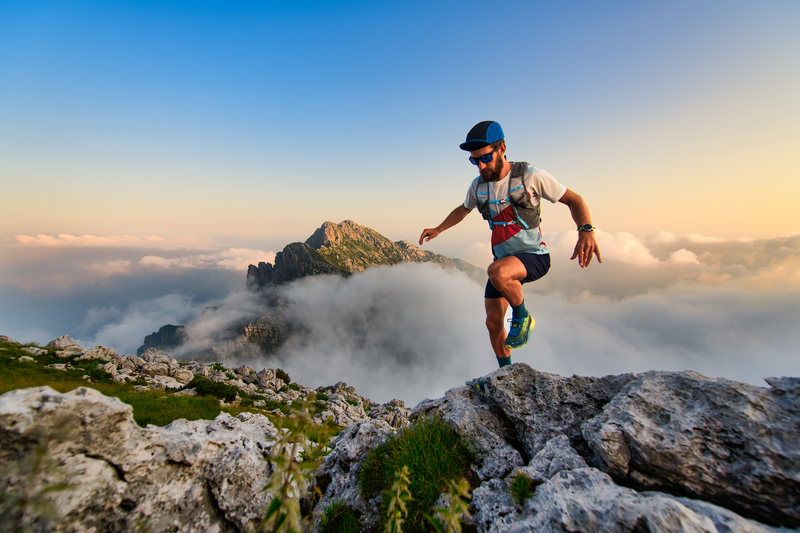
95% of researchers rate our articles as excellent or good
Learn more about the work of our research integrity team to safeguard the quality of each article we publish.
Find out more
MINI REVIEW article
Front. Immunol. , 25 November 2022
Sec. Inflammation
Volume 13 - 2022 | https://doi.org/10.3389/fimmu.2022.1038744
This article is part of the Research Topic Cellular and Molecular Communication Networks within the Cutaneous Immune System View all 11 articles
Single-cell RNA sequencing (scRNA-seq) is a novel technology that characterizes molecular heterogeneity at the single-cell level. With the development of more automated, sensitive, and cost-effective single-cell isolation methods, the sensitivity and efficiency of scRNA-seq have improved. Technological advances in single-cell analysis provide a deeper understanding of the biological diversity of cells present in tissues, including inflamed skin. New subsets of cells have been discovered among common inflammatory skin diseases, such as atopic dermatitis (AD) and psoriasis. ScRNA-seq technology has also been used to analyze immune cell distribution and cell-cell communication, shedding new light on the complex interplay of components involved in disease responses. Moreover, scRNA-seq may be a promising tool in precision medicine because of its ability to define cell subsets with potential treatment targets and to characterize cell-specific responses to drugs or other stimuli. In this review, we briefly summarize the progress in the development of scRNA-seq technologies and discuss the latest scRNA-seq-related findings and future trends in AD and psoriasis. We also discuss the limitations and technical problems associated with current scRNA-seq technology.
Inflammatory skin diseases are induced by skin barrier disorders and dysregulation of innate and adaptive immunity. Atopic dermatitis (AD) and psoriasis are two of the most common chronic inflammatory skin diseases (1, 2). The Global Burden of Disease study showed that AD is the 15th most common non-fatal disease and the skin disorder with the highest disease burden, with a prevalence of 15% to 20% among children and up to 10% in adults (3). Approximately 125 million people worldwide are estimated to have psoriasis (4). Although genetic, immune dysregulation, and environmental factors play important roles in the pathogenesis of AD and psoriasis (1, 2), the detailed mechanisms of AD and psoriasis remain unclear. In recent years, specific immune component targeted therapies have been reported in AD and psoriasis, with substantially positive effects. However, some patients do not respond to these treatments, have a secondary failure, or relapse after drug withdrawal; thus, the underlying mechanisms regarding the treatment of these diseases remain unclear.
Bulk RNA sequencing is an indispensable tool for analyzing transcriptional variations, which determine the average gene expression among pooled populations of cells and reported as single data. However, tissues consist of multiple cell types in various states; hence, the results of such a technique can be misleading. Newly developed single-cell RNA sequencing (scRNA-seq) technologies facilitate the analysis of transcriptional activity at the single-cell level (5). scRNA-seq facilitates the assessment of cellular heterogeneity, identification of new or rare cell populations, and clarification of cellular transition states at a high resolution. In addition, Thus, organ- or tissue-specific transcriptomic characteristics of keratinocytes (KCs), fibroblasts, endothelial cells, and immune cells hosted within or infiltrated after inflammation can be assessed to elucidate the function of cell heterogeneity in AD and psoriasis.
scRNA-seq is a powerful tool for providing precision and detail to individual cells (6). The workflow of scRNA-seq usually includes sample preparation, single-cell capture, reverse transcription of full-length mRNA, cDNA amplification, preparation of a sequencing library, high-throughput sequencing, and bioinformatics analyses. Single-cell isolation and amplification of cDNA are the main steps in various single-cell sequencing strategies.
Methods of traditional single-cell isolation have eventually developed after many optimizations. Micromanipulation is a classic technique for manually capturing under a microscope (7, 8). It can accurately select single cells under microscopic observation and is suitable for analyzing a limited number of cells (9). However, this method is time-consuming, has low throughput, and may cause cellular injury due to mechanical shearing (10). Laser capture microdissection (LCM) is another approach for obtaining single cells from solid tissue. In this technique, a laser beam is used to capture the cells of interest from the tissue specimen quickly and accurately, attaching these cells to a thin and transparent film (11). Here, the spatial positional information of the target cells is retained (12); however, in addition to being laborious and inconvenient, there is a risk of destroying the integrity of cells and damaging cellular RNA (13), which could impact subsequent analyses. Fluorescence-activated cell sorting (FACS) is a specialized type of flow cytometry that sorts large numbers of cells based on cell surface markers and physicochemical properties and completes quantitative analyses quickly (14). Time consumption and low throughput limit the use of these traditional technologies. To circumvent this, scRNA-seq technology platforms have been rapidly developed based on the application of microfluidic and single-cell identification technologies.
Novel single-cell capture methods based on microfluidics include integrated fluidic circuits (IFCs), droplets, microwells, traps, and the SlipChip (15). Popular platforms have recently enabled droplet-based scRNA-seq, which sorts cells into aqueous compartments in a lipid suspension (16). Using this system, the cell capture rate of a single sample can be as high as 65%, and 80,000 cells can be simultaneously isolated and amplified in minutes (17). Split pool ligation-based transcriptome sequencing (SPLiT-Seq) uses combinatorial barcodes to label individual cells (18), which is expected to decrease operational costs and does not involve microfluidic devices. The development of these technologies has led to the widespread use of scRNA-seq. The advantages and disadvantages of these single-cell isolation methods are summarized in Table 1.
Single-cell bioinformatics analysis, typically involves fundamental analytical procedures such as quality control, normalization, dimensionality reduction, differential expression gene analysis, visualization, clustering, and cell type annotation. Thus, this analysis can obtain the basic single-cell landscape of specific disease. Some advanced analyses, such as trajectory and cell-cell communications, can help us further capture specific disease-related cells, genes, functional pathways, and cell-cell interactions. scRNA-seq technology has been applied in skin cancer and autoimmune skin diseases. For example, ferroptosis-related genes and resident memory CD8+ T cells in regional lymph nodes have been identified to predict the prognosis of melanoma using scRNA-seq analyses (19, 20). Moreover, patterns of dedifferentiation in melanoma are predictive of the response to immune checkpoint inhibitor therapy (21). Type 1 cytokine signaling plays a central role in vitiligo, and Treg cells inhibit disease progression in non-lesional skin (22). A unique cluster of CXCL13+ T cells identified via scRNA-seq appears to promote B- cell responses within the inflamed skin of patients with systemic sclerosis (23). These data provide critical insights into the pathogenesis of melanoma, vitiligo, and systemic sclerosis, respectively.
Atopic dermatitis (AD) is one of the most common inflammatory skin diseases. The prevalence of AD has been reported up to 20% among children and 10% among adults (24), and the causes are complex. Genetic susceptibility, a dysfunctional epidermal barrier, skin microbiome abnormalities, and type-2 immune dysregulation predominantly play a role in the pathogenesis of AD (2). The endotype is the molecular mechanism underlying the disease phenotypes (25, 26). Aside from the presence of IgE that can distinguish between intrinsic and extrinsic AD, AD is characterized by a highly diverse endotype repertoire, including the dysregulation of Th1/Th2/Th17/Th22 cells and impaired epidermal barrier integrity (27).
In addition to the unclear specific pathogenic mechanisms of AD, the treatment options for this disease vary. Targeted therapies for specific AD endotypes, such as those directed against Th2/Tc2, and Th17 cells and general anti-inflammatory agents, have been proved by the FDA or are currently in different phases of clinical trials (28). Additionally, addressing the unsatisfactory efficacy of current therapies and identifying biomarkers that will improve therapy selection for biological agents and small-molecule drugs should be the focus for AD.
RNA sequencing and gene microarray analysis of skin biopsy specimens have provided insights into AD pathogenesis (29, 30). scRNA-seq was performed on lesional and non-lesional samples from patients with AD and skin from healthy individuals. It was found that COL6A5+COL18A1 + fibroblast, which express the cytokines CCL2 and CCL19, were a novel cell subpopulation unique to AD lesional skin. A dendritic cell population that expresses the CCL19 receptor CCR7 is also unique to AD lesions (31). Prx1+ fibroblasts overexpressing the eosinophilic chemokine CCL11 may also contribute to the pathogenesis of AD by dysregulating IKKβ/NF-κB signaling; hence, targeting CCL11 upregulation in Prx1+ fibroblasts may be a way to treat AD-like skin diseases (32). Whether these subpopulations of fibroblasts recruit T cells and other inflammatory cells into the local lesions or play a role in the initiation, maintenance, and regression phases needs further research.
Meanwhile, myeloid dendritic cells (DCs), including inflammatory dendritic epidermal cells, form the most expanded immune cell population in AD lesions (33). It has been demonstrated that unique inflammatory fibroblasts may interact with immune cells, such as DCs expressing CCR7, to regulate type 2 inflammation. Moreover, innate lymphoid cells (ILC), especially ILC2, have been implicated in AD pathogenesis. ILC2s are activated by various tissue-derived factors and exhibit different functions in both the steady- state and inflammation. The number of ILC2s in lesional skin biopsies from patients with AD was significantly higher than in healthy individuals (34, 35). Skin ILC2s were further sub-classified into skin-resident and circulating ILC2s through scRNA-seq in a transgenic mouse line expressing skin-specific IL-33 expression. Here, these transgenic mice showed ILC2- dependent atopic dermatitis-like skin inflammation (36). Patients with AD have a high percentage of ILC2s in their peripheral blood that respond better to IL-4/13 inhibitors, such as dupilumab (37). Furthermore, ILC2s in lesional AD skin have been shown to be biologically heterogeneous and are involved in the IL-33 signaling pathway. ILC2s are flexible and co-express typical genes, either type 2 or type 3/17 immunity markers, within individual cells (38). It is well known that type3/17 immunity is associated with the development of psoriasis. However, whether the plasticity of ILC2s is responsible for psoriasis-related dermatitis remains unclear.
AD typically starts in infancy or early childhood, showing spontaneous regression after puberty in a subset of patients while waxing and waning for life in many others. However, the factors that modify the natural course of spontaneous remission remain to be elucidated. The overall cell composition of patients with spontaneously healed AD was comparable to that of healthy individuals. Compared to healthy controls, melanocytes exhibit many differentially expressed genes in all cell types in spontaneously healed atopic dermatitis. Specifically, the expression of the potential anti-inflammatory, maker PLA2G7 (Lipoprotein-associated phospholipase A2 or “platelet-activating factor acetylhydrolase) is increased. Regulatory markers are also upregulated in conventional T-cells (39). Moreover, skin-resident memory T cells showed the greatest transcriptional dysregulation in AD (40), which may be responsible for the recurrence of the disease. KCs also play an important role in the pathogenesis of AD. Epidermal proliferation and chemokines (CCL2 and CCL27) were significantly upregulated in the KCs of lesional AD. Such KCs were found to be enriched during epidermis development and immune responses (31).
Based on previous studies, innate immune cells (ILC2s and DCs), fibroblasts, and KCs, as well as their interplay and interactions, play a role in AD development. Melanocytes and skin-resident memory T cells may contribute to the specific regulatory microenvironment in the spontaneous remission and recurrence of AD, respectively.
Psoriasis is also a common, chronic inflammatory skin disease, and its incidence in ethnic groups and countries is significantly different (41). The pathogenesis of psoriasis is complex and multifactorial, involving genetic, immune, and environmental factors. The IL-23/Th17 pathway is thought to be the predominant pathway governing the progression and development of psoriasis (42). Biologics that target IL-17/IL-17 receptor and IL-23 have shown significant clinical efficacy in patients with psoriasis (43, 44). Cutaneous type 17 T-cells showed markedly different g transcriptome profiles depending on various cytokines, including IL-17A, IL-17F, and IL-10 (45). CD8+ T cells are increased in abundance within psoriatic lesions (46) and are found to produce inflammatory cytokines, such as IL-17, at sites of the active phase of psoriasis (47). However, CD8+ T cells are phenotypically heterogeneous and have distinct functional properties with cytotoxic and cytokine-producing features (48). Two pathogenic cytotoxic type 17 T-cell (Tc17) subsets of CD8+ T cells were identified in psoriatic skin from lesional skin biopsies of 11 patients with psoriasis and five healthy control individuals via single-cell transcriptomics. CXCL13- expressing Tc17 cells appear to be specific to psoriatic lesions and are associated with disease severity (49). Up to 30% patients with psoriasis may develop psoriatic arthritis (PsA), presenting with peripheral arthritis, enthesitis, and (or) dactylitis (50). The expansion of memory CD8+ T cells in the joints of PsA patients was significantly higher than that in their peripheral blood. CD8+ T cells have also been previously reported in the synovial fluid of PsA patients (51). single-cell sequencing showed that in the synovial fluid, CD8+T cells that express CXCR3, a tissue-homing receptor, are increased in abundance and that the expression of its ligands (CXCL9 and CXCL10) were elevated, providing molecular insight into the cellular immune mechanism of PsA (52). Compared to healthy, patients with psoriasis are characterized by Treg expansion and CD8+ T cell exhaustion. Moreover, differentially expressed genes in skin-resident memory T cells have been recently reported to discriminate psoriasis vulgaris from AD. Other T cell subsets, such as dysfunctional T cells that regulate and express NR4A1, are also involved in psoriasis (53).
In addition to adaptive immunity, innate immunity plays an important role in the pathogenesis of psoriasis. Cutaneous antigen-presenting cells (APCs) are divided into three groups: Langerhans cells in the epidermis, classical dendritic cell type 1(cDC1) and cDC2 in the dermis, and macrophages (54). A new subset of inflammatory DCs expressing CD5-CD163+CD14+ (DC3) was identified in human blood (55). CD14+ DC3 cells expressing genes related to IL-17 and neutrophil activation signaling were enriched in psoriatic lesions, which were considered potential promoters of inflammation in psoriasis. Higher proportions of macrophage-expressing genes related to inflammatory chemokines and cytokines (CXCL8 and CXCL2) were found in psoriatic lesions compared to non-lesional skin (56). ILC3s have been reported in human and mouse psoriatic lesions (57, 58). The response to therapeutic compounds has decreased the number of ILC3 cells (59). Fate mapping analysis suggested that ILC3-like cells may arise from quiescent-like cells and ILC2s, highlighting the flexibility of skin ILC responses and driving the pathological remodeling process (60).
Even though immune cell infiltration plays a fundamental role in cutaneous inflammation, KCs can also influence the inflammatory microenvironment (61). scRNA-seq analysis showed that aberrant inflammatory transcription of A20 in KCs in psoriasis is related to the IL-17 and tumor necrosis factor-α(TNF-α) signaling pathways (62), suggesting a potential targeted therapy. Hence, Th17 cells, CD8+ T cells, DC3 cells, macrophages, ILCs and KCs play an important role in the development of psoriasis. And Tc17 cells, a subtype of CD8+ T cells, are associated with disease activity.
Since 2009, the first conceptual and technical breakthrough of the single-cell RNA sequencing method was made by Tang et al. (8). An increasing number of improved scRNA-seq technologies were developed to introduce essential modifications and improvements in sample collection, single-cell capture, barcoded reverse transcription, cDNA amplification, library preparation, sequencing and streamlined bioinformatics analysis. Freshly tissues, high cost of per sample and scRNA-seq data analysis still remain challenge. Subsequent studies are expected to explore fixed tissue sample and to downregulate costs. And automatic sc-RNA-seq data analysis pipelines and visualization platforms are expected to be available in the future.
Most of studies related in this review prepare the libraries and sequence depending on 10X Genomics platform. These findings highlight KCs, fibroblasts, and different types of immune cells in mechanisms for coping with the different stages of AD and psoriasis. scRNA-seq offers a novel method to identify the receptors, ligands, and cytokines expressed in each cell type to further highlight intercellular communication in the skin microenvironment. However, the detailed mechanisms driving the pathogenicity of these cells and the relationship between them require further study. And the clinical characteristics of AD and psoriasis varied. Therefore, more specific studies are necessary to elucidate the characteristics of AD and psoriasis based on the differences in the stages and subtypes of these diseases. Patients with clinical characteristics of both AD and psoriasis have been described as having psoriasis dermatitis, typically found in children (63). In addition to the co-existence of both AD and psoriasis, disease co-occurrence but alternating flare-ups or co-occurrence at different life stages may also be observed (64). The overlap condition not only presented in children but also in adult patients. With the increasing application of biologics agents, the psoriasiform reaction during dupilumab therapy has been reported in AD, and an eczematous reaction to anti-interleukin (IL)-17 treatment has been reported in psoriasis (65, 66). However, the pathophysiology should be further clarified. Moreover, it will be beneficial to analyze cell lineage trajectories and patient-specific cell heterogeneity using scRNA-seq data.
Analyzing the non-coding RNAs (ncRNAs) with scRNA-seq and combining proteomics with epigenomics will get closer to a ture global examination of single cell. In addition, the analysis of minimally invasive or non-invasive samples, such as blood or urine specimens, may also hold promise in diagnosis process and treatment response prediction. Considering the rapid development of sequencing methods, scRNA-seq can be expected to enter the clinics soon and facilitate personalized therapeutic decisions for patients with inflammatory skin diseases.
Although scRNA-seq can identify cell type-specific transcriptional regulation and cellular heterogeneity, its limitations can be challenged. Firstly, high-throughput single-cell analysis requires cell dissociation, quality control, and are largely tend to examine freshly isolated cells. More research will use such technology to explore cryopreserved and fixed tissue samples. And the preparation of single-cell suspensions destroys spatial information of tissues. In addition, researchers have mostly focused on protein-coding RNA. An increasing number of studies have indicated that ncRNAs have important roles in cell function and specialization (67–69). Even though it has been neglected in previous scRNA-seq studies, nanopore sequencing and Smart-seq-total technology may also address current gaps in the technology (70, 71). Bioinformatics analysis of DEGs in scRNA-seq strongly depends on the cell count in each identified cluster, whether the transcriptional changes obtained via scRNA-seq are specific biological findings, or a biased subset clustering data that is prone to misinterpretation (72, 73). The development of scRNA-seq technology has raised a wide range of computational and analytical challenges. Even though several methods have now been designed to efficiently perform upstream (quality control and normalization) and downstream (cell-, gene- and pathway-level) analyses of scRNA-seq data (74), there are limited guidelines on how to define quality control standards, remove technical artifacts, and interpret results. Deep-learning based methods, such as machine learning, may also provide more benefits than traditional statistical models in dealing with high-dimensional data.
In recent years, transcriptomics has made a great leap from bulk RNA-seq, which measures the average gene expression, to analyzing gene expression data in individual cells. This mini-review summarizes and discusses the applications of scRNA-seq in AD and psoriasis (Figure 1). Single-cell RNA sequencing has provided new insights into inflammatory skin disease heterogeneity, revealed complex interactions between cell types, and allowed a more comprehensive understanding of inflammatory skin disease initiation, progression, and regression. New insights are crucial for developing targeted and innovative therapeutic strategies, to advance precision medicine for inflammatory skin diseases. Although some limitations remain, scRNA-seq will pave the way for personalized medicine once solving the challenges.
Figure 1 Application of scRNA-seq in AD and PS. Single-cell RNA sequencing (scRNA-seq) is especially useful in the detection of rare cell populations, identifying cell-to-cell interactions, reconstructing skin cell trajectories, spatial transcriptomic mapping of skin (75), and developing more precision medicine tools for the better prediction of patient-specific drug responses. The future trends involved cell-to-cell communication, skin cell trajectories, spatial transcriptomic mapping of skin, and precision medicines in these diseases. AD, atopic dermatitis; PS, psoriasis; FBs, fibroblasts; KCs, keratinocytes; MCs, melanocytes; Th1, T helper 1; Th17, T helper 17; Tc17, expressing IL-17 cytotoxic CD+8 T cell; Trm, skin-resident memory T cell; Treg, regulatory T cell; DC, dendritic cell; Mreg DC, mature dendritic cell enriched in immunoregulatory molecules; ILC, innate lymphoid cell; Mø, macrophage; CCL, CC chemokine ligand; CXCL, C-X-C motif ligand; GRN, gene regulatory network; t-SNE, t-distributed stochastic neighbor embedding.
DX wrote the manuscript. YW, YX, and WL contributed to the revision of the manuscript. All authors approved the final submitted version.
1·3·5 project for disciplines of excellence, West China Hospital, Sichuan University.
The authors declare that the research was conducted in the absence of any commercial or financial relationships that could be construed as a potential conflict of interest.
All claims expressed in this article are solely those of the authors and do not necessarily represent those of their affiliated organizations, or those of the publisher, the editors and the reviewers. Any product that may be evaluated in this article, or claim that may be made by its manufacturer, is not guaranteed or endorsed by the publisher.
1. Boehncke W-H, Schön MP. Psoriasis. Lancet (2015) 386(9997):983–94. doi: 10.1016/s0140-6736(14)61909-7
2. Langan SM, Irvine AD, Weidinger S. Atopic dermatitis. Lancet (2020) 396(10247):345–60. doi: 10.1016/s0140-6736(20)31286-1
3. Laughter MR, Maymone MBC, Mashayekhi S, Arents BWM, Karimkhani C, Langan SM, et al. The global burden of atopic dermatitis: Lessons from the global burden of disease study 1990-2017. Br J Dermatol (2021) 184(2):304–9. doi: 10.1111/bjd.19580
4. World Health Organization. Global report on psoriasis: World health organization (2016). Available at: https://apps.who.int/iris/handle/10665/204417 (Accessed February 13, 2020).
5. Shapiro E, Biezuner T, Linnarsson S. Single-cell sequencing-based technologies will revolutionize whole-organism science. Nat Rev Genet (2013) 14(9):618–30. doi: 10.1038/nrg3542
6. Qi R, Ma A, Ma Q, Zou Q. Clustering and classification methods for single-cell rna-sequencing data. Brief Bioinform (2020) 21(4):1196–208. doi: 10.1093/bib/bbz062
7. Morris J, Singh JM, Eberwine JH. Transcriptome analysis of single cells. J Visualized Exp: JoVE (2011) 50:2634. doi: 10.3791/2634
8. Tang F, Barbacioru C, Wang Y, Nordman E, Lee C, Xu N, et al. Mrna-seq whole-transcriptome analysis of a single cell. Nat Methods (2009) 6(5):377–82. doi: 10.1038/nmeth.1315
9. Guo F, Li L, Li J, Wu X, Hu B, Zhu P, et al. Single-cell multi-omics sequencing of mouse early embryos and embryonic stem cells. Cell Res (2017) 27(8):967–88. doi: 10.1038/cr.2017.82
10. Gross A, Schoendube J, Zimmermann S, Steeb M, Zengerle R, Koltay P. Technologies for single-cell isolation. Int J Mol Sci (2015) 16(8):16897–919. doi: 10.3390/ijms160816897
11. Kamme F, Salunga R, Yu J, Tran DT, Zhu J, Luo L, et al. Single-cell microarray analysis in hippocampus Ca1: Demonstration and validation of cellular heterogeneity. J Neurosci: Off J Soc Neurosci (2003) 23(9):3607–15. doi: 10.1523/jneurosci.23-09-03607.2003
12. Chen J, Suo S, Tam PP, Han JJ, Peng G, Jing N. Spatial transcriptomic analysis of cryosectioned tissue samples with geo-seq. Nat Protoc (2017) 12(3):566–80. doi: 10.1038/nprot.2017.003
13. Keays KM, Owens GP, Ritchie AM, Gilden DH, Burgoon MP. Laser capture microdissection and single-cell rt-pcr without rna purification. J Immunol Methods (2005) 302(1-2):90–8. doi: 10.1016/j.jim.2005.04.018
14. Adan A, Alizada G, Kiraz Y, Baran Y, Nalbant A. Flow cytometry: Basic principles and applications. Crit Rev Biotechnol (2017) 37(2):163–76. doi: 10.3109/07388551.2015.1128876
15. García Alonso D, Yu M, Qu H, Ma L, Shen F. Advances in microfluidics-based technologies for single cell culture. Adv Biosyst (2019) 3(11):e1900003. doi: 10.1002/adbi.201900003
16. Macosko EZ, Basu A, Satija R, Nemesh J, Shekhar K, Goldman M, et al. Highly parallel genome-wide expression profiling of individual cells using nanoliter droplets. Cell (2015) 161(5):1202–14. doi: 10.1016/j.cell.2015.05.002
17. Valihrach L, Androvic P, Kubista M. Platforms for single-cell collection and analysis. Int J Mol Sci (2018) 19(3):807. doi: 10.3390/ijms19030807
18. Rosenberg AB, Roco CM, Muscat RA, Kuchina A, Sample P, Yao Z, et al. Single-cell profiling of the developing mouse brain and spinal cord with split-pool barcoding. Sci (New York NY) (2018) 360(6385):176–82. doi: 10.1126/science.aam8999
19. Liu Y, Shou Y, Zhu R, Qiu Z, Zhang Q, Xu J. Construction and validation of a ferroptosis-related prognostic signature for melanoma based on single-cell rna sequencing. Front Cell Dev Biol (2022) 10:818457. doi: 10.3389/fcell.2022.818457
20. Molodtsov AK, Khatwani N, Vella JL, Lewis KA, Zhao Y, Han J, et al. Resident memory Cd8(+) T cells in regional lymph nodes mediate immunity to metastatic melanoma. Immunity (2021) 54(9):2117–32 e7. doi: 10.1016/j.immuni.2021.08.019
21. Belote RL, Le D, Maynard A, Lang UE, Sinclair A, Lohman BK, et al. Human melanocyte development and melanoma dedifferentiation at single-cell resolution. Nat Cell Biol (2021) 23(9):1035–47. doi: 10.1038/s41556-021-00740-8
22. Gellatly KJ, Strassner JP, Essien K, Refat MA, Murphy RL, Coffin-Schmitt A, et al. Scrna-seq of human vitiligo reveals complex networks of subclinical immune activation and a role for Ccr5 in treg function. Sci Trans Med (2021) 13(610):eabd8995. doi: 10.1126/scitranslmed.abd8995
23. Gaydosik AM, Tabib T, Domsic R, Khanna D, Lafyatis R, Fuschiotti P. Single-cell transcriptome analysis identifies skin-specific T-cell responses in systemic sclerosis. Ann Rheumatic Dis (2021) 80(11):1453–60. doi: 10.1136/annrheumdis-2021-220209
25. Agache I, Akdis C, Jutel M, Virchow JC. Untangling asthma phenotypes and endotypes. Allergy (2012) 67(7):835–46. doi: 10.1111/j.1398-9995.2012.02832.x
26. Lötvall J, Akdis CA, Bacharier LB, Bjermer L, Casale TB, Custovic A, et al. Asthma endotypes: A new approach to classification of disease entities within the asthma syndrome. J Allergy Clin Immunol (2011) 127(2):355–60. doi: 10.1016/j.jaci.2010.11.037
27. Brunner PM, Leung DYM, Guttman-Yassky E. Immunologic, microbial, and epithelial interactions in atopic dermatitis. Ann Allergy Asthma Immunol (2018) 120(1):34–41. doi: 10.1016/j.anai.2017.09.055
28. Czarnowicki T, He H, Krueger JG, Guttman-Yassky E. Atopic dermatitis endotypes and implications for targeted therapeutics. J Allergy Clin Immunol (2019) 143(1):1–11. doi: 10.1016/j.jaci.2018.10.032
29. Nomura I, Gao B, Boguniewicz M, Darst MA, Travers JB, Leung DY. Distinct patterns of gene expression in the skin lesions of atopic dermatitis and psoriasis: A gene microarray analysis. J Allergy Clin Immunol (2003) 112(6):1195–202. doi: 10.1016/j.jaci.2003.08.049
30. Suárez-Fariñas M, Ungar B, Correa da Rosa J, Ewald DA, Rozenblit M, Gonzalez J, et al. Rna sequencing atopic dermatitis transcriptome profiling provides insights into novel disease mechanisms with potential therapeutic implications. J Allergy Clin Immunol (2015) 135(5):1218–27. doi: 10.1016/j.jaci.2015.03.003
31. He H, Suryawanshi H, Morozov P, Gay-Mimbrera J, Del Duca E, Kim HJ, et al. Single-cell transcriptome analysis of human skin identifies novel fibroblast subpopulation and enrichment of immune subsets in atopic dermatitis. J Allergy Clin Immunol (2020) 145(6):1615–28. doi: 10.1016/j.jaci.2020.01.042
32. Ko KI, Merlet JJ, DerGarabedian BP, Zhen H, Suzuki-Horiuchi Y, Hedberg ML, et al. Nf-kappab perturbation reveals unique immunomodulatory functions in Prx1(+) fibroblasts that promote development of atopic dermatitis. Sci Trans Med (2022) 14(630):eabj0324. doi: 10.1126/scitranslmed.abj0324
33. Rojahn TB, Vorstandlechner V, Krausgruber T, Bauer WM, Alkon N, Bangert C, et al. Single-cell transcriptomics combined with interstitial fluid proteomics defines cell type-specific immune regulation in atopic dermatitis. J Allergy Clin Immunol (2020) 146(5):1056–69. doi: 10.1016/j.jaci.2020.03.041
34. Kim BS, Siracusa MC, Saenz SA, Noti M, Monticelli LA, Sonnenberg GF, et al. Tslp elicits il-33-Independent innate lymphoid cell responses to promote skin inflammation. Sci Trans Med (2013) 5(170):170ra16. doi: 10.1126/scitranslmed.3005374
35. Salimi M, Barlow JL, Saunders SP, Xue L, Gutowska-Owsiak D, Wang X, et al. A role for il-25 and il-33-Driven type-2 innate lymphoid cells in atopic dermatitis. J Exp Med (2013) 210(13):2939–50. doi: 10.1084/jem.20130351
36. Nakatani-Kusakabe M, Yasuda K, Tomura M, Nagai M, Yamanishi K, Kuroda E, et al. Monitoring cellular movement with photoconvertible fluorescent protein and single-cell rna sequencing reveals cutaneous group 2 innate lymphoid cell subtypes, circulating Ilc2 and skin-resident Ilc2. JID Innov (2021) 1(3):100035. doi: 10.1016/j.xjidi.2021.100035
37. Imai Y, Kusakabe M, Nagai M, Yasuda K, Yamanishi K. Dupilumab effects on innate lymphoid cell and helper T cell populations in patients with atopic dermatitis. JID Innov (2021) 1(1):100003. doi: 10.1016/j.xjidi.2021.100003
38. Alkon N, Bauer WM, Krausgruber T, Goh I, Griss J, Nguyen V, et al. Single-cell analysis reveals innate lymphoid cell lineage infidelity in atopic dermatitis. J Allergy Clin Immunol (2022) 149(2):624–39. doi: 10.1016/j.jaci.2021.07.025
39. Rindler K, Krausgruber T, Thaler FM, Alkon N, Bangert C, Kurz H, et al. Spontaneously resolved atopic dermatitis shows melanocyte and immune cell activation distinct from healthy control skin. Front Immunol (2021) 12:630892. doi: 10.3389/fimmu.2021.630892
40. Liu Y, Wang H, Taylor M, Cook C, Martínez-Berdeja A, North JP, et al. Classification of human chronic inflammatory skin disease based on single-cell immune profiling. Sci Immunol (2022) 7(70):eabl9165. doi: 10.1126/sciimmunol.abl9165
41. Michalek IM, Loring B, John SM. A systematic review of worldwide epidemiology of psoriasis. J Eur Acad Dermatol Venereol: JEADV (2017) 31(2):205–12. doi: 10.1111/jdv.13854
42. Alwan W, Nestle FO. Pathogenesis and treatment of psoriasis: Exploiting pathophysiological pathways for precision medicine. Clin Exp Rheumatol (2015) 33(5 Suppl 93):S2–6.
43. Haugh IM, Preston AK, Kivelevitch DN, Menter AM. Risankizumab: An anti-Il-23 antibody for the treatment of psoriasis. Drug Design Dev Ther (2018) 12:3879–83. doi: 10.2147/dddt.S167149
44. Leonardi C, Matheson R, Zachariae C, Cameron G, Li L, Edson-Heredia E, et al. Anti-Interleukin-17 monoclonal antibody ixekizumab in chronic plaque psoriasis. N Engl J Med (2012) 366(13):1190–9. doi: 10.1056/NEJMoa1109997
45. Kim J, Lee J, Kim HJ, Kameyama N, Nazarian R, Der E, et al. Single-cell transcriptomics applied to emigrating cells from psoriasis elucidate pathogenic versus regulatory immune cell subsets. J Allergy Clin Immunol (2021) 148(5):1281–92. doi: 10.1016/j.jaci.2021.04.021
46. Cheuk S, Wikén M, Blomqvist L, Nylén S, Talme T, Ståhle M, et al. Epidermal Th22 and Tc17 cells form a localized disease memory in clinically healed psoriasis. J Immunol (2014) 192(7):3111–20. doi: 10.4049/jimmunol.1302313
47. Hijnen D, Knol EF, Gent YY, Giovannone B, Beijn SJ, Kupper TS, et al. Cd8(+) T cells in the lesional skin of atopic dermatitis and psoriasis patients are an important source of ifn-Γ, il-13, il-17, and il-22. J Invest Dermatol (2013) 133(4):973–9. doi: 10.1038/jid.2012.456
48. Cheuk S, Schlums H, Gallais Sérézal I, Martini E, Chiang SC, Marquardt N, et al. Cd49a expression defines tissue-resident Cd8(+) T cells poised for cytotoxic function in human skin. Immunity (2017) 46(2):287–300. doi: 10.1016/j.immuni.2017.01.009
49. Liu J, Chang HW, Huang ZM, Nakamura M, Sekhon S, Ahn R, et al. Single-cell rna sequencing of psoriatic skin identifies pathogenic Tc17 cell subsets and reveals distinctions between Cd8(+) T cells in autoimmunity and cancer. J Allergy Clin Immunol (2021) 147(6):2370–80. doi: 10.1016/j.jaci.2020.11.028
50. FitzGerald O, Ogdie A, Chandran V, Coates LC, Kavanaugh A, Tillett W, et al. Psoriatic arthritis. Nat Rev Dis Primers (2021) 7(1):59. doi: 10.1038/s41572-021-00293-y
51. Costello PJ, Winchester RJ, Curran SA, Peterson KS, Kane DJ, Bresnihan B, et al. Psoriatic arthritis joint fluids are characterized by Cd8 and Cd4 T cell clonal expansions appear antigen driven. J Immunol (2001) 166(4):2878–86. doi: 10.4049/jimmunol.166.4.2878
52. Penkava F, Velasco-Herrera MDC, Young MD, Yager N, Nwosu LN, Pratt AG, et al. Single-cell sequencing reveals clonal expansions of pro-inflammatory synovial Cd8 T cells expressing tissue-homing receptors in psoriatic arthritis. Nat Commun (2020) 11(1):4767. doi: 10.1038/s41467-020-18513-6
53. Hughes TK, Wadsworth MH 2nd, Gierahn TM, Do T, Weiss D, Andrade PR, et al. Second-strand synthesis-based massively parallel scrna-seq reveals cellular states and molecular features of human inflammatory skin pathologies. Immunity (2020) 53(4):878–94.e7. doi: 10.1016/j.immuni.2020.09.015
54. Kashem SW, Haniffa M, Kaplan DH. Antigen-presenting cells in the skin. Annu Rev Immunol (2017) 35:469–99. doi: 10.1146/annurev-immunol-051116-052215
55. Dutertre CA, Becht E, Irac SE, Khalilnezhad A, Narang V, Khalilnezhad S, et al. Single-cell analysis of human mononuclear phagocytes reveals subset-defining markers and identifies circulating inflammatory dendritic cells. Immunity (2019) 51(3):573–89.e8. doi: 10.1016/j.immuni.2019.08.008
56. Nakamizo S, Dutertre CA, Khalilnezhad A, Zhang XM, Lim S, Lum J, et al. Single-cell analysis of human skin identifies Cd14+ type 3 dendritic cells Co-producing Il1b and Il23a in psoriasis. J Exp Med (2021) 218(9):e20202345. doi: 10.1084/jem.20202345
57. Pantelyushin S, Haak S, Ingold B, Kulig P, Heppner FL, Navarini AA, et al. Rorγt+ innate lymphocytes and Γδ T cells initiate psoriasiform plaque formation in mice. J Clin Invest (2012) 122(6):2252–6. doi: 10.1172/jci61862
58. Teunissen MBM, Munneke JM, Bernink JH, Spuls PI, Res PCM, Te Velde A, et al. Composition of innate lymphoid cell subsets in the human skin: Enrichment of ncr(+) Ilc3 in lesional skin and blood of psoriasis patients. J Invest Dermatol (2014) 134(9):2351–60. doi: 10.1038/jid.2014.146
59. Villanova F, Flutter B, Tosi I, Grys K, Sreeneebus H, Perera GK, et al. Characterization of innate lymphoid cells in human skin and blood demonstrates increase of Nkp44+ Ilc3 in psoriasis. J Invest Dermatol (2014) 134(4):984–91. doi: 10.1038/jid.2013.477
60. Bielecki P, Riesenfeld SJ, Hütter JC, Torlai Triglia E, Kowalczyk MS, Ricardo-Gonzalez RR, et al. Skin-resident innate lymphoid cells converge on a pathogenic effector state. Nature (2021) 592(7852):128–32. doi: 10.1038/s41586-021-03188-w
61. Rupec RA, Boneberger S, Ruzicka T. What is really in control of skin immunity: Lymphocytes, dendritic cells, or keratinocytes? facts and controversies. Clinics Dermatol (2010) 28(1):62–6. doi: 10.1016/j.clindermatol.2009.04.004
62. Harirchian P, Lee J, Hilz S, Sedgewick AJ, Perez White BE, Kesling MJ, et al. A20 and Abin1 suppression of a keratinocyte inflammatory program with a shared single-cell expression signature in diverse human rashes. J Invest Dermatol (2019) 139(6):1264–73. doi: 10.1016/j.jid.2018.10.046
63. Kouwenhoven TA, Bronckers I, van de Kerkhof PCM, Kamsteeg M, Seyger MMB. Psoriasis dermatitis: An overlap condition of psoriasis and atopic dermatitis in children. J Eur Acad Dermatol Venereol (2019) 33(2):e74–e6. doi: 10.1111/jdv.15213
64. Bozek A, Zajac M, Krupka M. Atopic dermatitis and psoriasis as overlapping syndromes. Mediators Inflammation (2020) 2020:7527859. doi: 10.1155/2020/7527859
65. Napolitano M, Caiazzo G, Fabbrocini G, Balato A, Di Caprio R, Scala E, et al. Increased expression of interleukin-23a in lesional skin of patients with atopic dermatitis with psoriasiform reaction during dupilumab treatment. Br J Dermatol (2021) 184(2):341–3. doi: 10.1111/bjd.19459
66. Napolitano M, Gallo L, Patruno C, Fabbrocini G, Megna M. Eczematous reaction to ixekizumab successfully treated with dupilumab. Dermatol Ther (2020) 33(2):e13218. doi: 10.1111/dth.13218
67. Derrien T, Johnson R, Bussotti G, Tanzer A, Djebali S, Tilgner H, et al. The gencode V7 catalog of human long noncoding rnas: Analysis of their gene structure, evolution, and expression. Genome Res (2012) 22(9):1775–89. doi: 10.1101/gr.132159.111
68. Flynn RA, Chang HY. Long noncoding rnas in cell-fate programming and reprogramming. Cell Stem Cell (2014) 14(6):752–61. doi: 10.1016/j.stem.2014.05.014
69. Gil N, Ulitsky I. Regulation of gene expression by cis-acting long non-coding rnas. Nat Rev Genet (2020) 21(2):102–17. doi: 10.1038/s41576-019-0184-5
70. Isakova A, Neff N, Quake SR. Single-cell quantification of a broad rna spectrum reveals unique noncoding patterns associated with cell types and states. Proc Natl Acad Sci U.S.A. (2021) 118(51):e2113568118. doi: 10.1073/pnas.2113568118
71. Wang Y, Zhao Y, Bollas A, Wang Y, Au KF. Nanopore sequencing technology, bioinformatics and applications. Nat Biotechnol (2021) 39(11):1348–65. doi: 10.1038/s41587-021-01108-x
72. Chung KB, Oh J, Roh WS, Kim TG, Kim DY. Core gene signatures of atopic dermatitis using public rna-sequencing resources: Comparison of bulk approach with single-cell approach. J Invest Dermatol (2022) 142(3 Pt A):717–21.e5. doi: 10.1016/j.jid.2021.07.169
73. Kim D, Chung KB, Kim TG. Application of single-cell rna sequencing on human skin: Technical evolution and challenges. J Dermatol Sci (2020) 99(2):74–81. doi: 10.1016/j.jdermsci.2020.06.002
74. Bao S, Li K, Yan C, Zhang Z, Qu J, Zhou M. Deep learning-based advances and applications for single-cell rna-sequencing data analysis. Brief Bioinform (2022) 23(1):bbab473. doi: 10.1093/bib/bbab473
Keywords: single-cell RNA sequencing, inflammation, atopic dermatitis, psoriasis, transcriptomics
Citation: Xia D, Wang Y, Xiao Y and Li W (2022) Applications of single-cell RNA sequencing in atopic dermatitis and psoriasis. Front. Immunol. 13:1038744. doi: 10.3389/fimmu.2022.1038744
Received: 07 September 2022; Accepted: 27 October 2022;
Published: 25 November 2022.
Edited by:
Eva Reali, University of Milano-Bicocca, ItalyReviewed by:
H. Robert Frost, Dartmouth College, United StatesCopyright © 2022 Xia, Wang, Xiao and Li. This is an open-access article distributed under the terms of the Creative Commons Attribution License (CC BY). The use, distribution or reproduction in other forums is permitted, provided the original author(s) and the copyright owner(s) are credited and that the original publication in this journal is cited, in accordance with accepted academic practice. No use, distribution or reproduction is permitted which does not comply with these terms.
*Correspondence: Wei Li, bGl3ZWloeF9oeHl5QHNjdS5lZHUuY24=
Disclaimer: All claims expressed in this article are solely those of the authors and do not necessarily represent those of their affiliated organizations, or those of the publisher, the editors and the reviewers. Any product that may be evaluated in this article or claim that may be made by its manufacturer is not guaranteed or endorsed by the publisher.
Research integrity at Frontiers
Learn more about the work of our research integrity team to safeguard the quality of each article we publish.