- Department of Medical Oncology Cancer Center, West China Hospital, Sichuan University, Sichuan, China
Immunotherapy has revolutionized colon cancer treatment. Immune checkpoint inhibitors (ICIs) have shown clinical benefits for colon cancer patients, especially those with high microsatellite instability (MSI-H). In 2020, the US Food and Drug Administration (FDA)-approved ICI pembrolizumab as the first-line treatment for metastatic MSI-H colon cancer patients. Additionally, neoadjuvant immunotherapy has presented efficacy in treating early-stage colon cancer patients. Although MSI has been thought of as an effective predictive biomarker for colon cancer immunotherapy, only a small proportion of colon cancer patients were MSI-H, and certain colon cancer patients with MSI-H presented intrinsic or acquired resistance to immunotherapy. Thus, further search for predictive biomarkers to stratify patients is meaningful in colon cancer immunotherapy. Except for MSI, other biomarkers, such as PD-L1 expression level, tumor mutation burden (TMB), tumor-infiltrating lymphocytes (TILs), certain gut microbiota, ctDNA, and circulating immune cells were also proposed to be correlated with patient survival and ICI efficacy in some colon cancer clinical studies. Moreover, developing new diagnostic techniques helps identify accurate predictive biomarkers for colon cancer immunotherapy. In this review, we outline the reported predictive biomarkers in colon cancer immunotherapy and further discuss the prospects of technological changes for biomarker development in colon cancer immunotherapy.
Introduction
Colon cancer is currently one of the malignant tumors with a high incidence and death rate worldwide (1). Traditionally, the main therapeutic strategies in colon cancer include surgery, chemotherapy, and targeted therapy. Among these therapy methods, surgery is applicable to early-stage patients with lesions confined to the colon, while approximately 20% of colon cancer patients have distant metastases at the time of diagnosis and miss the opportunity for surgery (2). Chemotherapy is the main treatment option for metastatic colon cancer patients. In addition, depending on the RAS gene mutational status and tumor location, corresponding targeted agents, such as bevacizumab or cetuximab, were combined to enhance the anti-tumor effect of chemotherapy agents. Even so, the patients’ prognosis is still dismal (3). Recently, immunotherapy has revolutionized colon cancer treatment. In the clinical study, pembrolizumab, an anti-programmed cell death 1 (PD-1) agent, resulted in significant improvements in progression-free survival (16.5 vs. 8.2 months) and fewer adverse events than chemotherapy as a first-line treatment in patients with microsatellite instability-high (MSI-H) or mismatch repair-deficient (dMMR) metastatic colorectal cancer (mCRC) (4). This promising result led to the US Food and Drug Administration’s (FDA) approval of pembrolizumab as the first-line treatment for MSI-H mCRC patients. Moreover, double ICI combination, anti-cytotoxic T lymphocyte-associated antigen 4 (CTLA-4) agent, and anti-PD-1 agent combination therapy presented promising anti-tumor efficacy in MSI-H/dMMR mCRC (5, 6). Furthermore, neoadjuvant immunotherapy also presented promising efficacy in treating early-stage MSI-H/dMMR colon cancer patients (7).
Currently, MSI-H/dMMR is the only well-recognized biomarker that can be used to guide the immunotherapy of colon cancer. However, the mechanism of why MSI-H/dMMR can be used as a biomarker for colon cancer immunotherapy is still not clarified (8). The possible mechanism was thought to be that MSI-H/dMMR may be correlated with a higher mutational load, which leads to neoantigen formation and activation of the body’s immunity (9, 10). The efficiency of MSI as the biomarker for cancer immunotherapy is relatively low. The reported objective response rate (ORR) in MSI-H/dMMR colon cancer patients varies between 30%-70% (4, 5, 11–14). Additionally, colon cancer patients with MSI-H/dMMR are not in a high proportion of the total number of colon cancer patients. Only approximately 20% of colon cancer patients are MSI-H (8); whereas, in stage IV colon cancer patients, MSI-H is less than 5% (15). In addition, a small percentage of patients with microsatellite stable (MSS) or proficient MMR (pMMR) can benefit from immunotherapy, while a significant proportion of MSI-H/dMMR patients demonstrate intrinsic or acquired resistance to immunotherapy (16–19). These results suggest that more precise biomarkers are needed to stratify colon cancer patients that could benefit from immunotherapy.
Other potential predictive biomarkers which are proposed in colon cancer immunotherapy include PD-L1 expression level, tumor mutation burden (TMB), tumor-infiltrating lymphocytes (TILs), gut microbiota, ctDNA, and circulating immune cells (20–24). In addition, relevant indicators that reflect the tumor microenvironment (TME) have also been proposed for use as biomarkers for immunotherapy in colon cancer (25). Immunoscore and consensus molecular subtypes (CMS) based on immune cells and molecular typing have implications for clinical management and are predictive of prognosis and treatment response in patients with colon cancer (26, 27). New techniques, such as multiplex immunohistochemistry (mIHC) and single-cell RNA sequencing, could provide a more comprehensive evaluation of the TME and genetic heterogeneity in colon cancer. This will help find precise biomarkers for screening and efficacy assessment of colon cancer immunotherapy beneficiary populations (28, 29). In this review, we comprehensively summarize the reported biomarkers of colon cancer immunotherapy and further discuss the prospects of technological changes for biomarker development in colon cancer immunotherapy.
MSI and dMMR as the predictive biomarker for colon cancer immunotherapy
Microsatellites (MS) are short tandem repeats (STRs) in the human genome that are composed of several short and repetitive DNA sequences. Microsatellite instability (MSI) refers to the failure of the DNA mismatch repair mechanism during the DNA replication process, which results in length changes in MS (8). MSI was first identified in hereditary nonpolyposis colorectal cancer syndrome, known as Lynch syndrome (30). Subsequently, multiple types of malignant tumor patients were found to present with MSI (31–35). MSI is an indicator of tumor prognosis and treatment response (36). According to the status of MMR, tumors can be classified as dMMR and pMMR. IHC is the main method to test the MMR status. Tumors with loss of expression of MMR genes, including MutS homolog 2 (MSH2), MutL homolog 1 (MLH1), MutS homolog 6 (MSH6), or postmeiotic segregation increased by 2 (PMS2), were defined as dMMR; otherwise, they were defined as pMMR. In addition, according to the mutation frequency of MS, tumors can be termed MSI-H, low-frequency MSI (MSI-L), and MSS. Polymerase chain reaction (PCR) is the main method used to assess the frequency of MSI mutations. Due to the respective limitations of these two methods, a combination test of both IHC and PCR is usually required to evaluate the status of MS (37). In general, MSI-H is equivalent to dMMR.
Approximately 20% of colon cancer patients are MSI-H/dMMR (8). Except for approximately 3% of MSI-H/dMMR colon cancer patients who were hereditary with Lynch syndrome, most MSI-H/dMMR colon cancer patients are sporadic. The mechanisms of MSI in hereditary and sporadic MSI-H/dMMR patients are different (8). MSI-H/dMMR colon cancer patients all present unique clinicopathological features that correlate with patient prognosis and treatment response. For example, colon cancer patients with MSI-H/dMMR were reported to have a better prognosis than those with MSI-L/pMMR (38, 39). Colon cancer patients with MSI-H/dMMR did not benefit from fluorouracil-based therapy (40). In particular, there is now considerable clinical evidence that MSI-H/dMMR colon cancer patients present a high ORR to ICIs. The relationship between MSI and colon cancer immunotherapy response was initially found in a phase I clinical trial. In this study, the safety and tolerability of the anti-PD-1 antibody BMS-936558 were evaluated in treatment-refractory solid tumor patients. A post-operative recurrent colon cancer patient who reported a durable complete response after therapy was MSI-H (41). Then, the KEYNOTE-016 trial was conducted to identify the role of MMR status as a biomarker for predicting the clinical benefit of ICI treatment. In this study, progressive metastatic carcinoma patients with or without dMMR were treated with the PD-1 antibody pembrolizumab. In the cohort of patients with dMMR colorectal adenocarcinomas, the reported ORR was 40% versus 0% in the cohort of pMMR patients (11). A similar result was observed in another clinical study, KEYNOTE164. In this study, the antitumor activity of pembrolizumab was tested in previously treated and metastatic MSI-H/dMMR colorectal cancer (CRC) patients. The reported ORR was 33% (14). In addition, in CheckMate 142, another PD-1 agent called nivolumab also induced durable responses and disease control in pretreated dMMR/MSI-H metastatic CRC patients. At a median follow-up of 12.0 months, 31.1% of patients achieved an investigator-assessed objective response (13). Based on these results, the FDA approved ICIs (pembrolizumab and nivolumab) for the treatment of MSI-H/dMMR metastatic CRC patients in 2017. Then, in KEYNOTE-177, the efficacy of pembrolizumab was compared with chemotherapy as first-line therapy for MSI-H/dMMR advanced or metastatic CRC. After a median follow-up of 32.4 months, pembrolizumab therapy demonstrated superiority over chemotherapy in terms of median progression-free survival (16.5 months vs. 8.2 months). ORR was observed in 43.8% of the patients in the pembrolizumab group versus 33.1% in the chemotherapy group (4). This result prompted the FDA to approve pembrolizumab as the first-line treatment for metastatic CRC patients with MSI-H. In addition, in the GERCOR NIPICOL phase II study, an impressive DCR was observed in MSI/dMMR mCRC patients treated with anti-PD-1 inhibitor nivolumab combined with anti-CTLA-4 inhibitor ipilimumab (6). Recently, the preliminary results of nivolumab plus low-dose ipilimumab as the first-line therapy cohort from the CheckMate 142 study were reported. This double ICI combination demonstrated a durable clinical benefit as a first-line treatment for MSI-H/dMMR mCRC. The ORR is 69% in the combination group (5).
However, although MSI-H/dMMR has a promising prospect as the biomarker for colon cancer immunotherapy, the reported ORRs in MSI-H mCRC patients vary from 30%-70% (5, 6, 11–14); this implies that a certain number of MSI-H mCRC patients also do not benefit from immunotherapy. In contrast, a small subset of MSS colon cancer patients responded to immunotherapy (42). The diagnostic mistake caused by the test method is one of the reasons for this phenomenon (18). The current methods could be used for detecting MSI, including IHC, PCR, and next-generation sequencing (NGS). By using IHC to detect the expression of four MMR genes (MLH1, MSH2, MSH6, and PMS2) in the tumor cell nucleus, the presence of one or more negative proteins was defined as dMMR; otherwise, they were defined as pMMR. The advantage of IHC lies in that it is easy to perform and allows direct identification of the MMR gene status. The disadvantages of IHC are its subjectivity and lack of a uniform standard (43). PCR was based on comparing DNA extracted from tumor tissue and normal tissue to detect MSI status. In 1997, the National Cancer Institute (NCI) first formalized the guidelines for PCR testing of MSI, which contained 2 single nucleotide repeat sites (BAT-25 and BAT-26) and 3 dinucleotide repeat sites (D2S123, D5S346, and D17S250) (44). Subsequent studies identified the limitations of this criterion and improved the criteria for PCR detection of MSI. Currently, the five poly-A panel (BAT-25, BAT-26, NR-21, NR-24, and NR-27) is the usually recommended panel for MSI-PCR tests. MSI at more than two loci out of five is defined as MSI-high (MSI-H); MSI at one of five loci is defined as MSI-low (MSI-L); and no instability at any of the loci is defined as MSS (45). PCR is the currently accepted gold standard for the detection of MSI, with high accuracy and standardization. The disadvantage of PCR for MSI detection is that it cannot directly determine abnormal proteins. In addition, considering the difference in MSI incidences among different ethnicities, although there are standardized recommendations, the selection of the appropriate panel for different populations is also essential for the detection of MSI PCR tests (46). NGS is the third alternative method for MSI measurement, which determines MSI by directly measuring the length of altered MS. The NGS method does not require normal tissue as a control, requires lower sample quality, and is more compatible than PCR. NGS can simultaneously provide information on MSI loci, MMR gene status, and information on other gene statuses. In addition, NGS can detect MSI status by peripheral blood samples, unlike traditional PCR methods. However, what limit the clinical promotions and uses of NGS are the high cost, complexity of the data analysis process, and lack of uniform evaluation criteria (47).
Furthermore, reasonable direct evidence for MSI-H/dMMR as a biomarker for immunotherapy is still lacking. Existing studies suggest that the main reason for the response of MSI-H patients to immunotherapy is that MSI may cause the production of new antigens, leading to the recruitment of immune cells and the release of proinflammatory factors. A higher TMB and infiltration of TILs were found in patients with MSI-H/dMMR (48). However, TMB and MSI do not always match perfectly (49). Immunosuppressive cells, such as myeloid-derived suppressor cells (MDSCs) and T-regulatory cells (Tregs), were also found in MSI-H/dMMR patients, which means that a more comprehensive biomarker portfolio is needed for immunotherapy efficacy prediction (50).
TMB as the predictive biomarker for colon cancer immunotherapy
TMB is another potential effective biomarker in the field of tumor immunotherapy. TMB is usually defined as the total number of somatic mutations detected per million bases (muts/Mb). It was thought that a high TMB (TMB-H) status was related to more tumor neoantigens, and more tumor neoantigens presented on the surface of tumor cells may be recognized by immune cells and activate the body immune system to kill the tumor (51). In KEYNOTE-158, pembrolizumab was tested in several types of advanced solid tumor patients but did not include colon cancer patients. The results show that patients with TMB-H status (TMB≥10 muts/Mb) presented higher objective responses to pembrolizumab. The ORRs were reported to be 29% in the TMB-H group versus 6% in the non-TMB-H group (52). Based on this result, in June 2020, the FDA approved the use of pembrolizumab for unresectable or metastatic solid tumor patients with TMB-H status. With a cutoff value of 10 muts/Mb, higher response rates to immunotherapy were confirmed in melanoma and non-small cell lung cancer (NSCLC) patients with TMB-H (53, 54).
In colon cancer, TMB was found to be potentially correlated with patient survival. It was reported that colon cancer patients with high TMB (TMB≥8 muts/Mb) presented longer OS than those with low TMB (55). Additionally, TMB was reported as an additional predictive biomarker for MSI in metastatic CRC. Among MSI-H mCRC patients, patients with TMB-H (the TMB cutoff point was defined between 37 and 41 mutations/Mb) have shown a better prognosis than those with TMB-L after receiving immunotherapy (18). However, the independent application of TMB for the prediction of immunotherapy response in colon cancer is still controversial. In the KEYNOTE 177 trial, the limits of TMB as a predictor of the response of CRC to anti-PD1 immunotherapy were observed (56). However, in the Canadian cancer trials group CO.26 study, elevated plasma TMB levels (≥28 muts/Mb) showed a predictable response to anti-PD-L1 agent durvalumab and anti-CTLA4 agent tremelimumab combination therapy in MSS colon cancer patients (57).
One of the greatest obstacles causing this controversy is the difficulty in defining the TMB cutoff value. Nonetheless, the cutoff of 10 muts/Mb presented a relatively good sensitivity in the prediction of immunotherapy in NSCLC and melanoma, but this cutoff value cannot be generalized for different tumor types (58). Currently, there is no uniform TMB cutoff value for colon cancer. In a recently reported study, TMB≥16 mut/Mb was proposed as the optimal threshold for ICI atezolizumab monotherapy in advanced solid tumor types. In patients with TMB ≥16 mut/Mb, durable clinical activity was observed, and particularly high response rates (70%) were reported in CRC patients, including both MSI-H and MSI-L (59). Nonetheless, this finding still awaits further validation by prospective studies. In addition, TMB is proposed as a predictive biomarker for immunotherapy because it may represent a useful estimation of tumor neoantigens. However, not all neoantigens presented on the cell surface are immunogenic. Only mutations resulting in higher ‘quality’ antigens can induce an antitumor immune response (60, 61). This may explain why TMB cannot be used as an independent marker of the effectiveness of tumor immunotherapy. Furthermore, the testing method hinders the clinical application of TMB. TMB was initially performed using whole-exome sequencing (WES), and this technology is complex and costly. Currently, NGS has been used in the clinic as a substitute for WES; however, there are different algorithms for WES-based and NGS-panel-based methods. In addition, the advantage of TMB as the biomarker for immunotherapy is that TMB can be not only obtained from tumor tissue but also detected by peripheral blood. Therefore, in some studies, tissue TMB was substituted with plasma TMB when unavailable. But there are differences in the criteria for assessing TMB in blood samples versus tissue samples, which cause inconsistencies among different studies and interfere with TMB standardization (62).
In summary, although several pieces of evidence indicate that TMB cannot be used as an independent predictive biomarker in colon cancer immunotherapy, it is still valuable in immunotherapy efficacy prediction, especially when sufficient evidence is obtained for a valid TMB cutoff value in colon cancer. TMB can be used as an important complementary biomarker, such as when combined with MSI, and for identifying other significant gene mutations; it was found, that some MSS colon cancer patients with high TMB had polymerase epsilon (POLE) mutation, and they responded well to immunotherapy (63).
The value of molecular subtype and consensus molecular subtype as predictive biomarkers in colon cancer immunotherapy
Colon cancer is a heterogeneous disease. Colon cancer patients usually present different molecular subtypes. Some molecular subtypes, such as RAS and BRAF mutations, have already been used to guide the treatment and prognostic assessment of colon cancer (64). Among these molecular subtypes, the encoded DNA POLE and delta 1 (POLD1) mutation has attracted much attention due to its potential association with immunotherapy response. POLE/POLD1 play an important role in proofreading and ensuring the fidelity of DNA replication. The somatic or germline mutations in POLE and POLD1 lead to defects in DNA repair and, consequently, to tumorigenesis (65). In colon cancer, about 7.4% of patients harbor POLE or POLD1 mutations, and most of this population was MSS or MSI-L (66). In 2019, Wang and his colleagues, through analyzing medical data of 47,721 patients with various cancer types with POLE/POLD1 mutations, proposed that POLE/POLD1 mutations are promising potential predictive biomarkers for positive ICI outcomes (66). However, in a previous clinical study, which enrolled three CRC patients with POLE mutations, three of the patients did not show an achieved response to anti-PD-L1 inhibitor avelumab (67). In a recent study, where anti-PD-L1 inhibitor durvalumab monotherapy was used to treat previously treated MSI-H/dMMR or POLE-mutated mCRC patients, the results showed that POLE mutation mCRC patients had a clinical response to durvalumab, only those with exonuclease domain mutation (68). Furthermore, there is a limited role of other molecular mutations as biomarkers in predicting the response of colon cancer to immunotherapy; this includes KRAS mutation, a common molecular subtype in colon cancer. Although KRAS mutation is proposed to modulate tumor immunity (69), its biomarker value in colon cancer immunotherapy was found to be weak. According to Lal et al., KRAS mutation is proposed to be associated with suppressed cytotoxic immunity in CRC, and the extent of the effect is modulated by consensus molecular subtype (CMS) (70).
The CRC Subtyping Consortium, based on the gene expression of the tumor, proposed four CMSs for CRC using transcriptomics in 2015. The CMS classification included CMS1, CMS2, CMS3, and CMS4. CMS1 is categorized as MSI immune and presents with strong immune activation; CMS2 is categorized as canonical and is characterized by chromosomal instability as well as WNT and MYC signaling activation; CMS3 is categorized as metabolic and is associated with metabolic dysregulation; CMS4 is categorized as mesenchymal and is associated with prominent transforming growth factor β (TGF-β) activation, stromal invasion, and angiogenesis (71). CMS classification can be used in guiding colon cancer treatment strategies and predicting patient prognosis (72–74). The correlation between CMS and tumor immune characteristics has been proposed in several studies (27, 75). In 2016, Becht E et al. integrated the CMS classification with the TME of CRC. Their results show that the good prognosis of CMS1 is related to the overexpression of cytotoxic lymphocytes. In contrast, the poor prognosis of CMS4 is related to a high density of fibroblasts, which produce chemokines and cytokines, resulting in inflammatory and immunosuppressive TMEs. The CMS2 and CMS3 groups presented an intermediate prognosis, exhibiting low immune and inflammatory signatures (75). In a recent study, Hu et al, based on data from multiple databases and using algorithms, further analyzed the molecular characteristics of colon cancer CMS and their immunotherapy responses. Their results indicate that CMS1 patients present a higher positive response to immunotherapy among the four CMS subtypes due to immune infiltration and activation. TILs were significantly higher in the CMS1 subtype than in the other three subtypes. In contrast, CMS4 patients may not respond well to immunotherapy, due to the high Treg and NK-cell infiltration found in the CMS4 subtype (27). Meanwhile, in Chida K and his colleagues’ study, transcriptomic profiling for MSI-H/dMMR gastrointestinal tumors was performed to determine the predictors of response to PD-1 blockade. The results show among 13 CRC patients, the reported ORR for CMS1 was 100%, for CMS4 was 16.7%, but for CMS2 and CMS3 all were 0%. This study indicates that CMS classification may serve as a predictive biomarker for colon cancer immunotherapy (76).
However, existing CMS classification still has certain limits. CMS classification relies on transcriptome analysis of the entire tumor, which has inherent limitations such as stromal confounding and the presence of varied cell-type mixtures. Moreover, the differences in cancer cells and other stromal cells (e.g., immune cells, fibroblasts, and vascular cells) are masked and indistinguishable (77, 78). There is transcriptomic intratumor heterogeneity in CMS classification, which may impact its accuracy (79). To solve this, Joanito et al, using single-cell and bulk transcriptome sequencing, identified two epithelial tumor cells and refined the CMS classification of colon cancer. The refined CMS classification includes intrinsic epithelial subtype, MSI status, and fibrosis. By this classification, a specific subtype of MSS was identified. They proved that despite a lower TMB, iCMS3_MSS tumors are more similar to MSI-H colon cancers, and this refined classification may provide new clues for screening the population benefiting from immunotherapy in colon cancer (80). Recently, Khaliq et al. also refined CRC classification and clinical stratification through a single-cell atlas; they proved that distinct cancer-associated fibroblasts (CAFs) and tumor-associated macrophages (TAMs) are sufficient to explain CMS predictive ability and, based on these cellular phenotypes, could stratify CRC patient prognosis with greater precision (81).
In conclusion, existing molecular subtypes and CMS may not be suitable for stratifying colon cancer patients for immunotherapy. The molecular subtypes and CMS help define the molecular and immunological characteristics of colon cancer, which contribute to the precise therapy of colon cancer. As research progresses, the understanding of the molecules subtype of colon cancer patients continues to improve and a more precise molecular subtype of colon cancer may be recognized, and a more precise CMS classification may be refined, which will further contribute to colon cancer immunotherapy.
TILs, immunescore, and PD-L1 expression as predictive biomarkers for colon cancer immunotherapy
TME plays an essential role in tumorigenesis, development, and immune escape (82). Including immune cells, other components of the TME can influence the immune state and response to immunotherapy in tumors (83). TILs are core components of immune cells involved in tumor immunity. TIL is a global term for a variety of lymphocytes in the TME, including T cells, B cells, and NK cells. Several studies have proven that TILs play prominent roles in malignant tumor development and progression and have been proposed as predictive biomarkers for patient prognosis (84–86). The relationship between TILs and colon cancer patient prognosis was first reported in 1998. In this study, CD8+ T cells infiltrated within cancer cell nests were observed to be a prognostic factor in human CRC (87). A series of studies then reported the role of TILs in the prognosis of patients with colon cancer (88–91).
In several clinical trials of colon cancer immunotherapy, TILs showed potential in being used as predictive biomarkers for immune response. In an analysis study of the KEYNOTE 177 trial, colon cancer patients’ response to immunotherapy was found to be not associated with TMB, but rather with TILs. Immunotherapy-responsive CRC patients were found rich in CD-8+PD-1+ T cells (56). In Loupakis F et al’ study, they proposed that there was a significant positive correlation between high TMB and the number of TILs in the ICI-responsive MSI-H mCRC patients (92). In the pilot clinical trial of perioperative durvalumab combined with tremelimumab for treating resectable CRC liver metastases, the treatment induced activation of CD8+ and CD4+ T cells, and an increase in B-cell density was correlated with patients’ prolonged relapse-free survival (93). In the study of neoadjuvant immunotherapy for early-stage colon cancer patients, CD8+PD-1+ T cell infiltration was a predictive biomarker of response in pMMR patients (7). For MSS mCRC patients, higher CD8+ TIL density at baseline was associated with a greater likelihood of benefit from immunotherapy treatment and activated TILs are considered as the biomarker of effective immune induction (94). And according to Kuang C et al’s study, immune modulation may result from treatment with azacitidine, chemotherapy refractory mCRC patients with higher CD8+TIL density at baseline are more likely to benefit from the combination therapy of pembrolizumab and azacitidine combination (95). In addition to CD8+T cells, the role of CD3+T cells as the predictive biomarker in colon cancer immunotherapy has also been reported in some studies (96, 97). In Turksma et al’s study, they found that high numbers of pre-existing stromal CD3+ T cells are own positive predictive value in adjuvant immunotherapy treatment for MSS colon cancer patients (96). In Chakrabarti S. et al’s study, higher CD3+ and CD8+ T-cell densities were associated with higher ORR in dMMR mCRC patients treated with pembrolizumab (97).
CD3+ T cells and CD8+ T cells are two important types of TILs that represent the total T cells and cytotoxic T cell subsets, respectively. In 2018, the Society for Immunotherapy of Cancer proposed using Immunoscore (IS) to estimate the risk of recurrence in colon cancer patients, and their findings proved the powerful role of IS in CRC recurrence risk assessment (98). IS is based on the quantification of CD3+ T cells and CD8+ T cells at the tumor center and at the invasive margin using IHC. A scoring system ranging from IS0 (I0) to IS4 (I4) and high IS was associated with prolonged survival in CRC (99). In Mlecnik et al’s study, IS is proposed to play a bigger role in predicting CRC patient survival than MSI (100). However, IS as the predictive biomarker for colon cancer immunotherapy has certain limitations. First, IS assays are mainly performed by IHC, which is a semiquantitative test, and the results are susceptible to subjectivity. Second, the IS test requires simultaneous assessment of lymphocytes in the center and margin of the tumor, which is difficult to achieve in metastatic tumors. In addition, intratumoral heterogeneity can also affect the accuracy of IS. For example, heterogeneity of T cells was observed in the primary tumor and hepatic metastases of CRC patients (97). Finally, current studies have shown that the effectiveness of tumor immunotherapy is influenced by the immune landscape rather than by a single immune cell (63, 101–103). Except for CD8+T cells, other immune cells, such as Treg cells, NK cells, DC cells, and B cells, are also closely related to the immune response of tumors (104, 105). Recently, a special lymph node structure, tertiary lymphoid structure (TLS), was also proposed as a biomarker in cancer prognosis and response to immunotherapy (106). The effect of TLS on the prognosis of colon cancer has now been demonstrated in several studies (107–110). But the role of TLS in colon cancer immunotherapy still needs to be verified. In addition, the phenotypic profiles and subsets of TILs were also found to affect the patient’s response to ICI and have the potential to be biomarkers of immunotherapy (96, 111–113). For example, a CD39 subgroup of CD8+ T cells was reported in colorectal and lung tumors, the absence of CD39 in CD8+ TILs causes them to act as bystanders that lack an immune response (112). A similar phenomenon has also been observed in B cells; it was found that B cells with CD86 expression were enriched in tumors with increased numbers of TLSs, induced specific T-cell responses, and enhanced the antitumor effect of ICI (114). Epigenetic alterations of TILs, such as DNA methylation, are also involved in the colon cancer immune response. According to Zou et al’s study, the DNA methylation-based signature of CD8+ TILs was related to the immune response and prognosis of CRC patients (22). Thus, further screening TIL subgroups and studying the immune landscape of colon cancer are key to improving the accuracy of screening for beneficial ICI populations.
PD-L1 is another important indicator for TME. Tumor cells induce tumor immune escape by upregulating PD-L1 expression, which binds to PD-1 on the surface of T cells, causing T-cell deactivation. ICIs can reactivate the body’s antitumor immunity by blocking the binding of PD-1 and PD-L1 (115). Thus, in theory, the higher level of PD-L1 expression in tumor tissues, the better the response to ICI treatment. PD-L1 expression is postulated as a predictive biomarker of immunotherapy response in some solid tumors, such as NSCLC, melanoma, and renal cell cancer (116–118). Positive PD-L1 expression (with a cut-off value of 10%) is reported in more than half of colon cancer patients (119, 120). Although a high PD-L1 expression is associated with a better prognosis in colon cancer patients (20, 121–123). The current clinical data suggest that PD-L1 expression alone cannot be used to precisely predict immunotherapy response in colon cancer (Table 1). Several factors limit PD-L1 expression as a biomarker for colon cancer immunotherapy response: First, there is intratumoral heterogeneity of PD-L1 expression (135), which makes assessing tumor PD-L1 expression level. Second, PD-L1 expression is dynamic, and treatment modalities can affect the expression level of PD-L1. PD-L1 expression varies widely between tumor types and presents a significant intrapatient heterogeneity with a frequent discordance between primary tumors and metastases. Third, the test method also affects the assessment of PD-L1 expression. IHC is now widely used in clinical practice to detect PD-L1 expression in tumor tissues. However, this method is difficult to quantify, the consistency of detecting PD-L1 expression levels between different platforms is poor, and there is still lack of a standardized testing criteria (136). Lastly, tumor cells and immune cells can both express PD-L1. Thus, the predictive effects of PD-L1 expression by tumor cells and PD-L1 expression by lymphocytes on immunotherapy need to be clarified separately. This is illustrated when PD-L1-expressing tumor cells were reported to be a marker of poor prognosis; in contrast, PD-L1-expressing TILs were a marker of good prognosis (137).
Nonetheless, PD-L1 expression still has value in immunotherapy for colon cancer patients. The PD-L1 expression level is an important indicator of the immune status of cancer patients (138–140), and the immune status indicates the tumor response to immunotherapy. PD-L1 combined with other immune indicators demonstrated a promising predictive role in colon cancer immunotherapy. Such as, Llosa et al. proposed the incorporation of histopathologic characteristics (percentage of extracellular mucin) and PD-L1 expression at the invasive front to generate a composite score (CPM score). The CPM score has the potential of discriminating mCRC patients who exhibited clinical benefits from pembrolizumab (124). Additionally, using multiplex immunohistochemistry (mIHC), multiple immune indicators combined with PD-L1 expression can be analyzed simultaneously as well as report TME in various solid tumors, including colon cancer (28, 141, 142).
Therefore, TME immune landscape is significantly related to tumor immunotherapy response. It is not sufficient to evaluate tumor response to immunotherapy by a single index only, such as PD-L1 expression or the number of TILs. A more comprehensively quantified TME immune landscape is necessary for the prediction of colon cancer immunotherapy response.
Other gene signatures of the TME as predictive biomarkers for colon cancer immunotherapy
With the development of gene sequencing technology, several TME-related gene signatures have been proposed as predictive biomarkers for colon cancer immunotherapy. Previously, Ravensbergen et al, using bioinformatics approaches, proved that combined assessment of the tumor-stroma ratio and TILs could be used as a response prediction biomarker of ICI therapy in colon cancer (143). This result reveals the role of the tumor stoma in the response to tumor immunotherapy in patients with colon cancer. CAFs are the main cell type within the tumor stroma, and they are also thought to be an available indicator for assessing the response to immunotherapy. CAFs can interact with tumor cells and TILs via the secretion of various cytokines and chemokines, shaping an immunosuppressive TME and helping tumor cell immune evasion. In addition, CAFs play a significant role in constituting the inflammatory TME of colon cancer (144). Some studies have proven that CAF-derived gene signatures can determine prognosis in colon cancer patients (29, 145, 146). In the area of immunotherapy, it was proven that CAFs promote the upregulation of PD-L1 expression in CRC (147). CAFs have an impact on the prognosis of CRC patients by inhibiting the immune response; thus, patients with higher CAF levels were more prone to be unresponsive to immunotherapy (29, 148). Additionally, among the CMSs, CMS4 is typically characterized by infiltration of adjacent tumor tissues by CAFs and transforming growth factor β (TGF-β) signaling activation, and this subtype presented insensitivity to immunotherapy. Recently, the refinement of CMS through single-cell characterization based on specific CAF subtypes presented the potential role of identifying immunotherapy responses in CRC patients (81). Thus, further study of CAF gene signatures may contribute to the precise stratification of immunotherapy efficacy for colon cancer.
TME metabolic characteristics also influence patients’ response to immunotherapy. Hypoxia is one of the metabolic characteristics of the tumor TME. Hypoxia can play an essential role not only in tumor proliferation, apoptosis, angiogenesis, invasion, and metastasis but also in immune evasion. Hypoxia and the related acidic TME greatly impair the functions of TILs, while alleviating hypoxia could improve the efficacy of ICIs (149). Transcriptomic profiling of MSI-H/dMMR gastrointestinal tumors showed that hypoxia-related signaling pathways were upregulated in ICI nonresponders (76). Recently, several studies proposed that hypoxia-related genes can be classified as predicting immune cell infiltration and prognosis of colon cancer patients (25, 28, 150). These genes provide potential therapeutic targets for immunotherapy as well as prognostic biomarkers for colon cancer patients. In addition to hypoxia, ferroptosis-related gene signatures are another study hot spot in the TME-related prognostic assessment of immunotherapy for colon cancer. Several studies support that ferroptosis plays a vital role in tumor immunotherapy and TME regulation, and ferroptosis-related gene signatures were proposed as potential targets for tumor immunotherapy and patient prognosis (151–153). In recent studies, several ferroptosis-related gene signatures were proposed for the prediction of prognosis and immunotherapy response in colon cancer patients (154–156). These findings further confirm the relevance of ferroptosis to the immune microenvironment and prognosis of colon cancer. Therefore, further understanding of the metabolic characteristics of the TME and the search for metabolism-related gene signatures are valuable for the identification of new biomarkers of colon cancer immunotherapy.
Furthermore, the inflammatory microenvironment of colon cancer induces immune-related genetic alterations, and inflammatory-related genes affect the response of patients to immunotherapy. Wang et al. explored the relationship between inflammation-related genes and the immune TME in CRC. Eight prognostic genes (CX3CL1, CCL22, SERPINE1, LTB4R, XCL1, GAL, TIMP1, ADIPOQ, and CRH) were identified and used to construct a risk-scoring model. The results of this study show that the inflammatory response has a direct impact on CRC patient prognosis and immune infiltration. Thus, further classifying inflammatory response-related genes may help find predictive biomarkers for immunotherapy in colon cancer (157).
Through analysis by transgenomic techniques, some TME-related gene signatures were proposed for use as biomarkers for colon cancer immunotherapy. However, most of these studies were derived from bioinformatic analyses of databases; further validation of these genes in large prospective clinical studies is necessary.
The potential role of certain gut microbiota as the predictive biomarker for colon cancer immunotherapy
The gut microbiota is another hot topic in the current field of immunotherapy. Several studies have proposed that gut microbiota are involved in tumor formation and progression and correlate with patient therapy response in solid cancers (158–164). It has been established that tumor patients have distinct microbiota compared with healthy subjects (165, 166). In addition, compared to patients that did not respond to immunotherapy, a unique intestinal microbiome was found in cancer patients that did respond to immunotherapy (167). Increasing evidence indicates that transplanting the gut microbiome of immunotherapy responders can activate immune cells and make immunotherapy nonresponders respond to immunotherapy (168–171). Therefore, the gut microbiome could be a promising therapeutic target as well as a predictive biomarker in cancer immunotherapy.
Colon cancer presents with an altered state of gut microbiota, which is known as dysbiosis (165). The gut microbiome plays a significant role in the formation of the inflammatory microenvironment during the development of colon cancer. Gut microbes can interact with TILs and influence the tumor immune microenvironment and host sensitivity in favor of immunotherapy in colon cancer (172–174). Recently, certain gut microbes have been proposed as promising predictive biomarkers of colon cancer immunotherapy (23). In 2020, Peng and his colleagues recruited advanced-stage GI cancer patients receiving anti-PD-1/PD-L1 treatment and collected their fecal samples. By comparing the gut microbes of patients before and after treatment, they found an elevation of the Prevotella/Bacteroides ratio in patients with a preferred response to immunotherapy (175). In another phase Ib/II clinical trial of regorafenib plus toripalimab treatment for mCRC, gut microbiome analysis presented a significantly increased relative abundance and positive detection rate of Fusobacterium in nonresponders compared to responders (125). In a recent clinical study, Agathobacter and Blautia species were proposed as potential biomarkers of outcome in mCRC and NSCLC patients treated with cetuximab and avelumab (126). However, the evidence related to the role of gut microbiome as a prognostic marker of colon cancer immunotherapy is still lacking, and further investigations are still required to consider the gut microbiome as a predictive biomarker for the immunotherapy response in colon cancer.
The testing method of gut microbiome detection also needs to be optimized and unified. Current methods for testing the gut microbiome are mainly stool-based genetic tests. The two commonly used methods are PCR-based 16S amplicon sequencing and macrogenome sequencing. PCR-based sequencing of 16S amplicons is relatively less costly, however this method is limited to the genus level and can easily miss microbiomes with low expression levels. In contrast, macrogenomic sequencing has several potential advantages over 16S amplicon sequencing. Macrogenomic sequencing can extend gut microbiome taxonomic resolution to the species level and can also provide information on metabolic pathways of the microbiome. However, the cost of macrogenomic sequencing is relatively high, and the interpretation of analysis results is complex (127). Measurement differences may exist between the two methods due to differences in stool sample collection, storage, and handling, as well as nucleic acid extraction protocols and data analysis methods. Therefore, to make better use of gut microbiota as the predictive biomarker for immunotherapy of colon cancer, it is also necessary to further optimize the testing methods and standardize the testing criteria.
Peripheral blood biomarkers in colon cancer immunotherapy
CtDNA is the most used peripheral blood biomarker. Previously, ctDNA already presented high sensitivity in colon cancer early diagnosis, recurrence detection, and treatment outcome prediction (176, 177). CtDNA is derived from apoptotic and necrotic tumor cells that release their fragmented DNA into circulation. Information on genetic variation could be detected through ctDNA test (178). In 2017, Cabel et al. proposed a proof-of-concept study, they enrolled patients with NSCLC, uveal melanoma, or MSI CRC who were treated by nivolumab or pembrolizumab monotherapy, their results demonstrated that quantitative ctDNA monitoring can be used as a valuable tool to assess tumor patients’ response to anti-PD-1 agents (179). Several studies also focused on the predictive value of ctDNA in colon cancer immunotherapy. Wang et al. proposed ctDNA can be used as a powerful tool for predicting MSS CRC patients’ response to regorafenib and nivolumab combination therapy (180). Gong et al. through four cases illustrated that ctDNA can be used as a dynamic predictive biomarker for colon cancer immunotherapy (128). In the CAVE trial, cetuximab and avelumab combination therapy were tested in RAS wild-type mCRC. In this study, patients’ KRAS, NRAS, BRAF, and EGFR-S492R mutation was analyzed through ctDNA, and the result show patients with RAS/BRAF WT ctDNA presented with better mOS and mPFS compared to patients with mutated ctDNA. These findings presented the potential role of ctDNA for colon cancer immunotherapy (181). However, the reports of ctDNA in the immunotherapy of colon cancer are still limited and ctDNA’s predictive role in immunotherapy needs to be verified by larger clinical studies. In addition, the following questions also need to be paid attention to in the further study of ctDNA (129, 182). First, the concentration of ctDNA in blood is relatively low. Thus, the sensitivity of the detection method is strictly required. Second, ctDNA is vulnerable to a variety of factors, such as trauma, which is a crucial factor affecting the determination of ctDNA. Therefore, strict avoidance of interfering factors is important for accurate measurement of ctDNA. Third, ctDNA is dynamically changing, thus the ctDNA results from different studies sampled at different points in time are difficult to unify and quantify, and there is yet to be a uniform detection standard for ctDNA. Furthermore, the testing method for blood ctDNA still needs to be optimized. Digital PCR (dPCR), amplification refractory mutation system (ARMS), and NGS are currently the main available methods for the detection of ctDNA. Each method has its strengths and limitations. dPCR with a low cost and relatively high sensitivity is the most used method for ctDNA detection. But the limitations of the dPCR are low throughput and the inability to detect unknown mutations. ARMS is moderate in cost, simple to operate, and can sequentially detect multiple mutations in a single gene, but it is not as sensitive as dPCR. The advantages of the NGS method lie mainly in the high throughput and sequencing of unknown mutations, but its economic cost is relatively high. Moreover, some study proposed the optimization of ctDNA detection (183). With the optimization of detection methods and the uniformization of standards, the value of ctDNA in immunotherapy of colon cancer will be better demonstrated.
In addition to ctDNA, various immune cells can also be tested in the peripheral blood, including T cells, B cells, NK cells, and myeloid cells (184). These circulating immune cells are proposed as predictive biomarkers for therapeutic response and clinical benefit of ICIs in solid cancer patients (185, 186). However, based on the existing study, the role of circulating immune cells in colon cancer immunotherapy is controversial. In a phase II study of durvalumab and tremelimumab with concurrent radiotherapy for pMMR mCRC patients, an increase in circulating CD8+T lymphocyte activation was observed in patients with an objective response. However, this combination of radiotherapy plus ICI did not meet the study endpoint criteria (187). In another study of mFOLFOX6 combined bevacizumab alone or with AdCEA vaccine combined avelumab immunotherapy for untreated mCRC, combination therapy generated brachyury-specific CD4+/CD8+T cells but did not improve patients’ PFS (130). In Cheng et al’s study, the peripheral blood of dMMR mCRC patients receiving anti-PD-1 immunotherapy was analyzed, the results show that the ratio of CD4+/CD8+ in peripheral blood and the frequency of CD4+ T cells are promising predictive biomarkers for dMMR mCRC patients responding to immunotherapy (131). According to Herting CJ et al’s study, the baseline levels and changes in circulating immunosuppressive myeloid and T cell subsets were not associated with advanced CRC patients’ response to pembrolizumab combined modified FOLFOX6 therapy (132). Moreover, according to Clouthier et al’s study, they found that the immune biomarkers were significantly varied between the blood and tissue (131). The reasons for this phenomenon are mainly related to the small sample size included in the existing studies and, similar to the reports of ctDNA, the different sampling times and analysis methods can also have an impact on the results. Test methods for the detection of various immune cell subsets including multi-color fluorescence flow cytometry, mass cytometry, and NGS, are still developing, and evaluation criteria need to be normalized (133). In addition, a larger population-based cohort study is necessary to further test the value of circulating tumor cells in immunotherapy of colon cancer.
Inflammation also plays an essential role in colon cancer tumorigenesis and influences patients’ immunotherapy response (188). Lymphocytes and neutrophils are two common indicators of the inflammatory state of the body in the peripheral blood (189). Some studies reported the predictive role of neutrophil-lymphocyte ratio (NLR) in colon cancer immunotherapy (134, 190, 191). In Saied et al’s study, they proposed that NLR changes correlated with CEA+ liver metastases CRC patients’ early responses to chimeric antigen receptor-modified T-cell (CAR-T) hepatic artery infusions (HAI) variations. Increased NLR levels were proven to be associated with poor responses following CAR-T HAI (190). The final results of the CAVE trial show that a baseline NLR <3 significantly correlated with improved survival of ctDNA RAS/BRAF WT patients after cetuximab plus avelumab therapy (191). Furthermore, through a retrospective study, Corti et al. proposed a blood-based biomarker, Pan-Immune-Inflammation Value which integrates neutrophil, platelet, monocyte, and lymphocyte counts, as a strong predictor of outcomes in MSI-H mCRC patients receiving ICIs (192). However, based on the limited number of studies conducted so far, the role of peripheral inflammatory cell-related involvement (e.g., NLR) in the immunotherapy of colon cancer remains to be further demonstrated.
In summary, tissue-based predictive biomarkers are more accurate and closely related to TME. However, there are still some challenges in some clinical situations. Tissue biopsies are invasive, and may be difficult to obtain in advanced and metastatic patients. In addition, intratumoral heterogeneity is prevalent among tumor tissues. Multipoint sampling is necessary to obtain more accurate results, but it is difficult to achieve in clinical settings, as it requires invasive procedures for the patient (193). Under such conditions, liquid biopsies through peripheral blood provide a method with minimally invasive, reproducible sampling and dynamically observe changes in indicators. The variety of information that can be acquired through liquid biopsy includes inflammatory cells, ctDNA, circulating immune cells, cytokines, and so on. Several peripheral blood biomarkers are now being proposed as predictive biomarkers for colon cancer immunotherapy response in existing clinical studies (Table 1). The development of high-throughput sequencing technology provides a deeper and broader view of peripheral blood biomarkers. However, since various multiplexed assays are employed for peripheral blood analyses, these assay protocols and their reporting methods need to be standardized, and additional studies will also be needed on the sampling time points, sensitivity, and specificity of each assay for clinical applicability. In further clinical studies, peripheral blood biomarkers will be developed as dynamic indicators for colon cancer immunotherapy.
Conclusions and perspectives
In recent years, ICI-based immunotherapy has brought revolutionary breakthroughs in the treatment of colon cancer. While immunotherapeutic agents continue to be researched and developed, it is also worth focusing on precisely screening beneficial patients through predictive biomarkers. Currently, MSI is the only approved biomarker for screening colon cancer immunotherapy-benefiting patients. However, the results of existing clinical studies indicated the low efficacy of MSI as a predictive biomarker for colon cancer immunotherapy. Several new predictive biomarkers have been proposed in colon cancer immunotherapy. Developments have also been made in the detection method of predictive biomarkers for immunotherapy of colon cancer. In this review, we summarized the currently reported predictive biomarkers in existing studies of colon cancer immunotherapy.
We concluded that immunotherapeutic biomarkers reported in the clinical studies for colon cancer can be divided into four main categories (Figure 1), the first category of biomarkers related to genetic alterations, such as MSI, TMB, and POLE/POLD1; the second category of biomolecular markers related to TME, mainly included TILs, PD-L1 expression, TLS, and CAF related genes; the third category is certain specific gut microbiome; the fourth category is peripheral blood biomarkers, such as ctDNA, bTMB, circulating immune cells, and inflammatory cell related indicators. Based on currently reported clinical studies (Table 1), only MSI’s predictive role in colon cancer immunotherapy has been demonstrated in a larger cohort. The other immunotherapeutic biomarkers have only been reported in some small cohorts of colon cancer and are pending justification in larger cohorts. Some predictive biomarkers for colon cancer immunotherapy come from database analysis or retrospective studies, also waiting to be demonstrated by large cohort clinical studies. In addition, despite the attention given to the TME and gut microbiota in colon cancer immunotherapy, reliable biomarkers for colon cancer immunotherapy beneficial population selection are still lacking. Peripheral blood markers have been favored by many studies in recent years due to their non-invasive and multi-sampling advantages. However, a unified evaluation criterion is yet to be established. Furthermore, the testing methods of each biomarker are all waiting to be optimized to obtain more accurate testing results, and a unified judgment standard must be developed.
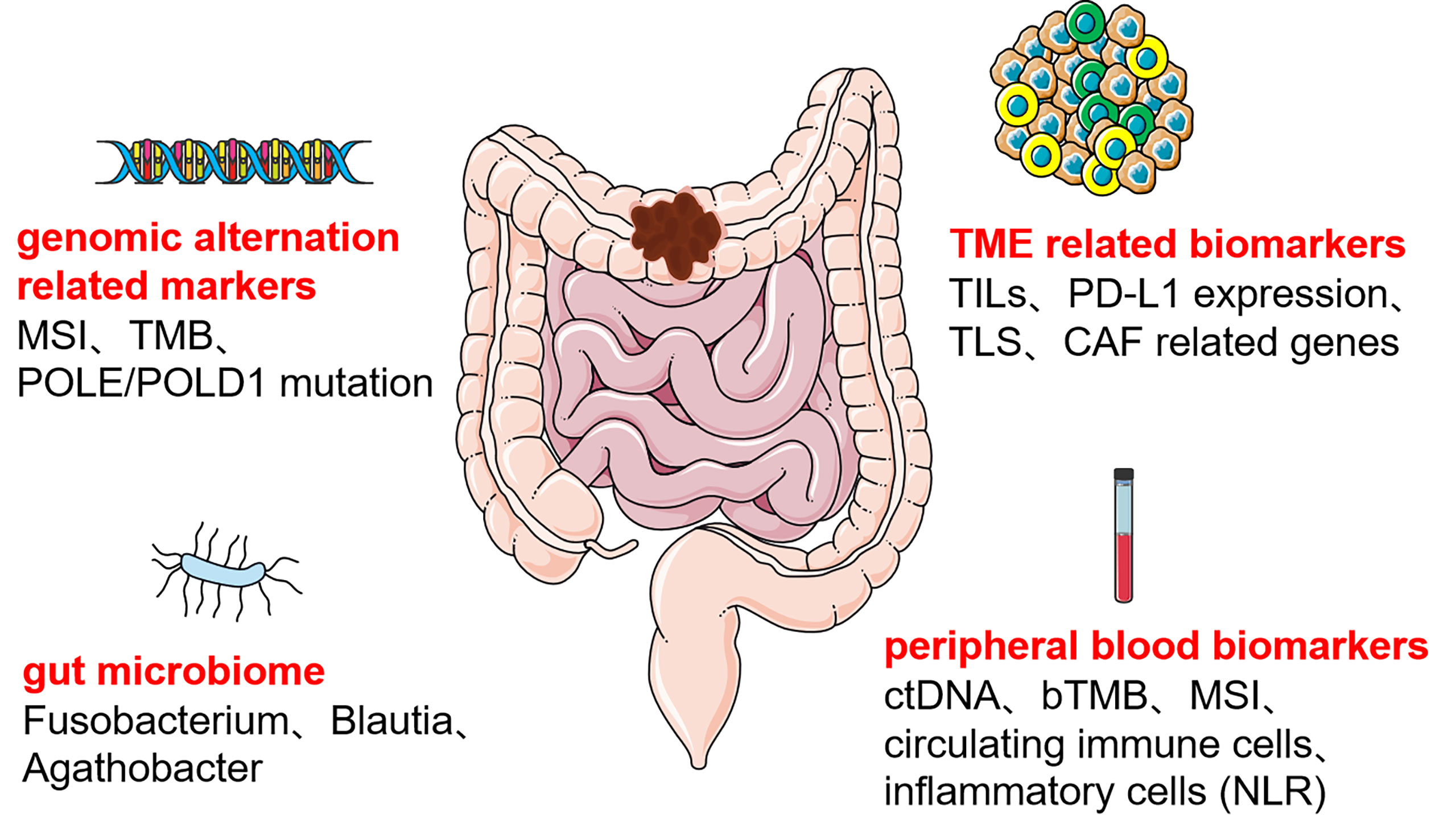
Figure 1 Predictive biomarkers of colon cancer immunotherapy MSI, microsatellite instability; TMB, total mutation burden; POLE:/POLD1, DNA polymerase ϵ (POLE) and δ (POLD1); TILs, tumor-infiltrating lymphocytes; PD-L1, programmed cell death-ligand 1; TLS, tertiary lymphoid structure; CAF, cancer-associated fibroblast; ctDNA, circulating tumor DNA; bTMB, blood total mutation burden; NLR, neutrophil-lymphocyte ratio.
In summary, there is no optimal predictive biomarker for immunotherapy of colon cancer till now, and each biomarker has its limitations. Although the combined application of multi-methods for recognizing multi-indicators could improve the accuracy of biomarkers for colon cancer immunotherapy, large numbers of clinical trials are needed to verify that. With the optimization and improvement of the technology, more accurate biomarkers for predicting immunotherapy of colon cancer will help to stratify patients, which will also greatly improve the prognosis and the overall survival rate of patients
Author contributions
(I) Conception and design: WH, CY, HZ; (II) Administrative support: CY; (III) Provision of study materials or patients: CY, HZ; (IV) Collection and assembly of data: WH, CY, HZ; (V) Manuscript writing: WH, CY, HZ. All authors contributed to the article and approved the submitted version.
Funding
This study was supported by grants from the Key research projects of Science & Technology of Sichuan Province (2022YFS0189) and the Fundamental Research Funds for the Central Universities (2022SCU12058).
Conflict of interest
The authors declare that the research was conducted in the absence of any commercial or financial relationships that could be construed as a potential conflict of interest.
Publisher’s note
All claims expressed in this article are solely those of the authors and do not necessarily represent those of their affiliated organizations, or those of the publisher, the editors and the reviewers. Any product that may be evaluated in this article, or claim that may be made by its manufacturer, is not guaranteed or endorsed by the publisher.
References
1. Siegel RL, Miller KD, Fuchs HE, Jemal A. Cancer statistics, 2022. CA: Cancer J Clin (2022) 72(1):7–33. doi: 10.3322/caac.21708
2. Dienstmann R, Salazar R, Tabernero J. Personalizing colon cancer adjuvant therapy: selecting optimal treatments for individual patients. J Clin Oncol Off J Am Soc Clin Oncol (2015) 33(16):1787–96. doi: 10.1200/JCO.2014.60.0213
3. Wu C. Systemic therapy for colon cancer. Surg Oncol Clinics North America. (2018) 27(2):235–42. doi: 10.1016/j.soc.2017.11.001
4. André T, Shiu KK, Kim TW, Jensen BV, Jensen LH, Punt C, et al. Pembrolizumab in microsatellite-Instability-High advanced colorectal cancer. New Engl J Med (2020) 383(23):2207–18. doi: 10.1056/NEJMoa2017699
5. Lenz HJ, Van Cutsem E, Luisa Limon M, Wong KYM, Hendlisz A, Aglietta M, et al. First-line nivolumab plus low-dose ipilimumab for microsatellite instability-High/Mismatch repair-deficient metastatic colorectal cancer: The phase II CheckMate 142 study. J Clin Oncol (2022) 40(2):161–70. doi: 10.1200/JCO.21.01015
6. Cohen R, Bennouna J, Meurisse A, Tournigand C, de la Fouchardière C, Tougeron D, et al. RECIST and iRECIST criteria for the evaluation of nivolumab plus ipilimumab in patients with microsatellite instability-high/mismatch repair-deficient metastatic colorectal cancer: the GERCOR NIPICOL phase II study. J Immunother Cancer. (2020) 8(2):e001499. doi: 10.1136/jitc-2020-001499
7. Chalabi M, Fanchi LF, Dijkstra KK, Van den Berg JG, Aalbers AG, Sikorska K, et al. Neoadjuvant immunotherapy leads to pathological responses in MMR-proficient and MMR-deficient early-stage colon cancers. Nat Med (2020) 26(4):566–76. doi: 10.1038/s41591-020-0805-8
8. Li SKH, Martin A. Mismatch repair and colon cancer: Mechanisms and therapies explored. Trends Mol Med (2016) 22(4):274–89. doi: 10.1016/j.molmed.2016.02.003
9. Viale G, Trapani D, Curigliano G. Mismatch repair deficiency as a predictive biomarker for immunotherapy efficacy. BioMed Res Int (2017) 2017:4719194. doi: 10.1155/2017/4719194
10. Timmermann B, Kerick M, Roehr C, Fischer A, Isau M, Boerno ST, et al. Somatic mutation profiles of MSI and MSS colorectal cancer identified by whole exome next generation sequencing and bioinformatics analysis. PloS One (2010) 5(12):e15661. doi: 10.1371/journal.pone.0015661
11. Le DT, Uram JN, Wang H, Bartlett BR, Kemberling H, Eyring AD, et al. PD-1 blockade in tumors with mismatch-repair deficiency. New Engl J Med (2015) 372(26):2509–20. doi: 10.1056/NEJMoa1500596
12. Le DT, Durham JN, Smith KN, Wang H, Bartlett BR, Aulakh LK, et al. Mismatch repair deficiency predicts response of solid tumors to PD-1 blockade. Science. (2017) 357(6349):409–13. doi: 10.1126/science.aan6733
13. Overman MJ, McDermott R, Leach JL, Lonardi S, Lenz HJ, Morse MA, et al. Nivolumab in patients with metastatic DNA mismatch repair-deficient or microsatellite instability-high colorectal cancer (CheckMate 142): an open-label, multicentre, phase 2 study. Lancet Oncol (2017) 18(9):1182–91. doi: 10.1016/S1470-2045(17)30422-9
14. Le DT, Kim TW, Van Cutsem E, Geva R, Jäger D, Hara H, et al. Phase II open-label study of pembrolizumab in treatment-refractory, microsatellite instability-High/Mismatch repair-deficient metastatic colorectal cancer: KEYNOTE-164. J Clin Oncol Off J Am Soc Clin Oncol (2020) 38(1):11–9. doi: 10.1200/JCO.19.02107
15. Fujiyoshi K, Yamamoto G, Takenoya T, Takahashi A, Arai Y, Yamada M, et al. Metastatic pattern of stage IV colorectal cancer with high-frequency microsatellite instability as a prognostic factor. Anticancer Res (2017) 37(1):239–47. doi: 10.21873/anticanres.11313
16. Amodio V, Mauri G, Reilly NM, Sartore-Bianchi A, Siena S, Bardelli A, et al. Mechanisms of immune escape and resistance to checkpoint inhibitor therapies in mismatch repair deficient metastatic colorectal cancers. Cancers (2021) 13(11):2638. doi: 10.3390/cancers13112638
17. Sahin IH, Akce M, Alese O, Shaib W, Lesinski GB, El-Rayes B, et al. Immune checkpoint inhibitors for the treatment of MSI-H/MMR-D colorectal cancer and a perspective on resistance mechanisms. Br J cancer. (2019) 121(10):809–18. doi: 10.1038/s41416-019-0599-y
18. Cohen R, Hain E, Buhard O, Guilloux A, Bardier A, Kaci R, et al. Association of primary resistance to immune checkpoint inhibitors in metastatic colorectal cancer with misdiagnosis of microsatellite instability or mismatch repair deficiency status. JAMA Oncol (2019) 5(4):551–5. doi: 10.1001/jamaoncol.2018.4942
19. Wang C, Sandhu J, Ouyang C, Ye J, Lee PP, Fakih M. Clinical response to immunotherapy targeting programmed cell death receptor 1/Programmed cell death ligand 1 in patients with treatment-resistant microsatellite stable colorectal cancer with and without liver metastases. JAMA network Open (2021) 4(8):e2118416. doi: 10.1001/jamanetworkopen.2021.18416
20. Li Y, Liang L, Dai W, Cai G, Xu Y, Li X, et al. Prognostic impact of programed cell death-1 (PD-1) and PD-ligand 1 (PD-L1) expression in cancer cells and tumor infiltrating lymphocytes in colorectal cancer. Mol cancer. (2016) 15(1):55. doi: 10.1186/s12943-016-0539-x
21. Schrock AB, Ouyang C, Sandhu J, Sokol E, Jin D, Ross JS, et al. Tumor mutational burden is predictive of response to immune checkpoint inhibitors in MSI-high metastatic colorectal cancer. Ann Oncol (2019) 30(7):1096–103. doi: 10.1093/annonc/mdz134
22. Zou Q, Wang X, Ren D, Hu B, Tang G, Zhang Y, et al. DNA Methylation-based signature of CD8+ tumor-infiltrating lymphocytes enables evaluation of immune response and prognosis in colorectal cancer. J Immunother Cancer (2021) 9(9):e002671. doi: 10.1136/jitc-2021-002671
23. Temraz S, Nassar F, Nasr R, Charafeddine M, Mukherji D, Shamseddine A. Gut microbiome: A promising biomarker for immunotherapy in colorectal cancer. Int J Mol Sci (2019) 20(17):4155. doi: 10.3390/ijms20174155
24. Zeng Z, Yang B, Liao Z. Biomarkers in immunotherapy-based precision treatments of digestive system tumors. Front Oncol (2021) 11:650481. doi: 10.3389/fonc.2021.650481
25. Wang C, Tang Y, Ma H, Wei S, Hu X, Zhao L, et al. Identification of hypoxia-related subtypes, establishment of prognostic models, and characteristics of tumor microenvironment infiltration in colon cancer. Front Genet (2022) 13:919389. doi: 10.3389/fgene.2022.919389
26. El Sissy C, Kirilovsky A, Zeitoun G, Marliot F, Haicheur N, Lagorce-Pagès C, et al. Therapeutic implications of the immunoscore in patients with colorectal cancer. Cancers (Basel) (2021) 13(6):1281. doi: 10.3390/cancers13061281
27. Hu F, Wang J, Zhang M, Wang S, Zhao L, Yang H, et al. Comprehensive analysis of subtype-specific molecular characteristics of colon cancer: Specific genes, driver genes, signaling pathways, and immunotherapy responses. Front Cell Dev Biol (2021) 9:758776. doi: 10.3389/fcell.2021.758776
28. Xu Y, Cao C, Zhu Z, Wang Y, Tan Y, Xu X. Novel hypoxia-associated gene signature depicts tumor immune microenvironment and predicts prognosis of colon cancer patients. Front Genet (2022) 13:901734. doi: 10.3389/fgene.2022.901734
29. Zheng H, Liu H, Ge Y, Wang X. Integrated single-cell and bulk RNA sequencing analysis identifies a cancer associated fibroblast-related signature for predicting prognosis and therapeutic responses in colorectal cancer. Cancer Cell Int (2021) 21(1):552. doi: 10.1186/s12935-021-02252-9
30. Thibodeau SN, Bren G, Schaid D. Microsatellite instability in cancer of the proximal colon. Science. (1993) 260(5109):816–9. doi: 10.1126/science.8484122
31. Gonzalez-Zulueta M, Ruppert JM, Tokino K, Tsai YC, Spruck CH, Miyao N 3rd, et al. Microsatellite instability in bladder cancer. Cancer Res (1993) 53(23):5620–3.
32. Peltomäki P, Lothe RA, Aaltonen LA, Pylkkänen L, Nyström-Lahti M, Seruca R, et al. Microsatellite instability is associated with tumors that characterize the hereditary non-polyposis colorectal carcinoma syndrome. Cancer Res (1993) 53(24):5853–5.
33. Rhyu MG, Park WS, Meltzer SJ. Microsatellite instability occurs frequently in human gastric carcinoma. Oncogene. (1994) 9(1):29–32.
34. Burks RT, Kessis TD, Cho KR, Hedrick L. Microsatellite instability in endometrial carcinoma. Oncogene. (1994) 9(4):1163–6.
35. Yee CJ, Roodi N, Verrier CS, Parl FF. Microsatellite instability and loss of heterozygosity in breast cancer. Cancer Res (1994) 54(7):1641–4.
36. van Velzen MJM, Derks S, van Grieken NCT, Haj Mohammad N, van Laarhoven HWM. MSI as a predictive factor for treatment outcome of gastroesophageal adenocarcinoma. Cancer Treat Rev (2020) 86:102024. doi: 10.1016/j.ctrv.2020.102024
37. McCarthy AJ, Capo-Chichi JM, Spence T, Grenier S, Stockley T, Kamel-Reid S, et al. Heterogenous loss of mismatch repair (MMR) protein expression: a challenge for immunohistochemical interpretation and microsatellite instability (MSI) evaluation. J Pathol Clin Res (2019) 5(2):115–29. doi: 10.1002/cjp2.120
38. Taieb J, Shi Q, Pederson L, Alberts S, Wolmark N, Van Cutsem E, et al. Prognosis of microsatellite instability and/or mismatch repair deficiency stage III colon cancer patients after disease recurrence following adjuvant treatment: results of an ACCENT pooled analysis of seven studies. Ann Oncol Off J Eur Soc Med Oncol (2019) 30(9):1466–71. doi: 10.1093/annonc/mdz208
39. Roth AD, Delorenzi M, Tejpar S, Yan P, Klingbiel D, Fiocca R, et al. Integrated analysis of molecular and clinical prognostic factors in stage II/III colon cancer. J Natl Cancer Institute. (2012) 104(21):1635–46. doi: 10.1093/jnci/djs427
40. Sargent DJ, Marsoni S, Monges G, Thibodeau SN, Labianca R, Hamilton SR, et al. Defective mismatch repair as a predictive marker for lack of efficacy of fluorouracil-based adjuvant therapy in colon cancer. J Clin Oncol Off J Am Soc Clin Oncol (2010) 28(20):3219–26. doi: 10.1200/JCO.2009.27.1825
41. Lipson EJ, Sharfman WH, Drake CG, Wollner I, Taube JM, Anders RA, et al. Durable cancer regression off-treatment and effective reinduction therapy with an anti-PD-1 antibody. Clin Cancer Res an Off J Am Assoc Cancer Res (2013) 19(2):462–8. doi: 10.1158/1078-0432.CCR-12-2625
42. Huyghe N, Baldin P, Van den Eynde M. Immunotherapy with immune checkpoint inhibitors in colorectal cancer: what is the future beyond deficient mismatch-repair tumours? Gastroenterol Rep (2020) 8(1):11–24. doi: 10.1093/gastro/goz061
43. Amato M, Franco R, Facchini G, Addeo R, Ciardiello F, Berretta M, et al. Microsatellite instability: From the implementation of the detection to a prognostic and predictive role in cancers. Int J Mol Sci (2022) 23(15):8726. doi: 10.3390/ijms23158726
44. Rodriguez-Bigas MA, Boland CR, Hamilton SR, Henson DE, Jass JR, Khan PM, et al. A national cancer institute workshop on hereditary nonpolyposis colorectal cancer syndrome: meeting highlights and Bethesda guidelines. J Natl Cancer Institute. (1997) 89(23):1758–62. doi: 10.1093/jnci/89.23.1758
45. Suraweera N, Duval A, Reperant M, Vaury C, Furlan D, Leroy K, et al. Evaluation of tumor microsatellite instability using five quasimonomorphic mononucleotide repeats and pentaplex PCR. Gastroenterology. (2002) 123(6):1804–11. doi: 10.1053/gast.2002.37070
46. Bai W, Ma J, Liu Y, Liang J, Wu Y, Yang X, et al. Screening of MSI detection loci and their heterogeneity in East Asian colorectal cancer patients. Cancer Med (2019) 8(5):2157–66. doi: 10.1002/cam4.2111
47. Luchini C, Bibeau F, Ligtenberg MJL, Singh N, Nottegar A, Bosse T, et al. ESMO recommendations on microsatellite instability testing for immunotherapy in cancer, and its relationship with PD-1/PD-L1 expression and tumour mutational burden: a systematic review-based approach. Ann Oncol Off J Eur Soc Med Oncol (2019) 30(8):1232–43. doi: 10.1093/annonc/mdz116
48. Shin SJ, Kim SY, Choi YY, Son T, Cheong JH, Hyung WJ, et al. Mismatch repair status of gastric cancer and its association with the local and systemic immune response. oncologist. (2019) 24(9):e835–e44. doi: 10.1634/theoncologist.2018-0273
49. Lizardo DY, Kuang C, Hao S, Yu J, Huang Y, Zhang L. Immunotherapy efficacy on mismatch repair-deficient colorectal cancer: From bench to bedside. Biochim Biophys Acta Rev cancer. (2020) 1874(2):188447. doi: 10.1016/j.bbcan.2020.188447
50. Lin A, Zhang J, Luo P. Crosstalk between the MSI status and tumor microenvironment in colorectal cancer. Front Immunol (2020) 11:2039. doi: 10.3389/fimmu.2020.02039
51. Chan TA, Yarchoan M, Jaffee E, Swanton C, Quezada SA, Stenzinger A, et al. Development of tumor mutation burden as an immunotherapy biomarker: utility for the oncology clinic. Ann Oncol Off J Eur Soc Med Oncol (2019) 30(1):44–56. doi: 10.1093/annonc/mdy495
52. Marabelle A, Fakih M, Lopez J, Shah M, Shapira-Frommer R, Nakagawa K, et al. Association of tumour mutational burden with outcomes in patients with advanced solid tumours treated with pembrolizumab: prospective biomarker analysis of the multicohort, open-label, phase 2 KEYNOTE-158 study. Lancet Oncol (2020) 21(10):1353–65. doi: 10.1016/S1470-2045(20)30445-9
53. Pan D, Hu AY, Antonia SJ, Li CY. A gene mutation signature predicting immunotherapy benefits in patients with NSCLC. J Thorac Oncol Off Publ Int Assoc Study Lung Cancer. (2021) 16(3):419–27. doi: 10.1016/j.jtho.2020.11.021
54. Kang K, Xie F, Mao J, Bai Y, Wang X. Significance of tumor mutation burden in immune infiltration and prognosis in cutaneous melanoma. Front Oncol (2020) 10:573141. doi: 10.3389/fonc.2020.573141
55. Innocenti F, Ou FS, Qu X, Zemla TJ, Niedzwiecki D, Tam R, et al. Mutational analysis of patients with colorectal cancer in CALGB/SWOG 80405 identifies new roles of microsatellite instability and tumor mutational burden for patient outcome. J Clin Oncol Off J Am Soc Clin Oncol (2019) 37(14):1217–27. doi: 10.1200/JCO.18.01798
56. Bortolomeazzi M, Keddar MR, Montorsi L, Acha-Sagredo A, Benedetti L, Temelkovski D, et al. Immunogenomics of colorectal cancer response to checkpoint blockade: Analysis of the KEYNOTE 177 trial and validation cohorts. Gastroenterology. (2021) 161(4):1179–93. doi: 10.1053/j.gastro.2021.06.064
57. Chen EX, Jonker DJ, Loree JM, Kennecke HF, Berry SR, Couture F, et al. Effect of combined immune checkpoint inhibition vs best supportive care alone in patients with advanced colorectal cancer: The Canadian cancer trials group CO. 26 Study. JAMA Oncol (2020) 6(6):831–8. doi: 10.1001/jamaoncol.2020.0910
58. McGrail DJ, Pilié PG, Rashid NU, Voorwerk L, Slagter M, Kok M, et al. High tumor mutation burden fails to predict immune checkpoint blockade response across all cancer types. Ann Oncol Off J Eur Soc Med Oncol (2021) 32(5):661–72. doi: 10.1016/j.annonc.2021.02.006
59. Friedman CF, Hainsworth JD, Kurzrock R, Spigel DR, Burris HA, Sweeney CJ, et al. Atezolizumab treatment of tumors with high tumor mutational burden from MyPathway, a multicenter, open-label, phase IIa multiple basket study. Cancer discovery. (2022) 12(3):654–69. doi: 10.1158/2159-8290.CD-21-0450
60. Jardim DL, Goodman A, de Melo Gagliato D, Kurzrock R. The challenges of tumor mutational burden as an immunotherapy biomarker. Cancer Cell (2021) 39(2):154–73. doi: 10.1016/j.ccell.2020.10.001
61. Strickler JH, Hanks BA, Khasraw M. Tumor mutational burden as a predictor of immunotherapy response: Is more always better? Clin Cancer Res an Off J Am Assoc Cancer Res (2021) 27(5):1236–41. doi: 10.1158/1078-0432.CCR-20-3054
62. Fancello L, Gandini S, Pelicci PG, Mazzarella L. Tumor mutational burden quantification from targeted gene panels: major advancements and challenges. J immunotherapy cancer. (2019) 7(1):183. doi: 10.1186/s40425-019-0647-4
63. Picard E, Verschoor CP, Ma GW, Pawelec G. Relationships between immune landscapes, genetic subtypes and responses to immunotherapy in colorectal cancer. Front Immunol (2020) 11:369. doi: 10.3389/fimmu.2020.00369
64. Sveen A, Kopetz S, Lothe RA. Biomarker-guided therapy for colorectal cancer: strength in complexity. Nat Rev Clin Oncol (2020) 17(1):11–32. doi: 10.1038/s41571-019-0241-1
65. Mur P, García-Mulero S, Del Valle J, Magraner-Pardo L, Vidal A, Pineda M, et al. Role of POLE and POLD1 in familial cancer. Genet Med (2020) 22(12):2089–100. doi: 10.1038/s41436-020-0922-2
66. Wang F, Zhao Q, Wang YN, Jin Y, He MM, Liu ZX, et al. Evaluation of POLE and POLD1 mutations as biomarkers for immunotherapy outcomes across multiple cancer types. JAMA Oncol (2019) 5(10):1504–6. doi: 10.1001/jamaoncol.2019.2963
67. Kim JH, Kim SY, Baek JY, Cha YJ, Ahn JB, Kim HS, et al. A phase II study of avelumab monotherapy in patients with mismatch repair-Deficient/Microsatellite instability-high or POLE-mutated metastatic or unresectable colorectal cancer. Cancer Res Treat (2020) 52(4):1135–44. doi: 10.4143/crt.2020.218
68. Oh CR, Kim JE, Hong YS, Kim SY, Ahn JB, Baek JY, et al. Phase II study of durvalumab monotherapy in patients with previously treated microsatellite instability-high/mismatch repair-deficient or POLE-mutated metastatic or unresectable colorectal cancer. Int J Cancer. (2022) 150(12):2038–45. doi: 10.1002/ijc.33966
69. Hamarsheh S, Groß O, Brummer T, Zeiser R. Immune modulatory effects of oncogenic KRAS in cancer. Nat Commun (2020) 11(1):5439. doi: 10.1038/s41467-020-19288-6
70. Lal N, White BS, Goussous G, Pickles O, Mason MJ, Beggs AD, et al. And consensus molecular subtypes 2 and 3 are independently associated with reduced immune infiltration and reactivity in colorectal cancer. Clin Cancer Res (2018) 24(1):224–33. doi: 10.1158/1078-0432.CCR-17-1090
71. Guinney J, Dienstmann R, Wang X, de Reyniès A, Schlicker A, Soneson C, et al. The consensus molecular subtypes of colorectal cancer. Nat Med (2015) 21(11):1350–6. doi: 10.1038/nm.3967
72. Ten Hoorn S, de Back TR, Sommeijer DW, Vermeulen L. Clinical value of consensus molecular subtypes in colorectal cancer: A systematic review and meta-analysis. J Natl Cancer Institute. (2022) 114(4):503–16. doi: 10.1093/jnci/djab106
73. Dienstmann R, Vermeulen L, Guinney J, Kopetz S, Tejpar S, Tabernero J. Consensus molecular subtypes and the evolution of precision medicine in colorectal cancer. Nat Rev Cancer. (2017) 17(2):79–92. doi: 10.1038/nrc.2016.126
74. Rodriguez-Salas N, Dominguez G, Barderas R, Mendiola M, García-Albéniz X, Maurel J, et al. Clinical relevance of colorectal cancer molecular subtypes. Crit Rev oncology/hematology. (2017) 109:9–19. doi: 10.1016/j.critrevonc.2016.11.007
75. Becht E, de Reyniès A, Giraldo NA, Pilati C, Buttard B, Lacroix L, et al. Immune and stromal classification of colorectal cancer is associated with molecular subtypes and relevant for precision immunotherapy. Clin Cancer Res an Off J Am Assoc Cancer Res (2016) 22(16):4057–66. doi: 10.1158/1078-0432.CCR-15-2879
76. Chida K, Kawazoe A, Suzuki T, Kawazu M, Ueno T, Takenouchi K, et al. Transcriptomic profiling of MSI-H/dMMR gastrointestinal tumors to identify determinants of responsiveness to anti-PD-1 therapy. Clin Cancer Res (2022) 28(10):2110–7. doi: 10.1158/1078-0432.CCR-22-0041
77. Fontana E, Eason K, Cervantes A, Salazar R, Sadanandam A. Context matters-consensus molecular subtypes of colorectal cancer as biomarkers for clinical trials. Ann Oncol Off J Eur Soc Med Oncol (2019) 30(4):520–7. doi: 10.1093/annonc/mdz052
78. Dunne PD, McArt DG, Bradley CA, O'Reilly PG, Barrett HL, Cummins R, et al. Challenging the cancer molecular stratification dogma: Intratumoral heterogeneity undermines consensus molecular subtypes and potential diagnostic value in colorectal cancer. Clin Cancer Res an Off J Am Assoc Cancer Res (2016) 22(16):4095–104. doi: 10.1158/1078-0432.CCR-16-0032
79. Marisa L, Blum Y, Taieb J, Ayadi M, Pilati C, Le Malicot K, et al. Intratumor CMS heterogeneity impacts patient prognosis in localized colon cancer. Clin Cancer Res an Off J Am Assoc Cancer Res (2021) 27(17):4768–80. doi: 10.1158/1078-0432.CCR-21-0529
80. Joanito I, Wirapati P, Zhao N, Nawaz Z, Yeo G, Lee F, et al. Single-cell and bulk transcriptome sequencing identifies two epithelial tumor cell states and refines the consensus molecular classification of colorectal cancer. Nat Genet (2022) 54(7):963–75. doi: 10.1038/s41588-022-01100-4
81. Khaliq AM, Erdogan C, Kurt Z, Turgut SS, Grunvald MW, Rand T, et al. Refining colorectal cancer classification and clinical stratification through a single-cell atlas. Genome Biol (2022) 23(1):113. doi: 10.1186/s13059-022-02677-z
82. Bader JE, Voss K, Rathmell JC. Targeting metabolism to improve the tumor microenvironment for cancer immunotherapy. Mol Cell (2020) 78(6):1019–33. doi: 10.1016/j.molcel.2020.05.034
83. Trimaglio G, Tilkin-Mariamé AF, Feliu V, Lauzéral-Vizcaino F, Tosolini M, Valle C, et al. Colon-specific immune microenvironment regulates cancer progression versus rejection. Oncoimmunology. (2020) 9(1):1790125. doi: 10.1080/2162402X.2020.1790125
84. Stanton SE, Disis ML. Clinical significance of tumor-infiltrating lymphocytes in breast cancer. J immunotherapy cancer. (2016) 4:59. doi: 10.1186/s40425-016-0165-6
85. Laumont CM, Banville AC, Gilardi M, Hollern DP, Nelson BH. Tumour-infiltrating b cells: immunological mechanisms, clinical impact and therapeutic opportunities. Nat Rev Cancer. (2022) 22(7):414–30. doi: 10.1038/s41568-022-00466-1
86. Zheng M, Li YM, Liu ZY, Zhang X, Zhou Y, Jiang JL, et al. Prognostic landscape of tumor-infiltrating T and b cells in human cancer. Front Immunol (2021) 12:731329. doi: 10.3389/fimmu.2021.731329
87. Naito Y, Saito K, Shiiba K, Ohuchi A, Saigenji K, Nagura H, et al. CD8+ T cells infiltrated within cancer cell nests as a prognostic factor in human colorectal cancer. Cancer Res (1998) 58(16):3491–4.
88. Galon J, Costes A, Sanchez-Cabo F, Kirilovsky A, Mlecnik B, Lagorce-Pagès C, et al. Type, density, and location of immune cells within human colorectal tumors predict clinical outcome. Science. (2006) 313(5795):1960–4. doi: 10.1126/science.1129139
89. Foroutan M, Molania R, Pfefferle A, Behrenbruch C, Scheer S, Kallies A, et al. The ratio of exhausted to resident infiltrating lymphocytes is prognostic for colorectal cancer patient outcome. Cancer Immunol Res (2021) 9(10):1125–40. doi: 10.1158/2326-6066.CIR-21-0137
90. Giannakis M, Mu XJ, Shukla SA, Qian ZR, Cohen O, Nishihara R, et al. Genomic correlates of immune-cell infiltrates in colorectal carcinoma. Cell Rep (2016) 15(4):857–65. doi: 10.1016/j.celrep.2016.03.075
91. Roxburgh CS, McMillan DC. The role of the in situ local inflammatory response in predicting recurrence and survival in patients with primary operable colorectal cancer. Cancer Treat Rev (2012) 38(5):451–66. doi: 10.1016/j.ctrv.2011.09.001
92. Loupakis F, Depetris I, Biason P, Intini R, Prete AA, Leone F, et al. Prediction of benefit from checkpoint inhibitors in mismatch repair deficient metastatic colorectal cancer: Role of tumor infiltrating lymphocytes. oncologist. (2020) 25(6):481–7. doi: 10.1634/theoncologist.2019-0611
93. Kanikarla Marie P, Haymaker C, Parra ER, Kim YU, Lazcano R, Gite S, et al. Pilot clinical trial of perioperative durvalumab and tremelimumab in the treatment of resectable colorectal cancer liver metastases. Clin Cancer Res (2021) 27(11):3039–49. doi: 10.1158/1078-0432.CCR-21-0163
94. Johnson B, Haymaker CL, Parra ER, Soto LMS, Wang X, Thomas JV, et al. Phase II study of durvalumab (anti-PD-L1) and trametinib (MEKi) in microsatellite stable (MSS) metastatic colorectal cancer (mCRC). J Immunother Cancer. (2022) 10(8):e005332. doi: 10.1136/jitc-2022-005332
95. Kuang C, Park Y, Augustin RC, Lin Y, Hartman DJ, Seigh L, et al. Pembrolizumab plus azacitidine in patients with chemotherapy refractory metastatic colorectal cancer: a single-arm phase 2 trial and correlative biomarker analysis. Clin Epigenetics. (2022) 14(1):3. doi: 10.1186/s13148-021-01226-y
96. Turksma AW, Coupé VM, Shamier MC, Lam KL, de Weger VA, Belien JA, et al. Extent and location of tumor-infiltrating lymphocytes in microsatellite-stable colon cancer predict outcome to adjuvant active specific immunotherapy. Clin Cancer Res (2016) 22(2):346–56. doi: 10.1158/1078-0432.CCR-13-2462
97. Chakrabarti S, Huebner LJ, Finnes HD, Muranyi A, Clements J, Singh S, et al. Intratumoral CD3+ and CD8+ T-cell densities in patients with DNA mismatch repair–deficient metastatic colorectal cancer receiving programmed cell death-1 blockade. JCO Precis Oncol (2019) 3):1–7. doi: 10.1200/PO.19.00055
98. Pagès F, Mlecnik B, Marliot F, Bindea G, Ou FS, Bifulco C, et al. International validation of the consensus immunoscore for the classification of colon cancer: a prognostic and accuracy study. Lancet (London England). (2018) 391(10135):2128–39. doi: 10.1016/S0140-6736(18)30789-X
99. Stanton SE, Disis ML. Clinical significance of tumor-infiltrating lymphocytes in breast 843 cancer. J immunotherapy cancer. (2016) 4:59. doi: 10.1186/s40425-016-0165-6
100. Mlecnik B, Bindea G, Angell HK, Maby P, Angelova M, Tougeron D, et al. Integrative analyses of colorectal cancer show immunoscore is a stronger predictor of patient survival than microsatellite instability. Immunity. (2016) 44(3):698–711. doi: 10.1016/j.immuni.2016.02.025
101. Bruni D, Angell HK, Galon J. The immune contexture and immunoscore in cancer prognosis and therapeutic efficacy. Nat Rev Cancer. (2020) 20(11):662–80. doi: 10.1038/s41568-020-0285-7
102. de Vries NL, van Unen V, Ijsselsteijn ME, Abdelaal T, van der Breggen R, Farina Sarasqueta A, et al. High-dimensional cytometric analysis of colorectal cancer reveals novel mediators of antitumour immunity. Gut. (2020) 69(4):691–703. doi: 10.1136/gutjnl-2019-318672
103. Bindea G, Mlecnik B, Tosolini M, Kirilovsky A, Waldner M, Obenauf AC, et al. Spatiotemporal dynamics of intratumoral immune cells reveal the immune landscape in human cancer. Immunity. (2013) 39(4):782–95. doi: 10.1016/j.immuni.2013.10.003
104. Saito T, Nishikawa H, Wada H, Nagano Y, Sugiyama D, Atarashi K, et al. Two FOXP3(+)CD4(+) T cell subpopulations distinctly control the prognosis of colorectal cancers. Nat Med (2016) 22(6):679–84. doi: 10.1038/nm.4086
105. Kitsou M, Ayiomamitis GD, Zaravinos A. High expression of immune checkpoints is associated with the TIL load, mutation rate and patient survival in colorectal cancer. Int J Oncol (2020) 57(1):237–48. doi: 10.3892/ijo.2020.5062
106. Jacquelot N, Tellier J, Nutt Sl, Belz Gt. Tertiary lymphoid structures and b lymphocytes in cancer prognosis and response to immunotherapies. Oncoimmunology. (2021) 10(1):1900508. doi: 10.1080/2162402X.2021.1900508
107. Posch F, Silina K, Leibl S, Mündlein A, Moch H, Siebenhüner A, et al. Maturation of tertiary lymphoid structures and recurrence of stage II and III colorectal cancer. Oncoimmunology. (2018) 7(2):e1378844. doi: 10.1080/2162402X.2017.1378844
108. Berntsson J, Nodin B, Eberhard J, Micke P, Jirström K. Prognostic impact of tumour-infiltrating b cells and plasma cells in colorectal cancer. Int J cancer. (2016) 139(5):1129–39. doi: 10.1002/ijc.30138
109. Di Caro G, Bergomas F, Grizzi F, Doni A, Bianchi P, Malesci A, et al. Occurrence of tertiary lymphoid tissue is associated with T-cell infiltration and predicts better prognosis in early-stage colorectal cancers. Clin Cancer Res an Off J Am Assoc Cancer Res (2014) 20(8):2147–58. doi: 10.1158/1078-0432.CCR-13-2590
110. Coppola D, Nebozhyn M, Khalil F, Dai H, Yeatman T, Loboda A, et al. Unique ectopic lymph node-like structures present in human primary colorectal carcinoma are identified by immune gene array profiling. Am J Pathol (2011) 179(1):37–45. doi: 10.1016/j.ajpath.2011.03.007
111. Klapholz M, Drage MG, Srivastava A, Anderson AC. Presence of Tim3(+) and PD-1(+) CD8(+) T cells identifies microsatellite stable colorectal carcinomas with immune exhaustion and distinct clinicopathological features. J pathology. (2022) 257(2):186–97. doi: 10.1002/path.5877
112. Hamada T, Soong TR, Masugi Y, Kosumi K, Nowak JA, da Silva A, et al. TIME (Tumor immunity in the MicroEnvironment) classification based on tumor CD274 (PD-L1) expression status and tumor-infiltrating lymphocytes in colorectal carcinomas. Oncoimmunology. (2018) 7(7):e1442999. doi: 10.1080/2162402X.2018.1442999
113. Simoni Y, Becht E, Fehlings M, Loh CY, Koo SL, Teng KWW, et al. Bystander CD8(+) T cells are abundant and phenotypically distinct in human tumour infiltrates. Nature. (2018) 557(7706):575–9. doi: 10.1038/s41586-018-0130-2
114. Wennhold K, Thelen M, Lehmann J, Schran S, Preugszat E, Garcia-Marquez M, et al. CD86(+) antigen-presenting b cells are increased in cancer, localize in tertiary lymphoid structures, and induce specific T-cell responses. Cancer Immunol Res (2021) 9(9):1098–108. doi: 10.1158/2326-6066.CIR-20-0949
115. Hou W, Zhou X, Yi C, Zhu H. Immune check point inhibitors and immune-related adverse events in small cell lung cancer. Front Oncol (2021) 11:604227. doi: 10.3389/fonc.2021.604227
116. Aguilar EJ, Ricciuti B, Gainor JF, Kehl KL, Kravets S, Dahlberg S, et al. Outcomes to first-line pembrolizumab in patients with non-small-cell lung cancer and very high PD-L1 expression. Ann Oncol Off J Eur Soc Med Oncol 730 (2019) 30(10):1653–9. doi: 10.1093/annonc/mdz288
117. Chen G, Huang AC, Zhang W, Zhang G, Wu M, Xu W, et al. Exosomal PD-L1 contributes to immunosuppression and is associated with anti-PD-1 response. Nature. (2018) 560(7718):382–6. doi: 10.1038/s41586-018-0392-8
118. Wang X, Teng F, Kong L, Yu J. PD-L1 expression in human cancers and its association with clinical outcomes. OncoTargets Ther (2016) 9:5023–39. doi: 10.2147/OTT.S105862
119. Patel SP, Kurzrock R. PD-L1 expression as a predictive biomarker in cancer immunotherapy. Mol Cancer Ther (2015) 14(4):847–56. doi: 10.1158/1535-7163.MCT-14-0983
120. Ahn AR, Kim KM, Jang KY, Moon WS, Ha GW, Lee MR, et al. Correlation of PIK3CA mutation with programmed death ligand-1 (PD-L1) expression and their clinicopathological significance in colorectal cancer. Ann Trans Med (2021) 9(18):1406. doi: 10.21037/atm-21-2315
121. Yang L, Xue R, Pan C. Prognostic and clinicopathological value of PD-L1 in colorectal cancer: a systematic review and meta-analysis. OncoTargets Ther (2019) 12:3671–82. doi: 10.2147/OTT.S190168
122. Li Y, He M, Zhou Y, Yang C, Wei S, Bian X, et al. The prognostic and clinicopathological roles of PD-L1 expression in colorectal cancer: A systematic review and meta-analysis. Front Pharmacol (2019) 10:139. doi: 10.3389/fphar.2019.00139
123. Wang S, Yuan B, Wang Y, Li M, Liu X, Cao J, et al. Clinicopathological and prognostic significance of PD-L1 expression in colorectal cancer: a meta-analysis. Int J colorectal disease. (2021) 36(1):117–30. doi: 10.1007/s00384-020-03734-4
124. Llosa NJ, Luber B, Siegel N, Awan AH, Oke T, Zhu Q, et al. Immunopathologic stratification of colorectal cancer for checkpoint blockade immunotherapy. Cancer Immunol Res (2019) 7(10):1574–9. doi: 10.1158/2326-6066.CIR-18-0927
125. Peng Z, Cheng S, Kou Y, Wang Z, Jin R, Hu H, et al. The gut microbiome is associated with clinical response to anti-PD-1/PD-L1 immunotherapy in gastrointestinal cancer. Cancer Immunol Res (2020) 8(10):1251–61. doi: 10.1158/2326-6066.CIR-19-1014
126. Wang F, MM He, YC Y, Zhao X, ZQ W, Jin Y, et al. Regorafenib plus toripalimab in patients with metastatic colorectal cancer: a phase Ib/II clinical trial and gut microbiome analysis. Cell Rep Med (2021) 2(9):100383. doi: 10.1016/j.xcrm.2021.100383
127. Martini G, Ciardiello D, Dallio M, Famiglietti V, Esposito L, Corte CMD, et al. Gut microbiota correlates with antitumor activity in patients with mCRC and NSCLC treated with cetuximab plus avelumab. Int J cancer. (2022) 151(3):473–80. doi: 10.1002/ijc.34033
128. Wang C, Chevalier D, Saluja J, Sandhu J, Lau C, Fakih M. Regorafenib and nivolumab or pembrolizumab combination and circulating tumor DNA response assessment in refractory microsatellite stable colorectal cancer. oncologist. (2020) 25(8):e1188–e94. doi: 10.1634/theoncologist.2020-0161
129. Martinelli E, Martini G, Famiglietti V, Troiani T, Napolitano S, Pietrantonio F, et al. Cetuximab rechallenge plus avelumab in pretreated patients with RAS wild-type metastatic colorectal cancer: The phase 2 single-arm clinical CAVE trial. JAMA Oncol (2021) 7(10):1529–35. doi: 10.1001/jamaoncol.2021.2915
130. Segal NH, Cercek A, Ku G, Wu AJ, Rimner A, Khalil DN, et al. Phase II single-arm study of durvalumab and tremelimumab with concurrent radiotherapy in patients with mismatch repair-proficient metastatic colorectal cancer. Clin Cancer Res (2021) 27(8):2200–8. doi: 10.1158/1078-0432.CCR-20-2474
131. Redman JM, Tsai YT, Weinberg BA, Donahue RN, Gandhy S, Gatti-Mays ME, et al. A randomized phase II trial of mFOLFOX6 + bevacizumab alone or with AdCEA vaccine + avelumab immunotherapy for untreated metastatic colorectal cancer. Oncologist. (2022) 27(3):198–209. doi: 10.1093/oncolo/oyab046
132. Cheng YK, Chen DW, Chen P, He X, Li PS, Lin ZS, et al. Association of peripheral blood biomarkers with response to anti-PD-1 immunotherapy for patients with deficient mismatch repair metastatic colorectal cancer: A multicenter cohort study. Front Immunol (2022) 13:809971. doi: 10.3389/fimmu.2022.809971
133. Herting CJ, Farren MR, Tong Y, Liu Z, O'Neil B, Bekaii-Saab T, et al. A multi-center, single-arm, phase ib study of pembrolizumab (MK-3475) in combination with chemotherapy for patients with advanced colorectal cancer: HCRN GI14-186. Cancer Immunol Immunother. (2021) 70(11):3337–48. doi: 10.1007/s00262-021-02986-5
134. Saied A, Licata L, Burga RA, Thorn M, McCormack E, Stainken BF, et al. Neutrophil:lymphocyte ratios and serum cytokine changes after hepatic artery chimeric antigen receptor-modified T-cell infusions for liver metastases. Cancer Gene Ther (2014) 21(11):457–62. doi: 10.1038/cgt.2014.50
135. McLaughlin J, Han G, Schalper KA, Carvajal-Hausdorf D, Pelekanou V, Rehman J, et al. Quantitative assessment of the heterogeneity of PD-L1 expression in non-Small-Cell lung cancer. JAMA Oncol (2016) 2(1):46–54. doi: 10.1001/jamaoncol.2015.3638
136. Paver EC, Cooper WA, Colebatch AJ, Ferguson PM, Hill SK, Lum T, et al. Programmed death ligand-1 (PD-L1) as a predictive marker for immunotherapy in solid tumours: a guide to immunohistochemistry implementation and interpretation. Pathology. (2021) 53(2):141–56. doi: 10.1016/j.pathol.2020.10.007
137. Ho HL, Chou TY, Yang SH, Jiang JK, Chen WS, Chao Y, et al. PD-L1 is a double-edged sword in colorectal cancer: the prognostic value of PD-L1 depends on the cell type expressing PD-L1. J Cancer Res Clin Oncol (2019) 145(7):1785–94. doi: 10.1007/s00432-019-02942-y
138. Peng QH, Wang CH, Chen HM, Zhang RX, Pan ZZ, Lu ZH, et al. CMTM6 and PD-L1 coexpression is associated with an active immune microenvironment and a favorable prognosis in colorectal cancer. J immunotherapy Cancer (2021) 9(2):e001638. doi: 10.1136/jitc-2020-001638
139. Omura Y, Toiyama Y, Okugawa Y, Yin C, Shigemori T, Kusunoki K, et al. Prognostic impacts of tumoral expression and serum levels of PD-L1 and CTLA-4 in colorectal cancer patients. Cancer immunology immunotherapy CII. (2020) 69(12):2533–46. doi: 10.1007/s00262-020-02645-1
140. Masugi Y, Nishihara R, Yang J, Mima K, da Silva A, Shi Y, et al. Tumour CD274 (PD-L1) expression and T cells in colorectal cancer. Gut. (2017) 66(8):1463–73. doi: 10.1136/gutjnl-2016-311421
141. Ng HHM, Lee RY, Goh S, Tay ISY, Lim X, Lee B, et al. Immunohistochemical scoring of CD38 in the tumor microenvironment predicts responsiveness to anti-PD-1/PD-L1 immunotherapy in hepatocellular carcinoma. J immunotherapy Cancer (2020) 8(2):e000987. doi: 10.1136/jitc-2020-000987
142. Yeong J, Tan T, Chow ZL, Cheng Q, Lee B, Seet A, et al. Multiplex immunohistochemistry/immunofluorescence (mIHC/IF) for PD-L1 testing in triple-negative breast cancer: a translational assay compared with conventional IHC. J Clin pathology. (2020) 73(9):557–62. doi: 10.1136/jclinpath-2019-206252
143. Ravensbergen CJ, Polack M, Roelands J, Crobach S, Putter H, Gelderblom H, et al. Combined assessment of the tumor-stroma ratio and tumor immune cell infiltrate for immune checkpoint inhibitor therapy response prediction in colon cancer. Cells. (2021) 10(11):2935. doi: 10.3390/cells10112935
144. Vega PN, Nilsson A, Kumar MP, Niitsu H, Simmons AJ, Ro J, et al. Cancer-associated fibroblasts and squamous epithelial cells constitute a unique microenvironment in a mouse model of inflammation-induced colon cancer. Front Oncol (2022) 12:878920. doi: 10.3389/fonc.2022.878920
145. Herrera M, Berral-González A, López-Cade I, Galindo-Pumariño C, Bueno-Fortes S, Martín-Merino M, et al. Cancer-associated fibroblast-derived gene signatures determine prognosis in colon cancer patients. Mol cancer. (2021) 20(1):73. doi: 10.1186/s12943-021-01367-x
146. Pan H, Pan J, Wu J. Development and validation of a cancer-associated fibroblast-derived lncRNA signature for predicting clinical outcomes in colorectal cancer. Front Immunol (2022) 13:934221. doi: 10.3389/fimmu.2022.934221
147. Gao Y, Sun Z, Gu J, Li Z, Xu X, Xue C, et al. Cancer-associated fibroblasts promote the upregulation of PD-L1 expression through akt phosphorylation in colorectal cancer. Front Oncol (2021) 11:748465. doi: 10.3389/fonc.2021.748465
148. Chen YF, Yu ZL, Lv MY, Cai ZR, Zou YF, Lan P, et al. Cancer-associated fibroblasts impact the clinical outcome and treatment response in colorectal cancer via immune system modulation: a comprehensive genome-wide analysis. Mol Med (Cambridge Mass). (2021) 27(1):139. doi: 10.1186/s10020-021-00402-3
149. Jiang M, Qin B, Luo L, Li X, Shi Y, Zhang J, et al. A clinically acceptable strategy for sensitizing anti-PD-1 treatment by hypoxia relief. J Controlled release Off J Controlled Release Society. (2021) 335:408–19. doi: 10.1016/j.jconrel.2021.06.001
150. Xu Y, Hu J, Cao C, Zhang M, Liu Y, Chen H, et al. Distinct hypoxia-related gene profiling characterizes clinicopathological features and immune status of mismatch repair-deficient colon cancer. J Oncol (2021) 2021:2427427. doi: 10.1155/2021/2427427
151. Xu H, Ye D, Ren M, Zhang H, Bi F. Ferroptosis in the tumor microenvironment: perspectives for immunotherapy. Trends Mol Med (2021) 27(9):856–67. doi: 10.1016/j.molmed.2021.06.014
152. Wang W, Green M, Choi JE, Gijón M, Kennedy PD, Johnson JK, et al. CD8(+) T cells regulate tumour ferroptosis during cancer immunotherapy. Nature. (2019) 569(7755):270–4. doi: 10.1038/s41586-019-1170-y
153. Wan RJ, Peng W, Xia QX, Zhou HH, Mao XY. Ferroptosis-related gene signature predicts prognosis and immunotherapy in glioma. CNS Neurosci Ther (2021) 27(8):973–86. doi: 10.1111/cns.13654
154. Zhang HC, Deng SH, Pi YN, Guo JN, Xi H, Shi X, et al. Identification and validation in a novel quantification system of ferroptosis patterns for the prediction of prognosis and immunotherapy response in left- and right-sided colon cancer. Front Immunol (2022) 13:855849. doi: 10.3389/fimmu.2022.855849
155. Shao Y, Jia H, Huang L, Li S, Wang C, Aikemu B, et al. An original ferroptosis-related gene signature effectively predicts the prognosis and clinical status for colorectal cancer patients. Front Oncol (2021) 11:711776. doi: 10.3389/fonc.2021.711776
156. Yang YB, Zhou JX, Qiu SH, He JS, Pan JH, Pan YL. Identification of a novel ferroptosis-related gene prediction model for clinical prognosis and immunotherapy of colorectal cancer. Dis markers. (2021) 2021:4846683. doi: 10.1155/2021/4846683
157. Luo W, Dai W, Li Q, Mo S, Han L, Xiao X, et al. Ferroptosis-associated molecular classification characterized by distinct tumor microenvironment profiles in colorectal cancer. Int J Biol Sci (2022) 18(5):1773–94. doi: 10.7150/ijbs.69808
158. Wang Y, Zhang L, Shi G, Liu M, Zhao W, Zhang Y, et al. Effects of inflammatory response genes on the immune microenvironment in colorectal cancer. Front Genet (2022) 13:886949. doi: 10.3389/fgene.2022.886949
159. Wong SH, Zhao L, Zhang X, Nakatsu G, Han J, Xu W, et al. Gavage of fecal samples from patients with colorectal cancer promotes intestinal carcinogenesis in germ-free and conventional mice. Gastroenterology. (2017) 153(6):1621–33.e6. doi: 10.1053/j.gastro.2017.08.022
160. Kostic AD, Chun E, Robertson L, Glickman JN, Gallini CA, Michaud M, et al. Fusobacterium nucleatum potentiates intestinal tumorigenesis and modulates the tumor-immune microenvironment. Cell Host Microbe (2013) 14(2):207–15. doi: 10.1016/j.chom.2013.07.007
161. Helmink BA, Khan MAW, Hermann A, Gopalakrishnan V, Wargo JA. The microbiome, cancer, and cancer therapy. Nat Med (2019) 25(3):377–88. doi: 10.1038/s41591-019-0377-7
162. Iida N, Dzutsev A, Stewart CA, Smith L, Bouladoux N, Weingarten RA, et al. Commensal bacteria control cancer response to therapy by modulating the tumor microenvironment. Science. (2013) 342(6161):967–70. doi: 10.1126/science.1240527
163. Routy B, Le Chatelier E, Derosa L, Duong CPM, Alou MT, Daillère R, et al. Gut microbiome influences efficacy of PD-1-based immunotherapy against epithelial tumors. Science. (2018) 359(6371):91–7. doi: 10.1126/science.aan3706
164. Nejman D, Livyatan I, Fuks G, Gavert N, Zwang Y, Geller LT, et al. The human tumor microbiome is composed of tumor type-specific intracellular bacteria. Science. (2020) 368(6494):973–80. doi: 10.1126/science.aay9189
165. Allen J, Sears CL. Impact of the gut microbiome on the genome and epigenome of colon epithelial cells: contributions to colorectal cancer development. Genome Med (2019) 11(1):11. doi: 10.1186/s13073-019-0621-2
166. Flemer B, Lynch DB, Brown JM, Jeffery IB, Ryan FJ, Claesson MJ, et al. Tumour-associated and non-tumour-associated microbiota in colorectal cancer. Gut. (2017) 66(4):633–43. doi: 10.1136/gutjnl-2015-309595
167. Zong Y, Zhou Y, Liao B, Liao M, Shi Y, Wei Y, et al. The interaction between the microbiome and tumors. Front Cell infection Microbiol (2021) 11:673724. doi: 10.3389/fcimb.2021.673724
168. Vétizou M, Pitt JM, Daillère R, Lepage P, Waldschmitt N, Flament C, et al. Anticancer immunotherapy by CTLA-4 blockade relies on the gut microbiota. Science. (2015) 350(6264):1079–84. doi: 10.1126/science.aad1329
169. Sharma P, Allison JP. The future of immune checkpoint therapy. Science. (2015) 348(6230):56–61. doi: 10.1126/science.aaa8172
170. Sivan A, Corrales L, Hubert N, Williams JB, Aquino-Michaels K, Earley ZM, et al. Commensal bifidobacterium promotes antitumor immunity and facilitates anti-PD-L1 efficacy. Science. (2015) 350(6264):1084–9. doi: 10.1126/science.aac4255
171. Baruch EN, Youngster I, Ben-Betzalel G, Ortenberg R, Lahat A, Katz L, et al. Fecal microbiota transplant promotes response in immunotherapy-refractory melanoma patients. Science. (2021) 371(6529):602–9. doi: 10.1126/science.abb5920
172. Davar D, Dzutsev AK, McCulloch JA, Rodrigues RR, Chauvin JM, Morrison RM, et al. Fecal microbiota transplant overcomes resistance to anti-PD-1 therapy in melanoma patients. Science. (2021) 371(6529):595–602. doi: 10.1126/science.abf3363
173. Mola S, Pandolfo C, Sica A, Porta C. The macrophages-microbiota interplay in colorectal cancer (CRC)-related inflammation: Prognostic and therapeutic significance. Int J Mol Sci (2020) 21(18):6866. doi: 10.3390/ijms21186866
174. Borowsky J, Haruki K, Lau MC, Dias Costa A, Väyrynen JP, Ugai T, et al. Association of fusobacterium nucleatum with specific T-cell subsets in the colorectal carcinoma microenvironment. Clin Cancer Res an Off J Am Assoc Cancer Res (2021) 27(10):2816–26. doi: 10.1158/1078-0432.CCR-20-4009
175. Kikuchi T, Mimura K, Ashizawa M, Okayama H, Endo E, Saito K, et al. Characterization of tumor-infiltrating immune cells in relation to microbiota in colorectal cancers. Cancer immunology immunotherapy CII. (2020) 69(1):23–32. doi: 10.1007/s00262-019-02433-6
176. Frankel AE, Coughlin LA, Kim J, Froehlich TW, Xie Y, Frenkel EP, et al. Metagenomic shotgun sequencing and unbiased metabolomic profiling identify specific human gut microbiota and metabolites associated with immune checkpoint therapy efficacy in melanoma patients. Neoplasia (New York NY). (2017) 19(10):848–55. doi: 10.1016/j.neo.2017.08.004
177. Marcuello M, Vymetalkova V, Neves RPL, Duran-Sanchon S, Vedeld HM, Tham E, et al. Circulating biomarkers for early detection and clinical management of colorectal cancer. Mol aspects Med (2019) 69:107–22. doi: 10.1016/j.mam.2019.06.002
178. Tie J, Cohen JD, Wang Y, Christie M, Simons K, Lee M, et al. Circulating tumor DNA analyses as markers of recurrence risk and benefit of adjuvant therapy for stage III colon cancer. JAMA Oncol (2019) 5(12):1710–7. doi: 10.1001/jamaoncol.2019.3616
179. Pessoa LS, Heringer M, Ferrer VP. ctDNA as a cancer biomarker: A broad overview. Crit Rev oncology/hematology. (2020) 155:103109. doi: 10.1016/j.critrevonc.2020.103109
180. Cabel L, Riva F, Servois V, Livartowski A, Daniel C, Rampanou A, et al. Circulating tumor DNA changes for early monitoring of anti-PD1 immunotherapy: a proof-of-concept study. Ann Oncol Off J Eur Soc Med Oncol (2017) 28(8):1996–2001. doi: 10.1093/annonc/mdx212
181. Gong J, Aguirre F, Hazelett D, Alvarez R, Zhou L, Hendifar A, et al. Circulating tumor DNA dynamics and response to immunotherapy in colorectal cancer. Mol Clin Oncol (2022) 16(5):100. doi: 10.3892/mco.2022.2533
182. Liu Z, Han Y, Dang Q, Xu H, Zhang Y, Duo M, et al. Roles of circulating tumor DNA in PD-1/PD-L1 immune checkpoint inhibitors: Current evidence and future directions. Int Immunopharmacol. (2022) 111:109173. doi: 10.1016/j.intimp.2022.109173
183. Osumi H, Shinozaki E, Yamaguchi K, Zembutsu H. Clinical utility of circulating tumor DNA for colorectal cancer. Cancer Sci (2019) 110(4):1148–55. doi: 10.1111/cas.13972
184. Henriksen TV, Reinert T, Rasmussen MH, Demuth C, Løve US, Madsen AH, et al. Comparing single-target and multitarget approaches for postoperative circulating tumour DNA detection in stage II-III colorectal cancer patients. Mol Oncol (2022) 16(20):3654–65. doi: 10.1002/1878-0261.13294
185. Krijgsman D, de Vries NL, Skovbo A, Andersen MN, Swets M, Bastiaannet E, et al. Characterization of circulating T-, NK-, and NKT cell subsets in patients with colorectal cancer: the peripheral blood immune cell profile. Cancer immunology immunotherapy CII. (2019) 68(6):1011–24. doi: 10.1007/s00262-019-02343-7
186. Ferrucci PF, Gandini S, Battaglia A, Alfieri S, Di Giacomo AM, Giannarelli D, et al. Baseline neutrophil-to-lymphocyte ratio is associated with outcome of ipilimumab-treated metastatic melanoma patients. Br J cancer. (2015) 112(12):1904–10. doi: 10.1038/bjc.2015.180
187. Soyano AE, Dholaria B, Marin-Acevedo JA, Diehl N, Hodge D, Luo Y, et al. Peripheral blood biomarkers correlate with outcomes in advanced non-small cell lung cancer patients treated with anti-PD-1 antibodies. J immunotherapy cancer. (2018) 6(1):129. doi: 10.1186/s40425-018-0447-2
188. Clouthier DL, Lien SC, Yang SYC, Nguyen LT, Manem VSK, Gray D, et al. An interim report on the investigator-initiated phase 2 study of pembrolizumab immunological response evaluation (INSPIRE). J Immunother Cancer. (2019) 7(1):72. doi: 10.1186/s40425-019-0541-0
189. An HJ, Chon HJ, Kim C. Peripheral blood-based biomarkers for immune checkpoint inhibitors. Int J Mol Sci (2021) 22(17):9414. doi: 10.3390/ijms22179414
190. Lichtenstern CR, Ngu RK, Shalapour S, Karin M. Immunotherapy, inflammation and colorectal cancer. Cells. (2020) 9(3):618. doi: 10.3390/cells9030618
191. Mei Z, Shi L, Wang B, Yang J, Xiao Z, Du P, et al. Prognostic role of pretreatment blood neutrophil-to-lymphocyte ratio in advanced cancer survivors: A systematic review and meta-analysis of 66 cohort studies. Cancer Treat Rev (2017) 58:1–13. doi: 10.1016/j.ctrv.2017.05.005
192. Corti F, Lonardi S, Intini R, Salati M, Fenocchio E, Belli C, et al. The pan-Immune-Inflammation value in microsatellite instability-high metastatic colorectal cancer patients treated with immune checkpoint inhibitors. Eur J Cancer (Oxford Engl 1990). (2021) 150:155–67. doi: 10.1016/j.ejca.2021.03.043
Keywords: biomarker, immune checkpoint inhibitor (ICI), colon cancer, predictive, immunotherapy
Citation: Hou W, Yi C and Zhu H (2022) Predictive biomarkers of colon cancer immunotherapy: Present and future. Front. Immunol. 13:1032314. doi: 10.3389/fimmu.2022.1032314
Received: 30 August 2022; Accepted: 08 November 2022;
Published: 22 November 2022.
Edited by:
Udo S. Gaipl, University Hospital Erlangen, GermanyReviewed by:
Oscar J. Cordero, University of Santiago de Compostela, SpainSrijayaprakash Babu Uppada, University of Alabama, United States
Copyright © 2022 Hou, Yi and Zhu. This is an open-access article distributed under the terms of the Creative Commons Attribution License (CC BY). The use, distribution or reproduction in other forums is permitted, provided the original author(s) and the copyright owner(s) are credited and that the original publication in this journal is cited, in accordance with accepted academic practice. No use, distribution or reproduction is permitted which does not comply with these terms.
*Correspondence: Hong Zhu, NDQxNjk1MTMxQHFxLmNvbQ==