- 1Department of Thoracic Surgery, Beijing Chest Hospital/Beijing Tuberculosis and Thoracic Tumor Research Institute, Capital Medical University, Beijing, China
- 2Department of Medical Oncology, Beijing Tuberculosis and Thoracic Tumor Research Institute/Beijing Chest Hospital, Capital Medical University, Beijing, China
- 3Cancer Research Center, Beijing Tuberculosis and Thoracic Tumor Research Institute/Beijing Chest Hospital, Capital Medical University, Beijing, China
Background: At present, immunotherapy is a very promising treatment method for lung cancer patients, while the factors affecting response are still controversial. It is crucial to predict the efficacy of lung squamous carcinoma patients who received immunotherapy.
Methods: In our retrospective study, we enrolled lung squamous carcinoma patients who received immunotherapy at Beijing Chest Hospital from January 2017 to November 2021. All patients were grouped into two cohorts randomly, the training cohort (80% of the total) and the test cohort (20% of the total). The training cohort was used to build neural network models to assess the efficacy and outcome of immunotherapy in lung squamous carcinoma based on clinical information. The main outcome was the disease control rate (DCR), and then the secondary outcomes were objective response rate (ORR), progression-free survival (PFS), and overall survival (OS).
Results: A total of 289 patients were included in this study. The DCR model had area under the receiver operating characteristic curve (AUC) value of 0.9526 (95%CI, 0.9088–0.9879) in internal validation and 0.9491 (95%CI, 0.8704–1.0000) in external validation. The ORR model had AUC of 0.8030 (95%CI, 0.7437–0.8545) in internal validation and 0.7040 (95%CI, 0.5457–0.8379) in external validation. The PFS model had AUC of 0.8531 (95%CI, 0.8024–0.8975) in internal validation and 0.7602 (95%CI, 0.6236–0.8733) in external validation. The OS model had AUC of 0.8006 (95%CI, 0.7995–0.8017) in internal validation and 0.7382 (95%CI, 0.7366–0.7398) in external validation.
Conclusions: The neural network models show benefits in the efficacy evaluation of immunotherapy to lung squamous carcinoma patients, especially the DCR and ORR models. In our retrospective study, we found that neoadjuvant and adjuvant immunotherapy may bring greater efficacy benefits to patients.
Highlights
- The expression level of PD-L1 is not an ideal tool for evaluating efficacy.
- The neural network models show benefits in the efficacy evaluation of immunotherapy to lung squamous carcinoma patients, especially the DCR and ORR models.
- Neoadjuvant and adjuvant immunotherapy may bring greater efficacy benefits to lung squamous carcinoma patients.
Background
Lung cancer occupies first place in mortality and second place in morbidity globally (1, 2). Non-small cell lung cancer (NSCLC) accounts for about 85% of lung cancer cases, and the 5-year survival rate is only 18% (3, 4). Recently, the advent of targeted drugs and the emergence of immunotherapy have significantly prolonged the survival of lung cancer patients and improved their quality of life (3). Lung squamous carcinoma accounts for 25%–30% of lung cancer cases, while the occurrence rate of common driver gene mutation is less than 7% (5–9). Patients with lung squamous carcinoma have few chances to receive targeted therapy. Fortunately, immunotherapy brings a new light to patients with lung squamous carcinoma, which can significantly improve objective response rate (ORR) and prolong progression-free survival (PFS) and overall survival (OS) (10–12). However, it also brings challenges to the selection of biomarkers for predicting efficacy, the treatment plan for lung cancer, and treatment-related adverse events.
At present, the expression level of programmed cell death ligand 1 (PD-L1) is an indicator that may predict the effectiveness of anti-programmed cell death 1 (anti-PD-1)/PD-L1 immunotherapy and screen the population sensitive to them (13). However, studies also found that some patients with high PD-L1 expression have a poor immune response. On the contrary, up to 10% of patients with negative PD-L1 expression have a good immune response (14). It suggested that the expression level of PD-L1 is not an ideal tool for evaluating efficacy. Several studies showed that traditional clinical or pathological features, including smoking status, age, pathological type, and tumor grade, are associated with immunotherapy for NSCLC (15–17). Therefore, it is necessary to use a new method to evaluate the efficacy of immunotherapy and identify the dominant population sensitive to immunotherapy.
Deep learning neural network, as a subdiscipline of artificial intelligence (AI), has shown good performance in predicting and monitoring treatment response, which is also gradually gaining the attention of clinicians (18, 19). Convolutional neural network is currently used to diagnose solid tumors (lung cancer, melanoma, gastrointestinal tumors, etc.) through automatic quantification of radiological images, digital histopathological image interpretation, or biomarker analysis (18, 20–22). However, little research focused on the evaluation of immunotherapy efficacy in NSCLC based on AI.
To better predict the efficacy of the immunotherapy of lung squamous carcinoma patients and thus further provide more optimal treatment strategies, we introduced the neural network algorithm to build a fully connected neural network (also known as a dense neural network (DNN)) based on clinical information of the above patients. The original codes and data have been uploaded for use by clinicians and future visualization platforms.
Method
Study design and clinical information
This study was designed as a retrospective cohort study. Eligible patients aged ≥18 years were diagnosed with lung squamous carcinoma pathologically and received immunotherapy in Beijing Chest Hospital affiliated with Capital Medical University between 16 January 2017 and 10 December 2021. Patients with active autoimmune disease, symptomatic interstitial lung disease, multiple primary pulmonary carcinomas, or missing any of the included clinical characteristics, like status or follow-up records, were excluded. Tumor PD-L1 expression was assessed using the PD-L1 immunohistochemistry 22C3 pharmDx kit (Agilent Technologies, Carpinteria, CA, USA) at the Pathology Department of Beijing Chest Hospital. The expression level of PD-L1 protein of archival tumor tissue or tissue obtained through biopsy was determined by the tumor proportion score (TPS). Then, all patients were grouped into two cohorts randomly, the training cohort (80% of the total) and the test cohort (20% of the total). The training cohort was used to build neural network models to assess the efficacy and outcome of immunotherapy in lung squamous carcinoma based on clinical information, with evaluated by internal validation (using training cohort data) and external validation (using test cohort data) (Figure 1). The last follow-up date was 30 April 2022.
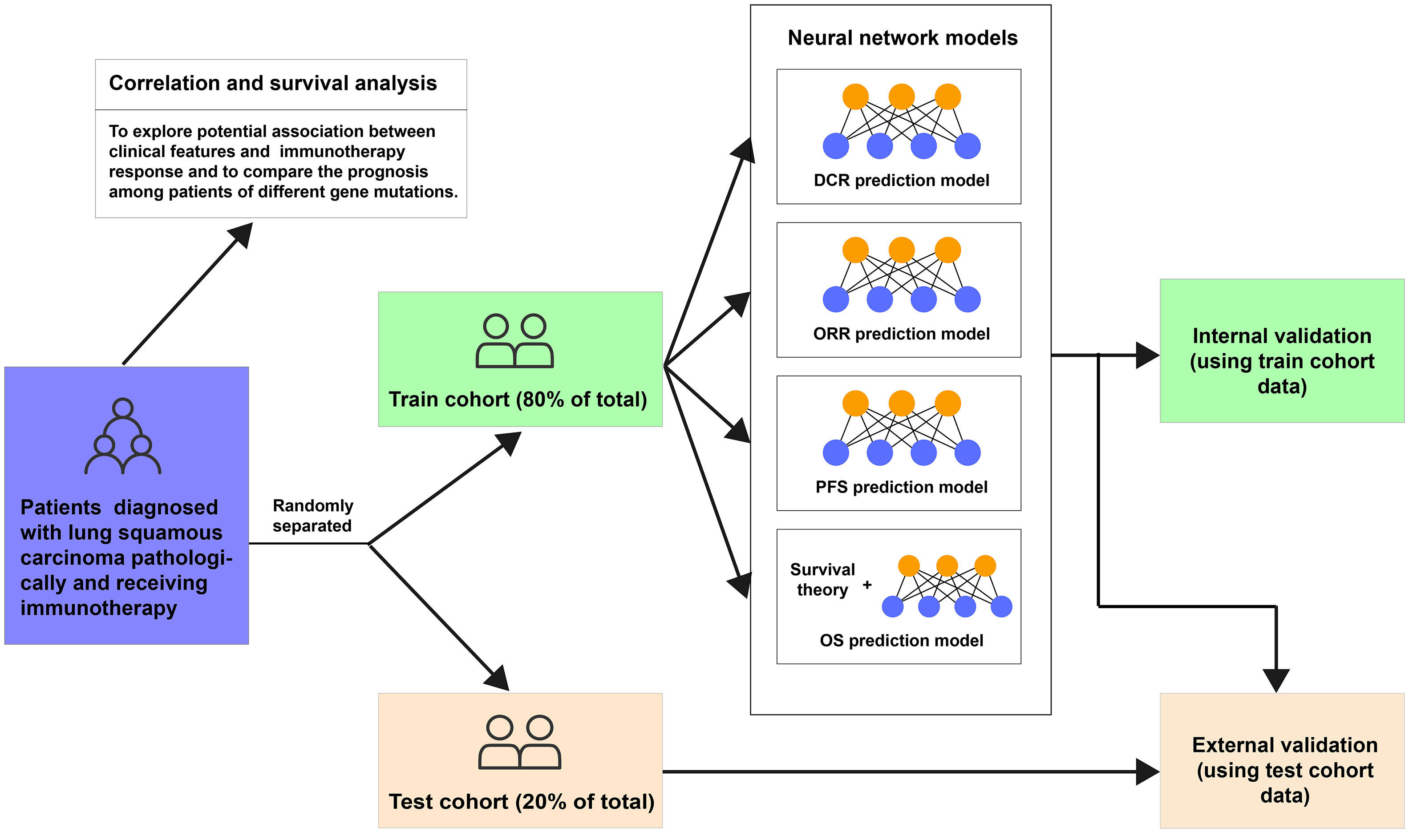
Figure 1 Flowchart of this study. DCR, disease control rate; ORR, objective response rate; PFS, progression-free survival; OS, overall survival.
This research has been approved by the Ethics Committee of Beijing Chest Hospital affiliated with Capital Medical University. Given that this was a retrospective analysis, individual consent was waived.
Potential predictive variables
The potential clinical predictive variables were as follows: age, sex, smoking status, performance status (PS) score before receiving immunotherapy, PD-L1 expression, TNM and clinical stage, vascular invasion, pleural metastasis, extra-thoracic metastasis, brain metastasis, liver metastasis, bone metastasis, adrenal metastasis, received chemoimmunotherapy or not, received immunotherapy plus antiangiogenic therapy or not, neoadjuvant immunotherapy, immunotherapy lines, and gene mutations. Considering that gene mutations contained much missing value, we did not include them in the final models. Supplementary Figure 1 shows the distribution of missing values. TNM and clinical stage were evaluated by at least two senior clinicians, referring to the 8th edition of the American Joint Committee on Cancer (AJCC) stage. All patients have received immunotherapy, of whom some accepted another therapy such as chemotherapy or antiangiogenic medicine. Partial patients in this study were treated with neoadjuvant immunotherapy, which meant they would undergo operations later.
Multiple imputations of missing values have been performed before the above predictive variables were included in models, with the help of the R package (23).
Outcome
The main outcome was the disease control rate (DCR), and secondary outcome indicators were ORR, PFS, and OS. The imaging manifestations have been interpreted by local researchers according to the Response Evaluation Criteria in Solid Tumors (RECIST version 1.1). The best overall response (BOR) was assessed including complete response (CR), partial response (PR), or stable disease (SD) after immunotherapy, regarded as DCR. ORR included CR or PR patients. PFS was defined as the time from the day of receiving immunotherapy to objective tumor progression, surgery, or death. We processed PFS as a binary variable, PFS ≤6 months or >6 months.
Data pre-processing
Standardization of data is a common process in many machine learning situations, which implies numerical variables subtracting their means and dividing by their standard deviations. Categorical variables have been converted into dummy variables, such as replacing the sex variable with two dummy variables (female = 0 or male = 1). Age and PS score before receiving immunotherapy were standardized, while sex, smoking status, PD-L1 expression, TNM and clinical stage, vascular invasion, pleural metastasis, extra-thoracic metastasis, brain metastasis, liver metastasis, bone metastasis, adrenal metastasis, received chemoimmunotherapy or not, received immunotherapy plus antiangiogenic therapy or not, neoadjuvant immunotherapy, and immunotherapy lines were transformed to dummy variables. Data from the test cohort were standardized according to the training cohort, and Supplementary Table 1 exhibits the mean and standard deviations of numerical variables.
Python package pandas and scikit-learn helped us to achieve the above processing (24, 25).
Model training and validation
To assess the DCR, ORR, and PFS, three dense neural networks have been built. To obtain accurate predictions, batch training and normalization were used. Dropout layers were used to avoid overfitting (which means performing well in the training cohort but badly in the test cohort), and early stopping functions were used to end training epochs if necessary.
To predict the OS possibility of lung squamous carcinoma patients after immunotherapy, we conducted a neural network survival model based on Katzman’s DeepSurv (26). It has to be mentioned that the neural network was designed to tackle traditional classification issues instead of time-dependent tasks, so the performance of OS prediction might be moderate.
As mentioned above, since our models were applied to solve classification problems, we used the area under the receiver operating characteristic curve (AUC) to evaluate their performance. The closer the AUC is to 1, the better the performance of the model. In order to obtain AUC with detailed 95% confidence interval (CI), we run bootstrap 1,000 times.
Python and its packages PyTorch, torchtuples, NumPy, pycox, and matplotlib and the R package pROC helped us in these analyses (27–32).
Correlation and survival analysis
We used correlation analysis to explore the potential association between clinical features and patients’ immunotherapy response with Spearman’s rank correlation, visualized by heatmap and chord diagram. Finally, survival analysis was performed to compare the prognoses of patients with different gene mutations. R and packages corrplot, circlize, and survminer were used during these procedures (33–35).
Statistical analysis
The numerical data of skewed distribution were analyzed by the Wilcoxon test, while categorical data were compared by chi-square or Fisher’s exact test. A two-sided p-value of less than 0.05 was considered statistically significant. These analyses and relevant plotting were completed using R software and packages epiDisplay, ggplot2, and ggridges (36, 37).
Result
Characteristics of patients
A total of 289 patients were included; the PFS of 138 patients was shorter or equal to 6 months, and the PFS of 151 patients was longer than 6 months. In the group with PFS ≤ 6 months, there were 11 women and 127 men. As for the expression of PD-L1, 30 patients had <1% expression, 37 patients had 1%–49% expression, and 42 patients had ≥50% expression. Among them, four patients had CR, 44 patients had PR, 65 patients had SD, and 24 patients were diagnosed with PD. The group with PFS > 6 months included 12 women and 139 men. The PD-L1 expression of 29 patients was <1%; 36 patients, between 1% and 49%; and 55 patients, ≥50%. Among them, five had CR, 108 had PR, and 38 had PD. The median follow-up time was 7.75 months in the group with PFS ≤ 6 months and 12.8 months in the group with PFS > 6 months. The detailed information of patients is illustrated in Table 1 and Figure 2.
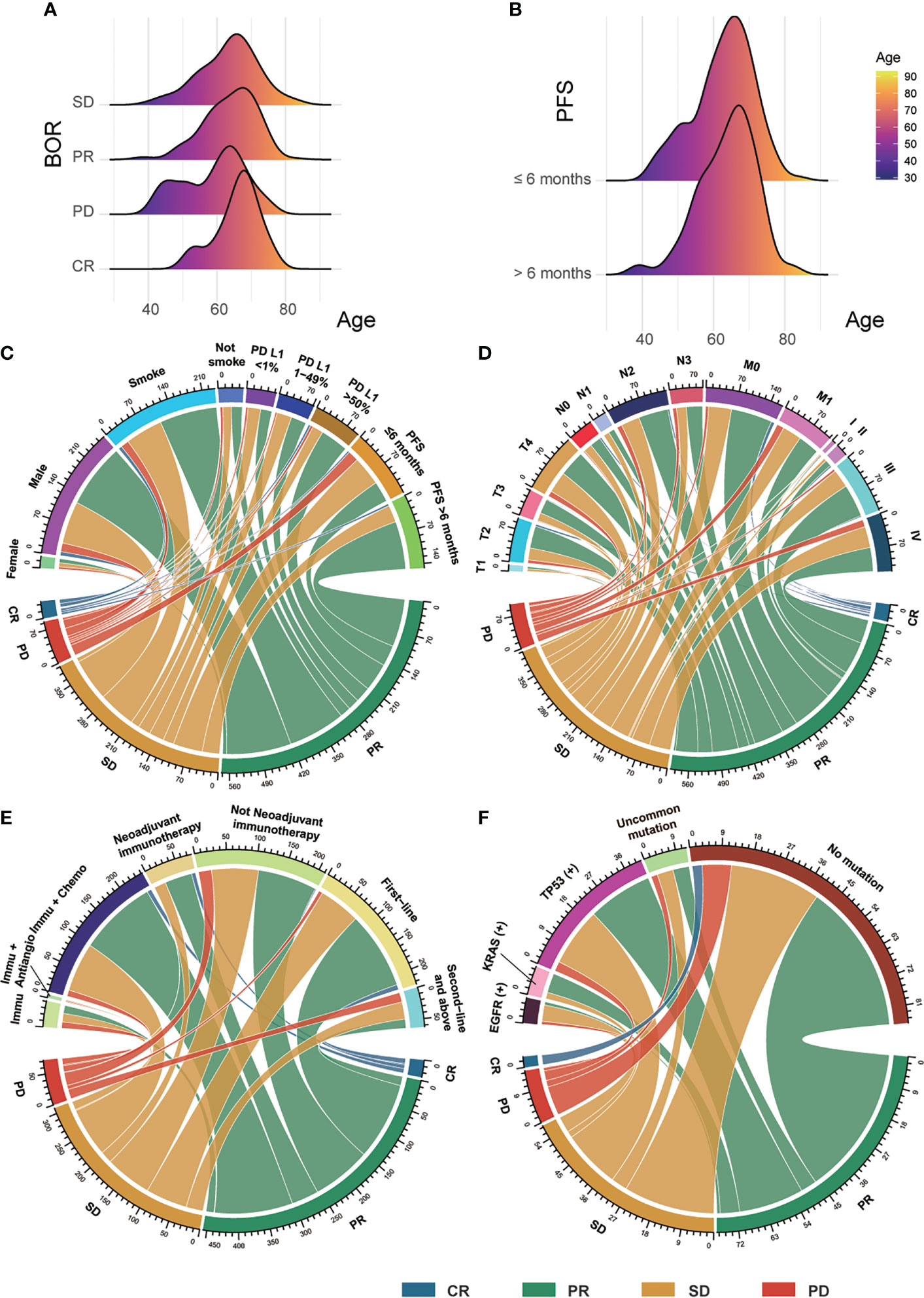
Figure 2 The clinical features of patients. (A) The distribution between BOR and patients’ age. (B) The distribution between PFS and patients’ age. (C) The connections among patients’ sex, smoking status, PD-L1 expression, and PFS with their BOR. (D) The connections among patients’ TNM stage with their BOR. (E) The correlations between patients’ therapy and their BOR. (F) The correlations between patients’ gene mutation and their BOR. BOR, best overall response; CR, complete response; PR, partial response; SD, stable disease; PD, progressive disease; PFS, progression-free survival; PD-L1, programmed cell death ligand 1; Immu, immunotherapy; Antiangio, antiangiogenic therapy; Chemo, chemotherapy; uncommon gene mutation.
Model training
We conducted four neural networks to assess the efficacy and outcome of immunotherapy in lung squamous carcinoma (Figure 1). The DCR model was designed to return the probabilities of patients showing DCR after immunotherapy, with the ORR model predicting the ORR possibilities and the PFS model judging their PFS longer or shorter than 6 months. The OS model was used to predict patients’ OS possibility based on a neural network survival algorithm. The training curves are shown in Supplementary Figure 2.
Model performance and inference
The DCR model had AUC of 0.9526 (95%CI, 0.9088–0.9879) in internal validation and 0.9491 (95%CI, 0.8704–1.0000) in external validation. The ORR model had AUC of 0.8030 (95%CI, 0.7437–0.8545) in internal validation and 0.7040 (95%CI, 0.5457–0.8379) in external validation. The PFS model had AUC of 0.8531 (95%CI, 0.8024–0.8975) in internal validation and 0.7602 (95%CI, 0.6236–0.8733) in external validation. The receiver operating characteristic curve valuess of the DCR model, ORR model, and PFS model are shown in Figure 3, and their original codes are shown in Supplementary Material File 1. The OS model had AUC of 0.8006 (95%CI, 0.7995–0.8017) in internal validation and 0.7382 (95%CI, 0.7366–0.7398) in external validation (Table 2). The codes of the OS model are shown in Supplementary Material File 2. The weights and hyper-parameters of the above four models are shown in Supplementary Material File 3.
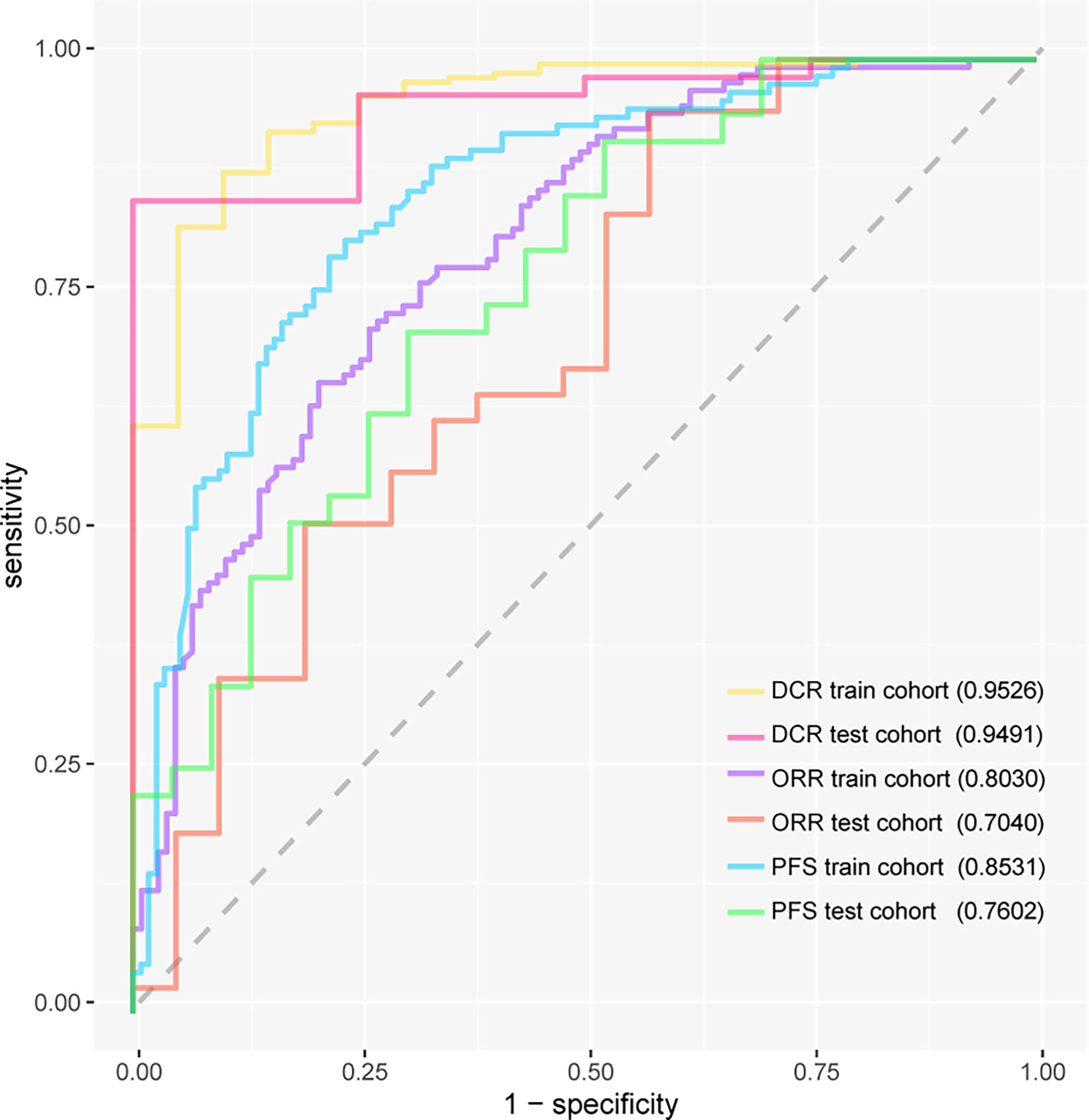
Figure 3 The receiver operating characteristic curves of three models. DCR, disease control rate; ORR, objective response rate; PFS, progression-free survival.
Age, sex, smoking status, PS score, PD-L1 expression, TNM and clinical stage, vascular invasion, pleural metastasis, extra-thoracic metastasis, brain metastasis, liver metastasis, bone metastasis, adrenal metastasis, received chemoimmunotherapy or not, received immunotherapy plus antiangiogenic therapy or not, neoadjuvant immunotherapy, and immunotherapy lines were the predictive clinical features. When these codes are open in a python environment (https://www.python.org/) and jupyter notebook software (https://www.jupyter.org/), with predictive clinical features inputted, models will return the predictive possibility of DCR, ORR, PFS, or OS.
Correlation analysis
General overview, PS score, PD-L1 expression, TNM stage, distant metastasis, and invasion (vascular, pleural, brain, liver, and bone) had statistical relation with immunotherapy (BOR, DCR, ORR, PFS, or OS) (Figure 4). We also compared the OS of patients with different gene mutations but did not find some statistical discrepancies (Supplementary Figure 3).
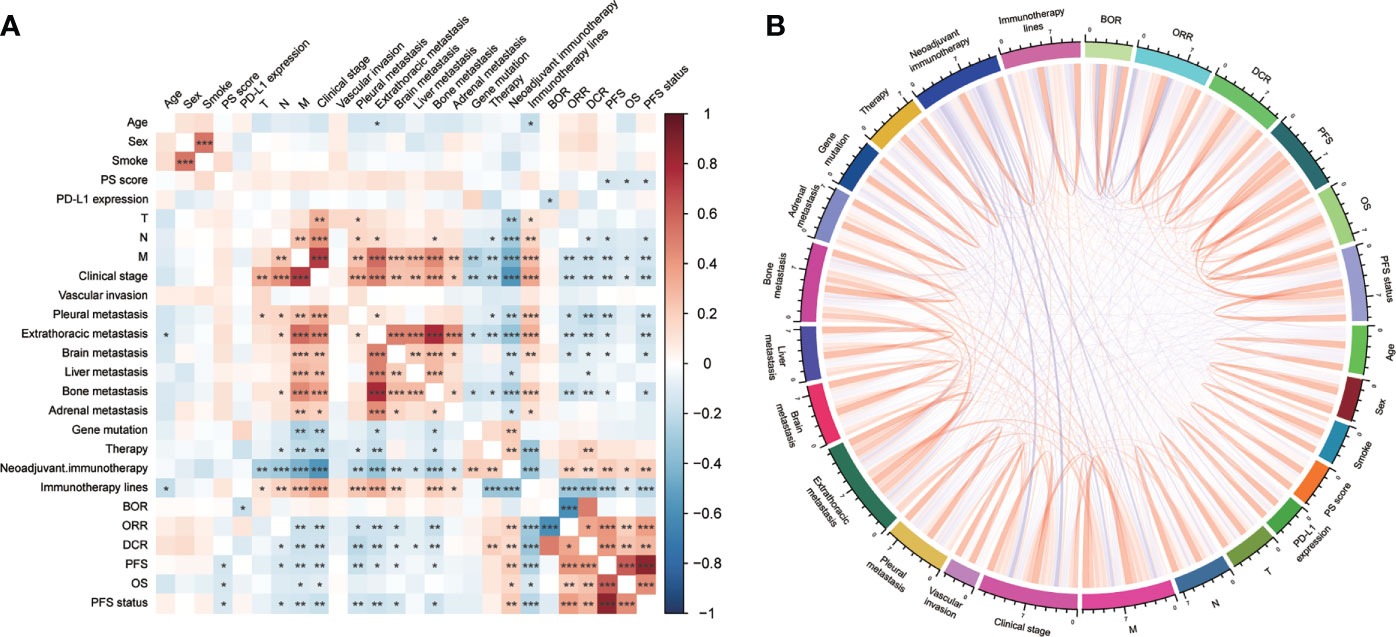
Figure 4 The correlation among clinical features with immunotherapy was visualized by (A) heatmap and (B) chord diagram. PD-L1, programmed cell death ligand 1; BOR, best overall response; ORR, objective response rate; DCR, disease control rate; PFS, progression-free survival; OS, overall survival. *P<0.05, **P<0.01, ***P<0.001.
Discussion
Immunotherapy significantly improves the prognosis of lung cancer patients, but not everyone who receives immunotherapy can benefit from it (38). Hence, it is essential to choose a better method to predict immunotherapy efficacy and screen the population sensitive to immunotherapy. We developed and validated deep learning neural network models based on clinical data for immune efficacy prediction for lung squamous carcinoma. The results of our study verified that the deep learning model showed good predictive performance in these patients.
Currently, multiple clinical trials have shown that the expression level of PD-L1 not only could decide who to treat but also could hint at whom to benefit (16, 39–42). Interestingly, several trials have examined PD-L1 as a viable biomarker to predict response to immune checkpoint inhibitors (ICIs) (43–45). Moreover, due to the heterogeneity of PD-L1 expression in tumors, there is a certain difference between puncture biopsy specimens and surgical resection specimens (46, 47). Some studies have shown that the positive rate of PD-L1 was related to clonal selection, biopsy sites, and detection time, and researchers should further coordinate the harmonization of utilized clones, scores, and interobserver variability (48). It is suggested that the independent predictive effect of tumor PD-L1 expression is still imperfect.
We developed and validated the immunotherapy predictive deep learning models using clinical information in lung squamous carcinoma. Raw data were divided into two independent groups, the training cohort (80% of the total) and the test cohort (20% of the total). Age, sex, smoking status, PS score before receiving immunotherapy, PD-L1 expression, TNM and clinical stage, vascular invasion, pleural metastasis, extra-thoracic metastasis, brain metastasis, liver metastasis, bone metastasis, adrenal metastasis, received chemoimmunotherapy or not, received immunotherapy plus antiangiogenic therapy or not, neoadjuvant immunotherapy, and immunotherapy lines were chosen as the predictive variables. The train cohort was used to conduct the ORR model, DCR model, PFS model, and OS model, which were validated using both train cohort and test cohort. Teo avoid overfitting, early stopping function and dropout layers were adopted after numerical variables were standardized and categorical variables were converted into dummy variables. Finally, the above models showed satisfactory performances. The DCR model had AUC of 0.9526 (95%CI, 0.9088–0.9879) in internal validation and 0.9491 (95%CI, 0.8704–1.0000) in external validation. The ORR model had AUC of 0.8030 (95%CI, 0.7437–0.8545) in internal validation and 0.7040 (95%CI, 0.5457–0.8379) in external validation. The PFS model had AUC of 0.8531 (95%CI, 0.8024–0.8975) in internal validation and 0.7602 (95%CI, 0.6236–0.8733) in external validation. The benefit of immunotherapy can be predicted by deep learning models that integrate patient clinical information. Compared with PD-L1 expression, the efficacy indicators DCR and ORR predicted it more accurately.
As a new efficacy prediction model, the deep learning model will have the potential to support clinical decision-making more accurately. She et al. evaluated the use of deep learning algorithms to evaluate the specific survival of NSCLC patients and concluded that deep learning was significantly better than previous models in lung cancer prognosis assessment and treatment recommendations (18). Mu et al. used PET/CT image deep learning to measure the PD-L1 state to predict immunotherapy response non-invasively and then found that deep learning can replace PD-L1 detected by immunohistochemistry (IHC) (49). In our study, we enrolled immunotherapy patients with lung squamous carcinoma, including stage I–IV, for model training and testing. The deep learning models have multiple hidden layers, each of which contains multiple nodes. The node weights between different layers are updated in time according to the loss function and the reverse propagation of the optimizer. Coupled with the application of activation functions, deep learning can better learn and simulate the non-linear relationship between predictive variables and outcomes than traditional statistical models and some machine learning algorithms. Realistic data, especially clinical data, have a relationship that is not simple linear but intricate. Deep learning is more suitable for the analysis and modeling of clinical data. Therefore, our research found that this will be an interesting attempt, and the models also showed satisfactory performance and could precisely predict the efficacy of immunotherapy.
Of note, ICIs have contributed to improving the survival of patients with lung squamous carcinoma. Our results found that first-line immunotherapy can increase DCR to 86.7% and ORR to 46.7%, which is similar to other clinical studies. In KEYNOTE-024 (50), pembrolizumab, compared with chemotherapy, can remarkably improve the ORR (44.8% vs. 27.8%).
With the development of immunotherapy, many studies have found that chemo-immunotherapy strategy can significantly improve the response of NSCLC patients (51–55). The KEYNOTE-407 (53) study found that first-line immunotherapy combined with chemotherapy was better than chemotherapy; the ORR was 66.6% versus 38.4%, respectively. A plethora of phase III clinical trials such as IMpower110 (54), CameL-Sq (52), ORIT-12 (51), and GEMSTONE-302 (55) showed superior efficacy with ICIs plus chemotherapy compared with chemotherapy alone. In our study, we observed that the DCR of patients who received chemoimmunotherapy was 97.7% and ORR was 62.93%, which were similar to the above studies. To be brief, first-line immunotherapy combined with chemotherapy has rapidly expanded first-line treatment options for advanced NSCLC patients without sensitizing epidermal growth factor receptor (EGFR) mutations or anaplastic lymphoma kinase (ALK) fusions.
The success of ICIs in NSCLC has expanded to unresectable stage III and more recently to resectable stage II–IIIA disease. The NADIM study supported the addition of neoadjuvant nivolumab to platinum-based chemotherapy in patients with resectable stage IIIA NSCLC; the major pathological response (MPR) rate was 83%, pathological complete response (pCR) rate was 71%, and 90% of patients had tumor stage decline (33 cases) (56). In CheckMate 816, the neoadjuvant treatment of nivolumab plus chemotherapy improved the pCR rate (24.0% vs. 2.2%) in resectable NSCLC patients, and median OS showed a beneficial trend (57). In a neoadjuvant study of sintilimab combined with chemotherapy, 40.5% and 10.8% of patients attained MPR and pCR, respectively, and 3-year OS and disease-free survival (DFS) rates were 95.5% and 81.8%, respectively (58). In our study, we found that the MPR rate of patients with neoadjuvant immunotherapy was 58.4% and the pCR rate was 38.9%, which was similar to the above studies. Neoadjuvant chemoimmunotherapy could change the perception of locally advanced lung cancer from being a potentially lethal disease to one that is curable.
In summary, it is found that immunotherapy can improve the efficacy of patients to a certain extent, but the factors affecting immune response and specific immune resistance mechanisms are multifaceted.
However, this study still has some limitations. Neural network algorithms have the disadvantages of black boxes, which are complex to explain with time-consuming training. Biomarkers such as tumor mutation burden (TMB) were not included. Additionally, this model requires more multi-center prospective data to validate. Further study is needed to validate the advantages of deep learning networks in immunotherapy predictive models.
Conclusion
In conclusion, this study found that the neural network model based on clinical information can accurately predict the efficacy benefits of ICI therapies for lung squamous carcinoma patients, especially DCR and ORR. This novel predictive model may provide reliable individual response information and treatment recommendations. In our retrospective study, we found that neoadjuvant and adjuvant immunotherapy may bring greater efficacy benefits to patients.
Data availability statement
The raw data supporting the conclusions of this article will be made available by the authors, without undue reservation.
Ethics statement
This research has been approved by the Ethics Committee of Beijing Chest Hospital affiliated with Capital Medical University. Given that this was a retrospective analysis, individual consent was waived.
Author contributions
JW, SL, WL and TM came up with the idea for the study. SL, TM, SF, XG, NQ, YW, XZ and JW collected the data and conducted the follow-up. WL carried out the statistical analysis. SL, TM and WL wrote the manuscript. JW, ZL and YP edited and reviewed the manuscript. All authors discussed the results and commented on the manuscript. All the authors contributed to the article and approved the submitted version.
Conflict of interest
The authors declare that the research was conducted in the absence of any commercial or financial relationships that could be construed as a potential conflict of interest.
Publisher’s note
All claims expressed in this article are solely those of the authors and do not necessarily represent those of their affiliated organizations, or those of the publisher, the editors and the reviewers. Any product that may be evaluated in this article, or claim that may be made by its manufacturer, is not guaranteed or endorsed by the publisher.
Supplementary material
The Supplementary Material for this article can be found online at: https://www.frontiersin.org/articles/10.3389/fimmu.2022.1024707/full#supplementary-material
Supplementary Figure 1 | The distribution of missing values of clinical features in this study. PD-L1, programmed cell death ligand 1. BOR, best of response. ORR, objective response rate. DCR, disease control rate. PFS, progression-free survival. OS, overall survival.
Supplementary Figure 2 | The training curves of (A) DCR model, (B) ORR model, (C) PFS model and (D) OS model. DCR, disease control rate. ORR, objective response rate. PFS, progression-free survival. OS, overall survival.
Supplementary Figure 3 | The Kaplan-Meier curve compared the overall survival of patients with different gene mutations. Uncommon gene mutation.
Addition File 1 | The original codes of DCR model, ORR model and PFS model. DCR, disease control rate. ORR, objective response rate. PFS, progression-free survival.
Addition File 2 | The original codes of OS model. OS, overall survival.
Addition File 3 | The weights and hyper-parameters of DCR model, ORR model, PFS model and OS model. DCR, disease control rate. ORR, objective response rate. PFS, progression-free survival. OS, overall survival.
Addition File 4 | The clinical data used by this study.
Abbreviations
AJCC, American Joint Committee on Cancer; AI, artificial intelligence; ALK, anaplastic lymphoma kinase; AUC, area under the receiver operating characteristic curve; BOR, best overall response; CI, confidence interval; CR, complete response; DCR, disease control rate; DNN, dense neural network; EGFR, epidermal growth factor receptor; IHC, immunohistochemistry; MPR, major pathological response; NSCLC, non-small cell lung cancer; ORR, objective response rate; OS, overall survival; PD, progressive disease; PD-1, programmed cell death 1; PD-L1, programmed cell death ligand 1; PFS, progression-free survival; pCR, pathological complete response; PR, partial response; SD, stable disease, TPS, tumor proportion score.
References
1. Siegel RL, Miller KD, Fuchs HE, Jemal A. cancer statistics, 2021. CA Cancer J Clin (2021) 71(1):7–33. doi: 10.3322/caac.21654
2. Siegel RL, Miller KD, Fuchs HE, Jemal A. Cancer statistics, 2022. CA Cancer J Clin (2022) 72(1):1–27. doi: 10.3322/caac.21708
3. Duma N, Santana-Davila R, Molina JR. Non-small cell lung cancer: epidemiology, screening, diagnosis, and treatment. Mayo Clin Proc (2019) 94(8):1623–40. doi: 10.1016/j.mayocp.2019.01.013
4. Goldstraw P, Chansky K, Crowley J, Rami-Porta R, Asamura H, Eberhardt WEE, et al. The IASLC lung cancer staging project: Proposals for revision of the tnm stage groupings in the forthcoming (eighth) edition of the tnm classification for lung cancer. J Thorac Oncol (2016) 11(1):39–51. doi: 10.1016/j.jtho.2015.09.009
5. Wang J, Shen Q, Shi Q, Yu B, Wang X, Cheng K, et al. Detection of ALK protein expression in lung squamous cell carcinomas by immunohistochemistry. J Exp Clin Cancer Res (2014) 33:109. doi: 10.1186/s13046-014-0109-2
6. Houston KA, Henley SJ, Li J, White MC, Richards TB. Patterns in lung cancer incidence rates and trends by histologic type in the United States, 2004-2009. Lung Cancer (2014) 86(1):22–8. doi: 10.1016/j.lungcan.2014.08.001
7. Caliò A, Nottegar A, Gilioli E, Bria E, Pilotto S, Peretti U, et al. ALK/EML4 fusion gene may be found in pure squamous carcinoma of the lung. J Thorac Oncol (2014) 9(5):729–32. doi: 10.1097/JTO.0000000000000109
8. Youlden DR, Cramb SM, Baade PD. The international epidemiology of lung cancer: geographical distribution and secular trends. J Thorac Oncol (2008) 3(8):819–31. doi: 10.1097/JTO.0b013e31818020eb
9. Forbes SA, Bhamra G, Bamford S, Dawson E, Kok C, Clements J, et al. The Catalogue of Somatic Mutations in Cancer (COSMIC). Curr Protoc Hum Genet (2008). doi: 10.1002/0471142905.hg1011s57
10. Mazieres J, Rittmeyer A, Gadgeel S, Hida T, Gandara DR, Cortinovis DL, et al. Atezolizumab versus docetaxel in pretreated patients with nsclc: Final results from the randomized phase 2 poplar and phase 3 oak clinical trials. J Thorac Oncol (2021) 16(1):140–50. doi: 10.1016/j.jtho.2020.09.022
11. Herbst RS, Garon EB, Kim D-W, Cho BC, Gervais R, Perez-Gracia JL, et al. Five year survival update from keynote-010: Pembrolizumab versus docetaxel for previously treated, programmed death-ligand 1-positive advanced nsclc. J Thorac Oncol (2021) 16(10):1718–32. doi: 10.1016/j.jtho.2021.05.001
12. Borghaei H, Gettinger S, Vokes EE, Chow LQM, Burgio MA, de Castro Carpeno J, et al. Five-year outcomes from the randomized, phase iii trials checkmate 017 and 057: Nivolumab versus docetaxel in previously treated non-small-cell lung cancer. J Clin Oncol (2021) 39(7):723–33. doi: 10.1200/JCO.20.01605
13. Bie F, Tian H, Sun N, Zang R, Zhang M, Song P, et al. Research progress of anti-pd-1/pd-l1 immunotherapy related mechanisms and predictive biomarkers in nsclc. Front Oncol (2022) 12:769124. doi: 10.3389/fonc.2022.769124
14. Patel SP, Kurzrock R. PD-L1 expression as a predictive biomarker in cancer immunotherapy. Mol Cancer Ther (2015) 14(4):847–56. doi: 10.1158/1535-7163.MCT-14-0983
15. Shimoda Y, Yoshida T, Shirasawa M, Mizuno T, Jo H, Matsumoto Y, et al. Smoking history predicts high presence of tils and efficacy of pd-1 blockade in pd-l1 expression-negative non-small cell lung cancer patients. Anticancer Res (2021) 41(11):5739–47. doi: 10.21873/anticanres.15390
16. Nosaki K, Saka H, Hosomi Y, Baas P, de Castro G, Reck M, et al. Safety and efficacy of pembrolizumab monotherapy in elderly patients with PD-L1-positive advanced non-small-cell lung cancer: Pooled analysis from the KEYNOTE-010, KEYNOTE-024, and KEYNOTE-042 studies. Lung Cancer (2019) 135:188–95. doi: 10.1016/j.lungcan.2019.07.004
17. Gettinger SN, Horn L, Gandhi L, Spigel DR, Antonia SJ, Rizvi NA, et al. Overall survival and long-term safety of nivolumab (anti-programmed death 1 antibody, bms-936558, ono-4538) in patients with previously treated advanced non-small-cell lung cancer. J Clin Oncol (2015) 33(18):2004–12. doi: 10.1200/JCO.2014.58.3708
18. She Y, Jin Z, Wu J, Deng J, Zhang L, Su H, et al. Development and validation of a deep learning model for non-small cell lung cancer survival. JAMA Netw Open (2020) 3(6):e205842. doi: 10.1001/jamanetworkopen.2020.5842
19. Xu Y, Hosny A, Zeleznik R, Parmar C, Coroller T, Franco I, et al. Deep learning predicts lung cancer treatment response from serial medical imaging. Clin Cancer Res (2019) 25(11):3266–75. doi: 10.1158/1078-0432.CCR-18-2495
20. Nam JG, Park S, Hwang EJ, Lee JH, Jin K-N, Lim KY, et al. Development and validation of deep learning-based automatic detection algorithm for malignant pulmonary nodules on chest radiographs. Radiology (2019) 290(1):218–28. doi: 10.1148/radiol.2018180237
21. Kather JN, Pearson AT, Halama N, Jäger D, Krause J, Loosen SH, et al. Deep learning can predict microsatellite instability directly from histology in gastrointestinal cancer. Nat Med (2019) 25(7):1054–6. doi: 10.1038/s41591-019-0462-y
22. Hwang EJ, Park S, Jin K-N, Kim JI, Choi SY, Lee JH, et al. Development and validation of a deep learning-based automated detection algorithm for major thoracic diseases on chest radiographs. JAMA Netw Open (2019) 2(3):e191095. doi: 10.1001/jamanetworkopen.2019.1095
23. Van Buuren S, Groothuis-Oudshoorn K. Mice: Multivariate imputation by chained equations in r. J Stat software (2011) 45:1–67. doi: 10.18637/jss.v045.i03
24. McKinney W. (2010). Data structures for statistical computing in python, in: Proceedings of the 9th Python in Science Conference, Austin, TX. 51–56
25. Pedregosa F, Varoquaux G, Gramfort A, Michel V, Thirion B, Grisel O, et al. Scikit-learn: Machine learning in Python. J Mach Learn Res (2011) 12:2825–30.
26. Katzman JL, Shaham U, Cloninger A, Bates J, Jiang T, Kluger Y. DeepSurv: personalized treatment recommender system using a Cox proportional hazards deep neural network. BMC Med Res Methodol (2018) 18(1):24. doi: 10.1186/s12874-018-0482-1
28. Paszke A, Gross S, Massa F, Lerer A, Bradbury J, Chanan G, et al. PyTorch: An imperative style, high-performance deep learning library. Adv Neur In (2019), 32.
29. Harris CR, Millman KJ, van der Walt SJ, Gommers R, Virtanen P, Cournapeau D, et al. Array programming with NumPy. Nature (2020) 585(7825):357–62. doi: 10.1038/s41586-020-2649-2
30. Hunter JD. Matplotlib: A 2D graphics environment. Computing Sci Eng (2007) 9(03):90–5. doi: 10.1109/MCSE.2007.55
31. Kvamme H, Borgan Ø, Scheel I. Time-to-event prediction with neural networks and Cox regression. arXiv preprint arXiv:190700825 (2019).
32. Robin X, Turck N, Hainard A, Tiberti N, Lisacek F, Sanchez J-C, et al. pROC: an open-source package for R and S+ to analyze and compare ROC curves. BMC Bioinf (2011) 12(1):1–8. doi: 10.1186/1471-2105-12-77
33. Wei T, Simko V, Levy M, Xie Y, Jin Y, Zemla J. Package ‘corrplot’. Statistician (2017) 56(316):e24.
34. Gu Z, Gu L, Eils R, Schlesner M, Brors B. Circlize implements and enhances circular visualization in R. Bioinformatics (2014) 30(19):2811–2. doi: 10.1093/bioinformatics/btu393
35. Kassambara A, Kosinski M, Biecek P, Fabian S. Package ‘survminer’. Drawing Survival Curves using ‘ggplot2’(R Package version 03 1) (2017).
38. Sunshine J, Taube JM. PD-1/PD-L1 inhibitors. Curr Opin In Pharmacol (2015) 23:32–8. doi: 10.1016/j.coph.2015.05.011
39. Rittmeyer A, Barlesi F, Waterkamp D, Park K, Ciardiello F, von Pawel J, et al. Atezolizumab versus docetaxel in patients with previously treated non-small-cell lung cancer (OAK): a phase 3, open-label, multicentre randomised controlled trial. Lancet (2017) 389(10066):255–65. doi: 10.1016/S0140-6736(16)32517-X
40. Mamdani H, Matosevic S, Khalid AB, Durm G, Jalal SI. Immunotherapy in lung cancer: Current landscape and future directions. Front Immunol (2022) 13:823618. doi: 10.3389/fimmu.2022.823618
41. Fehrenbacher L, Spira A, Ballinger M, Kowanetz M, Vansteenkiste J, Mazieres J, et al. Atezolizumab versus docetaxel for patients with previously treated non-small-cell lung cancer (POPLAR): a multicentre, open-label, phase 2 randomised controlled trial. Lancet (2016) 387(10030):1837–46. doi: 10.1016/S0140-6736(16)00587-0
42. Gandhi L, Rodríguez-Abreu D, Gadgeel S, Esteban E, Felip E, De Angelis F, et al. Pembrolizumab plus chemotherapy in metastatic non-small-cell lung cancer. New Engl J Med (2018) 378(22):2078–92. doi: 10.1056/NEJMoa1801005
43. Brahmer J, Reckamp KL, Baas P, Crinò L, Eberhardt WEE, Poddubskaya E, et al. Nivolumab versus docetaxel in advanced squamous-cell non-small-cell lung cancer. New Engl J Med (2015) 373(2):123–35. doi: 10.1056/NEJMoa1504627
44. Borghaei H, Paz-Ares L, Horn L, Spigel DR, Steins M, Ready NE, et al. Nivolumab versus docetaxel in advanced nonsquamous non-small-cell lung cancer. New Engl J Med (2015) 373(17):1627–39. doi: 10.1056/NEJMoa1507643
45. Carbone DP, Reck M, Paz-Ares L, Creelan B, Horn L, Steins M, et al. First-line nivolumab in stage iv or recurrent non-small-cell lung cancer. New Engl J Med (2017) 376(25):2415–26. doi: 10.1056/NEJMoa1613493
46. Satturwar S, Girolami I, Munari E, Ciompi F, Eccher A, Pantanowitz L. Program death ligand-1 immunocytochemistry in lung cancer cytological samples: A systematic review. Diagn Cytopathol (2022) 50(6):313–23. doi: 10.1002/dc.24955
47. Paolino G, Pantanowitz L, Barresi V, Pagni F, Munari E, Moretta L, et al. PD-L1 evaluation in head and neck squamous cell carcinoma: Insights regarding specimens, heterogeneity and therapy. Pathol Res Pract (2021) 226:153605. doi: 10.1016/j.prp.2021.153605
48. Marletta S, Fusco N, Munari E, Luchini C, Cimadamore A, Brunelli M, et al. Atlas of pd-l1 for pathologists: indications, scores, diagnostic platforms and reporting systems. J Pers Med (2022) 12(7):1–16. doi: 10.3390/jpm12071073
49. Mu W, Jiang L, Shi Y, Tunali I, Gray JE, Katsoulakis E, et al. Non-invasive measurement of PD-L1 status and prediction of immunotherapy response using deep learning of PET/CT images. J Immunother Cancer (2021) 9(6):1–15. doi: 10.1136/jitc-2020-002118
50. Reck M, Rodríguez-Abreu D, Robinson AG, Hui R, Csőszi T, Fülöp A, et al. Pembrolizumab versus chemotherapy for pd-l1-positive non-small-cell lung cancer. New Engl J Med (2016) 375(19):1823–33. doi: 10.1056/NEJMoa1606774
51. Zhou C, Wu L, Fan Y, Wang Z, Liu L, Chen G, et al. Sintilimab plus platinum and gemcitabine as first-line treatment for advanced or metastatic squamous nsclc: Results from a randomized, double-blind, phase 3 trial (orient-12). J Thorac Oncol (2021) 16(9):1501–11. doi: 10.1016/j.jtho.2021.04.011
52. Ren S, Chen J, Xu X, Jiang T, Cheng Y, Chen G, et al. Camrelizumab plus carboplatin and paclitaxel as first-line treatment for advanced squamous nsclc (camel-sq): A phase 3 trial. J Thorac Oncol (2022) 17(4):544–57. doi: 10.1016/j.jtho.2021.11.018
53. Paz-Ares L, Vicente D, Tafreshi A, Robinson A, Soto Parra H, Mazieres J, et al. A randomized, placebo-controlled trial of pembrolizumab plus chemotherapy in patients with metastatic squamous nsclc: Protocol-specified final analysis of keynote-407. J Thorac Oncol (2020) 15(10):1657–69. doi: 10.1016/j.jtho.2020.06.015
54. Herbst RS, Giaccone G, de Marinis F, Reinmuth N, Vergnenegre A, Barrios CH, et al. Atezolizumab for first-line treatment of pd-l1-selected patients with nsclc. N Engl J Med (2020) 383(14):1328–39. doi: 10.1056/NEJMoa1917346
55. Zhou C, Wang Z, Sun Y, Cao L, Ma Z, Wu R, et al. Sugemalimab versus placebo, in combination with platinum-based chemotherapy, as first-line treatment of metastatic non-small-cell lung cancer (GEMSTONE-302): interim and final analyses of a double-blind, randomised, phase 3 clinical trial. Lancet Oncol (2022) 23(2):220–33. doi: 10.1016/S1470-2045(21)00650-1
56. Provencio M, Nadal E, Insa A, García-Campelo MR, Casal-Rubio J, Dómine M, et al. Neoadjuvant chemotherapy and nivolumab in resectable non-small-cell lung cancer (NADIM): an open-label, multicentre, single-arm, phase 2 trial. Lancet Oncol (2020) 21(11):1413–22. doi: 10.1016/s1470-2045(20)30453-8
57. Forde PM, Spicer J, Lu S, Provencio M, Mitsudomi T, Awad MM, et al. Neoadjuvant nivolumab plus chemotherapy in resectable lung cancer. N Engl J Med (2022) 386(21):1973–85. doi: 10.1056/NEJMoa2202170
Keywords: immunotherapy, lung squamous carcinoma, neural network, deep learning, predictive model
Citation: Li S, Li W, Ma T, Fu S, Gao X, Qin N, Wu Y, Zhang X, Wang J, Pan Y and Liu Z (2022) Assessing the efficacy of immunotherapy in lung squamous carcinoma using artificial intelligence neural network. Front. Immunol. 13:1024707. doi: 10.3389/fimmu.2022.1024707
Received: 22 August 2022; Accepted: 07 November 2022;
Published: 28 November 2022.
Edited by:
Jinzhuang Dou, University of Texas MD Anderson Cancer Center, United StatesReviewed by:
Albino Eccher, Integrated University Hospital Verona, ItalyHaiyu Zhou, Guangdong Provincial People’s Hospital, China
Copyright © 2022 Li, Li, Ma, Fu, Gao, Qin, Wu, Zhang, Wang, Pan and Liu. This is an open-access article distributed under the terms of the Creative Commons Attribution License (CC BY). The use, distribution or reproduction in other forums is permitted, provided the original author(s) and the copyright owner(s) are credited and that the original publication in this journal is cited, in accordance with accepted academic practice. No use, distribution or reproduction is permitted which does not comply with these terms.
*Correspondence: Jinghui Wang, amluZ2h1aXdhbmcyMDA2QDE2My5jb20=; Zhidong Liu, bGl1emhpZG9uZ0BianhreXkuY24=; Yuanming Pan, cGV0ZXIuZi5wYW5AaHNjLnBrdS5lZHUuY24=
†These authors have contributed equally to this work