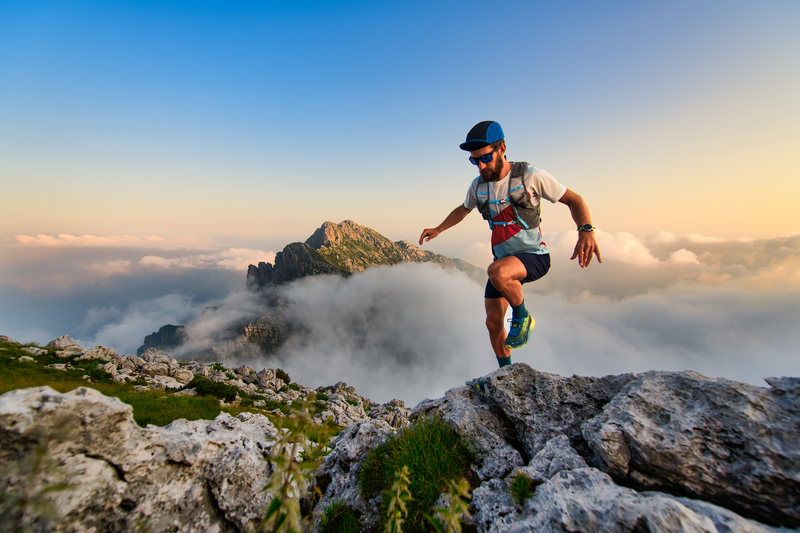
95% of researchers rate our articles as excellent or good
Learn more about the work of our research integrity team to safeguard the quality of each article we publish.
Find out more
ORIGINAL RESEARCH article
Front. Immunol. , 01 December 2022
Sec. Cancer Immunity and Immunotherapy
Volume 13 - 2022 | https://doi.org/10.3389/fimmu.2022.1060051
This article is part of the Research Topic Biomarkers and Immunotherapy of Hepatic-Biliary-Pancreatic Cancers View all 53 articles
Background: The combination of immune checkpoint inhibitors (ICIs) and anti-angiogenic agents has shown promising efficacy in unresectable hepatocellular carcinoma (HCC), but until now no clinical prognostic models or predictive biomarkers have been established.
Methods: From 2016 to 2021, a total of 258 HCCs treated with ICIs and tyrosine kinase inhibitors (TKIs) were retrospectively enrolled, as the study cohort. Patients’ baseline data was extracted by least absolute and shrinkage selection operator (LASSO) and Cox regression. Finally, a prognostic model in the form of nomogram was developed. Model performance was assessed in terms of discrimination, calibration, and clinical utility. A 5-fold cross-validation was used to evaluate the internal repeatability of the model. In addition, the patient cohort was divided into three subgroups according to nomogram scores. Their survivals were estimated by Kaplan-Meier methods and the differences were analyzed using log-rank tests.
Results: Seven clinical parameters were selected: Eastern Cooperative Oncology Group performance status (ECOG PS), combination of transarterial chemoembolization (TACE), extrahepatic metastasis (EHM), platelet to lymphocyte ratio (PLR), alanine aminotransferase (ALT), alpha-fetoprotein (AFP), and Child-Pugh score. The model had an area under the curve (AUC) of 0.777 at 1 year and 0.772 at 2 years. Receiver operating characteristic (ROC) curve, calibration curve and decision curve analysis (DCA) showed that the discrimination, consistency and applicability of the model were good. In addition, cross-validation validated the discrimination of the model, and the C index value of the model is 0.7405. The median overall survival (OS) of the high-, medium- and low-risk subgroups was 7.58, 17.50 and 53.17 months, respectively, with a significant difference between the groups (P < 0.0001).
Conclusion: We developed a comprehensive and simple prognostic model for the combination of ICIs plus TKIs. And it may predict the efficacy of the combination regimen for unresectable HCC.
Primary liver cancer is ranked the sixth of cancer morbidity and the third of cancer mortality worldwide (1). And hepatocellular carcinoma (HCC) is the main pathological type of liver cancer (2). HCC has a poor prognosis, possibly due to untimely diagnosis and complex tumor microenvironment (3, 4). Sorafenib was the first approved front-line tyrosine kinase inhibitor (TKI) for unresectable HCC (5, 6). In recent years, four other TKIs (lenvatinib, regorafenib, cabozantinib, and ramucirumab) have been used for first-line or second-line treatment of HCC (7–10). While immune checkpoint inhibitors (ICIs) have shown clinical prospects in other cancers, these programmed cell death protein 1 (PD-1) monoclonal antibodies including nivolumab, pembrolizumab, camrelizumab and sintilimab, are approved in advanced HCC based on clinical trials (11–14). In the light of the synergic efficacy for the combination of anti-angiogenic TKIs or monoclonal antibody plus ICIs, the combination regimens have been validated and approved (15, 16). More notably, IMbrave150 and ORIENT-32 showed that the combination of ICIs and anti-angiogenic agents resulted in longer overall survival (OS) of 19.2 months and progression-free survival (PFS) of 6.9 months compared with mono-sorafenib (17, 18). Though with the increasing evidence for ICIs plus TKIs in advanced HCC, the prognosis of advanced HCC varies a lot and clinical predictive biomarkers are limited (15). Considering that some patients could not benefit at all, they would be screened by the prognostic model to further guide clinical choice.
As for the prognostic biomarkers, unlike other malignancy, tumor mutation burden (TMB) and programmed cell death protein ligand-1 (PD-L1) expression seems less predictive in HCC (15, 19, 20). The diversity of response to treatment and the potential severe adverse events makes the discovery of biomarkers of great clinical importance. Radiomics nomograms have achieved good predictive performance, with an area under the receiver operating characteristic (ROC) curve (AUC) of 0.880, but the acquisition and analysis of images often require professional radiologists and professional equipment (21, 22). Two radiomics models involved a sample size of less than 60 and uncertain treatment regimens, either mono-ICIs or the combination of anti-angiogenic agents plus ICI. Genomics utilizes tumor tissue for testing and it is invasive (23–25). Although these clinical predictive models are convenient to use, none of the current models involve clinical inflammatory factors (15). Indeed, the tumor microenvironment is closely related to the prognosis of HCC, and neutrophil-to-lymphocyte ratio (NLR), platelet-to-lymphocyte ratio (PLR), lymphocyte-to-monocyte ratio (LMR), prognostic nutritional index (PNI), and systemic immune inflammatory (SII) index are representative systemic inflammatory markers (26–29). The comprehensive model including the above factors could be developed, thus, the patients with poor prognosis will be promptly identified and invalid treatment might be avoided.
Therefore, this study retrospectively analyzed unresectable HCC treated with ICIs combined with TKIs. The predictive factors affecting patient survival were explored and a prognostic model for advanced HCC in Chinese population was developed, in whom HBV-infection was the main etiology.
Patients with unresectable HCC were retrospectively enrolled, who received PD-1 inhibitors and anti-angiogenic agents at Beijing Ditan Hospital, Capital Medical University from 2016 to 2021. The study conformed to the 1975 Declaration of Helsinki and has been approved by the Ethics Review Committee of Beijing Ditan Hospital, Capital Medical University. All patients provided written informed consent.
Inclusion criteria for this study included: (1) aged ≥ 18 years; (2) HCC diagnosed by imaging or pathological examination; (3) Child-Pugh class A or B; (4) Eastern Cooperative Oncology Group performance status (ECOG PS) < 2; (5) BCLC stage C or B unable to tolerate radical treatment. Meanwhile, the following patients were excluded: (1) combined with a history of other malignancy outside HCC or metastatic liver tumor; (2) pregnant or lactating women; (3) severe organ or blood dysfunction; (4) abdominal computed tomography (CT) and/or magnetic resonance imaging (MRI) were not performed. (5) loss of follow-up.
Patients’ clinical data were queried by electronic medical records, mainly including age, gender, ECOG PS, etiology (“HBV”or”HCV”or”other”), previous and present treatment (surgery, ablation, transarterial chemoembolization (TACE) and treatment line), Child-Pugh class and BCLC stage. In addition, imaging data (tumor number, tumor size, portal vein tumor thrombus (PVTT), extrahepatic metastasis (EHM)) and laboratory parameters (peripheral blood count, liver function, and tumor marker AFP) were collected before the start of treatment, and NLR (absolute neutrophil count/absolute lymphocyte count), PLR (absolute platelet count/absolute lymphocyte count), LMR (absolute lymphocyte count/absolute monocyte count), PNI (serum albumin + 0.5 * absolute lymphocyte count), and SII (absolute neutrophil count * platelet count/absolute lymphocyte count) were calculated.
All patients were treated with ICIs, and the selected PD-1 inhibitors mainly included camrelizumab (Hengrui Medicine, China) and sintilimab (Innovent Biologics, China), 200 mg intravenously every three weeks. In addition, the above patients could be included in this study whether they were TKI ‐ naïve or TKI ‐ experienced. The TKIs involved were sorafenib (Bayer Pharma AG, Germany) 400-800 mg/day orally; lenvatinib (Eisai Co., Ltd., Japan) 8 mg/day for body weight < 60 kg and 12 mg/day orally for body weight ≥ 60 kg; and regorafenib (Bayer Pharma AG, Germany) 160 mg/day orally every 4 weeks 1-3. Patient management was to modify dose and interrupt treatment according to local drug regulations.
Response to treatment and questioning survival status were assessed every 6-12 weeks after the start of treatment, and medication modifications were documented.
Histograms were plotted to view the data distribution, and logarithmic transformation was performed for continuous variables with a clearly skewed distribution. Alternative predictors were screened by least absolute shrinkage and selection operator (LASSO) regression, and then independent predictors were selected by multivariate Cox regression (30). Based on the above results, a nomogram was constructed as the final model. Model performance was then assessed by ROC curve, calibration curve, and decision curve analysis (DCA). ROC curve measures the discriminatory power of the model, and AUC value quantitatively describes discrimination, with 0.5 indicating no discriminatory power and 1 indicating the best discriminatory power (31). The consistency between the model predicted risk and the actual risk is represented by the calibration curve, and the closer to the slope line, the better the calibration (32). DCA curves assess the clinical utility of the model, with greater area under the curve having better applicability (33). To test the reproducibility of the model, we intend to use a 5-fold cross-validation. We randomly selected 80% of patients as the training set, and the rest as the validation set, calculate AUC value, repeat 1000 times, and record the results in box plot and scatter plot. A total score was calculated for each patient based on the nomogram assigned ratio and divided into three risk groups: low, medium, and high using X-tile software.
All statistical analyses were implemented by R software (version 4.1.1, Chicago, IL). Quantitative data were presented as median (range), while qualitative data were presented as number (percentage). OS was calculated from the start of treatment until death or last follow-up, survival curves were plotted by Kaplan-Meier method, and median survival (mOS) and 95% confidence interval (95% CI) were reported. Log-rank test was used when comparing different risk groups and P < 0.05 was considered statistically significant. The software packages involved in this study were “ggplot2”, “survminer”, “survival”, “glmnet”, “rms”, “riskRegression”, “dcurves”, “caret”, “nomogramFormula” and “dplyr”.
Two hundred and fifty-eight patients treated with ICIs and TKIs were recruited retrospectively in this study (Figure 1). Patients’ baseline characteristics are shown in Table 1, with a median age of 59 (range, 51-65) years, 39 (15.1%) women and 219 (84.9%) men. And the majority of HCC was caused by HBV infection (n = 230, 89.1%). Ninety-seven (37.6%) patients had ECOG PS of 1, 157 (60.9%) had intrahepatic tumors > 3, 157 (60.9%) had maximum tumor diameter > 5 cm, and 76 (29.5%) had Child-Pugh class B. Overall, 195 (75.6%) patients were in BCLC stage C and had indications for PD-1 inhibitors and anti-angiogenic agents. In addition, although one-quarter of patients were in the middle stage of HCC, progression after TACE required the combination of systemic therapy. Other treatment history before ICI varied, with 40 (15.5%) and 109 (42.2%) cases receiving surgery and ablation, respectively, and 225 (87.2%) cases had combined TACE, 109 (42.2%) patients were first-line and the rest were later-line. In addition, 137 (53.1%) patients had PVTT and 101 (39.1%) had EHM. Until the last follow-up, a total of 97 (37.6%) patients had died, with a mOS of 30.2 (95% CI, 25.1 – 40.8) months, and their survival curves are shown in Figure 2.
Histograms were used to view the data distribution of continuous variables. NLR, PLR, SII, alanine aminotransferase (ALT), aspartate aminotransferase (AST) and AFP showed a significantly skewed distribution, and logarithms were taken for the above factors. While other variables (age, LMR, PNI and albumin/globulin ratio (A/G)) were not converted. Eleven variables were selected by lambda values corresponding to partial Likelihood Deviance in Lasso regression: ECOG PS, the combination of TACE, tumor number, PVTT, EHM, A/G, log (PLR), log (ALT), log (AFP), ALBI class and Child-Pugh score (Figures 3A, B). A stepwise backward regression was then used to refit the model and the Akaike Information Criterion (AIC) minimum was selected as the final model (Supplementary 1). We screened independent predictors of ICI including ECOG PS, combination of TACE, EHM, log (PLR), log (ALT), log (AFP), Child-Pugh score (Supplementary 2), and established nomogram to predict the 1-year and 2-years survival probability of patients (Figure 3C). In addition, to facilitate the application of this model, we construct an online tool: https://xiaomili.shinyapps.io/DynNomapp/.
Figure 3 Result of Lasso regression (A, B) and nomogram for combination regimen for unresectable hepatocellular carcinoma (C).
In all study cohorts, we plotted ROC curve for the model, which had higher AUC value (0.777, 95% CI 0.704 – 0.850) at 1 year and (0.772, 95% CI 0.690 – 0.855) at 2 years, indicating better predictive performance of the nomogram (Figures 4A, B). The calibration curve of this model showed good agreement between prediction and observation in survival probability (Figures 4C, D). According to the DCA curve, the model predicted a clear net benefit of patients compared to “none” or “full”, and the clinical utility was better (Figures 4E, F). In addition, cross-validation showed that the model predicted a median AUC value of 0.7613 (95% CI, 0.7044–0.8185) for 1-year survival and 0.7520 (95% CI, 0.6948–0.8141) for 2-year survival, again demonstrating its discriminant ability (Figures 4G, H). Finally, we calculated the C-index for this predictive model, which has a value of 0.7405.
Figure 4 Receiver operating curve (A, B), calibration curve (C, D), decision curve analysis (E, F) and cross-validation of AUC (G, H) for model predicting survival at 1 year and 2 years.
The nomogram integrated seven variables and assigned a score to each predictor, and the total score was calculated according to the actual situation of the patient. And the corresponding survival probability scale was the survival probability of HCC after combination regimen. To visually demonstrate the power of the constructed model, we stratified the total patient score using X-tile software with cutoff values of 182.7 and 240.3. Thus, the study cohort was divided into three risk groups, the high-risk group consisted of 36 patients and 23 deaths, with a mOS of 7.58 (95% CI, 4.9 – 15.1) months; the medium-risk group consisted of 82 patients and 37 deaths, with a mOS of 17.50 (95% CI, 14.1 – 29.0) months; and finally, the low-risk group included 140 patients and 37 deaths, with a mOS of 53.17 (95% CI, 40.0 – not reached (NR)) months. Kaplan-Meier among the three groups was shown in Figure 5, with significant differences between groups (P < 0.0001).
The advent of ICIs has greatly improved the prognosis of a variety of solid tumors, including HCC, and has shown synergy in combination with anti-angiogenic agents (16). All patients in our study received a combination of ICIs and anti-angiogenic drugs, and the mOS was as long as 30.2 months, far better than mono-PD-1 inhibitor or mono-TKI in previous studies. Considering the etiological differences between HCC patients worldwide and China, the response to the combination regimen of TKIs plus PD-1 inhibitors will also be different. Therefore, we need to construct a prognostic model of unresectable HCC in Chinese populations, in whom the main etiology was HBV infection, which is different from HCV-, alcohol- or NAFLD- related HCC. However, there is an urgent need in the era of precision medicine to explore reliable predictors and identify subgroups of patients who may benefit from the combination therapy. In this study, we included seven variables and constructed a prognostic model in advanced HCC, with good discrimination, calibration, and applicability. The patient population was divided into three subgroups, with mOS of 53.17, 17.50, and 7.58 months in the low-, medium-, and high- risk groups, respectively, and internal validation again demonstrated its accuracy.
Because there are differences in treatment response to the above combination regimen, the identification and integration of molecular markers is currently a problem to be addressed. Nivolumab tends to improve survival and response in HCC with PD-L1 expression ≥ 1%, but heterogeneity of PD-L1 expression and diversity of detection methods limit its utility (19, 34). High TMB status is a predictive biomarker for second-line treatment with pembrolizumab in advanced solid tumors, but genomic instability makes detection difficult (20, 35). Also, neither PD-L1 expression or TMB were determined to predict the efficacy of mono-PD-1 inhibitors. In the era of comprehensive treatment, it is important to select HCC that may benefit from ICIs combined with TKIs by identifying biomarkers and translate into clinical applications.
As one of the common forms of prediction models, nomograms are widely used in tumor prognosis assessment (36). The mechanism of advanced HCC is complex. Its survival is not only associated with patient characteristics, but also with tumor features including tumor immune microenvironment and genic alterations following treatment. We used Lasso regression and multivariate Cox regression to screen variables, and the potential predictors included ECOG PS, combination of TACE, EHM, PLR, ALT, AFP, and Child-Pugh score.
BCLC stage is a commonly used stage in clinical practice, and ECOG PS, EHM and Child-Pugh score included in our model are important components (37). ECOG PS is an important independent prognostic factor for anti-PD-1 therapy in advanced HCC, which is consistent with the results presented in our study (38). Patients with extrahepatic metastasis were defined as BCLC stage C and the difference in organ tumor microenvironment made their different response. As the most common site of extrahepatic metastasis, lung metastasis has been shown to respond best to treatment in two studies (39, 40). Unfortunately, we did not record specific metastatic sites and further exploration of specific responses in different organs is warranted. Most clinical trials have included only Child-Pugh class A patients to minimize the impact of liver dysfunction on outcomes, whereas we have also investigated Child-Pugh calss B patients. Unlike BCLC stage, we divided according to Child-Pugh score, adding more detail information, thereby improving the accuracy of the model.
Although BCLC stage is widely used in clinical practice, there is a lack of treatment history and inflammatory markers. Other treatment modalities in addition to systemic therapy also affect the survival of HCC, and a small sample study found that TACE tends to delay HCC progression, although no randomized intervention was performed (38). The sequence and time interval between TACE and ICIs are currently inconclusive. The heterogeneity of the tumor microenvironment makes treatment responses different, systemic inflammatory responses play an important role in immune monitoring and treatment assessment (41). NLR and PLR, as the most commonly used inflammatory markers, have been shown to be independent predictors of HCC (42–44). However, no prognostic effect of NLR was found in our study, whereas PLR was included in the model as a continuous variable. High-affinity lymphocytes have antitumor activity and are associated with cancer immune escape (45). Although advanced HCC is often accompanied by thrombocytopenia, platelets can promote the formation of tumor metastasis (46). Therefore, platelet binding to lymphocytes is predictive of HCC with combination regimen, and tends to predict a poor prognosis. In addition, we report the association of other nutritional inflammatory markers (LMR, PNI, and SII) with prognosis, but no significant correlation was found.
Immunotherapy related hepatotoxicity is one of the immune-related AEs and often manifests as an increase in ALT or AST (47). We have found that elevated ALT levels before ICI also predict shorter survival, therefore, management of liver function is challenging. AFP is one of the markers of HCC, and elevated AFP is associated with progression and survival (48). Ramucirumab is used in HCC previously treated with sorafenib and is currently the only agent to select patient populations based on AFP (10). We found that AFP levels were negatively correlated with HCC prognosis treated with ICIs and TKIs and could be used as potential markers to predict patient survival.
The above indicators provide clinicians with direct information on whether to use combination therapy and maximize drug efficacy. Our model contains not only important components of BCLC stage, but also liver function, tumor and inflammatory markers, as well as treatment history. Multiple rather than single prognostic factors were included in the study, which is more comprehensive and optimizes its predictive performance. In addition, the above indicators are routine clinical examinations, non-invasive and easy to obtain.
Limitations of this study are: first, the ICIs and TKIs received by patients are heterogeneous, and no studies have yet demonstrated differences in efficacy among different PD-1 inhibitors; second, there is an inevitable selection bias in the retrospective design, we did not perform stratified analysis according to genes or proteins, and lack predictive analysis of tumor molecular mechanisms for immune prognostic models; finally, the patient population comes from a single center and lacks external validation in other regions, and etiological differences between regions are also non-negligible factors. Additional potential predictors need to be explored prospectively in large multicenter studies in the future, and appropriate subgroups of patients have been selected.
We constructed a prognostic model for predicting ICIs combined with anti-angiogenic agents for unresectable HCC. The included indicators are comprehensive and simple, and the model has good performance, realizing individualized prediction of patients. The model may predict the efficacy of the combination regimen for advanced HCCs.
The raw data supporting the conclusions of this article will be made available by the authors, without undue reservation.
The studies involving human participants were reviewed and approved by the ethics review committee of Beijing Ditan Hospital, Capital Medical University. The patients/participants provided their written informed consent to participate in this study.
Study conception and design, JC and WL. Conception, data collection, assembly of data, project administration, and manuscript preparation, XL and WS. Data collection, data analysis, and manuscript review, XD. All authors contributed to the article and approved the submitted version.
We thank all participants for their endeavor and contribution to this study.
The authors declare that the research was conducted in the absence of any commercial or financial relationships that could be construed as a potential conflict of interest.
All claims expressed in this article are solely those of the authors and do not necessarily represent those of their affiliated organizations, or those of the publisher, the editors and the reviewers. Any product that may be evaluated in this article, or claim that may be made by its manufacturer, is not guaranteed or endorsed by the publisher.
The Supplementary Material for this article can be found online at: https://www.frontiersin.org/articles/10.3389/fimmu.2022.1060051/full#supplementary-material
1. Sung H, Ferlay J, Siegel RL, Laversanne M, Soerjomataram I, Jemal A, et al. Global cancer statistics 2020: GLOBOCAN estimates of incidence and mortality worldwide for 36 cancers in 185 countries. CA: Cancer J Clin (2021) 71(3):209–49. doi: 10.3322/caac.21660
2. Villanueva A. Hepatocellular carcinoma. N Engl J Med (2019) 380(15):1450–62. doi: 10.1056/NEJMra1713263
3. Park JW, Chen M, Colombo M, Roberts LR, Schwartz M, Chen PJ, et al. Global patterns of hepatocellular carcinoma management from diagnosis to death: the BRIDGE study. Liver Int (2015) 35(9):2155–66. doi: 10.1111/liv.12818
4. Fu Y, Liu S, Zeng S, Shen H. From bench to bed: the tumor immune microenvironment and current immunotherapeutic strategies for hepatocellular carcinoma. J Exp Clin Cancer Res (2019) 38(1):396. doi: 10.1186/s13046-019-1396-4
5. Llovet JM, Ricci S, Mazzaferro V, Hilgard P, Gane E, Blanc JF, et al. Sorafenib in advanced hepatocellular carcinoma. N Engl J Med (2008) 359(4):378–90. doi: 10.1056/NEJMoa0708857
6. Cheng AL, Kang YK, Chen Z, Tsao CJ, Qin S, Kim JS, et al. Efficacy and safety of sorafenib in patients in the Asia-pacific region with advanced hepatocellular carcinoma: a phase III randomised, double-blind, placebo-controlled trial. Lancet Oncol (2009) 10(1):25–34. doi: 10.1016/S1470-2045(08)70285-7
7. Kudo M, Finn RS, Qin S, Han KH, Ikeda K, Piscaglia F, et al. Lenvatinib versus sorafenib in first-line treatment of patients with unresectable hepatocellular carcinoma: a randomised phase 3 non-inferiority trial. Lancet (London England) (2018) 391(10126):1163–73. doi: 10.1016/S0140-6736(18)30207-1
8. Bruix J, Qin S, Merle P, Granito A, Huang YH, Bodoky G, et al. Regorafenib for patients with hepatocellular carcinoma who progressed on sorafenib treatment (RESORCE): a randomised, double-blind, placebo-controlled, phase 3 trial. Lancet (London England) (2017) 389(10064):56–66. doi: 10.1016/S0140-6736(16)32453-9
9. Abou-Alfa GK, Meyer T, Cheng AL, El-Khoueiry AB, Rimassa L, Ryoo BY, et al. Cabozantinib in patients with advanced and progressing hepatocellular carcinoma. N Engl J Med (2018) 379(1):54–63. doi: 10.1056/NEJMoa1717002
10. Zhu AX, Kang YK, Yen CJ, Finn RS, Galle PR, Llovet JM, et al. Ramucirumab after sorafenib in patients with advanced hepatocellular carcinoma and increased α-fetoprotein concentrations (REACH-2): a randomised, double-blind, placebo-controlled, phase 3 trial. Lancet Oncol (2019) 20(2):282–96. doi: 10.1016/S1470-2045(18)30937-9
11. Yau T, Kang YK, Kim TY, El-Khoueiry AB, Santoro A, Sangro B, et al. Efficacy and safety of nivolumab plus ipilimumab in patients with advanced hepatocellular carcinoma previously treated with sorafenib: The CheckMate 040 randomized clinical trial. JAMA Oncol (2020) 6(11):e204564. doi: 10.1001/jamaoncol.2020.4564
12. Zhu AX, Finn RS, Edeline J, Cattan S, Ogasawara S, Palmer D, et al. Pembrolizumab in patients with advanced hepatocellular carcinoma previously treated with sorafenib (KEYNOTE-224): a non-randomised, open-label phase 2 trial. Lancet Oncol (2018) 19(7):940–52. doi: 10.1016/S1470-2045(18)30351-6
13. Xu J, Shen J, Gu S, Zhang Y, Wu L, Wu J, et al. Camrelizumab in combination with apatinib in patients with advanced hepatocellular carcinoma (RESCUE): A nonrandomized, open-label, phase II trial. Clin Cancer Res (2021) 27(4):1003–11. doi: 10.1158/1078-0432.CCR-20-2571
14. Zhang W, Gong C, Peng X, Bi X, Sun Y, Zhou J, et al. Serum concentration of CD137 and tumor infiltration by M1 macrophages predict the response to sintilimab plus bevacizumab biosimilar in advanced hepatocellular carcinoma patients. Clin Cancer Res (2022) 28(16):3499–508. doi: 10.1158/1078-0432.CCR-21-3972
15. Pinter M, Jain RK, Duda DG. The current landscape of immune checkpoint blockade in hepatocellular carcinoma: A review. JAMA Oncol (2021) 7(1):113–23. doi: 10.1001/jamaoncol.2020.3381
16. Leone P, Solimando AG, Fasano R, Argentiero A, Malerba E, Buonavoglia A, et al. The evolving role of immune checkpoint inhibitors in hepatocellular carcinoma treatment. Vaccines (Basel) (2021) 9(5):532. doi: 10.3390/vaccines9050532
17. Finn RS, Qin S, Ikeda M, Galle PR, Ducreux M, Kim TY, et al. Atezolizumab plus bevacizumab in unresectable hepatocellular carcinoma. N Engl J Med (2020) 382(20):1894–905. doi: 10.1056/NEJMoa1915745
18. Ren Z, Xu J, Bai Y, Xu A, Cang S, Du C, et al. Sintilimab plus a bevacizumab biosimilar (IBI305) versus sorafenib in unresectable hepatocellular carcinoma (ORIENT-32): a randomised, open-label, phase 2-3 study. Lancet Oncol (2021) 22(7):977–90. doi: 10.1016/S1470-2045(21)00252-7
19. Pinato DJ, Mauri FA, Spina P, Cain O, Siddique A, Goldin R, et al. Clinical implications of heterogeneity in PD-L1 immunohistochemical detection in hepatocellular carcinoma: the blueprint-HCC study. Br J Cancer (2019) 120(11):1033–6. doi: 10.1038/s41416-019-0466-x
20. Ang C, Klempner SJ, Ali SM, Madison R, Ross JS, Severson EA, et al. Prevalence of established and emerging biomarkers of immune checkpoint inhibitor response in advanced hepatocellular carcinoma. Oncotarget (2019) 10(40):4018–25. doi: 10.18632/oncotarget.26998
21. Sheng R, Zeng M, Jin K, Zhang Y, Wu D, Sun H. MRI-Based nomogram predicts the risk of progression of unresectable hepatocellular carcinoma after combined lenvatinib and anti-PD-1 antibody therapy. Acad Radiol (2022) 29(6):819–29. doi: 10.1016/j.acra.2021.09.004
22. Yuan G, Song Y, Li Q, Hu X, Zang M, Dai W, et al. Development and validation of a contrast-enhanced CT-based radiomics nomogram for prediction of therapeutic efficacy of anti-PD-1 antibodies in advanced HCC patients. Front Immunol (2020) 11:613946. doi: 10.3389/fimmu.2020.613946
23. Gu X, Guan J, Xu J, Zheng Q, Chen C, Yang Q, et al. Model based on five tumour immune microenvironment-related genes for predicting hepatocellular carcinoma immunotherapy outcomes. J Trans Med (2021) 19(1):26. doi: 10.1186/s12967-020-02691-4
24. Dai Y, Qiang W, Lin K, Gui Y, Lan X, Wang D. An immune-related gene signature for predicting survival and immunotherapy efficacy in hepatocellular carcinoma. Cancer Immunol Immunother (2021) 70(4):967–79. doi: 10.1007/s00262-020-02743-0
25. Ou Q, Yu Y, Li A, Chen J, Yu T, Xu X, et al. Association of survival and genomic mutation signature with immunotherapy in patients with hepatocellular carcinoma. Ann Trans Med (2020) 8(5):230. doi: 10.21037/atm.2020.01.32
26. Ha Y, Mohamed Ali MA, Petersen MM, Harmsen WS, Therneau TM, Lee HC, et al. Lymphocyte to monocyte ratio-based nomogram for predicting outcomes of hepatocellular carcinoma treated with sorafenib. Hepatol Int (2020) 14(5):776–87. doi: 10.1007/s12072-020-10076-4
27. Conroy G, Salleron J, Belle A, Bensenane M, Nani A, Ayav A, et al. The prognostic value of inflammation-based scores in advanced hepatocellular carcinoma patients prior to treatment with sorafenib. Oncotarget (2017) 8(56):95853–64. doi: 10.18632/oncotarget.21401
28. Wang Z, Wang J, Wang P. The prognostic value of prognostic nutritional index in hepatocellular carcinoma patients: A meta-analysis of observational studies. PloS One (2018) 13(10):e0202987. doi: 10.1371/journal.pone.0202987
29. Diaz-Beveridge R, Bruixola G, Lorente D, Caballero J, Rodrigo E, Segura Á, et al. An internally validated new clinical and inflammation-based prognostic score for patients with advanced hepatocellular carcinoma treated with sorafenib. Clin Trans Oncol (2018) 20(3):322–9. doi: 10.1007/s12094-017-1720-4
30. Lee S, Seo MH, Shin Y. The lasso for high dimensional regression with a possible change point. J R Stat Soc Ser B Stat Method (2016) 78(1):193–210. doi: 10.1111/rssb.12108
31. Carrington AM, Fieguth PW, Qazi H, Holzinger A, Chen HH, Mayr F, et al. A new concordant partial AUC and partial c statistic for imbalanced data in the evaluation of machine learning algorithms. BMC Med Inf Decision Making (2020) 20(1):4. doi: 10.1186/s12911-019-1014-6
32. Van Calster B, McLernon DJ, van Smeden M, Wynants L, Steyerberg EW. Calibration: the Achilles heel of predictive analytics. BMC Med (2019) 17(1):230. doi: 10.1186/s12916-019-1466-7
33. Vickers AJ, Elkin EB. Decision curve analysis: a novel method for evaluating prediction models. Med decision making: an Int J Soc Med Decision Making (2006) 26(6):565–74. doi: 10.1177/0272989X06295361
34. Sangro B, Melero I, Wadhawan S, Finn RS, Abou-Alfa GK, Cheng AL, et al. Association of inflammatory biomarkers with clinical outcomes in nivolumab-treated patients with advanced hepatocellular carcinoma. J Hepatol (2020) 73(6):1460–9. doi: 10.1016/j.jhep.2020.07.026
35. Marabelle A, Fakih M, Lopez J, Shah M, Shapira-Frommer R, Nakagawa K, et al. Association of tumour mutational burden with outcomes in patients with advanced solid tumours treated with pembrolizumab: prospective biomarker analysis of the multicohort, open-label, phase 2 KEYNOTE-158 study. Lancet Oncol (2020) 21(10):1353–65. doi: 10.1016/S1470-2045(20)30445-9
36. Iasonos A, Schrag D, Raj GV, Panageas KS. How to build and interpret a nomogram for cancer prognosis. J Clin Oncol (2008) 26(8):1364–70. doi: 10.1200/JCO.2007.12.9791
37. Reig M, Forner A, Rimola J, Ferrer-Fàbrega J, Burrel M, Garcia-Criado Á, et al. BCLC strategy for prognosis prediction and treatment recommendation: The 2022 update. J Hepatol (2022) 76(3):681–93. doi: 10.1016/j.jhep.2021.11.018
38. Kuo HY, Chiang NJ, Chuang CH, Chen CY, Wu IC, Chang TT, et al. Impact of immune checkpoint inhibitors with or without a combination of tyrosine kinase inhibitors on organ-specific efficacy and macrovascular invasion in advanced hepatocellular carcinoma. Oncol Res Treat (2020) 43(5):211–20. doi: 10.1159/000505933
39. Xie D, Sun Q, Wang X, Zhou J, Fan J, Ren Z, et al. Immune checkpoint inhibitor plus tyrosine kinase inhibitor for unresectable hepatocellular carcinoma in the real world. Ann Trans Med (2021) 9(8):652. doi: 10.21037/atm-20-7037
40. Lu LC, Hsu C, Shao YY, Chao Y, Yen CJ, Shih IL, et al. Differential organ-specific tumor response to immune checkpoint inhibitors in hepatocellular carcinoma. Liver Cancer (2019) 8(6):480–90. doi: 10.1159/000501275
41. Grivennikov SI, Greten FR, Karin M. Immunity, inflammation, and cancer. Cell (2010) 140(6):883–99. doi: 10.1016/j.cell.2010.01.025
42. Wen S, Chen Y, Hu C, Du X, Xia J, Wang X, et al. Combination of tertiary lymphoid structure and neutrophil-to-Lymphocyte ratio predicts survival in patients with hepatocellular carcinoma. Front Immunol (2021) 12:788640. doi: 10.3389/fimmu.2021.788640
43. Muhammed A, Fulgenzi CAM, Dharmapuri S, Pinter M, Balcar L, Scheiner B, et al. The systemic inflammatory response identifies patients with adverse clinical outcome from immunotherapy in hepatocellular carcinoma. Cancers (2021) 14(1):186. doi: 10.3390/cancers14010186
44. Dharmapuri S, Özbek U, Lin JY, Sung M, Schwartz M, Branch AD, et al. Predictive value of neutrophil to lymphocyte ratio and platelet to lymphocyte ratio in advanced hepatocellular carcinoma patients treated with anti-PD-1 therapy. Cancer Med (2020) 9(14):4962–70. doi: 10.1002/cam4.3135
45. Rosenberg SA. Progress in human tumour immunology and immunotherapy. Nature (2001) 411(6835):380–4. doi: 10.1038/35077246
46. Labelle M, Begum S, Hynes RO. Direct signaling between platelets and cancer cells induces an epithelial-mesenchymal-like transition and promotes metastasis. Cancer Cell (2011) 20(5):576–90. doi: 10.1016/j.ccr.2011.09.009
47. De Martin E, Michot JM, Rosmorduc O, Guettier C, Samuel D. Liver toxicity as a limiting factor to the increasing use of immune checkpoint inhibitors. JHEP reports: Innovation Hepatol (2020) 2(6):100170. doi: 10.1016/j.jhepr.2020.100170
Keywords: hepatocellular carcinoma, immune checkpoint inhibitors, anti-angiogenic agents, predictive model, platelet to lymphocyte ratio, alpha-fetoprotein
Citation: Li X, Sun W, Ding X, Li W and Chen J (2022) Prognostic model of immune checkpoint inhibitors combined with anti-angiogenic agents in unresectable hepatocellular carcinoma. Front. Immunol. 13:1060051. doi: 10.3389/fimmu.2022.1060051
Received: 02 October 2022; Accepted: 21 November 2022;
Published: 01 December 2022.
Edited by:
Yunfei Xu, Shandong University, ChinaReviewed by:
Antonio Giovanni Solimando, University of Bari Aldo Moro, ItalyCopyright © 2022 Li, Sun, Ding, Li and Chen. This is an open-access article distributed under the terms of the Creative Commons Attribution License (CC BY). The use, distribution or reproduction in other forums is permitted, provided the original author(s) and the copyright owner(s) are credited and that the original publication in this journal is cited, in accordance with accepted academic practice. No use, distribution or reproduction is permitted which does not comply with these terms.
*Correspondence: Jinglong Chen, Y2psNjQxMkBjY211LmVkdS5jbg==; Wei Li, dmlzaW9uOTg4QDEyNi5jb20=
†These authors have contributed equally to this work and share first authorship
Disclaimer: All claims expressed in this article are solely those of the authors and do not necessarily represent those of their affiliated organizations, or those of the publisher, the editors and the reviewers. Any product that may be evaluated in this article or claim that may be made by its manufacturer is not guaranteed or endorsed by the publisher.
Research integrity at Frontiers
Learn more about the work of our research integrity team to safeguard the quality of each article we publish.