- 1Division of Molecular Regulation of Inflammatory and Immune Diseases, Research Institute for Biomedical Sciences, Tokyo University of Science, Chiba, Japan
- 2Department of Hygiene, Graduate School of Medicine, The University of Tokyo, Tokyo, Japan
CD8+ T cells are the key effector cells that contribute to the antitumor immune response. They comprise various T-cell clones with diverse antigen-specific T-cell receptors (TCRs). Thus, elucidating the overall antitumor responses of diverse T-cell clones is an emerging challenge in tumor immunology. With the recent advancement in next-generation DNA sequencers, comprehensive analysis of the collection of TCR genes (TCR repertoire analysis) is feasible and has been used to investigate the clonal responses of antitumor T cells. However, the immunopathological significance of TCR repertoire indices is still undefined. In this review, we introduce two approaches that facilitate an immunological interpretation of the TCR repertoire data: inter-organ clone tracking analysis and single-cell TCR sequencing. These approaches for TCR repertoire analysis will provide a more accurate understanding of the response of tumor-specific T cells in the tumor microenvironment.
Introduction
Each T cell has a unique antigen receptor (T-cell receptor; TCR). TCR is composed of alpha and beta chains, whose specificity is determined by the gene rearrangement that occurs in the thymus. T cells specifically recognize their cognate antigens via the TCRs and are activated. These activated T cells proliferate in the periphery, eliciting responses against the corresponding antigen, including cancer antigens. Conventional preclinical and clinical studies on antitumor T-cell responses have analyzed a limited number of tumor-specific T-cell clones using TCR transgenic mice (1) or peptide-major histocompatibility complex multimer technology (2). However, it is becoming increasingly clear that antitumor T-cell responses are driven by a wide variety of T-cell clones (3, 4).
The collection of TCRs in vivo, termed as the TCR repertoire, is considered to be a new indicator to evaluate T-cell responses based on antigen specificity. Recent advances in next-generation sequencing (NGS) technology have enabled TCR sequencing (TCRseq), which allows a comprehensive determination of the TCR sequences in individuals (5). TCR repertoire analysis is currently being used for studies in various medical fields, including infectious diseases (6), transplantation immunity (7), and tumor immunity (8). In this review, we provide an overview of the TCRseq methods, and summarize the findings and limitations of current TCR repertoire analysis in the field of cancer immunotherapy. Additionally, we introduce two novel approaches in TCR repertoire analysis that facilitate the immunological interpretation of TCR repertoire data: inter-organ clone tracking analysis, which identifies and analyzes T-cell clones present in the tumor and other tissues, and single-cell (sc) TCRseq, which identifies TCR sequences and their gene expression profiles at a single cell resolution.
Overview of TCR Sequencing Methods
TCRs are produced through rearrangement of the variable (V), diversity (D), joining (J), and constant (C) gene segments as well as insertions and deletions, resulting in a vast diversity (9). In TCRseq, the TCR gene, including complementary determining region 3 (CDR3), which is the most variable region in the TCR and a significant contributor to antigen specificity, is amplified and then sequenced by NGS. There are several TCRseq methods, which can be classified based on (i) whether genomic DNA (gDNA) or messenger RNA (mRNA) is used as a template, and (ii) whether multiple V region-specific primers are used to amplify the TCR sequence (multiplex PCR) or universal primers for the adapter sequence are used (5′ rapid amplification of cDNA ends [RACE]) (10) (Figure 1A). Because each method has its advantages and disadvantages (Table 1) (11, 12), it is preferable to adopt the most appropriate TCRseq method for a given research purpose based on these characteristics. In addition, the impact of each TCRseq method on the repertoire data should be considered when comparing TCR repertoire data obtained by different methods.
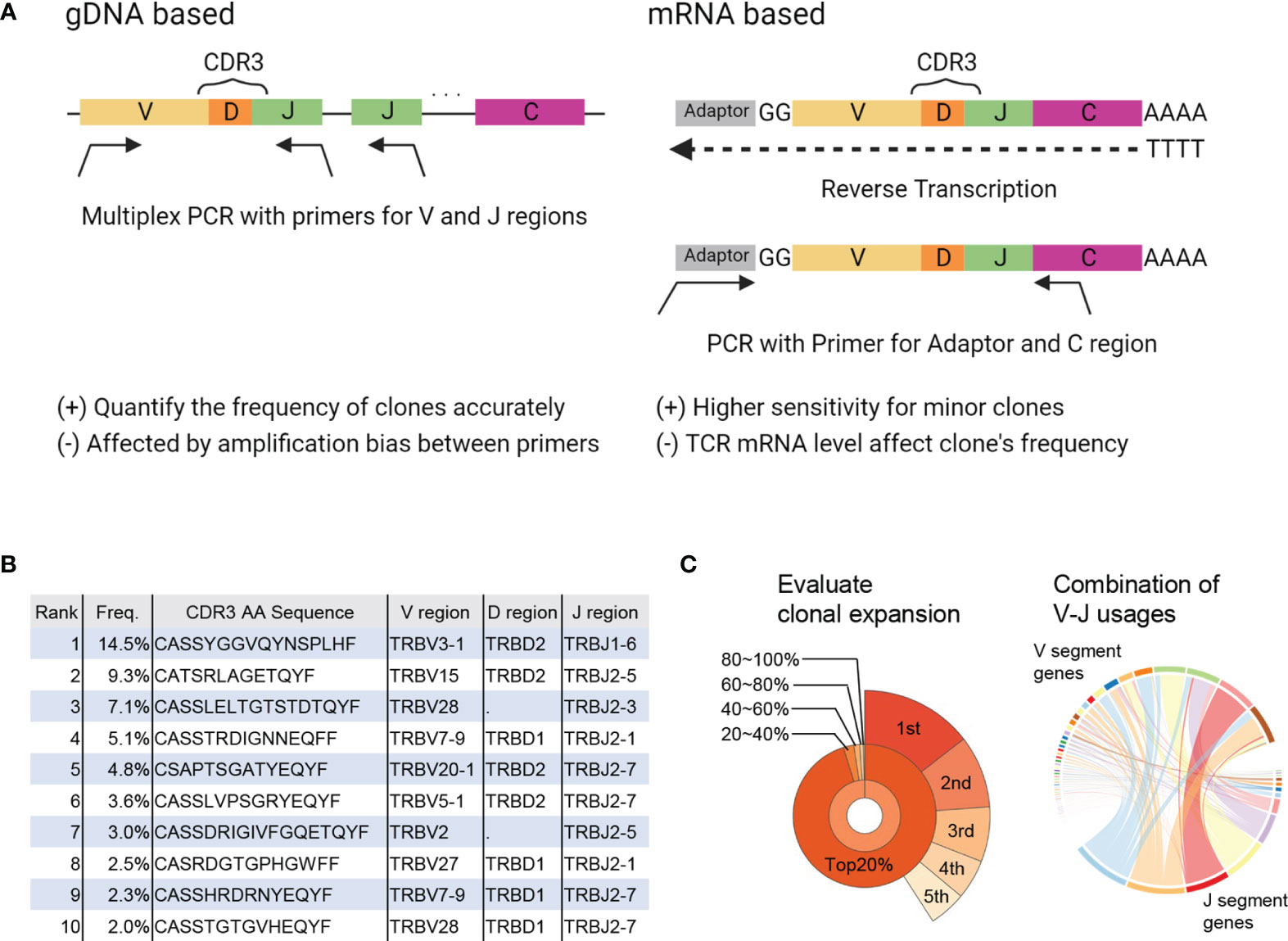
Figure 1 Summary of TCRseq and TCR repertoire analysis. (A) Exemplary workflow of TCR library preparation methods. Genomic (g) DNA-based methods use a primer set designed to cover all V or J regions. Multiplex PCR is used to amplify CDR3 generated by V(D)J recombination. In the messenger (m)RNA-based method, an adaptor sequence is attached to the 5′ end of mRNA in the reverse transcription reaction. Then, TCR cDNA containing CDR3 is amplified using primers targeting the C region and adaptor sequence. (B) Output of primary analysis: table of T-cell clones with their frequency, CDR3 sequence, and usage of V, D, and J gene segments. (C) Output of secondary analysis. (left) Pie chart of the frequencies of top1–5 clones (outer layer) and total frequencies of top20%, 20–40%, 40–60%, 60–80%, and <80% of clones (inner layer). (right) Circos plot representing the proportion of each combination of V-J segment usage. The widths of the ribbons connecting V (upper side) and J (lower side) segments correspond to the total frequency of clones with the given combination of V-J segment usage.
TCR sequences obtained by NGS are aligned to reference sequences of the V, D, and J regions and then assembled into T-cell clones that share the same CDR3 sequence. Algorithms, such as IgBLAST (13), IMGT/HighV-QUEST (14), MiXCR (15), and RTCR (16) are used to analyze TCR sequence data (Table 2). The results are presented in a table summarizing all detected T-cell clones and their frequencies (primary analysis, Figure 1B). Based on this clone table, the characteristics of TCR repertoires, including diversity (i.e., the number of T-cell clones present in the repertoire) and clonality (i.e., the extent to which specific T-cell clones are expanded in the repertoire), can be evaluated (secondary analysis, Figure 1C).
TCR Repertoire Analysis in Tumor Immunology: Achievements and Challenges
As cancer immunotherapies, such as anti-CTLA4 antibody and PD-1 inhibitor therapies, are applied to a wide range of cancer types, biomarkers that can predict treatment responders are urgently required (19). Treatment responses are reportedly associated with the level of PD-L1 expression in the tumor (20) and the mismatch repair deficiency (MMRd) of the tumor (21, 22), which are tumor-related factors. However, predictive biomarkers reflecting the patient’s immune status have not been established to date. TCR repertoire has been suggested as a candidate. The association between TCR repertoires and therapeutic effects in patients receiving cancer immunotherapy was reported around 2014, as summarized in Table 3. A clinical study on anti-PD-1 antibody therapy in melanoma patients showed that the clonality of the TCR repertoire in the tumor before treatment was higher in responders than in non-responders (23–26). Similarly, the total frequency of the top 1% clones in tumor repertoire was greater in responders than in non-responders in studies on neoadjuvant immunotherapy in patients with non-small cell lung cancer (NSCLC) (27, 28). Furthermore, responders showed more T-cell clones with increased frequency (23) and increased clonality of the T-cell repertoire (29) in the tumor after treatment. These results suggest that the clonality of the TCR repertoire in the tumor may reflect and predict the therapeutic effect of cancer immunotherapy. However, CD4+ and CD8+ tumor-infiltrating lymphocytes (TILs) were not separated prior to TCRseq in these studies. Considering that CD8+ TILs generally exhibit higher clonality than CD4+ (35, 36), the higher clonality of the total TIL repertoire may simply reflect a higher proportion of CD8+ cells (37).
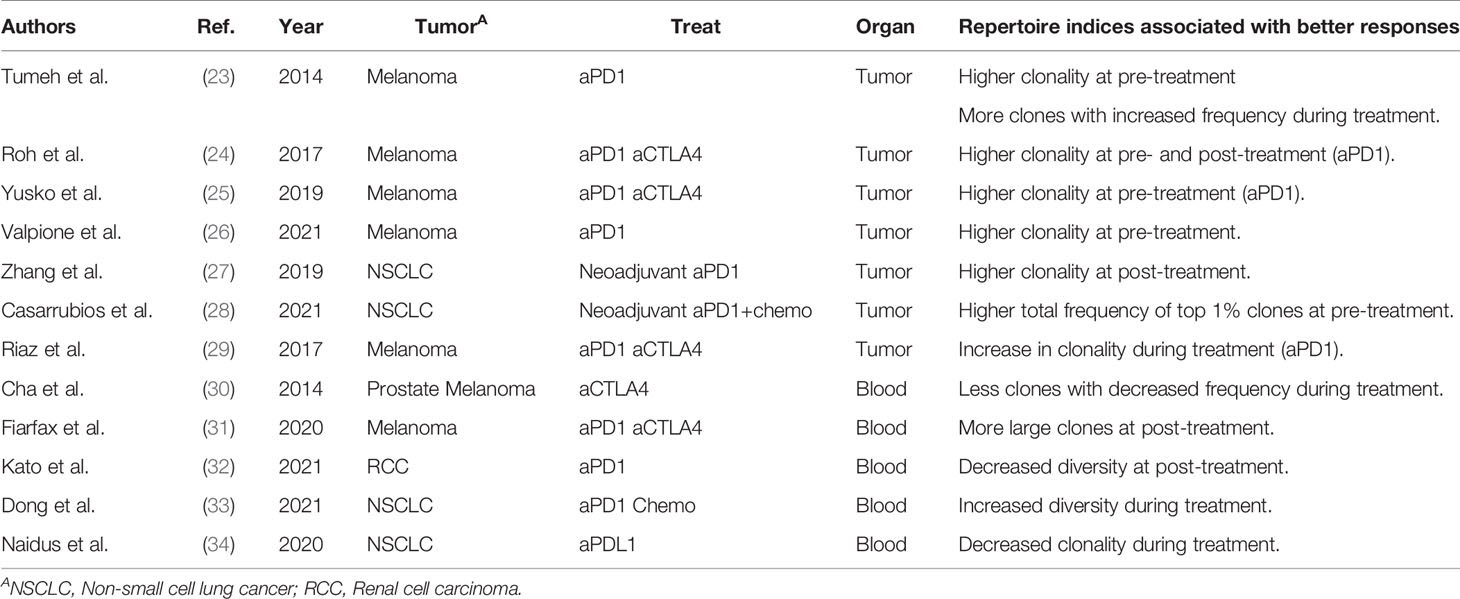
Table 3 Previous studies analyzing the association between repertoire clonality/diversity and clinical responses.
As blood sampling is less invasive than a tumor biopsy, peripheral blood samples are suitable for long-term immune monitoring of patients with cancer. Cha et al. reported that responders among prostate cancer and melanoma patients treated with anti-CTLA4 antibody had fewer contracted T-cell clones in the peripheral blood after treatment (30). Fairfax et al. found that responders among melanoma patients treated with anti-PD-1 antibody alone or in combination with anti-CTLA4 antibody had higher numbers of expanded CD8+ T-cell clones in their peripheral blood (31). A similar trend has been reported in a cohort of renal cell carcinoma patients treated with anti-PD-1 antibodies (32). In contrast, responders among NSCLC patients showed decreased clonality and increased diversity in peripheral blood TCR repertoires after treatment (33, 34). Therefore, there is currently no consensus on the characteristics of peripheral blood TCR repertoires associated with therapeutic responses. This is because the diversity and clonality of the TCR repertoire vary greatly among individuals and the peripheral blood comprises a large fraction of T-cell clones that are not related to the antitumor immune response.
Despite these issues, the diversity and clonality of the TCR repertoire are becoming recognized as factors associated with prognosis and/or therapeutic effects. However, the immunopathological significance of these TCR repertoire indices is still undefined because clonality or diversity as determined by TCR repertoire analysis does not consider the immunological features of individual T-cell clones. In other words, whether a clone is CD8+ or CD4+, tumor-reactive or bystander, effector or exhausted, is ignored, and only the frequency distribution of clones is analyzed. Therefore, we introduce two approaches in TCR repertoire analysis to support the immunological interpretation of TCR repertoire data. The first approach is inter-organ clone tracking analysis, in which T-cell clones in the tumor are tracked in the blood and/or draining lymph node (dLN). The second is single-cell (sc) TCRseq, in which gene expression profile is integrated into individual T-cell clones.
TCR Repertoire Analysis Based on Inter-Organ Overlapping Clones
Tumor-reactive T cells are activated and proliferate after antigen presentation in lymph nodes where cancer antigens enter (i.e., dLNs) and then infiltrate into the tumor tissue via the peripheral blood (Cancer Immunity Cycle: 38). Therefore, T-cell clones detected in both the tumor and the dLNs (dLN-tumor overlapping repertoire) represent tumor-reactive clones that are mobilized for the antitumor T-cell response (Figure 2A). Thus, an increase in overlapping clones is considered to be associated with enhanced priming of tumor-reactive clones and stronger antitumor effects (Figure 2B). In the peripheral blood, there exist many T-cell clones that are unrelated to the antitumor response. Hence, the TCR repertoire in the peripheral blood that overlaps with that in the tumor (peripheral blood-tumor overlapping repertoire) is expected to be enriched in tumor-reactive T-cell clones. Therefore, the blood-tumor overlapping repertoire may be useful for the monitoring of antitumor T-cell responses. For example, patients with a higher blood-tumor overlap in the blood at baseline and an increase in the blood-tumor overlap following treatment may exhibit a better antitumor response (Figure 2C). Moreover, blood-tumor overlapping clones in the unfractionated tumor T-cell repertoire can be annotated as CD4+ or CD8+ based on whether overlapping clones are detected in the blood CD4+ or CD8+ T-cell repertoire. Given that isolating sufficient numbers of CD4+ and CD8+ T cells from tumor biopsy is technically difficult and laborious, the ability to analyze the responses of CD4+ and CD8+ T-cell clones in the tumor without the need for preparing CD4+ and CD8+ TILs is another advantage of blood-tumor overlapping repertoire analysis.
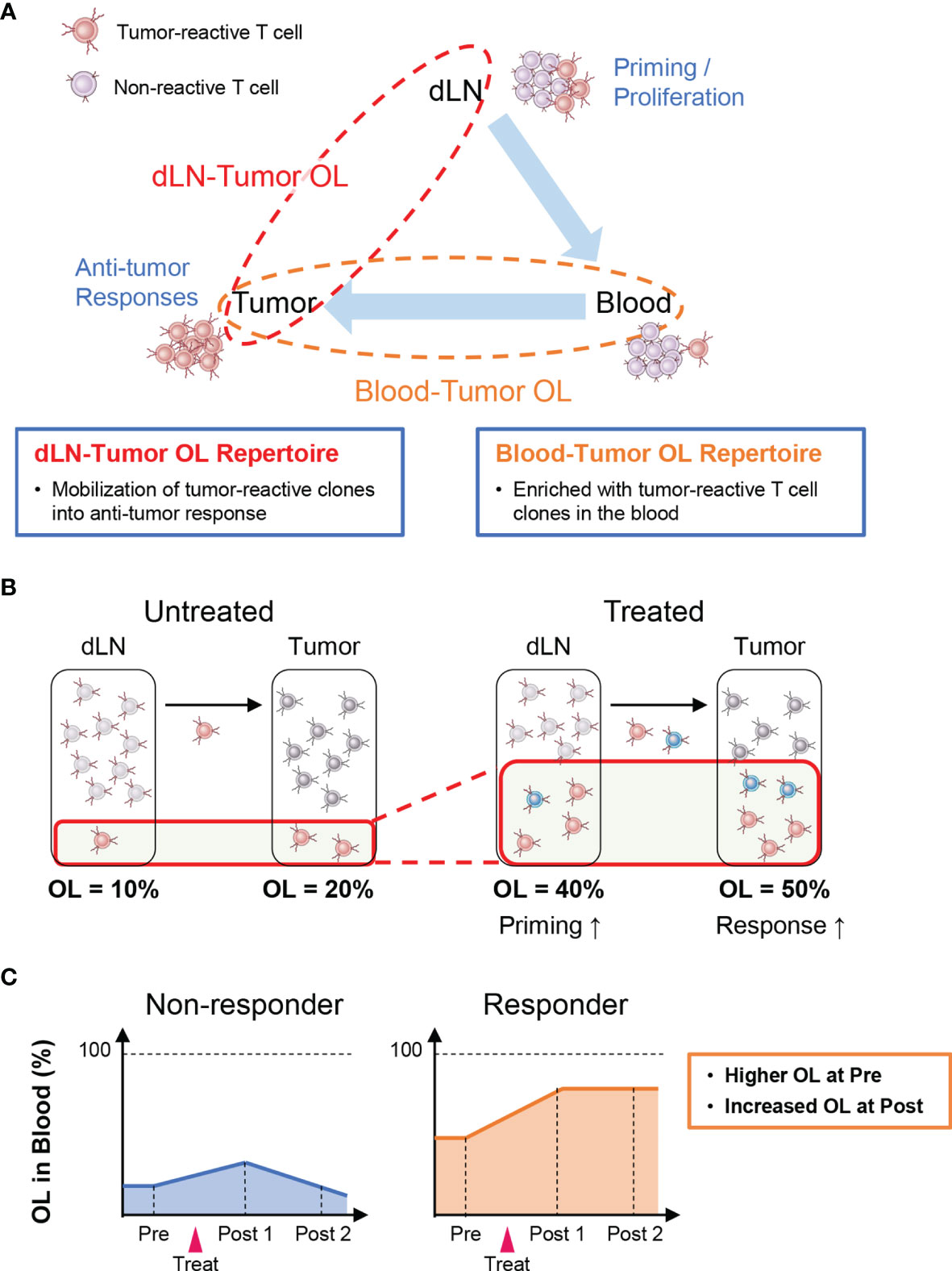
Figure 2 Outline of inter-organ clone tracking analysis. (A) Tumor-reactive T cells are activated and proliferate after antigen presentation in tumor-draining lymph nodes (dLNs) and then infiltrate into the tumor tissue via the blood circulation. Therefore, the dLN-tumor overlapping (OL) repertoire reflects a novel mobilization of tumor-reactive clones into the antitumor T-cell response. In addition, the blood-tumor OL repertoire is expected to be enriched in tumor-reactive T-cell clones. (B) Overview of dLN-tumor overlapping repertoire analysis. An increase in overlapping clones is associated with enhanced priming of tumor-reactive clones and stronger antitumor effects. (C) Overview of blood-tumor OL repertoire analysis. Patients with a higher blood-tumor overlap in the blood at baseline and an increase in blood-tumor overlap following treatment show a better antitumor response.
dLN-Tumor Overlapping Repertoire Analysis
A few studies have performed dLN-tumor overlapping analysis on samples surgically resected from patients with cancer (Table 4). Inamori et al. reported that patients with MMRd colorectal cancer (CRC), for which ICIs are more effective, exhibited a greater extent of dLN-tumor overlap than those with MMR proficient CRC (39). This result is consistent with that of MMRd tumors harboring tumor-reactive T-cell clones specific to mutation-associated neoantigens (22). Considering this result, other tumor types that exhibit greater dLN-tumor overlap may also be responsive to ICIs. However, it is noted that metastasis-positive dLN showed a significantly higher extent of dLN-tumor overlap (40, 41). Thus, the degree of overlap between metastasis-positive dLN and the tumor may not necessarily reflect the enhanced priming of tumor-reactive clones.
In a preclinical study, we demonstrated the increased dLN-tumor overlapping repertoire in B16-bearing mice receiving an anti-CD4 depleting antibody treatment (42). Anti-CD4 depleting antibody exhibits a potent anti-tumor effect by transiently removing CD4+ immunosuppressive cells, including regulatory T cells, and activating tumor-reactive CD8+ T cells (47). While the clonality of CD8+ T cells in the tumor was equivalent between the untreated and anti-CD4-treated mice, the total frequency and diversity of the dLN-tumor overlapping repertoire were significantly increased in the anti-CD4-treated mice. In addition, the expansion of adoptively transferred or endogenous melanoma-reactive clones was detected in the dLN-tumor overlapping repertoire (42). These results support that treatment-induced changes in TCR repertoire are enriched in dLN-tumor overlap. Moreover, the dLN-tumor overlapping clones could be classified into three patterns: “TumorMajor clones”, which were dominant (>0.1%) in the tumor, but not in the dLNs; “dLNMajor clones”, which were dominant in the dLNs, but not in the tumor; and “DoubleMajor clones”, which were dominant in both the dLNs and the tumor (42). Anti-CD4 treatment increased the dLNMajor and DoubleMajor clones, which strengthens the evidence that the primary target of anti-CD4 antibody in the cancer-immunity cycle is priming the tumor-reactive T cells in dLN. The anti-CD4 antibody produced a synergistic anti-tumor effect in combination with PD1 blockade in a preclinical model (47). Considering that the primary target of PD1 blockade is preventing tumor-reactive T cells from exhaustion in the tumor (38), other treatments that increase dLN-tumor overlap may also work synergistically with PD1 blockade by targeting dLN and mobilize more tumor-reactive clones.
Blood-Tumor Overlapping Repertoire Analysis
A number of studies reported the association between blood-tumor overlapping repertoire and clinical responses (Table 4). Chow et al. reported that TumorMajor clones in blood-tumor overlap expanded in the blood within two weeks after initiating stereotactic body radiation therapy (43). In addition, responders for PD-1 blockade therapy showed a higher total frequency of blood-tumor overlapping clones in the blood before treatment (44), a greater number of blood-tumor overlapping clones after treatment (32), and more pronounced expansion of blood-tumor overlapping clones in the blood after treatment (45). Similar observations were reported for neoadjuvant chemoimmunotherapy (28) and clinical trial of a humanized anti-CD4 antibody (46, 48). The association between the blood-tumor overlapping repertoire in the tumor and clinical responses has also been analyzed. Zhang et al. reported that, in a trial of neoadjuvant PD-1 blockade therapy for NSCLC, the tumor T-cell repertoire of responders contained a higher proportion of clones that expanded in the blood after treatment than that of non-responders (27). Moreover, Yost et al. showed that T-cell clones in the tumor were replaced during treatment and that some of the newly emerged clones in the tumor were also present in the peripheral blood before treatment (49).
These observations suggest that PD-1 blockade therapy activates novel tumor-reactive T-cell clones outside the tumor and promotes their infiltration into the tumor (50). This “tumor-extrinsic” response may coincide with the reactivation of exhausted T cells in the tumor, which has been previously considered the central mechanism of PD-1 blockade. In addition, scTCR analysis of tumors and peripheral blood (see below) has shown that genes related to effector function are highly expressed in tumor-overlapping clones in the peripheral blood (51, 52). These results suggested the potential of tumor-overlapping clones in the peripheral blood as a prognostic and early diagnostic marker for PD-1 blockade therapy: patients with more tumor-overlapping clones may benefit from PD-1 blockade, while those with less tumor-overlapping clones may need more aggressive immunotherapy like a combination with anti-CTLA4 antibody. Moreover, whether these overlapping clones indeed recognize tumor cells and contribute to the antitumor effect will require verification in preclinical and clinical studies.
Overview of Single-Cell TCR Sequencing Methods
In the TCRseq methods described above, the total DNA or RNA in a T-cell population is pooled for library preparation (bulk TCRseq). Therefore, it is impossible to associate a gene expression profile with a particular clone, which poses a major hurdle to understanding the function of T-cell clones in TCR repertoire analysis.
scTCRseq is a potent approach to assigning immunological phenotypes to individual T-cell clones (11). scTCRseq identifies paired TCR alpha and beta sequences of thousands of T cells in parallel using unique DNA barcodes for individual cells (cellular barcodes) (53, 54). Single-cell gene expression profiles can be obtained simultaneously (scRNAseq), and the scRNAseq and scTCRseq results can be integrated using cellular barcodes. By extracting T cells with a particular TCR sequence using the cellular barcode and analyzing the gene expression profile, the phenotypes of individual T-cell clones (including naïve, effector, memory, and exhausted T cells) can be evaluated.
With the advancements in single-cell analysis, more high-throughput scTCRseq methods are being developed, which can be roughly divided into two types: (1) methods reconstructing TCR sequences by extracting TCR reads from standard scRNAseq data and (2) those amplifying TCR genes selectively from the scRNAseq library and sequencing both the TCR gene and scRNAseq libraries. Experimental overview and characteristics of these two categories of scTCRseq methods are summarized in Figure 3 and Tables 2, 5 (17, 18, 55, 56).
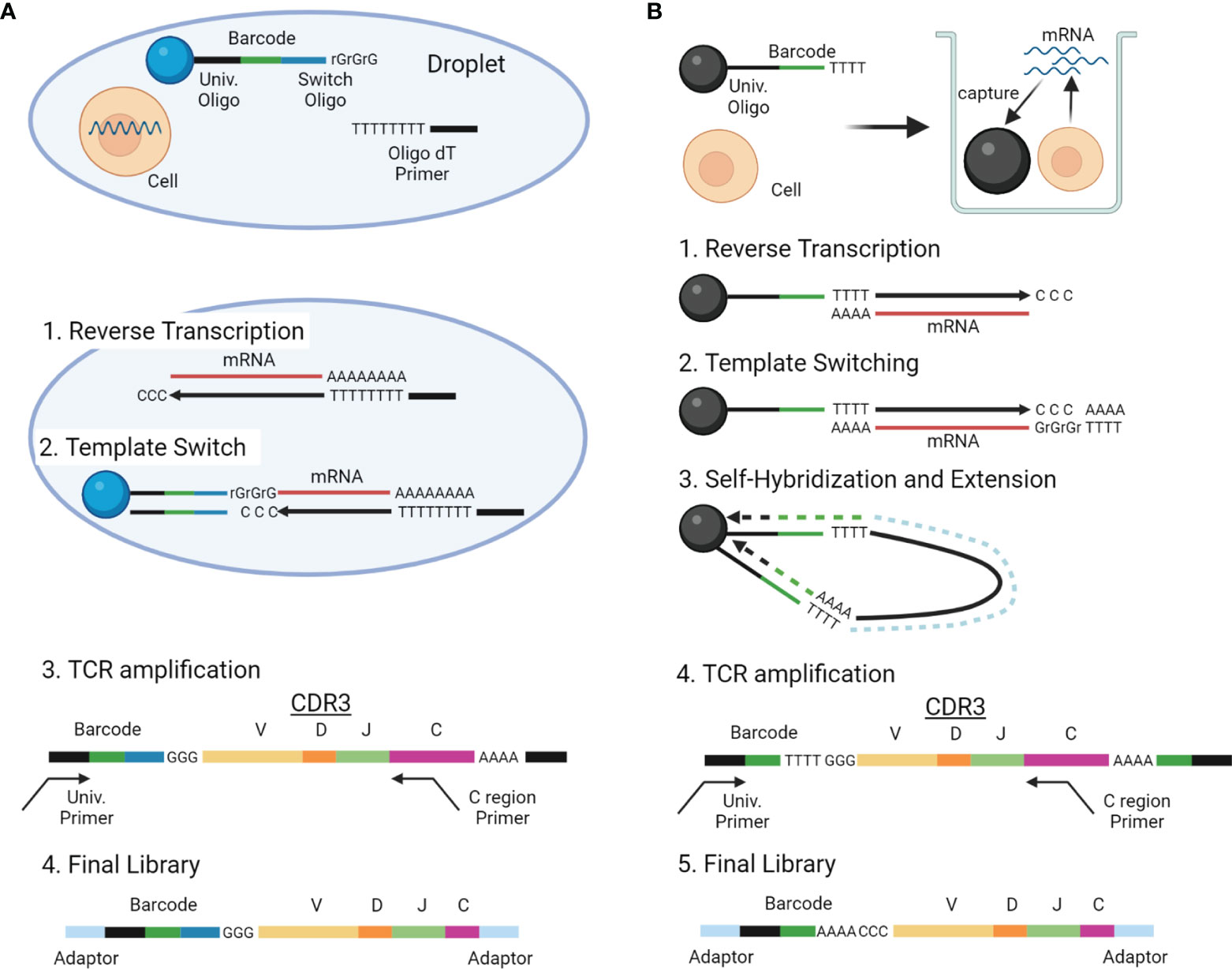
Figure 3 Overview of scTCRseq methods. (A) Overview of the scTCRseq method using 5′ RNA amplification. Cells are isolated in oil-in-water droplets. After cell lysis, mRNAs from a single cell are reverse-transcribed using oligo-dT primers. An adapter containing a cellular barcode and a universal oligo is added at the 5′ end of cDNA by template switching. TCR transcripts containing CDR3 and the cellular barcode are specifically amplified using primers designed to target the TCR C region and the universal oligo. The amplified TCRs are sequenced using NGS. (B) Overview of the scTCRseq method using self-hybridization. Single cells and beads with cellular barcodes are loaded into micro-wells. After cell lysis, mRNAs from the single cells are captured by the oligo-dT sequences on the beads. After reverse transcription, a synthetic poly-A tail is added to the 3′ end of the cDNA by template switching. Through denaturation and annealing, the synthetic poly-A tail at the 3′ end of the cDNA hybridizes to the oligo-dT sequence on its own bead. The second strand of cDNA is then stretched by DNA polymerase to copy the cellular barcode of the bead. TCR transcripts containing CDR3 and a cellular barcode are specifically amplified using primers designed to target the TCR C region and the universal oligo. The amplified TCRs are sequenced using NGS.
Application of scTCR Analysis to Elucidating T-Cell Responses in the Tumor Microenvironment
One of the applications of scTCRseq in tumor immunology is estimating the developmental relationships among T-cell subsets based on the T-cell clone overlap. scRNAseq has identified several clusters of tumor-infiltrating CD8+ T cells (36, 57–61), including a cytotoxic cluster that expresses high levels of perforin and granzyme, an exhausted cluster that is highly tumor-responsive, but expresses high levels of inhibitory receptors, and a tissue-resident memory cluster that expresses integrin alpha E. The exhausted cluster is further classified into a terminally exhausted cluster, which expresses high levels of inhibitory receptors, and a progenitor exhausted cluster, which expresses molecules related to self-renewal capacity, such as TCF1. However, the developmental relationship between these T-cell clusters has not been clarified.
The differentiation trajectory of TILs can be estimated by quantifying the overlapping T-cell clones among the clusters. T cells within a particular TCR clone are derived from a single naïve T cell. Thus, the presence of multiple overlapping clones between two clusters suggests that they are closely related in terms of differentiation trajectory. For example, Li et al. found a considerable overlap of T-cell clones between the late and early exhaustion clusters of CD8+ TILs in melanoma, whereas there was limited clonal overlap between the cytotoxic cluster and these exhaustion clusters (57). This finding indicated that the exhaustion and cytotoxic clusters have different differentiation trajectories. In addition, TCR overlap analysis in NSCLC suggested that terminally exhausted CD8+ T cells in tumors had two origins: GZMK+ circulating precursors infiltrating from the peripheral blood and XCL1+ resident precursors showing tissue-resident characteristics (35, 62). Inter-cluster TCR overlap analysis will be a useful method for inferring the differentiation trajectories of T cells in silico in addition to trajectory analysis based on gene expression. However, the number of T-cell clones that can be analyzed by scTCRseq is limited. Therefore, a higher throughput method, such as bulk TCRseq of specific T-cell subsets, will be required to validate scTCRseq results in the future.
Another application of scTCRseq is the identification of TCRs that respond to tumor antigens. Tumor-infiltrating T cells include not only tumor-reactive T-cell clones that recognize tumor antigens, but also clones that recognize viral antigens unrelated to tumors (63, 64). Since the antigen specificity of a T-cell clone is determined by the TCR alpha and beta chains, scTCRseq identifying the TCR alpha and beta chain pair is required to reconstitute TCRs of T-cell clones and examine their tumor-reactivity. Oliveira et al. identified TCR alpha- and beta-chain pairs of CD8+ TILs by scTCRseq and introduced them into peripheral blood T cells using lentiviral vectors. The antigen specificity of the TCRs was identified by coculturing the TCR-transfected T cells with immortalized lymphoblastoid cell lines pulsed with peptides of tumor antigens or tumor-unrelated viral antigens. In addition, the gene expression profiles of the T-cell clones were determined by scRNAseq. Using this approach, the authors found that the clones recognizing tumor antigens showed an exhaustion phenotype, whereas the bystander clones recognizing viral antigens showed a memory-like phenotype (65). Tumor-reactive TCRs identified by scTCRseq as above can be applied to tailor-made adoptive T-cell therapy. A recent study demonstrated that only a small percentage of TILs can be cultured ex vivo (66). Considering this, direct identification of functional T-cell clones using scTCRseq will expand the choice of T-cell clones for T-cell therapy, which will lead to improved therapeutic outcomes.
Conclusion
The repertoire of tumor-infiltrating T cells, which reflects the antigen-specific T-cell-mediated anti-tumor responses, is an important characteristic feature of the tumor microenvironment. In this review, we introduce two novel approaches for immunological interpretation of the TCR repertoire. Inter-organ clone tracking analysis based on bulk TCRseq can enrich tumor-reactive T-cell clones that are mobilized into the cancer-immunity cycle, providing a more direct index of the antitumor T-cell response than repertoire diversity or clonality. scTCRseq technology enables linking individual T-cell clones with their gene expression profiles, allowing an improved immunological interpretation of the TCR repertoire. Moreover, high-throughput identification of antigen-specific T cells is becoming possible by combining scTCRseq and DNA-barcoded peptide-MHC multimer technology (56, 67, 68). While scTCRseq provides a more precise characterization of individual clones, the number of T-cell clones that can be analyzed per sample is only the “tip of the iceberg” of the TCR repertoire. Thus, combined application of high-throughput bulk repertoire overlap analysis and scTCRseq will improve our understanding of the antitumor responses of diverse T-cell clones. Overall, the approaches for TCR repertoire analysis described in this review will not only provide a more precise characterization of the tumor microenvironment, but also help develop efficient immunotherapeutic strategies to combat cancer.
Author Contributions
HA and SU wrote the initial draft of manuscript. All authors contributed to the article and approved the submitted version.
Funding
This work was supported by the Japan Society for the Promotion of Science under Grant Number 20281832 and 17929397, and by the Japan Agency for Medical Research and Development (AMED) under Grant Number JP 21gm6210025 and JP21fk0210049.
Conflict of Interest
HA reports stock for ImmunoGeneTeqs, Inc. SU reports advisory role for ImmunoGeneTeqs, Inc; stock for ImmunoGeneTeqs, Inc, IDAC Theranostics, Inc. SS reports advisory role for ImmunoGeneTeqs, Inc; stock for ImmunoGeneTeqs, Inc, KM reports consulting or advisory role for Kyowa-Hakko Kirin, ImmunoGeneTeqs, Inc; research funding from Kyowa-Hakko Kirin, and Ono; stock for ImmunoGeneTeqs, Inc, IDAC Theranostics, Inc.
Publisher’s Note
All claims expressed in this article are solely those of the authors and do not necessarily represent those of their affiliated organizations, or those of the publisher, the editors and the reviewers. Any product that may be evaluated in this article, or claim that may be made by its manufacturer, is not guaranteed or endorsed by the publisher.
Acknowledgments
HA was supported by the Tadamitsu Kishimoto Fellowship Program. We would like to thank Editage (www.editage.com) for English language editing. Figures 1A and 3 were created with BioRender.com.
References
1. Overwijk WW, Theoret MR, Finkelstein SE, Surman DR, De Jong LA, Vyth-Dreese FA, et al. Tumor Regression and Autoimmunity After Reversal of a Functionally Tolerant State of Self-Reactive CD8+ T Cells. J Exp Med (2003) 198:569–80. doi: 10.1084/jem.20030590
2. Ahmadzadeh M, Johnson LA, Heemskerk B, Wunderlich JR, Dudley ME, White DE, et al. Tumor Antigen-Specific CD8 T Cells Infiltrating the Tumor Express High Levels of PD-1 and Are Functionally Impaired. Blood (2009) 114:1537–44. doi: 10.1182/blood-2008-12-195792
3. Pasetto A, Gros A, Robbins PF, Deniger DC, Prickett TD, Matus-Nicodemos R, et al. Tumor- and Neoantigen-Reactive T-Cell Receptors Can Be Identified Based on Their Frequency in Fresh Tumor. Cancer Immunol Res (2016) 4:734–43. doi: 10.1158/2326-6066.CIR-16-0001
4. Gros A, Robbins PF, Yao X, Li YF, Turcotte S, Tran E, et al. PD-1 Identifies the Patient-Specific CD8+ Tumor-Reactive Repertoire Infiltrating Human Tumors. J Clin Invest (2014) 124:2246–59. doi: 10.1172/JCI73639
5. Schrama D, Ritter C, Becker JC. T Cell Receptor Repertoire Usage in Cancer as a Surrogate Marker for Immune Responses. Semin Immunopathol (2017) 39:255–68. doi: 10.1007/s00281-016-0614-9
6. DeWitt WS, Emerson RO, Lindau P, Vignali M, Snyder TM, Desmarais C, et al. Dynamics of the Cytotoxic T Cell Response to a Model of Acute Viral Infection. J Virol (2015) 89:4517–26. doi: 10.1128/JVI.03474-14
7. Whangbo JS, Kim HT, Nikiforow S, Koreth J, Alho AC, Falahee B, et al. Functional Analysis of Clinical Response to Low-Dose IL-2 in Patients With Refractory Chronic Graft-Versus-Host Disease. Blood Adv (2019) 3:984–94. doi: 10.1182/bloodadvances.2018027474
8. Aversa I, Malanga D, Fiume G, Palmieri C. Molecular T-Cell Repertoire Analysis as Source of Prognostic and Predictive Biomarkers for Checkpoint Blockade Immunotherapy. Int J Mol Sci (2020) 21(7):2378. doi: 10.3390/ijms21072378
9. Davis MM, Bjorkman PJ. The T Cell Receptor Genes and T-Cell Recognition. Nature (1988) 334:395–402. doi: 10.1038/334395a0
10. Rosati E, Dowds CM, Liaskou E, Henriksen EKK, Karlsen TH, Franke A. Overview of Methodologies for T-Cell Receptor Repertoire Analysis. BMC Biotechnol (2017) 17:1–16. doi: 10.1186/s12896-017-0379-9
11. Pai JA, Satpathy AT. High-Throughput and Single-Cell T Cell Receptor Sequencing Technologies. Nat Methods (2021) 18:881–92. doi: 10.1038/s41592-021-01201-8
12. Barennes P, Quiniou V, Shugay M, Egorov ES, Davydov AN, Chudakov DM, et al. Benchmarking of T Cell Receptor Repertoire Profiling Methods Reveals Large Systematic Biases. Nat Biotechnol (2021) 39:236–45. doi: 10.1038/s41587-020-0656-3
13. Ye J, Ma N, Madden TL, Ostell JM. IgBLAST: An Immunoglobulin Variable Domain Sequence Analysis Tool. Nucleic Acids Res (2013) 41:34–40. doi: 10.1093/nar/gkt382
14. Alamyar E, Duroux P, Lefranc M, Giudicelli V. IMGT ® Tools for the Nucleotide Analysis of Immunoglobulin (IG) and T Cell Receptor (TR) V-(D)-J Repertoires, Polymorphisms, and IG Mutations: IMGT/V-QUEST and IMGT/HighV-QUEST for NGS. In: Christiansen FT, Tait BD, editors. Immunogenetics: Methods and Applications in Clinical Practice. Methods in Molecular Biology, vol. 882 . Totowa, NJ: Humana Press (2012). p. 569–604.
15. Bolotin DA, Poslavsky S, Mitrophanov I, Shugay M, Mamedov IZ, Putintseva EV, et al. MiXCR: Software for Comprehensive Adaptive Immunity Profiling. Nat Methods (2015) 12:380–1. doi: 10.1038/nmeth.3364
16. Gerritsen B, Pandit A, Andeweg AC, De Boer RJ. RTCR: A Pipeline for Complete and Accurate Recovery of T Cell Repertoires From High Throughput Sequencing Data. Bioinformatics (2016) 32:3098–106. doi: 10.1093/bioinformatics/btw339
17. Stubbington MJT, Lönnberg T, Proserpio V, Clare S, Speak AO, Dougan G, et al. T Cell Fate and Clonality Inference From Single-Cell Transcriptomes. Nat Methods (2016) 13:329–32. doi: 10.1038/nmeth.3800
18. Rizzetto S, Koppstein DNP, Samir J, Singh M, Reed JH, Cai CH, et al. B-Cell Receptor Reconstruction From Single-Cell RNA-Seq With VDJPuzzle. Bioinformatics (2018) 34:2846–7. doi: 10.1093/bioinformatics/bty203
19. Havel JJ, Chowell D, Chan TA. The Evolving Landscape of Biomarkers for Checkpoint Inhibitor Immunotherapy. Nat Rev Cancer (2019) 19:133–50. doi: 10.1038/s41568-019-0116-x
20. Remon J, Besse B, Soria JC. Successes and Failures: What did We Learn From Recent First-Line Treatment Immunotherapy Trials in Non-Small Cell Lung Cancer? BMC Med (2017) 15:1–7. doi: 10.1186/s12916-017-0819-3
21. Le DT, Uram JN, Wang H, Bartlett BR, Kemberling H, Eyring AD, et al. PD-1 Blockade in Tumors With Mismatch-Repair Deficiency. N Engl J Med (2015) 372:2509–20. doi: 10.1056/NEJMoa1500596
22. Le DT, Durham JN, Smith KN, Wang H, Bartlett BR, Aulakh LK, et al. Mismatch Repair Deficiency Predicts Response of Solid Tumors to PD-1 Blockade. Science (2017) 357:409–13. doi: 10.1126/science.aan6733
23. Tumeh PC, Harview CL, Yearley JH, Shintaku IP, Taylor EJM, Robert L, et al. PD-1 Blockade Induces Responses by Inhibiting Adaptive Immune Resistance. Nature (2014) 515:568–71. doi: 10.1038/nature13954
24. Roh W, Chen P, Reuben A, Spencer CN, Peter A, Miller JP, et al. Integrated Molecular Analysis of Tumor Biopsies on Sequential CTLA-4 and PD-1 Blockade Reveals Markers of Response and Resistance. Sci Transl Med (2017) 9:1–24. doi: 10.1126/scitranslmed.aah3560.Integrated
25. Yusko E, Vignali M, Wilson RK, Mardis ER, Hodi FS, Horak C, et al. Association of Tumor Microenvironment T-Cell Repertoire and Mutational Load With Clinical Outcome After Sequential Checkpoint Blockade in Melanoma. Cancer Immunol Res (2019) 7:458–65. doi: 10.1158/2326-6066.cir-18-0226
26. Valpione S, Mundra PA, Galvani E, Campana LG, Lorigan P, De Rosa F, et al. The T Cell Receptor Repertoire of Tumor Infiltrating T Cells Is Predictive and Prognostic for Cancer Survival. Nat Commun (2021) 12:4098. doi: 10.1038/s41467-021-24343-x
27. Zhang J, Ji Z, Caushi JX, El Asmar M, Anagnostou V, Cottrell TR, et al. Compartmental Analysis of T-Cell Clonal Dynamics as a Function of Pathologic Response to Neoadjuvant PD-1 Blockade in Resectable Non-Small Cell Lung Cancer. Clin Cancer Res (2020) 26:1327–37. doi: 10.1158/1078-0432.CCR-19-2931
28. Casarrubios M, Cruz-Bermúdez A, Nadal E, Insa A, García Campelo M del R, Lázaro M, et al. Pretreatment Tissue TCR Repertoire Evenness Is Associated With Complete Pathologic Response in Patients With NSCLC Receiving Neoadjuvant Chemoimmunotherapy. Clin Cancer Res (2021) 27(21):5878–90. doi: 10.1158/1078-0432.ccr-21-1200
29. Riaz N, Havel JJ, Makarov V, Desrichard A, Urba WJ, Sims JS, et al. Tumor and Microenvironment Evolution During Immunotherapy With Nivolumab. Cell (2017) 171:934–49.e15. doi: 10.1016/j.cell.2017.09.028
30. Cha E, Klinger M, Hou Y, Cummings C, Ribas A, Faham M, et al. Improved Survival With T Cell Clonotype Stability After Anti-CTLA-4 Treatment in Cancer Patients. Sci Transl Med (2014) 6:238ra70. doi: 10.1126/scitranslmed.3008211
31. Fairfax BP, Taylor CA, Watson RA, Nassiri I, Danielli S, Fang H, et al. Peripheral CD8+ T Cell Characteristics Associated With Durable Responses to Immune Checkpoint Blockade in Patients With Metastatic Melanoma. Nat Med (2020) 26:193–9. doi: 10.1038/s41591-019-0734-6
32. Kato T, Kiyotani K, Tomiyama E, Koh Y, Matsushita M, Hayashi Y, et al. Peripheral T Cell Receptor Repertoire Features Predict Durable Responses to Anti-PD-1 Inhibitor Monotherapy in Advanced Renal Cell Carcinoma. Oncoimmunology (2021) 10(1):1862948. doi: 10.1080/2162402X.2020.1862948
33. Dong N, Moreno-Manuel A, Calabuig-Fariñas S, Gallach S, Zhang F, Blasco A, et al. Characterization of Circulating T Cell Receptor Repertoire Provides Information About Clinical Outcome After Pd-1 Blockade in Advanced Non-Small Cell Lung Cancer Patients. Cancers (Basel) (2021) 13:2950. doi: 10.3390/cancers13122950
34. Naidus E, Bouquet J, Oh DY, Looney TJ, Yang H, Fong L, et al. Early Changes in the Circulating T Cells Are Associated With Clinical Outcomes After PD-L1 Blockade by Durvalumab in Advanced NSCLC Patients. Cancer Immunol Immunother (2021) 70:2095–102. doi: 10.1007/s00262-020-02833-z
35. Gueguen P, Metoikidou C, Dupic T, Lawand M, Goudot C, Baulande S, et al. Contribution of Resident and Circulating Precursors to Tumor-Infiltrating CD8+ T Cell Populations in Lung Cancer. Sci Immunol (2021) 6(55):eabd5778. doi: 10.1126/SCIIMMUNOL.ABD5778
36. Zhang L, Yu X, Zheng L, Zhang Y, Li Y, Fang Q, et al. Lineage Tracking Reveals Dynamic Relationships of T Cells in Colorectal Cancer. Nature (2018) 564:268–72. doi: 10.1038/s41586-018-0694-x
37. Rudqvist NP, Pilones KA, Lhuillier C, Wennerberg E, Sidhom JW, Emerson RO, et al. Radiotherapy and CTLA-4 Blockade Shape the Tcr Repertoire of Tumor-Infiltrating T Cells. Cancer Immunol Res (2018) 6:139–50. doi: 10.1158/2326-6066.CIR-17-0134
38. Chen DS, Mellman I. Oncology Meets Immunology: The Cancer-Immunity Cycle. Immunity (2013) 39:1–10. doi: 10.1016/j.immuni.2013.07.012
39. Inamori K, Togashi Y, Fukuoka S, Akagi K, Ogasawara K, Irie T, et al. Importance of Lymph Node Immune Responses in MSI-H/dMMR Colorectal Cancer. JCI Insight (2021) 6. doi: 10.1172/jci.insight.137365
40. Wang T, Wang C, Wu J, He C, Zhang W, Liu J, et al. The Different T-Cell Receptor Repertoires in Breast Cancer Tumors, Draining Lymph Nodes, and Adjacent Tissues. Cancer Immunol Res (2017) 5:148–56. doi: 10.1158/2326-6066.CIR-16-0107
41. Matsuda T, Miyauchi E, Hsu YW, Nagayama S, Kiyotani K, Zewde M, et al. TCR Sequencing Analysis of Cancer Tissues and Tumor Draining Lymph Nodes in Colorectal Cancer Patients. Oncoimmunology (2019) 8:1–8. doi: 10.1080/2162402X.2019.1588085
42. Aoki H, Ueha S, Shichino S, Ogiwara H, Hashimoto SI, Kakimi K, et al. TCR Repertoire Analysis Reveals Mobilization of Novel CD8 + T Cell Clones Into the Cancer-Immunity Cycle Following Anti-CD4 Antibody Administration. Front Immunol (2019) 10:3185. doi: 10.3389/fimmu.2018.03185
43. Chow J, Hoffend NC, Abrams SI, Schwaab T, Singh AK, Muhitch JB. Radiation Induces Dynamic Changes to the T Cell Repertoire in Renal Cell Carcinoma Patients. Proc Natl Acad Sci USA (2020) 117:23721–9. doi: 10.1073/pnas.2001933117
44. Aoki H, Ueha S, Nakamura Y, Shichino S, Nakajima H, Shimomura M, et al. Greater Extent of Blood-Tumor TCR Repertoire Overlap Is Associated With Favorable Clinical Responses to PD-1 Blockade. Cancer Sci (2021) 112:2993–3004. doi: 10.1111/cas.14975
45. Snyder A, Nathanson T, Funt SA, Ahuja A, Buros Novik J, Hellmann MD, et al. Contribution of Systemic and Somatic Factors to Clinical Response and Resistance to PD-L1 Blockade in Urothelial Cancer: An Exploratory Multi-Omic Analysis. PloS Med (2017) 14:1–24. doi: 10.1371/journal.pmed.1002309
46. Aoki H, Ueha S, Shichino S, Ogiwara H, Shitara K, Shimomura M, et al. Transient Depletion of CD4+ Cells Induces Remodeling of the TCR Repertoire in Gastrointestinal Cancer. Cancer Immunol Res (2021) 9:624–36. doi: 10.1158/2326-6066.CIR-20-0989
47. Ueha S, Yokochi S, Ishiwata Y, Ogiwara H, Chand K, Nakajima T, et al. Robust Antitumor Effects of Combined Anti-CD4-Depleting Antibody and Anti-PD-1/PD-L1 Immune Checkpoint Antibody Treatment in Mice. Cancer Immunol Res (2015) 3:631–40. doi: 10.1158/2326-6066.CIR-14-0190
48. Shitara K, Ueha S, Shichino S, Aoki H, Ogiwara H, Nakatsura T, et al. First-In-Human Phase 1 Study of IT1208, A Defucosylated Humanized Anti-CD4 Depleting Antibody, in Patients With Advanced Solid Tumors. J Immunother Cancer (2019) 7:1–11. doi: 10.1186/s40425-019-0677-y
49. Yost KE, Satpathy AT, Wells DK, Qi Y, Wang C, Kageyama R, et al. Clonal Replacement of Tumor-Specific T Cells Following PD-1 Blockade. Nat Med (2019) 25:1251–9. doi: 10.1038/s41591-019-0522-3
50. Yost KE, Chang HY, Satpathy AT. Recruiting T Cells in Cancer Immunotherapy. Sci (80 ) (2021) 372:130–1. doi: 10.1126/science.abd1329
51. Lucca LE, Axisa PP, Lu B, Harnett B, Jessel S, Zhang L, et al. Circulating Clonally Expanded T Cells Reflect Functions of Tumor-Infiltrating T Cells. J Exp Med (2021) 218(4):e20200921. doi: 10.1084/JEM.20200921
52. Pauken KE, Shahid O, Lagattuta KA, Mahuron KM, Luber JM, Lowe MM, et al. Single-Cell Analyses Identify Circulating Anti-Tumor CD8 T Cells and Markers for Their Enrichment. J Exp Med (2021) 218(4):e20200920. doi: 10.1084/JEM.20200920
53. Friedensohn S, Khan TA, Reddy ST. Advanced Methodologies in High-Throughput Sequencing of Immune Repertoires. Trends Biotechnol (2017) 35:203–14. doi: 10.1016/j.tibtech.2016.09.010
54. De Simone M, Rossetti G, Pagani M. Single Cell T Cell Receptor Sequencing: Techniques and Future Challenges. Front Immunol (2018) 9:1638. doi: 10.3389/fimmu.2018.01638
55. Neal JT, Li X, Zhu J, Giangarra V, Grzeskowiak CL, Ju J, et al. Organoid Modeling of the Tumor Immune Microenvironment. Cell (2018) 175:1972–88.e16. doi: 10.1016/j.cell.2018.11.021
56. Ma KY, Schonnesen AA, He C, Xia AY, Sun E, Chen E, et al. High-Throughput and High-Dimensional Single-Cell Analysis of Antigen-Specific CD8+ T Cells. Nat Immunol (2021) 22:1590–8. doi: 10.1038/s41590-021-01073-2
57. Li H, van der Leun AM, Yofe I, Lubling Y, Gelbard-Solodkin D, van Akkooi ACJ, et al. Dysfunctional CD8 T Cells Form a Proliferative, Dynamically Regulated Compartment Within Human Melanoma. Cell (2019) 176:775–89.e18. doi: 10.1016/j.cell.2018.11.043
58. Sade-Feldman M, Yizhak K, Bjorgaard SL, Ray JP, de Boer CG, Jenkins RW, et al. Defining T Cell States Associated With Response to Checkpoint Immunotherapy in Melanoma. Cell (2018) 175:998–1013.e20. doi: 10.1016/j.cell.2018.10.038
59. Zheng C, Zheng L, Yoo JK, Guo H, Zhang Y, Guo X, et al. Landscape of Infiltrating T Cells in Liver Cancer Revealed by Single-Cell Sequencing. Cell (2017) 169:1342–56.e16. doi: 10.1016/j.cell.2017.05.035
60. Clarke J, Panwar B, Madrigal A, Singh D, Gujar R, Wood O, et al. Single-Cell Transcriptomic Analysis of Tissue-Resident Memory T Cells in Human Lung Cancer. J Exp Med (2019) 216:2128–49. doi: 10.1084/jem.20190249
61. van der Leun AM, Thommen DS, Schumacher TN. CD8+ T Cell States in Human Cancer: Insights From Single-Cell Analysis. Nat Rev Cancer (2020) 20:218–32. doi: 10.1038/s41568-019-0235-4
62. Guo X, Zhang Y, Zheng L, Zheng C, Song J, Zhang Q, et al. Global Characterization of T Cells in Non-Small-Cell Lung Cancer by Single-Cell Sequencing. Nat Med (2018) 24:978–85. doi: 10.1038/s41591-018-0045-3
63. Simoni Y, Becht E, Fehlings M, Loh CY, Koo SL, Teng KWW, et al. Bystander CD8+ T Cells Are Abundant and Phenotypically Distinct in Human Tumour Infiltrates. Nature (2018) 557:575–9. doi: 10.1038/s41586-018-0130-2
64. Scheper W, Kelderman S, Fanchi LF, Linnemann C, Bendle G, de Rooij MAJ, et al. Low and Variable Tumor Reactivity of the Intratumoral TCR Repertoire in Human Cancers. Nat Med (2019) 25:89–94. doi: 10.1038/s41591-018-0266-5
65. Oliveira G, Stromhaug K, Klaeger S, Kula T, Frederick DT, Le PM, et al. Phenotype, Specificity and Avidity of Antitumour CD8+ T Cells in Melanoma. Nature (2021) 596:119–25. doi: 10.1038/s41586-021-03704-y
66. Poschke IC, Hassel JC, Rodriguez-Ehrenfried A, Lindner KAM, Heras-Murillo I, Appel LM, et al. The Outcome of Ex Vivo TIL Expansion Is Highly Influenced by Spatial Heterogeneity of the Tumor T-Cell Repertoire and Differences in Intrinsic In Vitro Growth Capacity Between T-Cell Clones. Clin Cancer Res (2020) 26:4289–301. doi: 10.1158/1078-0432.CCR-19-3845
67. Francis JM, Leistritz-Edwards D, Dunn A, Tarr C, Lehman J, Dempsey C, et al. Allelic Variation in Class I HLA Determines CD8+ T Cell Repertoire Shape and Cross-Reactive Memory Responses to SARS-CoV-2. Sci Immunol (2021) 3070:eabk3070. doi: 10.1126/sciimmunol.abk3070
Keywords: CD8+ T cell, T-cell receptor repertoire, single-cell TCR-seq, immune check inhibitors, cancer-immunity cycle, inter-organ clone tracking
Citation: Aoki H, Shichino S, Matsushima K and Ueha S (2022) Revealing Clonal Responses of Tumor-Reactive T-Cells Through T Cell Receptor Repertoire Analysis. Front. Immunol. 13:807696. doi: 10.3389/fimmu.2022.807696
Received: 02 November 2021; Accepted: 12 January 2022;
Published: 27 January 2022.
Edited by:
Dominik Wolf, Innsbruck Medical University, AustriaReviewed by:
David Kranz, University of Illinois at Urbana-Champaign, United StatesAlexander Yermanos, ETH Zürich, Switzerland
Copyright © 2022 Aoki, Shichino, Matsushima and Ueha. This is an open-access article distributed under the terms of the Creative Commons Attribution License (CC BY). The use, distribution or reproduction in other forums is permitted, provided the original author(s) and the copyright owner(s) are credited and that the original publication in this journal is cited, in accordance with accepted academic practice. No use, distribution or reproduction is permitted which does not comply with these terms.
*Correspondence: Satoshi Ueha, ueha@rs.tus.ac.jp