- 1Department of Orthopedics, 900th Hospital of Joint Logistics Support Force, Fuzhou, China
- 2Department of Paediatrics, Fujian Provincial Hospital South Branch, Fuzhou, China
- 3Department of Pharmacology and Toxicology, University of Mississippi Medical Center, Jackson, MS, United States
- 4Key Laboratory of Zoonose Prevention and Control at Universities of Inner Mongolia Autonomous Region, Medical College, Inner Mongolia Minzu University, Tongliao, China
- 5Department of Orthopedics, First Affiliated Hospital of Fujian Medical University, Fuzhou, China
Background: The coronavirus disease (COVID-19) is a pandemic disease that threatens worldwide public health, and rheumatoid arthritis (RA) is the most common autoimmune disease. COVID-19 and RA are each strong risk factors for the other, but their molecular mechanisms are unclear. This study aims to investigate the biomarkers between COVID-19 and RA from the mechanism of pyroptosis and find effective disease-targeting drugs.
Methods: We obtained the common gene shared by COVID-19, RA (GSE55235), and pyroptosis using bioinformatics analysis and then did the principal component analysis(PCA). The Co-genes were evaluated by Gene Ontology (GO), Kyoto Encyclopedia of Genes and Genomes (KEGG), and ClueGO for functional enrichment, the protein-protein interaction (PPI) network was built by STRING, and the k-means machine learning algorithm was employed for cluster analysis. Modular analysis utilizing Cytoscape to identify hub genes, functional enrichment analysis with Metascape and GeneMANIA, and NetworkAnalyst for gene-drug prediction. Network pharmacology analysis was performed to identify target drug-related genes intersecting with COVID-19, RA, and pyroptosis to acquire Co-hub genes and construct transcription factor (TF)-hub genes and miRNA-hub genes networks by NetworkAnalyst. The Co-hub genes were validated using GSE55457 and GSE93272 to acquire the Key gene, and their efficacy was assessed using receiver operating curves (ROC); SPEED2 was then used to determine the upstream pathway. Immune cell infiltration was analyzed using CIBERSORT and validated by the HPA database. Molecular docking, molecular dynamics simulation, and molecular mechanics-generalized born surface area (MM-GBSA) were used to explore and validate drug-gene relationships through computer-aided drug design.
Results: COVID-19, RA, and pyroptosis-related genes were enriched in pyroptosis and pro-inflammatory pathways(the NOD-like receptor family pyrin domain containing 3 (NLRP3) inflammasome complex, death-inducing signaling complex, regulation of interleukin production), natural immune pathways (Network map of SARS-CoV-2 signaling pathway, activation of NLRP3 inflammasome by SARS-CoV-2) and COVID-19-and RA-related cytokine storm pathways (IL, nuclear factor-kappa B (NF-κB), TNF signaling pathway and regulation of cytokine-mediated signaling). Of these, CASP1 is the most involved pathway and is closely related to minocycline. YY1, hsa-mir-429, and hsa-mir-34a-5p play an important role in the expression of CASP1. Monocytes are high-caspase-1-expressing sentinel cells. Minocycline can generate a highly stable state for biochemical activity by docking closely with the active region of caspase-1.
Conclusions: Caspase-1 is a common biomarker for COVID-19, RA, and pyroptosis, and it may be an important mediator of the excessive inflammatory response induced by SARS-CoV-2 in RA patients through pyroptosis. Minocycline may counteract cytokine storm inflammation in patients with COVID-19 combined with RA by inhibiting caspase-1 expression.
Introduction
In 2019, SARS-CoV-2-caused COVID-19 was recognized as a public health emergency of international concern (PHIEC) and subsequently identified as a pandemic by the World Health Organization (WHO) (1–6). SARS-CoV-2 is the third widespread coronavirus outbreak after SARS CoV in 2003 (7, 8) and MERS CoV in 2012 (9, 10). Droplets and aerosols mostly transmit SARS-CoV-2 at close range (11–13). From the COVID-19 dashboard of the Johns Hopkins Coronavirus Resource Center: As of 2022.8.28, more than 200 countries/regions worldwide have recorded over 600 million confirmed cases and over 6.48 million deaths, with a total of 12.124 billion vaccine doses administered (14). Coronaviruses (CoVs) are a group of enveloped viruses with a single-stranded RNA genome (+ssRNA) that exhibits a high mutation rate and variable recombination rates (15–17). SARS-CoV-2 is the ninth coronavirus threatening human health (18, 19) and has a high degree of host genetic variation (20–23). SARS-CoV-2 can encode 29 proteins (24, 25), consisting of 16 non-structural proteins (NSP) (26), 4 structural proteins (spike [S], envelope [E], membrane [M], and nucleocapsid [N]) (27), and 9 auxiliary proteins (28). COVID-19 is not just a respiratory disease but also a systemic disease that affects many of the body’s systems and organs (29, 30). SARS-CoV-2 infection frequently disrupts the immune system (31), resulting in increased expression of autoantigens during infection and the development of autoantibodies due to the organism’s potential antigenic cross-reactivity (32–34). SARS-CoV-2 is not only predisposed to the onset and progression of autoimmune diseases (35–37), but even SARS-CoV-2 vaccination can trigger autoimmune phenomena (38, 39). Consequently, patients with autoimmune illnesses have a higher risk of contracting COVID-19 (40, 41).
The COVID-19 Global Rheumatology Alliance Global Registry records: As of 2022.08.31, the most common autoimmune/rheumatic disease among COVID-19 patients is RA (40.92%) (42). RA is one of the most prevalent autoimmune diseases, with a prevalence of up to 1 percent (43–46), and its expanding population coverage has posed a significant threat to global public health (47). The three primary causes of RA development are genetic, environmental, and immunological factors (48, 49), with viruses, as part of the environmental factors, playing a significant role in the development of RA (50, 51). Correspondingly, the immunological dysregulation in RA patients favors the invasion of SARS-CoV-2 (52, 53). Additionally, the traditional use of DMARDs and glucocorticoids in RA enhances viral replication via immunosuppression, and the use of biological agents (e.g., TNF-α-inhibitors) also raises the likelihood of viral infection in RA (54–57). Therefore, there may be a potential mutual pathogenic factor between COVID-19 and RA that contributes to disease progression, and we need to find appropriate therapeutic agents to combat it.
Pyroptosis is an emerging form of regulated cell death (RCD) and an active area of research (58). It is caused by innate immune dysregulation and disruption of organism/cellular homeostasis due to pathogen invasion (59), as shown by increased plasma membrane permeability, cell swelling, and rupture (60, 61). caspase-1 is one of the first pro-pyroptosis inflammatory cystathiases identified (62–65), creating NLRP3 inflammasome by binding to NLRP3, apoptosis-associated speck-like Protein (ASC), which establishes the canonical route of pyroptosis leading to cell lysis and the release of IL-1β and IL-18 (66–70). Firstly, active NLRP3 inflammasome and caspase-1 are detected in the peripheral blood and tissues of COVID-19 patients and are positively correlated with severity markers for COVID-19 (e.g., IL-6) (71). In SARS-CoV-2 infected cells, NLRP3 inflammasome and caspase-1 activity increase and promote pyroptosis and cytokine storm (72–74). Secondly, the overactivation of NLRP3 inflammasome and caspase-1 in individuals with RA’s serum, synovium, and synovial fluid induces pyroptosis and inflammatory responses and is positively linked with disease activity (75–79). Thus, the caspase-1-mediated classical pyroptosis pathway may be an important cause of the vicious cycle of cytokine storm caused by the interaction between COVID-19 and RA disease. This study investigates the pathogenesis and disease targets of COVID-19 associated with RA through bioinformatics and network pharmacology analysis as well as computer-aided drug design methods and explores the drug and pharmacology of this target.
Methods
Data collection and processing
Three RA datasets (GSE55235, GSE55457, GSE93272) were screened using the National Center for Biotechnology Information (NCBI) Gene Expression Omnibus (GEO) (https://www.ncbi.nlm.nih.gov/geo/) (Table 1). GSE55235 contains synovial tissue samples from 10 RA cases and 10 healthy people. GSE55457 contains synovial tissue samples from 13 RA cases and 10 healthy people, and GSE93272 contains 232 whole blood samples from RA patients and 43 healthy people. The GeneCards database (https://www.genecards.org/) (80) platform searched for the keywords “SARS-CoV-2” and “COVID-19” and found 4055 and 4778 related genes. Xiong et al., 2020 (81), Ziegler et al., 2020 (82), and Jain et al., 2020 (83), respectively, contributed an additional 25, 17, and 28 COVID-19-related genes (Supplementary Table 1). A total of 5103 COVID-19-related genes were obtained by pooling and de-duplicating these genes. Similarly, a search of the GeneCards database using the keyword “pyroptosis” yielded 254 related genes.
Identification of co-genes
The empirical Bayesian method in the limma package (http://www.bioconductor.org/packages/release/bioc/html/limma.html) (84) was used to analyze the RA and healthy controls (HC) groups of the GSE55235 dataset in different gene expression analyses. |log2 FC| >0.5 and P< 0.05 as the cutoff. Further mapping of volcanoes using the ggplot2 package to reflect RA-differentially expressed genes (DEGs). Co-genes were obtained from the intersection of COVID-19, RA-DEGs (GSE55235), and pyroptosis-related genes using the Venn-diagram package in R software and subjected to PCA.
GO, KEGG, and ClueGO enrichment analyses of co-genes
For the investigation of the pathway and function of the Co-genes, the R package “clusterProfiler” (85) was used to conduct GO and KEGG enrichment analyses. Co-genes are visualized through ClueGO (a plug-in for Cytoscape, using kappa’s statistical analysis method) to differentiate between up-and down-regulated genes to construct interactive gene network maps and analyze the function of target gene sets.
PPI network analysis and machine learning for the identification of hub genes
The STRING database (https://string-db.org/) (86) was utilized to analyze the Co-genes and build a PPI network with a confidence score > 0.40 as the threshold. The k-means algorithm is an effective unsupervised machine learning technique (87). It enables the prediction of protein-protein interactions without explicit data labeling. We used the k-means algorithm (the network was clustered to a specified number of clusters, the number clusters: 3) Clustering analysis of Co-genes. The Cytoscape platform (88) is utilized to visualize PPI network data, while the MCODE (a Cytoscape plug-in) is utilized for modular analysis of PPI networks. The cytoHubba uses the Degree algorithm to identify Hub genes from Co-genes.
Metascape, geneMANIA and network analyst analyses of hub genes
Metascape (https://metascape.org/gp/index.html#/main/step1) (89) is a gene function analysis website that aggregates over 40 databases and groups genes into clusters based on Terms with a P< 0.01, a minimum count of 3, and an enrichment factor >1.5 to group genes into clusters and find pathways for the enrichment of Hub genes and associated functional annotations. Cytoscape connected terms with similarity > 0.30 to further build a network graphic to capture the linkages between gene clusters. GeneMANIA (http://www.genemania.org) (90) is a website that integrates different databases and technologies, including Gene Expression Omnibus (GEO) and the Biological General Repository for Interaction Datasets (BioGRID), for predicting the functions of Hub genes and identifying gene priority and interconnections. NetworkAnalyst (https://www.networkanalyst.ca/) (91) is a website for visual analysis of gene expression profiling and meta-analysis. The hub genes were analyzed for associations with potentially relevant medications (DrugBank Version 5.0) by the site’s Protein-drug interactions function (minimum network).
Screening for minocycline-related target genes and co-hub genes
CASP1, CASP3, and ILB in the hub genes were closely related to minocycline from NetworkAnalyst analysis. Therefore, minocycline was hypothesized to be an effective drug against this mechanism, and relevant validation was carried out. We used SwissTargetPrediction (http://www.swisstargetprediction.ch/) (92), CTD (http://ctdbase.org/) (93), Drugbank (https://go.drugbank.com/drugs/DB01017) (94) and STITCH (http://stitch.embl.de/cgi/input.pl) which are four databases to search for potentially related genes of minocycline. The STITCH database unifies drug-gene connections between more than 68,000 distinct compounds and 1.5 million genes; we utilize STITCH to visualize minocycline and target genes. COVID-19, RA-DEGs (GSE55235), pyroptosis-related genes, and minocycline-related target genes were intersected to determine the set of Co-targets. Subsequently, the Hub genes were intersected with the Co-targets to obtain Co-hub genes.
Establishment of the TF-hub genes and miRNA-hub genes network
Co-hub genes were submitted to the NetworkAnalyst platform, TFs were obtained from the ENCODE database, and miRNAs were obtained from miRTarBase and TarBase. Visualization of TF-hub genes and miRNA-hub genes network using Cytoscape.
Validation of co-hub genes and identification of key gene
To increase the reliability of the results as well as comprehensiveness, we included GSE55457 and GSE93272 as validation sets in this study. The intersection of the co-hub genes, RA-DEGs (GSE55457) and RA-DEGs (GSE93272), was identified as a key gene. Boxplot analyzed the expression of the key gene, and ROC (95) was used to determine the sensitivity and specificity of the key gene. The area under the curve (AUC) > 0.8 is considered to have a significant diagnostic value.
Upstream pathway activity
SPEED2 (https://speed2.sys-bio.net/) (96) is an upstream signaling pathway enrichment analysis platform that evaluates the significance of 16 classical signaling pathways based on P-values using gene set data from human cell biology research. We used the bates test in SPEED2 to predict the upstream signaling pathways of the co-hub genes and the Key gene.
Analysis of immune cell infiltration
The CIBERSORT algorithm (http://CIBERSORT.stanford.edu/) is a linear support vector regression-based methodology (97) applied to assess the makeup and number of immune cells in RA and HC. The relationship between the expression of the key gene and the abundance of immune cells in RA was revealed using person correlation coefficient analysis to find the immune cells closely related to it. The Human Protein Atlas (https://www.proteinatlas.org/) contains data on the tissue and cellular distribution and expression abundance of nearly all human proteins. The HPA database was utilized to validate the key gene-immune cell associations to guarantee the accuracy of the results.
Molecular docking
Molecular docking techniques were used to verify the affinity of minocycline to the crystal structure of the protein expressed by the Key gene. First, a two-dimensional (2D) structure of minocycline was obtained in sdf format from the Drugbank database or the PubChem database (https://pubchem.ncbi.nih.gov/) (98) for use as a ligand. Entry for Key gene obtained from Uniport database (https://www.uniprot.org/) (99) (CASP1: P29466). Enter the entry into the RCSB PDB database (https://www.rcsb.org/) (100) and download the protein structure in pdb format to use as a receptor. Second, using ChemBio 3D Ultra 12.0 software, the 2D structure of the ligand (minocycline) was transformed to a 3D structure, optimized, and saved in mol2 format. The receptor (caspase-1) was processed using PyMOL 2.4.0 software to remove solvent molecules and ligands and then saved in pdb format. Third, After processing the ligands and receptors in Autodock 1.5.6 software and saving the results in pdbqt format, molecular docking was used to identify the activity pockets of candidate loci and export the results in gpf format. Finally, the AutoDock Vina software was used to carry out the molecular docking commands, and PyMOL 2.4.0 was used to visualize and analyze the results.
Molecular dynamics simulation and molecular mechanics-generalized born solvent accessibility
Further investigation of the dynamic properties, stability, and structural flexibility of protein-drug complexes can be done by molecular dynamics simulations. It permits the examination of the interaction between the drug and the amino acid residues of the target protein and acts as an in-depth validation of molecular docking. MD to MDS and MM-GBSA calculations are a series of workflows for computer-aided drug design to study the properties of ligand-receptor interactions.
AMBER 18 was used to examine the stability of the complexes by simulating the molecular docking of ligands and receptors using all-atom MDS of ligands and receptors. Before the simulation, the charge of the minocycline was determined using the HF-SCF (6-31G**) computation with the antechamber module and gauss 09 software. The GAFF2 small molecule force field and the ff14SB protein force field were utilized to describe, respectively, the ligand (minocycline) and the receptor (caspase-1) (101, 102). The LEaP module was utilized to introduce hydrogen atoms, and a TIP3P solvent cartridge was added at 10Å. The system’s charge is then balanced by adding Na+/Cl-, and the topology and parameter files required for the molecular simulation are then output. Optimization of system energy via a 2500-step steepest descent method and a 2500-step conjugate gradient method. The system was warmed up at 200 ps and stabilized from 0 K to 298.15 K, followed by a 500 ps NVT ensemble simulation and a 500 ps equilibrium simulation. The system was warmed up at 200 ps, from 0 K to 298.15 K, followed by an NVT system simulation (isothermal isomer) at 500 ps, followed by an equilibrium simulation (isothermal isobaric) at 500 ps. The final NVT system simulation (isothermal isobaric) was carried out for 100 ns. Other parameters: truncation distance set to 10 Å, collision frequency γ set to 2 ps-1, system pressure 1 atm, integration step 2 fs, trajectory saved at 10 ps intervals.
The free energy of binding between receptor and ligand is calculated by the MM/GBSA method (103, 104). The specific formula is as follows:
ΔEinternal : Internal energy, ΔEVDW : Van der Waals interactions, ΔEelec : Electrostatic interactions, ΔGGB and ΔGSA : solvation-free energy.
The flowchart shows all of our study’s key and important procedures (Figure 1). The GitHub page for this study is HTTPS(https://github.com/zheng5862/COVID-19-RA.git).
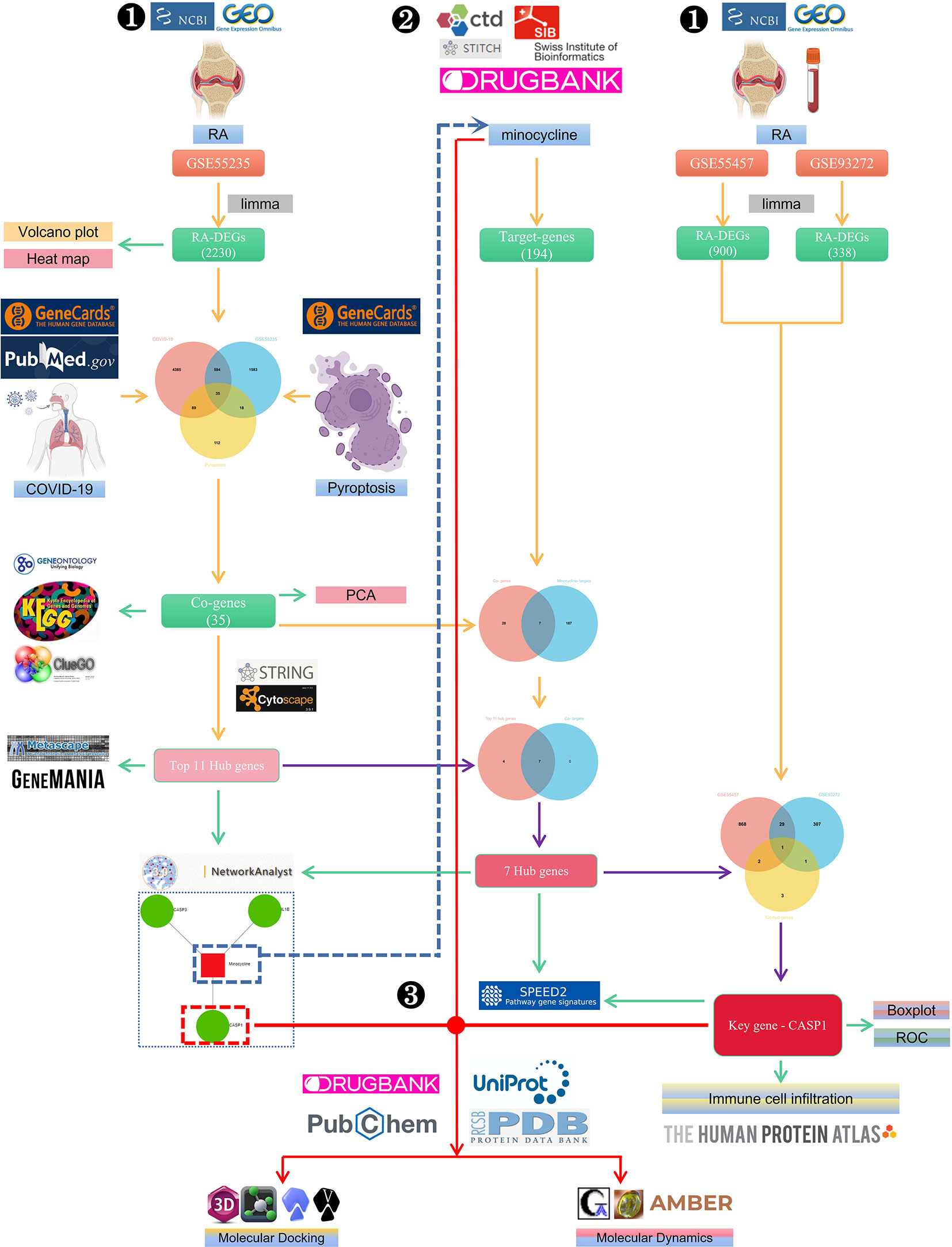
Figure 1 The schematic block diagram of the entire workflow of this study. ❶ Bioinformatics analysis. ❷ Network pharmacology. ❸ Computer-aided drug design.
Results
Identification of co-genes
2230 RA-DEGs were obtained from the GSE55235 dataset and visualized using volcano maps and clustered heat maps (Figures 2, 3). Co-genes are intersecting genes for COVID-19, RA-DEGs (GSE55235), and pyroptosis and include 35 genes, of which 23 are upregulated and 12 are down-regulated (Figure 4A). PCA analysis of the Co-genes in the GSE55235 dataset revealed that PC1 (54.84%) and PC2 (7.91%) confirmed the Co-genes’ significant reliability and between-group variability (Figure 4B).
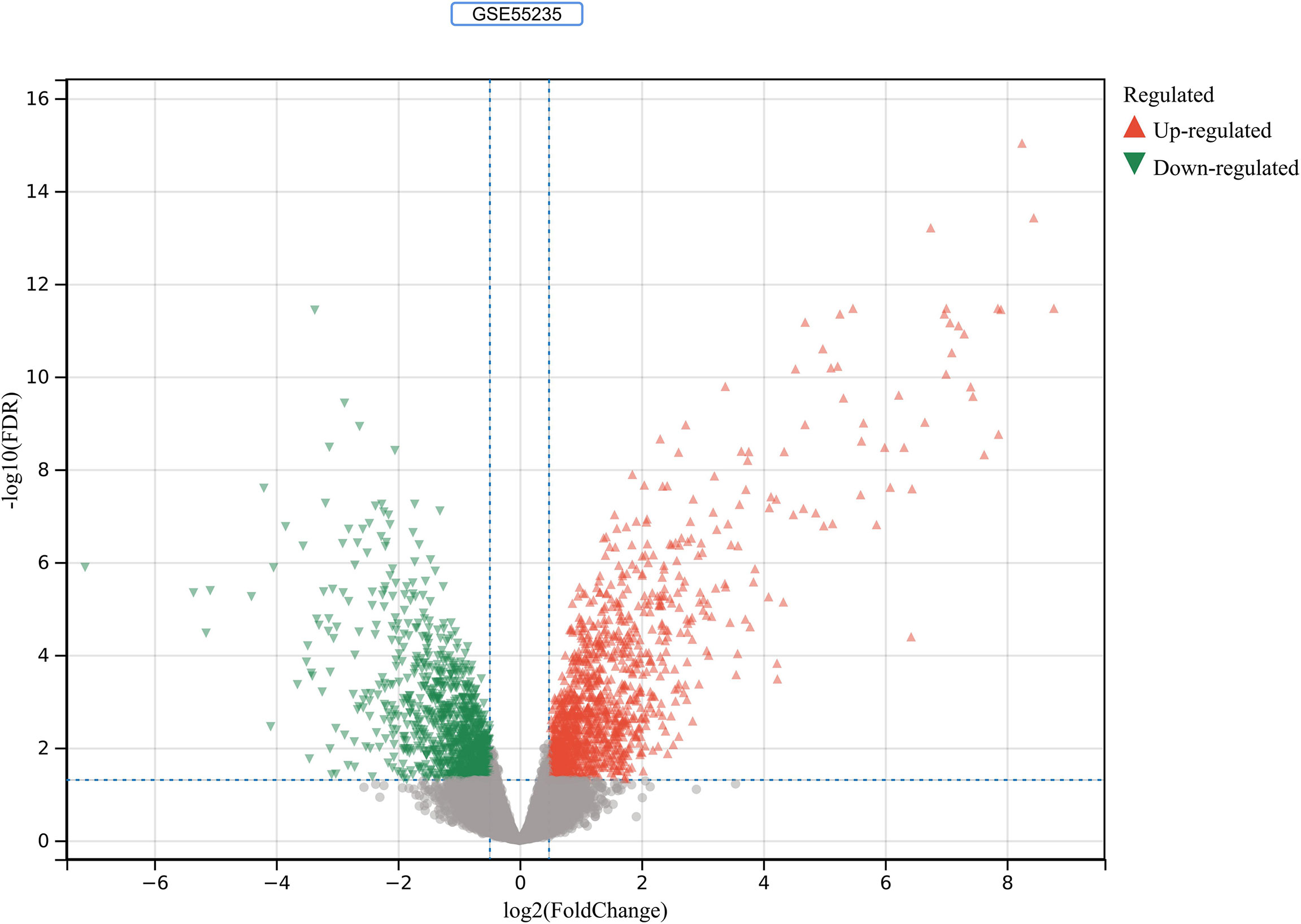
Figure 2 RA-DEGs identification. In the GSE55235 dataset, red triangles represent upregulated genes (P < 0.05), green triangles represent downregulated genes (P < 0.05), and gray dots represent genes not significantly differentially expressed across the RA and HC groups (P > 0.05).
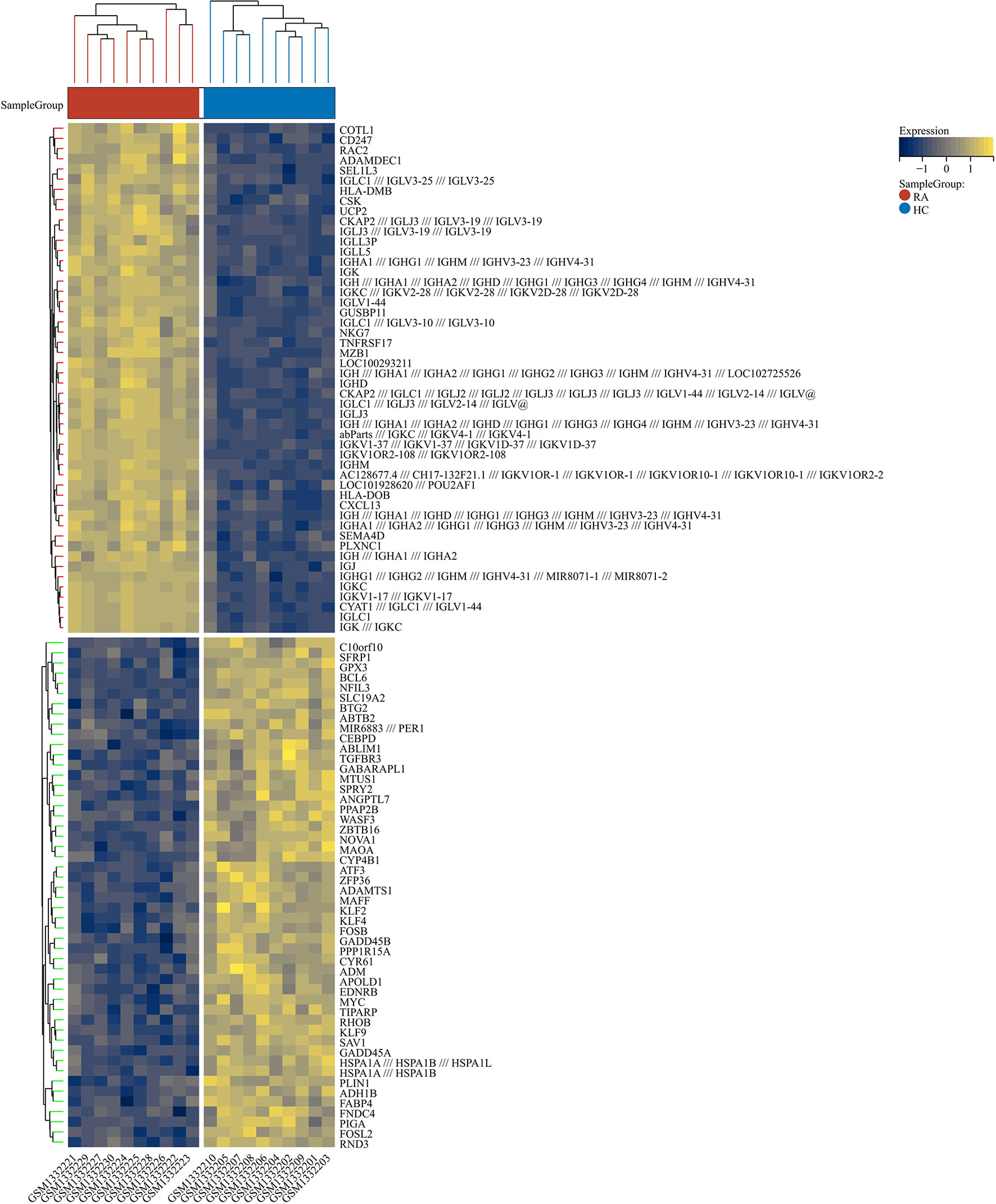
Figure 3 RA-DEGs distribution. The Clustering heat map displays the top one hundred DEGs from the GSE55235 dataset. The samples from the RA group were colored red, while those from the HC group were colored blue. Yellow rectangles represent highly expressed genes (P < 0.05), while blue rectangles represent lowly expressed genes (P < 0.05).
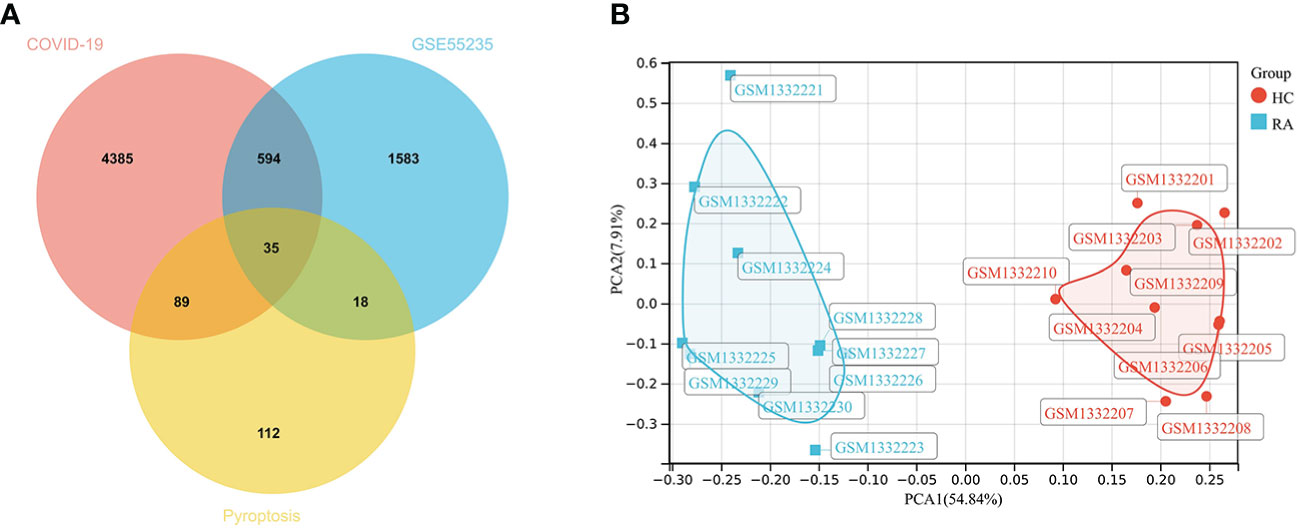
Figure 4 Screening Co-genes. (A) Venn-diagram on COVID-19, RA-DEGs (GSE55235), pyroptosis-related genes. Co-genes include 35 genes. (B) PCA analysis of Co-genes in the GSE55235 dataset: PC1 (54.84%) and PC2 (7.91%).
Functional enrichment analyses of co-genes
GO analysis showed that the biological process (BP) was mainly enriched in the immune system process (Figure 5A). Cellular component (CC) was mainly enriched in the cytoplasm, inflammasome complex, death-inducing signaling complex, NLRP3, and NLRP1 inflammasome complex (Figure 5B). Molecular function (MF) was mainly enriched in signaling receptor binding, protein domain-specific binding, cytokine receptor binding, tumor necrosis factor receptor superfamily binding, and death receptor binding (Figure 5C). The ClueGO analysis showed visually that the upregulated genes of Co-genes were mainly enriched in NLRP3 inflammasome complex, positive response to cytokine stimulus, cytokine production involved in immune response, and regulation of interleukin (IL-1β, IL-6, IL-8, IL-17) production (Figure 5D). KEGG analysis was mainly enriched in the NOD-like receptor (NLR) signaling pathway, the IL-17 signaling pathway, and the Toll-like receptor (TLR) signaling pathway (Figure 5E).
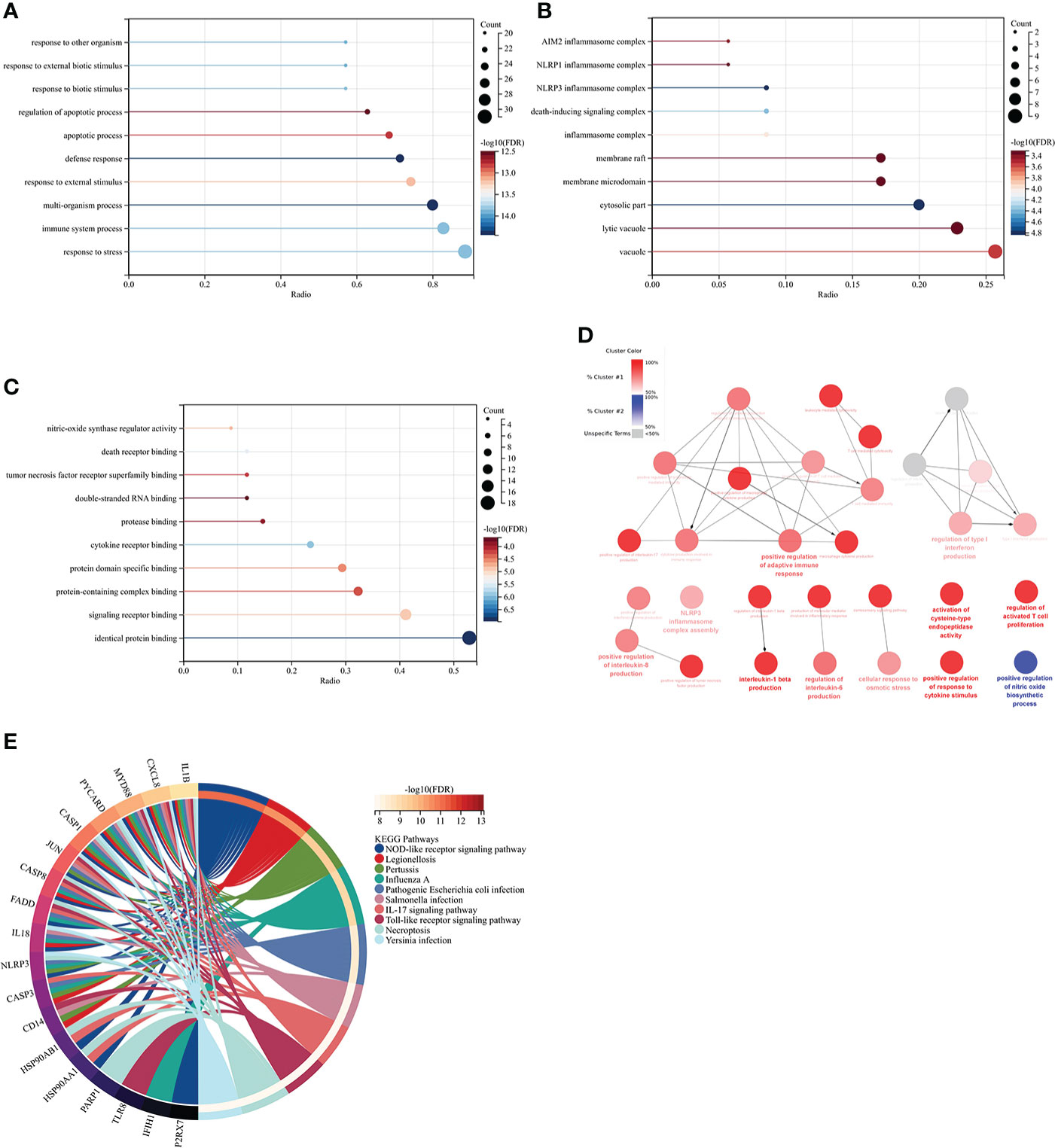
Figure 5 Co-genes functional enrichment analysis using GO, ClueGO, and KEGG. (A) Enrichment of Co-genes in BP. (B) Enrichment of Co-genes in CC. (C) Enrichment of Co-genes in MF. (D) Co-genes Analysis Using ClueGO. Red-denoted pathways for upregulated genes, while blue-denoted pathways for downregulated genes. (E) Co-genes Analysis Using KEGG.
PPI network analysis and machine learning for hub genes
This PPI network has 35 nodes, 202 edges, an average node degree of 11.5, and an average local clustering coefficient of 0.632 (Figure 6A). Using a machine learning algorithm, the k-means clustering analysis of the PPI data predicted the four genes in the lower right corner of the amplified content to be CASP1, NLRP3, IL1B, and IL18 (Figure 6B). These are the genes for the four most important proteins in the caspase-1-driven classical pyroptosis pathway. By using the degree algorithm of the CytoHubba program to the PPI data, the distribution of genes becomes specific and hierarchical, and it can be seen that the top 11 hub genes in the center of the ring were: IL1B, CASP1, CASP3, JUN, MYD88, CASP8, NLRP3, HSP90AA1, CXCL8, IL18, EGFR (where the Degree algorithm values for IL18 and EGFR were equal) (Figure 6C).
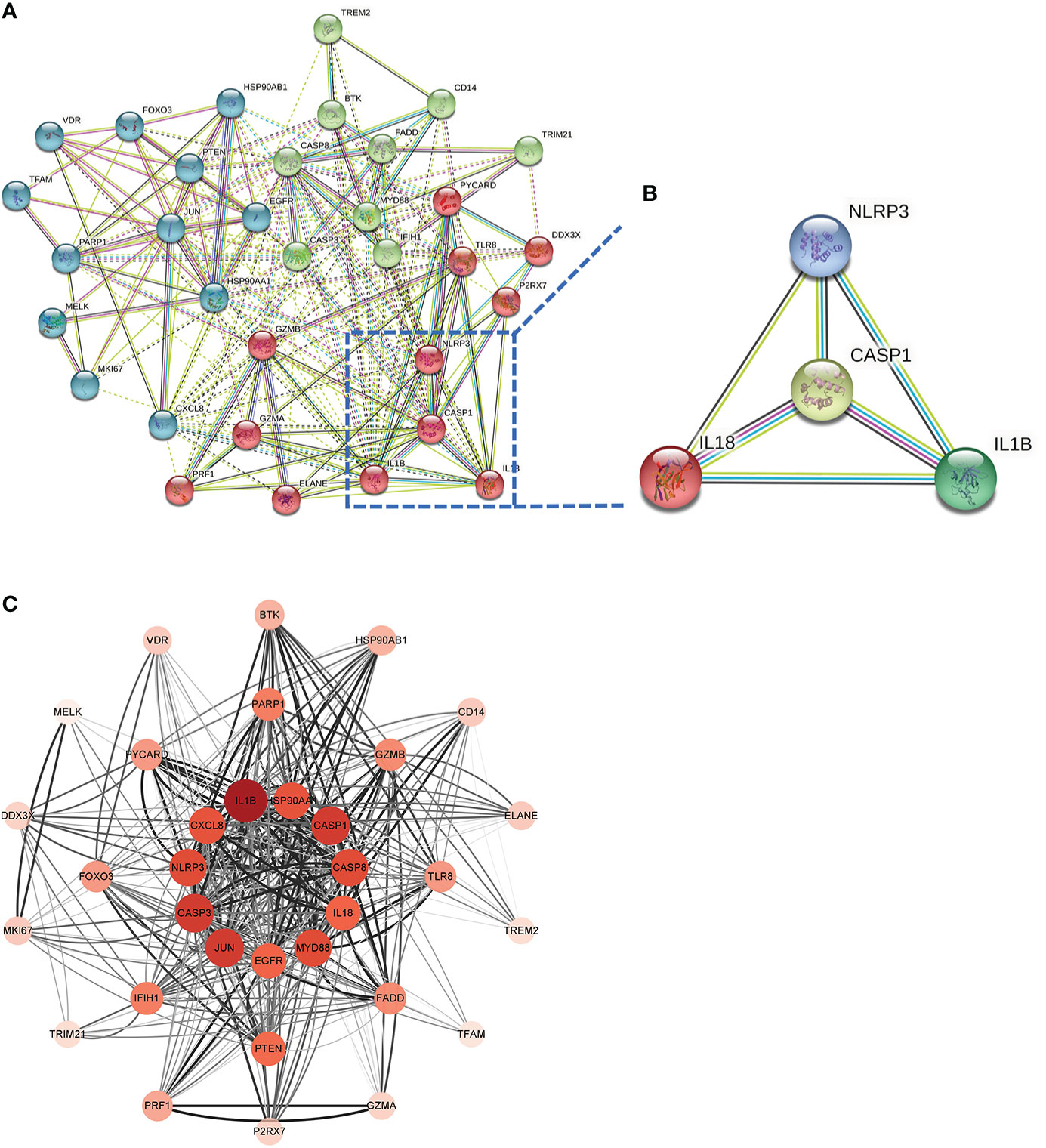
Figure 6 Screening Hub genes. (A) PPI network diagram obtained after applying the k-means algorithm based on machine learning to the Co-genes. The four genes in the lower right-hand corner of the enlarged diagram are CASP1, NLRP3, IL1B, and IL18. (B) PPI network diagram after processing with Cytoscape software. (C) The Top 11 hub genes are filtered using the Degree algorithm under the CytoHubba package condition.
Functional network analysis of the top 11 hub genes
The results of the Metascape analysis were as follows. In pathway and process enrichment analysis, the main enrichments were in the network map of the SARS-CoV-2 signaling pathway; Nucleotide-binding oligomerization domain (NOD) pathway; and Signaling by Interleukins (Table 2) (Figure 7A). Network diagrams will allow visualization of the associations between the pathways (Figure 7B). In the PPI enrichment analysis, the main enrichments were in the NOD pathway, the activation of the NLRP3 inflammasome by SARS-CoV-2 (Figure 7C), and the NLR signaling pathway (Figure 7D). Inflammasome complex, positive regulation of cysteine-type endopeptidase activity, production of IL(LI-1β, IL-6), NF-κB signaling, TNF-mediated signaling pathway, and regulation of cytokine-mediated signaling pathway were all enriched in GeneMANIA analysis of the top 11 hub genes (Figure 8A). Of these, CASP1 is the most involved in the pathway. The protein-drug interactions function on NetworkAnalyst (DrugBank database 5.0) found minocycline to be closely related to CASP1, CASP3, and IL1B (Figure 8B).
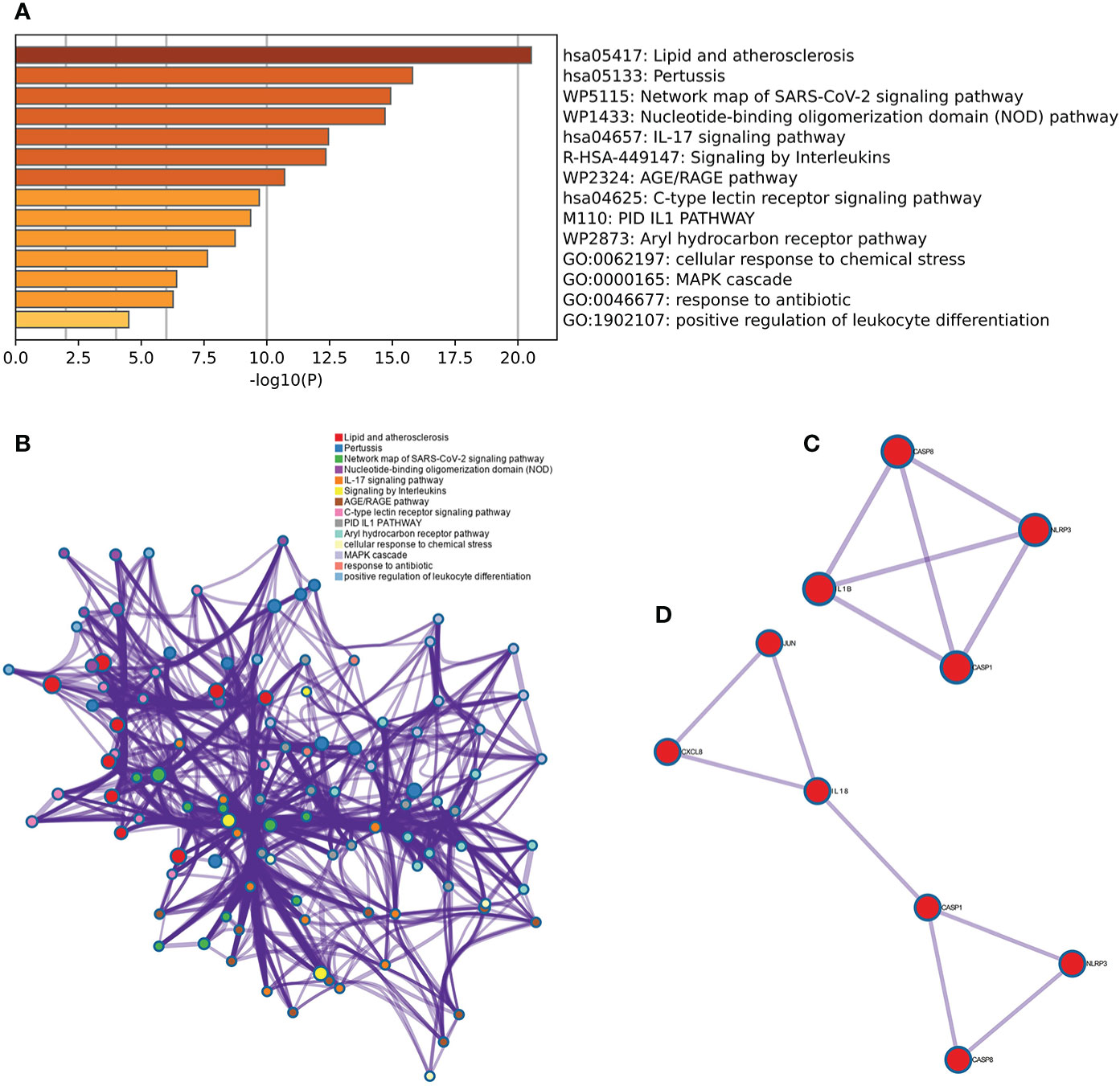
Figure 7 Metascape analysis of Hub genes. (A) Pathway and process richness analysis. (B) The network is shown using Cytoscape5, with nodes with the same cluster ID typically located close to one another. (C, D) Protein-protein Interaction Enrichment Analysis.
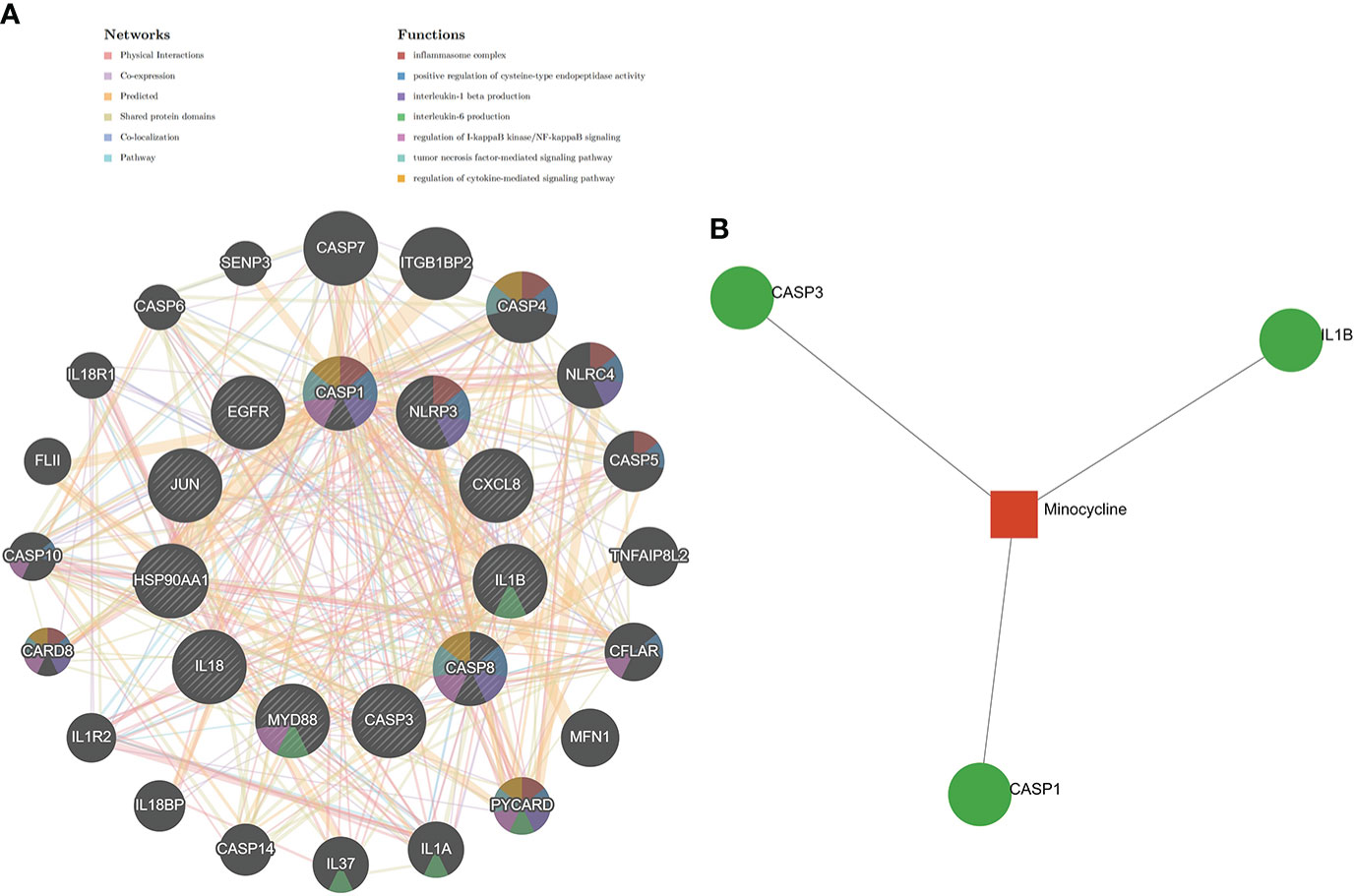
Figure 8 GeneMANIA and NetworkAnalyst analysis of Hub genes. (A) The GeneMANIA database examined the gene-gene interaction network of the top 11 hub genes and the 20 most nearby genes. Each node represents a gene. The color of the node links shows the relationship between the relevant genes. (B) Results for the top 11 Hub genes by NetworkAnalyst’s Protein-Drug Interaction Function (DrugBank database 5.0). Drugs were in red and target genes were in green.
Identification of minocycline-related target genes and co-hub genes
Top 100, 92, 12, and 10 minocycline-related target genes from SwissTargetPrediction, CTD, Drugbank, and STITCH databases, respectively (Supplementary Table 2). We can visualize the connection between minocycline, each target gene, and gene to gene in the STITCH interaction network diagram (Figure 9A). A total of 194 minocycline-related Targets were obtained by pooling the total genes and removing duplicates. Co-targets were 194 genes intersecting with COVID-19, RA-DEGs (GSE55235), and pyroptosis-related genes, including 7 genes: CASP1, CASP8, IL1B, CASP3, JUN, EGFR, CXCL8 (Figure 9B). Co-targets were intersected with the top 11 hub genes to obtain the Co-hub genes (Figure 9C). All 7 genes in the Co- Targets were contained in the top 11 hub genes, suggesting that the targets of minocycline action may be proteins of core genes involved in the pyroptosis mechanism of COVID-19 and RA.
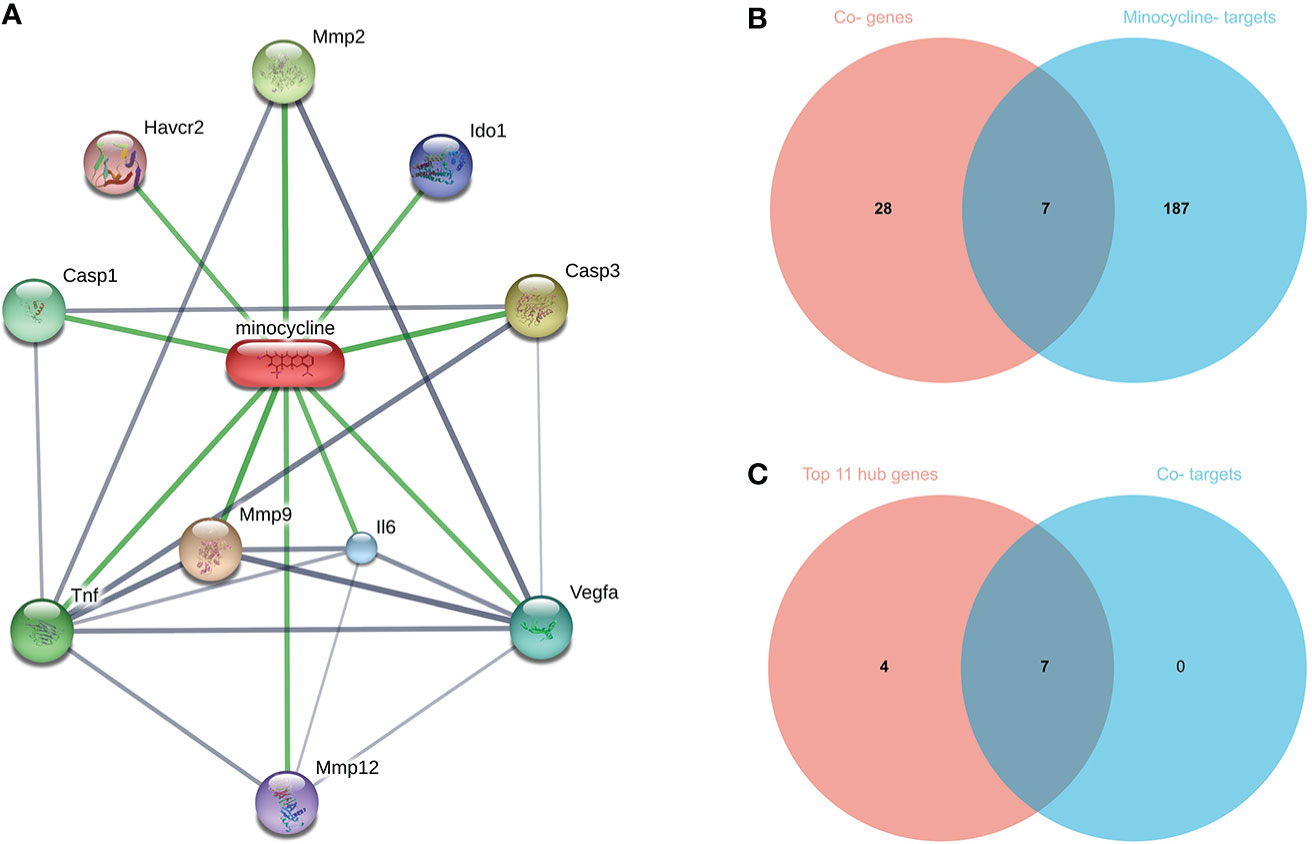
Figure 9 (A) Network diagram of minocycline and related target genes on the STITCH platform, with minocycline in capsules and related target genes in circles. (B) Venn-diagram of Co-genes versus minocycline-targets. (C) Venn diagram of the top 11 hub genes versus Co-targets, with Co-targets all contained in the top 11 hub genes.
TF-hub genes and miRNA-hub genes network for co-hub genes
The TF-hub genes network consists of 7 seeds, 51 edges, and 40 nodes (Figure 10A), and the simplified minimum network consists of 7 seeds, 19 edges, and 14 nodes (Figure 10B). YY1 has the potential to regulate CASP1, CASP8, and CXCL8. The miRNA-hub genes analyzed using the TarBase package consisted of 7 seeds, 407 edges, and 267 nodes (Figure 10C), and the simplified minimum network consisted of 7 seeds, 40 edges, and 17 nodes (Figure 10D). CASP1, CASP3, IL1B, CXCL8, and JUN were all closely related to hsa-mir-429. The miRNA-hub genes analyzed using the miRTarBase package consisted of 7 seeds, 210 edges, and 189 nodes (Figure 10E), and the simplified minimum network consisted of 7 seeds, 19 edges, and 14 nodes (Figure 10F). CASP1, CASP3, and CASP8 were all closely related to hsa-mir-34a-5p. In conclusion, YY1, hsa-mir-429, and hsa-mir-34a-5p may play an important role in the expression of CASP1.
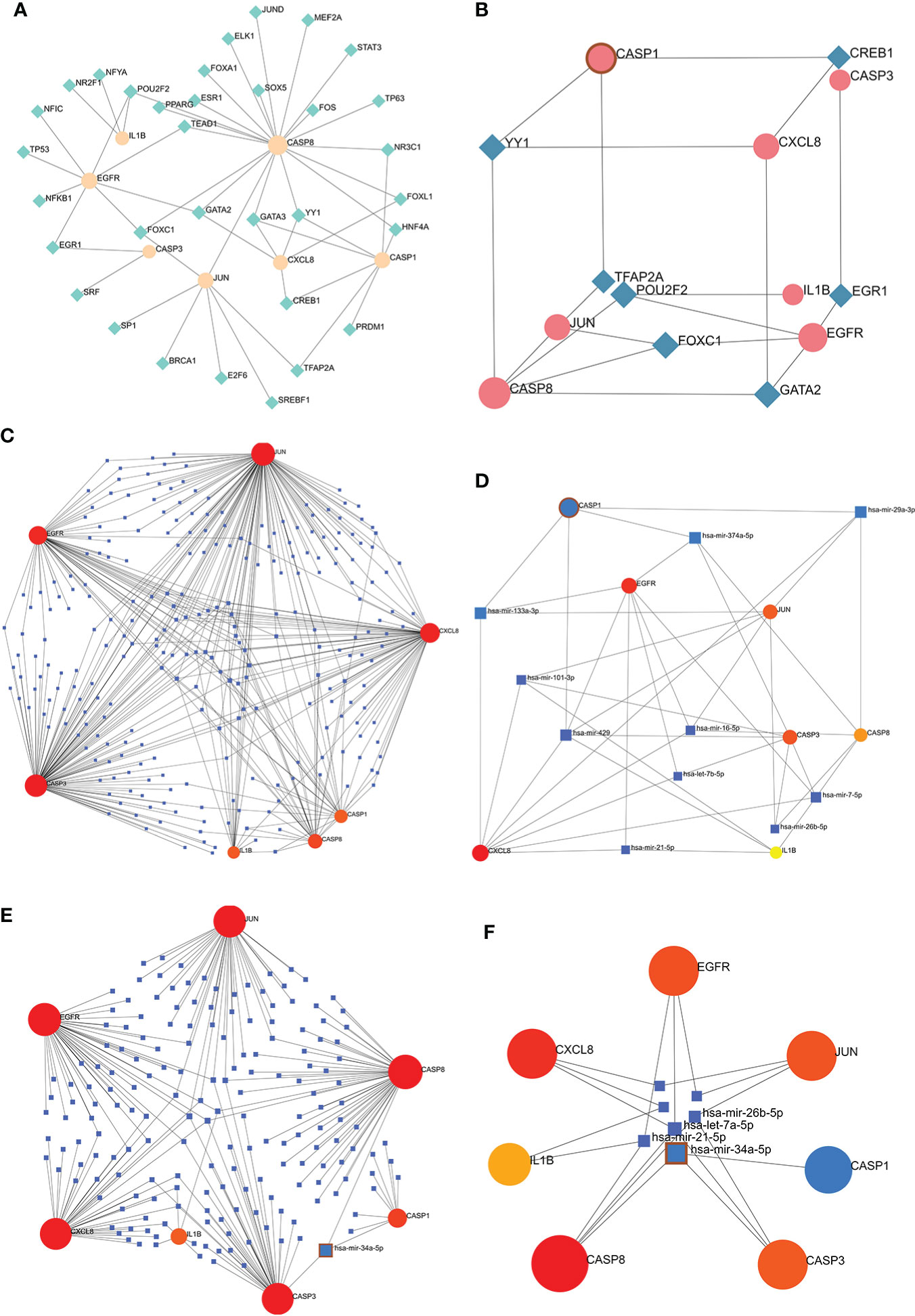
Figure 10 TF-hub genes and miRNA-hub genes network construction using NetworkAnalyst. (A, B) TF-hub genes network and simplified diagram. Circles were genes, while squares were TFs. (C, D) miRNA-hub genes network and simplified diagram (TarBase version 8.0). Circles represent genes, while squares are miRNAs. (E, F) miRNA-hub genes network and simplified diagram (miRTarBase v8.0). Circles represent genes, while squares are miRNAs.
Validation of co-hub genes and identification of key gene
900 DEGs were obtained from the GSE55457 validation set, of which 470 were upregulated genes and 430 were down-regulated genes (Figure 11A). 338 DEGs were obtained from the GSE93272 validation set, 322 upregulated genes, and 16 down-regulated genes (Figure 11B). The distribution of these two RA-DEGs was visualized separately using volcano plots. The only Key gene in the Venn-diagram intersection of the Co-hub genes with these two RA-DEGs is CASP1 (Figure 11C). CASP1 was highly expressed in the RA group in all three datasets (P<0.01) (Figures 11D–F). The AUC values of CASP1 in the GSE55235, GSE55457, and GSE93272 datasets were 0.97 (0.91-1.00), 0.88 (0.72-1.00), and 0.85 (0.79-0.90), respectively, all of which were greater than 0.8, using ROC curves to verify the diagnostic validity of CASP1 as a biomarker with good specificity and sensitivity (Figures 11G–I).
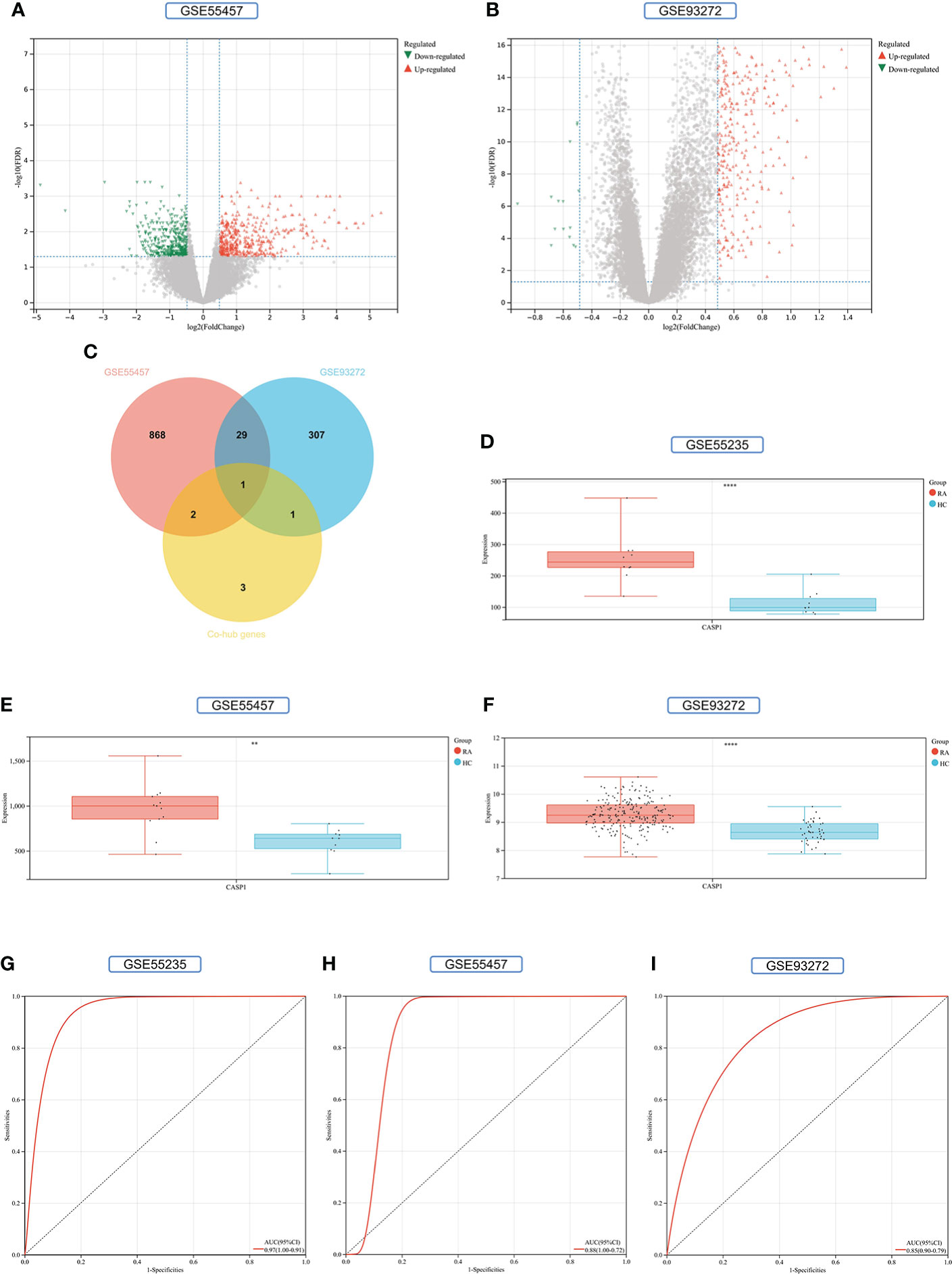
Figure 11 Screening and validation of key gene. (A) Volcano map of the GSE55457 dataset. (B) Volcano map of the GSE93272 dataset. Red triangles represent upregulated genes (P < 0.05), green triangles represent downregulated genes (P < 0.05), and gray dots represent genes not significantly differentially expressed across the RA and HC groups (P > 0.05). (C) Venn-diagram of RA-DEGs of GSE55457 and GSE93272 with Co-hub genes. (D–F) Expression of CASP1 in the GSE55235, GSE5457, and GSE93272 datasets, Red for the RA group and cyan for the HC group (**P < 0.01 and ****P < 0.0001). (G–I) The AUC of the ROC curve verifies the diagnostic validity of CASP1 in GSE55235, GSE55457和GSE93272 (P < 0.05).
Upstream pathway activity
SPEED2 analysis in the context of all human gene sets showed that Co-Hub Genes were associated with the IL-1 signaling pathway (Figure 12A), and the Key gene (CASP1) was associated with the Janus kinase/signal transducer and activator of transcription (JAK-STAT) signaling pathway (Figure 12B).
Immune infiltration analysis
In this study, LM22 immune cell infiltration data in RA (GSE93272) was obtained by the CIBERSORT algorithm. CASP1 was positively correlated with monocytes, dendritic cells activated, and neutrophils by Pearson correlation coefficient analysis (Figure 13A–C). Both the HPA and Monaco datasets in the HPA platform showed that the top three immune cells with high CASP1 expression were monocytes, dendritic cells (DCs), and neutrophils (Figures 13D, E), thus validating our results for immune infiltration analysis.
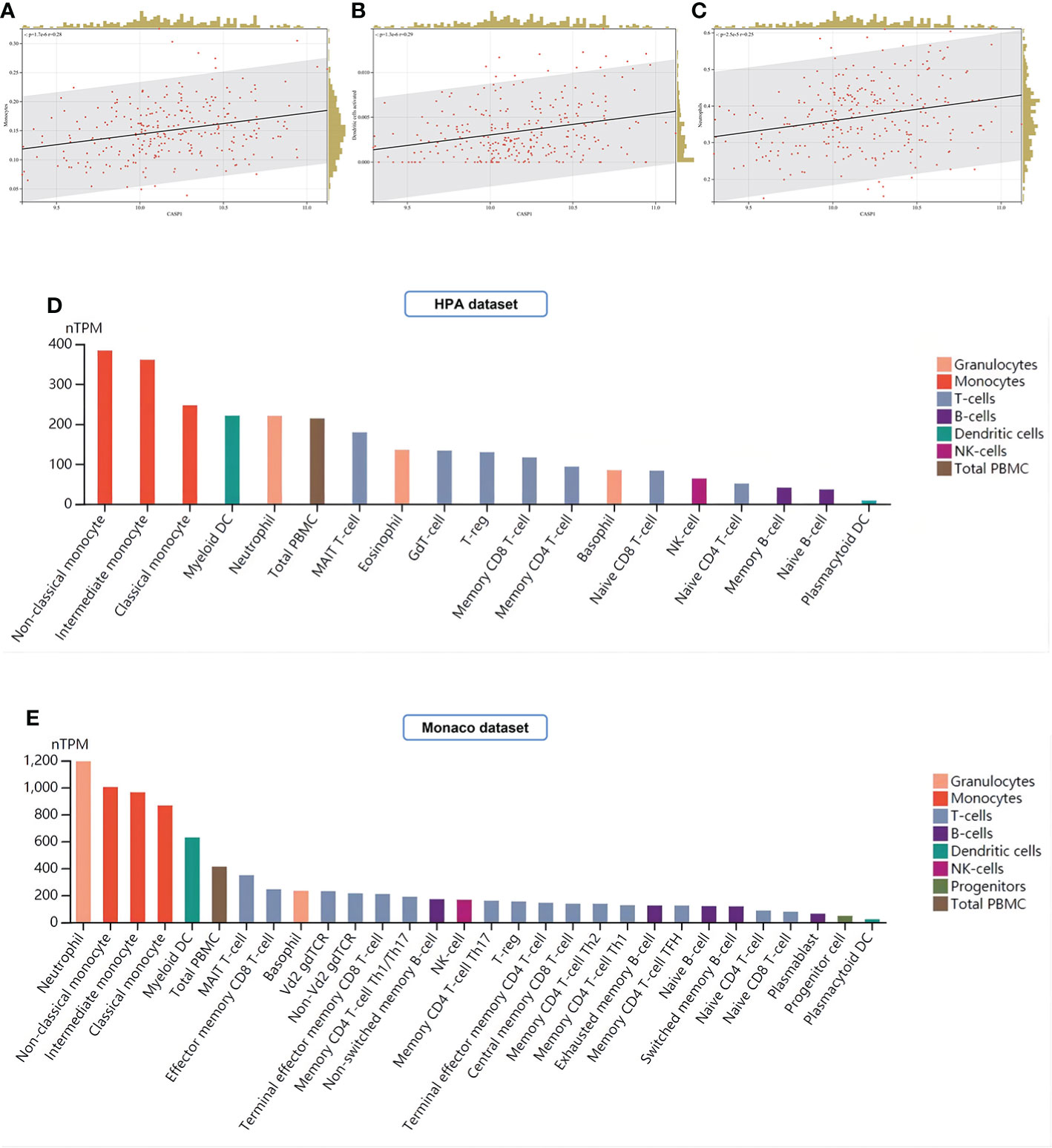
Figure 13 Analysis of immune cell infiltration. (A–C) Immune infiltrating cells positively associated with high CASP1 expression in LM22: Monocytes, Dendritic cells activated, and Neutrophils. (D, E) Distribution of CASP1 expression in immune cells from HPA datasets and Monaco datasets.
Molecular docking
A drug’s conformation within a protein target binding site can be predicted by molecular docking, which can also predict the binding affinity. We obtained the 2D and 3D structures of minocycline (Figures 14A, B) and showed by MD analysis that minocycline forms four hydrogen bonds with the four amino acid residues ASP-157, LYS-158, SER-159, and HIS-404 of caspase-1, allowing minocycline to bind tightly to the active pocket of caspase-1 to form a stable complex (Figure 14C).
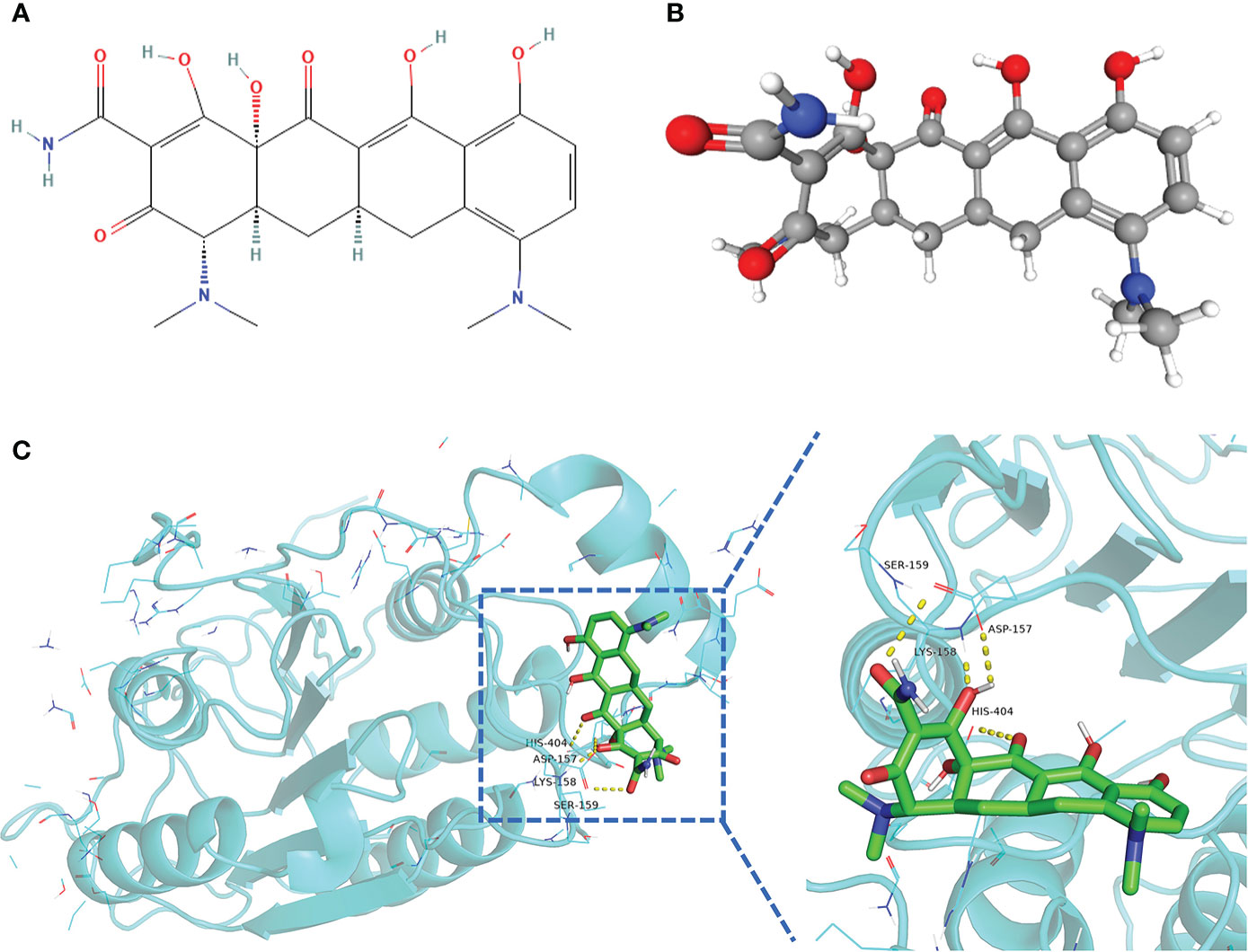
Figure 14 Structure of minocycline and molecular docking. (A, B) 2D and 3D structures of minocycline. (C) Results of molecular docking of minocycline with caspase-1 protein.
Molecular dynamics simulation and MM-GBSA
The MDS’s root-mean-square deviation (RMSD) depicts the movement of caspase-1 and minocycline; a greater value and amplitude of the RMSD suggests an intense movement and vice versa for a smooth movement. In Figure 15A, caspase-1 (red line) swings widely in the early portion of the simulation, begins to converge at 40 ns and plateaus later in the simulation, and caspase-1 fluctuates within 5Å overall, indicating that there has been no major disintegration. Minocycline’s (black line) value and amplitude were minor, fluctuating steadily around 1 Å and not reaching 1.5 Å. Typically, the RMSD of small molecules does not exceed 2 Å, indicating a weak conformational change. In conclusion, caspase-1 binds stably to the minocycline, almost tightly bound to the active site docked with caspase-1. The root-mean-square fluctuation (RMSF) indicates the flexibility of caspase-1 during the MDS process. When the drug attaches to the protein’s active site, its flexibility diminishes, stabilizing the protein and allowing the drug to have its biochemically active action. In Figure 15B, caspase-1 is composed of Chain A and Chain B. Overall, Chain A has a lower RMSF than Chain B, indicating that Chain A is less flexible. Minocycline interacts with Chain The start sequence of caspase-1 (the yellow background highlights the binding site) and the fact that the RMSF value for this region is less than 2 Å, indicating low protein flexibility, indicates that the binding of minocycline to caspase-1 is in a highly stable state.
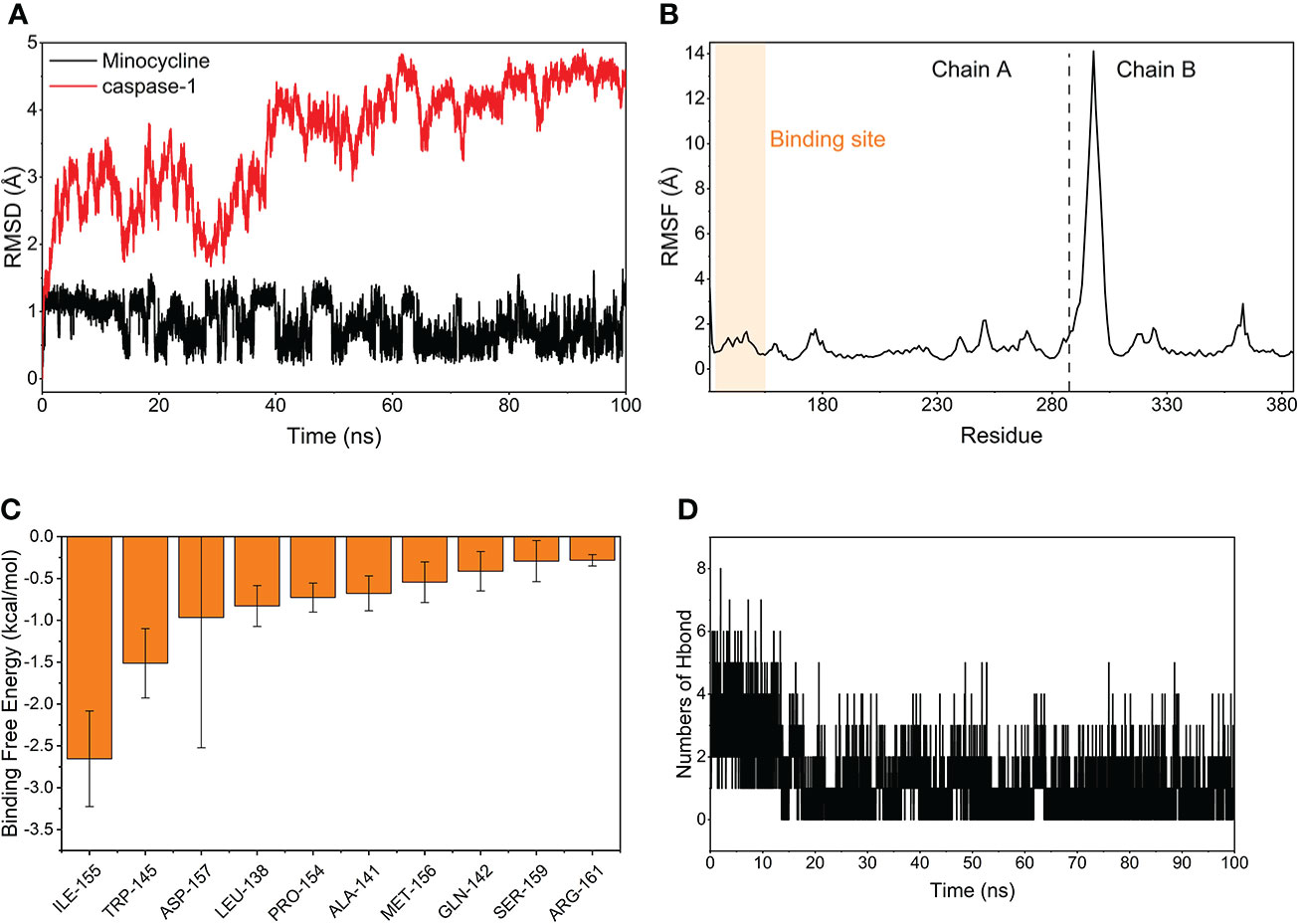
Figure 15 Molecular Dynamics Simulation and MM-GBSA. (A) Variation of the root means square deviation (RMSD) difference with time for small molecule compounds (black line) and proteins (red line) during molecular dynamics simulations. (B) Root mean square fluctuations (RMSF) are calculated based on molecular dynamics simulation trajectories. (C) The top 10 amino acids that contribute to small molecule and protein binding. (D) Changes in the number of hydrogen bonds between small molecules and proteins result from molecular dynamics simulations.
Based on MDS, the binding energy of minocycline to caspase-1 was determined using MM-GBSA. It can reflect the binding pattern of the medication to the protein more precisely. A negative binding energy value (ΔGbind) implies that the medication binds to the protein with affinity, whereas a smaller value indicates a greater binding capability. The binding energy of minocycline/caspase-1 was -21.43 ± 3.89 kcal/mol, showing that minocycline has a strong binding affinity for caspase-1. The energy decomposition reveals that van der Waals and electrostatic forces are the primary contributors to their binding (Table 3). The amino acid residue decomposition results of MM-GBSA can be more accurate than the active amino acid residues obtained by molecular docking. In Figure 15C, The top 10 amino acids that play a key role in minocycline/caspase-1 were: ILE-155, TRP-145, ASP-157, LEU-154, ALA-141, MET-156, GLN-142, SER-159, ARG-161. The ILE-155 ΔGbind is -2.625 kcal/mol, TRP-145 is -1.513 kcal/mol, and ASP-157 is -0.967 kcal/mol (Table 4). Thus ILE-155, TRP-145, and ASP-157 are the major and maintained by hydrogen bonding minocycline/caspase-1 tightly bound amino acids. Hydrogen bonding is one of the greatest forces for the non-covalent binding of medicines and proteins, and an investigation of the number of hydrogen bonds is required to comprehend the relationship between minocycline and caspase-1. Based on MDS trajectory monitoring, we acquired the coordinates of the number of hydrogen bond formations between minocycline and caspase-1 over time. In Figure 15D, In the early part of the simulation (0-20 ns), the number of hydrogen bonds fluctuated in the range of 1-5, and in the middle and late part of the simulation (20-100 ns), the number of hydrogen bonds was mainly concentrated in 1-2. Thus, minocycline interaction with caspase-1 relies heavily on 1-2 hydrogen bonding forces.
Discussion
35 Co-genes were obtained by the intersection of COVID-19, RA (GSE55235), and pyroptosis-related genes enriched in NLR/TLR signaling pathway, NLRP3 inflammasome complex, death-inducing signaling complex, regulation of interleukin production and cytokine production involved in immune responses. The top 11 hub genes in Metascape were enriched in the network map of the SARS-CoV-2 signaling pathway, activation of the NLRP3 inflammasome by SARS-CoV-2, NLR signaling pathway, and interleukins signaling pathway. While they were enriched in GeneMANIA in inflammasome complex, IL production pathway, NF-κB signaling, TNF signaling, and regulation of cytokine-mediated signaling pathway. CASP1 was most involved in these enrichment pathways. Minocycline was found to be closely associated with CASP1 by NetworkAnalyst analysis. Therefore, based on bioinformatics analysis and further network pharmacology analysis, it was surprising to find that the 7 Co-hub genes obtained from the intersection of minocycline with COVID-19, RA (GSE55235), and pyroptosis were all contained in the top 11 hub genes of COVID-19, RA (GSE55235), and pyroptosis. One important TF (YY1) and two important miRNAs (hsa-mir-429 and hsa-mir-34a-5p) associated with CASP1 were obtained by TF-hub genes and miRNA-hub genes network. The key gene was validated by the GSE55457 and GSE93272 validation sets and obtained as CASP1, which was highly expressed in the RA group in all three datasets and validated with ROC for significantly good test performance. This gene coincided with the results of previous pathway analysis. SPEED2 analysis indicates that CASP1 is associated with the JAK-STAT signaling pathway. Immune cell infiltration analysis revealed that monocytes, dendritic cells activated, and neutrophils were able to express CASP1 at high levels, and the reliability of the results was verified by using the HPA dataset and Monaco dataset databases. Finally, the relationship between minocycline and caspase-1 was investigated and verified by MD, MDS, and MM-GBSA: minocycline can dock close to the active site of caspase-1 to form a highly stable state and exert the biochemical activity of the drug.
Caspase-1 induces the classical pathway of pyroptosis
In this study, COVID-19, the crossover genes between RA and pyroptosis were enriched in the NLR/TLR signaling pathway, NLRP3 inflammasome complex, death-inducing signaling complex, regulation of interleukin production, NF-κB signaling, and TNF signaling. These pathways are all closely related to the caspase-1-induced pyroptosis pathway.
It is known that the innate immune system can recognize the viral pathogen-associated molecular pattern (PAMP) and host cell-derived damage-associated molecular pattern (DAMP) using the pathogen recognition receptor (PRR) (105–107). PRRs are divided into 2 main categories of 4 sensors: transmembrane proteins (TLRs, C-type lectin-receptors (CLRs)) and cytoplasmic proteins (RIG-I-like receptors (RLRs), NLRs) (108–110). NLRs, also known as versatile cytosolic sentinels (111, 112), play a significant role in the molecular processes (antigen presentation, inflammatory response, and cell death) linked to viral infectious diseases and autoimmune diseases (111, 113, 114). Five isoforms of NLRs, NLRA, NLRB, NLRC, NLRP, and NLRX1, activate two downstream signaling pathways: NOD1/NOD2 signaling and inflammasome signaling pathways (115), which recruit immune cells to produce pro-inflammatory cytokines (116). Caspases are a class of conserved cysteinyl proteases that activate themselves and other caspases by aspartate-specific cleavage (117) and can also cleave vast quantities of cellular substrates to drive cell death (e.g., apoptosis, pyroptosis) and inflammation (118). Caspases are classified as either apoptotic or inflammatory (119), with caspase-1 being the first member of the protease family of cysteases to be found (120) and the apical caspase of the inflammasome (121). caspase-1, one of the most typical inflammatory caspases, plays a crucial function in the regulation of pyroptosis and pro-inflammatory activities (122, 123). Since inflammatory caspases are inactive zymogens, they must be activated by the inflammasome to become proteolytically active (124). Inflammasomes are multiprotein complexes activated in response to endogenous and microbiological stimuli (125). The NLRP3 inflammasome is one of the most thoroughly researched and best-characterized inflammasomes in recent years (126), and it is the canonical activation platform for caspase-1 (127). The NLRP3 inflammasome is made up of a sensor (NLRP3), an adaptor (ASC), and an effector (caspase-1) (128). NLRP3 has a C-terminal Leucine rich repeat (LRR), a central nucleotide-binding and oligomerization domain (NACHT), and an N-terminal pyrin domain (PYD) (129, 130), whereas ASC has an N-terminal PYD and a C-terminal caspase recruitment domain (CARD) (131). full-length caspase-1 is composed of an N-terminal CARD, a main big catalytic domain (p20), and a C-terminal small catalytic subunit domain (p10) (132). PYD and CARD structural domains belong to the death domain (DD) fold superfamily (133).
NLRP3 inflammasome requires an initiation and activation pathway. The beginning step is the NF-κB-NLRP3 axis, in which the detection of PAMP/DAMP by a particular PRR (e.g., TLR) activates the NF-κB pathway, increasing NLRP3 expression (134, 135). During the initiation phase, phosphorylation and ubiquitination are further post-translational modifications of NLRP3 (136). The activation phase is the NLRP3/ASC/pro-caspase-1/caspase-1 axis, with NLRP3 recruiting the adaptor ASC through PYD-PYD interactions (137, 138), then ASC recruiting pro-Caspase-1 through CARD-CARD interactions (139, 140). Since autocatalytic activity permits autoconversion into p33 (both CARD and p20) and p10, removing CARD from the inflammasome after secondary autoconversion of caspase-1 p33/p10 releases an enzymatically active caspase-1 tetramer comprising p20/p10 subunits (141–143). There are two primary caspase-1 effector routes. One is the cleavage of pro-IL-1β and pro-IL-18 by the p20/p10 subunit of active caspase-1, which results in the release of IL-1β and IL-18 and the initiation of an inflammatory response (144–147). The second is for active caspase-1 to cleave and activate the executioner gasdermin D (GSDMD), cleave and remove its inhibitory GSDMD-C domain, and release the GSDMD-N domain (GSDMD-NT), allowing it to generate pores in the cell membrane and initiate pyroptosis (148–150).
Therefore, pyroptosis is a classical cytolytic type of PCD induced by caspase-1 (151). The pyroptosis pathway can be activated by various viral infections (64, 152–154) and can also be induced by autoantibodies to autoimmune diseases (AID) (155, 156). COVID-19 and RA share a tight relationship with the pyroptosis mechanism, which may be one of the pathogenic mechanisms by which COVID-19 interacts with RA to induce deterioration.
Caspase-1 in COVID-19
In this study, the top 11 hub genes pathways of COVID-19, RA, and pyroptosis were enriched in the Network map of the SARS-CoV-2 signaling pathway, Activation of the NLRP3 inflammasome by SARS-CoV-2, IL, NF-κB, TNF signaling pathway and regulation of cytokine-mediated signaling pathway. Caspase-1 activation is not only a critical effector molecule in the development of acute respiratory distress syndrome (ARDS) (157, 158), but it is also a major contributor to the development of ALI (159, 160). In peripheral blood immune cells and tissues of COVID-19 patients, activated NLRP3 inflammasome, caspase-1, and high levels of GSDMD-NT were found, as well as elevated expression of IL-1β and IL-18 in serum (161–166). In animal investigations, high caspase-1 expression was also detected in the peripheral immune cells of SARS-CoV-2-infected rhesus monkeys (167). With the in-depth study of the mechanism of pyroptosis triggered by SARS-CoV-2, it was found that NSP6 in non-structural proteins (74, 168), N-protein (169), and S-protein (170) in structural proteins, and ORF3a protein (171) in auxiliary proteins all lead to overexpression and activation of NLRP3 inflammasome and caspase-1 and are positively correlated with the severity of COVID-19 (164). SARS-CoV-2 ultimately leads to an excessive inflammatory response in the form of a “cytokine storm” (172–174) and severe host cell pyroptosis (175). Cytokine storm is an uncontrolled, lethal immune disease characterized by the excessive release of pro-inflammatory cytokines and chemical mediators from immune cells (176, 177), capable of causing damage to multiple organs, including the respiratory system (165, 178), and it is believed to be a major cause of deterioration and death in COVID-19 patients (179).
In this study, immune cell infiltration analysis of COVID-19, RA, and the key gene for pyroptosis (CASP1) was found to be positively correlated with Monocytes, and the reliability of the results was verified by the HPA dataset and Monaco dataset databases. Among the numerous immune cells, monocytes play a vital part in the cytokine storm of COVID-19 patients (180). It was demonstrated that monocytes in COVID-19 patients are the outposts of SARS-CoV-2 invasion via TLR sensing and can release inflammatory cytokines by assembling NLRP3, activating caspase-1 to generate a “cytokine storm,” and synthesizing GSDMD-NT to induce cellular pyroptosis (72, 168). Monocytes from COVID-19 patients not only overexpress IL-1β and IL-18 but also show pyroptosis morphology, suggesting that pyroptosis is a possible key mechanism for cytokine storm in COVID-19 (123, 166).
Caspase-1 in RA
The peripheral blood and synovial tissue of RA patients have been reported to contain a high level of expression and activation of the NLRP3 inflammasome and caspase-1, as well as a high level of expression of IL-1β and IL-18 (181–183). In animal investigations, inhibition of NLRP3 and caspase-1 was also found to be useful in alleviating the symptoms of arthritis in RA (CIA mouse model) (79). A cytokine network in the form of a cytokine storm, similar to that in COVID-19, is also present in RA and is a major factor in the disease’s onset, persistence, and progression (184, 185). The most important pro-inflammatory cytokines in RA are IL-1β and IL-18, and the expression of these cytokines is positively correlated with active disease status (186–188). In recent years the mechanism of pyroptosis has been shown to play a key role in the development of autoimmune diseases. In the course of the pro-inflammatory process, activation of the pyroptosis pathway causes host cells to release large amounts of pro-inflammatory cytokines and directs innate immune cells to the site of injury (119), which ultimately results in an overreactive immune response akin to a “cytokine storm” that sustains an ongoing autoimmune disease (189, 190).
In this study, immune cell infiltration analysis of the CASP1 in the RA dataset revealed that its expression was positively correlated with monocytes, dendritic cells activated, and neutrophils. It was found that high expression of NLRP3 and activated caspase-1 was detected in monocytes, dendritic cells, and neutrophils in the peripheral blood of RA patients, most notably in monocytes (181, 191, 192). Blood that circulates in the periphery Monocytes from RA patients can cleave GSDMD via the TLR4-NLRP3-caspase-1 pathway, resulting in pyroptosis and the production of a significant variety of cytokines, including IL-1β and IL-18, and are positively linked with disease activity (75, 193).
In conclusion, COVID-19 and RA are both capable of high expression of activated caspase-1 in peripheral blood and tissues. The invasion of SARS-CoV-2 in RA patients may enhance the caspase-1-induced pyroptosis mechanism, creating a vicious cycle of common outbreaks of “cytokine storm” and cell death, leading to increased hospitalization, morbidity, and mortality (194–197).
The JAK-STAT pathway upstream of caspase-1
In this study, the functional enrichment of the collection of Co-genes and the Top 11 Hub Genes included the regulation of IL-6 production, and the Upstream Pathway of the key gene (CASP1) was closely related to the JAK-STAT signaling pathway. The JAK/STAT pathway, also called the IL-6 signaling pathway, can be activated by IL-6 (198, 199), which is also a significant indication of COVID-19 severity (1, 200). Activation of the JAK/STAT pathway, which produces pro-inflammatory cytokines, also a significant role in the development of rheumatoid arthritis (RA) (201). Thus the JAK/STAT pathway is also one of the crosstalk pathways of COVID-19 and RA (202, 203). JAK inhibitors, represented by Tofacitinib, have been approved by the FDA to treat moderately and severely active RA (204, 205). However, it increases the risk of viral infection (206, 207). Since IFN can trigger the JAK/STAT pathway to launch a cascade response against viral infection (208), JAK inhibitors would interfere with the natural IFN/ISG antiviral immune system in the context of SARS-CoV-2 infection. Currently, the WHO only advises baricitinib for the treatment of severe COVID-19 (209), and the evidence for the use of JAK inhibitors in the treatment of COVID-19 is weak and requires additional investigation (210–212). Since the JAK/STAT pathway can promote caspase-1 expression and activation via cytokines (e.g., GM-CSF) and interferons (e.g., IFN-γ) (213–216), this study, in conjunction with other evidence, suggests that the NLRP3/caspase-1 pathway is a key mechanism by which COVID-19 and RA disease exacerbate each other.
Therefore, we can look for drug targets downstream of the JAK/STAT pathway to avoid interfering with the IFN/ISG system by inhibiting the JAK/STAT pathway, but also to effectively inhibit the pyroptosis link, interrupting the “cytokine storm” that erupts from each other and thus interrupting the vicious cycle. Interestingly, caspase-1 is one of the common crosstalk targets between JAK/STAT and pyroptosis pathways.
Minocycline and caspase-1
In the present COVID-19 pandemic, the discovery of new medications is challenging, time-consuming, risky, and less successful, and drug repurposing is a good option (217, 218). Minocycline is a second-generation semi-synthetic tetracycline derivative with a good safety profile (219). In addition to being a broad-spectrum antibiotic (220), it is also a broad-spectrum antiviral agent (e.g., HIV, WNV, DENV) (221–223) and possesses anti-inflammatory, antioxidant, anti-cell death (e.g., pyroptosis), immunomodulatory effects in terms of non-anti-microbial action (224–226). Fundamental investigations have demonstrated that minocycline inhibits caspase-1 activity in mice suffering from traumatic brain injury (TBI) (227); reduces the expression of caspase-1 to alleviate stress-induced depression in mice (228); acts as a caspase-1 inhibitor to delay the death of mice with Huntington’s disease (229); reduces caspase-1 activity in the retina of diabetic mice (230) and suppresses caspase-1 activation in mice with acute lung injury to reduce inflammation (231). Retrospective multicentre cohort studies have shown that minocycline inhibits caspase-1 to reduce the incidence of acute renal failure (232). In conclusion, minocycline can reduce IL-1β and IL-18 levels by selectively inhibiting caspase-1 expression and activation, and it can have anti-inflammatory and anti-pyroptosis effects in the lung and throughout the body. Minocycline could play an important potential role in treating patients with COVID-19 through these properties (233) and exert a powerful antimicrobial effect against co-infections/secondary bacterial infections in patients with COVID-19 (234, 235). A current clinical study indicates that the combination of minocycline and favipiravir has significant efficacy and safety in treating COVID-19 inpatients (236). Minocycline has also demonstrated efficacy in treating COVID-19 individuals who are secluded at home (237). In addition, minocycline has been known to be clearly and effectively used in treating RA for many years (238–240).
Thus, minocycline can counteract the “cytokine storm” inflammatory response and resist pyroptosis in patients with COVID-19 combined with RA by inhibiting the expression and activation of caspase-1. This process also indirectly demonstrates a potential caspase-1-directed pyroptosis and a shared pro-inflammatory mechanism between COVID-19 and RA, which requires further basic and clinical research.
Conclusions
Bioinformatic analysis revealed that COVID-19, RA, and pyroptosis-related genes were enriched in pyroptosis and pro-inflammatory pathways (NLR/TLR signaling pathway, NLRP3 inflammasome complex, death-inducing signaling complex, regulation of interleukin production), natural immune pathways (activation of the NLRP3 inflammasome by SARS-CoV-2) and COVID-19-and RA-related cytokine storm pathways (IL, NF-κB, TNF signaling pathway and regulation of cytokine-mediated signaling). Of these, CASP1 is involved in most pathways. The genes related to minocycline were then obtained by network pharmacology analysis and intersected with COVID-19, RA, and pyroptosis to obtain the common hub gene, and then the key gene was verified as CASP1 by two validation sets. Caspase-1 may be an important mediator of the excessive inflammatory response induced by SARS-CoV-2 in RA patients through pyroptosis. Finally, minocycline was analyzed by computer-aided drug design as an effective drug against the mechanism of caspase-1-induced pyroptosis. Our study provides insight into the causes of the high hospitalization and mortality rates of COVID-19 combined with RA from a new perspective of pyroptosis and offers potentially effective drugs that could provide new directions for further analysis of its pathogenesis and the development of targeted clinical treatments.
Data availability statement
The datasets presented in this study can be found in online repositories. The names of the repository/repositories and accession number(s) can be found in the article/Supplementary Material.
Author contributions
QZ, RL and YC: Consulted the literature and prepared materials. QZ, RL, YC, QL, JZ and JBZ: Experimented and analyzed the data. QZ, RL and YC: Drawn up the manuscript. WW and WX devised the concept and supervised the study. All authors contributed to the article and approved the submitted version.
Acknowledgments
We acknowledge the GEO and Genecards databases for providing their platforms and contributors for uploading meaningful datasets.
Conflict of interest
The authors declare that the research was conducted in the absence of any commercial or financial relationships that could be construed as a potential conflict of interest.
Publisher’s note
All claims expressed in this article are solely those of the authors and do not necessarily represent those of their affiliated organizations, or those of the publisher, the editors and the reviewers. Any product that may be evaluated in this article, or claim that may be made by its manufacturer, is not guaranteed or endorsed by the publisher.
Supplementary material
The Supplementary Material for this article can be found online at: https://www.frontiersin.org/articles/10.3389/fimmu.2022.1058884/full#supplementary-material
Glossary
References
1. Huang C, Wang Y, Li X, Ren L, Zhao J, Hu Y, et al. Clinical features of patients infected with 2019 novel coronavirus in wuhan, China. Lancet (2020) 395(10223):497–506. doi: 10.1016/S0140-6736(20)30183-5
2. Chen N, Zhou M, Dong X, Qu J, Gong F, Han Y, et al. Epidemiological and clinical characteristics of 99 cases of 2019 novel coronavirus pneumonia in wuhan, China: a descriptive study. Lancet (2020) 395(10223):507–13. doi: 10.1016/S0140-6736(20)30211-7
3. Lu R, Zhao X, Li J, Niu P, Yang B, Wu H, et al. Genomic characterisation and epidemiology of 2019 novel coronavirus: implications for virus origins and receptor binding. Lancet (2020) 395(10224):565–74. doi: 10.1016/S0140-6736(20)30251-8
4. Poon LLM, Peiris M. Emergence of a novel human coronavirus threatening human health. Nat Med (2020) 26(3):317–9. doi: 10.1038/s41591-020-0796-5
5. Jiang S, Xia S, Ying T, Lu L. A novel coronavirus (2019-nCoV) causing pneumonia-associated respiratory syndrome. Cell Mol Immunol (2020) 17(5):554. doi: 10.1038/s41423-020-0372-4
6. Zhou P, Yang XL, Wang XG, Hu B, Zhang L, Zhang W, et al. A pneumonia outbreak associated with a new coronavirus of probable bat origin. Nature (2020) 579(7798):270–3. doi: 10.1038/s41586-020-2012-7
7. Parry J. WHO issues guidelines to manage any future SARS outbreak. BMJ (2003) 327(7412):411. doi: 10.1136/bmj.327.7412.411-a
8. Drazen JM. SARS–looking back over the first 100 days. N Engl J Med (2003) 349(4):319–20. doi: 10.1056/NEJMp038118
9. Assiri A, McGeer A, Perl TM, Price CS, Al Rabeeah AA, Cummings DA, et al. Hospital outbreak of middle East respiratory syndrome coronavirus. N Engl J Med (2013) 369(5):407–16. doi: 10.1056/NEJMoa1306742
10. de Groot RJ, Baker SC, Baric RS, Brown CS, Drosten C, Enjuanes L, et al. Middle East respiratory syndrome coronavirus (MERS-CoV): announcement of the coronavirus study group. J Virol (2013) 87(14):7790–2. doi: 10.1128/JVI.01244-13
11. Wiersinga WJ, Rhodes A, Cheng AC, Peacock SJ, Prescott HC. Pathophysiology, transmission, diagnosis, and treatment of coronavirus disease 2019 (COVID-19): A review. JAMA (2020) 324(8):782–93. doi: 10.1001/jama.2020.12839
12. Guan WJ, Ni ZY, Hu Y, Liang WH, Ou CQ, He JX, et al. China Medical treatment expert group for covid-19. clinical characteristics of coronavirus disease 2019 in China. N Engl J Med (2020) 382(18):1708–20. doi: 10.1056/NEJMoa2002032
13. Riou J, Althaus CL. Pattern of early human-to-human transmission of wuhan 2019 novel coronavirus (2019-nCoV), December 2019 to January 2020. Euro Surveill. (2020) 25(4):2000058. doi: 10.2807/1560-7917.ES.2020.25.4.2000058
14. Johns Hopkins Coronavirus Resource Center. COVID-19 dashboard (2021). Available at: https://coronavirus.jhu.edu/map.html.
15. Masters PS. The molecular biology of coronaviruses. Adv Virus Res (2006) 66:193–292. doi: 10.1016/S0065-3527(06)66005-3
16. Xu Z, Shi L, Wang Y, Zhang J, Huang L, Zhang C, et al. Pathological findings of COVID-19 associated with acute respiratory distress syndrome. Lancet Respir Med (2020) 8(4):420–2. doi: 10.1016/S2213-2600(20)30076-X
17. Cui J, Li F, Shi ZL. Origin and evolution of pathogenic coronaviruses. Nat Rev Microbiol (2019) 17(3):181–92. doi: 10.1038/s41579-018-0118-9
18. Holmes EC, Goldstein SA, Rasmussen AL, Robertson DL, Crits-Christoph A, Wertheim JO, et al. The origins of SARS-CoV-2: A critical review. Cell (2021) 184(19):4848–56. doi: 10.1016/j.cell.2021.08.017
19. Ye ZW, Yuan S, Yuen KS, Fung SY, Chan CP, Jin DY. Zoonotic origins of human coronaviruses. Int J Biol Sci (2020) 16(10):1686–97. doi: 10.7150/ijbs.45472
20. Bai C, Zhong Q, Gao GF. Overview of SARS-CoV-2 genome-encoded proteins. Sci China Life Sci (2022) 65(2):280–94. doi: 10.1007/s11427-021-1964-4
21. Brant AC, Tian W, Majerciak V, Yang W, Zheng ZM. SARS-CoV-2: from its discovery to genome structure, transcription, and replication. Cell Biosci (2021) 11(1):136. doi: 10.1186/s13578-021-00643-z
22. Tonkin-Hill G, Martincorena I, Amato R, Lawson ARJ, Gerstung M, Johnston I, et al. Wellcome Sanger institute COVID-19 surveillance team. patterns of within-host genetic diversity in SARS-CoV-2. Elife (2021) 10:e66857. doi: 10.7554/eLife.66857
23. Focosi D, Maggi F. Recombination in coronaviruses, with a focus on SARS-CoV-2. Viruses (2022) 14(6):1239. doi: 10.3390/v14061239
24. Yadav R, Chaudhary JK, Jain N, Chaudhary PK, Khanra S, Dhamija P, et al. Role of structural and non-structural proteins and therapeutic targets of SARS-CoV-2 for COVID-19. Cells (2021) 10(4):821. doi: 10.3390/cells10040821
25. Wong NA, Saier MH Jr. The SARS-coronavirus infection cycle: A survey of viral membrane proteins, their functional interactions and pathogenesis. Int J Mol Sci (2021) 22(3):1308. doi: 10.3390/ijms22031308
26. Rohaim MA, El Naggar RF, Clayton E, Munir M. Structural and functional insights into non-structural proteins of coronaviruses. Microb Pathog (2021) 150:104641. doi: 10.1016/j.micpath.2020.104641
27. Yang H, Rao Z. Structural biology of SARS-CoV-2 and implications for therapeutic development. Nat Rev Microbiol (2021) 19(11):685–700. doi: 10.1038/s41579-021-00630-8
28. Naqvi AAT, Fatima K, Mohammad T, Fatima U, Singh IK, Singh A, et al. Insights into SARS-CoV-2 genome, structure, evolution, pathogenesis and therapies: Structural genomics approach. Biochim Biophys Acta Mol Basis Dis (2020) 1866(10):165878. doi: 10.1016/j.bbadis.2020.165878
29. Ashraf UM, Abokor AA, Edwards JM, Waigi EW, Royfman RS, Hasan SA, et al. SARS-CoV-2, ACE2 expression, and systemic organ invasion. Physiol Genomics (2021) 53(2):51–60. doi: 10.1152/physiolgenomics.00087.2020
30. Flaumenhaft R, Enjyoji K, Schmaier AA. Vasculopathy in COVID-19. Blood (2022) 140(3):222–35. doi: 10.1182/blood.2021012250
31. Kim H, Byun JE, Yoon SR, Koohy H, Jung H, Choi I. SARS-CoV-2 peptides bind to NKG2D and increase NK cell activity. Cell Immunol (2022) 371:104454. doi: 10.1016/j.cellimm.2021.104454
32. Saheb Sharif-Askari N, Saheb Sharif-Askari F, Ahmed SBM, Hannawi S, Hamoudi R, Hamid Q, et al. Enhanced expression of autoantigens during SARS-CoV-2 viral infection. Front Immunol (2021) 12:686462. doi: 10.3389/fimmu.2021.686462
33. Vojdani A, Kharrazian D. Potential antigenic cross-reactivity between SARS-CoV-2 and human tissue with a possible link to an increase in autoimmune diseases. Clin Immunol (2020) 217:108480. doi: 10.1016/j.clim.2020.108480
34. Dotan A, Muller S, Kanduc D, David P, Halpert G, Shoenfeld Y. The SARS-CoV-2 as an instrumental trigger of autoimmunity. Autoimmun Rev (2021) 20(4):102792. doi: 10.1016/j.autrev.2021.102792
35. Liu Y, Sawalha AH, Lu Q. COVID-19 and autoimmune diseases. Curr Opin Rheumatol (2021) 33(2):155–62. doi: 10.1097/BOR.0000000000000776
36. Zhong J, Shen G, Yang H, Huang A, Chen X, Dong L, et al. COVID-19 in patients with rheumatic disease in hubei province, China: a multicentre retrospective observational study. Lancet Rheumatol (2020) 2(9):e557–64. doi: 10.1016/S2665-9913(20)30227-7
37. Tang KT, Hsu BC, Chen DY. Autoimmune and rheumatic manifestations associated with COVID-19 in adults: An updated systematic review. Front Immunol (2021) 12:645013. doi: 10.3389/fimmu.2021.645013
38. Ishay Y, Kenig A, Tsemach-Toren T, Amer R, Rubin L, Hershkovitz Y, et al. Autoimmune phenomena following SARS-CoV-2 vaccination. Int Immunopharmacol. (2021) 99:107970. doi: 10.1016/j.intimp.2021.107970
39. Bartels LE, Ammitzbøll C, Andersen JB, Vils SR, Mistegaard CE, Johannsen AD, et al. Local and systemic reactogenicity of COVID-19 vaccine BNT162b2 in patients with systemic lupus erythematosus and rheumatoid arthritis. Rheumatol Int (2021) 41(11):1925–31. doi: 10.1007/s00296-021-04972-7
40. Ferri C, Giuggioli D, Raimondo V, L’Andolina M, Tavoni A, Cecchetti R, et al. COVID-19 & ASD Italian study group. COVID-19 and rheumatic autoimmune systemic diseases: report of a large Italian patients series. Clin Rheumatol (2020) 39(11):3195–204. doi: 10.1007/s10067-020-05334-7
41. Freites Nuñez DD, Leon L, Mucientes A, Rodriguez-Rodriguez L, Font Urgelles J, Madrid García A, et al. Risk factors for hospital admissions related to COVID-19 in patients with autoimmune inflammatory rheumatic diseases. Ann Rheum Dis (2020) 79(11):1393–9. doi: 10.1136/annrheumdis-2020-217984
42. The COVID-19 global rheumatology alliance global registry (2020). Available at: https://rheum-covid.org/updates/combined-data.html (Accessed 17 Aug).
43. Scott DL, Wolfe F, Huizinga TW. Rheumatoid arthritis. Lancet (2010) 376(9746):1094–108. doi: 10.1016/S0140-6736(10)60826-4
44. Smolen JS, Aletaha D, McInnes IB. Rheumatoid arthritis. Lancet (2016) 388(10055):2023–38. doi: 10.1016/S0140-6736(16)30173-8
45. McInnes IB, Schett G. The pathogenesis of rheumatoid arthritis. N Engl J Med (2011) 365(23):2205–19. doi: 10.1056/NEJMra1004965
46. van der Woude D, van der Helm-van Mil AHM. Update on the epidemiology, risk factors, and disease outcomes of rheumatoid arthritis. Best Pract Res Clin Rheumatol (2018) 32:174–87. doi: 10.1016/j.berh.2018.10.005
47. Safiri S, Kolahi AA, Hoy D, Smith E, Bettampadi D, Mansournia MA, et al. Global, regional and national burden of rheumatoid arthritis 1990-2017: A systematic analysis of the global burden of disease study 2017. Ann Rheum Dis (2019) 78(11):1463–71. doi: 10.1136/annrheumdis-2019-215920
48. Karami J, Aslani S, Jamshidi A, Garshasbi M, Mahmoudi M. Genetic implications in the pathogenesis of rheumatoid arthritis; an updated review. Gene (2019) 702:8–16. doi: 10.1016/j.gene.2019.03.033
49. Firestein GS, McInnes IB. Immunopathogenesis of rheumatoid arthritis. Immunity (2017) 46(2):183–96. doi: 10.1016/j.immuni.2017.02.006
50. Joo YB, Lim YH, Kim KJ, Park KS, Park YJ. Respiratory viral infections and the risk of rheumatoid arthritis. Arthritis Res Ther (2019) 21(1):199. doi: 10.1186/s13075-019-1977-9
51. Klatt T, Ouyang Q, Flad T, Koetter I, Bühring HJ, Kalbacher H, et al. Expansion of peripheral CD8+ CD28- T cells in response to Epstein-Barr virus in patients with rheumatoid arthritis. J Rheumatol (2005) 32(2):239–51.
52. van Delft MAM, Huizinga TWJ. An overview of autoantibodies in rheumatoid arthritis. J Autoimmun (2020) 110:102392. doi: 10.1016/j.jaut.2019.102392
53. Scherer HU, Häupl T, Burmester GR. The etiology of rheumatoid arthritis. J Autoimmun (2020) 110:102400. doi: 10.1016/j.jaut.2019.102400
54. Furst DE. The risk of infections with biologic therapies for rheumatoid arthritis. Semin Arthritis Rheumatol (2010) 39(5):327–46. doi: 10.1016/j.semarthrit.2008.10.002
55. Roongta R, Ghosh A. Managing rheumatoid arthritis during COVID-19. Clin Rheumatol (2020) 39(11):3237–44. doi: 10.1007/s10067-020-05358-z
56. Dixon WG. Rheumatoid arthritis: biological drugs and risk of infection. Lancet (2015) 386(9990):224–5. doi: 10.1016/S0140-6736(14)61907-3
57. Bongartz T, Sutton AJ, Sweeting MJ, Buchan I, Matteson EL, Montori V. Anti-TNF antibody therapy in rheumatoid arthritis and the risk of serious infections and malignancies: systematic review and meta-analysis of rare harmful effects in randomized controlled trials. JAMA (2006) 295(19):2275–85. doi: 10.1001/jama.295.19.2275
58. Galluzzi L, Vitale I, Aaronson SA, Abrams JM, Adam D, Agostinis P, et al. Molecular mechanisms of cell death: recommendations of the nomenclature committee on cell death 2018. Cell Death Differ (2018) 25(3):486–541. doi: 10.1038/s41418-017-0012-4
59. Jorgensen I, Miao EA. Pyroptotic cell death defends against intracellular pathogens. Immunol Rev (2015) 265(1):130–42. doi: 10.1111/imr.12287
60. Fink SL, Cookson BT. Caspase-1-dependent pore formation during pyroptosis leads to osmotic lysis of infected host macrophages. Cell Microbiol (2006) 8(11):1812–25. doi: 10.1111/j.1462-5822.2006.00751.x
61. Kovacs SB, Miao EA. Gasdermins: Effectors of pyroptosis. Trends Cell Biol (2017) 27(9):673–84. doi: 10.1016/j.tcb.2017.05.005
62. Cookson BT, Brennan MA. Pro-inflammatory programmed cell death. Trends Microbiol (2001) 9(3):113–4. doi: 10.1016/s0966-842x(00)01936-3
63. Black RA, Kronheim SR, Merriam JE, March CJ, Hopp TP. A pre-aspartate-specific protease from human leukocytes that cleaves pro-interleukin-1 beta. J Biol Chem (1989) 264:5323–6. doi: 10.1016/S0021-9258(18)83546-3
64. Bergsbaken T, Fink SL, Cookson BT. Pyroptosis: host cell death and inflammation. Nat Rev Microbiol (2009) 7(2):99–109. doi: 10.1038/nrmicro2070
65. Zychlinsky A, Prevost MC, Sansonetti PJ. Shigella flexneri induces apoptosis in infected macrophages. Nature (1992) 358(6382):167–9. doi: 10.1038/358167a0
66. Kayagaki N, Stowe IB, Lee BL, O’Rourke K, Anderson K, Warming S, et al. Caspase-11 cleaves gasdermin d for non-canonical inflammasome signalling. Nature (2015) 526(7575):666–71. doi: 10.1038/nature15541
67. Qiu S, Liu J, Xing F. ‘Hints’ in the killer protein gasdermin d: unveiling the secrets of gasdermins driving cell death. Cell Death Differ (2017) 24(4):588–96. doi: 10.1038/cdd.2017.24
68. Shi J, Zhao Y, Wang K, Shi X, Wang Y, Huang H, et al. Cleavage of GSDMD by inflammatory caspases determines pyroptotic cell death. Nature (2015) 526(7575):660–5. doi: 10.1038/nature15514
69. He WT, Wan H, Hu L, Chen P, Wang X, Huang Z, et al. Gasdermin d is an executor of pyroptosis and required for interleukin-1β secretion. Cell Res (2015) 25(12):1285–98. doi: 10.1038/cr.2015.139
70. Liu X, Zhang Z, Ruan J, Pan Y, Magupalli VG, Wu H, et al. Inflammasome-activated gasdermin d causes pyroptosis by forming membrane pores. Nature (2016) 535(7610):153–8. doi: 10.1038/nature18629
71. Rodrigues TS, de Sá KSG, Ishimoto AY, Becerra A, Oliveira S, Almeida L, et al. Inflammasomes are activated in response to SARS-CoV-2 infection and are associated with COVID-19 severity in patients. J Exp Med (2021) 218(3):e20201707. doi: 10.1084/jem.20201707
72. Junqueira C, Crespo Â, Ranjbar S, de Lacerda LB, Lewandrowski M, Ingber J, et al. FcγR-mediated SARS-CoV-2 infection of monocytes activates inflammation. Nature (2022) 606(7914):576–84. doi: 10.1038/s41586-022-04702-4
73. Lara PC, Macías-Verde D, Burgos-Burgos J. Age-induced NLRP3 inflammasome over-activation increases lethality of SARS-CoV-2 pneumonia in elderly patients. Aging Dis (2020) 11(4):756–62. doi: 10.14336/AD.2020.0601
74. Sun X, Liu Y, Huang Z, Xu W, Hu W, Yi L, et al. SARS-CoV-2 non-structural protein 6 triggers NLRP3-dependent pyroptosis by targeting ATP6AP1. Cell Death Differ (2022) 29(6):1240–54. doi: 10.1038/s41418-021-00916-7
75. Wu XY, Li KT, Yang HX, Yang B, Lu X, Zhao LD, et al. Complement C1q synergizes with PTX3 in promoting NLRP3 inflammasome over-activation and pyroptosis in rheumatoid arthritis. J Autoimmun (2020) 106:102336. doi: 10.1016/j.jaut.2019.102336
76. Spel L, Martinon F. Inflammasomes contributing to inflammation in arthritis. Immunol Rev (2020) 294(1):48–62. doi: 10.1111/imr.12839
77. Zhang Y, Yang W, Li W, Zhao Y. NLRP3 inflammasome: Checkpoint connecting innate and adaptive immunity in autoimmune diseases. Front Immunol (2021) 12:732933. doi: 10.3389/fimmu.2021.732933
78. Shen HH, Yang YX, Meng X, Luo XY, Li XM, Shuai ZW, et al. NLRP3: A promising therapeutic target for autoimmune diseases. Autoimmun Rev (2018) 17(7):694–702. doi: 10.1016/j.autrev.2018.01.020
79. Guo C, Fu R, Wang S, Huang Y, Li X, Zhou M, et al. NLRP3 inflammasome activation contributes to the pathogenesis of rheumatoid arthritis. Clin Exp Immunol (2018) 194(2):231–43. doi: 10.1111/cei.13167
80. Safran M, Dalah I, Alexander J, Rosen N, Iny Stein T, Shmoish M, et al. GeneCards version 3: the human gene integrator. Database (Oxford). (2010) 2010:baq020. doi: 10.1093/database/baq020
81. Xiong Y, Liu Y, Cao L, Wang D, Guo M, Jiang A, et al. Transcriptomic characteristics of bronchoalveolar lavage fluid and peripheral blood mononuclear cells in COVID-19 patients. Emerg Microbes Infect (2020) 9(1):761–70. doi: 10.1080/22221751.2020.1747363
82. Ziegler CGK, Allon SJ, Nyquist SK, Mbano IM, Miao VN, Tzouanas CN, et al. HCA lung biological network. electronic address:bHVuZy1uZXR3b3JrQGh1bWFuY2VsbGF0bGFzLm9yZw==; HCA lung biological network. SARS-CoV-2 receptor ACE2 is an interferon-stimulated gene in human airway epithelial cells and is detected in specific cell subsets across tissues. Cell (2020) 181(5):1016–1035.e19. doi: 10.1016/j.cell.2020.04.035
83. Jain R, Ramaswamy S, Harilal D, Uddin M, Loney T, Nowotny N, et al. Host transcriptomic profiling of COVID-19 patients with mild, moderate, and severe clinical outcomes. Comput Struct Biotechnol J (2020) 19:153–60. doi: 10.1016/j.csbj.2020.12.016
84. Ritchie ME, Phipson B, Wu D, Hu Y, Law CW, Shi W, et al. Limma powers differential expression analyses for RNA-sequencing and microarray studies. Nucleic Acids Res (2015) 43(7):e47. doi: 10.1093/nar/gkv007
85. Yu G, Wang LG, Han Y, He QY. clusterProfiler: an r package for comparing biological themes among gene clusters. OMICS (2012) 16(5):284–7. doi: 10.1089/omi.2011.0118
86. Szklarczyk D, Gable AL, Lyon D, Junge A, Wyder S, Huerta-Cepas J, et al. STRING v11: protein-protein association networks with increased coverage, supporting functional discovery in genome-wide experimental datasets. Nucleic Acids Res (2019) 47(D1):D607–13. doi: 10.1093/nar/gky1131
87. Sarkar D, Saha S. Machine-learning techniques for the prediction of protein-protein interactions. J Biosci (2019) 44(4):104. doi: 10.1007/s12038-019-9909-z
88. Shannon P, Markiel A, Ozier O, Baliga NS, Wang JT, Ramage D, et al. Cytoscape: a software environment for integrated models of biomolecular interaction networks. Genome Res (2003) 13(11):2498–504. doi: 10.1101/gr.1239303
89. Zhou Y, Zhou B, Pache L, Chang M, Khodabakhshi AH, Tanaseichuk O, et al. Metascape provides a biologist-oriented resource for the analysis of systems-level datasets. Nat Commun (2019) 10(1):1523. doi: 10.1038/s41467-019-09234-6
90. Warde-Farley D, Donaldson SL, Comes O, Zuberi K, Badrawi R, Chao P, et al. The GeneMANIA prediction server: biological network integration for gene prioritization and predicting gene function. Nucleic Acids Res (2010) 38(Web Server issue):W214–2. doi: 10.1093/nar/gkq537
91. Zhou G, Soufan O, Ewald J, Hancock REW, Basu N, Xia J. NetworkAnalyst 3.0: a visual analytics platform for comprehensive gene expression profiling and meta-analysis. Nucleic Acids Res (2019) 47(W1):W234–41. doi: 10.1093/nar/gkz240
92. Gfeller D, Grosdidier A, Wirth M, Daina A, Michielin O, Zoete V. SwissTargetPrediction: a web server for target prediction of bioactive small molecules. Nucleic Acids Res (2014) 42(Web Server issue):W32–8. doi: 10.1093/nar/gku293
93. Davis AP, Grondin CJ, Johnson RJ, Sciaky D, Wiegers J, Wiegers TC, et al. Comparative toxicogenomics database (CTD): update 2021. Nucleic Acids Res (2021) 49(D1):D1138–43. doi: 10.1093/nar/gkaa891
94. Wishart DS, Feunang YD, Guo AC, Lo EJ, Marcu A, Grant JR, et al. DrugBank 5.0: a major update to the DrugBank database for 2018. Nucleic Acids Res (2018) 46(D1):D1074–82. doi: 10.1093/nar/gkx1037
95. Robin X, Turck N, Hainard A, Tiberti N, Lisacek F, Sanchez JC, et al. pROC: an open-source package for r and s+ to analyze and compare ROC curves. BMC Bioinf (2011) 12:77. doi: 10.1186/1471-2105-12-77
96. Rydenfelt M, Klinger B, Klünemann M, Blüthgen N. SPEED2: inferring upstream pathway activity from differential gene expression. Nucleic Acids Res (2020) 48(W1):W307–12. doi: 10.1093/nar/gkaa236
97. Chen B, Khodadoust MS, Liu CL, Newman AM, Alizadeh AA. Profiling tumor infiltrating immune cells with CIBERSORT. Methods Mol Biol (2018) 1711:243–59. doi: 10.1007/978-1-4939-7493-1_12
98. Kim S, Chen J, Cheng T, Gindulyte A, He J, He S, et al. PubChem in 2021: new data content and improved web interfaces. Nucleic Acids Res (2021) 49(D1):D1388–95. doi: 10.1093/nar/gkaa971
99. UniProt Consortium. UniProt: the universal protein knowledgebase in 2021. Nucleic Acids Res (2021) 49(D1):D480–9. doi: 10.1093/nar/gkaa1100
100. Berman HM, Westbrook J, Feng Z, Gilliland G, Bhat TN, Weissig H, et al. The protein data bank. Nucleic Acids Res (2000) 28(1):235–42. doi: 10.1093/nar/28.1.235
101. Wang J, Wolf RM, Caldwell JW, Kollman PA, Case DA. Development and testing of a general amber force field. J Comput Chem (2004) 25(9):1157–74. doi: 10.1002/jcc.20035
102. Maier JA, Martinez C, Kasavajhala K, Wickstrom L, Hauser KE, Simmerling C. ff14SB: Improving the accuracy of protein side chain and backbone parameters from ff99SB. J Chem Theory Comput (2015) 11(8):3696–713. doi: 10.1021/acs.jctc.5b00255
103. Genheden S, Ryde U. The MM/PBSA and MM/GBSA methods to estimate ligand-binding affinities. Expert Opin Drug Discovery (2015) 10(5):449–61. doi: 10.1517/17460441.2015.1032936
104. Rastelli G, Del Rio A, Degliesposti G, Sgobba M. Fast and accurate predictions of binding free energies using MM-PBSA and MM-GBSA. J Comput Chem (2010) 31(4):797–810. doi: 10.1002/jcc.21372
105. Sellge G, Kufer TA. PRR-signaling pathways: Learning from microbial tactics. Semin Immunol (2015) 27(2):75–84. doi: 10.1016/j.smim.2015.03.009
106. Carty M, Guy C, Bowie AG. Detection of viral infections by innate immunity. Biochem Pharmacol (2021) 183:114316. doi: 10.1016/j.bcp.2020.114316
107. Iwasaki A, Medzhitov R. Control of adaptive immunity by the innate immune system. Nat Immunol (2015) 16(4):343–53. doi: 10.1038/ni.3123
108. Takeuchi O, Akira S. Pattern recognition receptors and inflammation. Cell (2010) 140(6):805–20. doi: 10.1016/j.cell.2010.01.022
109. Broz P, Monack DM. Newly described pattern recognition receptors team up against intracellular pathogens. Nat Rev Immunol (2013) 13(8):551–65. doi: 10.1038/nri3479
110. Kumagai Y, Akira S. Identification and functions of pattern-recognition receptors. J Allergy Clin Immunol (2010) 125(5):985–92. doi: 10.1016/j.jaci.2010.01.058
111. Barbé F, Douglas T, Saleh M. Advances in nod-like receptors (NLR) biology. Cytokine Growth Factor Rev (2014) 25(6):681–97. doi: 10.1016/j.cytogfr.2014.07.001
112. Motta V, Soares F, Sun T, Philpott DJ. NOD-like receptors: versatile cytosolic sentinels. Physiol Rev (2015) 95(1):149–78. doi: 10.1152/physrev.00009.2014
113. Neerincx A, Castro W, Guarda G, Kufer TA. NLRC5, at the heart of antigen presentation. Front Immunol (2013) 4:397. doi: 10.3389/fimmu.2013.00397
114. Wang L, Hauenstein AV. The NLRP3 inflammasome: Mechanism of action, role in disease and therapies. Mol Aspects Med (2020) 76:100889. doi: 10.1016/j.mam.2020.100889
115. Chen L, Cao SQ, Lin ZM, He SJ, Zuo JP. NOD-like receptors in autoimmune diseases. Acta Pharmacol Sin (2021) 42(11):1742–56. doi: 10.1038/s41401-020-00603-2
116. Zhang Y, Liang C. Innate recognition of microbial-derived signals in immunity and inflammation. Sci China Life Sci (2016) 59(12):1210–7. doi: 10.1007/s11427-016-0325-6
117. Lamkanfi M, Declercq W, Kalai M, Saelens X, Vandenabeele P. Alice In caspase land. a phylogenetic analysis of caspases from worm to man. Cell Death Differ (2002) 9(4):358–61. doi: 10.1038/sj.cdd.4400989
118. Lamkanfi M, Festjens N, Declercq W, Vanden Berghe T, Vandenabeele P. Caspases in cell survival, proliferation and differentiation. Cell Death Differ (2007) 14(1):44–55. doi: 10.1038/sj.cdd.4402047
119. Kesavardhana S, Malireddi RKS, Kanneganti TD. Caspases in cell death, inflammation, and pyroptosis. Annu Rev Immunol (2020) 38:567–95. doi: 10.1146/annurev-immunol-073119-095439
120. Martinon F, Tschopp J. Inflammatory caspases and inflammasomes: master switches of inflammation. Cell Death Differ (2007) 14(1):10–22. doi: 10.1038/sj.cdd.4402038
121. Sagulenko V, Vitak N, Vajjhala PR, Vince JE, Stacey KJ. Caspase-1 is an apical caspase leading to caspase-3 cleavage in the AIM2 inflammasome response, independent of caspase-8. J Mol Biol (2018) 430(2):238–47. doi: 10.1016/j.jmb.2017.10.028
122. Martinon F, Tschopp J. Inflammatory caspases: linking an intracellular innate immune system to autoinflammatory diseases. Cell (2004) 117(5):561–74. doi: 10.1016/j.cell.2004.05.004
123. Shi J, Gao W, Shao F. Pyroptosis: Gasdermin-mediated programmed necrotic cell death. Trends Biochem Sci (2017) 42(4):245–54. doi: 10.1016/j.tibs.2016.10.004
124. Yazdi AS, Guarda G, D’Ombrain MC, Drexler SK. Inflammatory caspases in innate immunity and inflammation. J Innate Immun (2010) 2(3):228–37. doi: 10.1159/000283688
125. Bryant C, Fitzgerald KA. Molecular mechanisms involved in inflammasome activation. Trends Cell Biol (2009) 19(9):455–64. doi: 10.1016/j.tcb.2009.06.002
126. Schroder K, Tschopp J. The inflammasomes. Cell (2010) 140(6):821–32. doi: 10.1016/j.cell.2010.01.040
127. Kelley N, Jeltema D, Duan Y, He Y. The NLRP3 inflammasome: An overview of mechanisms of activation and regulation. Int J Mol Sci (2019) 20(13):3328. doi: 10.3390/ijms20133328
128. Swanson KV, Deng M, Ting JP. The NLRP3 inflammasome: molecular activation and regulation to therapeutics. Nat Rev Immunol (2019) 19(8):477–89. doi: 10.1038/s41577-019-0165-0
129. Weber ANR, Bittner ZA, Shankar S, Liu X, Chang TH, Jin T, et al. Recent insights into the regulatory networks of NLRP3 inflammasome activation. J Cell Sci (2020) 133(23):jcs248344. doi: 10.1242/jcs.248344
130. Broderick L, De Nardo D, Franklin BS, Hoffman HM, Latz E. The inflammasomes and autoinflammatory syndromes. Annu Rev Pathol (2015) 10:395–424. doi: 10.1146/annurev-pathol-012414-040431
131. Nambayan RJT, Sandin SI, Quint DA, Satyadi DM, de Alba E. The inflammasome adapter ASC assembles into filaments with integral participation of its two death domains, PYD and CARD. J Biol Chem (2019) 294(2):439–52. doi: 10.1074/jbc.RA118.004407
132. Guey B, Bodnar M, Manié SN, Tardivel A, Petrilli V. Caspase-1 autoproteolysis is differentially required for NLRP1b and NLRP3 inflammasome function. Proc Natl Acad Sci U S A. (2014) 111(48):17254–9. doi: 10.1073/pnas.1415756111
133. Ferrao R, Wu H. Helical assembly in the death domain (DD) superfamily. Curr Opin Struct Biol (2012) 22(2):241–7. doi: 10.1016/j.sbi.2012.02.006
134. Chen MY, Ye XJ, He XH, Ouyang DY. The signaling pathways regulating NLRP3 inflammasome activation. Inflammation (2021) 44(4):1229–45. doi: 10.1007/s10753-021-01439-6
135. Bauernfeind FG, Horvath G, Stutz A, Alnemri ES, MacDonald K, Speert D, et al. Cutting edge: NF-kappaB activating pattern recognition and cytokine receptors license NLRP3 inflammasome activation by regulating NLRP3 expression. J Immunol (2009) 183(2):787–91. doi: 10.4049/jimmunol.0901363
136. Yang J, Liu Z, Xiao TS. Post-translational regulation of inflammasomes. Cell Mol Immunol (2017) 14(1):65–79. doi: 10.1038/cmi.2016.29
137. Vajjhala PR, Mirams RE, Hill JM. Multiple binding sites on the pyrin domain of ASC protein allow self-association and interaction with NLRP3 protein. J Biol Chem (2012) 287(50):41732–43. doi: 10.1074/jbc.M112.381228
138. Franklin BS, Bossaller L, De Nardo D, Ratter JM, Stutz A, Engels G, et al. The adaptor ASC has extracellular and ‘prionoid’ activities that propagate inflammation. Nat Immunol (2014) 15(8):727–37. doi: 10.1038/ni.2913
139. Lu A, Magupalli VG, Ruan J, Yin Q, Atianand MK, Vos MR, et al. Unified polymerization mechanism for the assembly of ASC-dependent inflammasomes. Cell (2014) 156(6):1193–206. doi: 10.1016/j.cell.2014.02.008
140. Lu A, Wu H. Structural mechanisms of inflammasome assembly. FEBS J (2015) 282(3):435–44. doi: 10.1111/febs.13133
141. Malik A, Kanneganti TD. Inflammasome activation and assembly at a glance. J Cell Sci (2017) 130(23):3955–63. doi: 10.1242/jcs.207365
142. Davis BK, Wen H, Ting JP. The inflammasome NLRs in immunity, inflammation, and associated diseases. Annu Rev Immunol (2011) 29:707–35. doi: 10.1146/annurev-immunol-031210-101405
143. Boucher D, Monteleone M, Coll RC, Chen KW, Ross CM, Teo JL, et al. Caspase-1 self-cleavage is an intrinsic mechanism to terminate inflammasome activity. J Exp Med (2018) 215(3):827–40. doi: 10.1084/jem.20172222
144. He Y, Hara H, Núñez G. Mechanism and regulation of NLRP3 inflammasome activation. Trends Biochem Sci (2016) 41(12):1012–21. doi: 10.1016/j.tibs.2016.09.002
145. Yang CA, Chiang BL. Inflammasomes and human autoimmunity: A comprehensive review. J Autoimmun (2015) 61:1–8. doi: 10.1016/j.jaut.2015.05.001
146. Rathinam VA, Vanaja SK, Fitzgerald KA. Regulation of inflammasome signaling. Nat Immunol (2012) 13(4):333–42. doi: 10.1038/ni.2237
147. Segovia JA, Tsai SY, Chang TH, Shil NK, Weintraub ST, Short JD, et al. Nedd8 regulates inflammasome-dependent caspase-1 activation. Mol Cell Biol (2015) 35(3):582–97. doi: 10.1128/MCB.00775-14
148. Wang K, Sun Q, Zhong X, Zeng M, Zeng H, Shi X, et al. Structural mechanism for GSDMD targeting by autoprocessed caspases in pyroptosis. Cell (2020) 180(5):941–955.e20. doi: 10.1016/j.cell.2020.02.002
149. Ding J, Wang K, Liu W, She Y, Sun Q, Shi J, et al. Pore-forming activity and structural autoinhibition of the gasdermin family. Nature (2016) 535(7610):111–6. doi: 10.1038/nature18590
150. Sborgi L, Rühl S, Mulvihill E, Pipercevic J, Heilig R, Stahlberg H, et al. GSDMD membrane pore formation constitutes the mechanism of pyroptotic cell death. EMBO J (2016) 35(16):1766–78. doi: 10.15252/embj.201694696
151. Frank D, Vince JE. Pyroptosis versus necroptosis: similarities, differences, and crosstalk. Cell Death Differ (2019) 26(1):99–114. doi: 10.1038/s41418-018-0212-6
152. Kuriakose T, Man SM, Malireddi RK, Karki R, Kesavardhana S, Place DE, et al. ZBP1/DAI is an innate sensor of influenza virus triggering the NLRP3 inflammasome and programmed cell death pathways. Sci Immunol (2016) 1(2):aag2045. doi: 10.1126/sciimmunol.aag2045
153. Man SM, Karki R, Kanneganti TD. Molecular mechanisms and functions of pyroptosis, inflammatory caspases and inflammasomes in infectious diseases. Immunol Rev (2017) 277(1):61–75. doi: 10.1111/imr.12534
154. Vande Walle L, Lamkanfi M. Pyroptosis. Curr Biol (2016) 26(13):R568–72. doi: 10.1016/j.cub.2016.02.019
155. Li Z, Guo J, Bi L. Role of the NLRP3 inflammasome in autoimmune diseases. BioMed Pharmacother. (2020) 130:110542. doi: 10.1016/j.biopha.2020.110542
156. Shin JI, Lee KH, Joo YH, Lee JM, Jeon J, Jung HJ, et al. Inflammasomes and autoimmune and rheumatic diseases: A comprehensive review. J Autoimmun (2019) 103:102299. doi: 10.1016/j.jaut.2019.06.010
157. Dolinay T, Kim YS, Howrylak J, Hunninghake GM, An CH, Fredenburgh L, et al. Inflammasome-regulated cytokines are critical mediators of acute lung injury. Am J Respir Crit Care Med (2012) 185(11):1225–34. doi: 10.1164/rccm.201201-0003OC
158. Grailer JJ, Canning BA, Kalbitz M, Haggadone MD, Dhond RM, Andjelkovic AV, et al. Critical role for the NLRP3 inflammasome during acute lung injury. J Immunol (2014) 192(12):5974–83. doi: 10.4049/jimmunol.1400368
159. Hoshino T, Okamoto M, Sakazaki Y, Kato S, Young HA, Aizawa H. Role of proinflammatory cytokines IL-18 and IL-1beta in bleomycin-induced lung injury in humans and mice. Am J Respir Cell Mol Biol (2009) 41(6):661–70. doi: 10.1165/rcmb.2008-0182OC
160. Jones HD, Crother TR, Gonzalez-Villalobos RA, Jupelli M, Chen S, Dagvadorj J, et al. The NLRP3 inflammasome is required for the development of hypoxemia in LPS/mechanical ventilation acute lung injury. Am J Respir Cell Mol Biol (2014) 50(2):270–80. doi: 10.1165/rcmb.2013-0087OC
161. Plassmeyer M, Alpan O, Corley MJ, Premeaux TA, Lillard K, Coatney P, et al. Caspases and therapeutic potential of caspase inhibitors in moderate-severe SARS-CoV-2 infection and long COVID. Allergy (2022) 77(1):118–29. doi: 10.1111/all.14907
162. Kroemer A, Khan K, Plassmeyer M, Alpan O, Haseeb MA, Gupta R, et al. Inflammasome activation and pyroptosis in lymphopenic liver patients with COVID-19. J Hepatol (2020) 73(5):1258–62. doi: 10.1016/j.jhep.2020.06.034
163. Conti P, Caraffa A, Gallenga CE, Ross R, Kritas SK, Frydas I, et al. Coronavirus-19 (SARS-CoV-2) induces acute severe lung inflammation. via IL-1 causing Cytokine storm COVID-19: promising inhibitory strategy. J Biol Regul Homeost Agents. (2020) 34(6):1971–5. doi: 10.23812/20-1-E
164. Toldo S, Bussani R, Nuzzi V, Bonaventura A, Mauro AG, Cannatà A, et al. Inflammasome formation in the lungs of patients with fatal COVID-19. Inflammation Res (2021) 70(1):7–10. doi: 10.1007/s00011-020-01413-2
165. Costela-Ruiz VJ, Illescas-Montes R, Puerta-Puerta JM, Ruiz C, Melguizo-Rodríguez L. SARS-CoV-2 infection: The role of cytokines in COVID-19 disease. Cytokine Growth Factor Rev (2020) 54:62–75. doi: 10.1016/j.cytogfr.2020.06.001
166. Zhang J, Wu H, Yao X, Zhang D, Zhou Y, Fu B, et al. Pyroptotic macrophages stimulate the SARS-CoV-2-associated cytokine storm. Cell Mol Immunol (2021) 18(5):1305–7. doi: 10.1038/s41423-021-00665-0
167. Aid M, Busman-Sahay K, Vidal SJ, Maliga Z, Bondoc S, Starke C, et al. Vascular disease and thrombosis in SARS-CoV-2-Infected rhesus macaques. Cell (2020) 183(5):1354–1366.e13. doi: 10.1016/j.cell.2020.10.005
168. Naqvi I, Giroux N, Olson L, Morrison SA, Llanga T, Akinade TO, et al. DAMPs/PAMPs induce monocytic TLR activation and tolerance in COVID-19 patients; nucleic acid binding scavengers can counteract such TLR agonists. Biomaterials (2022) 283:121393. doi: 10.1016/j.biomaterials.2022.121393
169. Pan P, Shen M, Yu Z, Ge W, Chen K, Tian M, et al. SARS-CoV-2 n protein promotes NLRP3 inflammasome activation to induce hyperinflammation. Nat Commun (2021) 12(1):4664. doi: 10.1038/s41467-021-25015-6
170. Theobald SJ, Simonis A, Georgomanolis T, Kreer C, Zehner M, Eisfeld HS, et al. Long-lived macrophage reprogramming drives spike protein-mediated inflammasome activation in COVID-19. EMBO Mol Med (2021) 13(8):e14150. doi: 10.15252/emmm.202114150
171. Zhang J, Ejikemeuwa A, Gerzanich V, Nasr M, Tang Q, Simard JM, et al. Understanding the role of SARS-CoV-2 ORF3a in viral pathogenesis and COVID-19. Front Microbiol (2022) 13:854567. doi: 10.3389/fmicb.2022.854567
172. Vardhana SA, Wolchok JD. The many faces of the anti-COVID immune response. J Exp Med (2020) 217(6):e20200678. doi: 10.1084/jem.20200678
173. Hu B, Huang S, Yin L. The cytokine storm and COVID-19. J Med Virol (2021) 93(1):250–6. doi: 10.1002/jmv.26232
174. Ye Q, Wang B, Mao J. The pathogenesis and treatment of the `Cytokine storm’ in COVID-19. J Infect (2020) 80(6):607–13. doi: 10.1016/j.jinf.2020.03.037
175. Ferreira AC, Soares VC, de Azevedo-Quintanilha IG, Dias SDSG, Fintelman-Rodrigues N, Sacramento CQ, et al. SARS-CoV-2 engages inflammasome and pyroptosis in human primary monocytes. Cell Death Discovery (2021) 7(1):43. doi: 10.1038/s41420-021-00428-w
176. Teijaro JR, Walsh KB, Rice S, Rosen H, Oldstone MB. Mapping the innate signaling cascade essential for cytokine storm during influenza virus infection. Proc Natl Acad Sci U S A. (2014) 111(10):3799–804. doi: 10.1073/pnas.1400593111
177. Li X, Geng M, Peng Y, Meng L, Lu S. Molecular immune pathogenesis and diagnosis of COVID-19. J Pharm Anal (2020) 10(2):102–8. doi: 10.1016/j.jpha.2020.03.001
178. Pedersen SF, Ho YC. SARS-CoV-2: a storm is raging. J Clin Invest. (2020) 130(5):2202–5. doi: 10.1172/JCI137647
179. Mehta P, McAuley DF, Brown M, Sanchez E, Tattersall RS, Manson JJ. HLH across speciality collaboration, UK. COVID-19: consider Cytokine storm syndromes immunosuppression. Lancet (2020) 395(10229):1033–4. doi: 10.1016/S0140-6736(20)30628-0
180. Zhou Y, Fu B, Zheng X, Wang D, Zhao C, Qi Y, et al. Pathogenic T-cells and inflammatory monocytes incite inflammatory storms in severe COVID-19 patients. Natl Sci Rev (2020) 7(6):998–1002. doi: 10.1093/nsr/nwaa041
181. Cheng L, Liang X, Qian L, Luo C, Li D. NLRP3 gene polymorphisms and expression in rheumatoid arthritis. Exp Ther Med (2021) 22(4):1110. doi: 10.3892/etm.2021.10544
182. Jäger E, Murthy S, Schmidt C, Hahn M, Strobel S, Peters A, et al. Calcium-sensing receptor-mediated NLRP3 inflammasome response to calciprotein particles drives inflammation in rheumatoid arthritis. Nat Commun (2020) 11(1):4243. doi: 10.1038/s41467-020-17749-6
183. Mathews RJ, Robinson JI, Battellino M, Wong C, Taylor JC, Biologics in Rheumatoid Arthritis Genetics and Genomics Study Syndicate (BRAGGSS), et al. Evidence of NLRP3-inflammasome activation in rheumatoid arthritis (RA); genetic variants within the NLRP3-inflammasome complex in relation to susceptibility to RA and response to anti-TNF treatment. Ann Rheum Dis (2014) 73(6):1202–10. doi: 10.1136/annrheumdis-2013-203276
184. Kondo N, Kuroda T, Kobayashi D. Cytokine networks in the pathogenesis of rheumatoid arthritis. Int J Mol Sci (2021) 22(20):10922. doi: 10.3390/ijms222010922
185. Burska A, Boissinot M, Ponchel F. Cytokines as biomarkers in rheumatoid arthritis. Mediators Inflamm (2014) 2014:545493. doi: 10.1155/2014/545493
186. Dai SM, Shan ZZ, Xu H, Nishioka K. Cellular targets of interleukin-18 in rheumatoid arthritis. Ann Rheum Dis (2007) 66(11):1411–8. doi: 10.1136/ard.2006.067793
187. Vervoordeldonk MJ, Tak PP. Cytokines in rheumatoid arthritis. J Curr Rheumatol Rep (2002) 4(3):208–17. doi: 10.1007/s11926-002-0067-0
188. Song P, Yang C, Thomsen JS, Dagnæs-Hansen F, Jakobsen M, Brüel A, et al. Lipidoid-siRNA nanoparticle-mediated IL-1β gene silencing for systemic arthritis therapy in a mouse model. Mol Ther (2019) 27(8):1424–35. doi: 10.1016/j.ymthe.2019.05.002
189. Deets KA, Vance RE. Inflammasomes and adaptive immune responses. Nat Immunol (2021) 22(4):412–22. doi: 10.1038/s41590-021-00869-6
190. You R, He X, Zeng Z, Zhan Y, Xiao Y, Xiao R. Pyroptosis and its role in autoimmune disease: A potential therapeutic target. Front Immunol (2022) 13:841732. doi: 10.3389/fimmu.2022.841732
191. McInnes IB, Schett G. Cytokines in the pathogenesis of rheumatoid arthritis. Nat Rev Immunol (2007) 7(6):429–42. doi: 10.1038/nri2094
192. Choulaki C, Papadaki G, Repa A, Kampouraki E, Kambas K, Ritis K, et al. Enhanced activity of NLRP3 inflammasome in peripheral blood cells of patients with active rheumatoid arthritis. Arthritis Res Ther (2015) 17(1):257. doi: 10.1186/s13075-015-0775-2
193. Ruscitti P, Cipriani P, Di Benedetto P, Liakouli V, Berardicurti O, Carubbi F, et al. Monocytes from patients with rheumatoid arthritis and type 2 diabetes mellitus display an increased production of interleukin (IL)-1β via the nucleotide-binding domain and leucine-rich repeat containing family pyrin 3(NLRP3)-inflammasome activation: a possible implication for therapeutic decision in these patients. Clin Exp Immunol (2015) 182(1):35–44. doi: 10.1111/cei.12667
194. Elemam NM, Maghazachi AA, Hannawi S. COVID-19 infection and rheumatoid arthritis: mutual outburst cytokines and remedies. Curr Med Res Opin (2021) 37(6):929–38. doi: 10.1080/03007995.2021.1906637
195. Raiker R, DeYoung C, Pakhchanian H, Ahmed S, Kavadichanda C, Gupta L, et al. Outcomes of COVID-19 in patients with rheumatoid arthritis: A multicenter research network study in the united states. Semin Arthritis Rheumatol (2021) 51(5):1057–66. doi: 10.1016/j.semarthrit.2021.08.010
196. England BR, Roul P, Yang Y, Kalil AC, Michaud K, Thiele GM, et al. Risk of COVID-19 in rheumatoid arthritis: A national veterans affairs matched cohort study in At-risk individuals. Arthritis Rheumatol (2021) 73(12):2179–88. doi: 10.1002/art.41800
197. Favalli EG, Ingegnoli F, De Lucia O, Cincinelli G, Cimaz R, Caporali R. COVID-19 infection and rheumatoid arthritis: Faraway, so close! Autoimmun Rev (2020) 19(5):102523. doi: 10.1016/j.autrev.2020.102523
198. Mihara M, Hashizume M, Yoshida H, Suzuki M, Shiina M. IL-6/IL-6 receptor system and its role in physiological and pathological conditions. Clin Sci (Lond). (2012) 122(4):143–59. doi: 10.1042/CS20110340
199. Huang YP, Chen DR, Lin WJ, Lin YH, Chen JY, Kuo YH, et al. Ergosta-7,9(11),22-trien-3β-ol attenuates inflammatory responses via inhibiting MAPK/AP-1 induced IL-6/JAK/STAT pathways and activating Nrf2/HO-1 signaling in LPS-stimulated macrophage-like cells. Antioxidants (Basel). (2021) 10(9):1430. doi: 10.3390/antiox10091430
200. Chen G, Wu D, Guo W, Cao Y, Huang D, Wang H, et al. Clinical and immunological features of severe and moderate coronavirus disease 2019. J Clin Invest. (2020) 130(5):2620–9. doi: 10.1172/JCI137244
201. Malemud CJ. The role of the JAK/STAT signal pathway in rheumatoid arthritis. Ther Adv Musculoskelet Dis (2018) 10(5-6):117–27. doi: 10.1177/1759720X18776224
202. Simon LS, Taylor PC, Choy EH, Sebba A, Quebe A, Knopp KL, et al. The Jak/STAT pathway: A focus on pain in rheumatoid arthritis. Semin Arthritis Rheumatol (2021) 51(1):278–84. doi: 10.1016/j.semarthrit.2020.10.008
203. Luo W, Li YX, Jiang LJ, Chen Q, Wang T, Ye DW. Targeting JAK-STAT signaling to control cytokine release syndrome in COVID-19. Trends Pharmacol Sci (2020) 41(8):531–43. doi: 10.1016/j.tips.2020.06.007
204. Smolen JS, Landewé R, Bijlsma J, Burmester G, Chatzidionysiou K, Dougados M, et al. EULAR recommendations for the management of rheumatoid arthritis with synthetic and biological disease-modifying antirheumatic drugs: 2016 update. Ann Rheum Dis (2017) 76(6):960–77. doi: 10.1136/annrheumdis-2016-210715
205. You H, Xu D, Zhao J, Li J, Wang Q, Tian X, et al. JAK inhibitors: Prospects in connective tissue diseases. Clin Rev Allergy Immunol (2020) 59(3):334–51. doi: 10.1007/s12016-020-08786-6
206. Winthrop KL. The emerging safety profile of JAK inhibitors in rheumatic disease. Nat Rev Rheumatol (2017) 13(5):320. doi: 10.1038/nrrheum.2017.51
207. Curtis JR, Xie F, Yang S, Bernatsky S, Chen L, Yun H, et al. Risk for herpes zoster in tofacitinib-treated rheumatoid arthritis patients with and without concomitant methotrexate and glucocorticoids. Arthritis Care Res (Hoboken). (2019) 71(9):1249–54. doi: 10.1002/acr.23769
208. Schneider WM, Chevillotte MD, Rice CM. Interferon-stimulated genes: a complex web of host defenses. Annu Rev Immunol (2014) 32:513–45. doi: 10.1146/annurev-immunol-032713-120231
209. Agarwal A, Rochwerg B, Lamontagne F, Siemieniuk RA, Agoritsas T, Askie L, et al. A living WHO guideline on drugs for covid-19. BMJ (2020) 370:m3379. doi: 10.1136/bmj.m3379
210. Reuken PA, Teich N, Stallmach A. Safety of tofacitinib in the COVID-19 pandemic-enough is not enough. Inflammation Bowel Dis (2021) 27(8):e89. doi: 10.1093/ibd/izab051
211. Levy G, Guglielmelli P, Langmuir P, Constantinescu SN. JAK inhibitors and COVID-19. J Immunother Cancer. (2022) 10(4):e002838. doi: 10.1136/jitc-2021-002838
212. Kramer A, Prinz C, Fichtner F, Fischer AL, Thieme V, Grundeis F, et al. Janus kinase inhibitors for the treatment of COVID-19. Cochrane Database Syst Rev (2022) 6(6):CD015209. doi: 10.1002/14651858.CD015209
213. Yang X, Zhan N, Jin Y, Ling H, Xiao C, Xie Z, et al. Tofacitinib restores the balance of γδTreg/γδT17 cells in rheumatoid arthritis by inhibiting the NLRP3 inflammasome. Theranostics (2021) 11(3):1446–57. doi: 10.7150/thno.47860
214. Furuya MY, Asano T, Sumichika Y, Sato S, Kobayashi H, Watanabe H, et al. Tofacitinib inhibits granulocyte-macrophage colony-stimulating factor-induced NLRP3 inflammasome activation in human neutrophils. Arthritis Res Ther (2018) 20(1):196. doi: 10.1186/s13075-018-1685-x
215. Chin YE, Kitagawa M, Kuida K, Flavell RA, Fu XY. Activation of the STAT signaling pathway can cause expression of caspase 1 and apoptosis. Mol Cell Biol (1997) 17(9):5328–37. doi: 10.1128/MCB.17.9.5328
216. Voutsadakis IA. Interferon-alpha and the pathogenesis of myeloproliferative disorders. Med Oncol (2000) 17(4):249–57. doi: 10.1007/BF02782189
217. Jiang S. Don’t rush to deploy COVID-19 vaccines and drugs without sufficient safety guarantees. Nature (2020) 579(7799):321. doi: 10.1038/d41586-020-00751-9
218. Singh H, Kakkar AK, Chauhan P. Repurposing minocycline for COVID-19 management: mechanisms, opportunities, and challenges. Expert Rev Anti Infect Ther (2020) 18(10):997–1003. doi: 10.1080/14787210.2020.1782190
219. FDA. Minocycline label . Available at: https://www.accessdata.fda.gov/drugsatfda_docs/label/2010/050649023lbl.pdf.
220. Bishburg E, Bishburg K. Minocycline–an old drug for a new century: emphasis on methicillin-resistant staphylococcus aureus (MRSA) and acinetobacter baumannii. Int J Antimicrob Agents. (2009) 34(5):395–401. doi: 10.1016/j.ijantimicag.2009.06.021
221. Quick ED, Seitz S, Clarke P, Tyler KL. Minocycline has anti-inflammatory effects and reduces cytotoxicity in an. Ex Vivo Spinal Cord Slice Culture Model West Nile Virus Infection. J Virol (2017) 91(22):e00569-17. doi: 10.1128/JVI.00569-17
222. Leela SL, Srisawat C, Sreekanth GP, Noisakran S, Yenchitsomanus PT, Limjindaporn T. Drug repurposing of minocycline against dengue virus infection. Biochem Biophys Res Commun (2016) 478(1):410–6. doi: 10.1016/j.bbrc.2016.07.029
223. Singh M, Singh P, Vaira D, Amand M, Rahmouni S, Moutschen M. Minocycline attenuates HIV-1 infection and suppresses chronic immune activation in humanized NOD/LtsZ-scidIL-2Rγ(null) mice. Immunology (2014) 142(4):562–72. doi: 10.1111/imm.12246
224. Yang F, Zhu W, Cai X, Zhang W, Yu Z, Li X, et al. Minocycline alleviates NLRP3 inflammasome-dependent pyroptosis in monosodium glutamate-induced depressive rats. Biochem Biophys Res Commun (2020) 526(3):553–9. doi: 10.1016/j.bbrc.2020.02.149
225. Garrido-Mesa N, Zarzuelo A, Gálvez J. Minocycline: far beyond an antibiotic. Br J Pharmacol (2013) 169(2):337–52. doi: 10.1111/bph.12139
226. Garrido-Mesa N, Zarzuelo A, Gálvez J. What is behind the non-antibiotic properties of minocycline? Pharmacol Res (2013) 67(1):18–30. doi: 10.1016/j.phrs.2012.10.006
227. Sanchez Mejia RO, Ona VO, Li M, Friedlander RM. Minocycline reduces traumatic brain injury-mediated caspase-1 activation, tissue damage, and neurological dysfunction. Neurosurgery (2001) 48(6):1393–9; discussion 1399-401. doi: 10.1097/00006123-200106000-00051
228. Wong ML, Inserra A, Lewis MD, Mastronardi CA, Leong L, Choo J, et al. Inflammasome signaling affects anxiety- and depressive-like behavior and gut microbiome composition. Mol Psychiatry (2016) 21(6):797–805. doi: 10.1038/mp.2016.46
229. Chen M, Ona VO, Li M, Ferrante RJ, Fink KB, Zhu S, et al. Minocycline inhibits caspase-1 and caspase-3 expression and delays mortality in a transgenic mouse model of huntington disease. Nat Med (2000) 6(7):797–801. doi: 10.1038/77528
230. Vincent JA, Mohr S. Inhibition of caspase-1/interleukin-1beta signaling prevents degeneration of retinal capillaries in diabetes and galactosemia. Diabetes (2007) 56(1):224–30. doi: 10.2337/db06-0427
231. Peukert K, Fox M, Schulz S, Feuerborn C, Frede S, Putensen C, et al. Inhibition of caspase-1 with tetracycline ameliorates acute lung injury. Am J Respir Crit Care Med (2021) 204(1):53–63. doi: 10.1164/rccm.202005-1916OC
232. Lodise TP, Fan W, Griffith DC, Dudley MN. Sulham KA. a retrospective cohort analysis shows that coadministration of minocycline with colistin in critically ill patients is associated with reduced frequency of acute renal failure. Antimicrob Agents Chemother (2017) 62(1):e01165-17. doi: 10.1128/AAC.01165-17
233. Gautam SS, Gautam CS, Garg VK, Singh H. Combining hydroxychloroquine and minocycline: potential role in moderate to severe COVID-19 infection. Expert Rev Clin Pharmacol (2020) 13(11):1183–90. doi: 10.1080/17512433.2020.1832889
234. Garcia-Vidal C, Sanjuan G, Moreno-García E, Puerta-Alcalde P, Garcia-Pouton N, Chumbita M, et al. COVID-19 researchers group. incidence of co-infections and superinfections in hospitalized patients with COVID-19: a retrospective cohort study. Clin Microbiol Infect (2021) 27(1):83–8. doi: 10.1016/j.cmi.2020.07.041
235. Hughes S, Troise O, Donaldson H, Mughal N, Moore LSP. Bacterial and fungal coinfection among hospitalized patients with COVID-19: a retrospective cohort study in a UK secondary-care setting. Clin Microbiol Infect (2020) 26(10):1395–9. doi: 10.1016/j.cmi.2020.06.025
236. Itoh K, Sakamaki I, Hirota T, Iwasaki H. Evaluation of minocycline combined with favipiravir therapy in coronavirus disease 2019 patients: A case-series study. J Infect Chemother (2022) 28(1):124–7. doi: 10.1016/j.jiac.2021.09.016
237. Gironi LC, Damiani G, Zavattaro E, Pacifico A, Santus P, Pigatto PDM, et al. Tetracyclines in COVID-19 patients quarantined at home: Literature evidence supporting real-world data from a multicenter observational study targeting inflammatory and infectious dermatoses. Dermatol Ther (2021) 34(1):e14694. doi: 10.1111/dth.14694
238. Kloppenburg M, Mattie H, Douwes N, Dijkmans BA, Breedveld FC. Minocycline in the treatment of rheumatoid arthritis: relationship of serum concentrations to efficacy. J Rheumatol (1995) 22(4):611–6.
239. Greenwald RA. The road forward: the scientific basis for tetracycline treatment of arthritic disorders. Pharmacol Res (2011) 64(6):610–3. doi: 10.1016/j.phrs.2011.06.010
Keywords: SARS-CoV-2, COVID-19, rheumatoid arthritis, pyroptosis, caspase-1, minocycline
Citation: Zheng Q, Lin R, Chen Y, Lv Q, Zhang J, Zhai J, Xu W and Wang W (2022) SARS-CoV-2 induces “cytokine storm” hyperinflammatory responses in RA patients through pyroptosis. Front. Immunol. 13:1058884. doi: 10.3389/fimmu.2022.1058884
Received: 30 September 2022; Accepted: 15 November 2022;
Published: 01 December 2022.
Edited by:
Chenhe Su, Wistar Institute, United StatesReviewed by:
Sharah Jabeen, Bangladesh Agricultural University, BangladeshYuexiu Zhang, The Ohio State University, United States
Weizheng Liang, First Affiliated Hospital of Hebei North University, China
Copyright © 2022 Zheng, Lin, Chen, Lv, Zhang, Zhai, Xu and Wang. This is an open-access article distributed under the terms of the Creative Commons Attribution License (CC BY). The use, distribution or reproduction in other forums is permitted, provided the original author(s) and the copyright owner(s) are credited and that the original publication in this journal is cited, in accordance with accepted academic practice. No use, distribution or reproduction is permitted which does not comply with these terms.
*Correspondence: Weihong Xu, eHV3ZWlob25nODE1QDEyNi5jb20=; Wanming Wang, d2FuZ3dtOTNAMTI2LmNvbQ==
†These authors have contributed equally to this work