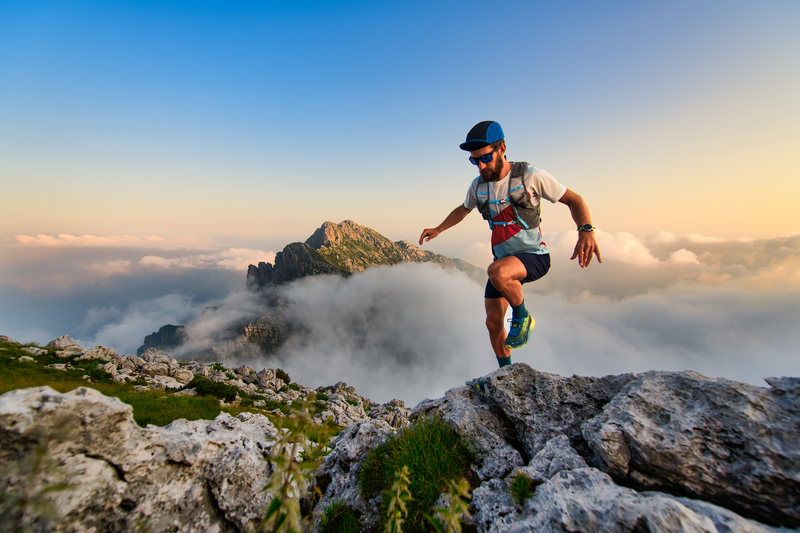
95% of researchers rate our articles as excellent or good
Learn more about the work of our research integrity team to safeguard the quality of each article we publish.
Find out more
ORIGINAL RESEARCH article
Front. Immunol. , 24 November 2022
Sec. Cancer Immunity and Immunotherapy
Volume 13 - 2022 | https://doi.org/10.3389/fimmu.2022.1046790
This article is part of the Research Topic Regulation of Tumor-immune Microenvironment by Non-coding RNAs View all 7 articles
Clear cell renal cell carcinoma (ccRCC) is a common aggressive malignant tumor of the urinary system. Given the heterogeneity of the tumor microenvironment, immunotherapy may not fully exert its role in the treatment of advanced patients. Long noncoding RNA (lncRNA) has been reported to be critically associated with the differentiation and maturation of tumor-infiltrating lymphocytes (TILs), which work against tumor cells. In this study, we identified 10 TIL-related lncRNAs (AL590094.1, LINC02027, LINC00460, AC147651.1, AC026401.3, LINC00944, LINC01615, AP000439.2, AL162586.1, and AC084876.1) by Pearson correlation, univariate Cox regression, Lasso regression, and multivariate Cox regression based on The Cancer Genome Atlas (TCGA) database. A risk score model was established based on these lncRNAs. Next, a nomogram was constructed to predict the overall survival. By employing differentially expressed genes (DEGs) between groups with high and low risk scores, gene ontology (GO) enrichment analysis was performed to identify the major biological processes (BP) related to immune DEGs. We analyzed the mutation data of the groups and demonstrated that SETD2 and BAP1 had the highest mutation frequency in the high-risk group. The “CIBERSORT” R package was used to detect the abundance of TILs in the groups. The expression of lymphocyte markers was compared. We also determined the expression of two lncRNAs (AC084876.1 and AC026401.3) and their relationship with lymphocyte markers in the kidney tissue of ccRCC patients and showed that there was a positive correlation between AC084876.1 and FoxP3. Proliferation, migration, and invasion of AC084876.1-downregulated ccRCC cell lines were inhibited, and the expression of PD-L1 and TGF-β secretion decreased. To our knowledge, this is the first bioinformatics study to establish a prognostic model for ccRCC using TIL-related lncRNAs. These lncRNAs were associated with T-cell activities and may serve as biomarkers of disease prognosis.
Renal cell carcinoma (RCC) is a common malignant tumor of the urinary system. Globally, 431,288 new cases (accounting for 2.2% of all cancer cases) and 179,368 new deaths (accounting for 1.8% of all cancer deaths) occurred in 2020, second only to prostate cancer and bladder cancer (1). In 2021, the number of new cases of kidney cancer in the United States was 76,080, ranking sixth in the male cancer incidence rate and ninth in the female cancer incidence rate (2). RCC has several subtypes, and about 70% of individuals receive a diagnosis of clear cell RCC (ccRCC). Although ccRCC is a disease that can be detected early and successfully treated by surgery, up to one-third of cases relapse or develop metastasis (3). ccRCC is not sensitive to radiotherapy and chemotherapy. The application of immunotherapy for different targets brings hope to these patients (4, 5); however, the effective response rate of the treatment is limited. Therefore, it is necessary to find new effective immunotherapeutic targets to improve patients’ prognoses.
Immune cells in the tumor microenvironment play an important role in regulating tumor progression and serve as attractive therapeutic targets (6). Moreover, ccRCC is prone to immune infiltration, and the characteristics of the tumor microenvironment strongly affect the response to immunotherapy (7). A successful antitumor immune response requires the activation and synergy of multiple tumor-infiltrating lymphocytes, including T cells, B cells, natural killer cells (NK cells), and their subtypes. These cells play a positive and negative regulatory role in the process of antitumor immunity and kill tumor cells (8). Available studies have constructed signatures related to immune infiltration based on different biological characteristics in ccRCC. Moreover, specific lymphocyte related signatures such as CD8+ T cells (9), CD39+CD8+ T cells (10), and TNFRSF9+CD8+ T cells (11) have been identified in ccRCC. However, previous studies did not focus on the impact of tumor-infiltrating lymphocyte profile on the prognosis of patients with ccRCC, so they could not accurately demonstrate the potential role of immune cells in the treatment of ccRCC.
Long noncoding RNAs (lncRNA) have a length of over 200 nucleotides and are dynamically expressed in the immune system and regulate the differentiation and function of immune cells (12). Previous studies have shown that lncRNAs are dysregulated in cancer and that they have important effects on tumor proliferation, angiogenesis, apoptosis, and metastasis by regulating the formation of the tumor immune microenvironment (13). For instance, lncRNA LIMIT has been identified to be associated with tumor-infiltrating T cells. Silencing LIMIT could impair antitumor immunity and blunt immunotherapy efficacy (14). Breast cancer–derived exosomal lncRNA SNHG16/miR-16-5p/SMAD5-regulatory axis can induce CD73 expression in γδT cells, thereby enhancing the immunosuppressive function (15). LINC00301 is highly expressed in non-small cell lung cancer and functions to increase regulatory T cells while decreasing the CD8+ T cell population (16). A clinical trial suggested that lncRNA was associated with immunotherapeutic overall survival benefits superior tumor alteration burden, programmed cell death ligand 1 (PD-L1) expression, and cytotoxic T-lymphocyte (CTL) infiltration (17). Based on the above findings, lncRNAs are considered a potential target for immunotherapy and have attracted extensive attention in the field of cancer treatment research.
With the maturation in the application of high-throughput sequencing technology, it has become possible to explore the expression levels of lncRNAs in ccRCC and their relationship with tumor-infiltrating lymphocytes; additionally, bioinformatics analysis can be applied to further explore the relevant mechanisms. In this study, we identified a signature consisting of 10 TIL-related lncRNAs to predict the prognosis of patients with ccRCC and explored the potential mechanism. Human renal tissues were used to detect the expression levels of related lncRNAs and immune markers. Finally, the biological functional effect of AC084876.1 on the ccRCC cell line was examined by in vitro experiments.
This study was approved by the Ethics Committee of Guangzhou First People’s Hospital, School of Medicine, South China University of Technology. Informed consent forms were signed by all patients. In accordance with the ethical and legal standards, seven pairs of matched frozen samples of ccRCC and benign renal tissue adjacent to cancer from ccRCC patients were handled and made anonymous.
A human ccRCC cell line 786-O was obtained from ATCC (American Type Culture Collection, Manassas, Virginia, USA). The cells were cultured at 37°C in a 5% CO2 incubator with RPMI-1640 (MA0215, Meilunbio, Dalian, China) medium containing 10% fetal bovine serum, streptomycin, and penicillin.
The RNA-sequencing data and corresponding clinical information of patients with ccRCC were downloaded from the Cancer Genome Atlas (TCGA-KIRC, https://cancergenome.nih.gov/) database. A total of 507 patients with follow-up information were identified and used for further analysis. The total RNA expression data were standardized through log2 transformation.
Seven pairs of the matched frozen samples of ccRCC and benign renal tissue adjacent to cancer used in this study were all from the Guangzhou First People’s Hospital. The included patients did not receive chemotherapy or radiotherapy before surgery. Each case was diagnosed and graded by two pathologists separately and re-examined by hematoxylin-eosin staining.
The correlation between lncRNAs and TIL-related genes (18) was calculated using the Pearson correlation analysis. The correlation coefficient |R| > 0.3 and P< 0.001 were the criteria for TIL-related lncRNAs. Univariate Cox regression was applied to assess the prognostic value of TIL-related lncRNAs. TIL-related lncRNAs with P< 0.001 in univariate analysis were incorporated into least absolute shrinkage and selection operator (Lasso) regression. Then, TIL-related lncRNAs identified by Lasso were included in a multivariate Cox model to establish a risk score. Finally, we identified 10 TIL-lncRNAs associated with the prognostic risk to construct a prognostic risk score. The risk score of KIRC patients was calculated as follows: Risk score = . Patients in the cohort were divided into high- and low-risk groups according to the median risk score.
Based on the TCGA-KIRC cohort, the expression of TIL-related lncRNAs was measured by ‘tinyarray’ R package. The Kaplan–Meier (K–M) survival curves of overall survival (OS) were used to evaluate the clinical prognostic value of TIL-related lncRNAs. Cytoscape software 3.7.2 was applied to visualize coexpression networks between TIL-related lncRNAs and TIL-related genes. Sankey diagram was used to assess the association between prognostic TIL-related lncRNAs, TIL-related genes, and risk types. The K–M survival curves were created to compare the overall survival of high- and low-risk groups according to predictive signatures. The receiver operating characteristic (ROC) curve from the “survivalROC” R package was used to assess the sensitivity and specificity of the signature. Univariate Cox regression and multivariate Cox regression were applied to assess the prognostic value of the signature.
Based on age, clinical stage, risk score, and pathological grade, a nomogram for OS was developed with the R package “rms” to predict the 1-year, 3-year, and 5-year relapse-free survival probability. The concordance index (C-index) was used to evaluate the consistency between the nomogram-predicted results and the actual observed results. A calibration curve was used to show the difference between the predictions of the model and the real outcomes. ROC analysis was performed to evaluate the predictive ability of the risk score. The web-based OS probability calculators were built using packages “DynNom” and “shiny” in R software.
Differentially expressed genes (DEGs) of mRNA between the high-risk and low-risk groups were identified using package limma in R, with thresholds of |log2 fold change (FC)| > 2 and adjusted P< 0.05. Then, gene ontology (GO) enrichment analysis was performed to find the major biological process (BP) related to immune DEGs. The visual GO enrichment maps of the annotation analysis results were obtained by R with the “ggplot2” and “GOplot” packages.
Mutation data of the high-risk group and the low-risk group were analyzed and visualized using the “maftools” package. Mutation information for each gene in each sample was demonstrated by waterfall plots.
To evaluate the effect of the signature on immune cells, CIBERSORT (Cell-type Identification by Estimating Relative Subsets of RNA Transcripts; http://cibersort.stanford.edu) was used to measure the abundance of tumor-infiltrating immune cells in a gene expression matrix by linear support vector regression (19). The abundance of lymphocyte profiles in the TCGA-KIRC dataset was obtained via the “CIBERSORT” R package. The infiltration levels in the high-risk group and the low-risk group were visualized by the “ggplot” R package.
Total RNA was extracted from ccRCC and corresponding benign renal tissue samples using NucleoZOL reagent (740404.200, Meilunbio). Total RNA was then reverse-transcribed into cDNA using HiScript® III RT SuperMix for qPCR (R323-01, Vazyme, Nanjing, China). Real-time quantitative PCR (RT-qPCR) was carried out using the AceQ® qPCR SYBR Green Master Mix (Q141-02, Vazyme). The relative expression of lncRNA was calculated based on the internal reference β-actin. All experiments were carried out in three replicates. The primers applied are listed in Supplementary Table S1.
Quantitative analysis of protein expression in clinical tissues was performed using western blotting in accordance with the protocol of our previous study (20). The following antibodies were applied in the assay: β-Actin (1:5000; ab8227, Abcam, Cambridge, UK), CD4 (1:2000; 19068-1-AP, Proteintech, Chicago, IL, USA), CD8α (1:2000; 66868-1-Ig, Proteintech), PD-1 (1:2000; 66220-1-Ig, Proteintech), FoxP3 (1:2000; 22228-1-AP, Proteintech), and PD-L1 (1:2000, PTM-5075, PTM BIO, Hangzhou, China). Correlations between the expression levels of lncRNAs and immune markers were determined by Pearson correlation analysis.
Human AC084876.1-specific siRNA and negative control siRNA were purchased from Tsingke Biotechnology Co., Ltd. (Beijing, China). The siRNA sequences are shown in the Supplementary Table S1. RT-qPCR analysis was conducted 72 h after transfection to test the transfection efficacy.
Cell proliferation was measured by CCK-8 (Cell Counting Kit-8, MA0218, Meilunbio) assay as previously described (20).
Cell migration and invasion were measured by a wound healing assay and transwell assay in line with the protocol from our previous study (20).
The assay was conducted using a human TGF-β ELISA kit (JM-04929H1, Jingmei, Jiangsu, China). The cell culture medium was tested in accordance with the manufacturer’s instructions. The results were normalized using cell counts.
All statistical analyses were performed in R software (version 4.03) and GraphPad Prism 8 (GraphPad Software, United States). Continuous variables are shown as the means ± standard deviations. Student’s t test or analysis of variance (ANOVA) was used to determine the statistical significance of quantitative data. P< 0.05 was regarded as statistically significant.
This study was performed following the workflow shown in Figure 1. A total of 589 candidate lncRNAs related to tumor-infiltrating lymphocytes (TIL) were identified by Pearson’s correlation analysis (Supplementary Table S2). To further evaluate the prognostic value of these candidate lncRNAs, we performed univariate Cox regression analysis with the p-value of 0.05 as the cutoff threshold; 334 lncRNAs were detected as prognostic TIL-lncRNAs (Figure 2A and Supplementary Table S3). Next, by leveraging the Lasso algorithm, these 334 TIL-lncRNAs were narrowed down to 20 with the optimal lambda of 0.06175523 (Figures 2B, C and Supplementary Table S4). Furthermore, among the 20 TIL-lncRNAs, 10 lncRNAs were found by multivariate Cox regression analysis to be independent prognostic factors in ccRCC (Figure 2D and Supplementary Table S5). Among these 10 TIL-lncRNAs, seven lncRNAs, i.e., AL590094.1 (HR = 1.23, 95% CI 1.03 to 1.16, P = 0.019), LINC00460 (HR = 1.05, 95% CI 1.00 to 1.10, P = 0.031), AC026401.3 (HR = 1.06, 95% CI 0.99 to 1.15, P = 0.115), LINC00944 (HR = 1.24, 95% CI 1.09 to 1.42, P = 0.001), LINC01615 (HR = 1.05, 95% CI 1.01 to 1.10, P = 0.023), AL162586.1 (HR = 1.26, 95% CI 1.11 to 1.42, P< 0.001), and AC084876.1 (HR = 1.24, 95% CI 1.01 to 1.52, P = 0.041) served as negative prognostic factors, while other three lncRNAs, i.e., LINC02027 (HR = 0.84, 95% CI 0.71 to 0.98, P = 0.031), AC147651.1 (HR = 0.98, 95% CI 0.96 to 1.00, P = 0.017), and AP000439.2 (HR = 0.99, 95% CI 0.97 to 1.00, P = 0.103) were favorable prognostic factors in ccRCC.
Figure 2 Identifying 10 TIL-related Long noncoding RNAs (lncRNAs)s. (A) Prognostic factors identified by univariate Cox regression. (B) Cross-validation for tuning parameter selection in the proportional hazards model. (C) Profiles of Lasso coefficients. (D) Multivariate Cox model of 10 TIL-related lncRNAs. (E) Correlation analysis between 10 TIL-related lncRNAs. “Corr” indicates the correlation coefficient generated by Pearson’s correlation analysis. *P < 0.05; **P < 0.01; ***P < 0.001.
Next, correlation analysis was conducted. As shown in Figure 2E, AC084876.1, LINC00944, and AC026401.3 had an obvious positive correlation with other lncRNAs, whereas AP000439.2 had a negative correlation with other lncRNAs. Taken together, 10 TIL-lncRNAs, which significantly correlated with TIL and were significant in the univariate Cox regression model, LASSO algorithm, and multivariate Cox proportional hazards regression model, were selected as the most important TIL-lncRNAs for further analysis.
We compared the abundance of 10 candidate TIL-lncRNAs between tumor and normal tissue in TCGA-KIRC by Wilcoxon signed-rank test and found that AL590094.1 (P< 2.2e-16), LINC00460 (P = 2.1e-06), AC147651.1 (P = 6.6e-07), AC026401.3 (P< 2.2e-16), LINC00944 (P< 2.2e-16), LINC01615 (P< 2.2e-16), AP000439.2 (P< 2.2e-16), AL162586.1 (P< 2.2e-16), and AC084876.1 (P< 2.2e-16) were significantly upregulated, while LINC02027 (P = 2.1e-06) was significantly downregulated in tumor tissue compared with normal tissue (Figure 3A). The prognostic value of the 10 TIL-lncRNAs was determined by K–M survival analysis (Figure 3B). Based on the median expression, patients were classified into high- and low-expression groups. Patients with high expression of AL590094.1, LINC00460, AC026401.3, LINC00944, LINC01615, AL162586.1, and AC084876.1 had poorer overall survival than those with low expression, whereas patients with high expression of LINC02027, AC147651.1, and AP000439.2 had better overall survival than those with low expression (log-rank test, all P< 0.05, Figure 3B), indicating that these 10 TIL-lncRNAs play a role in ccRCC. Considering that lncRNAs may participate in the splicing, maturation, transportation or localization, and stability of messenger RNAs (mRNAs), and thus regulate the translation and biological functions of mRNA (21), we explored the relationship between TIL-lncRNAs and TIL-mRNAs by conducting coexpression networks (Figure 3C) and summarized their relationships with risk type in Sankey diagram (Figure 3D). Briefly, AC026401.3, AP000439.2, and LINC02027 were protective factors related to TNFRSF14, HHLA2, and CD276. The other seven lncRNAs were risk factors related to CTLA4, LAG3, TNFRSF18, TNFSF14, TNFRSF14, TNFRSF25, CD70, CD44, DCD1LG2, TNFSF4, CD244, CD27, CD40, CD48, CD86, CTLA4, ICOS, LAG3, PDCD1, TIGIT, and TNFRSF9.
Figure 3 Evaluation of the 10 TIL-related lncRNAs. (A) Expression of the 10 TIL-related lncRNAs. (B) Prognostic value of the 10 TIL-related lncRNAs. (C) Coexpression network between prognostic lncRNAs and TIL-related genes. (D) Sankey diagram showing the association between prognostic TIL-related lncRNAs, TIL-related genes, and risk types.
Then, 10 TIL-lncRNAs were enrolled to construct a TIL-related lncRNA signature with the following formula for the risk score: Risk score = (0.2068 × expression level of AL590094.1) + (−0.1762 × expression level of LINC02027) + (0.0487 × expression level of LINC00460) + (−0.0199 × expression level of AC147651.1) + (0.0604 × expression level of AC026401.3) + (0.2158 × expression level of LINC00944) + (0.0509 × expression level of LINC01615) + (−0.0134 × expression level of AP000439.2) + (0.2292 × expression level of AL162586.1) + (0.2125 × expression level of AC084876.1).
Based on the median cutoff value, patients in the cohort were divided into the high-risk group (n = 253) and the low-risk group (n = 254) (Figure 4A). As shown in Figure 4B, the death probability of high-risk patients was higher than that of low-risk patients. It was also found that the expression levels of AC026401.3, LINC00944, and AC084876.1 were visibly higher, and the expression levels of AP00439.2, AC14765.1, and LINC02027 were downregulated in the high-risk group (Figure 4C).
Figure 4 Analysis of TIL-related lncRNA signature for patients with ccRCC. (A–C) lncRNA predictor-score analysis of 507 ccRCC patients. The horizontal axis represents the 507 patients. Heat map of lncRNA expression level. (D) Survival time of patients between the groups. (E) Areas under the ROC curve. Forest plots for univariate (F) and multivariate (G) Cox regression analysis.
The K–M survival curves showed that patients with a high risk score had significantly poorer overall survival than those with a low risk score (Figure 4D). Then, time-dependent ROC curves were used to assess the predictive performance of the TIL-related lncRNA signature (Figure 4E). The area under the ROC (AUC) was 0.750, indicating that the TIL-lncRNA signature was a reliable prognostic indicator for predicting OS in ccRCC. In addition, univariate Cox regression and multivariate Cox regression analysis were employed to assess the independent prognostic value of the signature with the following factors: risk score and relevant clinical factors (age, gender, grade, clinical stage, tumor stage [T], and metastasis stage [M]). Node stage was excluded as much data were missing. All of the factors except gender were significantly associated with OS in univariate analysis (Figure 4F). Multivariate analysis indicated that risk score was still significantly related to OS, suggesting the TIL-related lncRNA signature could serve as an independent prognostic factor for patients with ccRCC (Figure 4G).
The risk score, age, clinical stage, and pathologic grade were included in the nomogram. As indicated in the nomogram, the risk score had the largest contribution to OS of patients with ccRCC (Figure 5A). The C-index of the nomogram was 0.693. The calibration curve revealed good agreement between the predicted and observed probabilities. All calibration curves of 1-year, 3-year, and 5-year (Figure 5B) OS were close to the 45-degree line.
Figure 5 Construction of a TIL-related lncRNA prognostic model to predict survival. (A) The nomogram of 1-year, 3-year, or 5-year OS. (B) Calibration plots for evaluating the agreement between the predicted and the actual 1-year, 3-year, and 5-year OS for the prognosis model. (C–E) The 1-year, 3-year, and 5-year ROC curves of the nomogram and other clinicopathological parameters. (F) A dynamic nomogram for clinical application to predict survival. The K–M analysis is shown on the left. The corresponding 95% CI is shown on the right.
As shown in Figures 5C–E, the area under curve (AUC) of the nomograms was 0.853, 0.802, and 0.755 for 1-, 3-, and 5-year OS, respectively. These AUC values of the nomograms were greater than those of every single clinical predictor (i.e., age, grade, and stage), indicating an advantage of combining these risk factors for ccRCC prognosis.
For the clinical usability of the model, a dynamic nomogram was created for the prediction of OS probability in patients with ccRCC (Figure 5F), which was convenient and intuitive for individual prognosis prediction based on the personal characteristics of ccRCC patients (https://zhonglab.shinyapps.io/dynnomapp/).
As shown in the Volcano plots (Figure 6A), DEGs were identified with fdr< 0.05 and |logFC| > 0.5. A total of 408 genes were upregulated and 455 genes were downregulated in the low-risk group compared with the high-risk group (Supplementary Table S6). GO enrichment analysis (Figure 6B) indicated that these DEGs were mostly related to the pathways involved in T-cell activation, differentiation, proliferation, co-stimulation, and migration.
Figure 6 Correlation between the signature and TILs in ccRCC tissues. (A) DEGs between the groups by volcano map. (B) GO-BP enrichment analysis. (C) Waterfall plots represent mutation information in each ccRCC patient sample in the high- and low-risk groups. (D) Expression of SETD2 and BAP1. (E) TILs composition analysis in ccRCC of TCGA cohort. (F) Expression of immune markers of lymphocytes of TCGA cohort. (G, H) The expression of AC084876.1 and AC026401.3 in seven kidney samples from ccRCC patients. (H) The expression of CD4, CD8α, PD-1, and FoxP3 in seven kidney samples from ccRCC patients. “B” indicates benign renal tissue adjacent to cancer. “T” indicates tumor tissues of ccRCC. (I) Correlation between the expression of AC084876.1 and FoxP3 in seven ccRCC samples. *P < 0.05; **P < 0.01; ***P < 0.001; ****P < 0.0001.
Somatic mutations often affect the distribution of tumor-infiltrating immune cells in the tumor microenvironment, thereby causing tumor heterogeneity. The results of somatic mutation analysis (Figure 6C) indicated that the top five mutated genes in the high-risk group were VHL (43%), PBRM1 (40%), TTN (26%), SETD2 (18%), and BAP1 (14%). The top five mutated genes in the high-risk group were VHL (41%), PBRM1 (38%), TTN (26%), DST (8%), and MUC16 (8%). Considering the higher mutation frequency of SETD2 and BAP1 in the high-risk group, we measured their expression levels. The expression levels of SETD2 and BAP1 were downregulated in the high-risk group (Figure 6D).
CIBERSORT analysis (Figure 6E) indicated that the abundance of activated CD4+ memory T cells, CD8+ T cells, CD4+ follicular helper T cells (TFH), and regulatory T cells (Tregs) was higher in the high-risk group. A higher abundance of activated NK cells was observed in the low-risk group. As shown in Figure 6F, immune markers CD4, CD8α, CD69, CD25, PD-1, LAG3, CD62L, CCR7, FoxP3, CD56, and CD16 were significantly upregulated in the high-risk group with a decrease in TIM-3.
Considering the above results, we performed an RT-qPCR assay to detect the expression of AC084876.1 and AC026401.3 in seven pairs of matched frozen samples of ccRCC and benign renal tissue adjacent to cancer from ccRCC patients. Moreover, a western blot assay was conducted to measure the expression of immune markers (including CD4, CD8α, PD-1, and FoxP3, Figure 6H). As shown in Figure 6G, the expression of AC084876.1 and AC026401.3 tended to be higher in cancer tissues although the threshold of statistical significance was not reached. The results of the western blot showed a higher trend for all of the immune markers detected in cancer tissues, but without reaching statistical significance (Figure S1A). For the correlation analysis, we found that the expression of AC084876.1 positively correlated with FoxP3 (Figure 6I, 95% CI 0.173 to 0.972, R2 = 0.671, P = 0.024). No statistically significant results were found in other correlation analyses (Figure S1B).
siRNAs were used to knock down the expression of AC084876.1 in the 786-O cell line. The transfection efficiency was measured by RT-qPCR, and we showed that si-1 and si-2 significantly interfered with the expression of AC084876.1 (Figure 7A, P< 0.01). The growth curve suggested that the knockdown of AC084876.1 inhibited the growth of the 786-O cell line (Figure 7B, P< 0.01). The migration ability (Figure 7C, P< 0.01) and invasion ability (Figure 7D, P< 0.01) were attenuated in AC084876.1-downregulated 786-O cell lines.
Figure 7 Experimental validation of AC084876.1. (A) Transfection efficiency of siRNAs targeting AC084876.1 in the 786-O cell line. (B) Growth curve of AC084876.1-downregulated 786-O cell line. (C, D) Knockdown of AC084876.1 inhibited migration and invasion of the 786-O cell line. (E) Eexpression of PD-L1 decreased in the AC084876.1-downregulated 786-O cell line. (F) TGF-β secretion decreased in the AC084876.1-downregulated 786-O cell line. **P < 0.01.
PD-L1 expressed on the surface of tumor cells and TGF-β secreted by tumor cells have been shown to regulate the activity and differentiation of tumor-infiltrating Tregs (22). To further analyze the potential role of AC084876.1 to regulate tumor-infiltrating Tregs, the expression level of PD-L1 and secreted TGF-β level were also examined. We found that the knockdown of AC084876.1 resulted in decreased expression of PD-L1 (Figure 7E, P< 0.01) and decreased secreted TGF-β (Figure 7F, P< 0.01).
ccRCC is the most common pathological subtype of renal carcinoma. Although at an early stage patients can benefit from surgical treatment, advanced patients have finite treatment options (23). Immunotherapy has ignited their hope, but the treatment effectiveness is still limited. The important role of lncRNAs in tumor progression has been gradually explored. To date, several prognostic models based on the immune-related lncRNAs have suggested that lncRNAs are involved in the regulation of immune cell–mediated tumor killing in the tumor microenvironment (TME) (24–26). A large number of immune cells infiltrate the TME, and tumor-infiltrating lymphocytes (TIL) play a key role in the response to immunotherapy (27). Thus, it is worth noting that investigating the potential role of TIL-related lncRNA may identify novel biomarkers for the treatment and prognosis of patients with ccRCC.
In the present study, we established a novel risk coefficient model based on TIL-related lncRNAs through Pearson correlation analysis, univariate Cox regression, Lasso regression, and multivariate Cox regression in 507 ccRCC patients from the TCGA-KIRC dataset. Finally, 10 TIL-related lncRNAs (AL590094.1, LINC02027, LINC00460, AC147651.1, AC026401.3, LINC00944, LINC01615, AP000439.2, AL162586.1, and AC084876.1) were identified and included to construct the prognostic signature. Considering the results of survival analysis, Sankey diagram, and expression analysis of lncRNAs in the high-risk group, AC026401.3, LINC00944, and AC084876.1 were risk factors, whereas AP00439.2, AC14765.1, and LINC02027 were protective factors. Exploring the expression of biomarkers in experiments, we found that the expression trends of AC084876.1 and AC026401.3 in benign and malignant kidney tissues were consistent with the results of bioinformatics analysis, although the differences did not reach the threshold of statistical significance. A larger sample size is needed for further confirmation. The overall survival of ccRCC patients in the TCGA cohort with high expression of AC084876.1 was worse, and AC084876.1 was present as a risk factor in the prognostic model. The experimental validation indicated that the knockdown of AC084876.1 inhibited the growth, migration, and invasion ability of the ccRCC cell line in vitro. Thus, lncRNAs in the signature were tightly related to TILs and reliable to further investigate the potential role of TIL-related lncRNA signature in the progression of ccRCC.
The survival analysis indicated that the survival rate of patients in the low-risk group was significantly higher. Univariate Cox regression and multivariate Cox regression analysis showed that the risk score could be an independent predictor of overall survival in patients with ccRCC. In the nomogram model, risk score contributed the most, and the calibration curves of 1-, 3-, and 5-year survival prediction were close to the ideal value. Also, the AUC values of the 1-, 3-, and 5-year nomograms were greater than those of every single clinical predictor, indicating that the nomogram could be clinically helpful. To make the model applicable for clinical use, we constructed a dynamic nomogram for convenient and intuitive individual prognosis prediction based on the personal characteristics of ccRCC patients.
Consistent with previous studies based on the immunotherapy effectiveness in ccRCC (28, 29), we found that the mutation frequencies of SETD2 and BAP1 were higher and their expression were both downregulated in the high-risk group. SETD2 and BAP1 mutations are associated with metastasis and poor prognosis in ccRCC (30, 31). SETD2 mutations also play an important role in promoting ccRCC progression through cellular autophagy inhibition, DNA repair inhibition, and genomic stability perturbation (32, 33). Mutation of BAP1 may engender genomic instability and promote defects in DNA repair pathways (34). Thus, our novel TIL-related lncRNA signature may contribute to the understanding of the potential mechanism of the progression of ccRCC.
GO-BP analysis of DEGs showed that the immune-related biological processes were mostly involved in T-cell activation, differentiation, proliferation, co-stimulation, and migration. By applying CIBERSORT, we found that the abundance of activated CD4+ memory T cells, CD8+ T cells, TFH, and Tregs was higher in the high-risk group. CD8+ T cells are the main executors of killing tumor cells in the immune system. However, high abundance of CD8+ T cells was not associated with a favorable prognosis in ccRCC (35), and it has been reported that CD8+ T cells did not show functional status as exhausted CD8+ TILs (36). Consistently, our study showed that the exhausted T-cell markers, including PD-1, LAG3, and FoxP3, were upregulated in the high-risk group. Tumor-infiltrating CD8+T cells lose the ability to recognize antigens and activate proliferation under the long-term effect of inhibitory cells and factors, thereby leading to the failure of tumor-killing function (37). Moreover, as an important suppressive immune cell, Tregs can inhibit the proliferation of CD8+T cells by secreting TGF-β, IL-10, and IL-35 (38).
Our results demonstrated that the expression of AC084876.1 positively correlated with FoxP3 in ccRCC. FoxP3 is considered an important biomarker to characterize the Tregs, which are an immunosuppressive subset of CD4+ T cells (39, 40). It is widely accepted that Tregs, the central mediators of immune suppression, are activated by PD-L1 and are induced to differentiate by TGF-β synthesized from tumor cells (22). PD-L1 expressed on tumor cells interacts with PD-1 on tumor-infiltrating lymphocytes, attenuating effector T-cell responses and allowing tumors to escape immune attack (41). In addition, Foxp3 in naive T cells could be induced by TGF-β, thereby promoting Tregs development (42). Interestingly, we found that knockdown of AC084876.1 resulted in decreased expression of PD-L1 and reduced level of secreted TGF-β. These results suggest that AC084876.1 may play an important role in the progression of ccRCC by regulating the activation and differentiation of Tregs. Tumor-infiltrating Tregs are highly activated (43) and exert suppressive activities on effector cells by inducing apoptosis and inhibiting activation/proliferation (44). It has been reported that Tregs promote tumorigenesis and immunosuppression via increasing consumption of IL-2 and upregulating inhibitory immune checkpoints (45). Importantly, the functional enrichment analysis suggested that our signature was related to T-cell activation, differentiation, and proliferation signaling pathways, and Tregs were significantly increased in the high-risk groups. Thus, we believe this may be related to the potential regulatory mechanism between AC084876.1 and tumor-infiltrating Tregs, which is worthy of further investigation.
Despite a number of immune-related lncRNA signatures, to our knowledge, the present study was the first to identify a TIL-related lncRNA signature to predict the prognosis of ccRCC patients. Compared with previous studies, which only established signatures (26, 46), our novel signature was used to establish a nomogram combined with clinical indicators to accurately predict survival. As for the study with nomogram construction (24), the potential mechanism of our signature was verified with experiments. We showed that the signature was mainly related to the function of T cells. Furthermore, we found that AC084876.1 may serve as a potential therapy target associated with the activation and differentiation of tumor-infiltrating Tregs. However, our study was retrospective, and a larger validation cohort is needed to confirm our conclusions. Moreover, the underlying mechanism of the identified lncRNAs in regulating TILs and tumor progression in ccRCC remains to be further explored.
The original contributions presented in the study are included in the article/Supplementary Material. Further inquiries can be directed to the corresponding authors.
The studies involving human participants were reviewed and approved by the Ethics Committee of Guangzhou First People’s Hospital, School of Medicine, South China University of Technology. The patients/participants provided their written informed consent to participate in this study.
WZ, GZ, and ZH supervised the whole project and participated in study design and coordination. YD and KG analyzed data and wrote and revised the paper. YD, ZFT, and YF processed and performed the bioinformatic analysis. SC, YX, JL, RL, ZHT, and YZ collected clinical tissues and performed the experimental assays. YF, JY, and CC checked the revised the figures. All authors contributed to the article and approved the submitted version.
This work was supported by grants from the National Natural Science Foundation of China 82072813 (WZ); Guangdong Basic and Applied Basic Research Foundation 2017A030310100 (KG); Science and Technology Projects in Guangzhou 202201010726 (ZH); Science and Technology Development Fund of Macau SAR 0031/2021/A (WZ); Emergency Key Program of Guangzhou Laboratory EKPG21-04 (WZ); Guangdong Basic and Applied Basic Research Foundation 2020A1515110792, 2022A1515010342 (JY).
The authors declare that the research was conducted in the absence of any commercial or financial relationships that could be construed as a potential conflict of interest.
All claims expressed in this article are solely those of the authors and do not necessarily represent those of their affiliated organizations, or those of the publisher, the editors and the reviewers. Any product that may be evaluated in this article, or claim that may be made by its manufacturer, is not guaranteed or endorsed by the publisher.
The Supplementary Material for this article can be found online at: https://www.frontiersin.org/articles/10.3389/fimmu.2022.1046790/full#supplementary-material
1. Sung H, Ferlay J, Siegel RL, Laversanne M, Soerjomataram I, Jemal A, et al. Global cancer statistics 2020: Globocan estimates of incidence and mortality worldwide for 36 cancers in 185 countries. CA: Cancer J Clin (2021) 71(3):209–49. doi: 10.3322/caac.21660
2. Siegel RL, Miller KD, Fuchs HE, Jemal A. Cancer statistics, 2021. CA Cancer J Clin (2021) 71(1):7–33. doi: 10.3322/caac.21654
3. Jonasch E, Gao J, Rathmell WK. Renal cell carcinoma. BMJ (2014) 349:g4797. doi: 10.1136/bmj.g4797
4. Considine B, Hurwitz ME. Current status and future directions of immunotherapy in renal cell carcinoma. Curr Oncol Rep (2019) 21(4):34. doi: 10.1007/s11912-019-0779-1
5. Grimm MO, Bex A, De Santis M, Ljungberg B, Catto JWF, Rouprêt M, et al. Safe use of immune checkpoint inhibitors in the multidisciplinary management of urological cancer: The European association of urology position in 2019. Eur Urol (2019) 76(3):368–80. doi: 10.1016/j.eururo.2019.05.041
6. Grivennikov SI, Greten FR, Karin M. Immunity, inflammation, and cancer. Cell (2010) 140(6):883–99. doi: 10.1016/j.cell.2010.01.025
7. Vuong L, Kotecha RR, Voss MH, Hakimi AA. Tumor microenvironment dynamics in clear-cell renal cell carcinoma. Cancer Discov (2019) 9(10):1349–57. doi: 10.1158/2159-8290.Cd-19-0499
8. Paijens ST, Vledder A, de Bruyn M, Nijman HW. Tumor-infiltrating lymphocytes in the immunotherapy era. Cell Mol Immunol (2021) 18(4):842–59. doi: 10.1038/s41423-020-00565-9
9. Wu X, Jiang D, Liu H, Lu X, Lv D, Liang L. Cd8(+) T cell-based molecular classification with heterogeneous immunogenomic landscapes and clinical significance of clear cell renal cell carcinoma. Front Immunol (2021) 12:745945. doi: 10.3389/fimmu.2021.745945
10. Qi Y, Xia Y, Lin Z, Qu Y, Qi Y, Chen Y, et al. Tumor-infiltrating Cd39(+)Cd8(+) T cells determine poor prognosis and immune evasion in clear cell renal cell carcinoma patients. Cancer Immunol Immunother (2020) 69(8):1565–76. doi: 10.1007/s00262-020-02563-2
11. Li Y, Wang Z, Jiang W, Zeng H, Liu Z, Lin Z, et al. Tumor-infiltrating Tnfrsf9(+) Cd8(+) T cells define different subsets of clear cell renal cell carcinoma with prognosis and immunotherapeutic response. Oncoimmunology (2020) 9(1):1838141. doi: 10.1080/2162402x.2020.1838141
12. Atianand MK, Fitzgerald KA. Long non-coding rnas and control of gene expression in the immune system. Trends Mol Med (2014) 20(11):623–31. doi: 10.1016/j.molmed.2014.09.002
13. Wei B, Kong W, Mou X, Wang S. Comprehensive analysis of tumor immune infiltration associated with endogenous competitive rna networks in lung adenocarcinoma. Pathol Res Pract (2019) 215(1):159–70. doi: 10.1016/j.prp.2018.10.032
14. Li G, Kryczek I, Nam J, Li X, Li S, Li J, et al. Limit is an immunogenic lncrna in cancer immunity and immunotherapy. Nat Cell Biol (2021) 23(5):526–37. doi: 10.1038/s41556-021-00672-3
15. Ni C, Fang Q-Q, Chen W-Z, Jiang J-X, Jiang Z, Ye J, et al. Breast cancer-derived exosomes transmit lncrna Snhg16 to induce Cd73+Γ Δ1 treg cells. Signal Transduct Target Ther (2020) 5(1):41. doi: 10.1038/s41392-020-0129-7
16. Sun C-C, Zhu W, Li S-J, Hu W, Zhang J, Zhuo Y, et al. Foxc1-mediated Linc00301 facilitates tumor progression and triggers an immune-suppressing microenvironment in non-small cell lung cancer by regulating the Hif1α pathway. Genome Med (2020) 12(1):77. doi: 10.1186/s13073-020-00773-y
17. Yu Y, Zhang W, Li A, Chen Y, Ou Q, He Z, et al. Association of long noncoding rna biomarkers with clinical immune subt ype and prediction of immunotherapy response in patients with cancer. JAMA Netw Open (2020) 3(4):e202149. doi: 10.1001/jamanetworkopen.2020.2149
18. Liu JN, Kong XS, Huang T, Wang R, Li W, Chen QF. Clinical implications of aberrant pd-1 and Ctla4 expression for cancer immunity and prognosis: A pan-cancer study. Front Immunol (2020) 11:2048. doi: 10.3389/fimmu.2020.02048
19. Newman AM, Liu CL, Green MR, Gentles AJ, Feng W, Xu Y, et al. Robust enumeration of cell subsets from tissue expression profiles. Nat Methods (2015) 12(5):453–7. doi: 10.1038/nmeth.3337
20. Liu R, Feng Y, Deng Y, Zou Z, Ye J, Cai Z, et al. A Hif1 alpha-Gpd1 feedforward loop inhibits the progression of renal clear cell carcinoma via mitochondrial function and lipid metabolism. J Exp Clin Cancer Res (2021) 40(1):188. doi: 10.1186/s13046-021-01996-6
21. Khorkova O, Hsiao J, Wahlestedt C. Basic biology and therapeutic implications of lncrna. Advanced Drug Deliv Rev (2015) 87:15–24. doi: 10.1016/j.addr.2015.05.012
22. Ohue Y, Nishikawa H. Regulatory T (Treg) cells in cancer: Can treg cells be a new therapeutic target? Cancer Sci (2019) 110(7):2080–9. doi: 10.1111/cas.14069
23. MacLennan S, Imamura M, Lapitan MC, Omar MI, Lam TB, Hilvano-Cabungcal AM, et al. Systematic review of perioperative and quality-of-Life outcomes following surgical management of localised renal cancer. Eur Urol (2012) 62(6):1097–117. doi: 10.1016/j.eururo.2012.07.028
24. Zhang Z, Tang Y, Liu Y, Zhuang H, Lin E, Xie L, et al. A novel immune-related lncrna-based model for survival prediction in clear cell renal cell carcinoma. J Immunol Res (2021) 2021:9921466. doi: 10.1155/2021/9921466
25. Tang C, Qu G, Xu Y, Yang G, Wang J, Xiang M. An immune-related lncrna risk coefficient model to predict the outcomes in clear cell renal cell carcinoma. Aging (2021) 13(24):26046–62. doi: 10.18632/aging.203797
26. Sun JX, Xia QD, Liu CQ, Xu JZ, Xun Y, Lu JL, et al. Construction of a novel immune-related lncrna pair signature with prognostic significance for kidney clear cell renal cell carcinoma. Dis Markers (2021) 2021:8800358. doi: 10.1155/2021/8800358
27. Chevrier S, Levine JH, Zanotelli VRT, Silina K, Schulz D, Bacac M, et al. An immune atlas of clear cell renal cell carcinoma. Cell (2017) 169(4):736–49.e18. doi: 10.1016/j.cell.2017.04.016
28. Lin E, Zhu P, Ye C, Huang M, Liu X, Tian K, et al. Integrative analysis of the genomic and immune microenvironment characteristics associated with clear cell renal cell carcinoma progression: Implications for prognosis and immunotherapy. Front Immunol (2022) 13:830220. doi: 10.3389/fimmu.2022.830220
29. Braun DA, Hou Y, Bakouny Z, Ficial M, Sant' Angelo M, Forman J, et al. Interplay of somatic alterations and immune infiltration modulates response to pd-1 blockade in advanced clear cell renal cell carcinoma. Nat Med (2020) 26(6):909–18. doi: 10.1038/s41591-020-0839-y
30. Sato Y, Yoshizato T, Shiraishi Y, Maekawa S, Okuno Y, Kamura T, et al. Integrated molecular analysis of clear-cell renal cell carcinoma. Nat Genet (2013) 45(8):860–7. doi: 10.1038/ng.2699
31. Gu YF, Cohn S, Christie A, McKenzie T, Wolff N, Do QN, et al. Modeling renal cell carcinoma in mice: Bap1 and Pbrm1 inactivation drive tumor grade. Cancer Discovery (2017) 7(8):900–17. doi: 10.1158/2159-8290.Cd-17-0292
32. Li L, Miao W, Huang M, Williams P, Wang Y. Integrated genomic and proteomic analyses reveal novel mechanisms of the methyltransferase Setd2 in renal cell carcinoma development. Mol Cell Proteomics (2019) 18(3):437–47. doi: 10.1074/mcp.RA118.000957
33. González-Rodríguez P, Engskog-Vlachos P, Zhang H, Murgoci AN, Zerdes I, Joseph B. Setd2 mutation in renal clear cell carcinoma suppress autophagy Via regulation of Atg12. Cell Death Dis (2020) 11(1):69. doi: 10.1038/s41419-020-2266-x
34. Jonasch E, Walker CL, Rathmell WK. Clear cell renal cell carcinoma ontogeny and mechanisms of lethality. Nat Rev Nephrol (2021) 17(4):245–61. doi: 10.1038/s41581-020-00359-2
35. Fridman WH, Pagès F, Sautès-Fridman C, Galon J. The immune contexture in human tumours: Impact on clinical outcome. Nat Rev Cancer (2012) 12(4):298–306. doi: 10.1038/nrc3245
36. Giraldo NA, Becht E, Pages F, Skliris G, Verkarre V, Vano Y, et al. Orchestration and prognostic significance of immune checkpoints in the microenvironment of primary and metastatic renal cell cancer. Clin Cancer Res (2015) 21(13):3031–40. doi: 10.1158/1078-0432.Ccr-14-2926
37. Deleuze A, Saout J, Dugay F, Peyronnet B, Mathieu R, Verhoest G, et al. Immunotherapy in renal cell carcinoma: The future is now. Int J Mol Sci (2020) 21(7):2532. doi: 10.3390/ijms21072532
38. Nair VS, Elkord E. Immune checkpoint inhibitors in cancer therapy: A focus on T-regulatory cells. Immunol Cell Biol (2018) 96(1):21–33. doi: 10.1111/imcb.1003
39. Hori S, Nomura T, Sakaguchi S. Control of regulatory T cell development by the transcription factor Foxp3. Sci (New York NY) (2003) 299(5609):1057–61. doi: 10.1126/science.1079490
40. Khattri R, Cox T, Yasayko SA, Ramsdell F. An essential role for scurfin in Cd4+Cd25+ T regulatory cells. Nat Immunol (2003) 4(4):337–42. doi: 10.1038/ni909
41. Brahmer JR, Tykodi SS, Chow LQM, Hwu W-J, Topalian SL, Hwu P, et al. Safety and activity of anti-Pd-L1 antibody in patients with advanced c ancer. N Engl J Med (2012) 366(26):2455–65. doi: 10.1056/NEJMoa1200694
42. Kanamori M, Nakatsukasa H, Okada M, Lu Q, Yoshimura A. Induced regulatory T cells: Their development, stability, and applicat ions. Trends Immunol (2016) 37(11):803–11. doi: 10.1016/j.it.2016.08.012
43. Shitara K, Nishikawa H. Regulatory T cells: A potential target in cancer immunotherapy. Ann New York Acad Sci (2018) 1417(1):104–15. doi: 10.1111/nyas.13625
44. Saleh R, Elkord E. Acquired resistance to cancer immunotherapy: Role of tumor-mediated immunosuppression. Semin Cancer Biol (2020) 65:13–27. doi: 10.1016/j.semcancer.2019.07.017
45. Saleh R, Elkord E. Foxp3(+) T regulatory cells in cancer: Prognostic biomarkers and therapeutic targets. Cancer Lett (2020) 490:174–85. doi: 10.1016/j.canlet.2020.07.022
Keywords: tumor-infiltrating lymphocytes, long noncoding RNA, bioinformatics, clear cell renal cell carcinoma, prognostic
Citation: Deng Y, Guo K, Tang Z, Feng Y, Cai S, Ye J, Xi Y, Li J, Liu R, Cai C, Tan Z, Zhang Y, Han Z, Zeng G and Zhong W (2022) Identification and experimental validation of a tumor-infiltrating lymphocytes–related long noncoding RNA signature for prognosis of clear cell renal cell carcinoma. Front. Immunol. 13:1046790. doi: 10.3389/fimmu.2022.1046790
Received: 17 September 2022; Accepted: 07 November 2022;
Published: 24 November 2022.
Edited by:
Zong Sheng Guo, University at Buffalo, United StatesReviewed by:
Cong Liu, First Affiliated Hospital of Nanchang University, ChinaCopyright © 2022 Deng, Guo, Tang, Feng, Cai, Ye, Xi, Li, Liu, Cai, Tan, Zhang, Han, Zeng and Zhong. This is an open-access article distributed under the terms of the Creative Commons Attribution License (CC BY). The use, distribution or reproduction in other forums is permitted, provided the original author(s) and the copyright owner(s) are credited and that the original publication in this journal is cited, in accordance with accepted academic practice. No use, distribution or reproduction is permitted which does not comply with these terms.
*Correspondence: Weide Zhong, emhvbmd3ZDIwMDlAbGl2ZS5jbg==; Guohua Zeng, Z3pneXpnaEBzaW5hLnZpcC5jb20=; Zhaodong Han, aGFuemhhb2RvbmdAMjFjbi5jb20=
†These authors have contributed equally to this work
Disclaimer: All claims expressed in this article are solely those of the authors and do not necessarily represent those of their affiliated organizations, or those of the publisher, the editors and the reviewers. Any product that may be evaluated in this article or claim that may be made by its manufacturer is not guaranteed or endorsed by the publisher.
Research integrity at Frontiers
Learn more about the work of our research integrity team to safeguard the quality of each article we publish.