rev (2013) 254(1):78–101. doi: 10.1111/imr.12079
78. Monaco CL, Gootenberg DB, Zhao G, Handley SA, Ghebremichael MS, Lim ES, et al. Altered Virome and Bacterial Microbiome in Human Immunodeficiency Virus-Associated Acquired Immunodeficiency Syndrome. Cell Host Microbe (2016) 19(3):311–22. doi: 10.1016/j.chom.2016.02.011
79. Meyer-Myklestad MH, Medhus AW, Lorvik KB, Seljeflot I, Hansen SH, Holm K, et al. HIV-Infected Immunological Non-Responders Have Colon-Restricted Gut Mucosal Immune Dysfunction. J Infect Dis (2020) jiaa714. doi: 10.1093/infdis/jiaa714
80. Dillon SM, Guo K, Austin GL, Gianella S, Engen PA, Mutlu EA, et al. A Compartmentalized Type I Interferon Response in the Gut During Chronic HIV-1 Infection Is Associated With Immunopathogenesis. Aids (2018) 32(12):1599–611. doi: 10.1097/qad.0000000000001863
81. Pinacchio C, Scagnolari C, Iebba V, Santinelli L, Innocenti GP, Frasca F, et al. High Abundance of Genus Prevotella Is Associated With Dysregulation of IFN-I and T Cell Response in HIV-1-Infected Patients. Aids (2020) 34(10):1467–73. doi: 10.1097/qad.0000000000002574
Keywords: HIV, INR, CD4 recovery, IFI27, IFN response pathway
Citation: Liu X, Lin L, Lu L, Li X, Han Y, Qiu Z, Li X, Li Y, Song X, Cao W and Li T (2022) Comparative Transcriptional Analysis Identified Characteristic Genes and Patterns in HIV-Infected Immunological Non-Responders. Front. Immunol. 13:807890. doi: 10.3389/fimmu.2022.807890
Received: 02 November 2021; Accepted: 04 January 2022;
Published: 28 January 2022.
Edited by:
Shokrollah Elahi, University of Alberta, CanadaReviewed by:
Jean-Pierre Routy, McGill University, CanadaMartyn Andrew French, University of Western Australia, Australia
Copyright © 2022 Liu, Lin, Lu, Li, Han, Qiu, Li, Li, Song, Cao and Li. This is an open-access article distributed under the terms of the Creative Commons Attribution License (CC BY). The use, distribution or reproduction in other forums is permitted, provided the original author(s) and the copyright owner(s) are credited and that the original publication in this journal is cited, in accordance with accepted academic practice. No use, distribution or reproduction is permitted which does not comply with these terms.
*Correspondence: Taisheng Li, bGl0c2hAMjYzLm5ldA==