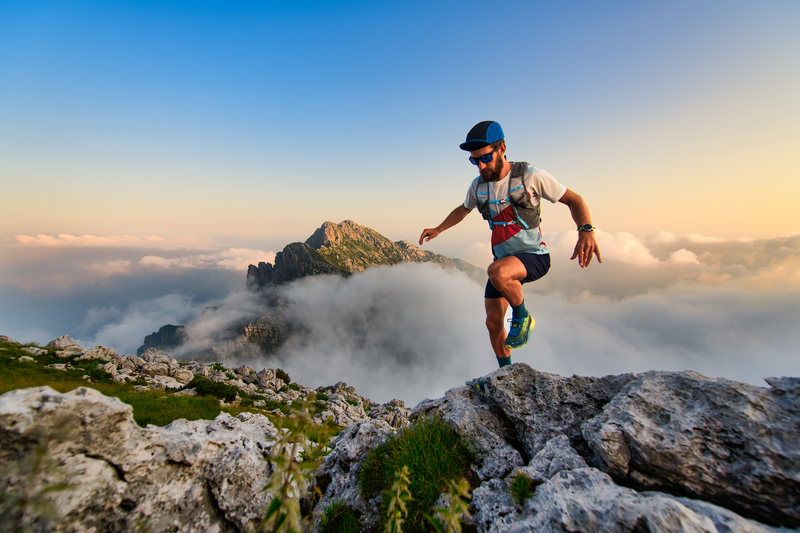
94% of researchers rate our articles as excellent or good
Learn more about the work of our research integrity team to safeguard the quality of each article we publish.
Find out more
REVIEW article
Front. Immunol. , 20 October 2022
Sec. Cancer Immunity and Immunotherapy
Volume 13 - 2022 | https://doi.org/10.3389/fimmu.2022.1049043
This article is part of the Research Topic Ionizing Radiation Reprograms Tumor Immune Microenvironment by Inducing Immunogenic Cell Death View all 11 articles
Cancer immunotherapy, especially immune-checkpoint inhibitors (ICIs), has paved a new way for the treatment of many types of malignancies, particularly advanced-stage cancers. Accumulating evidence suggests that as a molecular imaging modality, positron emission tomography/computed tomography (PET/CT) can play a vital role in the management of ICIs therapy by using different molecular probes and metabolic parameters. In this review, we will provide a comprehensive overview of the clinical data to support the importance of 18F-fluorodeoxyglucose PET/CT (18F-FDG PET/CT) imaging in the treatment of ICIs, including the evaluation of the tumor microenvironment, discovery of immune-related adverse events, evaluation of therapeutic efficacy, and prediction of therapeutic prognosis. We also discuss perspectives on the development direction of 18F-FDG PET/CT imaging, with a particular emphasis on possible challenges in the future. In addition, we summarize the researches on novel PET molecular probes that are expected to potentially promote the precise application of ICIs.
Cancer immunotherapy has paved a new way for the treatment of many types of malignancies, particularly advanced-stage cancers, by intervening in the abnormal immune processes, reshaping the tumor microenvironment (TME), and restoring immune surveillance (1). Immune-checkpoint inhibitors (ICIs), such as the blocking antibodies of programmed cell death protein-1 (PD-1), programmed death-ligand 1 (PD-L1), and cytotoxic T lymphocyte-associated protein 4 (CTLA-4), have brought considerable clinical benefits to cancer patients. However, only a subset of patients can benefit from ICIs therapies, and some might even experience severe immune-related adverse events (irAEs) and detrimental hyperprogressive disease (2). Emerging preclinical and clinical evidence indicates that the reciprocity between ICIs and TME may play a complex and important influence on ICIs therapy, but the specific mechanism is still unclear. How to characterize TME noninvasively and effectively, so as to deeply elucidate its potential mechanisms in immunotherapy and precisely guide the use of ICIs, is continually attracting research interest worldwide.
It is well-known that positron emission tomography (PET)/computed tomography (CT) can reflect the biological information of the living body noninvasively and dynamically by using different kinds of imaging agents. Fluorine-18 fluorodeoxyglucose (18F-FDG), the most commonly used PET/CT imaging agent, has been increasingly applied in the immunotherapeutic management. It can reflect the level of glucose accumulation in both primary tumor tissues and metastatic lesions by tracking glucose uptake through a single scan. The metabolic parameters obtained from 18F-FDG PET/CT arguably provide useful indications of the tumor burden (3). Accumulating evidence suggests that 18F-FDG PET/CT imaging can play a vital role in ICIs therapy, including TME characterization, irAEs assessment, efficacy evaluation, prognosis prediction, and so on.
In this review, we focus on the characteristics of TME associated with immunotherapy, and provide an overview of the clinical data associated with the application of 18F-FDG PET/CT imaging in the treatment of ICIs. Furthermore, we discuss perspectives on the development direction of 18F-FDG PET/CT imaging, with a particular emphasis on possible challenges in the future. We also summarize the researches on novel PET/CT molecular imaging, which may potentially promote the precise application of ICIs.
Compared with traditional treatments, such as radiotherapy and chemotherapy, ICIs treatment is more closely related to TME. The efficacy of ICIs may be influenced by various mechanisms related to the tumor or the host, among which TME is being widely investigated as a critical factor. The characteristics of TME vary in different individuals and cancer types, which will affect the immune response to ICIs treatment.
TME is composed of tumor cells, immune cells, stromal cells, extracellular matrix, and exosomes (4), thus forming a microenvironment with the characteristics of inflammation, hypoxia, acidity, and immunosuppression. Different types of cells in TME have their preferred metabolic phenotypes (Figure 1).
Figure 1 Tumor microenvironment (TME) is composed of tumor cells, immune cells, stromal cells, extracellular matrix, and exosomes, thus forming a microenvironment with the characteristics of inflammation, hypoxia, acidity, and immunosuppression. Different types of cells in TME have their preferred metabolic phenotypes. OXPHOS, oxidative phosphorylation; HBP, hexosamine biosynthesis pathway; PPP, pentose phosphate pathway; MDSC, myeloid-derived suppressor cell; Treg cell, regulatory T cell; M2-TAM, immunosuppressive macrophages; Tmem cell, CD8+ memory T cells; DC, dendritic cell; Teff cell, CD8+ effector T cells; NK cell, natural killer cell; M1-TAM, inflammatory tumor-associated macrophages; CAF, cancer-associated fibroblast; TGF-β, transforming growth factor-β; TNF-α, tumor necrosis factor-α; IL-2, Interleukin-2; IFN-γ, Interferon-γ; ECM, extracellular matrix.
Tumor cells generally experience metabolic reprogramming, especially glucose metabolism, to adapt to immunosuppressive TME. Even under aerobic conditions, tumor cells are typically characterized by glycolysis, resulting in high rates of glucose intake with high lactate excretion, which is known as the Warburg effect (5). There are fundamental differences between the metabolic programs of cancer cells and immune cells, as well as between different immune cells (6).
Immune cells can be divided into immune-activating cells and immunosuppressive cells. The characteristics of TME might inhibit antitumor immune cells and lead to their exhaustion or senescence (7), but tumor-promoting immune cells mostly show tolerance (8). Immune-activating cells include CD8+ effector T (Teff) cells, CD8+ memory T (Tmem) cells, CD4+ T helper 1 (Th 1) cells, dendritic cells (DCs), natural killer (NK) cells, inflammatory tumor-associated macrophages (M1-TAM), B cells, and neutrophils. Immunosuppressive cells include regulatory T (Treg) cells, myeloid-derived suppressor cells (MDSCs), and immunosuppressive macrophages (M2-TAM), which subvert antitumor immunity by secreting cytokines or interfering with metabolism (5).
Stromal cells include cancer-associated fibroblasts (CAFs), endothelial cells, and pericytes. Like immune cells, stromal cells could interact with tumor cells, modulate their metabolic behavior, and contribute to migration, invasion, and evasion of immune surveillance (9). CAFs can carry out aerobic glycolysis and secrete lactate and pyruvate as fuels for neighboring tumor cells. A metabolic cross-talk exists between tumor cells and CAFs, referred to as a reverse-Warburg effect. CAFs are also characterized by increased synthesis and secretion of glutamine, which is consumed by cancer cells, thus allowing them to sustain nucleotide generation and oxidative phosphorylation (OXPHOS) to obtain high proliferation (10).
With the continuous understanding of the dynamic interaction (promote or hinder) between the status of TME and the treatment with ICIs, several TME classifications based on immunotherapeutic rationale have been proposed. These types are associated with therapy response and might be helpful in selecting the appropriate immunotherapy strategy and suitable patients.
Tumor immune microenvironment (TIME) refers to the immunological characteristics of TME, mainly including the cell types, infiltration degrees, and molecular expression levels of immune cells. Based on the degree and location of tumor-infiltrating lymphocytes (TILs) in TIME, tumors can be classified into “cold” or “hot” ones, or more precisely, divided into three types of immune-inflamed, immune-excluded, and immune-desert (11). In addition, PD-L1 expression and TILs infiltration are essential features of TIME related to ICIs (12, 13). Hence, TIME can be divided into four tumor immune microenvironment types (TIMTs) according to these two characteristics, i.e., PD-L1−/TIL−, PD-L1+/TIL+, PD-L1−/TIL+, and PD-L1+/TIL− (14).
Considering that the metabolic and immune characteristics of TME are important theoretical bases for tumor immunotherapy, Siska et al. recommended the metabolic-tumor-stroma score (MeTS) to describe the characteristics of tumor metabolism and cell heterogeneity (15): (1) OXPHOS metabolic type and high T cell infiltration; (2) reverse Warburg type; (3) mixed type; and (4) Warburg type and low T cell infiltration.
Due to the Warburg effect, tumor cells are usually characterized by high glucose metabolism, i.e., increased FDG uptake is often induced in the case of over-expression of glucose transporters (GLUT), such as GLUT1 and GLUT3. A set of studies have shown that GLUT1 expression is correlated with tumor size and hypoxia of TME, and the latter activates hypoxia-inducible factor 1-alpha (HIF-1α) to trigger the Warburg effect and upregulate GLUT expression (16). It has also been elucidated that HIF-1α could directly bind to the hypoxia response element in the PD-L1 proximal promoter and control its expression under hypoxic conditions (17). Besides, activation of some immune cells, including CD4+ and CD8+ T cells, is accompanied by increased metabolism, such as upregulated aerobic glycolysis, tricarboxylic acid (TCA) cycle, and OXPHOS (6). The above mechanisms may provide a theoretical basis for the role of 18F-FDG PET/CT imaging in ICIs therapy, including characterization of TIME, assessment of irAEs, evaluation of therapeutic efficacy, and prediction of prognosis (Figure 2).
Figure 2 The application of 18F-FDG PET/CT imaging in tumor immunotherapy includes characterization of tumor immune microenvironment (TIME), assessment of immune-related adverse events (irAEs), evaluation of therapeutic efficacy, and prediction of prognosis.
Delineation of TIME characteristics can help treatment formulation, efficacy evaluation, and prognosis prediction (18). Due to the complexity of TIME components and their dynamic changes during the treatment process of ICIs, traditional methods such as biopsy have limitations in reflecting TIME. As a non-invasive and functional whole-body imaging modality, 18F-FDG PET/CT imaging has some potential advantages in characterizing the overall glucose metabolism of tumor cells, activated immune cells, and stromal cells in the TME of the primary lesions.
The majority of researches on the application of 18F-FDG PET/CT imaging on TIME characterization focused on non-small-cell lung cancer (NSCLC) (Table 1). Zhao et al. carried out two studies with the largest sample sizes (419 cases and 428 cases) to investigate the relationship between PD-L1 expression and 18F-FDG uptake, using 22C3 and SP142 assays, respectively (19, 20). They both showed that maximum standardized uptake value (SUVmax) was significantly associated with PD-L1 expression in NSCLC. A meta-analysis across seven studies (473 patients) showed that the predictive sensitivity of the SUVmax for the expression of PD-L1 in NSCLC patients was 75%, and the specificity was 73% (41). Other metabolic parameters, such as mean standardized uptake value (SUVmean) and the ratio of metabolic to morphological lesion volumes (MMVR), have been reported to be correlated with PD-1 or PD-L1 expression (26, 27). 18F-FDG PET/CT radiomics have also been used to explore predictive models based on images and clinical information for PD-L1 expression (28–30). Additionally, Mitchell et al. found that high SUVmax was associated with reduced CD57+ cell density and increased T cell exhaustion gene signature (21). Wang et al. revealed that high SUVmax was associated with high infiltration of CD8+ T cells, M2 macrophages, and Foxp3+ Treg cells (22).
In terms of TIMTs, Zhou et al. used dual-phase 18F-FDG PET/CT imaging to reflect metabolic dynamics in NSCLC and constructed a model combined metabolic signature (Meta-Sig) and clinical factors to predict PD-L1+/TIL+ tumors (AUC: 0.869, sensitivity: 77.27%, specificity: 82.61%) (42). Wu et al. analyzed the correlation between SUVmax and TIMT classification in patients with cell clear renal cell carcinoma (ccRCC) and suggested that SUVmax might be used as an indicator for TIMTs and thus help guide the treatment with ICIs (32).
In addition, the correlation between 18F-FDG PET/CT imaging and TIME in breast cancer, gastric cancer, colorectal cancer, bladder cancer, nasopharyngeal cancer, and oral squamous cell carcinoma has also been observed (Table 1).
The perturbation of ICIs on the balance of the immune system can lead to a loss of self-tolerance and excessive immune activation of normal tissues, resulting in irAEs (43). The irAEs can affect nearly all organ systems, such as the neurologic, pulmonary, cardiovascular, gastrointestinal, endocrine, genitourinary, integumentary, skeletal and joint systems, and so on (44, 45). The irAEs resulting from different ICIs may vary. A systematic review found that deaths from CTLA-4 inhibitors were mainly caused by the irAEs of colitis (70%), and deaths from PD-1/PD-L1 inhibitors were mainly pneumonia (35%), hepatitis (22%), and neurotoxicity (15%) (46). For different types of tumors, the most commonly affected sites of irAEs are also different. For example, patients with NSCLC mainly show endocrine system and skin irAEs, and patients with melanoma mostly involve the skin and liver, while irAEs occur in patients with RCC are more common in the skin and gastrointestinal tract (47).
A number of current reports support that some irAEs seem to be associated with improved tumor response and better survival (48). This association may stay robust in certain cancer types (NSCLC, melanoma, RCC, and advanced urothelial cancer) and organ-specific irAEs (the skin and endocrine system) (49–52). But some reports pointed out that grade 3-5 irAEs (severe irAEs) are not associated with increased overall survival (OS) and progression-free survival (PFS) in NSCLC patients (53, 54). Oncologists should weigh the risk of irAEs against the benefit of ICIs before immunotherapy and take appropriate management once irAEs occur.
Therefore, it is necessary to judge the appearances of irAEs timely by noninvasive imaging methods. CT or magnetic resonance imaging (MRI) has been widely used to detect irAEs, especially in the lung, pancreas, liver, and nervous system (55). However, the utility of 18F-FDG PET/CT imaging in irAEs screening and monitoring is largely under-recognized currently (2).
18F-FDG PET/CT imaging may be a sensitive method to identify the development and severity of irAEs, which usually present as a new non-neoplastic lesion with increased FDG accumulation after ICI treatment (56). For instance, elevated thyroid SUVmax commonly suggests ICI-related thyroiditis (57), while a diffuse increase of FDG uptake in the pancreas is a characteristic manifestation of ICI-related pancreatitis (58). A study on patients with metastatic melanoma treated with ICIs found that novel quantitative imaging biomarkers, i.e. the SUV percentiles (SUVX%) of 18F-FDG uptake within the target organs, could be predictive of irAEs in the bowel, stomach, and thyroid (56). This study also demonstrated that some irAEs could be detected on 18F-FDG PET/CT imaging before the onset of clinical symptoms, which showed increased 18F-FDG uptake in the affected organs. The typical 18F-FDG PET/CT manifestations of irAEs can be seen in Figure 3.
Figure 3 Typical images of irAEs in patients with ICI treatment. (A), thyroiditis; (B), hypophysitis; (C), pneumonia; (D), pancreatitis; (E), enteritis. The sites of irAEs were marked with blue arrows on maximum intensity projection (MIP) and PET images.
ICIs treatment can achieve antitumor effects by eliminating immunosuppression and reinvigorating Tmem cells, which are good for the patient’s long-term survival. However, ICIs can also lead to atypical response patterns, including pseudoprogression, hyperprogression, and mixed response.
In order to standardize the imaging evaluation of tumor treatment efficacy, a series of tumor treatment response evaluation criteria have been proposed. The CT-based evaluation criteria, i.e. Response Evaluation Criteria in Solid Tumors version 1.1 (RECIST 1.1) (59), were initially used directly. The typical cases evaluated by RECIST 1.1 were displayed in Figure 4. RECIST 1.1 was later adjusted for better ICI response evaluation. The modified RECIST 1.1 for immune-based therapeutics, i.e. iRECIST, classify the initial discovery of the suspected progression as initially unconfirmed progressive disease (iUPD) (60). Immune-modified RECIST (imRECIST) includes the measurable new lesions in the total tumor burden (61).
Figure 4 Typical cases evaluated by Response Evaluation Criteria in Solid Tumors (RECIST) 1.1 in patients with ICI treatment. (A), Immunotherapy response in a 64-year-old male patient with right lung adenocarcinoma. The baseline image shows intensive FDG uptake in the primary tumor, accompanied with multifocal intrapulmonary metastasis, lymphadenopathy, and the involvement of pleura. Follow-up images after 4 cycles and sequential 11 cycles of the combination of chemotherapy and ICI show partial response (PR). (B), Immunotherapy response in a 60-year-old female patient with right lung adenocarcinoma. Image after 7 cycles of the combination of chemotherapy and ICI shows the enlargement and increased metabolism in the primary tumor, and the onset of multiple new lesions in the lung, pleura, lymph nodes, liver, and bone, indicating progressive disease (PD).
Meanwhile, the 18F-FDG PET (PET/CT)-based evaluation criteria have also been successively proposed (Table 2). The European Organization for Research and Treatment of Cancer 1999 criteria (EORTC) is the first metabolism criterion using SUVmax changes to determine antitumor treatment response (62). In 2009, Wahl et al. proposed the PET Response Criteria in Solid Tumors (PERCIST) (63), which has also been modified further. Immune PERCIST (iPERCIST) introduced the concept of unconfirmed progressive metabolic disease (UPMD) (64). Since immunotherapy may induce new inflammatory lesions that are detectable on 18F-FDG PET/CT, immunotherapy-modified response classification (imPERCIST5) was introduced. According to imPERCIST5, progressive metabolic disease (PMD) was defined only by an increase of the sum of peak standardized uptake values normalized for body lean body mass (SULpeak) by 30% (65). In 2018, Anwar et al. proposed PET Response Evaluation Criteria for Immunotherapy (PERCIMT) to evaluate clinical benefit based on the number and size of new lesions (66). According to PERCIMT, 4 or more new lesions (regardless of size), or 3 or more new lesions (diameter > 1 cm), or 2 or more new lesions (diameter > 1.5 cm), are all defined as no-clinical benefit, while the other cases are considered clinically benefited.
The comparative studies of different response evaluation criteria are shown in Table 3. In short, the continuous adjustment of immunotherapy response evaluation criteria aims to guide immunotherapy management more precisely and effectively.
Since only a certain proportion of patients can benefit from ICIs therapy, how to conduct pretreatment assessments and identify eligible patients has important clinical significance. Up to now, some potentially prognostic biomarkers have been explored, including tumor PD-L1 expression, tumor mutation burden (TMB), microsatellite instability (MSI), gene expression profiles, gastrointestinal microbiome, and so on (73). Nevertheless, the values of these biomarkers remain controversial, and some biomarkers (such as TMB and MSI) require complex, expensive, and time-consuming analyses. Despite imperfection, PD-L1 expression is still the most commonly used biomarker in clinic, especially for NSCLC patients (74).
There have been a variety of studies committed to discovering the prognostic value of 18F-FDG PET/CT imaging on ICI treatment, but the results are inconsistent. The researches mainly focus on patients with NSCLC and melanoma, and the metabolic parameters include SUVmax, SUVmean, metabolic tumor volume (MTV), total MTV (tMTV), total lesion glycolysis (TLG), and so on (Table 4). Although SUVmax is the most commonly used metabolic parameter, its prognostic value may be controversial (81, 87). Some researchers have also advocated that SUVmean may be suggestive (83). Other studies support the prognostic value of MTV and TLG for immunotherapy, indicating that high MTV and TLG are associated with poor prognosis (78, 80). However, the multivariate analysis of a prospective study on nivolumab found no significant correlation between TLG and OS (88). In addition, tMTV provides a good indication of the total cancer burden (3). Seban et al. demonstrated that tMTV > 75 cm3 was associated with shorter OS and the absence of clinical disease benefit. They proposed a metabolic scoring system based on the derived neutrophil-to-lymphocyte ratio (dNLR) and tMTV, which stratified patients into three groups with different prognosis: poor prognosis (dNLR>3 and tMTV>75 cm3), moderate prognosis (dNLR>3 or tMTV>75 cm3) and good prognosis (dNLR ≤ 3 and tMTV ≤ 75 cm3) (77). However, Vekens et al. discovered that tMTV and TLG did not have a predictive effect (87).
The main reasons for the inconsistent results may lie in the heterogeneity of patients included and the difference of treatment schemes in the study cohorts. The discrepancies between adopted end-point events and treatment response evaluation criteria may be other confounding issues. Further researches should be carried out to establish an ideal and universal method from the metabolism perspective for predicting the prognosis of pan-cancer, which would be validated in a large dataset.
In recent years, the rapid development of artificial intelligence has further promoted the application of 18F-FDG PET/CT radiomics, which extracts a large number of quantitative features from PET/CT images through automated and high-throughput methods, in the prognosis evaluation after surgery, chemoradiotherapy, targeted therapy or immunotherapy. Mu et al. (91) constructed a deep learning model based on PET/CT images, namely the EGFR-deep learning score (EGFR-DLS), to provide non-invasive decision support for targeted therapy or immunotherapy for patients with NSCLC. Moreover, Mu et al. (92) established a nomogram including multi-parameter PET/CT radiomic features, Eastern Cooperative Oncology Group (ECOG) score, and distant metastasis to predict the prognosis of patients with stage IIIB-IV NSCLC receiving ICIs therapy.
With unprecedented advances in ICIs in cancer treatment, the value of 18F-FDG PET/CT has been especially emphasized. Nevertheless, several challenges associated with 18F-FDG PET/CT imaging need to be addressed to broaden its application in ICIs treatment. One major shortcoming is the lack of recognized guidelines to instruct the application of 18F-FDG PET/CT imaging in immunotherapy. On the other hand, although glucose metabolism parameters, such as SUVmax, have been shown to be significantly related to the immune characteristics of TME or prognosis, related studies were mostly retrospective, single-center and small-sample size, and there are controversies between the results of different studies. So, further prospective and large-sample cohort studies are still needed. Moreover, current studies mainly focus on NSCLC and melanoma. With the extensive development of ICIs treatment and the accumulation of cases, researches concentrating on other cancers can be investigated. Besides, there remains a challenging area of investigations on biological mechanisms of the association between TME and 18F-FDG PET/CT imaging, and basic and translational studies are encouraged to unravel the unknowns.
In addition to 18F-FDG, researchers are also committed to developing a series of new PET molecular probes targeting the compositions of TME, some of which have entered preclinical or clinical applications (Figure 5). The principles of these designs are mostly based on the specific binding of radiolabeled antibodies, peptides, or small molecules with the corresponding targets. The emergence of new molecular imaging agents is expected to provide more accurate means to obtain dynamic information about TME for promoting individualized treatment.
Figure 5 A series of new PET molecular probes targeting the compositions of tumor microenvironment (TME).
PD-L1 or PD-1 expression is reported to be related with prognosis after immunotherapy, and the detection of the two biomarkers mainly depends on immunohistochemistry (IHC) of biopsy or surgery materials. However, this invasive and snap-shot approach has some limitations, including the inability to reflect the heterogeneity and spatio-temporal dynamic expression of PD-L1 or PD-1 in tumor tissues, different antibody detection platforms and different thresholds leading to different results, and difficulties in obtaining histological specimens for some patients. Targeted molecular imaging can detect PD-L1 or PD-1 expression noninvasively and dynamically in vivo to compensate for the above shortcomings.
PD-1 imaging agents used in clinical trials include 89Zr-pembrolizumab, 64Cu-pembrolizumab, and 89Zr-nivolumab. The first-in-humans study of 89Zr-pembrolizumab in patients with advanced-stage NSCLC confirmed its safety and feasibility for immunotherapy. The findings indicated that patients with higher tumor uptake for 89Zr-pembrolizumab showed a tendency for better response to pembrolizumab (93). A later study showed that 89Zr-pembrolizumab uptake was positively associated with PFS and OS in melanoma and NSCLC patients (94).
PD-L1 targeting imaging agents mainly include 89Zr-atezolizumab, 89Zr-durvalumab, and 18F-BMS-986192, all of which have been in clinical trials (95). Researchers uncovered that 89Zr-atezolizumab uptake performed better than IHC or RNA sequencing-based predictive biomarkers in evaluating clinical responses for ICIs (96). 89Zr-atezolizumab is also reported to be helpful in identifying RCC patients who may benefit from anti-PD-1/PD-L1 therapy (97). Meanwhile, a number of preclinical studies on PD-L1-targeting molecular probes (including antibodies, peptides, and small molecules) using nuclear medicine, MRI, or optical imaging, have been documented (98, 99).
CTLA-4 is another well-known immunosuppressive checkpoint. It is expressed on T cells and binds with CD80/86 ligands on DCs with a high affinity to prevent uncontrolled expansion of activated T cells. Accordingly, the blockade of CTLA-4 with antibodies has been used in clinic as a promising option for cancer patients. CTLA-4 targeting imaging agents include 64Cu-NOTA-ipilimumab-F(ab’)2, 64Cu-NOTA-ipilimumab, and 64Cu-DOTA-ipilimumab (100, 101), and all of them have not yet entered clinical trials.
Apart from PD-1, PD-L1, and CTLA-4, several novel immune checkpoint molecules, both the inhibitory and stimulatory ones, have been discovered over the past decade (43). The former molecules include lymphocyte activation gene-3 (LAG-3), T cell immunoglobulin and mucin-domain containing-3 (TIM-3), T cell immunoglobulin and ITIM domain (TIGIT), sialic acid-binding immunoglobulin-like lectin 15 (Siglec-15), and V-domain Ig suppressor of T cell activation (VISTA), which express on a variety of immune cells and exhibit inhibitory roles in the context of malignancy.
The positive immune regulators, such as glucocorticoid-induced TNFR-related gene (GITR) and tumor necrosis factor receptor superfamily member 4 (OX40), are co-stimulatory molecules expressed on T cells. In addition, inducible T-cell co-stimulator (ICOS) is an indicator of T-cell-mediated immune response, and some animal experiments showed that 89Zr-DFO-ICOS mAb targeting ICOS could monitor immunotherapy response (102, 103). With the discovery of new immune checkpoints, molecular probes targeting these targets are emerging.
CD8+ TILs are an important feature reflecting TIME and significantly impact the tumorigenesis and development of tumors. Responders showed higher numbers of pre-existing CD8+ Teff cells within the tumor and at tumor margins prior to ICIs therapy (104). Therefore, targeted imaging of CD8+ T cells is of great significance for immunotherapy.
89Zr-Df-IAB22M2C, a new molecular probe targeting CD8+ TILs, is used to assess CD8+ TILs in tumors accurately. The first human trial of 89Zr-Df-IAB22M2C has proven its safety and validity in patients with solid malignancies (105). 89Zr-PEGylated VHH-X118 has also been confirmed to have the potential for CD8+ TIL targeting imaging (106). Iravani et al. demonstrated that 18F-FDG and other new imaging agents, such as those targeting CD8+ TILs or T cell function, can be used for PET/CT imaging to guide ICI treatment in the future (107). However, due to the complexity of lymphocyte subsets, CD8+ TILs imaging agents can only reflect part of the overall immune effect (108). Another problem with T cell targeting imaging is to determine the optimal timing of evaluation to reasonably reflect the activation degree of T cells.
Secretory substances exist in the extracellular environment and participate in information transmission and effect exertion. Imaging targeting such substances, such as granzyme B and interferon-gamma (IFN-γ), has potential advantages in showing the immune treatment response. With regards to granzyme B targeted imaging, 18F-Ara-Gand and 68Ga-NOTA-GZP can reflect the activation of CD8+ T cells and help to distinguish between pseudoprogression and true progression (107). Another study showed that the detection of granzyme B with 68Ga-NOTA-GZP helps differentiate the responders from non-responders to immunotherapy (109). 89Zr-DFO-AN-18, a novel probe targeting IFN-γ, has been indicated to monitor the response to immunotherapy in mouse mammary tumors (110).
In summary, an in-depth understanding of the underlying mechanisms of cancer immunotherapy is an important cornerstone for expanding the benefits of ICIs treatment to a larger cancer population. Hence, diagnostic approaches, especially PET/CT molecular imaging, should be vigorously developed to identify patients who might benefit from ICIs treatment. Concurrently, under the guidance of PET/CT molecular imaging, clinicians can shift the paradigm to improve the outcome of cancer patients, and even facilitate the development of novel therapeutic strategies to enhance therapeutic effectiveness. It is believed that with the extensive availability of standardized protocols, various affordable imaging agents, and user-friendly analysis platforms, PET/CT imaging will play a more important role in the era of immuno-oncology.
Conceptualization, ML. Methodology, ML, YG, and CW. Material preparation, YG, CW, XC, LM, XZ, JC, and XL. Writing-original draft preparation, YG and CW. Writing-review and editing, ML and XC. Funding acquisition, ML. Supervision, ML. All authors contributed to the article and approved the submitted version.
This study was supported by grants from the National Natural Science Foundation of China (82172052), Beijing Natural Science Foundation (Z210007), and National High-Level Hospital Clinical Research Funding (Interdepartmental Clinical Research Project of Peking University First Hospital) (2022CR34).
The authors declare that the research was conducted in the absence of any commercial or financial relationships that could be construed as a potential conflict of interest.
All claims expressed in this article are solely those of the authors and do not necessarily represent those of their affiliated organizations, or those of the publisher, the editors and the reviewers. Any product that may be evaluated in this article, or claim that may be made by its manufacturer, is not guaranteed or endorsed by the publisher.
1. Zhang Y, Zhang Z. The history and advances in cancer immunotherapy: understanding the characteristics of tumor-infiltrating immune cells and their therapeutic implications. Cell Mol Immunol (2020) 17:807–21. doi: 10.1038/s41423-020-0488-6
2. Iravani A, Hicks RJ. Imaging the cancer immune environment and its response to pharmacologic intervention, part 1: The role of (18)F-FDG PET/CT. J Nucl Med (2020) 61:943–50. doi: 10.2967/jnumed.119.234278
3. Dall'Olio FG, Marabelle A, Caramella C, Garcia C, Aldea M, Chaput N, et al. Tumour burden and efficacy of immune-checkpoint inhibitors. Nat Rev Clin Oncol (2022) 19:75–90. doi: 10.1038/s41571-021-00564-3
4. Park K, Veena MS, Shin DS. Key players of the immunosuppressive tumor microenvironment and emerging therapeutic strategies. Front Cell Dev Biol (2022) 10:830208. doi: 10.3389/fcell.2022.830208
5. Elia I, Haigis MC. Metabolites and the tumour microenvironment: from cellular mechanisms to systemic metabolism. Nat Metab (2021) 3:21–32. doi: 10.1038/s42255-020-00317-z
6. Leone RD, Powell JD. Metabolism of immune cells in cancer. Nat Rev Cancer (2020) 20:516–31. doi: 10.1038/s41568-020-0273-y
7. Cascone T, McKenzie JA, Mbofung RM, Punt S, Wang Z, Xu C, et al. Increased tumor glycolysis characterizes immune resistance to adoptive T cell therapy. Cell Metab (2018) 27:977–987.e4. doi: 10.1016/j.cmet.2018.02.024
8. Angelin A, Gil-de-Gómez L, Dahiya S, Jiao J, Guo L, Levine MH, et al. Foxp3 reprograms T cell metabolism to function in low-glucose, high-lactate environments. Cell Metab (2017) 25:1282–1293.e7. doi: 10.1016/j.cmet.2016.12.018
9. Bussard KM, Mutkus L, Stumpf K, Gomez-Manzano C, Marini FC. Tumor-associated stromal cells as key contributors to the tumor microenvironment. Breast Cancer Res (2016) 18:84. doi: 10.1186/s13058-016-0740-2
10. Pavlides S, Whitaker-Menezes D, Castello-Cros R, Flomenberg N, Witkiewicz AK, Frank PG, et al. The reverse warburg effect: aerobic glycolysis in cancer associated fibroblasts and the tumor stroma. Cell Cycle (2009) 8:3984–4001. doi: 10.4161/cc.8.23.10238
11. Chen DS, Mellman I. Elements of cancer immunity and the cancer-immune set point. Nature (2017) 541:321–30. doi: 10.1038/nature21349
12. Hamada T, Soong TR, Masugi Y, Kosumi K, Nowak JA, da Silva A, et al. TIME (Tumor immunity in the MicroEnvironment) classification based on tumor CD274 (PD-L1) expression status and tumor-infiltrating lymphocytes in colorectal carcinomas. Oncoimmunology (2018) 7:e1442999. doi: 10.1080/2162402x.2018.1442999
13. Taube JM, Anders RA, Young GD, Xu H, Sharma R, McMiller TL, et al. Colocalization of inflammatory response with B7-h1 expression in human melanocytic lesions supports an adaptive resistance mechanism of immune escape. Sci Transl Med (2012) 4:127ra37. doi: 10.1126/scitranslmed.3003689
14. Kim TK, Vandsemb EN, Herbst RS, Chen L. Adaptive immune resistance at the tumour site: mechanisms and therapeutic opportunities. Nat Rev Drug Discovery (2022) 21:529–40. doi: 10.1038/s41573-022-00493-5
15. Siska PJ, Singer K, Evert K, Renner K, Kreutz M. The immunological warburg effect: Can a metabolic-tumor-stroma score (MeTS) guide cancer immunotherapy? Immunol Rev (2020) 295:187–202. doi: 10.1111/imr.12846
16. Xie H, Simon MC. Oxygen availability and metabolic reprogramming in cancer. J Biol Chem (2017) 292:16825–32. doi: 10.1074/jbc.R117.799973
17. Noman MZ, Desantis G, Janji B, Hasmim M, Karray S, Dessen P, et al. PD-L1 is a novel direct target of HIF-1α, and its blockade under hypoxia enhanced MDSC-mediated T cell activation. J Exp Med (2014) 211:781–90. doi: 10.1084/jem.20131916
18. Riera-Domingo C, Audigé A, Granja S, Cheng WC, Ho PC, Baltazar F, et al. Immunity, hypoxia, and metabolism-the ménage à trois of cancer: Implications for immunotherapy. Physiol Rev (2020) 100:1–102. doi: 10.1152/physrev.00018.2019
19. Zhao L, Liu J, Wang H, Shi J. Association between (18)F-FDG metabolic activity and programmed death ligand-1 (PD-L1) expression using 22C3 immunohistochemistry assays in non-small cell lung cancer (NSCLC) resection specimens. Br J Radiol (2021) 94:20200397. doi: 10.1259/bjr.20200397
20. Zhao L, Liu J, Shi J, Wang H. Relationship between SP142 PD-L1 expression and (18)F-FDG uptake in non-Small-Cell lung cancer. Contrast Media Mol Imaging (2020) 2020:2010924. doi: 10.1155/2020/2010924
21. Mitchell KG, Amini B, Wang Y, Carter BW, Godoy MCB, Parra ER, et al. (18)F-fluorodeoxyglucose positron emission tomography correlates with tumor immunometabolic phenotypes in resected lung cancer. Cancer Immunol Immunother (2020) 69:1519–34. doi: 10.1007/s00262-020-02560-5
22. Wang Y, Zhao N, Wu Z, Pan N, Shen X, Liu T, et al. New insight on the correlation of metabolic status on (18)F-FDG PET/CT with immune marker expression in patients with non-small cell lung cancer. Eur J Nucl Med Mol Imaging (2020) 47:1127–36. doi: 10.1007/s00259-019-04500-7
23. Miyazawa T, Otsubo K, Sakai H, Kimura H, Chosokabe M, Morikawa K, et al. Combining PD-L1 expression and standardized uptake values in FDG-PET/CT can predict prognosis in patients with resectable non-Small-Cell lung cancer. Cancer Control (2021) 28:10732748211038314. doi: 10.1177/10732748211038314
24. Wu X, Huang Y, Zhao Q, Wang L, Song X, Li Y, et al. PD-L1 expression correlation with metabolic parameters of FDG PET/CT and clinicopathological characteristics in non-small cell lung cancer. EJNMMI Res (2020) 10:51. doi: 10.1186/s13550-020-00639-9
25. Wang L, Ruan M, Lei B, Yan H, Sun X, Chang C, et al. The potential of (18)F-FDG PET/CT in predicting PDL1 expression status in pulmonary lesions of untreated stage IIIB-IV non-small-cell lung cancer. Lung Cancer (2020) 150:44–52. doi: 10.1016/j.lungcan.2020.10.004
26. Lopci E, Toschi L, Grizzi F, Rahal D, Olivari L, Castino GF, et al. Correlation of metabolic information on FDG-PET with tissue expression of immune markers in patients with non-small cell lung cancer (NSCLC) who are candidates for upfront surgery. Eur J Nucl Med Mol Imaging (2016) 43:1954–61. doi: 10.1007/s00259-016-3425-2
27. Jreige M, Letovanec I, Chaba K, Renaud S, Rusakiewicz S, Cristina V, et al. (18)F-FDG PET metabolic-to-morphological volume ratio predicts PD-L1 tumour expression and response to PD-1 blockade in non-small-cell lung cancer. Eur J Nucl Med Mol Imaging (2019) 46:1859–68. doi: 10.1007/s00259-019-04348-x
28. Li J, Ge S, Sang S, Hu C, Deng S. Evaluation of PD-L1 expression level in patients with non-small cell lung cancer by (18)F-FDG PET/CT radiomics and clinicopathological characteristics. Front Oncol (2021) 11:789014. doi: 10.3389/fonc.2021.789014
29. Mu W, Jiang L, Shi Y, Tunali I, Gray JE, Katsoulakis E, et al. Non-invasive measurement of PD-L1 status and prediction of immunotherapy response using deep learning of PET/CT images. J Immunother Cancer (2021) 9(6):e002118. doi: 10.1136/jitc-2020-002118
30. Monaco L, De Bernardi E, Bono F, Cortinovis D, Crivellaro C, Elisei F, et al. The "digital biopsy" in non-small cell lung cancer (NSCLC): a pilot study to predict the PD-L1 status from radiomics features of [18F]FDG PET/CT. Eur J Nucl Med Mol Imaging (2022) 49(10):3401–11. doi: 10.1007/s00259-022-05783-z
31. Zhou J, Zou S, Kuang D, Yan J, Zhao J, Zhu X. A novel approach using FDG-PET/CT-Based radiomics to assess tumor immune phenotypes in patients with non-small cell lung cancer. Front Oncol (2021) 11:769272. doi: 10.3389/fonc.2021.769272
32. Wu C, Cui Y, Liu J, Ma L, Xiong Y, Gong Y, et al. Noninvasive evaluation of tumor immune microenvironment in patients with clear cell renal cell carcinoma using metabolic parameter from preoperative 2-[(18)F]FDG PET/CT. Eur J Nucl Med Mol Imaging (2021) 48:4054–66. doi: 10.1007/s00259-021-05399-9
33. Hirakata T, Fujii T, Kurozumi S, Katayama A, Honda C, Yanai K, et al. FDG uptake reflects breast cancer immunological features: the PD-L1 expression and degree of TILs in primary breast cancer. Breast Cancer Res Treat (2020) 181:331–8. doi: 10.1007/s10549-020-05619-0
34. Park S, Min EK, Bae SJ, Cha C, Kim D, Lee J, et al. Relationship of the standard uptake value of (18)F-FDG-PET-CT with tumor-infiltrating lymphocytes in breast tumors measuring ≥ 1 cm. Sci Rep (2021) 11:12046. doi: 10.1038/s41598-021-91404-y
35. Sasada S, Shiroma N, Goda N, Kajitani K, Emi A, Masumoto N, et al. The relationship between ring-type dedicated breast PET and immune microenvironment in early breast cancer. Breast Cancer Res Treat (2019) 177:651–7. doi: 10.1007/s10549-019-05339-0
36. Lee S, Choi S, Kim SY, Yun MJ, Kim HI. Potential utility of FDG PET-CT as a non-invasive tool for monitoring local immune responses. J Gastric Cancer (2017) 17:384–93. doi: 10.5230/jgc.2017.17.e43
37. Jiang H, Zhang R, Jiang H, Zhang M, Guo W, Zhang J, et al. Retrospective analysis of the prognostic value of PD-L1 expression and (18)F-FDG PET/CT metabolic parameters in colorectal cancer. J Cancer (2020) 11:2864–73. doi: 10.7150/jca.38689
38. Chen R, Zhou X, Liu J, Huang G. Relationship between the expression of PD-1/PD-L1 and (18)F-FDG uptake in bladder cancer. Eur J Nucl Med Mol Imaging (2019) 46:848–54. doi: 10.1007/s00259-018-4208-8
39. Zhao L, Zhuang Y, Fu K, Chen P, Wang Y, Zhuo J, et al. Usefulness of [(18)F]fluorodeoxyglucose PET/CT for evaluating the PD-L1 status in nasopharyngeal carcinoma. Eur J Nucl Med Mol Imaging (2020) 47:1065–74. doi: 10.1007/s00259-019-04654-4
40. Togo M, Yokobori T, Shimizu K, Handa T, Kaira K, Sano T, et al. Diagnostic value of (18)F-FDG-PET to predict the tumour immune status defined by tumoural PD-L1 and CD8(+)tumour-infiltrating lymphocytes in oral squamous cell carcinoma. Br J Cancer (2020) 122:1686–94. doi: 10.1038/s41416-020-0820-z
41. Seol HY, Kim YS, Kim SJ. Diagnostic test accuracy of (18)F-FDG PET/CT for prediction of programmed death ligand 1 (PD-L1) expression in solid tumours: a meta-analysis. Clin Radiol (2021) 76:863.e19–863.e25. doi: 10.1016/j.crad.2021.06.012
42. Zhou J, Zou S, Cheng S, Kuang D, Li D, Chen L, et al. Correlation between dual-Time-Point FDG PET and tumor microenvironment immune types in non-small cell lung cancer. Front Oncol (2021) 11:559623. doi: 10.3389/fonc.2021.559623
43. Morad G, Helmink BA, Sharma P, Wargo JA. Hallmarks of response, resistance, and toxicity to immune checkpoint blockade. Cell (2021) 184:5309–37. doi: 10.1016/j.cell.2021.09.020
44. Darnell EP, Mooradian MJ, Baruch EN, Yilmaz M, Reynolds KL. Immune-related adverse events (irAEs): Diagnosis, management, and clinical pearls. Curr Oncol Rep (2020) 22:39. doi: 10.1007/s11912-020-0897-9
45. Nobashi T, Mittra E. PD-1 blockade-induced inflammatory arthritis. Radiology (2018) 289:616. doi: 10.1148/radiol.2018181532
46. Wang DY, Salem JE, Cohen JV, Chandra S, Menzer C, Ye F, et al. Fatal toxic effects associated with immune checkpoint inhibitors: A systematic review and meta-analysis. JAMA Oncol (2018) 4:1721–8. doi: 10.1001/jamaoncol.2018.3923
47. Das S, Johnson DB. Immune-related adverse events and anti-tumor efficacy of immune checkpoint inhibitors. J Immunother Cancer (2019) 7:306. doi: 10.1186/s40425-019-0805-8
48. Fan Y, Xie W, Huang H, Wang Y, Li G, Geng Y, et al. Association of immune related adverse events with efficacy of immune checkpoint inhibitors and overall survival in cancers: A systemic review and meta-analysis. Front Oncol (2021) 11:633032. doi: 10.3389/fonc.2021.633032
49. Haratani K, Hayashi H, Chiba Y, Kudo K, Yonesaka K, Kato R, et al. Association of immune-related adverse events with nivolumab efficacy in non-Small-Cell lung cancer. JAMA Oncol (2018) 4:374–8. doi: 10.1001/jamaoncol.2017.2925
50. Paderi A, Giorgione R, Giommoni E, Mela MM, Rossi V, Doni L, et al. Association between immune related adverse events and outcome in patients with metastatic renal cell carcinoma treated with immune checkpoint inhibitors. Cancers (Basel) (2021) 13(4):860. doi: 10.3390/cancers13040860
51. Kijima T, Fukushima H, Kusuhara S, Tanaka H, Yoshida S, Yokoyama M, et al. Association between the occurrence and spectrum of immune-related adverse events and efficacy of pembrolizumab in Asian patients with advanced urothelial cancer: Multicenter retrospective analyses and systematic literature review. Clin Genitourin Cancer (2021) 19:208–216.e1. doi: 10.1016/j.clgc.2020.07.003
52. Nobashi T, Baratto L, Reddy SA, Srinivas S, Toriihara A, Hatami N, et al. Predicting response to immunotherapy by evaluating tumors, lymphoid cell-rich organs, and immune-related adverse events using FDG-PET/CT. Clin Nucl Med (2019) 44:e272–9. doi: 10.1097/rlu.0000000000002453
53. Wang D, Chen C, Gu Y, Lu W, Zhan P, Liu H, et al. Immune-related adverse events predict the efficacy of immune checkpoint inhibitors in lung cancer patients: A meta-analysis. Front Oncol (2021) 11:631949. doi: 10.3389/fonc.2021.631949
54. Morimoto K, Yamada T, Takumi C, Ogura Y, Takeda T, Onoi K, et al. Immune-related adverse events are associated with clinical benefit in patients with non-Small-Cell lung cancer treated with immunotherapy plus chemotherapy: A retrospective study. Front Oncol (2021) 11:630136. doi: 10.3389/fonc.2021.630136
55. Tang YZ, Szabados B, Leung C, Sahdev A. Adverse effects and radiological manifestations of new immunotherapy agents. Br J Radiol (2019) 92:20180164. doi: 10.1259/bjr.20180164
56. Hribernik N, Huff DT, Studen A, Zevnik K, Klaneček Ž, Emamekhoo H, et al. Quantitative imaging biomarkers of immune-related adverse events in immune-checkpoint blockade-treated metastatic melanoma patients: a pilot study. Eur J Nucl Med Mol Imaging (2022) 49:1857–69. doi: 10.1007/s00259-021-05650-3
57. Eshghi N, Garland LL, Nia E, Betancourt R, Krupinski E, Kuo PH. (18)F-FDG PET/CT can predict development of thyroiditis due to immunotherapy for lung cancer. J Nucl Med Technol (2018) 46:260–4. doi: 10.2967/jnmt.117.204933
58. Das JP, Postow MA, Friedman CF, Do RK, Halpenny DF. Imaging findings of immune checkpoint inhibitor associated pancreatitis. Eur J Radiol (2020) 131:109250. doi: 10.1016/j.ejrad.2020.109250
59. Eisenhauer EA, Therasse P, Bogaerts J, Schwartz LH, Sargent D, Ford R, et al. New response evaluation criteria in solid tumours: revised RECIST guideline (version 1.1). Eur J Cancer (2009) 45:228–47. doi: 10.1016/j.ejca.2008.10.026
60. Seymour L, Bogaerts J, Perrone A, Ford R, Schwartz LH, Mandrekar S, et al. iRECIST: guidelines for response criteria for use in trials testing immunotherapeutics. Lancet Oncol (2017) 18:e143–52. doi: 10.1016/s1470-2045(17)30074-8
61. Hodi FS, Ballinger M, Lyons B, Soria JC, Nishino M, Tabernero J, et al. Immune-modified response evaluation criteria in solid tumors (imRECIST): Refining guidelines to assess the clinical benefit of cancer immunotherapy. J Clin Oncol (2018) 36:850–8. doi: 10.1200/jco.2017.75.1644
62. Young H, Baum R, Cremerius U, Herholz K, Hoekstra O, Lammertsma AA, et al. Measurement of clinical and subclinical tumour response using [18F]-fluorodeoxyglucose and positron emission tomography: review and 1999 EORTC recommendations. European organization for research and treatment of cancer (EORTC) PET study group. Eur J Cancer (1999) 35:1773–82. doi: 10.1016/s0959-8049(99)00229-4
63. Wahl RL, Jacene H, Kasamon Y, Lodge MA. From RECIST to PERCIST: Evolving considerations for PET response criteria in solid tumors. J Nucl Med (2009) 50 Suppl 1:122s–50s. doi: 10.2967/jnumed.108.057307
64. Goldfarb L, Duchemann B, Chouahnia K, Zelek L, Soussan M. Monitoring anti-PD-1-based immunotherapy in non-small cell lung cancer with FDG PET: introduction of iPERCIST. EJNMMI Res (2019) 9:8. doi: 10.1186/s13550-019-0473-1
65. Ito K, Teng R, Schöder H, Humm JL, Ni A, Michaud L, et al. (18)F-FDG PET/CT for monitoring of ipilimumab therapy in patients with metastatic melanoma. J Nucl Med (2019) 60:335–41. doi: 10.2967/jnumed.118.213652
66. Anwar H, Sachpekidis C, Winkler J, Kopp-Schneider A, Haberkorn U, Hassel JC, et al. Absolute number of new lesions on (18)F-FDG PET/CT is more predictive of clinical response than SUV changes in metastatic melanoma patients receiving ipilimumab. Eur J Nucl Med Mol Imaging (2018) 45:376–83. doi: 10.1007/s00259-017-3870-6
67. Sachpekidis C, Anwar H, Winkler J, Kopp-Schneider A, Larribere L, Haberkorn U, et al. The role of interim (18)F-FDG PET/CT in prediction of response to ipilimumab treatment in metastatic melanoma. Eur J Nucl Med Mol Imaging (2018) 45:1289–96. doi: 10.1007/s00259-018-3972-9
68. Sachpekidis C, Kopp-Schneider A, Hakim-Meibodi L, Dimitrakopoulou-Strauss A, Hassel JC. 18F-FDG PET/CT longitudinal studies in patients with advanced metastatic melanoma for response evaluation of combination treatment with vemurafenib and ipilimumab. Melanoma Res (2019) 29:178–86. doi: 10.1097/cmr.0000000000000541
69. Beer L, Hochmair M, Haug AR, Schwabel B, Kifjak D, Wadsak W, et al. Comparison of RECIST, iRECIST, and PERCIST for the evaluation of response to PD-1/PD-L1 blockade therapy in patients with non-small cell lung cancer. Clin Nucl Med (2019) 44:535–43. doi: 10.1097/rlu.0000000000002603
70. Castello A, Rossi S, Toschi L, Lopci E. Comparison of metabolic and morphological response criteria for early prediction of response and survival in NSCLC patients treated with anti-PD-1/PD-L1. Front Oncol (2020) 10:1090. doi: 10.3389/fonc.2020.01090
71. Dimitriou F, Lo SN, Tan AC, Emmett L, Kapoor R, Carlino MS, et al. FDG-PET to predict long-term outcome from anti-PD-1 therapy in metastatic melanoma. Ann Oncol (2021) 33(1):99–106. doi: 10.1016/j.annonc.2021.10.003
72. Kitajima K, Watabe T, Nakajo M, Ishibashi M, Daisaki H, Soeda F, et al. Tumor response evaluation in patients with malignant melanoma undergoing immune checkpoint inhibitor therapy and prognosis prediction using (18)F-FDG PET/CT: multicenter study for comparison of EORTC, PERCIST, and imPERCIST. Jpn J Radiol (2022) 40:75–85. doi: 10.1007/s11604-021-01174-w
73. Zhu J, Armstrong AJ, Friedlander TW, Kim W, Pal SK, George DJ, et al. Biomarkers of immunotherapy in urothelial and renal cell carcinoma: PD-L1, tumor mutational burden, and beyond. J Immunother Cancer (2018) 6:4. doi: 10.1186/s40425-018-0314-1
74. Brody R, Zhang Y, Ballas M, Siddiqui MK, Gupta P, Barker C, et al. PD-L1 expression in advanced NSCLC: Insights into risk stratification and treatment selection from a systematic literature review. Lung Cancer (2017) 112:200–15. doi: 10.1016/j.lungcan.2017.08.005
75. Seban RD, Nemer JS, Marabelle A, Yeh R, Deutsch E, Ammari S, et al. Prognostic and theranostic 18F-FDG PET biomarkers for anti-PD1 immunotherapy in metastatic melanoma: association with outcome and transcriptomics. Eur J Nucl Med Mol Imaging (2019) 46:2298–310. doi: 10.1007/s00259-019-04411-7
76. Ito K, Schöder H, Teng R, Humm JL, Ni A, Wolchok JD, et al. Prognostic value of baseline metabolic tumor volume measured on (18)F-fluorodeoxyglucose positron emission tomography/computed tomography in melanoma patients treated with ipilimumab therapy. Eur J Nucl Med Mol Imaging (2019) 46:930–9. doi: 10.1007/s00259-018-4211-0
77. Seban RD, Mezquita L, Berenbaum A, Dercle L, Botticella A, Le Pechoux C, et al. Baseline metabolic tumor burden on FDG PET/CT scans predicts outcome in advanced NSCLC patients treated with immune checkpoint inhibitors. Eur J Nucl Med Mol Imaging (2020) 47:1147–57. doi: 10.1007/s00259-019-04615-x
78. Hashimoto K, Kaira K, Yamaguchi O, Mouri A, Shiono A, Miura Y, et al. Potential of FDG-PET as prognostic significance after anti-PD-1 antibody against patients with previously treated non-small cell lung cancer. J Clin Med (2020) 9(3):725. doi: 10.3390/jcm9030725
79. Castello A, Rossi S, Mazziotti E, Toschi L, Lopci E. Hyperprogressive disease in patients with non-small cell lung cancer treated with checkpoint inhibitors: The role of (18)F-FDG PET/CT. J Nucl Med (2020) 61:821–6. doi: 10.2967/jnumed.119.237768
80. Yamaguchi O, Kaira K, Hashimoto K, Mouri A, Shiono A, Miura Y, et al. Tumor metabolic volume by (18)F-FDG-PET as a prognostic predictor of first-line pembrolizumab for NSCLC patients with PD-L1 ≥ 50. Sci Rep (2020) 10:14990. doi: 10.1038/s41598-020-71735-y
81. Polverari G, Ceci F, Bertaglia V, Reale ML, Rampado O, Gallio E, et al. (18)F-FDG pet parameters and radiomics features analysis in advanced nsclc treated with immunotherapy as predictors of therapy response and survival. Cancers (Basel) (2020) 12(5):1163. doi: 10.3390/cancers12051163
82. Chardin D, Paquet M, Schiappa R, Darcourt J, Bailleux C, Poudenx M, et al. Baseline metabolic tumor volume as a strong predictive and prognostic biomarker in patients with non-small cell lung cancer treated with PD1 inhibitors: a prospective study. J Immunother Cancer (2020) 8(2):e000645. doi: 10.1136/jitc-2020-000645
83. Seban RD, Assie JB, Giroux-Leprieur E, Massiani MA, Soussan M, Bonardel G, et al. FDG-PET biomarkers associated with long-term benefit from first-line immunotherapy in patients with advanced non-small cell lung cancer. Ann Nucl Med (2020) 34:968–74. doi: 10.1007/s12149-020-01539-7
84. Wong A, Callahan J, Keyaerts M, Neyns B, Mangana J, Aberle S, et al. (18)F-FDG PET/CT based spleen to liver ratio associates with clinical outcome to ipilimumab in patients with metastatic melanoma. Cancer Imaging (2020) 20:36. doi: 10.1186/s40644-020-00313-2
85. Seban RD, Moya-Plana A, Antonios L, Yeh R, Marabelle A, Deutsch E, et al. Prognostic 18F-FDG PET biomarkers in metastatic mucosal and cutaneous melanoma treated with immune checkpoint inhibitors targeting PD-1 and CTLA-4. Eur J Nucl Med Mol Imaging (2020) 47:2301–12. doi: 10.1007/s00259-020-04757-3
86. Dall'Olio FG, Calabrò D, Conci N, Argalia G, Marchese PV, Fabbri F, et al. Baseline total metabolic tumour volume on 2-deoxy-2-[18F]fluoro-d-glucose positron emission tomography-computed tomography as a promising biomarker in patients with advanced non-small cell lung cancer treated with first-line pembrolizumab. Eur J Cancer (2021) 150:99–107. doi: 10.1016/j.ejca.2021.03.020
87. Vekens K, Everaert H, Neyns B, Ilsen B, Decoster L. The value of (18)F-FDG PET/CT in predicting the response to PD-1 blocking immunotherapy in advanced NSCLC patients with high-level PD-L1 expression. Clin Lung Cancer (2021) 22:432–40. doi: 10.1016/j.cllc.2021.03.001
88. Bauckneht M, Genova C, Rossi G, Rijavec E, Dal Bello MG, Ferrarazzo G, et al. The role of the immune metabolic prognostic index in patients with non-small cell lung cancer (NSCLC) in radiological progression during treatment with nivolumab. Cancers (Basel) (2021) 13(13):3117. doi: 10.3390/cancers13133117
89. Awada G, Jansen Y, Schwarze JK, Tijtgat J, Hellinckx L, Gondry O, et al. A comprehensive analysis of baseline clinical characteristics and biomarkers associated with outcome in advanced melanoma patients treated with pembrolizumab. Cancers (Basel) (2021) 13(2):168. doi: 10.3390/cancers13020168
90. Gulturk I, Yilmaz M, Yildiz Tacar S, Tural D. Prognostic importance of SUV-max values evaluated by 18F-FDG-PET/CT before nivolumab treatment in patients with metastatic renal cell carcinoma. Q J Nucl Med Mol Imaging (2021). doi: 10.23736/s1824-4785.21.03395-1
91. Mu W, Jiang L, Zhang J, Shi Y, Gray JE, Tunali I, et al. Non-invasive decision support for NSCLC treatment using PET/CT radiomics. Nat Commun (2020) 11:5228. doi: 10.1038/s41467-020-19116-x
92. Mu W, Tunali I, Gray JE, Qi J, Schabath MB, Gillies RJ. Radiomics of (18)F-FDG PET/CT images predicts clinical benefit of advanced NSCLC patients to checkpoint blockade immunotherapy. Eur J Nucl Med Mol Imaging (2020) 47:1168–82. doi: 10.1007/s00259-019-04625-9
93. Niemeijer AN, Oprea-Lager DE, Huisman MC, Hoekstra OS, Boellaard R, de Wit-van der Veen BJ, et al. Study of (89)Zr-pembrolizumab PET/CT in patients with advanced-stage non-small cell lung cancer. J Nucl Med (2022) 63:362–7. doi: 10.2967/jnumed.121.261926
94. Kok IC, Hooiveld JS, van de Donk PP, Giesen D, van der Veen EL, Lub-de Hooge MN, et al. (89)Zr-pembrolizumab imaging as a non-invasive approach to assess clinical response to PD-1 blockade in cancer. Ann Oncol (2022) 33:80–8. doi: 10.1016/j.annonc.2021.10.213
95. Rakhshandehroo T, Smith BR, Glockner HJ, Rashidian M, Pandit-Taskar N. Molecular immune targeted imaging of tumor microenvironment. Nanotheranostics (2022) 6:286–305. doi: 10.7150/ntno.66556
96. Bensch F, van der Veen EL, Lub-de Hooge MN, Jorritsma-Smit A, Boellaard R, Kok IC, et al. (89)Zr-atezolizumab imaging as a non-invasive approach to assess clinical response to PD-L1 blockade in cancer. Nat Med (2018) 24:1852–8. doi: 10.1038/s41591-018-0255-8
97. Vento J, Mulgaonkar A, Woolford L, Nham K, Christie A, Bagrodia A, et al. PD-L1 detection using (89)Zr-atezolizumab immuno-PET in renal cell carcinoma tumorgrafts from a patient with favorable nivolumab response. J Immunother Cancer (2019) 7:144. doi: 10.1186/s40425-019-0607-z
98. Gao H, Wu Y, Shi J, Zhang X, Liu T, Hu B, et al. Nuclear imaging-guided PD-L1 blockade therapy increases effectiveness of cancer immunotherapy. J Immunother Cancer (2020) 8(2):e001156. doi: 10.1136/jitc-2020-001156
99. Du Y, Jin Y, Sun W, Fang J, Zheng J, Tian J. Advances in molecular imaging of immune checkpoint targets in malignancies: current and future prospect. Eur Radiol (2019) 29:4294–302. doi: 10.1007/s00330-018-5814-3
100. Ehlerding EB, Lee HJ, Jiang D, Ferreira CA, Zahm CD, Huang P, et al. Antibody and fragment-based PET imaging of CTLA-4+ T-cells in humanized mouse models. Am J Cancer Res (2019) 9:53–63.
101. Ehlerding EB, England CG, Majewski RL, Valdovinos HF, Jiang D, Liu G, et al. ImmunoPET imaging of CTLA-4 expression in mouse models of non-small cell lung cancer. Mol Pharm (2017) 14:1782–9. doi: 10.1021/acs.molpharmaceut.7b00056
102. Xiao Z, Mayer AT, Nobashi TW, Gambhir SS. ICOS is an indicator of T-cell-Mediated response to cancer immunotherapy. Cancer Res (2020) 80:3023–32. doi: 10.1158/0008-5472.Can-19-3265
103. Simonetta F, Alam IS, Lohmeyer JK, Sahaf B, Good Z, Chen W, et al. Molecular imaging of chimeric antigen receptor T cells by ICOS-ImmunoPET. Clin Cancer Res (2021) 27:1058–68. doi: 10.1158/1078-0432.Ccr-20-2770
104. Tumeh PC, Harview CL, Yearley JH, Shintaku IP, Taylor EJ, Robert L, et al. PD-1 blockade induces responses by inhibiting adaptive immune resistance. Nature (2014) 515:568–71. doi: 10.1038/nature13954
105. Pandit-Taskar N, Postow MA, Hellmann MD, Harding JJ, Barker CA, O'Donoghue JA, et al. First-in-Humans imaging with (89)Zr-Df-IAB22M2C anti-CD8 minibody in patients with solid malignancies: Preliminary pharmacokinetics, biodistribution, and lesion targeting. J Nucl Med (2020) 61:512–9. doi: 10.2967/jnumed.119.229781
106. Rashidian M, Ingram JR, Dougan M, Dongre A, Whang KA, LeGall C, et al. Predicting the response to CTLA-4 blockade by longitudinal noninvasive monitoring of CD8 T cells. J Exp Med (2017) 214:2243–55. doi: 10.1084/jem.20161950
107. Iravani A, Hicks RJ. Imaging the cancer immune environment and its response to pharmacologic intervention, part 2: The role of novel PET agents. J Nucl Med (2020) 61:1553–9. doi: 10.2967/jnumed.120.248823
108. van de Donk PP, Kist de Ruijter L, Lub-de Hooge MN, Brouwers AH, van der Wekken AJ, Oosting SF, et al. Molecular imaging biomarkers for immune checkpoint inhibitor therapy. Theranostics (2020) 10:1708–18. doi: 10.7150/thno.38339
109. Larimer BM, Wehrenberg-Klee E, Dubois F, Mehta A, Kalomeris T, Flaherty K, et al. Granzyme b PET imaging as a predictive biomarker of immunotherapy response. Cancer Res (2017) 77:2318–27. doi: 10.1158/0008-5472.Can-16-3346
Keywords: positron emission tomography/computed tomography (PET/CT), molecular imaging, immune-checkpoint inhibitors (ICIs), tumor microenvironment (TME), metabolic parameter, molecular probe
Citation: Gao Y, Wu C, Chen X, Ma L, Zhang X, Chen J, Liao X and Liu M (2022) PET/CT molecular imaging in the era of immune-checkpoint inhibitors therapy. Front. Immunol. 13:1049043. doi: 10.3389/fimmu.2022.1049043
Received: 20 September 2022; Accepted: 10 October 2022;
Published: 20 October 2022.
Edited by:
Fei Yu, Tongji University School of Medicine, ChinaReviewed by:
Tomomi W. Nobashi, Kyoto University, JapanCopyright © 2022 Gao, Wu, Chen, Ma, Zhang, Chen, Liao and Liu. This is an open-access article distributed under the terms of the Creative Commons Attribution License (CC BY). The use, distribution or reproduction in other forums is permitted, provided the original author(s) and the copyright owner(s) are credited and that the original publication in this journal is cited, in accordance with accepted academic practice. No use, distribution or reproduction is permitted which does not comply with these terms.
*Correspondence: Meng Liu, bG91aXNhX2xpdUBiam11LmVkdS5jbg==
†These authors have contributed equally to this work and share first authorship
‡ORCID: Meng Liu, orcid.org/0000-0002-6960-3163
Disclaimer: All claims expressed in this article are solely those of the authors and do not necessarily represent those of their affiliated organizations, or those of the publisher, the editors and the reviewers. Any product that may be evaluated in this article or claim that may be made by its manufacturer is not guaranteed or endorsed by the publisher.
Research integrity at Frontiers
Learn more about the work of our research integrity team to safeguard the quality of each article we publish.