- 1Huaxi MR Research Center, Department of Radiology, Functional and Molecular Imaging Key Laboratory of Sichuan Province, West China Hospital, Sichuan University, Chengdu, China
- 2Department of Radiology, West China Hospital, Sichuan University, Chengdu, China
Objective: To explore multi-aspect radiologic assessment of immunotherapy response in intracranial malignancies based on a semi-automatic segmentation technique, and to explore volumetric thresholds with good performance according to RECIST 1.1 thresholds.
Methods: Patients diagnosed with intracranial malignancies and treated with immunotherapy were included retrospectively. In all MR images, target lesions were measured using a semi-automatic segmentation technique that could intelligently generate visual diagrams including RECIST 1.1, total volume, and max. 3D diameter. The changes in parameters were calculated for each patient after immunotherapy. The ROC curve was used to analyze the sensitivity and specificity of the size change of the legion. This was useful to find new volumetric thresholds with better efficiency in response assessment. The changes in total volume were assessed by conventional volumetric thresholds, while RECIST 1.1 thresholds were for the max. 3D diameter. A chi-square test was used to compare the concordance and diagnostic correlation between the response assessment results of the three criteria.
Results: A total of 20 cases (average age, 58 years; range, 23 to 84 years) and 58 follow-up MR examinations after immunotherapy were included in the analysis. The P-value of the chi-square test between RECIST 1.1 and total volume is 0 (P <0.05), same as that in RECIST 1.1 and max. 3D diameter. The kappa value of the former two was 0.775, and the kappa value for the latter two was 0.742. The above results indicate a significant correlation and good concordance for all three criteria. In addition, we also found that the volumetric assessment had the best sensitivity and specificity for the immunotherapy response in intracranial malignancies, with a PR threshold of −64.9% and a PD threshold of 21.4%.
Conclusions: Radiologic assessment of immunotherapy response in intracranial malignancy can be performed by multiple criteria based on semi-automatic segmentation technique on MR images, such as total volume, max. 3D diameter and RECIST 1.1. In addition, new volumetric thresholds with good sensitivity and specificity were found by volumetric assessment.
Introduction
Intracranial malignancies, including primary and metastatic tumors, seriously endanger human health. In recent years, in addition to surgery, radiotherapy, and chemotherapy, immunotherapy has achieved exciting progress in the treatment of intracranial malignancies (1). According to iRANO (immunotherapy Response Assessment for Neuro-Oncology) criteria, the assessment process of immunotherapy response in patients with neuro-oncological malignancies mainly involves two aspects: one is the assessment of radiological progression on follow-up oncologic imaging; the other is the assessment of new or substantially worsening neurological deficits indicating clinical decline unrelated to a comorbid event or concurrent medication. Both aspects together determine whether the neurosurgeon should change the clinical treatment decision for the patient (2, 3).
From the point of view of MR imaging, accurate radiological assessment of the size changes in intracranial malignancies after immunotherapy is still very important, which can help reflect the prognosis of patients more precisely and make better clinical treatment decisions. iRANO criteria guidelines stated that WHO (WHO = ∑ (long diameter ∗ short diameters) of target lesions) criteria were commonly used for radiological assessment of malignant gliomas, which had the same evaluation efficiency with RECIST 1.1 (4). For brain metastases, according to the RANO-BM (Response Assessment in Neuro-Oncology Brain Metastases) criteria, RECIST 1.1 (RECIST = ∑ (longest diameter of target tumors, shortest diameter of target lymph nodes)) criteria (5, 6) were used for radiological assessment. Although RECIST 1.1 is the most widely used criteria, it still has some shortcomings. Calculated values of RECIST 1.1 only represents the change in the longest axial diameter, but not the changes in all directions, so the authentic tumor size may be underestimated or overestimated, let alone the difficulty of accurate measurement of the tumor sizes with irregular shapes (7).
At present, a better way to solve the difficulties in RECIST 1.1 is volumetric assessment. Previous studies on size measurement of Vestibular Schwannomas (VS) found that linear measurements underestimated growth rate and were not as sensitive as volumetric measurements to tumor size changes (8). Meanwhile, an earlier study of brain metastases showed that the semi-automated segmentation technique based on CE-MRI (contrast-enhanced T1-weighted magnetic resonance imaging) showed lower intra-observer and inter-observer variability in volumetric measurement compared with unidimensional measurements, which could better reflect the real tumor size (7). Similarly, the potential applications of volumetric measurement have also been widely validated in other diseases, such as intrahepatic malignancies, lung metastases, rectal cancers, etc. (9–14).
Up until now, the main problem with volumetric assessment is the lack of a standardized threshold, which hinders the accurate assessment of remission and progress (10, 14). Because in some previous studies, the volumetric thresholds were derived from the RECIST 1.1 thresholds using a mathematically theoretical spherical formula (15). Therefore, in this study, we tried to find new volumetric thresholds for better diagnostic performance using the available data. Volumetric measurement was previously considered to be time-consuming and laborious. However, with the development of semi-automatic segmentation techniques, volumetric measurement may become more intelligent, efficient, and accurate.
Based on the existing semi-automatic segmentation technique, we attempt to find multiple radiologic criteria for immunotherapy response assessment in intracranial malignancies by analyzing CE-MRI images (16–18). Meanwhile, we will also seek new volumetric thresholds corresponding to the established RECIST 1.1 thresholds to assess the immunotherapy response of intracranial malignancies more sensitively and specifically.
Materials and methods
Screening of cases
We retrospectively scanned and analyzed the cases of patients diagnosed with intracranial malignancies and treated with immunotherapy from August 2018 to June 2022. The patients had primary gliomas or brain metastases from various malignancies, including lung adenocarcinoma, small cell lung cancer, and malignant melanoma, and follow-up MR (magnetic resonance) examinations were performed before and after immunotherapy. The inclusion criteria included the following:
1. At least one measurable lesion was present on the MR images (6).
2. The MR images had no artifacts that may affect the observation of target lesions, including motion artifacts, susceptibility artifacts, metal artifacts, etc.
3. The slice thickness of each follow-up CE-MRI images should be 1 mm.
4. Immunotherapy should be continued between the two follow-up MR examinations.
The flow chart for case screening is shown in Figure 1.
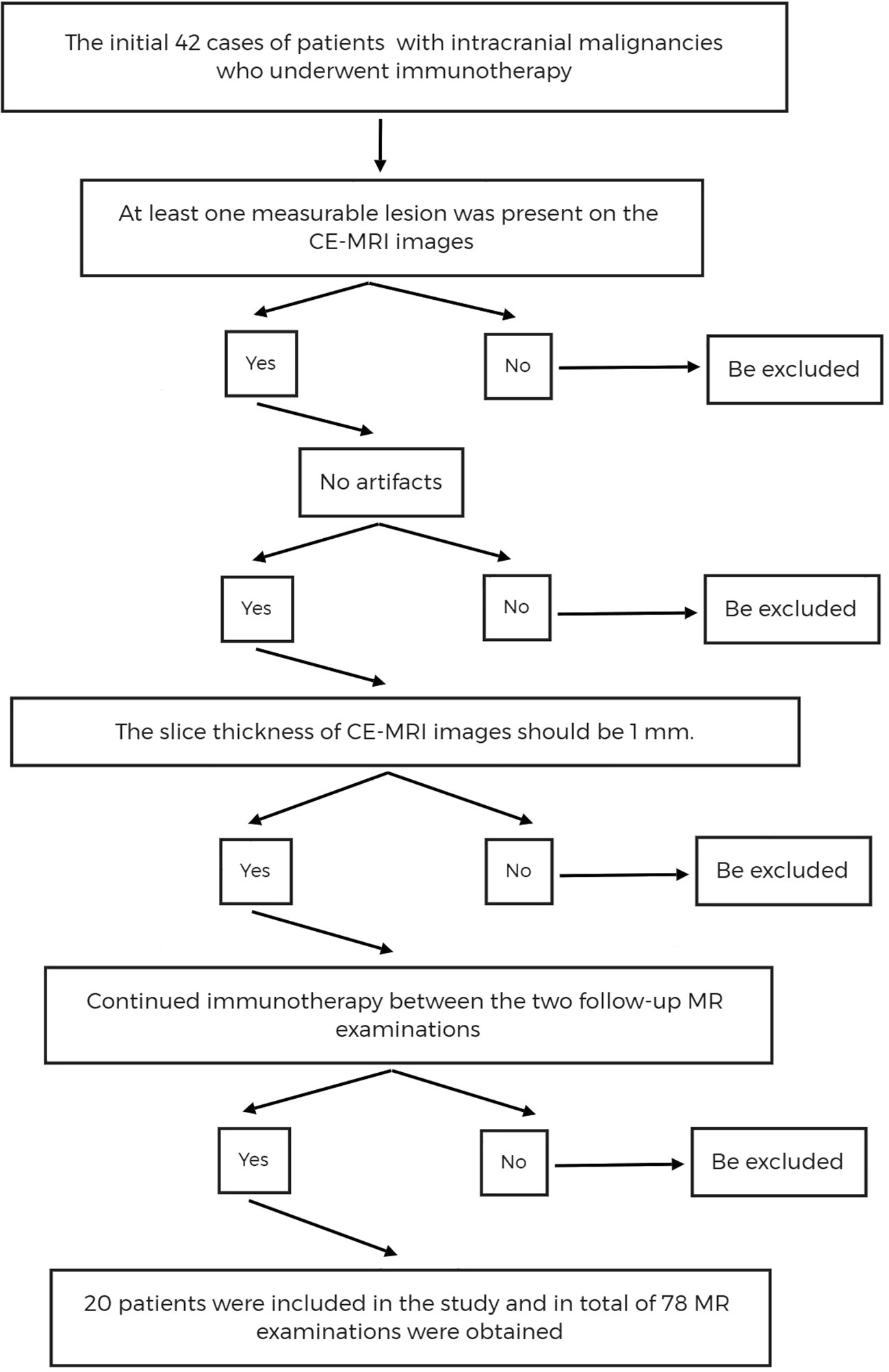
Figure 1 The flow chart of cases screening. CE-MRI, Contrast Enhanced T1-weighted Magnetic Resonance Imaging.
MRI acquisition
All the MR images were acquired randomly on 1.5 Tesla or 3.0 Tesla imaging systems from different scanner manufacturers, including Siemens, GE (General Electrical), Philips, and United Imaging. The critical parameters of CE-MRI protocols are shown in Table 1. The GBCAs (Gadolinium-based Contrast Agents) were used for CE-MRI, and the specific contrast agent information is as follows: gadoteridol injection (BIPSO GmbH), gadopentetate dimeglumine injection (Beijing Beilu Pharmaceutical Co., Ltd.), gadobutrol injection (Bayer Vital GmbH), and gadoteric acid meglumine salt injection (Jiangsu Hengrui Pharmaceutical Co., Ltd.). The dose of contrast agent was 0.1 mmol/kg.
Data analysis
All filtered MR data sets were loaded into the post-processing workstation, the ISP (Intellispace Philips Portal) and target lesions were semi-automatically delineated and analyzed using Multimodal Tumor Tracking (MMTT) software. MMTT software is a comprehensive technique based on regional growth and morphological image processing algorithms.
All routine follow-up MR images were reviewed by the senior radiologists (CL and YL) to qualitatively assess the presence of intracranial malignancy. Since previous studies had shown excellent intra-observer and inter-observer consistency with the semi-automated segmentation technique (7). Therefore, just an experienced radiologist independently measured, and all the target lesions were independently analyzed by the radiologist, but after discussing and reaching an agreement by two readers, some borderline cases were reviewed with two radiologists for consensus.
The number of target lesions was confirmed according to the RECIST 1.1 criteria, which required one lesion at least and five lesions at most. When the number of lesions is greater than five, only large and well-defined lesions should be selected. The target lesions included round-like lesions and heterotypic lesions. The delineation boundary was defined by the disruption area of the blood–brain barrier. Automatic segmentation could be started by clicking the mouse in the center of the enhanced area in axial view, and it could be adjusted manually if necessary. When delineating the target lesion, the boundary was identified in as many directions as possible (Figure 2). In the segmentation process, each time spent was measured in seconds. After that, multidimensional information could be obtained within a few seconds, including the detailed values of RECIST 1.1, total volume, max. 3D diameter (maximum three-dimensional diameter), etc.
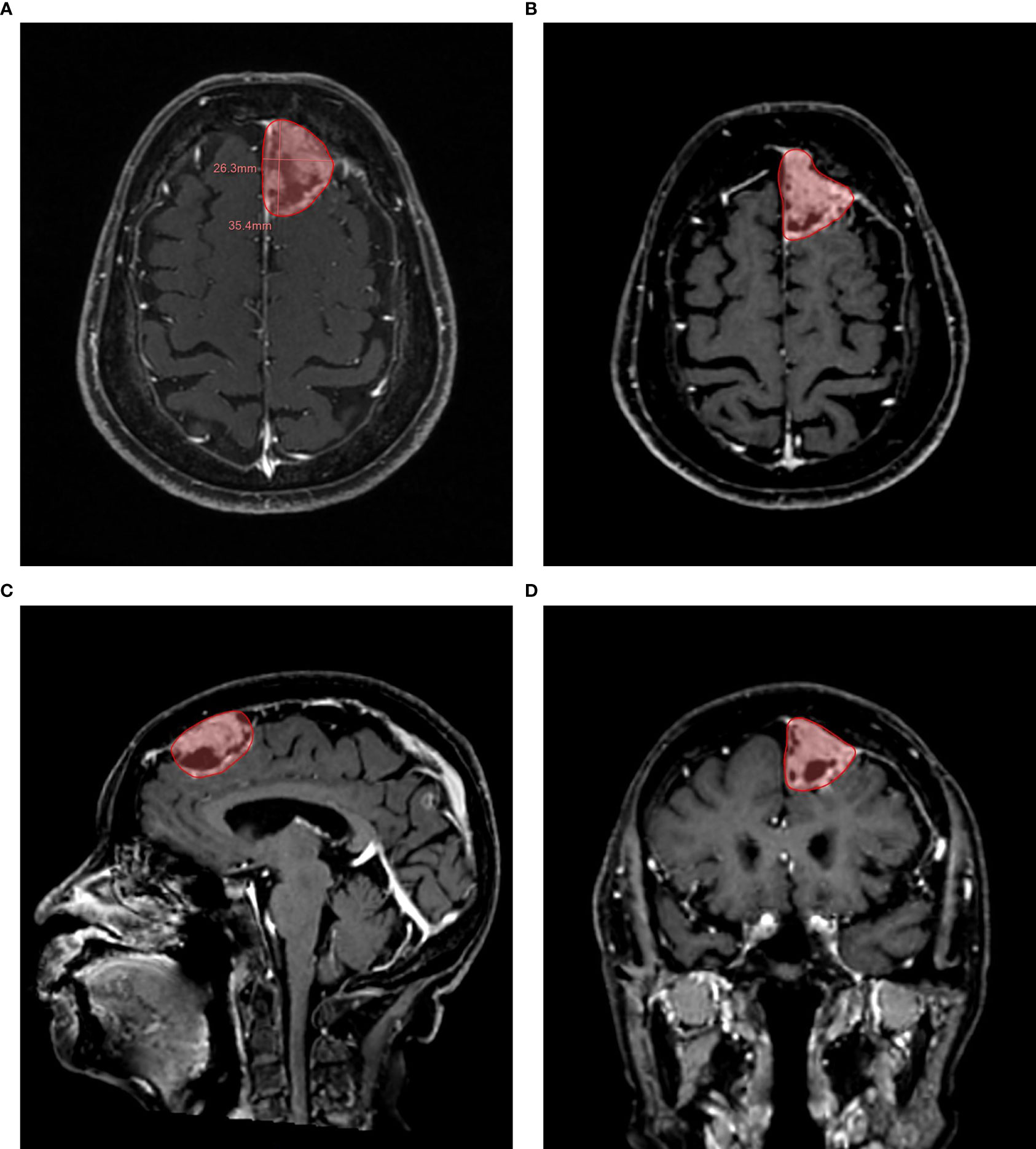
Figure 2 Example of a semi-automatic segmentation. The follow-up 3D CE-MRI of an 84-year-old female patient with brain metastases from lung adenocarcinoma. (A): Automatic measurement of maximum long diameter and maximum axial diameter. (B): Semi-automatic segmentation in axial plane. (C): Semi-automatic segmentation in sagittal plane. (D): Semi-automatic segmentation in coronal plane.
Taking the assessment results of target lesions before immunotherapy as a baseline, the changes in all results, including RECIST 1.1, total volume, and max. 3D diameter, were calculated for all patients at follow-up MR examinations after immunotherapy (7). Here, size changes of target lesions are calculated as the ratio of the difference between follow-up value and baseline value to the baseline value.
Radiographic response assessment for the change in max. 3D diameter was assessed based on RECIST 1.1 criteria, and radiographic response assessment for total volume was performed according to the theoretical volume thresholds. The detailed response thresholds are shown in Table 2.
Statistical analysis
A total of three groups of data were collected and analyzed, including changes in RECIST 1.1 data, total volume data, and max. 3D diameter data. After setting the PR (partial response) threshold to −30% and the PD (progressive disease) threshold to 20%, PR and PD were taken as the state variables. Differences in immunotherapy response between the three groups were compared pairwise using the chi-square test (21), and the null hypothesis called H0 was set to “the three groups of data were not correlated.” Both the Fisher exact text values and corresponding p-values were recorded. If P <0.05, it indicated that there was statistical significance among the three groups; if P >0.05, it indicated that there was no statistical significance among the three groups. Meanwhile, the Kappa value of each test was recorded to observe the concordance of the immunotherapy response among total volume, max. 3D diameter, and RECIST 1.1. Kappa value ≥0.75, the concordance was good; 0.75≥ Kappa value ≥0.4, the concordance was general; Kappa value <0.4 showed poor concordance.
Size changes of total volume and max. 3D diameter were taken as the test variables to draw the ROC curve (receiver operator characteristic curve) (22). Then, the size change corresponding to the maximum sum of sensitivity and specificity was selected as the optimal threshold. The AUC (area under the curve) value was also recorded at the same time. If AUC value = 1, the assessment effectiveness was perfect; if 0.85 <AUC value >0.95, the assessment effectiveness was very good; if 0.85 <AUC value >0.95, the assessment effectiveness was general; if AUC value <0.5, the assessment effectiveness was poor.
Finally, RECIST 1.1 was used as the “gold standard,” and cases were selected as examples to further analyze the response assessment between total volume, max. 3D diameter, and RECIST 1.1. All statistical analyses were performed using statistical software IBM SPSS Statistics 26.
Results
Patient data
After initial screening, there were a total of 42 cases of patients with intracranial malignancies who underwent immunotherapy. After further screening using inclusion criteria, 20 patients (males: 15; females: 5; average age: 58 years; range: 23–84 years) were included in this study. A total of 78 MRI scans obtained from these patients between September 2018 and June 2022 were used for analysis. Excluding the 20 MR scans performed before immunotherapy, the final number of MR scans used to calculate the size change rates was 58. There were three discrepant cases, all of which were derived from glioma patients, which may be due to the large boundary atypia of gliomas. Clinical information and follow-up MRI information for these cases are shown in Table 3.
Detailed information about the values and MR examinations are shown in Table 4.
Correlation and concordance
Detailed comparison results of immunotherapy response assessment between RECIST 1.1 and total volume by chi-square test are shown in Table 5. The Fisher exact test value was 59.70, and its corresponding P-value was zero, which was less than 0.05, indicating that there was statistically significance. The above results for total volume showed a strong correlation with RECIST 1.1. Similarly, Table 6 showed the assessment results between RECIST 1.1 and max. 3D diameter. While Fisher’s exact value was 57.382, its corresponding P-value was also zero. Besides, the Kappa value was higher than 0.75. There was high concordance between RECIST 1.1 and max. 3D diameter.
ROC curve and new volumetric thresholds
Furthermore, according to the PR threshold (−30%) of RECIST 1.1, we drew the ROC curve and obtained the AUC values for total volume and max. 3D diameter, which were 1 and 0.862 (Figure 3). We found that the maximum value of sensitivity plus specificity was 2. This corresponds to a volume size change of −64.9% and can be used as the new PR threshold for total volume. Similarly, according to the PD threshold of RECIST 1.1, the AUC values for total volume and max. 3D diameter were 0.945 and 0.968 (Figure 4) from the ROC curve. The maximum value of sensitivity plus specificity was 1.776. Its corresponding volumetric size change is 21.4%, which can be used as the new PD threshold for total volume.
Discussion
In this retrospective study of patients with intracranial malignancies after immunotherapy, using a semi-automated segmentation technique, we found that, compared with RECIST 1.1, volumetric assessment and max. 3D diameter assessment had consistent efficacy in assessing immunotherapy response (P <0.05; Kappa >0.75; AUC >085). Besides, our initial studies found that when the volumetric threshold of PR is −64.9% and the volumetric threshold of PD is 21.4%, the sensitivity and specificity are both the highest. At this point, the assessment efficacy of the immunotherapy response is equal to −30% and +20% of RECIST 1.1.
There were relatively few existing studies about the assessment of radiological response in the nervous system after immunotherapy recently (9, 23–25). Therefore, in this study, we investigated the radiologic response of intracranial malignancies after immunotherapy. According to the iRANO guidelines, the gold standard for determining recurrence or progression of intracranial malignancies is a biopsy or pathological section (2). However, currently, biopsies often obtain only very small tissue aliquots, which may lead to sampling artifacts. On the other hand, in clinical practice, many patients may undergo surgery before immunotherapy when the primary malignancy is found, such as high-grade glioma. As a result, the immunotherapy response of intracranial malignancies would be based on follow-up MR examinations rather than biopsies or pathological sections. Malignancies such as brain metastases, for which the patients did not easily receive surgery, were also assessed by follow-up MR examinations. Although initial studies had been conducted to monitor tumor response to radiotherapy or chemotherapy by assessing metabolic or functional parameters, such as using perfusion MRI or dynamic computed tomography (CT), size change of target lesions remains the most widely used parameter to monitor therapy response (2, 26). For size changes of target lesions, the iRANO working group stated that RECIST 1.1 criteria were the recommended radiological assessment criteria of immunotherapy response (2, 6). Therefore, based on all the above, RECIST 1.1 was used as the gold standard in this study.
Based on the optimized semi-automatic segmentation technology, one-stop intelligent identification and quantitative analysis of various tumors can be performed. It combines deep learning techniques to segment the boundaries of tumors accurately in a short time. The results support a variety of assessment criteria and can be output in a visual chart (Figure 5). Now, according to iRANO guidelines, one of the reasons why volumetric measurement remains controversial is that the technology increases costs and is not available in some centers. In this study, we performed a quantitative analysis of 78 MR examinations, including 20 MR examinations as a baseline and 58 longitudinal follow-up MR images as target assessments. The results showed that the time cost for quantitatively measuring the lesions on CE-MRI images is shorter (428.83 ± 88.47 s), and then we could obtain the results of RECIST 1.1, total volume, and max. 3D diameter simultaneously. According to our experiences, the semi-automatic segmentation time is equal to the time of post-processing in a brain CTA, so we consider that it can be practiced in clinical work.
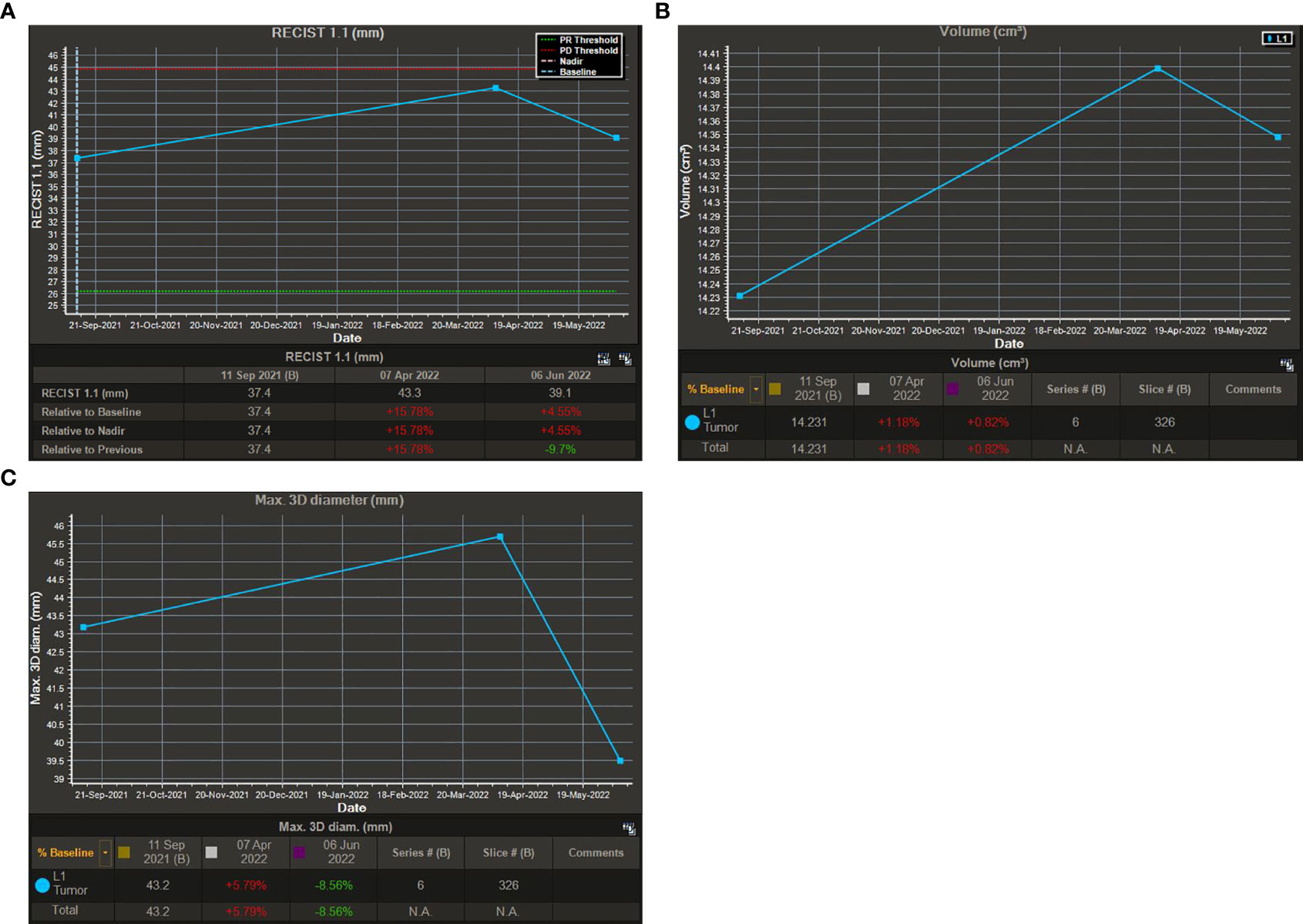
Figure 5 Visual charts of the semi-automatic segmentation technique. (A): Visual chart of RECIST 1.1. (B): Visual chart of total volume. (C): Visual chart of max. 3D diameter.
The RECIST 1.1 value is calculated as the sum of the longest axial diameter of the target lesions in intracranial malignancies. Because tumor growth is multidirectional and irregular, the axial size change does not represent all directional changes (15). This means that for irregular tumors, RECIST 1.1 may underestimate or overestimate the real tumor size. For assessing linear changes in maximum lesion diameter, the RECIST 1.0 criteria were originally established using a theoretical model of a solid tumor. RECIST 1.1 is an update of RECIST 1.0 for the entire population of patients with solid tumors. In actual clinical practice, accurate volumetric measurement shows certain advantages because of the varying tumor morphology. The RANO working group considered that the necessity for volumetric measurements would be confirmed with increasing clinical knowledge reserves and research on volumetric response assessment and reporting (2).
The chi-square test of the volumetric response assessment and the RECIST 1.1 response assessment showed that the volumetric assessment had a relatively high consistency efficiency in patients with intracranial malignancies undergoing immunotherapy (Table 5). Its corresponding P-value was zero, which was less than 0.05, indicating that there was a statistical significance. Besides, the Kappa value was 0.742. The above data showed concordance and a strong correlation between RECIST 1.1 and total volume. In addition, according to the ROC curve of PR threshold (−30%), the AUC value of total volume was 1 (Figure 4), and according to the ROC curve of the PD threshold (20%), the AUC value of total volume was 0.945, both of which reflect good diagnostic efficacy (Figure 5). Based on the above data, we consider that volume assessment using semi-automated segmentation is feasible for immunotherapy response assessment in intracranial malignancies and can be used as an updated method for RECIST 1.1. This is consistent with iRANO criteria, indicating that it would be encouraged as a secondary end point in the future when feasible.
Similarly, the sum of the longest axial diameters cannot represent the changes in all dimensions, while the max. 3D diameter based on semi-automatic segmentation techniques is intelligently identified in any dimensions. Using a unidimensional threshold (Table 2), we performed a chi-square test according to the response assessment results based on the sum of max. 3D diameter (Table 6, P <0; 0.05 Kappa >0.75). The above data showed concordance and a strong correlation between RECIST 1.1 and max. 3D diameter. According to the ROC curve (Figures 3, 4), the AUC of the size change in the max. 3D diameter was 0.862 for the PR threshold and 0.968 for the PD threshold, which indicated that the max. 3D diameter assessment had better diagnostic efficiency. Therefore, it is feasible to consider max. 3D diameter as another complementary parameter for immunotherapy response assessment.
If lesions grew proportionally and uniformly across all dimensions, according to the threshold for RECIST 1.1, a spherical volumetric threshold calculated by formula 4πr2/3 could be used as a reliable threshold for response assessment (Table 3). However, existing real-world data are insufficient to demonstrate the universality of volumetric response criteria in the population of patients with intracranial malignancies. At present, there are few studies focusing on volume response criteria, such as the optimization of volume threshold for liver metastases by Winter (10). While other studies on semi-automatic volumetric measurement mostly concern the comparison of repeatability and variability, there is no literature focusing on the optimization of volumetric threshold in intracranial malignancies (7).
Therefore, the volumetric threshold in different diseases needs more in-depth research. Our data showed that the PR threshold of volumetric change was −64.9%, which had a sensitivity of 100% and a specificity of 100% for the detection of target lesions with or without partial response. This was almost consistent with the conventional PR threshold of −65%. At the same time, our analysis showed that the volumetric threshold of PD was 21.4%, which was significantly different from the conventional threshold of 73%. The sensitivity and specificity of its detection of target lesions’ progression were 85.7% and 91.9%, respectively, which indicated that the accuracy of the current predicted PD threshold needs to be improved with more cases in the future.
Finally, due to the relatively small number of patients receiving immunotherapy for intracranial malignancies, it is better to enroll more patients in the future, as this is a meaningful and feasible study. Although MR images were obtained from different scanner manufacturers and there were inevitable differences between MR protocols, all MR scans met the minimum requirements for proper assessment according to RECIST 1.1; therefore, we think this did not significantly affect the results.
Conclusion
In conclusion, based on the semi-automatic segmentation technique, we found that volumetric assessment and max. 3D diameter assessment was reliable and consistent with RECIST 1.1 in response assessment of patients with intracranial malignancies undergoing immunotherapy. Total volume and max. 3D diameter can be used as complementary methods for RECIST 1.1 to assist neurosurgeons in the multi-aspect assessment of immunotherapy responses. Meanwhile, the initial analysis showed that when the volumetric threshold of PR was −64.9% and the volumetric threshold of PD was 21%, the sensitivity and specificity were the highest. The efficacy of volumetric thresholds for immunotherapy response assessment was equal to −30% and +20% of RECIST 1.1. This study will be useful for guiding further treatments for patients.
Data availability statement
The original contributions presented in the study are included in the article/supplementary material. Further inquiries can be directed to the corresponding author.
Ethics statement
Ethical review and approval were not required for the study on human participants in accordance with the local legislation and institutional requirements. Written informed consent for participation was not required for this study in accordance with the national legislation and the institutional requirements.
Author contributions
JT and CL provided the draft and performed the statistical analysis. JT, CL, ZL, HW, and YL collected the data. JT, MW, and CL evaluated the literature. JT wrote the first draft of the manuscript with support from other authors. RX, MW, SL, and CL revised the review. RX, YM, and MW contributed to the review. All authors contributed to the article and approved the submitted version.
Funding
This work was supported by the Sichuan Foundation for Distinguished Young Scholars (2021YFS0242); the Chengdu International Science and Technology Cooperation Funding (Grant 2019-GH02-00074-HZ); the Chengdu Science and Technology Bureau (Grant 2021-YF05-00698-SN); and the Scientific and technological Achievements Transformation Fund of West China Hospital, Sichuan University (Grant CGZH21002).
Conflict of interest
The authors declare that the research was conducted in the absence of any commercial or financial relationships that could be construed as a potential conflict of interest.
Publisher’s note
All claims expressed in this article are solely those of the authors and do not necessarily represent those of their affiliated organizations, or those of the publisher, the editors and the reviewers. Any product that may be evaluated in this article, or claim that may be made by its manufacturer, is not guaranteed or endorsed by the publisher.
Abbreviations
CE-MRI, Contrast-enhanced T1-weighted Magnetic Resonance Imaging; MRI, Magnetic Resonance Imaging; iRANO, immunotherapy Response Assessment for Neuro-Oncology; RECIST 1.1, Response Evaluation Criteria in Solid Tumors; Max. 3D diameter, Maximum three-dimensional diameter; GBCAs, Gadolinium-based Contrast Agents; ROC curve, Receiver Operator Characteristic curve; GE, General Electrical; AUC, Area Under the Curve.
References
1. Lim M, Xia Y, Bettegowda C, Weller M. Current state of immunotherapy for glioblastoma. Nat Rev Clin Oncol (2018) 15:422–42. doi: 10.1038/s41571-018-0003-5
2. Okada H, Weller M, Huang R, Finocchiaro G, Gilbert MR, Wick W, et al. Immunotherapy response assessment in neuro-oncology: A report of the rano working group. Lancet Oncol (2015) 16:e534–42. doi: 10.1016/s1470-2045(15)00088-1
3. Tan AC, Ashley DM, López GY, Malinzak M, Friedman HS, Khasraw M. Management of glioblastoma: State of the art and future directions. CA Cancer J Clin (2020) 70:299–312. doi: 10.3322/caac.21613
4. Miller AB, Hoogstraten B, Staquet M, Winkler A. Reporting results of cancer treatment. Cancer (1981) 47:207–14. doi: 10.1002/1097-0142(19810101)47:1<207::aid-cncr2820470134>3.0.co;2-6
5. Eisenhauer E, Therasse P, Bogaerts J, Schwartz L, Sargent D, Ford R, et al. New response evaluation criteria in solid tumours: Revised recist guideline (version 1.1). Eur J Cancer (2009) 45:228–47. doi: 10.1016/j.ejca.2008.10.026
6. Seymour L, Bogaerts J, Perrone A, Ford R, Schwartz LH, Mandrekar S, et al. Irecist: Guidelines for response criteria for use in trials testing immunotherapeutics. Lancet Oncol (2017) 18:e143–52. doi: 10.1016/s1470-2045(17)30074-8
7. Bauknecht HC, Romano VC, Rogalla P, Klingebiel R, Wolf C, Bornemann L, et al. Intra- and interobserver variability of linear and volumetric measurements of brain metastases using contrast-enhanced magnetic resonance imaging. Invest Radiol (2010) 45:49–56. doi: 10.1097/RLI.0b013e3181c02ed5
8. Harris GJ, Plotkin SR, Maccollin M, Bhat S, Urban T, Lev MH, et al. Three-dimensional volumetrics for tracking vestibular schwannoma growth in neurofibromatosis type ii. Neurosurgery (2008) 62:1314–9. doi: 10.1227/01.neu.0000333303.79931.83
9. Ertl-Wagner BB, Blume JD, Peck D, Udupa JK, Herman B, Levering A, et al. Reliability of tumor volume estimation from mr images in patients with malignant glioma. results from the american college of radiology imaging network (acrin) 6662 trial. Eur Radiol (2009) 19:599–609. doi: 10.1007/s00330-008-1191-7
10. Winter KS, Hofmann FO, Thierfelder KM, Holch JW, Hesse N, Baumann AB, et al. Towards volumetric thresholds in recist 1.1: Therapeutic response assessment in hepatic metastases. Eur Radiol (2018) 28:4839–48. doi: 10.1007/s00330-018-5424-0
11. Martens MH, van Heeswijk MM, van den Broek JJ, Rao SX, Vandecaveye V, Vliegen RA, et al. Prospective, multicenter validation study of magnetic resonance volumetry for response assessment after preoperative chemoradiation in rectal cancer: Can the results in the literature be reproduced? Int J Radiat Oncol Biol Phys (2015) 93:1005–14. doi: 10.1016/j.ijrobp.2015.09.008
12. Dicken V, Bornemann L, Moltz JH, Peitgen HO, Zaim S, Scheuring U. Comparison of volumetric and linear serial ct assessments of lung metastases in renal cell carcinoma patients in a clinical phase iib study. Acad Radiol (2015) 22:619–25. doi: 10.1016/j.acra.2014.12.018
13. Schramm N, Englhart E, Schlemmer M, Hittinger M, Übleis C, Becker CR, et al. Tumor response and clinical outcome in metastatic gastrointestinal stromal tumors under sunitinib therapy: Comparison of recist, choi and volumetric criteria. Eur J Radiol (2013) 82:951–8. doi: 10.1016/j.ejrad.2013.02.034
14. Pomerri F, Pucciarelli S, Gennaro G, Maretto I, Nitti D, Muzzio PC. Comparison between ct volume measurement and histopathological assessment of response to neoadjuvant therapy in rectal cancer. Eur J Radiol (2012) 81:3918–24. doi: 10.1016/j.ejrad.2012.04.038
15. Aykan NFÖzatlı T. Objective response rate assessment in oncology: Current situation and future expectations. World J Clin Oncol (2020) 11:53–73. doi: 10.5306/wjco.v11.i2.53
16. Amer AM, Zaid M, Chaudhury B, Elganainy D, Lee Y, Wilke CT, et al. Imaging-based biomarkers: Changes in the tumor interface of pancreatic ductal adenocarcinoma on computed tomography scans indicate response to cytotoxic therapy. Cancer (2018) 124:1701–9. doi: 10.1002/cncr.31251
17. Hoogeman M, Prévost JB, Nuyttens J, Pöll J, Levendag P, Heijmen B. Clinical accuracy of the respiratory tumor tracking system of the cyberknife: Assessment by analysis of log files. Int J Radiat Oncol Biol Phys (2009) 74:297–303. doi: 10.1016/j.ijrobp.2008.12.041
18. Yoon JH, Lee JM, Kang HJ, Ahn SJ, Yang H, Kim E, et al. Quantitative assessment of liver function by using gadoxetic acid-enhanced mri: Hepatocyte uptake ratio. Radiology (2019) 290:125–33. doi: 10.1148/radiol.2018180753
19. Sohaib SA, Turner B, Hanson JA, Farquharson M, Oliver RT, Reznek RH. Ct assessment of tumour response to treatment: Comparison of linear, cross-sectional and volumetric measures of tumour size. Br J Radiol (2000) 73:1178–84. doi: 10.1259/bjr.73.875.11144795
20. Singh AK, Hiroyuki Y, Sahani DV. Advanced postprocessing and the emerging role of computer-aided detection. Radiol Clin North Am (2009) 47:59–77. doi: 10.1016/j.rcl.2008.11.004
21. Sperry JL, Guyette FX, Brown JB, Yazer MH, Triulzi DJ, Early-Young BJ, et al. Prehospital plasma during air medical transport in trauma patients at risk for hemorrhagic shock. N Engl J Med (2018) 379:315–26. doi: 10.1056/NEJMoa1802345
22. Mandrekar JN. Receiver operating characteristic curve in diagnostic test assessment. J Thorac Oncol (2010) 5:1315–6. doi: 10.1097/JTO.0b013e3181ec173d
23. Qian JM, Mahajan A, Yu JB, Tsiouris AJ, Goldberg SB, Kluger HM, et al. Comparing available criteria for measuring brain metastasis response to immunotherapy. J Neurooncol (2017) 132:479–85. doi: 10.1007/s11060-017-2398-8
24. Lin NU, Lee EQ, Aoyama H, Barani IJ, Barboriak DP, Baumert BG, et al. Response assessment criteria for brain metastases: Proposal from the rano group. Lancet Oncol (2015) 16:e270–8. doi: 10.1016/s1470-2045(15)70057-4
25. Alexander BM, Brown PD, Ahluwalia MS, Aoyama H, Baumert BG, Chang SM, et al. Clinical trial design for local therapies for brain metastases: A guideline by the response assessment in neuro-oncology brain metastases working group. Lancet Oncol (2018) 19:e33–42. doi: 10.1016/s1470-2045(17)30692-7
Keywords: immunotherapy response, RECIST1.1, intracranial malignancy, magnetic resonance imaging, semi-automatic segmentation
Citation: Tan J, Liu C, Li Y, Ma Y, Xie R, Li Z, Wan H, Lui S and Wu M (2022) Assessment of immunotherapy response in intracranial malignancy using semi-automatic segmentation on magnetic resonance images. Front. Immunol. 13:1029656. doi: 10.3389/fimmu.2022.1029656
Received: 27 August 2022; Accepted: 25 November 2022;
Published: 14 December 2022.
Edited by:
Quan Cheng, Xiangya Hospital, Central South University, ChinaReviewed by:
Haiming Li, Fudan University, ChinaDejun Shr, First Affiliated Hospital of Fujian Medical University, China
Copyright © 2022 Tan, Liu, Li, Ma, Xie, Li, Wan, Lui and Wu. This is an open-access article distributed under the terms of the Creative Commons Attribution License (CC BY). The use, distribution or reproduction in other forums is permitted, provided the original author(s) and the copyright owner(s) are credited and that the original publication in this journal is cited, in accordance with accepted academic practice. No use, distribution or reproduction is permitted which does not comply with these terms.
*Correspondence: Min Wu, d3VtaW5zY3VAc2N1LmVkdS5jbg==
†These authors have contributed equally to this work