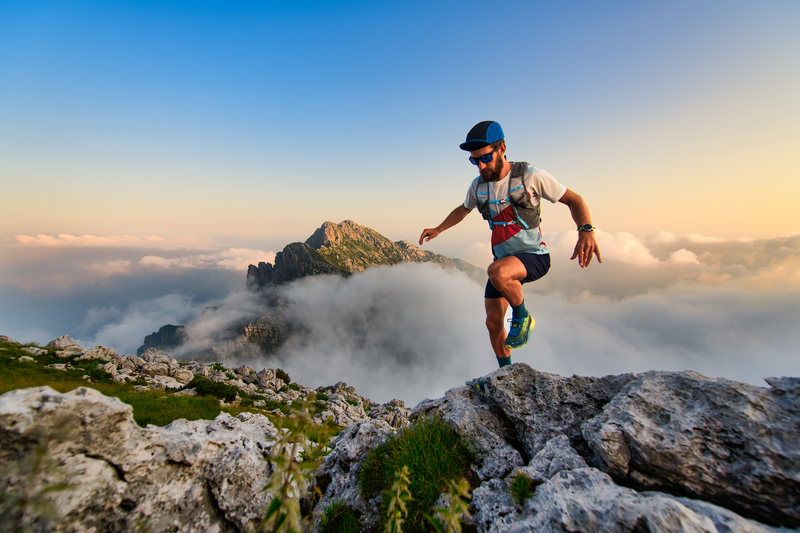
94% of researchers rate our articles as excellent or good
Learn more about the work of our research integrity team to safeguard the quality of each article we publish.
Find out more
ORIGINAL RESEARCH article
Front. Immunol. , 15 December 2022
Sec. Inflammation
Volume 13 - 2022 | https://doi.org/10.3389/fimmu.2022.1065739
Objective: Hyperuricemia and gout have become gradually more common. The effect of serum urate on organism aging and systematic inflammation is not determined. This study aims to evaluate whether serum urate is causally associated with cellular aging markers and serum inflammation markers.
Methods: A Mendelian randomization study was performed on summary-level data from the largest published genome-wide association studies. Single nucleotide polymorphisms with a genome-wide significance level were selected as instrumental variables for leukocyte telomere length (LTL), and serum soluble makers of inflammation (CRP, IL-6, TNF-α, and IGF-1). Standard inverse variance weighted (IVW) method was used as the primary statistical method. The weighted median, MR-Egger regression, and MR-PRESSO methods were used for sensitivity analysis.
Results: An inverse causal association of genetically predicted serum urate levels and LTL was found using IVW method (OR: 0.96, 95%CI 0.95, 0.97; β=-0.040; SE=0.0072; P=4.37×10-8). The association was also supported by MR results using MR-Egger method and weighted median method. The MR-PRESSO analysis and leave-one-out sensitivity analysis supported the robustness of the combined results. In terms of other aging-related serum biomarkers, there was no evidence supporting a causal effect of serum urate on CRP, IL-6, TNF-α, or IGF-1 levels.
Conclusions: Serum urate levels are negatively associated with telomere length but are not associated with serum soluble indicators of inflammation. Telomere length may be a critical marker that reflects urate-related organismal aging and may be a mechanism in the age-related pathologies and mortality caused by hyperuricemia.
Serum urate or uric acid is an end product of purine metabolism (1). High serum urate or hyperuricemia is the most critical risk factor for gout (2). Due to the spread of Western lifestyles and diets associated with excessive meat and alcohol consumption, hyperuricemia has become gradually more common (~20.0%; US; 2016) (3). Moreover, the extensive associations of serum urate with age-related diseases, such as cardiovascular diseases (4, 5), hypertension (6), obesity (6), type 2 diabetes mellitus (7), and metabolic syndrome (8), have been implied. The effects of urate on cellular functions and signaling events, such as promoting the production of reactive oxygen species (ROS) and activating inflammatory signaling pathways, have indicated the possible involvement in systematic inflammation (9–12). Due to its complicated connections within multiple diseases, the role of urate on multiple organ systems that may be facilitated by a perturbation in circulation has been a matter of discussion. Previous studies found the associations between serum urate and serum inflammation markers, such as C-reactive protein (CRP), IL-6, and TNF-α (13). Co-occurrence of high serum urate and high CRP is associated with elevated mortality (14, 15). However, whether these associations are causal remains unclear due to potential biases such as residual confounding and reverse causality.
Telomeres are nucleoprotein structures at the ends of chromosomes that protect linear chromosomes against damage by endogenous nucleases and erroneous recognition as unrepaired chromosomal breaks (16). Due to their critical functions in the maintenance of chromosomal structure and stability (17) and the determination of cell proliferation (18), telomere length has been considered an indicator of the biological age of organisms and a biomarker of aging, stress, and survival (19–21). Telomere shortening is associated with a series of age-related deteriorations and diseases, such as cardiovascular (22) and metabolic diseases (23). Interestingly, a significant negative association between serum urate and peripheral blood aging markers, including leukocyte telomere length (LTL) and mitochondrial DNA copy number (mtDNAcn) (24), has indicated that serum urate may play a role in promoting organismal aging by telomere shortening. Although reduced telomere length and elevated serum urate levels both come with aging, a recent Mendelian randomization (MR) study does not support the casual effect of serum urate on telomere shorten (25). However, the casual association between them remains elusive because of the limited numbers of single nucleotide polymorphisms (SNPs) employed as instrumental variables (IVs) in the previous study.
Here, we used an MR design to overcome the limitations of traditional observational studies and determine whether there are causal associations between serum urate and LTL and serum soluble makers of inflammation (CRP, IL-6, TNF-α, and IGF-1) (26). To detect whether serum urate causally affects LTL, more importantly, we employed a ~4-fold larger number of SNPs as IVs than the previous MR study (25). Confounding and reverse causality can be avoided in MR analysis. This study provides reliable insight into the causal associations between serum urate and the markers of aging.
To assess the causal association of genetically predicted urate level on aging-related biomarkers, we implemented an MR approach, assuming that (i) the SNPs used as instrumental variables (IVs) are robustly associated with urate level; (ii) the SNPs are not associated with any confounder of exposure-outcome associations; (iii) the SNPs exert effects only through urate level rather than via other pathways. For SNPs to instrument urate level, we obtained the results from a published meta-analysis of GWASs (27). Each GWAS in this study has been approved by relevant ethical review committees, all the participants gave written informed consent. Summary-level dataset for outcomes could be obtained from the UK Medical Research Council Integrative Epidemiology Unit (MRCIEU) Open GWAS Project database (http://gwas.mrcieu.au.uk). Therefore, no additional ethical approval is required for our study.
To instrument urate level, we extracted independent SNPs significantly associated with urate level from a published European-ancestry meta-analysis of GWASs (27). The meta-analysis of urate level (n=288,649) initially yielded a total of 123 genome-wide significant SNPs (P<5.0×10-8) using a workflow included methods that used linkage disequilibrium (LD) estimates from an ancestry-matched reference panel (28). Then 114 independent SNPs were identified using stepwise model selection, after LD-based combination into 99 larger genomic regions. These SNPs to instrument urate levels collectively explained 11.4% of the phenotypic variance, compared to 5.3% explained by the index SNPs previously reported from GWAS in European ancestry populations (29). SNPs were removed during the harmonizing process for being palindromic with intermediate allele frequencies. The F-statistics for each SNP was calculated using the previously reported approximation method (30). SNPs with F-statistics lower than 10 were removed from our MR study to avoid weak instrument bias. Previous MR studies have suggested that several factors can causally affect serum urate level, including estimated glomerular filtration rate (eGFR) (31), type 2 diabetes (T2DM) (32), physical activity (33), body mass index (BMI) (32, 34), high-density lipoprotein cholesterol (HDL-C) (32), systolic blood pressure (SBP) (32), and triglyceride (32, 34). Any aforementioned factor that is also causally associated with our study outcomes was deemed as a confounder, and the SNPs having a causal effect on related confounders were removed from the MR analysis. After a rigorous literature search, we found that physical activity was causally associated with both serum urate level and LTL (35), but none of the selected SNPs was significantly associated with any physical activity-associated phenotype. Detailed information of selected SNPs to instrument urate level in our MR study was summarized in Supplementary Tables S1, S2.
Summary-level data (betas and SEs) for mean LTL could be obtained from a publicly available GWAS (36), which was conducted with 488,400 DNA samples of participants in the UK Biobank (UKB). Mean LTL was measured using an established quantitative PCR technique and indicated as a ratio of the telomere repeat number (T) to a single-copy gene (S) (37, 38). After extensive quality checks and adjustments of technical variations, the LTL measurements (T/S ratio) were Z-standardized to allow direct comparison with previous studies. Finally, 472,174 LTL measurements were available and retained for MR study. For serum levels of CRP, IL-6, TNF-α, and IGF-1, we extracted the summary-level data from a GWAS using 3,301 available blood samples from the INTERVAL study (39). The main characteristics of selected GWASs were presented in Table 1.
All the analyses were performed with R (version 4.1.3), TwoSampleMR (0.5.5) (40), Mendelian Randomization (0.5.0) (41), and MR-PRESSO package (42). A scatter plot was generated to help illustrate genetic estimates (Figure 1). For the primary analysis, we used the standard inverse variance weighting (IVW) method under random-effect model, which assumes that each included SNP is a valid instrument (43). The IVW method combines the Wald ratios using a meta-analytic approach, but the estimates of effect could be biased by horizontal pleiotropy in the presence of invalid instruments (44). Heterogeneity between selected SNPs was evaluated using the Cochrane’s Q-statistics, significant heterogeneity could indicate the presence of horizontal pleiotropy (44).
Figure 1 MR plots for the causal association of urate level on LTL. (A) Scatter plot of SNP effect on LTL against that on urate level. The slope of each fitted line represents the combined effect using each MR analysis method. (B) Forest plot of effect from each SNP and pooled MR effect sizes for urate level on LTL. Each dot and its corresponding line represent the effect size and 95%CI. Each dot and its corresponding line represent the pooled estimates after the removal of the corresponding SNP. LTL, leukocyte telomere length; SNP, single nucleotide polymorphism; MR, mendelian randomization.
The weighted median method, MR-Egger regression, and MR-PRESSO methods, which relax some IV assumptions were performed as sensitivity analyses. The weighted median method provides consistent estimates of causal effect when more than half of the instruments are valid (30). Confidence should be given to the weighted median method when the heterogeneity is significant and the selected SNPs are free of pleiotropy. The MR-Egger regression analysis estimates the causal effect by calculating the slope from the weighted regression of the IVs-outcome associations on the IVs-exposure associations (30). The Egger intercept indicates average pleiotropic effect, and a non-zero intercept indicates significant pleiotropy (42). In case of significant pleiotropy, we used the PhenoScanner V2 to rule out SNPs associated with potential confounders at a genome-wide significance (P<5.0×10-8) (45, 46). The results obtained using IVW method were deemed as the most reliable if the selected instruments are free of directional pleiotropy (P for MR-Egger intercept >0.05) (47). The MR-PRESSO approach not only detects pleiotropy by identifying outlying SNPs, but also provides outliers-corrected estimates of causal effect (42). The P value for MR-PRESSO distortion test was used to denote a significant difference in estimates before and after the correction of outlying SNPs (42). The leave-one-out sensitivity analysis was also performed to test the robustness of results. In addition, the power of our MR study was calculated according to the previously published method to test whether our study had sufficient power to determine a clinically significant association between serum urate level and study outcomes (48).
Detailed information of selected 101 SNPs to instrument urate level was summarized in Supplementary Table 1. The F-statistics of these selected SNPs ranged from 62.9 to 5561.5, which were much higher than the conventional threshold of 10, suggesting a low possibility of weak-instrument bias. Based on a sample size of 472,174 individuals and the SNPs explaining 10.21% of the phenotypic variance, our study had 100% power to detect an OR of 1.20 (β=-0.2231, Table 1). The results of our MR study suggested an inverse association between urate level and LTL. The scatter plot of the association between genetically predicted urate level and LTL was shown in Figure 1A, where the slope of each fitted line represented the combined effect using each MR analysis method. Using the IVW method, genetically predicted urate level was negatively associated with LTL (0.040 SD higher urate per 1-SD shorter LTL, P=4.37×10-8; Table 1). The inverse causal association was also supported by MR results using MR-Egger method and weighted median method (Figure 1A and Table 2). As shown in Figure 1, the MR-Egger intercept was close to zero (MR-Egger intercept=0.00018), and the MR-Egger regression analysis revealed no horizontal pleiotropy (P-pleio=0.76). We observed significant heterogeneity across different SNPs (P-het=1.34×10-15), thus the random-effect model was used (forest plot shown in Figure 1B). The MR-PRESSO approach identified four outlying SNPs, but the P value for distortion test (P=1.00) suggested that there was no significant difference between the original estimate and the outlier-corrected estimate of the causal effect. The leave-one-out sensitivity analysis also supported the robustness of the conclusion (Supplementary Figure 1).
Table 2 Causal effects of urate level on leukocyte telomere length and inflammation markers using MR analyses.
In terms of inflammation markers, including serum levels of CRP, IL-6, TNF-α, and IGF-1, none of our analyses suggested a causal effect of urate level (Table 2). Based upon a sample size of 3,301 individuals and the phenotypic variance explained to 11.24%, our study had 99%/94% power to detect an OR of 0.80/1.20 (Table 1).
Although basic research and clinical investigations have strongly indicated the implications of urate in organismal aging, its causal association with aging is not determined. In conflict with the previous MR study which reported that serum urate has no significant causal effect on LTL (25), we found that serum urate levels are reversely associated with LTL. The possible reason is that we employed a ~4-fold larger number of SNPs as IVs than the previous MR study. Moreover, the sample size of the GWAS we used in this study (27) is 4-fold larger than the previous GWAS studies (29, 49, 50). The post hoc power calculation suggested that our study had 100% power to detect an OR of 0.80/1.20, while the previous MR did not.
Since serum urate increases with age (51, 52), this result suggests that increased serum urate results in telomere shortening during aging. Telomere shortening is a well-documented hallmark of both cellular senescence and organismal aging, and is a critical mechanism of age-related pathologies and mortality (53). It happens in each division as a normal cellular process in the dividing cells (54). Moreover, telomere shortening occurs due to oxidative damage and other end processing events in both dividing and non-dividing cells (20). The effects of urate on cellular stress and ROS production may be the mechanism fascinating the urate-induced telomere shortening found in this study. ROS are produced simultaneously with the formation of intracellular urate by xanthine oxidases (XO) (55). Enhanced activity of XO promotes intracellular urate production. Moreover, elevated extracellular urate concentration aggravates the urate flux into cells. These events cause the accumulation of intracellular urate that aggravates ROS production (56, 57), mitochondrial damage (58), endoplasmic reticulum stress (59), and apoptosis (56). These cellular events cause telomere shortening alone or in an interactive manner; in turn, telomere shortening further aggravates cell stress, forming a closed loop (20, 60).
The role of intracellular urate has been demonstrated that it can trigger inflammation and ROS. However, we did not find a causal effect of serum urate on the markers of systematic inflammation. Previous studies have observed the associations between serum urate and serum inflammation indicators (13, 61, 62). Our study indicates that these associations are not causal. The possible interpretation of these conflict findings may be the paradox of oxidant versus anti-oxidant properties of urate in multiple systems (9) and the net effect of urate on system is variable depending on various conditions, such as genetics and disease progress.
MR studies rely on strong assumptions, and biased and misleading results could be yielded if those assumptions fail (63). In our current study, a total of 114 SNPs significantly associated with serum urate level were obtained from the largest GWAS meta-analysis, which was in line with the Relevance assumption. We then performed a comprehensive literature search to identify potential confounders that affect the exposure-outcome association. Only physical activity was deemed as a confounder through the literature search, but none of our selected SNPs was significantly associated with any physical activity-associated phenotype. It should be noted, if a particular confounder was simply not considered as a confounder in the analysis, its effect could not be accounted for. We made an effort to reduce the possibility that the Independence assumption was violated. Furthermore, we used three robust analysis methods (weighted median method, MR-Egger regression, and MR-PRESSO) as sensitivity analysis, since robust methods can provide valid causal inferences under weaker assumptions than the standard IVW method (44). Strikingly, causal estimates from all methods were similar (Table 1), and the concordance of estimates from different methods made the causal claim more credible.
This study has several notable strengths. First, it is the first study revealing the causal association of serum urate with telomere length and highlighting the value of telomere length as a reliable serum marker reflecting urate-related aging. Second, an integrated and comprehensive MR analysis was conducted to support the robustness of causal estimates. A series of sensitivity analyses was also employed to ensure the consistency of causal association. Third, the number of SNPs employed as IVs is relatively large, enhancing the reliability of the conclusions.
Some limitations in this study need to be considered. First, the participants involved in the study were all of European descent, which may restrict the generalizability of the findings. Second, since a linear relationship between exposure and outcome was assumed in the MR design, the potential non-linear association of serum urate with leukocyte telomere length and inflammation markers could not be evaluated. Finally, other aging-related markers, such as immune parameters and indices of epigenetic age, were not included in this study.
Serum urate levels are negatively associated with telomere length but are not associated with inflammation markers. Telomere length may be a critical and reliable marker that reflects urate-related organismal aging and may be a mechanism in the age-related pathologies and mortality caused by hyperuricemia.
The original contributions presented in the study are included in the article/Supplementary Material. Further inquiries can be directed to the corresponding authors.
ZL: conception and design, data analysis, and draft writing. JC: draft writing. JZ: draft writing, and revision. All authors approved the final manuscript.
The authors declare that the research was conducted in the absence of any commercial or financial relationships that could be construed as a potential conflict of interest.
All claims expressed in this article are solely those of the authors and do not necessarily represent those of their affiliated organizations, or those of the publisher, the editors and the reviewers. Any product that may be evaluated in this article, or claim that may be made by its manufacturer, is not guaranteed or endorsed by the publisher.
The Supplementary Material for this article can be found online at: https://www.frontiersin.org/articles/10.3389/fimmu.2022.1065739/full#supplementary-material
1. Maiuolo J, Oppedisano F, Gratteri S, Muscoli C, Mollace V. Regulation of uric acid metabolism and excretion. Int J Cardiol (2016) 213:8–14. doi: 10.1016/j.ijcard.2015.08.109
2. Dalbeth N, Gosling AL, Gaffo A, Abhishek A. Gout. Lancet (2021) 397:1843–55. doi: 10.1016/S0140-6736(21)00569-9
3. Chen-Xu M, Yokose C, Rai SK, Pillinger MH, Choi HK. Contemporary prevalence of gout and hyperuricemia in the United States and decadal trends: The national health and nutrition examination survey, 2007-2016. Arthritis Rheumatol (2019) 71:991–9. doi: 10.1002/art.40807
4. Gill D, Cameron AC, Burgess S, Li X, Doherty DJ, Karhunen V, et al. Urate, blood pressure, and cardiovascular disease: Evidence from mendelian randomization and meta-analysis of clinical trials. Hypertension (2021) 77:383–92. doi: 10.1161/HYPERTENSIONAHA.120.16547
5. Yang F, Hu T, Cui H. Serum urate and heart failure: A bidirectional mendelian randomisation study. Eur J Prev Cardiol (2022) 29(11):1570–8. doi: 10.1093/eurjpc/zwac100
6. Sanchez-Lozada LG, Rodriguez-Iturbe B, Kelley EE, Nakagawa T, Madero M, Feig DI, et al. Uric acid and hypertension: An update with recommendations. Am J Hypertens (2020) 33:583–94. doi: 10.1093/ajh/hpaa044
7. Johnson RJ, Nakagawa T, Sanchez-Lozada LG, Shafiu M, Sundaram S, Le M, et al. Sugar, uric acid, and the etiology of diabetes and obesity. Diabetes (2013) 62:3307–15. doi: 10.2337/db12-1814
8. King C, Lanaspa MA, Jensen T, Tolan DR, Sanchez-Lozada LG, Johnson RJ. Uric acid as a cause of the metabolic syndrome. Contrib Nephrol (2018) 192:88–102. doi: 10.1159/000484283
9. Copur S, Demiray A, Kanbay M. Uric acid in metabolic syndrome: Does uric acid have a definitive role? Eur J Intern Med (2022) 103:4–12. doi: 10.1016/j.ejim.2022.04.022
10. Clavijo-Cornejo D, Hernandez-Gonzalez O, Gutierrez M. The current role of NLRP3 inflammasome polymorphism in gout susceptibility. Int J Rheumatic Dis (2021) 24:1257–65. doi: 10.1111/1756-185X.14205
11. Shen J, Xie Z, Liu Y, Zhao T, Li Z, Ren Y, et al. Aberrant messenger RNA expression in peripheral blood mononuclear cells is associated with gouty arthritis. Int J Rheumatic Dis (2022) 25:1152–63. doi: 10.1111/1756-185X.14395
12. Li G, Zhang H, Ma H, Qu S, Xing Q, Wang G. MiR-221-5p is involved in the regulation of inflammatory responses in acute gouty arthritis by targeting IL-1beta. Int J Rheumatic Dis (2021) 24:335–40. doi: 10.1111/1756-185X.14028
13. Luis-Rodriguez D, Donate-Correa J, Martin-Nunez E, Ferri C, Tagua VG, Perez Castro A, et al. Serum urate is related to subclinical inflammation in asymptomatic hyperuricaemia. Rheumatol (Oxford) (2021) 60:371–9. doi: 10.1093/rheumatology/keaa425
14. Li S, Cui L, Cheng J, Shu R, Chen S, Nguyen US, et al. Repeated measurements of serum urate and mortality: A prospective cohort study of 152,358 individuals over 8 years of follow-up. Arthritis Res Ther (2020) 22:84. doi: 10.1186/s13075-020-02173-4
15. Dao Bui Quy Q, Pham Ngoc Huy T, Nguyen Minh T, Nguyen Duc L, Nguyen Minh T, Nguyen Trung K, et al. High serum uric acid and high-sensitivity c reactive protein concentrations predict three-year cardiovascular mortality in patients treated with continuous ambulatory peritoneal dialysis. Cureus (2021) 13:e17900. doi: 10.7759/cureus.17900
16. Prochazkova Schrumpfova P, Schorova S, Fajkus J. Telomere- and telomerase-associated proteins and their functions in the plant cell. Front Plant Sci (2016) 7:851. doi: 10.3389/fpls.2016.00851
17. Aksenova AY, Mirkin SM. At The beginning of the end and in the middle of the beginning: Structure and maintenance of telomeric DNA repeats and interstitial telomeric sequences. Genes (Basel) (2019) 10(2):118. doi: 10.3390/genes10020118
18. Shay JW. Role of telomeres and telomerase in aging and cancer. Cancer Discov (2016) 6:584–93. doi: 10.1158/2159-8290.CD-16-0062
19. Turner KJ, Vasu V, Griffin DK. Telomere biology and human phenotype. Cells (2019) 8(1):73. doi: 10.3390/cells8010073
20. Lin J, Epel E. Stress and telomere shortening: Insights from cellular mechanisms. Ageing Res Rev (2022) 73:101507. doi: 10.1016/j.arr.2021.101507
21. Liu J, Wang L, Wang Z, Liu JP. Roles of telomere biology in cell senescence, replicative and chronological ageing. Cells (2019) 8(1):54. doi: 10.3390/cells8010054
22. Nilsson PM, Tufvesson H, Leosdottir M, Melander O. Telomeres and cardiovascular disease risk: An update 2013. Transl Res (2013) 162:371–80. doi: 10.1016/j.trsl.2013.05.004
23. Cheng F, Carroll L, Joglekar MV, Januszewski AS, Wong KK, Hardikar AA, et al. Diabetes, metabolic disease, and telomere length. Lancet Diabetes Endocrinol (2021) 9:117–26. doi: 10.1016/S2213-8587(20)30365-X
24. Yu J, Liu H, He S, Li P, Ma C, Ping F, et al. Negative association of serum URIC acid with peripheral blood cellular aging markers. J Nutr Health Aging (2019) 23:547–51. doi: 10.1007/s12603-019-1200-3
25. Mazidi M, Shekoohi N, Katsiki N, Rakowski M, Mikhailidis DP, Banach M. Serum anti-inflammatory and inflammatory markers have no causal impact on telomere length: A mendelian randomization study. Arch Med Sci (2021) 17:739–51. doi: 10.5114/aoms/119965
26. Wagner KH, Cameron-Smith D, Wessner B, Franzke B. Biomarkers of aging: From function to molecular biology. Nutrients (2016) 8. doi: 10.3390/nu8060338
27. Tin A, Marten J, Halperin Kuhns VL, Li Y, Wuttke M, Kirsten H, et al. Target genes, variants, tissues and transcriptional pathways influencing human serum urate levels. Nat Genet (2019) 51:1459–74. doi: 10.1038/s41588-019-0504-x
28. Benner C, Havulinna AS, Jarvelin MR, Salomaa V, Ripatti S, Pirinen M. Prospects of fine-mapping trait-associated genomic regions by using summary statistics from genome-wide association studies. Am J Hum Genet (2017) 101:539–51. doi: 10.1016/j.ajhg.2017.08.012
29. Kottgen A, Albrecht E, Teumer A, Vitart V, Krumsiek J, Hundertmark C, et al. Genome-wide association analyses identify 18 new loci associated with serum urate concentrations. Nat Genet (2013) 45:145–54. doi: 10.1038/ng.2500
30. Bowden J, Del Greco MF, Minelli C, Davey Smith G, Sheehan NA, Thompson JR. Assessing the suitability of summary data for two-sample mendelian randomization analyses using MR-egger regression: The role of the I2 statistic. Int J Epidemiol (2016) 45:1961–74. doi: 10.1093/ije/dyw220
31. Park S, Lee S, Kim Y, Cho S, Huh H, Kim K, et al. Mendelian randomization reveals causal effects of kidney function on various biochemical parameters. Commun Biol (2022) 5:713. doi: 10.1038/s42003-022-03659-4
32. Yang Y, Xian W, Wu D, Huo Z, Hong S, Li Y, et al. The role of obesity, type 2 diabetes, and metabolic factors in gout: A mendelian randomization study. Front Endocrinol (Lausanne) (2022) 13:917056. doi: 10.3389/fendo.2022.917056
33. Guan Y, Wei J, Meng L, Li Y, Wang T, Chen D, et al. Genetically predicted physical activity is associated with lower serum urate concentrations. Genes Genomics (2022) 44:843–53. doi: 10.1007/s13258-022-01239-8
34. Peng L, Jing J, He S, Wang J, Gao X, Wang T. The role of lipid traits in mediating the effect of body mass index on serum urate. Front Endocrinol (Lausanne) (2022) 13:938891. doi: 10.3389/fendo.2022.938891
35. Dempsey PC, Musicha C, Rowlands AV, Davies M, Khunti K, Razieh C, et al. Investigation of a UK biobank cohort reveals causal associations of self-reported walking pace with telomere length. Commun Biol (2022) 5:381. doi: 10.1038/s42003-022-03323-x
36. Codd V, Wang Q, Allara E, Musicha C, Kaptoge S, Stoma S, et al. Polygenic basis and biomedical consequences of telomere length variation. Nat Genet (2021) 53:1425–33. doi: 10.1038/s41588-021-00944-6
37. Cawthon RM. Telomere measurement by quantitative PCR. Nucleic Acids Res (2002) 30:e47. doi: 10.1093/nar/30.10.e47
38. Cawthon RM. Telomere length measurement by a novel monochrome multiplex quantitative PCR method. Nucleic Acids Res (2009) 37:e21. doi: 10.1093/nar/gkn1027
39. Sun BB, Maranville JC, Peters JE, Stacey D, Staley JR, Blackshaw J, et al. Genomic atlas of the human plasma proteome. Nature (2018) 558:73–9. doi: 10.1038/s41586-018-0175-2
40. Hemani G, Zheng J, Elsworth B, Wade KH, Haberland V, Baird D, et al. The MR-base platform supports systematic causal inference across the human phenome. Elife (2018) 7. doi: 10.7554/eLife.34408
41. Yavorska OO, Burgess S. MendelianRandomization: an r package for performing mendelian randomization analyses using summarized data. Int J Epidemiol (2017) 46:1734–9. doi: 10.1093/ije/dyx034
42. Verbanck M, Chen CY, Neale B, Do R. Detection of widespread horizontal pleiotropy in causal relationships inferred from mendelian randomization between complex traits and diseases. Nat Genet (2018) 50:693–8. doi: 10.1038/s41588-018-0099-7
43. Burgess S, Butterworth A, Thompson SG. Mendelian randomization analysis with multiple genetic variants using summarized data. Genet Epidemiol (2013) 37:658–65. doi: 10.1002/gepi.21758
44. Burgess S, Bowden J, Fall T, Ingelsson E, Thompson SG. Sensitivity analyses for robust causal inference from mendelian randomization analyses with multiple genetic variants. Epidemiology (2017) 28:30–42. doi: 10.1097/EDE.0000000000000559
45. Staley JR, Blackshaw J, Kamat MA, Ellis S, Surendran P, Sun BB, et al. PhenoScanner: a database of human genotype-phenotype associations. Bioinformatics (2016) 32:3207–9. doi: 10.1093/bioinformatics/btw373
46. Kamat MA, Blackshaw JA, Young R, Surendran P, Burgess S, Danesh J, et al. PhenoScanner V2: An expanded tool for searching human genotype-phenotype associations. Bioinformatics (2019) 35:4851–3. doi: 10.1093/bioinformatics/btz469
47. Holmes MV, Ala-Korpela M, Smith GD. Mendelian randomization in cardiometabolic disease: Challenges in evaluating causality. Nat Rev Cardiol (2017) 14:577–90. doi: 10.1038/nrcardio.2017.78
48. Brion MJ, Shakhbazov K, Visscher PM. Calculating statistical power in mendelian randomization studies. Int J Epidemiol (2013) 42:1497–501. doi: 10.1093/ije/dyt179
49. Sulem P, Gudbjartsson DF, Walters GB, Helgadottir HT, Helgason A, Gudjonsson SA, et al. Identification of low-frequency variants associated with gout and serum uric acid levels. Nat Genet (2011) 43:1127–30. doi: 10.1038/ng.972
50. Kanai M, Akiyama M, Takahashi A, Matoba N, Momozawa Y, Ikeda M, et al. Genetic analysis of quantitative traits in the Japanese population links cell types to complex human diseases. Nat Genet (2018) 50:390–400. doi: 10.1038/s41588-018-0047-6
51. Stiburkova B, Bleyer AJ. Changes in serum urate and urate excretion with age. Adv Chronic Kidney Dis (2012) 19:372–6. doi: 10.1053/j.ackd.2012.07.010
52. Zitt E, Fischer A, Lhotta K, Concin H, Nagel G. Sex- and age-specific variations, temporal trends and metabolic determinants of serum uric acid concentrations in a large population-based Austrian cohort. Sci Rep (2020) 10:7578. doi: 10.1038/s41598-020-64587-z
53. Vaiserman A, Krasnienkov D. Telomere length as a marker of biological age: State-of-the-Art, open issues, and future perspectives. Front Genet (2020) 11:630186. doi: 10.3389/fgene.2020.630186
54. Shammas MA. Telomeres, lifestyle, cancer, and aging. Curr Opin Clin Nutr Metab Care (2011) 14:28–34. doi: 10.1097/MCO.0b013e32834121b1
55. Kimura Y, Tsukui D, Kono H. Uric acid in inflammation and the pathogenesis of atherosclerosis. Int J Mol Sci (2021) 22. doi: 10.3390/ijms222212394
56. Shen S, He F, Cheng C, Xu B, Sheng J. Uric acid aggravates myocardial ischemia-reperfusion injury via ROS/NLRP3 pyroptosis pathway. BioMed Pharmacother (2021) 133:110990. doi: 10.1016/j.biopha.2020.110990
57. Demiray A, Afsar B, Covic A, Kuwabara M, Ferro CJ, Lanaspa MA, et al. The role of uric acid in the acute myocardial infarction: A narrative review. Angiology (2022) 73:9–17. doi: 10.1177/00033197211012546
58. Yang Y, Zhou Y, Cheng S, Sun JL, Yao H, Ma L. Effect of uric acid on mitochondrial function and oxidative stress in hepatocytes. Genet Mol Res (2016) 15. doi: 10.4238/gmr.15028644
59. Yan M, Chen K, He L, Li S, Huang D, Li J. Uric acid induces cardiomyocyte apoptosis via activation of calpain-1 and endoplasmic reticulum stress. Cell Physiol Biochem (2018) 45:2122–35. doi: 10.1159/000488048
60. Barnes RP, Fouquerel E, Opresko PL. The impact of oxidative DNA damage and stress on telomere homeostasis. Mech Ageing Dev (2019) 177:37–45. doi: 10.1016/j.mad.2018.03.013
61. Fayed A, El Nokeety MM, Heikal AA, Sadek KM, Hammad H, Abdulazim DO, et al. Urine albumin and serum uric acid are important determinants of serum 25 hydroxyvitamin d level in pre-dialysis chronic kidney disease patients. Ren Fail (2019) 41:540–6. doi: 10.1080/0886022X.2018.1563552
62. Liu F, Wang Y, Zhao Q, Zhang M, Ban B. Association between uric acid and insulin-like growth factor-1 in type 2 diabetes mellitus. Int J Gen Med (2021) 14:4017–23. doi: 10.2147/IJGM.S323579
Keywords: serum urate, telomere length, aging, causal effect, Mendelian randomization
Citation: Lv Z, Cui J and Zhang J (2022) Associations between serum urate and telomere length and inflammation markers: Evidence from UK Biobank cohort. Front. Immunol. 13:1065739. doi: 10.3389/fimmu.2022.1065739
Received: 10 October 2022; Accepted: 28 November 2022;
Published: 15 December 2022.
Edited by:
James Cheng-Chung Wei, Chung Shan Medical University Hospital, TaiwanReviewed by:
Chih-Wei Chen, Chung Shan Medical University, TaiwanCopyright © 2022 Lv, Cui and Zhang. This is an open-access article distributed under the terms of the Creative Commons Attribution License (CC BY). The use, distribution or reproduction in other forums is permitted, provided the original author(s) and the copyright owner(s) are credited and that the original publication in this journal is cited, in accordance with accepted academic practice. No use, distribution or reproduction is permitted which does not comply with these terms.
*Correspondence: Jiarui Cui, anJmZmZhaXJ5YmFiaUAxNjMuY29t; Jiaming Zhang, amlhbWluZ196aGFuZ3RqbWNAaWNsb3VkLmNvbQ==
†ORCID: Jiaming Zhang, orcid.org/0000-0001-8520-566X
Disclaimer: All claims expressed in this article are solely those of the authors and do not necessarily represent those of their affiliated organizations, or those of the publisher, the editors and the reviewers. Any product that may be evaluated in this article or claim that may be made by its manufacturer is not guaranteed or endorsed by the publisher.
Research integrity at Frontiers
Learn more about the work of our research integrity team to safeguard the quality of each article we publish.