- 1Sun Yat-sen University Cancer Center, State Key Laboratory of Oncology in South China, Collaborative Innovation Center for Cancer Medicine, Guangzhou, China
- 2School of Medicine, Sun Yat-sen University, Guangzhou, China
Tumor immunotherapy, particularly the use of immune checkpoint inhibitors, has yielded impressive clinical benefits. Therefore, it is critical to accurately screen individuals for immunotherapy sensitivity and forecast its efficacy. With the application of artificial intelligence (AI) in the medical field in recent years, an increasing number of studies have indicated that the efficacy of immunotherapy can be better anticipated with the help of AI technology to reach precision medicine. This article focuses on the current prediction models based on information from histopathological slides, imaging-omics, genomics, and proteomics, and reviews their research progress and applications. Furthermore, we also discuss the existing challenges encountered by AI in the field of immunotherapy, as well as the future directions that need to be improved, to provide a point of reference for the early implementation of AI-assisted diagnosis and treatment systems in the future.
1 Introduction
Tumor immunotherapy is the process of controlling and eliminating tumors by restarting the tumor immune cycle and restoring the body’s natural anti-tumor immune response. Immune checkpoint inhibitors (ICIs), chimeric antigen receptor T-cell therapy, tumor vaccines, and peripatetic immunotherapy are the main immunotherapy modalities currently used (1). These therapies, especially the use of ICIs such as PD-1 and CTLA-4, have achieved success in a major fraction of the patients, greatly enriching the prevailing clinical oncology treatments (2). However, it is still found in the clinic that some of the population is not sensitive to these drugs, and even the treatment outcome is not as good as traditional chemotherapy drugs. Therefore, it is crucial to screen the patients that will benefit from immunotherapy. While several of the current predictors such as PD-L1, tumor mutational burden, microsatellite instability, etc., do not sufficiently address this issue (3, 4).
In recent years, the application of artificial intelligence (AI) in the medical area has expanded significantly (5). Examples include surgical robots, which have proven to offer distinctive advantages. Scholars have made numerous attempts to apply AI to predict the efficacy of immunotherapy, for instance, by establishing immunotherapy prediction scores to predict treatment efficacy and effectively screen patients who can benefit from immunotherapy (6).
2 The overview of artificial intelligence to predict immunotherapy efficacy
The general strategy for using AI to predict the efficacy of immunotherapy (Figure 1) is to set up a training cohort and a validation cohort, take the multi-scale medical data from the training cohort, acquire, filter, segment, extract and select features, hand them over to the AI for learning and modeling, and then utilize the validation cohort to verify the learning results (7, 8). This multiscale medical data may include pathological tissue, CT/MR imaging-omics, genomics, proteomics, and more. The desired learning outcome is for the AI to be able to forecast whether a patient will benefit from immunotherapy or, at the very least, recommend which patients require more evaluation, like WGS. It’s also used to predict which immunotherapy drug will be most effective for the patient.
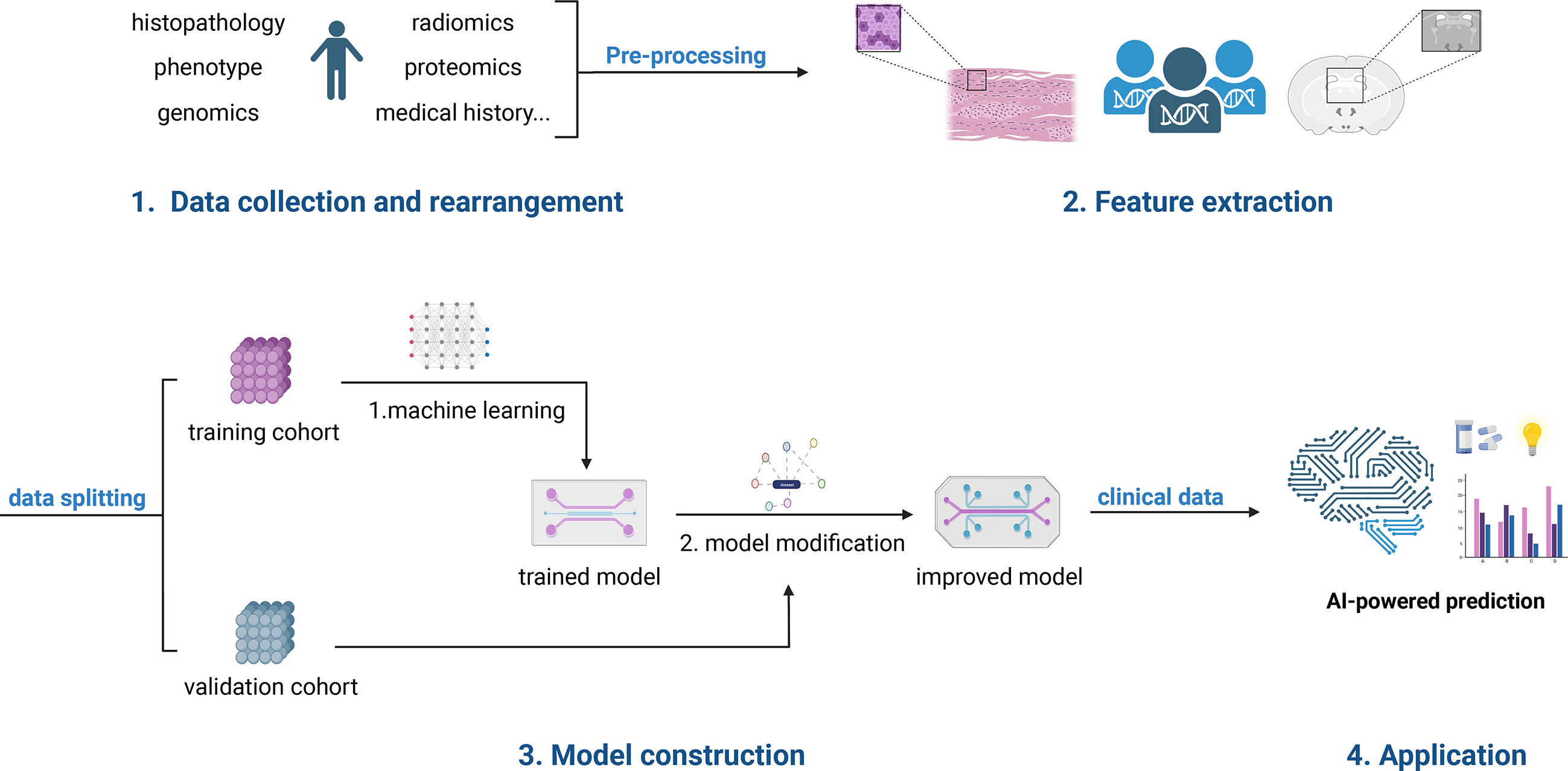
Figure 1 The workflow for using AI to predict immunotherapy efficacy. The First step is to collect multiscale medical data which include pathological tissue, CT/MR imaging-omics, genomics, proteomics, and more. The following steps are to gather, filter, segment, extract and select features. Then split these data into a training cohort and a validation cohort. Next, take the data from the training cohort, handing them over to the AI for learning and modeling. And then utilize the validation cohort to verify the learning results. Finally, a clinically applicable model will be developed.
3 The existing approaches to predicting immunotherapy outcomes
3.1 AI predicts immunotherapy efficacy by histopathological features
The gold standard for tumor identification is histopathological tissue sections, which also provide a wealth of information that can be utilized to determine disease progression, select individualized treatment plans, and predict patient survivorship. However, due to the enormous labor required of experts to extract information from complex images, traditional histopathology procedures are unable to satisfy the demands of precision medicine (9). Currently, AI-based digital pathology has been successfully used in tumor diagnosis and treatment. This technology has a wide range of future applications, including improving the accuracy of pathological diagnosis, formulating treatment plans, predicting patient prognosis, and reducing manual workload, and more (10). For instance, AI can segment and identify tumor cells in pathology slides and accurately quantify immunohistochemical staining results (11). Thus, machine learning techniques based on histopathological analysis provide novel strategies to predict response to tumor immunotherapy (12). Among these, immunohistochemical (IHC) analysis, tumor-infiltrating lymphocyte (TIL), tumor-stroma ratio (TSR), and microsatellite instability are extensively researched.
Tumor cells expressing PD-L1 can suppress the immune response by binding to PD-1 on T cells, and ICIs is an anti-tumor strategy to block this interaction. Studies have shown that PD-L1 expression levels correlate with immunotherapy response and clinical results. Previous research has demonstrated that an AI-driven PD-L1 tumor ratio score-based analyzer can identify non-small cell lung cancer (NSCLC) more effectively than a pathologist can when predicting the immunotherapy response, and its results are objective and repeatable without human error (13). In addition, the absence of DNA defective mis-match repair (MMR) mechanism caused by mutations in the MMR gene results in the accumulation of somatic genomic mutations, which are closely associated with the ICB response (14). For MMR-deficient and MMR-proficient colorectal tumors, Le et al. (15) discovered immune-related objective response rates of 40% and 0%, respectively, suggesting that MMR status can be used to predict clinical response in patients treated with immune checkpoint inhibitors. In further, many studies have confirmed that higher levels of T-cell infiltration and T-cell counts are related to improved immune checkpoint blockage (16).
Microsatellite refers to several short, repetitive DNA sequences in the genome. Microsatellite instability is associated with DNA mismatch repair and involved in the development of many malignancies. Tumors with high microsatellite instability were found to respond well to immunotherapy (17). Kather et al. (18) demonstrated that deep learning has the potential to rapidly and accurately screen patients suitable for immunotherapy by precisely identifying the microsatellite stability status of patients with gastrointestinal cancer. Furthermore, AI can recognize lymphocytes, tumor cells, and mesenchymal stroma in the section and use three-dimensional reconstruction to highlight the spatial distribution of different cell types, which is another potential factor to evaluate the efficacy of immunotherapy (19).
TIL is closely associated with immunotherapy, and deep convolutional neural network models can be used to determine its distribution from eosin-hematoxylin (HE) stained images. By assessing the immune cell types in the tumor tissue matrix and classifying patients into types A and B, Zheng et al. (20) constructed a prognostic-relevant immune phenotype classifier. Higher levels of ICB were observed in phenotype A, which indicated a superior immunotherapy effect and prognosis. Additionally, there is an end-to-end strategy to train DL directly on response or outcome data, which used convolutional neural networks (CNNs) or graph neural networks (GNNs) to predict immunotherapy responses. According to these trials, the AUCs for predicting responders in lung cancer and melanoma were 0.778 and 0.69, respectively (21).
Tumor mutational burden (TMB) is defined as the number of non-synonymous single nucleotide variants (NsSNV) on tumor cells that can be transcribed into new antigenic peptides and presented on the cell surface, thereby activating T cells (22). High TMB is shown to be a predictive biomarker for lung cancer (23). Recent research suggests that deep learning systems can be used to predict immune responses. The study used HE-stained images to predict the status of TMB and reached an AUC of 0.78-0.98 in the external validation group, much higher than utilizing clinical data alone (24).
AI has a promising development in predicting tumor immunotherapy response and can examine the state of tumor development through pathological data. Its algorithm and analysis results can be highly standardized and shared, which may assist advance medicine and actualizing precision medicine (Figure 2).
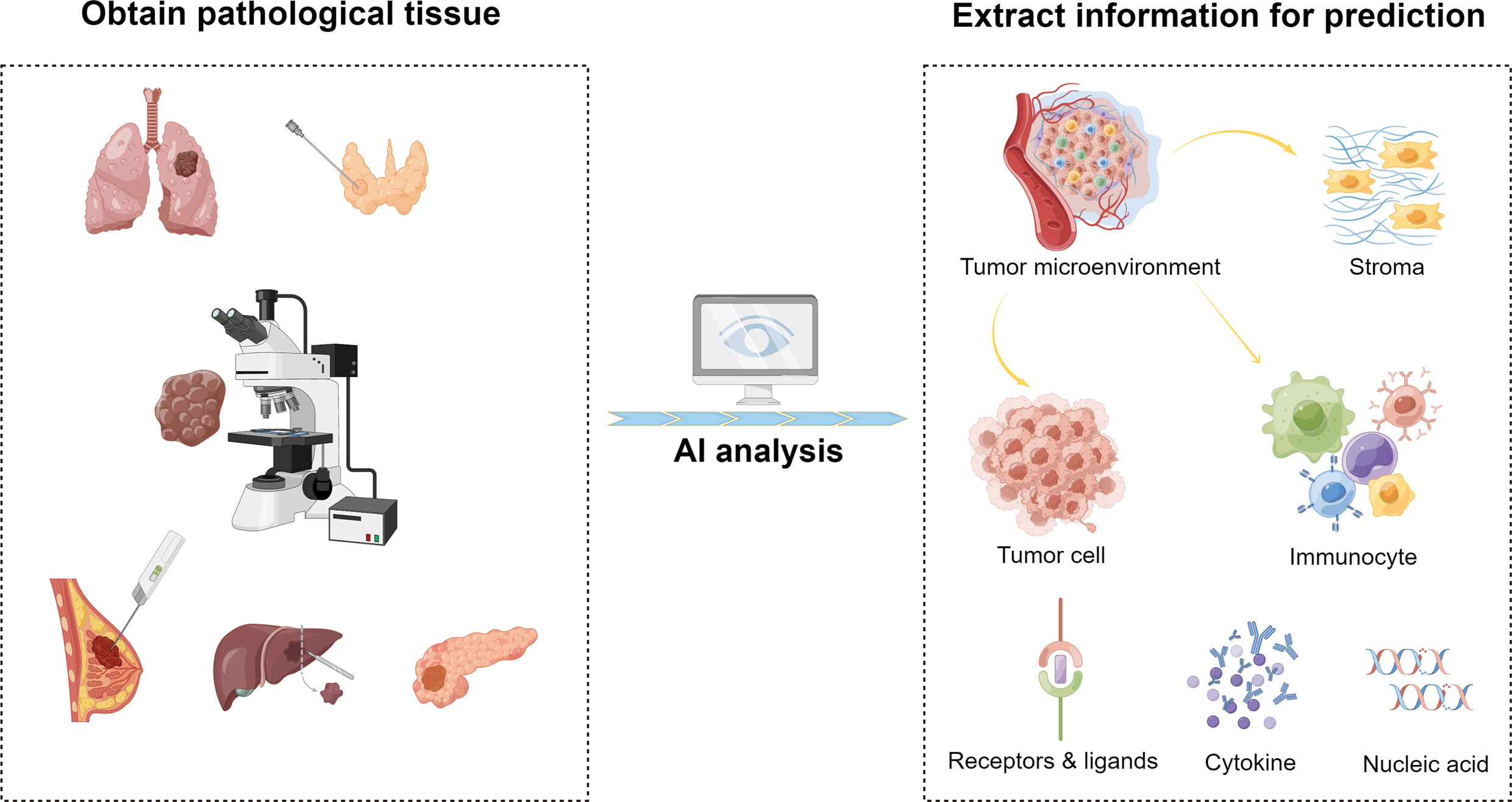
Figure 2 AI-based evaluation of immunotherapy efficacy by histopathological features. This is an illustration of the use of AI to forecast the effectiveness of immunotherapy. With the help of AI, more specific information can be extracted from clinical pathological tissues, including components of the tumor microenvironment and small molecular components like receptors, ligands, cytokines, nucleic acids, etc. From these elements, AI gathers data related to immunotherapy to forecast the efficacy.
3.2 AI predicts immunotherapy efficacy by imaging-omics features
With the constant advancement of medical imaging equipment and technology, medical images are no longer simply pictures or restricted to the traditional “computer assisted diagnostics (CAD)”, but also contain high-throughput mineable data that are not recognizable to the naked eye (25). Imaging histology refers to AI-based imaging characterization that can provide more detailed information than single graphics can, displaying macroscopic, molecular, and cellular features (26, 27). Additionally, AI-based predictive models can provide reliable non-invasive biomarkers for evaluating immunotherapeutic response. Among various biomarkers, PD-L1 expression has been well validated in immunotherapy, and a combined model based on CT radiomics and clinical features can assess PD-L1 expression levels non-invasively (28). Sun et al. (29) selected 135 patients with tumors at different sites derived from phase I PD-1/PD-L1 monotherapy clinical trials. They established a predictive imaging model based on CT imaging by combining enhanced CT images with RNA-seq genomic data from tumor biopsy tissues. This model can analyze tumor-infiltrating CD8 signals and distinguish between immune-infiltrating and immunodetect types, making it an efficient method for predicting clinical outcomes of patients with advanced solid tumors after immunotherapy. Mu et al. (30) analyzed baseline PET/CT data of 194 patients with stage IIIB-IV NSCLC treated with PD-1/PD-L1 inhibitors, and they developed multiparametric imaging histological signature models which successfully predict whether patients would receive sustained clinical benefit from immunotherapy. However, further research is still required to determine whether models utilizing several data sources can perform better than models that simply use radiomics.
TMB is also well known for being one of the important indicators of ICI efficacy. He et al. (31) used deep learning techniques to analyze CT images from patients with advanced NSCLC and establish TMB radiomic biomarkers, which have high predictive value for ICI treatment response, overall survival (OS), and progression-free survival (PFS). Furthermore, Trebeschi et al. (32) used AI techniques to study the pre-treatment enhanced CT image analysis of patients with progressive malignant melanoma and NSCLC treated with PD-1, and they discovered that lesions with more heterogeneous morphology, which means compact borders and inhomogeneous density, were more likely to respond to immunotherapy. Additionally, there is a substantial association between imaging histological indices and mitosis-related pathways, suggesting that higher proliferative potential may signal better immunotherapy efficacy.
However, despite the fact that hyperprogression—rapid tumor progression following immunotherapy—is associated with a poor prognosis, there are no validated biomarkers to identify patients at risk for it (33, 34). Vaidya et al. (35) retrospectively summarized clinical and imaging data from 109 patients with advanced NSCLC treated with PD-1/PD-L1 immunosuppressant monotherapy, 19 of whom showed hyper progression. And researchers extracted textural features from the patients’ baseline CT images reflecting the texture within and around the target lesion as well as histological features quantifying the degree of peri-lesion vascular tortuosity, which can somewhat predict whether patients will develop hyper progression.
In general, the majority of studies’ Radiomics Quality Scores (RQS) ranged from 11 to 20 out of a possible maximum score of 36 points (36). It indicates that AI-based imaging-omics analysis can delve into the spatiotemporal heterogeneity of tumors and plays an important role in predicting immunotherapy response, biomarker expression, and patient prognosis, particularly in the absence of histopathological specimens. Deeper studies of imaging histology can aid in the diagnosis of immunotherapy-eligible patients, disease risk assessment, and precision medicine.
3.3 AI predicts immunotherapy efficacy by genomics
Thanks to advances in sequencing technology, a large amount of cancer genomic data has now been accumulated, providing more precise recommendations for directing tumor immunotherapy. The development of next-generation sequencing (NGS) technologies has enabled comprehensive genomic and transcriptomic screening. This allows to the generation of datasets for analyzing tumor drivers, as well as sequencing cancer cells, stromal cells, and immune cells within the tumor microenvironment to reveal the characteristics of therapeutic effects (37). The human genome contains more than 3 billion base pairs, making it a vast space of high-dimensional data with very complicated information. Whole genome sequencing (WGS) offers the most comprehensive information about the genome, but sorting out the genes, phenotypes, and their inter-regulatory relationships requires the assistance of AI, particularly deep learning techniques. Xie et al. (38) developed a predictive model that integrated genomic data from multiple perspectives, such as TMB, microsatellite instability, and somatic cell copy number variation in many different tumor types to effectively differentiate between “cold” and “hot” immune patients. The model was further externally validated using data from clinical trials, showing that patients in the hyperimmune group were more responsive to immunotherapy and had a better prognosis. By sequencing WES and RNA sequences from 110 patients with metastatic melanoma, Van Allen et al. (39) found that individual response rates to anti-CTLA4 correlated with TMB and cytolytic markers. It has also been shown that the expression and variant levels of platelet-related genes are closely related to the prognosis and immunotherapy response in patients with triple-negative breast cancer (40). These studies provided a direction to explore the variable response to immune checkpoint inhibitors and the identification of prognostic biomarkers.
Moreover, some factors in the transcriptome are still required to be added to the genomic analysis in order to fully predict immune response and explain drug resistance (41). By combining exome and transcriptome sequencing with mass spectrometry, Yadav et al. (42) discovered immunogenic mutant peptides with MHC specificity that could be useful for individualized vaccine development. Furthermore, Mo et al. (43) developed a high-throughput screening platform using 384-well plates to observe tumor immune interactions by co-culturing peripheral blood mononuclear cells (PBMC) and cancer cells in each well to assess cellular value-added and viability and to detect cell growth phenotypes. They also tested the effects of multiple bioactive compounds and identified three potential antagonists for enhancing immune activity. Some scholars have also proved it by epigenetic profiling that the DNA methylation landscape of patient CART19 cells influences the efficacy of the cellular immunotherapy treatment in patients with B-cell malignancy (44).
3.4 Others
Numerous studies have utilized AI for a variety of tumor immunotherapy applications (Table 1). The current liquid biopsy technology offers a more accessible and flexible approach to tumor diagnosis and treatment, which is represented by the detection of circulating tumor cell DNA. In immunotherapy, liquid genetic biomarkers are increasingly being developed to predict the therapeutic efficacy of ICIs (59), and molecular biological information in liquid specimens can be automatically identified and detected by AI technology (45). Additionally, some biomarkers are used to exclude hyperprogressive or pseudoprogressive disease after immunotherapy, including plasma cytokines interleukins and circulating tumor cell DNA (60).
In addition to genomics and imaging, proteomics technologies have been extensively explored to identify biomarkers for the effectiveness of tumor immunotherapy (46). An AI-based serum proteomics test model has been developed to predict response to ICIs in patients with metastatic melanoma (48). The application of multi-omics-based AI models to predict tumor immunotherapy responses is also promising. Multi-omics integrates genomics, transcriptomics, epigenomics, proteomics, and radiomics, allowing for a more thoroughly characterization of the disease with data from different sources (49). Based on the multi-omics concept, Yi Yang et al. (50) constructed a deep learning-based 90-day prediction model using age, gender, medical history, baseline data, routine laboratory tests, and follow-up CT scans of NSCLC patients to better distinguish immunotherapy responders from non-responders. Similarly, Arsela Prelaj et al. (61) used machine learning techniques to predict OS and PFS in responders and non-responders in a real-world study. Additionally, Peng Song et al. (51) suggested combining DNA and RNA sequencing, immunohistochemical staining results, demographic baseline data, medical history, and laboratory test results to analyze the information related to efficacy and break the limitation of relying only on PD-1 expression level and TMB level to predict immunotherapy response, and develop a mature model for immunotherapy response prediction for Chinese lung cancer patients. Along with PD-L1 expression, TMB, and TIL, it has been demonstrated that miRNA abnormalities (62), EGFR mutations (54), TP53 mutations (63), ALK rearrangements (55), gut microbiota (56), Fc gamma receptor (FcγR) polymorphisms (57) and serum complement levels (58) all influence immunotherapy response to some extent. It would facilitate the robustness of prediction if this information could be merged with pathological sections and imaging histology to form multi-omics integrated data.
Moreover, tumor-like organs serve as a useful screening model for immunotherapy because they can accurately reproduce the tumor microenvironment while still generating in vitro immune-tumor interactions (47). Incorporating AI into organoids is anticipated to overcome the safety and individualization challenges of conventional prediction by establishing a productive platform for tissue collection, tumor in vitro culture, growth analysis, and medication screening (52). AI could also forecast ICB responses by focusing on antigen presentation pathways (64) and cell necroptosis index (CNI) (53).
4 Future directions
Although the majority of studies have proven that its models have performed as well as or better than doctors, there are only a few successful real-world applications (65). Lack of a uniform database, industry standards, specialized clinical application situations, policy and regulatory assistance, and so on may all contribute to implementation challenges (Figure 3).
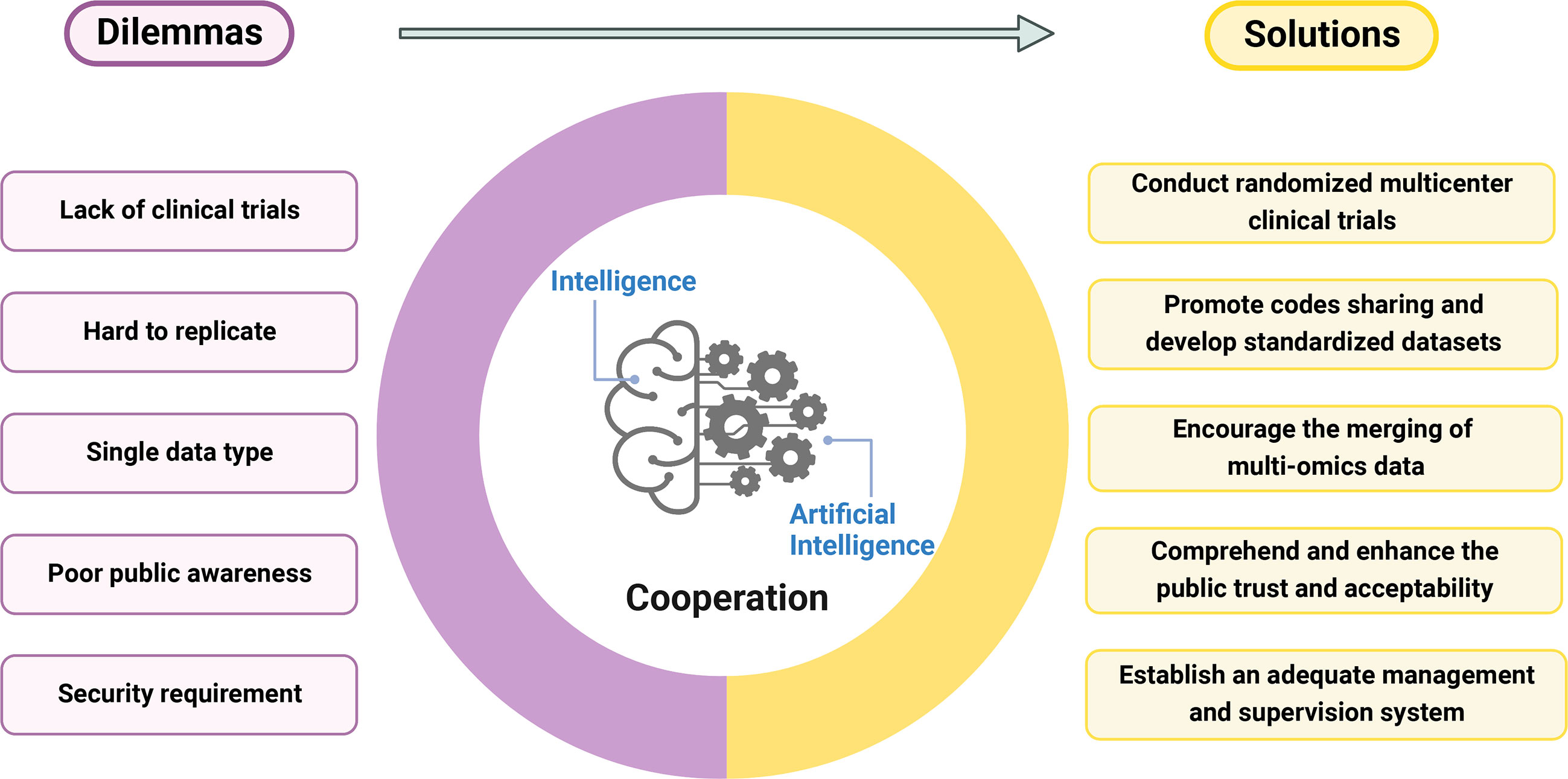
Figure 3 The dilemmas and solutions in predicting immunotherapy efficacy with AI. The left side outline the current difficulties AI encountered, and the right side proposes possible solutions. And only the excellent cooperation of artificial intelligence and human intelligence can achieve the best prediction effect.
Firstly, there are relatively few prospective research and randomized clinical trials pertaining to the use of AI in immunotherapy, and these studies are highly biased. Therefore, we should increase experimental design transparency, strengthen the correlation with clinical reality, and reduce systematic error. Additionally, we must protect patient interests, minimize research waste, consider the cohort’s rationality, and avoid irrationally exaggerating research findings and potential applications.
Second, due to the scarcity of codes and data sources, AI models are challenging to fully replicate. Additionally, it is challenging to evaluate the stability of different models because of various inconsistent preprocessing methods and prediction objectives. Moreover, biomarkers may differ from primary tumor to metastatic tumor (66), making determining the optimal prediction for a certain tumor type much more difficult. Therefore, we should promote code sharing across fields and the development of standardized datasets.
Thirdly, the merging of multi-omics data must be encouraged to create a medical system that is more precise, individualized, and predictable. AI has a unique recognition model that can quickly identify and combine vast amounts of information in a way that humans cannot. An ideal AI-based predictive model for immunotherapy should include all relevant clinical information about the patient (genomics, imaging, proteomics, pathological tissue, demographic information, medical history, etc.). Since concepts like pan-cancer analysis have been reflected in the evaluation of PD-1/PD-L1 efficacy (67), it is important to promote the integrity and objectivity of data collection to facilitate the sharing of large data from multiple centers. This shows great promise for the future of immunotherapy (68).
Fourth, we need to comprehend how patients, medical professionals, and the general public feel about the use of AI in the healthcare system. To encourage the application of AI in practical uses, we should also carefully consider the fee-for-service model and deal with the common interests of patients, health insurance, doctors, and information engineers. Additionally, a strong management and supervision system must be established to reduce actual hazards and guarantee the security of this developing system (69, 70).
Last but not least, a reasonable legal and ethical environment should be established to facilitate the sharing of big data and the multi-regional and multi-centered use of AI systems, ensuring that they are ethical and that the corresponding responsibilities of each sector are clear.
In summary, due to the complexity of immunotherapy prediction, scientific researchers, enterprises, and clinicians must collaborate to build databases and industry standards, remove technical barriers, and support the development of AI-assisted systems that can precisely identify the target population of immunotherapy, accurately predict the efficacy and prognosis, and promote the implementation of AI-assisted treatment while winning the trust of both doctors and patients.
5 Conclusion
With the aid of artificial intelligence, a cutting-edge technology, it is now possible to treat tumor patients on an individual basis by automating the prediction of tumor immunotherapy effects based on constructed models. However, it still faces many challenges and dilemmas. Future AI-assisted systems are anticipated to be better able to model tumor biological behavior and medication treatment response, which would ultimately help the vast majority of tumor patients and enhance medical effectiveness and quality.
Author contributions
XX, ZG, and YZ provided direction and guidance throughout the preparation of this manuscript. JX, XL, and XD wrote and edited the manuscript. JX, XL, XD, YT, and WT reviewed and made significant revisions to the manuscript. HC, and JZ collected and prepared the related papers. All authors contributed to the article and approved the submitted version.
Funding
This research was funded by the National Natural Science Foundation of China (81872152, XX).
Acknowledgments
We thank to Figdraw and Biorender for providing scientific researchers with exquisite materials and convenient drawing platforms. All the figures in this article are made by Figdraw (www.figdraw.com) and Biorender (biorender.com).
Conflict of interest
The authors declare that the research was conducted in the absence of any commercial or financial relationships that could be construed as a potential conflict of interest.
Publisher’s note
All claims expressed in this article are solely those of the authors and do not necessarily represent those of their affiliated organizations, or those of the publisher, the editors and the reviewers. Any product that may be evaluated in this article, or claim that may be made by its manufacturer, is not guaranteed or endorsed by the publisher.
References
1. Nishino M, Ramaiya NH, Hatabu H, Hodi FS. Monitoring immune-checkpoint blockade: response evaluation and biomarker development. Nat Rev Clin Oncol (2017) 14(11):655–68. doi: 10.1038/nrclinonc.2017.88
2. Anagnostou V, Landon BV, Medina JE, Forde P, Velculescu VE. Translating the evolving molecular landscape of tumors to biomarkers of response for cancer immunotherapy. Sci Transl Med (2022) 14(670):eabo3958. doi: 10.1126/scitranslmed.abo3958
3. Herbst RS, Baas P, Kim DW, Felip E, Perez-Gracia JL, Han JY, et al. Pembrolizumab versus docetaxel for previously treated, PD-L1-positive, advanced non-small-cell lung cancer (KEYNOTE-010): a randomised controlled trial. Lancet. (2016) 387(10027):1540–50. doi: 10.1016/S0140-6736(15)01281-7
4. Giustini N, Bazhenova L. Recognizing prognostic and predictive biomarkers in the treatment of non-small cell lung cancer (NSCLC) with immune checkpoint inhibitors (ICIs). Lung Cancer (Auckl). (2021) 12:21–34. doi: 10.2147/LCTT.S235102
5. Benjamens S, Dhunnoo P, Mesko B. The state of artificial intelligence-based FDA-approved medical devices and algorithms: an online database. NPJ Digit Med (2020) 3:118. doi: 10.1038/s41746-020-00324-0
6. Angell H, Galon J. From the immune contexture to the immunoscore: the role of prognostic and predictive immune markers in cancer. Curr Opin Immunol (2013) 25(2):261–7. doi: 10.1016/j.coi.2013.03.004
7. Kantarjian H, Yu PP. Artificial intelligence, big data, and cancer. JAMA Oncol (2015) 1(5):573–4. doi: 10.1001/jamaoncol.2015.1203
8. Hosny A, Parmar C, Quackenbush J, Schwartz LH, Aerts H. Artificial intelligence in radiology. Nat Rev Cancer. (2018) 18(8):500–10. doi: 10.1038/s41568-018-0016-5
9. van der Laak J, Litjens G, Ciompi F. Deep learning in histopathology: the path to the clinic. Nat Med (2021) 27(5):775–84. doi: 10.1038/s41591-021-01343-4
10. Lancellotti C, Cancian P, Savevski V, Kotha SRR, Fraggetta F, Graziano P, et al. Artificial intelligence & tissue biomarkers: Advantages, risks and perspectives for pathology. Cells (2021) 10(4):787. doi: 10.3390/cells10040787
11. Sirinukunwattana K, Ahmed Raza SE, Yee-Wah T, Snead DR, Cree IA, Rajpoot NM. Locality sensitive deep learning for detection and classification of nuclei in routine colon cancer histology images. IEEE Trans Med Imaging. (2016) 35(5):1196–206. doi: 10.1109/TMI.2016.2525803
12. Martins J, Magalhaes C, Rocha M, Osorio NS. Machine learning-enhanced T cell neoepitope discovery for immunotherapy design. Cancer Inform. (2019) 18:1176935119852081. doi: 10.1177/1176935119852081
13. Choi S, Cho SI, Ma M, Park S, Pereira S, Aum BJ, et al. Artificial intelligence-powered programmed death ligand 1 analyser reduces interobserver variation in tumour proportion score for non-small cell lung cancer with better prediction of immunotherapy response. Eur J Cancer. (2022) 170:17–26. doi: 10.1016/j.ejca.2022.04.011
14. Asaoka Y, Ijichi H, Koike K. PD-1 blockade in tumors with mismatch-repair deficiency. N Engl J Med (2015) 373(20):1979. doi: 10.1056/NEJMc1510353
15. Le DT, Uram JN, Wang H, Bartlett BR, Kemberling H, Eyring AD, et al. PD-1 blockade in tumors with mismatch-repair deficiency. N Engl J Med (2015) 372(26):2509–20. doi: 10.1056/NEJMoa1500596
16. Fridman WH, Pages F, Sautes-Fridman C, Galon J. The immune contexture in human tumours: impact on clinical outcome. Nat Rev Cancer. (2012) 12(4):298–306. doi: 10.1038/nrc3245
17. Li K, Luo H, Huang L, Luo H, Zhu X. Microsatellite instability: a review of what the oncologist should know. Cancer Cell Int (2020) 20:16. doi: 10.1186/s12935-019-1091-8
18. Kather JN, Pearson AT, Halama N, Jager D, Krause J, Loosen SH, et al. Deep learning can predict microsatellite instability directly from histology in gastrointestinal cancer. Nat Med (2019) 25(7):1054–6. doi: 10.1038/s41591-019-0462-y
19. Stanton SE, Disis ML. Clinical significance of tumor-infiltrating lymphocytes in breast cancer. J Immunother Cancer. (2016) 4:59. doi: 10.1186/s40425-016-0165-6
20. Zheng S, Zou Y, Xie X, Liang JY, Yang A, Yu K, et al. Development and validation of a stromal immune phenotype classifier for predicting immune activity and prognosis in triple-negative breast cancer. Int J Cancer. (2020) 147(2):542–53. doi: 10.1002/ijc.33009
21. Hu J, Cui C, Yang W, Huang L, Yu R, Liu S, et al. Using deep learning to predict anti-PD-1 response in melanoma and lung cancer patients from histopathology images. Transl Oncol (2021) 14(1):100921. doi: 10.1016/j.tranon.2020.100921
22. Ushio R, Murakami S, Saito H. Predictive markers for immune checkpoint inhibitors in non-small cell lung cancer. J Clin Med (2022) 11(7):1855. doi: 10.3390/jcm11071855
23. Rizvi NA, Hellmann MD, Snyder A, Kvistborg P, Makarov V, Havel JJ, et al. Cancer immunology. mutational landscape determines sensitivity to PD-1 blockade in non-small cell lung cancer. Science. (2015) 348(6230):124–8. doi: 10.1126/science.aaa1348
24. Sadhwani A, Chang HW, Behrooz A, Brown T, Auvigne-Flament I, Patel H, et al. Comparative analysis of machine learning approaches to classify tumor mutation burden in lung adenocarcinoma using histopathology images. Sci Rep (2021) 11(1):16605. doi: 10.1038/s41598-021-95747-4
25. Castellino RA. Computer aided detection (CAD): an overview. Cancer Imaging. (2005) 5:17–9. doi: 10.1102/1470-7330.2005.0018
26. Su X, Chen N, Sun H, Liu Y, Yang X, Wang W, et al. Automated machine learning based on radiomics features predicts H3 K27M mutation in midline gliomas of the brain. Neuro Oncol (2020) 22(3):393–401. doi: 10.1093/neuonc/noz184
27. Aerts HJ. The potential of radiomic-based phenotyping in precision medicine: A review. JAMA Oncol (2016) 2(12):1636–42. doi: 10.1001/jamaoncol.2016.2631
28. Wang C, Ma J, Shao J, Zhang S, Li J, Yan J, et al. Non-invasive measurement using deep learning algorithm based on multi-source features fusion to predict PD-L1 expression and survival in NSCLC. Front Immunol (2022) 13:828560. doi: 10.3389/fimmu.2022.828560
29. Sun R, Limkin EJ, Vakalopoulou M, Dercle L, Champiat S, Han SR, et al. A radiomics approach to assess tumour-infiltrating CD8 cells and response to anti-PD-1 or anti-PD-L1 immunotherapy: an imaging biomarker, retrospective multicohort study. Lancet Oncol (2018) 19(9):1180–91. doi: 10.1016/S1470-2045(18)30413-3
30. Mu W, Tunali I, Gray JE, Qi J, Schabath MB, Gillies RJ. Radiomics of (18)F-FDG PET/CT images predicts clinical benefit of advanced NSCLC patients to checkpoint blockade immunotherapy. Eur J Nucl Med Mol Imaging. (2020) 47(5):1168–82. doi: 10.1007/s00259-019-04625-9
31. He B, Dong D, She Y, Zhou C, Fang M, Zhu Y, et al. Predicting response to immunotherapy in advanced non-small-cell lung cancer using tumor mutational burden radiomic biomarker. J Immunother Cancer (2020) 8(2):e000550. doi: 10.1136/jitc-2020-000550
32. Trebeschi S, Drago SG, Birkbak NJ, Kurilova I, Cǎlin AM, Delli Pizzi A, et al. Predicting response to cancer immunotherapy using noninvasive radiomic biomarkers. Ann Oncol (2019) 30(6):998–1004. doi: 10.1093/annonc/mdz108
33. Frelaut M, Le Tourneau C, Borcoman E. Hyperprogression under immunotherapy. Int J Mol Sci (2019) 20(11):2674. doi: 10.3390/ijms20112674
34. Matos I, Garralda E. Clarification of definitions of hyperprogressive disease during immunotherapy. JAMA Oncol (2021) 7(1):136–7. doi: 10.1001/jamaoncol.2020.5582
35. Vaidya P, Bera K, Patil PD, Gupta A, Jain P, Alilou M, et al. Novel, non-invasive imaging approach to identify patients with advanced non-small cell lung cancer at risk of hyperprogressive disease with immune checkpoint blockade. J Immunother Cancer (2020) 8(2):e001343. doi: 10.1136/jitc-2020-001343
36. Dercle L, McGale J, Sun S, Marabelle A, Yeh R, Deutsch E, et al. Artificial intelligence and radiomics: fundamentals, applications, and challenges in immunotherapy. J Immunother Cancer (2022) 10(9):e005292. doi: 10.1136/jitc-2022-005292
37. Rosenberg JE, Hoffman-Censits J, Powles T, van der Heijden MS, Balar AV, Necchi A, et al. Atezolizumab in patients with locally advanced and metastatic urothelial carcinoma who have progressed following treatment with platinum-based chemotherapy: a single-arm, multicentre, phase 2 trial. Lancet. (2016) 387(10031):1909–20. doi: 10.1016/S0140-6736(16)00561-4
38. Xie F, Zhang J, Wang J, Reuben A, Xu W, Yi X, et al. Multifactorial deep learning reveals pan-cancer genomic tumor clusters with distinct immunogenomic landscape and response to immunotherapy. Clin Cancer Res (2020) 26(12):2908–20. doi: 10.1158/1078-0432.CCR-19-1744
39. Van Allen EM, Miao D, Schilling B, Shukla SA, Blank C, Zimmer L, et al. Genomic correlates of response to CTLA-4 blockade in metastatic melanoma. Science. (2015) 350(6257):207–11. doi: 10.1126/science.aad0095
40. Xie J, Zou Y, Ye F, Zhao W, Xie X, Ou X, et al. A novel platelet-related gene signature for predicting the prognosis of triple-negative breast cancer. Front Cell Dev Biol (2021) 9:795600. doi: 10.3389/fcell.2021.795600
41. Hugo W, Shi H, Sun L, Piva M, Song C, Kong X, et al. Non-genomic and immune evolution of melanoma acquiring MAPKi resistance. Cell. (2015) 162(6):1271–85. doi: 10.1016/j.cell.2015.07.061
42. Yadav M, Jhunjhunwala S, Phung QT, Lupardus P, Tanguay J, Bumbaca S, et al. Predicting immunogenic tumour mutations by combining mass spectrometry and exome sequencing. Nature. (2014) 515(7528):572–6. doi: 10.1038/nature14001
43. Mo X, Tang C, Niu Q, Ma T, Du Y, Fu H. HTiP: High-throughput immunomodulator phenotypic screening platform to reveal IAP antagonists as anti-cancer immune enhancers. Cell Chem Biol (2019) 26(3):331–9 e3. doi: 10.1016/j.chembiol.2018.11.011
44. Garcia-Prieto CA, Villanueva L, Bueno-Costa A, Davalos V, Gonzalez-Navarro EA, Juan M, et al. Epigenetic profiling and response to CD19 chimeric antigen receptor T-cell therapy in b-cell malignancies. J Natl Cancer Inst (2022) 114(3):436–45. doi: 10.1093/jnci/djab194
45. Ko J, Baldassano SN, Loh PL, Kording K, Litt B, Issadore D. Machine learning to detect signatures of disease in liquid biopsies - a user's guide. Lab Chip. (2018) 18(3):395–405. doi: 10.1039/C7LC00955K
46. Park Y, Kim MJ, Choi Y, Kim NH, Kim L, Hong SPD, et al. Role of mass spectrometry-based serum proteomics signatures in predicting clinical outcomes and toxicity in patients with cancer treated with immunotherapy. J Immunother Cancer (2022) 10(3):e003566. doi: 10.1136/jitc-2021-003566
47. Weeber F, van de Wetering M, Hoogstraat M, Dijkstra KK, Krijgsman O, Kuilman T, et al. Preserved genetic diversity in organoids cultured from biopsies of human colorectal cancer metastases. Proc Natl Acad Sci U S A. (2015) 112(43):13308–11. doi: 10.1073/pnas.1516689112
48. Bojar D, Lisacek F. Glycoinformatics in the artificial intelligence era. Chem Rev (2022) 122(20):15971–88. doi: 10.1021/acs.chemrev.2c00110
49. Hasin Y, Seldin M, Lusis A. Multi-omics approaches to disease. Genome Biol (2017) 18(1):83. doi: 10.1186/s13059-017-1215-1
50. Yang Y, Yang J, Shen L, Chen J, Xia L, Ni B, et al. A multi-omics-based serial deep learning approach to predict clinical outcomes of single-agent anti-PD-1/PD-L1 immunotherapy in advanced stage non-small-cell lung cancer. Am J Transl Res (2021) 13(2):743–56.
51. Song P, Cui X, Bai L, Zhou X, Zhu X, Zhang J, et al. Molecular characterization of clinical responses to PD-1/PD-L1 inhibitors in non-small cell lung cancer: Predictive value of multidimensional immunomarker detection for the efficacy of PD-1 inhibitors in Chinese patients. Thorac Cancer. (2019) 10(5):1303–9. doi: 10.1111/1759-7714.13078
52. Drost J, Clevers H. Organoids in cancer research. Nat Rev Cancer. (2018) 18(7):407–18. doi: 10.1038/s41568-018-0007-6
53. Xie J, Tian W, Tang Y, Zou Y, Zheng S, Wu L, et al. Establishment of a cell necroptosis index to predict prognosis and drug sensitivity for patients with triple-negative breast cancer. Front Mol Biosci (2022) 9:834593. doi: 10.3389/fmolb.2022.834593
54. Masuda K, Horinouchi H, Tanaka M, Higashiyama R, Shinno Y, Sato J, et al. Efficacy of anti-PD-1 antibodies in NSCLC patients with an EGFR mutation and high PD-L1 expression. J Cancer Res Clin Oncol (2021) 147(1):245–51. doi: 10.1007/s00432-020-03329-0
55. Oya Y, Kuroda H, Nakada T, Takahashi Y, Sakakura N, Hida T. Efficacy of immune checkpoint inhibitor monotherapy for advanced non-Small-Cell lung cancer with ALK rearrangement. Int J Mol Sci (2020) 21(7):2623. doi: 10.3390/ijms21072623
56. Uribe-Herranz M, Klein-Gonzalez N, Rodriguez-Lobato LG, Juan M, de Larrea CF. Gut microbiota influence in hematological malignancies: From genesis to cure. Int J Mol Sci (2021) 22(3):1026. doi: 10.3390/ijms22031026
57. Mata-Molanes JJ, Rebollo-Liceaga J, Martinez-Navarro EM, Manzano RG, Brugarolas A, Juan M, et al. Relevance of fc gamma receptor polymorphisms in cancer therapy with monoclonal antibodies. Front Oncol (2022) 12:926289. doi: 10.3389/fonc.2022.926289
58. Zhang D, Li Y, Li H, Tang T, Zheng Y, Guo X, et al. A preliminary study of the complement component 1q levels in predicting the efficacy of combined immunotherapy in patients with lung cancer. Cancer Manag Res (2021) 13:7131–7. doi: 10.2147/CMAR.S314369
59. Iinuma H. [Genomic analysis and immunotherapy by liquid biopsy]. Gan To Kagaku Ryoho (2019) 46(9):1361–6.
60. Yan Y, Chen X, Wei J, Gong Z, Xu Z. Immunotherapy combinations in patients with small cell lung cancers. J Thorac Oncol (2019) 14(10):e244–e5. doi: 10.1016/j.jtho.2019.05.021
61. Hopp L, Loffler-Wirth H, Galle J, Binder H. Combined SOM-portrayal of gene expression and DNA methylation landscapes disentangles modes of epigenetic regulation in glioblastoma. Epigenomics. (2018) 10(6):745–64. doi: 10.2217/epi-2017-0140
62. Fan J, Yin Z, Xu J, Wu F, Huang Q, Yang L, et al. Circulating microRNAs predict the response to anti-PD-1 therapy in non-small cell lung cancer. Genomics. (2020) 112(2):2063–71. doi: 10.1016/j.ygeno.2019.11.019
63. Biton J, Mansuet-Lupo A, Pecuchet N, Alifano M, Ouakrim H, Arrondeau J, et al. TP53, STK11, and EGFR mutations predict tumor immune profile and the response to anti-PD-1 in lung adenocarcinoma. Clin Cancer Res (2018) 24(22):5710–23. doi: 10.1158/1078-0432.CCR-18-0163
64. Huemer F, Leisch M, Geisberger R, Melchardt T, Rinnerthaler G, Zaborsky N, et al. Combination strategies for immune-checkpoint blockade and response prediction by artificial intelligence. Int J Mol Sci (2020) 21(8):2856. doi: 10.3390/ijms21082856
65. Nagendran M, Chen Y, Lovejoy CA, Gordon AC, Komorowski M, Harvey H, et al. Artificial intelligence versus clinicians: systematic review of design, reporting standards, and claims of deep learning studies. BMJ (2020) 368:m689. doi: 10.1136/bmj.m689
66. Zou Y, Hu X, Zheng S, Yang A, Li X, Tang H, et al. Discordance of immunotherapy response predictive biomarkers between primary lesions and paired metastases in tumours: A systematic review and meta-analysis. EBioMedicine. (2021) 63:103137. doi: 10.1016/j.ebiom.2020.103137
67. Shameer K, Johnson KW, Glicksberg BS, Dudley JT, Sengupta PP. The whole is greater than the sum of its parts: combining classical statistical and machine intelligence methods in medicine. Heart. (2018) 104(14):1228. doi: 10.1136/heartjnl-2018-313377
68. Stephansen JB, Olesen AN, Olsen M, Ambati A, Leary EB, Moore HE, et al. Neural network analysis of sleep stages enables efficient diagnosis of narcolepsy. Nat Commun (2018) 9(1):5229. doi: 10.1038/s41467-018-07229-3
69. Esteva A, Robicquet A, Ramsundar B, Kuleshov V, DePristo M, Chou K, et al. A guide to deep learning in healthcare. Nat Med (2019) 25(1):24–9. doi: 10.1038/s41591-018-0316-z
Keywords: artificial intelligence, immunotherapy, deep learning, multi-omics, genomics
Citation: Xie J, Luo X, Deng X, Tang Y, Tian W, Cheng H, Zhang J, Zou Y, Guo Z and Xie X (2023) Advances in artificial intelligence to predict cancer immunotherapy efficacy. Front. Immunol. 13:1076883. doi: 10.3389/fimmu.2022.1076883
Received: 22 October 2022; Accepted: 09 December 2022;
Published: 04 January 2023.
Edited by:
Manel Juan, Hospital Clinic of Barcelona, SpainReviewed by:
Sergio Navarro Velázquez, Hospital Clinic of Barcelona, SpainMichael Dennis, University of California, San Diego, United States
Copyright © 2023 Xie, Luo, Deng, Tang, Tian, Cheng, Zhang, Zou, Guo and Xie. This is an open-access article distributed under the terms of the Creative Commons Attribution License (CC BY). The use, distribution or reproduction in other forums is permitted, provided the original author(s) and the copyright owner(s) are credited and that the original publication in this journal is cited, in accordance with accepted academic practice. No use, distribution or reproduction is permitted which does not comply with these terms.
*Correspondence: Xiaoming Xie, eGlleG1Ac3lzdWNjLm9yZy5jbg==; Zhixing Guo, Z3Vvemh4QHN5c3VjYy5vcmcuY24=; Yutian Zou, em91eXRAc3lzdWNjLm9yZy5jbg==
†These authors have contributed equally to this work