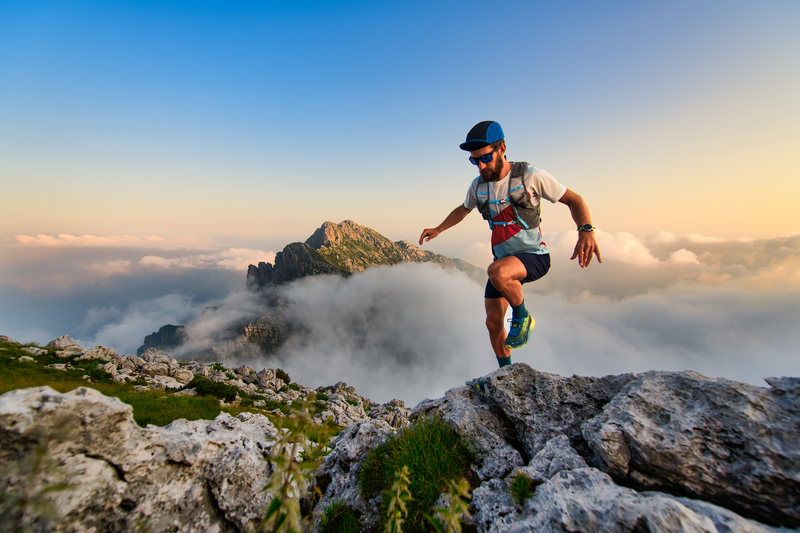
95% of researchers rate our articles as excellent or good
Learn more about the work of our research integrity team to safeguard the quality of each article we publish.
Find out more
MINI REVIEW article
Front. Immunol. , 27 October 2022
Sec. Cancer Immunity and Immunotherapy
Volume 13 - 2022 | https://doi.org/10.3389/fimmu.2022.1045957
This article is part of the Research Topic Community Series in Novel Insights into Immunotherapy Targeting Tumor Microenvironment in Ovarian Cancer, volume I View all 13 articles
Immune checkpoint blockade (ICB) therapy has evoked a prominent shift in anticancer therapy. Durable clinical antitumor activity to ICB has been observed in patients with ovarian cancer (OC). However, only a subset of patients derive clinical benefit, and immune-related adverse events (irAEs) caused by ICB therapy can lead to permanent tissue damage and even fatal consequences. It is thus urgent to develop predictive biomarkers to optimize patient outcomes and minimize toxicity risk. Herein, we review current predictive and prognostic biomarkers for checkpoint immunotherapy in OC and highlight emerging biomarkers to guide treatment with ICB. The prevalent biomarkers, such as PD-L1 expression status, tumor-infiltrating lymphocytes, mutational burden, and immune gene signatures, are further discussed. We provide a state-of-the-art survey on prognostic and predictive biomarkers for checkpoint immunotherapy and offer valuable information for guiding precision immunotherapy
Immune checkpoint blockade therapies (ICBs) can circumvent tumor-mediated immune suppression and reinvigorate antitumor immune responses, in contrast with conventional therapeutic strategies that exert direct cytotoxicity against tumor cells (1, 2). Immune checkpoint inhibitors (ICIs) that target the programmed cell death protein-1 (PD-1)/programmed death receptor ligand-1 (PD-L1) axis or cytotoxic T lymphocyte antigen 4 (CTLA4) have achieved impressive success against various cancer types (3). ICIs have achieved remarkable clinical activity with durable disease control across multiple advanced tumors (4). Accordingly, several ICIs have been approved by the United States Food and Drug Administration (FDA) for patients with malignancies, including melanoma, lung cancer, triple-negative breast cancer (TNBC), colorectal cancer, gastric cancer, renal cell cancer, head and neck squamous cell cancer, bladder cancer, lymphoma and so on (5). Albeit substantial advancements in clinical therapy, only a minority of patients receiving ICIs derive benefits. In addition, ICB therapy is significantly restricted by the occurrence of immune-related adverse events (irAEs), resulting from immune hyperactivation and subsequent immune homeostasis disturbance. Severe adverse events can lead to permanent disorders and can be lethal in some cases (6). Therefore, there is intense interest in developing predictive and prognostic biomarkers for ICI therapy to better understand the benefits and risks driven by ICB and effectively select patients.
Manipulating the immune environment with ICIs is an attractive therapeutic approach for antitumor therapy in ovarian cancer (OC) (Figure 1). There has been considerable progress in utilizing ICB therapy for OC over the past few years (Table 1; Supplementary Table S1). However, there is still confusion regarding patient selection and the choice of therapeutic regimen for patients with OC, underscoring the need for effective biomarkers to predict response and remission. In this review, we attempt to summarize published original research and clinical trials involving biomarker assessment in OC receiving ICI therapy and discuss ongoing efforts to develop predictive biomarkers of responsiveness and outcomes.
Figure 1 Biomarker development for immune-checkpoint inhibitor therapy in ovarian cancer. Key elements in biomarker development for immune checkpoint inhibitors therapy are briefly described, including PD-L1 expression, genomics alterations, immune cell infiltration, and transcriptomic signatures.
Direct measurement of PD-L1 expression is a logical biomarker for predicting response to anti-PD-1/PD-L1 therapies. PD-L1 immunohistochemistry (IHC) assay is now FDA-approved as a companion diagnostic biomarker to select patients most likely to benefit from ICI treatment for multiple cancer types, such as non-small cell lung cancer(NSCLC), metastatic TNBC, and melanoma (5).
The predictive value of PD-L1 expression was assessed in OC patients treated with anti-PD-1/PD-L1 antibodies (Table 1). KEYNOTE-100 (NCT02674061) investigated the clinical activity of pembrolizumab in patients with recurrent advanced OC and introduced PD-L1 stain score as a predictive biomarker, in which patients with higher PD-L1 expression (combined positive score≥10) had an increased overall response rate (ORR) and prolonged overall survival (OS) with pembrolizumab (8). More recently, Sanborn et al. evaluated the efficacy and safety of varlilumab plus nivolumab in patients with advanced solid tumors (10). Significantly, an absolute increase of 5% or more in tumor PD-L1 expression induced by treatment tended to improve progression-free survival (PFS) in OC (7.4 months vs. 3.5 months, p=0.07), whereas baseline pretreatment PD-L1 expression was not associated with ORR (10). Prespecified biomarker analysis in the JAVELIN-200 trial revealed a trend for prolonged PFS with the addition of avelumab to pegylated liposomal doxorubicin (PLD) compared with PLD alone among OC patients with PD-L1-positive tumors (12). Nevertheless, several trials yielded inconsistent or even contradictory results regarding the role of PD-L1 expression as a marker for predicting response to ICB and clinical outcomes in OC. Liu et al. (15) obtained the opposite results in evaluating the predictive and prognostic value of PD-L1 expression in recurrent OC patients receiving nivolumab and bevacizumab. Even patients with PD-L1-negative tumors (10/22) had higher therapeutic activity than those with PD-L1-positive expression (2/14) (15). In addition, several studies have shown that the expression of PD-L1 was not predictive of ICI outcome and prognosis in OC patients (36, 38–43). Potential reasons for these paradoxical results include the inability to accurately reflect PD-L1 status due to PD-L1 expression transiency and heterogeneity, differences in the disease status of patients, the poor uniformity between various detection assays, and the lack of standardized criteria and thresholds for assessing positivity (3, 44, 45). Therefore, PD-L1 status is likely insufficient to determine the suitability of ICI therapy for OC patients. Further refinement of the use of PD-L1 expression status as a robust biomarker for checkpoint immunotherapy is warranted.
TIICs can serve as an index to monitor the tumor microenvironment (TME) and play an increasingly important role in the immune response against cancer (46). Therefore, TIICs have also been speculated to be surrogate biomarkers for ICB immunotherapy in many types of cancer, including OC (Table 1). A comprehensive analysis of immune cells in patients with epithelial ovarian cancer (EOC) revealed a positive correlation between the infiltration of immune cells and the clinical outcome of EOC (16). The density of tumor-infiltrating lymphocytes (TILs), specifically CD8+ T cells, is a solid positive prognostic indicator for multiple cancer types regardless of ICI therapy. In fact, CD8 expression in tumors was predictive of clinical benefit with avelumab plus PLD treatment in OC (12). Of note, patients with dual PD-L1-positive and CD8-positive tumors seemed to benefit more from combination treatment than subgroups defined by only one of these biomarkers (12). Another potential predictor of ICI response is tumor-infiltrating mast cells (TIMs) within a tumor (Table 1). In high-grade plasmacytoid ovarian cancer (HGSOC), stromal TIMs (sTIMs) abundance was negatively associated with the ICB response (18). Remarkably, tumors with low sTIMs had enhanced effector functions of CD8+ T cells (18). This finding was corroborated in short-term HGSOC organoids. The effector molecules (GZMB and IFN-γ) on CD8+ T cells were marginally increased in organoids derived from low sTIMs tumors, compared to organoids from high sTIMs tumors (18). Overall, the abundance of sTIMs predicts a dismal prognosis in HGSOC patients treated with anti-PD-1 therapy.
Except for the spatial position and density of TIICs, their phenotype and activation status also impact the clinical benefit of ICIs (3). The immune-inflamed phenotype is usually accompanied by the expression of PD-L1 on infiltrating immune cells and tumor cells, which is associated with a better response to ICI therapy (3). In a trial investigating combination regimens with anti-PD-L1 antibody in women’s cancers, a trend toward a positive association of treatment response with the degree of PD-L1-positive TILs was observed (39). In contrast, melanoma patients with PD-L1-positive TILs had a significantly worse prognosis than those with PD-L1-negative TILs (P = 0.008) (47). Further investigations are needed to determine whether PD-L1-positive TILs are suitable to serve as predictors of ICB effectiveness. In addition, other non-neoplastic cells in the TME are also non-negligible, which are probably of biological significance. Therefore, increased awareness of the role of these distinct TME compartments is needed for comprehensive biomarker development to predict ICB response and prognosis.
Tumor development and progression generally occur along with the acquisition and accumulation of mutations (45). Neoantigens generated by mutations may lead to T-cell infiltration, thereby better response to immunotherapy (48). In fact, several studies have attempted to evaluate somatic mutations as biomarkers for predicting ICB response in OC (Table 1). ARID1A mutation or loss was associated with immune microenvironmental factors in clear cell ovarian cancer (CCC), suggesting that ARID1A status has potential as a biomarker to guide decisions concerning patient selection for ICB therapy in CCC (20). The phase I/II trial (NCT02657889) reported two novel biomarkers for the combination of poly (adenosine diphosphate-ribose) polymerase (PARP) and PD-1 inhibitors in the treatment of platinum-resistant OC (17). Mutational signature 3 reflected homologous recombination deficiency (HRD) status, and positive immune score (IS) was a surrogate of interferon-primed exhausted CD8+ T cells in TME. Specifically, the presence of one or both of the above alternative markers was associated with significantly prolonged PFS (HR = 0.32), while concurrent absence showed no response to PARP/PD-1 inhibitors(ORR= 0%) (17).
Another metric, known as tumor mutation burden (TMB), is a strong predictor of ICB efficacy. Unfortunately, its predictive performance in OC is disappointing. No significant correlation was found between TMB and immunotherapy response in recurrent OC (21). Furthermore, BRCA1/2 mutations and HRD status also did not predict the clinical benefit of ICI in heavily pretreated patients with OC (21). Notably, additional exploratory analyses identified the fraction of genome altered (FGA) as a promising biomarker of response to ICI in OC, which can characterize global copy number alterations. High FGA was significantly associated with improved OS (HR = 0.49; log-rank P = 0.01) and PFS (HR = 0.54; log-rank P = 0.014) after ICI therapy in OC (21). The optimal cutoff for defining high vs. low FGA is unclear; therefore, the predictive capacity of FGA warrants further validation.
TMB was also explored in the phase I/II trial (NCT03029598), which evaluated pembrolizumab and carboplatin for recurrent or refractory ovarian, fallopian tube, or primary peritoneal cancer (19). Stratification by the ratio of peripheral CD8+PD1+Ki67+ T cells to tumor burden at baseline yielded a significant survival advantage. Patients with a low ratio (<0.0375) had a median OS of only 8.72 months, while those with a high ratio (≥0.0375) had a significantly longer median OS of 18.37 months (p=0.0099). However, no significant survival difference was observed when using CD8+PD1+Ki67+ T cell (p=0.53) or tumor burden alone (p=0.24) as stratification criteria (19). Overall, TMB alone does not clearly discriminate responders from non-responders in OC patients treated with ICIs.
Gene expression analysis can uncover global tumor and microenvironment features, providing promise for predicting the clinical benefit of checkpoint inhibitor strategies. Multiplex characterization of the TME and gene expression signatures have been proposed as effective methods to dissect the immune contexture and cancer cell-intrinsic features. According to TME information derived from transcriptome data of OC, Li et al. (23) established immune cell infiltration (ICI) scores and an immune-related gene prognostic model to predict the clinical benefits of OC patients undergoing immunotherapy. Signal transducer and activator of transcription 1 (STAT1) has been demonstrated to be associated with TME. A recent study found that STAT1 expression was positively correlated with PD-L1 expression and had the potential to predict the response to ICB in patients with EOC (24). Integrins are transmembrane receptors that mediate the connection between cells and their external environment (49–51).
Several immune-related gene signatures have been confirmed to predict the immunotherapeutic response in OC. The TGF-β regulated signaling pathway was noted to contribute to immunotherapy resistance in OC (27). A significant negative correlation between the TGF-β score and ICI-PFS was observed in OC, with an ICI-PFS of 16.6 months in the low TGF-β score group compared to 2.65 months in the high TGF-β score group (p = 0.0012). As the most common RNA modification, N6-methyladenosine (m6A) plays a key role in epigenetics (52). A risk model based on m6A-related targets has an excellent clinical prognostic stratification effect in advanced OC. Importantly, the high- and low-risk groups divided by this model have significant differences in TME contexture, suggesting that this model may be able to predict immunotherapy response in OC (29).
Chemokines have essential roles in modulating immune homeostasis and inflammatory responses (53). Accumulating findings suggest that chemokines can influence cancer cell proliferation, invasion, angiogenesis, and therapy resistance by recruiting immune cells and modulating the TME (54, 55). The prognostic and predictive values of the CXC chemokine family have been addressed in the setting of OC, including CXCL9, CXCL11, and CXCL13 (Table 1). Tumors with high CXCL9 expression had significantly prolonged OS, implying the feasibility of CXCL9 expression as a novel prognostic marker for high-grade serous ovarian cancer (HGSC) (30). Similarly, Fan et al. (33) found a significant positive correlation between the expression of CXCL13, FCRLA, PLA2G2D, and MS4A1 and a better prognosis of OC. Meanwhile, these potential therapeutic genes could reflect OC immune status and allow better predictions of who will respond to ICI. Furthermore, Yang etal. (32) examined the therapeutic effects of CXCL13 and PD-1 blockade in human HGSC tumors and mouse models. They found that CXCL13 can augment the efficacy of PD-1 checkpoint blockade in HGSC by shaping the antitumor microenvironment. CXCL13 can facilitate CXCR5+CD8+ T-cell recruitment to tertiary lymphoid structures. Furthermore, the combination of CXCL13, CD8, and CXCR5 was confirmed as a potential prognostic indicator or response biomarker for ICB therapy in patients with HGSC. CXCL11 expression has been demonstrated as a biomarker for predicting the response to anti-PD-1/PD-L1 therapy in a clinical trial of OC (31). In OC patients with HRD, tumors with high CXCL11 expression had a more robust immune response to PD-L1 blockade than those with low CXCL11 expression. Notably, the tumor-infiltrating immunophenotype and neoantigen burden were significantly elevated in CXCL11-high tumors.
In addition, several genes have been demonstrated to be associated with immunotherapy efficacy and prognosis in OC (Table 1). For example, Capping Actin Protein, Gelsolin-Like (CAPG) (25) and Layilin (LAYN) (26) appeared to be indicators of ICI outcome. Tumors with high CAPG or LAYN expression showed a significantly shorter survival time. In a study, the predictive significance of NAD+ metabolism-related genes (NMRGs) on immunotherapy response in patients with OC was examined. The high-risk score obtained by the NMRG-based model was also associated with a poorer prognosis (28). Apolipoprotein B mRNA editing enzyme catalytic subunit 3A (APOBEC3A) has been recognized as an indicator of genomic instability and may aid in predicting the prognosis and response to immunotherapy in OC (22).
In recent years, there has been great interest in developing blood-derived predictive biomarkers of ICI response, owing to its convenient and non-invasive sampling (56). Cancer antigen 125 (CA-125) is an important tumor biomarker specific to OC (57); thus, several studies have carried out exploratory research on the predictive role of CA-125 in OC patients treated with ICIs (Table 1). A phase II trial (NCT02608684), designed for evaluating the combination of pembrolizumab and chemotherapy in platinum-resistant OC, found CA-125 to be a reliable marker that reflected response and progression (42). In a retrospective study of EOC patients treated with ICI (35), the magnitude of increase in CA-125 levels within the first 12 weeks of treatment was significantly smaller in patients with clinical benefit than in those without benefit, suggesting a possible predictive role for the degree of CA-125 increase. In a phase 1b study of avelumab in patients with heavily pretreated OC, 12 patients with an objective response, of whom all 7 patients evaluable for CA-125 levels showed decreased CA-125 concentrations (36).
Dynamic monitoring of circulating tumor DNA (ctDNA) in plasma samples offers a meaningful direction for biomarker identification for immunotherapy in OC patients (37). A satisfying finding was that ctDNA concentration was related to clinical response and benefit, although the effect sizes were modest (37). Additionally, in a phase II trial of olaparib combined with durvalumab for OC, increased IFNγ production and elevated VEGFR3 levels in blood samples showed positive and negative correlations with PFS, respectively (p=0.023; p=0.017) (34).
The clinical trials and original research outlined above have shown that classical biomarkers derived from the TME and tumor intrinsic features, such as PD-L1 expression, TMB, TIICs, and transcriptomic signatures, were correlated with ICI response and outcome in OC. Although these findings are intriguing, the implementation of these classical biomarkers has been hampered by inconsistencies and limitations. Promisingly, new biomarkers often designed as substitutes or complements to conventional biomarkers are constantly emerging, such as microbiome, tertiary lymphoid structures (TLSs), and tumor-associated antigens (TAAs). The potential of microbiome and its derived metabolome as biomarkers for predicting the efficacy of immunotherapy has been validated in melanoma (58), lung cancer (59), hepatobiliary cancer (60), and colorectal cancer (61). Several studies have demonstrated that clinical outcomes of immunotherapy for solid tumors are strongly correlated with the presence of TLSs, suggesting that TLSs may be a valid predictive indicator in the future (62). Elevated levels of carcinoembryonic antigen (CEA) have also been reported to negatively correlate with the prognosis of resected NSCLC patients receiving ICB therapy (63). More recently, a comprehensive predictive model for ICB response was developed across 16 different cancer types, which included the features of peripheral blood such as platelets, neutrophil-to-lymphocyte ratio, albumin, and hemoglobin (HGB) (64). These studies provide new perspectives to develop new biomarkers for OC patients treated with ICB therapy. The predictive values of these biomarkers in OC remain to be validated in routine clinical settings.
As evidenced by the fact that a single biomarker is often insufficient to determine the suitability of ICI therapy for OC patients, the combination of different biomarkers may be more valuable in predicting the clinical prognosis and therapeutic response to immunotherapy. Indeed, it has been proposed that the incorporation of dynamic and static biomarkers could improve decision-making to design tailored immunotherapy strategies. Moreover, the development of relevant biomarkers for the toxicity prediction of ICB therapy has become a research hotspot and is expected to offer effective ways to uncouple immunotherapy toxicity from its antitumor activity.
YX and XH: conceptualization and writing-original draft preparation. FZ: visualization. YX, FZ, HW, JJ and XH: writing-review and editing. XH and JJ: supervision and funding acquisition. All authors have read and agree to the published version of the manuscript.
This work was supported by (1) National Natural Science Foundation of China (No. 82172634 and 81902792); (2) Key Program of the Science and Technology Bureau of Sichuan (No. 2021YFSY0007); (3) 1.3.5 project for disciplines of excellence, West China Hospital, Sichuan University (No. ZYYC20013).
The authors declare that the research was conducted in the absence of any commercial or financial relationships that could be construed as a potential conflict of interest.
All claims expressed in this article are solely those of the authors and do not necessarily represent those of their affiliated organizations, or those of the publisher, the editors and the reviewers. Any product that may be evaluated in this article, or claim that may be made by its manufacturer, is not guaranteed or endorsed by the publisher.
The Supplementary Material for this article can be found online at: https://www.frontiersin.org/articles/10.3389/fimmu.2022.1045957/full#supplementary-material
1. Ott PA, Hodi FS, Robert C. CTLA-4 and PD-1/PD-L1 blockade: New immunotherapeutic modalities with durable clinical benefit in melanoma patients. Clin Cancer Res (2013) 19:5300–9. doi: 10.1158/1078-0432.CCR-13-0143
2. Nishino M, Ramaiya NH, Hatabu H, Hodi FS. Monitoring immune-checkpoint blockade: Response evaluation and biomarker development. Nat Rev Clin Oncol (2017) 14:655–68. doi: 10.1038/nrclinonc.2017.88
3. Havel JJ, Chowell D, Chan TA. The evolving landscape of biomarkers for checkpoint inhibitor immunotherapy. Nat Rev Cancer (2019) 19:133–50. doi: 10.1038/s41568-019-0116-x
4. Gong J, Chehrazi-Raffle A, Reddi S, Salgia R. Development of PD-1 and PD-L1 inhibitors as a form of cancer immunotherapy: A comprehensive review of registration trials and future considerations. J Immunother Cancer (2018) 6:1–18. doi: 10.1186/s40425-018-0316-z
5. Twomey JD, Zhang B. Cancer immunotherapy update: FDA-approved checkpoint inhibitors and companion diagnostics. AAPS J (2021) 23:1–11. doi: 10.1208/s12248-021-00574-0
6. Postow MA, Sidlow R, Hellmann MD. Immune-related adverse events associated with immune checkpoint blockade. N Engl J Med (2018) 378:158–68. doi: 10.1056/NEJMra1703481
7. Matulonis UA, Shapira R, Santin A, Lisyanskaya AS, Pignata S, Vergote I, et al. Antitumor activity and safety of pembrolizumab in patients with advanced recurrent ovarian cancer: results from the phase II KEYNOTE-100 study. Ann Oncol (2019) 30:1080–7. doi: 10.1093/annonc/mdz135
8. Matulonis UA, Shapira R, Santin A, Lisyanskaya AS, Pignata S, Vergote I, et al. Final results from the KEYNOTE-100 trial of pembrolizumab in patients with advanced recurrent ovarian cancer. J Clin Oncol (2020) 38:6005–5. doi: 10.1200/JCO.2020.38.15_suppl.6005
9. Nishio S, Matsumoto K, Takehara K, Kawamura N, Hasegawa K, Takeshima N, et al. Pembrolizumab monotherapy in Japanese patients with advanced ovarian cancer: Subgroup analysis from the KEYNOTE-100. Cancer Sci (2020) 111:1324–32. doi: 10.1111/cas.14340
10. Sanborn RE, Pishvaian MJ, Callahan MK, Weise A, Sikic BI, Rahma O, et al. Safety, tolerability and efficacy of agonist anti-CD27 antibody (varlilumab) administered in combination with anti-PD-1 (nivolumab) in advanced solid tumors. J Immunother Cancer (2022) 10:e005147. doi: 10.1136/jitc-2022-005147
11. Sanborn RE, Pishvaian MJ, Callahan MK, Weise AM, Sikic BI, Rahma OE, et al. Anti-CD27 agonist antibody varlilumab (varli) with nivolumab (nivo) for colorectal (CRC) and ovarian (OVA) cancer: Phase (Ph) 1/2 clinical trial results. J Clin Oncol (2018) 36:3001. doi: 10.1200/JCO.2018.36.15_suppl.3001
12. Pujade-Lauraine E, Fujiwara K, Ledermann JA, Oza AM, Kristeleit R, Ray-Coquard IL, et al. Avelumab alone or in combination with chemotherapy versus chemotherapy alone in platinum-resistant or platinum-refractory ovarian cancer (JAVELIN ovarian 200): an open-label, three-arm, randomised, phase 3 study. Lancet Oncol (2021) 22:1034–46. doi: 10.1016/S1470-2045(21)00216-3
13. Lakhani NJ, Patnaik A, Liao JB, Moroney JW, Miller DS, Fleming GF, et al. A phase Ib study of the anti-CD47 antibody magrolimab with the PD-L1 inhibitor avelumab (A) in solid tumor (ST) and ovarian cancer (OC) patients. J Clin Oncol (2020) 38:18–18. doi: 10.1200/JCO.2020.38.5_suppl.18
14. Lee EK, Xiong N, Cheng SC, Barry WT, Penson RT, Konstantinopoulos Lee Elizabeth PA K, et al. Combined pembrolizumab and pegylated liposomal doxorubicin in platinum resistant ovarian cancer: a phase 2 clinical trial. Gynecol Oncol (2020) 159:72–8. doi: 10.1016/j.ygyno.2020.07.028
15. Liu JF, Herold C, Gray KP, Penson RT, Horowitz N, Konstantinopoulos PA, et al. Assessment of combined nivolumab and bevacizumab in relapsed ovarian cancer: A phase 2 clinical trial. JAMA Oncol (2020) 5:1731–8. doi: 10.1001/jamaoncol.2019.3343
16. Liu S-Y, Zhu R-H, Wang Z-T, Tan W, Zhang L, Wang Y-Q, et al. Landscape of immune microenvironment in epithelial ovarian cancer and establishing risk model by machine learning. J Oncol (2020) 2021:1731–8. doi: 10.1155/2021/5523749
17. Färkkilä A, Gulhan DC, Casado J, Jacobson CA, Nguyen H, Kochupurakkal B, et al. Immunogenomic profiling determines responses to combined PARP and PD-1 inhibition in ovarian cancer. Nat Commun (2020) 11:1459. doi: 10.1038/s41467-020-15315-8
18. Cao K, Zhang G, Zhang X, Yang M, Wang Y, He M, et al. Stromal infiltrating mast cells identify immunoevasive subtype high-grade serous ovarian cancer with poor prognosis and inferior immunotherapeutic response. Oncoimmunology (2021) 10:1969075. doi: 10.1080/2162402X.2021.1969075
19. Liao JB, Gwin WR, Urban RR, Hitchcock-Bernhardt KM, Coveler AL, Higgins DM, et al. Pembrolizumab with low-dose carboplatin for recurrent platinum-resistant ovarian, fallopian tube, and primary peritoneal cancer: survival and immune correlates. J Immunother Cancer (2021) 9:e003122. doi: 10.1136/jitc-2021-003122
20. Kuroda Y, Chiyoda T, Kawaida M, Nakamura K, Aimono E, Yoshimura T, et al. ARID1A mutation/ARID1A loss is associated with a high immunogenic profile in clear cell ovarian cancer. Gynecol Oncol (2021) 162:679–85. doi: 10.1016/j.ygyno.2021.07.005
21. Liu YL, Selenica P, Zhou Q, Iasonos A, Callahan M, Feit NZ, et al. BRCA mutations, homologous DNA repair deficiency, tumor mutational burden, and response to immune checkpoint inhibition in recurrent ovarian cancer. JCO Precis Oncol (2020) 4:665–79. doi: 10.1200/PO.20.00069
22. Xu F, Liu T, Zhou Z, Zou C, Xu S. Comprehensive analyses identify APOBEC3A as a genomic instability-associated immune prognostic biomarker in ovarian cancer. Front Immunol (2021) 12. doi: 10.3389/fimmu.2021.749369
23. Li X, Liang W, Zhao H, Jin Z, Shi G, Xie W, et al. Immune cell infiltration landscape of ovarian cancer to identify prognosis and immunotherapy-related genes to aid immunotherapy. Front Cell Dev Biol (2021) 9. doi: 10.3389/fcell.2021.749157
24. Liu F, Liu J, Zhang J, Shi J, Gui L, Xu G. Expression of STAT1 is positively correlated with PD-L1 in human ovarian cancer. Cancer Biol Ther (2020) 21:963–71. doi: 10.1080/15384047.2020.1824479
25. Jiang S, Yang Y, Zhang Y, Ye Q, Song J, Zheng M, et al. Overexpression of CAPG is associated with poor prognosis and immunosuppressive cell infiltration in ovarian cancer. Dis Markers (2022) 2022:9719671. doi: 10.1155/2022/9719671
26. Li L, Ma H, Song C. LAYN acts as a prognostic biomarker in ovarian cancer by engaging T cell exclusion and dysfunction. Research Square (2022). doi: 10.21203/rs.3.rs-1943215/v1
27. Ni Y, Soliman A, Joehlin-Price A, Rose PG, Vlad A, Edwards RP, et al. High TGF-β signature predicts immunotherapy resistance in gynecologic cancer patients treated with immune checkpoint inhibition. NPJ Precis Oncol (2021) 5:1–11. doi: 10.1038/s41698-021-00242-8
28. Lin L, Chen L, Xie Z, Chen J, Li L, Lin A. Identification of NAD+ metabolism-derived gene signatures in ovarian cancer prognosis and immunotherapy. Front Genet (2022) 13. doi: 10.3389/fgene.2022.905238
29. Tan W, Liu S, Deng Z, Dai F, Yuan M, Hu W, et al. Gene signature of m6A-related targets to predict prognosis and immunotherapy response in ovarian cancer. J Cancer Res Clin Oncol (2022) 1–16. doi: 10.1007/s00432-022-04162-3
30. Seitz S, Dreyer TF, Stange C, Steiger K, Bräuer R, Scheutz L, et al. CXCL9 inhibits tumour growth and drives anti-PD-L1 therapy in ovarian cancer. Br J Cancer (2022) 126:1470–80. doi: 10.1038/s41416-022-01763-0
31. Shi Z, Zhao Q, Lv B, Qu X, Han X, Wang H, et al. Identification of biomarkers complementary to homologous recombination deficiency for improving the clinical outcome of ovarian serous cystadenocarcinoma. Clin Trans Med (2021) 11:e399. doi: 10.1002/ctm2.399
32. Yang M, Lu J, Zhang G, Wang Y, He M, Xu Q, et al. CXCL13 shapes immunoactive tumor microenvironment and enhances the efficacy of PD-1 checkpoint blockade in high-grade serous ovarian cancer. J Immunother Cancer (2021) 9:e001136. doi: 10.1136/jitc-2020-001136
33. Fan L, Lei H, Lin Y, Zhou Z, Shu G, Yan Z, et al. Identification of a gene set correlated with immune status in ovarian cancer by transcriptome-wide data mining. Front Mol Biosci (2021) 8. doi: 10.3389/fmolb.2021.670666
34. Lampert EJ, Zimmer A, Padget M, Cimino-Mathews A, Nair JR, Liu Y, et al. Combination of PARP inhibitor olaparib, and PD-L1 inhibitor durvalumab, in recurrent ovarian cancer: a proof-of-Concept phase II StudyPhase II study of olaparib with durvalumab in ovarian cancer. Clin Cancer Res (2020) 26:4268–79. doi: 10.1158/1078-0432.CCR-20-0056
35. Boland JL, Zhou Q, Iasonos AE, O'cearbhaill RE, Konner J, Callahan M, et al. Utility of serum CA-125 monitoring in patients with ovarian cancer undergoing immune checkpoint inhibitor therapy. Gynecol Oncol (2020) 158:303–8. doi: 10.1016/j.ygyno.2020.04.710
36. Disis ML, Taylor MH, Kelly K, Beck JT, Gordon M, Moore KM, et al. Efficacy and safety of avelumab for patients with recurrent or refractory ovarian cancer: Phase 1b results from the JAVELIN solid tumor trial. JAMA Oncol (2019) 5:393–401. doi: 10.1001/jamaoncol.2018.6258
37. Bratman SV, Yang S, Iafolla MA, Liu Z, Hansen AR, Bedard PL, et al. Personalized circulating tumor DNA analysis as a predictive biomarker in solid tumor patients treated with pembrolizumab. Nat Cancer (2020) 1:873–81. doi: 10.1038/s43018-020-0096-5
38. Disis ML, Patel MR, Pant S, Hamilton EP, Lockhart AC, Kelly K, et al. Avelumab (MSB0010718C; anti-PD-L1) in patients with recurrent/refractory ovarian cancer from the JAVELIN solid tumor phase ib trial: Safety and clinical activity. Am Soc Clin Oncol (2016) 34:5533. doi: 10.1200/JCO.2016.34.15_suppl.5533
39. Lee JM, Cimino-Mathews A, Peer CJ, Zimmer A, Lipkowitz S, Annunziata CM, et al. Safety and clinical activity of the programmed death-ligand 1 inhibitor durvalumab in combination with poly (ADP-ribose) polymerase inhibitor olaparib or vascular endothelial growth factor receptor 1-3 inhibitor cediranib in women's cancers: A dose-escalation, phase I study. J Clin Oncol (2017) 35:2193–202. doi: 10.1200/JCO.2016.72.1340
40. Zamarin D, Burger RA, Sill MW, Powell DJ Jr., Lankes HA, Feldman MD, et al. Randomized phase II trial of nivolumab versus nivolumab and ipilimumab for recurrent or persistent ovarian cancer: An NRG oncology study. J Clin Oncol (2020) 38:1814–23. doi: 10.1200/JCO.19.02059
41. Mirza M, Henriksen J, Maenpaa J, Christensen RD, Waldstroem M, Tandaric L, et al. 1195 results of NSGO-OV-UMB1/ENGOT-OV30 study: A phase II study of durvalumab and oleclumab in patients with relapsed ovarian cancer (OC). BMJ Specialist Journals (2021) 31:A376. doi: 10.1136/ijgc-2021-ESGO.668
42. Walsh CS, Kamrava M, Rogatko A, Kim S, Li A, Cass I, et al. Phase II trial of cisplatin, gemcitabine and pembrolizumab for platinum-resistant ovarian cancer. PloS One (2021) 16:e0252665. doi: 10.1371/journal.pone.0252665
43. Zsiros E, Lynam S, Attwood KM, Wang C, Chilakapati S, Gomez EC, et al. Efficacy and safety of pembrolizumab in combination with bevacizumab and oral metronomic cyclophosphamide in the treatment of recurrent ovarian cancer: A phase 2 nonrandomized clinical trial. JAMA Oncol (2021) 7:78–85. doi: 10.1001/jamaoncol.2020.5945
44. Gibney GT, Weiner LM, Atkins MB. Predictive biomarkers for checkpoint inhibitor-based immunotherapy. Lancet Oncol (2016) 17:e542–51. doi: 10.1016/S1470-2045(16)30406-5
45. Shen H, Yang ES, Conry M, Fiveash J, Contreras C, Bonner JA, et al. Predictive biomarkers for immune checkpoint blockade and opportunities for combination therapies. Genes Dis (2019) 6:232–46. doi: 10.1016/j.gendis.2019.06.006
46. Santoiemma PP, Powell DJ Jr. Tumor infiltrating lymphocytes in ovarian cancer. Cancer Biol Ther (2015) 16:807–20. doi: 10.1080/15384047.2015.1040960
47. Ren M, Dai B, Kong YY, Lv JJ, Cai X. PD-L1 expression in tumour-infiltrating lymphocytes is a poor prognostic factor for primary acral melanoma patients. Histopathology (2018) 73:386–96. doi: 10.1111/his.13527
48. Chic N, Brasó-Maristany F, Prat A. Biomarkers of immunotherapy response in breast cancer beyond PD-L1. Breast Cancer Res Treat (2021) 191:39–49. doi: 10.1007/s10549-021-06421-2
49. Ganguly KK, Pal S, Moulik S, Chatterjee A. Integrins and metastasis. Cell Adh Migr (2013) 7:251–61. doi: 10.4161/cam.23840
50. Bianconi D, Unseld M, Prager GW. Integrins in the spotlight of cancer. Int J Mol Sci (2016) 17:2037. doi: 10.3390/ijms17122037
51. Alday-Parejo B, Stupp R, Rüegg C. Are integrins still practicable targets for anticancer therapy? Cancers (Basel) (2019) 11:978. doi: 10.3390/cancers11070978
52. Xu T, He B, Sun H, Xiong M, Nie J, Wang S, et al. Novel insights into the interaction between N6-methyladenosine modification and circular RNA. Mol Therapy-Nucleic Acids (2022) 27:824–37. doi: 10.1016/j.omtn.2022.01.007
53. Tanegashima K, Suzuki K, Nakayama Y, Tsuji K, Shigenaga A, Otaka A, et al. CXCL14 is a natural inhibitor of the CXCL12–CXCR4 signaling axis. FEBS Lett (2013) 587:1731–5. doi: 10.1016/j.febslet.2013.04.046
54. Milligan G, Kostenis E. Heterotrimeric G-proteins: a short history. Br J Pharmacol (2006) 147:S46–55. doi: 10.1038/sj.bjp.0706405
55. Strieter RM, Burdick MD, Mestas J, Gomperts B, Keane MP, Belperio JA. Cancer CXC chemokine networks and tumour angiogenesis. Eur J Cancer (2006) 42:768–78. doi: 10.1016/j.ejca.2006.01.006
56. Di Capua D, Bracken-Clarke D, Ronan K, Baird A-M, Finn S. The liquid biopsy for lung cancer: state of the art, limitations and future developments. Cancers (2021) 13:3923. doi: 10.3390/cancers13163923
57. Razmi N, Hasanzadeh M. Current advancement on diagnosis of ovarian cancer using biosensing of CA 125 biomarker: Analytical approaches. TrAC Trends Analytical Chem (2018) 108:1–12. doi: 10.1016/j.trac.2018.08.017
58. Temraz S, Nassar F, Nasr R, Charafeddine M, Mukherji D, Shamseddine A. Gut microbiome: a promising biomarker for immunotherapy in colorectal cancer. Int J Mol Sci (2019) 20:4155. doi: 10.3390/ijms20174155
59. Malczewski AB, Navarro S, Coward JI, Ketheesan N. Microbiome-derived metabolome as a potential predictor of response to cancer immunotherapy. J Immunother Cancer (2020) 8:e001383. doi: 10.1136/jitc-2020-001383
60. Mao J, Wang D, Long J, Yang X, Lin J, Song Y, et al. Gut microbiome is associated with the clinical response to anti-PD-1 based immunotherapy in hepatobiliary cancers. J Immunother Cancer (2021) 9:e003334. doi: 10.1136/jitc-2021-003334
61. Shoji F, Yamashita T, Kinoshita F, Takamori S, Fujishita T, Toyozawa R, et al. Artificial intelligence-derived gut microbiome as a predictive biomarker for therapeutic response to immunotherapy in lung cancer: protocol for a multicentre, prospective, observational study. BMJ Open (2022) 12:e061674. doi: 10.1136/bmjopen-2022-061674
62. Trüb M, Zippelius A. Tertiary lymphoid structures as a predictive biomarker of response to cancer immunotherapies. Front Immunol (2021) 12:674565. doi: 10.3389/fimmu.2021.674565
63. Clevers MR, Kastelijn EA, Peters BJ, Kelder H, Schramel FM. Evaluation of serum biomarker CEA and Ca-125 as immunotherapy response predictors in metastatic non-small cell lung cancer. Anticancer Res (2021) 41:869–76. doi: 10.21873/anticanres.14839
Keywords: ovarian cancer, immune checkpoint blockade, biomarker, immunotherapy response, prognosis
Citation: Xu Y, Zuo F, Wang H, Jing J and He X (2022) The current landscape of predictive and prognostic biomarkers for immune checkpoint blockade in ovarian cancer. Front. Immunol. 13:1045957. doi: 10.3389/fimmu.2022.1045957
Received: 16 September 2022; Accepted: 14 October 2022;
Published: 27 October 2022.
Edited by:
Qi Zhao, University of Macau, ChinaReviewed by:
Bihui Cao, The Second Affiliated Hospital of Guangzhou Medical University, ChinaCopyright © 2022 Xu, Zuo, Wang, Jing and He. This is an open-access article distributed under the terms of the Creative Commons Attribution License (CC BY). The use, distribution or reproduction in other forums is permitted, provided the original author(s) and the copyright owner(s) are credited and that the original publication in this journal is cited, in accordance with accepted academic practice. No use, distribution or reproduction is permitted which does not comply with these terms.
*Correspondence: Jing Jing, ampfemN5QHZpcC4xNjMuY29t; Xiujing He, aGV4aXVqaW5nQHNjdS5lZHUuY24=
Disclaimer: All claims expressed in this article are solely those of the authors and do not necessarily represent those of their affiliated organizations, or those of the publisher, the editors and the reviewers. Any product that may be evaluated in this article or claim that may be made by its manufacturer is not guaranteed or endorsed by the publisher.
Research integrity at Frontiers
Learn more about the work of our research integrity team to safeguard the quality of each article we publish.