- 1Department of Medical Oncology, Clinical Oncology School of Fujian Medical University, Fujian Cancer Hospital, Fuzhou, China
- 2Cancer Bio-immunotherapy Center, Clinical Oncology School of Fujian Medical University, Fujian Cancer Hospital, Fuzhou, China
- 3Geneplus-Beijing Institute, Beijing, China
- 4Fujian Provincial Key Laboratory of Tumor Biotherapy, Clinical Oncology School of Fujian Medical University, Fujian Cancer Hospital, Fuzhou, China
- 5State Key Laboratory of Microbial Resources, Institute of Microbiology, Chinese Academy of Sciences, Beijing, China
- 6Department of Translational Medicine, GenePlus- Shenzhen Clinical Laboratory, ShenZhen, China
- 7Department of Radiation Oncology, Clinical Oncology School of Fujian Medical University, Fujian Cancer Hospital, Fuzhou, China
- 8Department of Pathology, Clinical Oncology School of Fujian Medical University, Fujian Cancer Hospital, Fuzhou, China
- 9The Third Affiliated Hospital of Soochow University, Institutes for Translational Medicine, Soochow University, Suzhou, China
Background: This study aimed to develop a vaccine that targets mutation-derived neoantigen in Chinese non-small-cell lung cancer (NSCLC).
Methods: A cohort of 1862 Chinese NSCLC patients who underwent targeted sequencing with a 1021-gene panel was investigated. HLA typing was done using OptiType v1.0 and neoantigens were predicted by netMHCpan v4.0. HLA LOH was inferred using the lohhla algorithm and TMB were quantified by counting the total number of non-synonymous ones based on our panel data. CIBERSORT was utilized to estimate the TME in different EGFR mutant subtype by using TCGA data.
Results: HLA-A*11:01(42.59%) was the top one allele and HLA-A*33:03(12.94%) ranked 12th. EGFR L858R (22.61%) was the most prevalent gene variant. The binding affinity (IC50 MT = 22.9 nM) and shared frequency (2.93%) of EGFR L858R in combination with HLA-A*33:03 were optimal. In a subsequent further analysis on immunological features of EGFR mutant subtypes, 63.1% HLA loss of heterozygosity LOH (HLA LOH) and 0.37% (7 of 1862) B2M aberrations were found in our population, both had no significant association with EGFR mutant subtypes suggesting that the process of antigen presentation involved HLA LOH and B2M mechanisms in EGFR L858R is working. Tumor mutation burden (TMB) was investigated by utilizing our panel and showed that EGFR L858R had the lowest TMB compared with other EGFR mutant subtypes. In addition, analysis of 22 immune cell types from The Cancer Genome Atlas (TCGA) data showed EGFR L858R was correlated with low level of CD8 T cells, activated CD4 memory T cells and elevated level of macrophage M2 suggesting an inhibited tumor microenvironment (TME).
Conclusion: Our study identified that EGFR L858R neoantigen had the potential to generate cancer vaccines in NSCLC patients with HLA A*33:03. The neoantigen-based vaccines may become an effective salvage regimen for EGFR L858R subgroup after targeted therapy or immune checkpoint inhibitors (ICIs) failure.
Introduction
Lung cancer is still the most common malignancy with morbidity and mortality both ranking first worldwide, and non-small-cell lung cancer (NSCLC) is a subset of lung cancer that has extensive clinical and molecular heterogeneity (1, 2). Epidermal growth factor receptor (EGFR) mutations are the most common driver genes in NSCLC, followed by RAS and ALK (3, 4). Only a subset of patients initially responds to targeted therapy, nonetheless, the majority inevitably acquire drug resistance (5–7).
Currently, immune checkpoint inhibitors (ICIs) have achieved positive laboratory results and remarkable clinical responses in the treatment of many kinds of cancer, including NSCLC (8–16). However, in the NSCLC clinical trials, EGFR mutant patients benefit less from ICIs than patients with KARS, BRAF, and MET mutations (8, 17–19). Previous studies have reported that antigen expression and presentation deficiency, the low mutation burden, immunosuppressive microenvironment, and upregulation of PD-L1 may be the mechanisms that limited efficacy of ICIs in EGFR mutant NSCLC patients (2, 20–23).
Yet, some NSCLC patients whose tumors are harboring EGFR mutations do respond to ICIs and studies have continued to focused on the tumor immune phenotype or somatic mutation features to develop novel and more effective treatments for this population. To date, the strategy that utilizes individualized neoantigen vaccines derived from mutated genes against cancers has achieved success in both mouse models and the clinical settings (7). Neoantigens generated from tumor-specific somatic mutations are the optimal targets for T-cells and are capable of mobilizing strong antitumor immune responses (24, 25).
To develop a vaccine that targets individualized neoantigen in NSCLC patients with EGFR mutations who do respond to ICIs, we performed a retrospective analysis of 1862 Chinese NSCLC tumor tissues matched with normal tissue samples which were previously profiled using our 1021-gene panel. We then assessed the expression of mutated alleles and predicted possible neoantigens. In this research, we have found that an EGFR L858R mutation could be a good target for the development of an individual vaccine for NSCLC patients with HLA A*33:03. We then presented a further investigation on immunological features (HLA LOH, B2M, TMB, and TME) of EGFR mutant subtypes to procure the evidence supporting the feasibility of EGFR L858R neoantigen. Our results not only provide useful information for predicting response to ICIs, but also introduce a promising treatment for Chinese NSCLC patients with EGFR mutations who were failed ICIs therapy and are without alternative therapy.
Materials and methods
Cohort
Clinical information of patients was collected from our records. Patients who were diagnosed with NSCLC and underwent targeted sequencing with a 1021-gene panel at Geneplus-Beijing (Beijing, China) were deemed eligible for analysis. For each patient tumor and normal (peripheral blood or normal tissue) samples were available. This study was approved by the Ethics Committee of Fujian Cancer Hospital. Written, informed consent was obtained from all participants before inclusion.
HLA typing
HLA typing was done using the OptiType v1.0 to obtain the four-digit HLA type at each locus of a patient (26). The Allele Frequency Net Database was utilized to retrieve the allele frequency (AF) of alleles in general Chinese Han populations and carrier frequencies were calculated according to this equation: carrier frequency = 1-(1-AF)2.
Neoantigen prediction and prioritization
For each patient, manually curated somatic mutations (missense or in-frame indel, AF≥0.05) in coding regions were also retrieved from previous records in our database. Neoantigens were predicted using netMHCpan v4.0 (27). Candidates with IC50 mut <500 nM and IC50 wild >=500 nM were considered for further analysis. A putative neoantigen was considered mutant-specific if the IC50 mut is <500 nM, and especially, it is considered as a “strong binder” if the IC50 mut is <50 nM.
Loss of Heterozygosity (LOH) in HLA genes
The LOH status at all three human leukocyte antigen (HLA) loci was inferred using the lohhla algorithm developed by McGranahan et al. (28). A locus was considered impacted by LOH if the computed p-value (‘PVal_unique’ in the output) was <0.01. A patient with a LOH at an HLA locus was defined as one who had at least one HLA locus impacted by LOH. All other patients (including those who have homozygous alleles at all three HLA loci) were considered not affected by HLA LOH.
Mutation number across four EGFR mutation type
Samples were categorized into four sub-groups: with L858R mutation, with deletions in exon 19 (19del), with other EGFR mutations, and EGFR wild types (WT). Mutations in each sample were quantified by counting the total number of non-synonymous ones. Group-wise Kruskal-Wallis tests were then performed.
NSCLC datasets and preprocessing in TCGA
Somatic mutations and RNA-sequencing (RNA-seq) data were downloaded from TCGA (https://portal.gdc.cancer.gov/). In consideration of no EGFR L858R mutation was found in 495 lung squamous cell carcinoma, therefore, we only mined mutation data from lung adenocarcinoma samples. The lung adenocarcinoma cohort was divided into four clusters as EGFR L858R (n=21), EGFR 19del (n=21), EGFR other (n=29) and EGFR WT (n=490). TCGA-LUAD (lung adenocarcinoma) FPKM data containing 594 cancer tissue samples were obtained. After exclusion, analysis was performed on a dataset of 513 lung adenocarcinoma patients who have EGFR mutation status data: EGFR L858R (n=21), EGFR 19del (n=19), EGFR other (n=28), EGFR WT (n=445).
Inference of infiltrating cells in TME
The CIBERSORT (http://cibersort.stanford.edu/) is an analytical tool developed by Newman et al. (29). To quantify the proportions of immune cells in tissue samples. We used the CIBERSORT algorithm and the LM22 gene signature, which was used to distinguish 22 immune cell phenotypes, including B-cells, T-cells, natural killer cells, macrophages, DCs, and myeloid subsets. We utilized CIBERSORT to estimate the fractions of 22 immune cell types among different EGFR mutant subtype.
Statistical analysis
P < 0.05 was considered statistically significant. All data were processed using the R software (version 3.6.0), GraphPad 7.0, and AdobeIllustratorCS6.
Results
Diversity and prevalence of HLA class I alleles
Data was collected from 1,862 patients who had been diagnosed with NSCLC and underwent targeted sequencing with a 1021-gene panel.
We recovered the HLA class I alleles (HLA-A, HLA-B, and HLA-C) for each patient from NGS data. We found 172 different alleles, of which 17 were carried by more than 10% of all patients (Figure 1). The most prevalent allele, A*11:01, was found in over 40% of all patients. HLA*11:01 and HLA-A*33:03 allele frequency (AF) are roughly comparable to the numbers retrieved from the Allele Frequency Net Database (30) (Supplementary Tables 1, 2). A rarefaction curve shows that the selected patients covered a large portion of HLA alleles, although it did not reach saturation (Supplementary Figure 1). Therefore, the selected population was not biased towards certain allele types and the reported carrier rates were reliable.
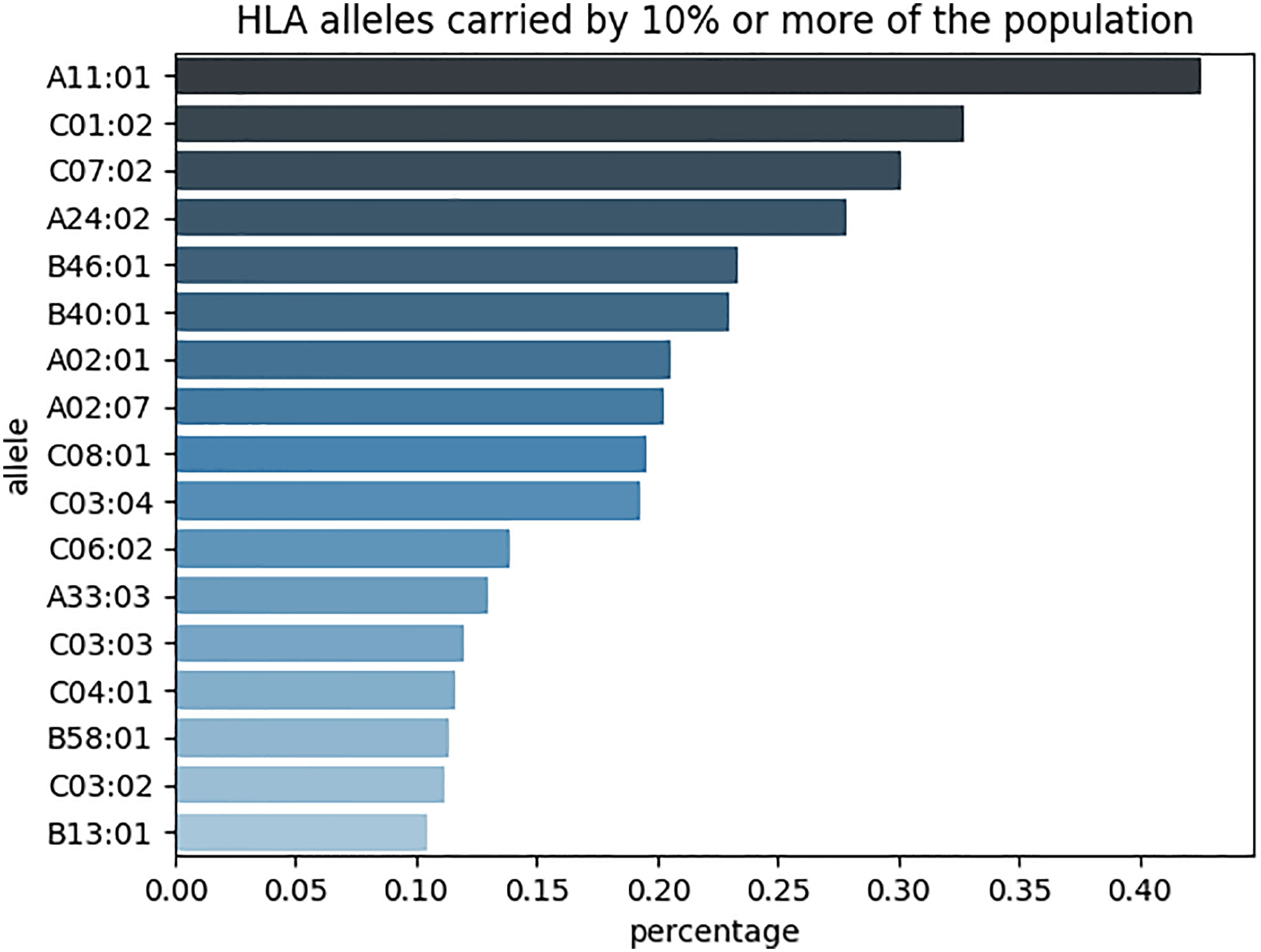
Figure 1 17 different HLA class I alleles (HLA-A, HLA-B, and HLA-C) were found in more than 10% of all patients (n=1862).
Recurrence of genes and mutations
Somatic missense or in-frame indel mutations were selected with an AF greater than or equal to 0.05 for neoantigen prediction and post-prediction analysis. We detected over 10,000 mutations across all patients (about 5 per patient). These mutations affected more than 800 genes. Genes EGFR and TP53 were the most frequently mutated genes, they were found mutated in 50% and 40% of all patients, respectively. They were followed by LRP1B and KRAS, which were mutated in 13% and 11% of all patients, respectively (Figure 2A). When inspected at variant level, EGFR mutations L858R and E746_A750del were overwhelmingly dominant. The frequencies were 23% and 13% for each, over 7-fold and 4-fold higher than the third mutation on the list. The EGFR genotyping results mainly agreed with previous studies in the prevalence of driver mutations in NSCLC patients (3, 4, 31). Interestingly, LRP1B mutations were not among the top at variant level (highest frequency at 0.11%), although the gene was mutated in a moderate proportion of patients (Figure 2B). Despite the aforementioned genes and mutations, a large majority of these genes and mutations were carried by few patients, typically less than 1% of the population.
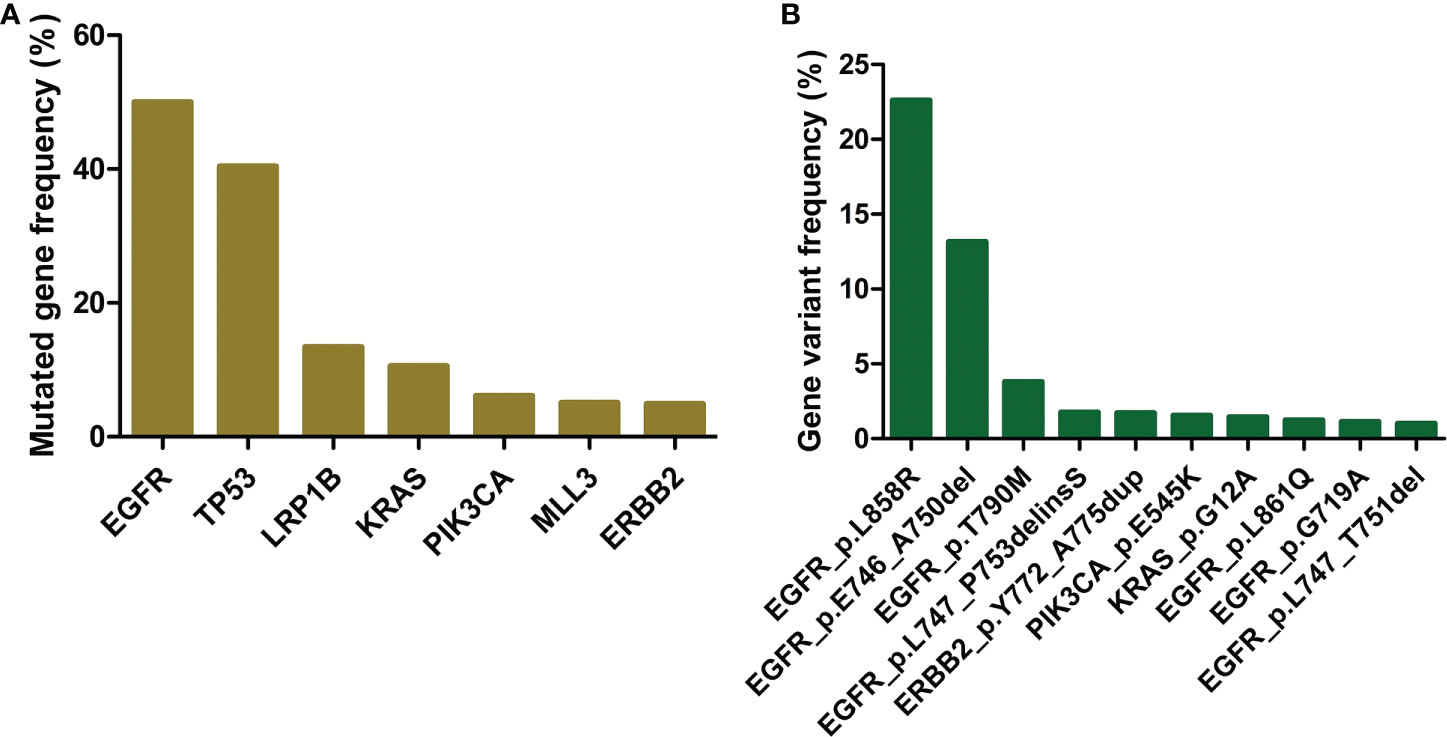
Figure 2 Recurrence of genes and mutations. (A) Mutated gene frequency among all patients. (B) Gene variant frequency among all patients.
Overview of putative neoantigens
All 8- to 11-mer peptides were derived from all selected mutations and predicted their binding affinity to the patient’s HLA class I complexes to identify patient-specific neoantigens. The prediction was performed for both mutant (MT) and wild type (WT) peptides. We considered a MT peptide a candidate neoantigen if the IC50 MT is smaller than 500 nM and the corresponding IC50 WT is greater than or equal to 500 nM. Furthermore, we categorized candidate neoantigens into “strong binder” and “weak binder” groups by the IC50 MT threshold of 50 nM.
We identified ~1900 candidate neoantigens in approximately 60% of all patients (1122/1862). The number of neoantigen ranged from 1 to 15, with a median of 1. More than half of the patients were predicted to possess only one neoantigen (Figure 3). We further shortened the list to 1438 unique neoantigens. A neoantigen was deemed “unique” if there was no other neoantigens derived from the same mutation and of the same amino acid sequence. About 35% of these unique neoantigens (504/1438) were derived from mutations found in less than 1% of patients. And among these, 19% (98/504) were strong binders (Figure 4A). Of the 934 unique neoantigens derived from frequently mutated genes (genes that were found mutated in more than 1% of all samples), 18% (169/934) were strong binders. Also, there were more than 1% of neoantigens (13/934) with ambiguous binding strength, as they were able to bind different HLA molecules with varying affinities. The neoantigens were further categorized into two groups by the mutation rate of the related genes (>1% samples vs. <=1% samples). No statistically significant difference was found between the proportions of strong and weak binders in the two groups (Chi-square Test, p = 0.61) (Figure 4B). The result indicates that concurrently mutated genes do not relate with increased proportion of strong binding neoantigens.
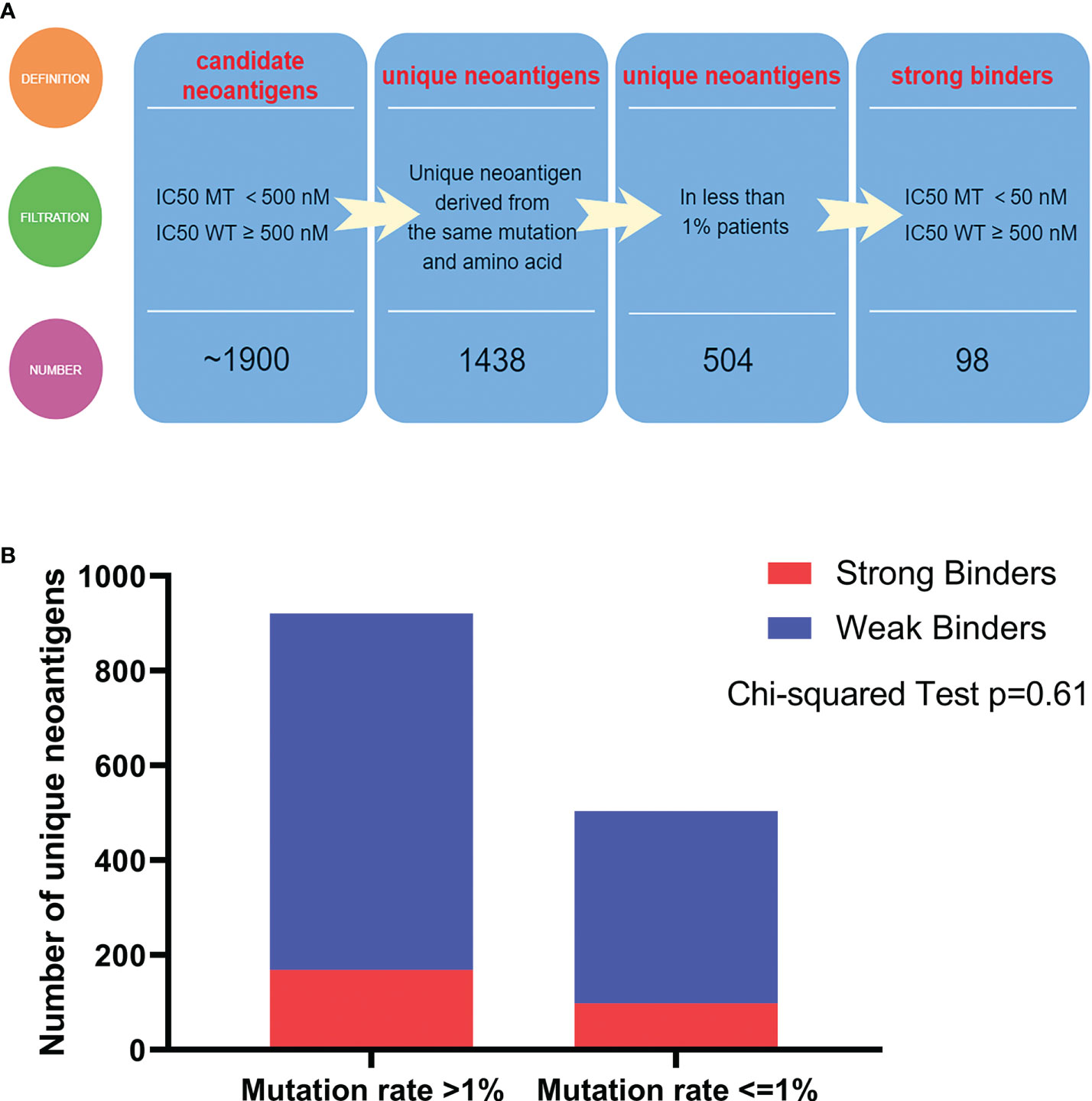
Figure 4 Overview of putative neoantigens. (A) Analytic pipelines to output putative neoantigens. (B) The proportions of strong and weak binders in two groups. The two groups were categorized by the mutation rate >1% samples vs. <=1% samples.
Quantifying neoantigens
The “neoantigen frequency” was calculated, defined in this study as the number of neoantigens related to a gene divided by the number of all mutations targeting this gene, for each neoantigen-producing gene (32). We did this from two perspectives: (1) the total neoantigen frequency, which reflects the “ability” of a gene to produce neoantigen, and (2) the neoantigen frequency corresponding to a specific HLA allele. Note that the latter might be larger than the former, because when calculating the total neoantigen frequency, neoantigens that were able to bind more than one HLA molecule were only counted once. Genes mutated in less than 1% of all patients were removed. By doing this, we also removed any genes targeted by less than 10 mutations. The EGFR, with a neoantigen frequency of 0.646, was the top one neoantigen producing gene, and most of these neoantigens were predicted to bind to A*11:01 (allele-specific neoantigen frequency 0.150). We noticed that some genes that were not so prevalent across patients still exhibited a high neoantigen frequency, like ERBB2 (0.563; mutated in 4.9% of patients), CTNNB1 (0.321; 3.2% of patients), and BRAF (0.357; 3.0% of patients) (Data not shown).
We repeated the above procedures at variant level. Instead of calculating frequency, we counted the number of neoantigens derived from a mutation directly, in total or HLA-specific. The number of per-mutation neoantigens ranged from 1 to 5. The EGFR L858R, the most prevalent mutation, produced four neoantigens. Two mutations were strong binders, while most mutations (except TP53 R110L, which was found in only 0.64% of all patients) produced no more than one strong binder (Figure 5).
Finding shared neoantigens
To find shared neoantigens that could be a good target for generalized neoantigen-based vaccines we further investigated the top two mutations by frequency; EGFR L858R and E746_A750del. The two strong binders derived from EGFR L858R were HVKITDFGR and RAKLLGAEEK. The latter binds to A*30:01 (IC50 MT = 47.5 nM and IC50 WT = 881.9 nM). Peptide HVKITDFGR binds to three HLA complexes; A*31:01 (IC50 MT = 18.9 nM and IC50 WT = 11653.7 nM), A*33:03 (IC50 MT = 22.9 nM and IC50 WT = 12734.0 nM), and A*68:01 (IC50 MT = 19.6 nM and IC50 WT = 8625.5 nM). The shared frequency of L858R and A*33:03 is 2.93% (22.61% × 12.94%).For the other two the percentages were 1.19% (A*31:01, 22.61% × 5.26%) and 0.28% (A*68:01, 22.61% × 1.24%). The most shared combination is E746_A750del and A*11:01 with a frequency of 5.60%. However, neither of the two neoantigens derived from this mutation was a strong binder (Table 1).
Prevalence of HLA LOH across NSCLC
In order to predict the ability to present neoantigens of different EGFR mutant subtypes (EGFR L858R, EGFR 19del, EGFR other rare, EGFR WT), we identified HLA LOH in our cohort. We analyzed 1731 tumor exomes and found 639 patients (36.9%) who were heterozygous at all HLA-I loci and 1092 patients (63.1%) who had LOH in at least one HLA-I locus in tumors in total (Supplementary Table 3). The HLA LOH occurrence rate was higher than the 40% reported in a previous study (28). HLA LOH was calculated for the EGFR WT (n=828) and EGFR mutant tumors harboring EGFR L858R (n=380), EGFR 19del (n=315), and EGFR other (n=139) (Figure 6A). We did not find that HLA LOH had any association with EGFR mutation status. Additionally, we examined the HLA LOH of selected HLA (A*33:03, A*31:01, and A*68:01) and also found no difference (Figure 6B).
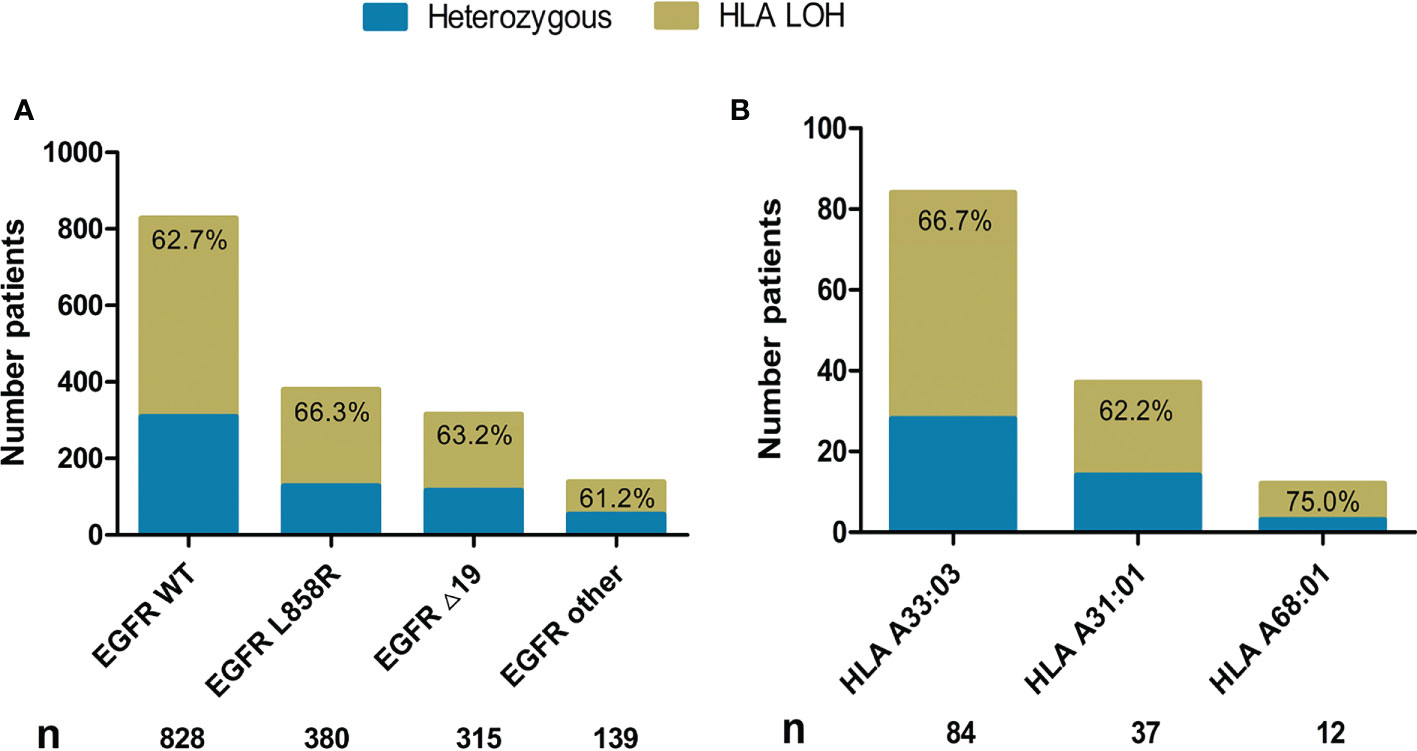
Figure 6 Frequency of HLA LOH in NSCLC. (A) The HLA LOH rate in the EGFR WT (n=828), EGFR L858R (n=380), EGFR 19del (n=315), and EGFR other (n=139). (B) The HLA LOH rate in HLA A*33:03 (n=84), HLA A*31:01 (n=37), and HLA A*68:01 (n=12).
Some mutations that are vital for antigen presentation and MHC class I expression were detected. In our cohort of 1862 NSCLC patients, only seven tumors were found to harbor β2-microglobulin (B2M) mutations and there was no difference among different EGFR mutant subtypes (Supplementary Table 4). No further mutations like TAP1, TAP2, LMP2 and LMP7 were identified in our cohort (Supplementary Table 5).
Association between EGFR mutant subtypes and mutation number
To examine whether the EGFR mutant status influenced the tumor mutation number, we determined the mutation numbers across EGFR mutation subtypes in NSCLC tumors from our cohorts. The median of EGFR WT (n=604) was five non-synonymous mutations, EGFR L858R (n=421) was three non-synonymous mutations, EGFR 19del (n=367) was three non-synonymous mutations, and EGFR other (n=145) was four non-synonymous mutations. The mutation number was significantly lower in EGFR L858R and EGFR 19del tumors compared with EGFR other and EGFR WT tumors. There was no difference between EGFR L858R and EGFR 19del, which was different from the previous report that EGFR 19del mutant lung cancers had a lower mutation number compared with EGFR L858R mutant lung cancers (2, 20) (Figure 7).
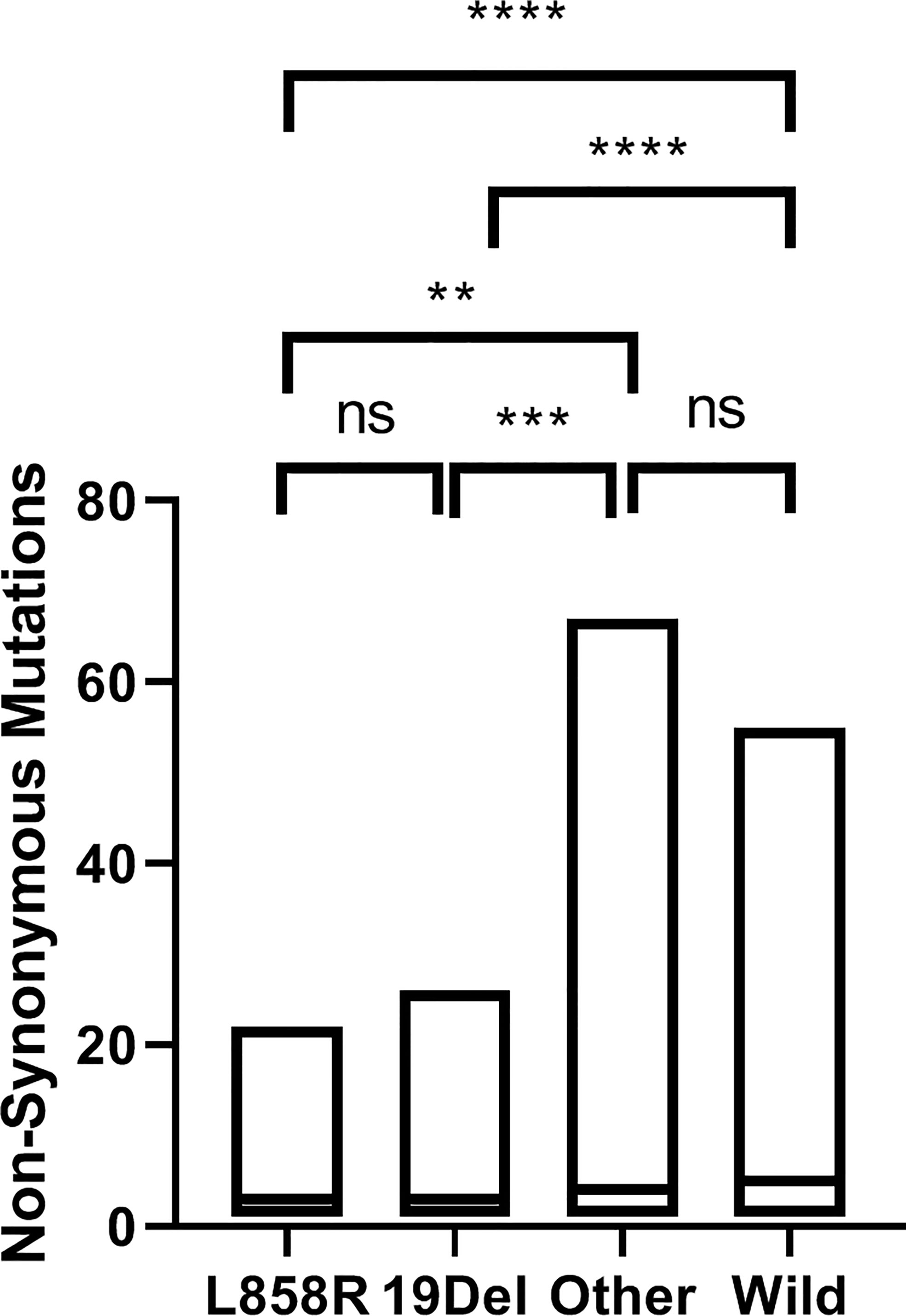
Figure 7 Non-synonymous mutations in NSCLC from our cohorts. The median non-synonymous mutations of EGFR L858R (n=421) was three, of EGFR 19del (n=367) was three, of EGFR other (n=145) was four and of EGFR WT (n=604) was five. **p < 0.01; ***p < 0.001; ****p < 0.0001 , ns, no significance.
Association between EGFR mutant subtypes and immune infiltration
When looking at the difference of 22 immune cells in EGFR mutant subtypes, EGFR L858R mutation were found to be associated with the relatively low level of CD8 T cells (P=0.00032), activated CD4 memory T cells signatures (P=0.0052) and elevated level of macrophage M2 (P=0.02) compared to EGFR WT tumors. However, the differences were not significant among EGFR L858R, EGFR 19del and EGFR other sites mutations (Figure 8).
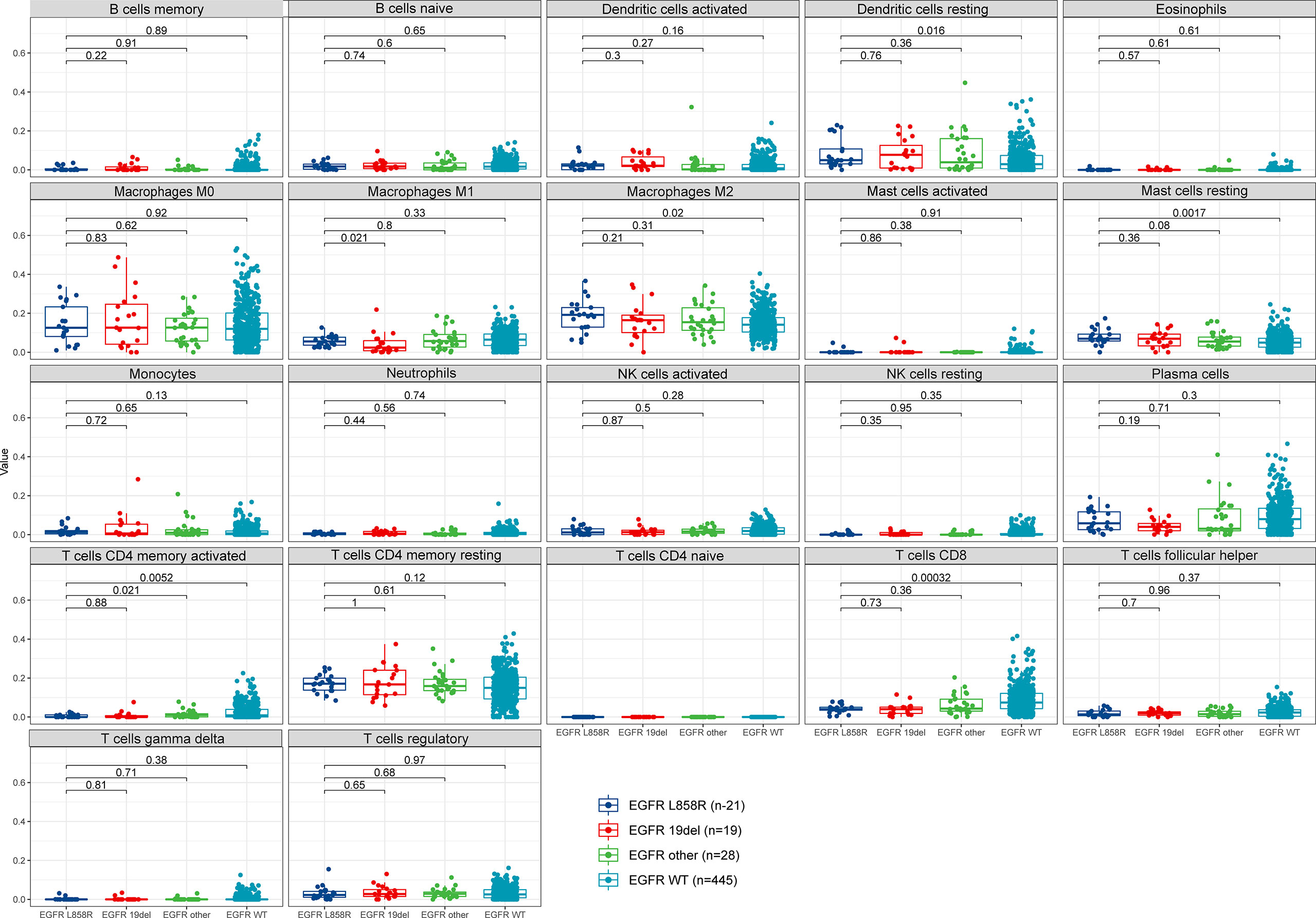
Figure 8 TME in NSCLC from TCGA database. TME, tumor microenvironment. TCGA, The Cancer Genome Atlas.
Discussion
NSCLC accounts for about 85% of all lung cancers and is a tumor with a high mutational load (33). Although NSCLC harbors many known driven mutations, the inter-individual genomic heterogeneity is extensive. Distinct molecular subtypes differ in sensitivity to various treatments (2). For instance, for treating EGFR-driven lung cancers, EGFR TKIs has been the first choice. However, the acquired resistance to TKIs is inevitable (5, 6, 34). As an emerging therapeutic approach with the potential for durable responses, ICIs are not recommended for EGFR-driven lung patients due to less benefit derived compared with other molecular subgroups (8, 17, 18, 35). However, an EGFR mutation is the most common gene alteration in NSCLC. There is a considerable need to find an effective treatment option to significantly increase immunotherapy efficacy in this subgroup.
In this study, we explored neoantigens in 1862 Chinese NSCLC patients who underwent targeted sequencing with a 1021-gene panel. Even though some of the same mutations were shared among different patients, not every mutation would play a role as a neoantigen as the binding affinity to each patient’s own HLA might vary (7, 36, 37). By combining the shared frequency and binding affinity to identify tumor specific somatic mutations, our data revealed that EGFR L858R was the top neoantigen producing gene allele, and most of these neoantigens were predicted to bind to A*33:03. We then presented a further analysis on immunological features of EGFR mutant subtypes to procure the evidence supporting the feasibility of EGFR L858R neoantigen.
A key step in neoantigen presentation and recognition by T-cell receptors is controlled by HLA. Hence, not only the binding affinity of the peptide to the HLA but also loss of HLA expression, which is caused by HLA haplotype loss or mutation of antigen presentation machinery genes such as B2M, needs to be taken into account (28, 38–40). We found that HLA LOH occurred in 63.1% NSCLC in our cohort, higher than 40% reported in a previous study and had no significant association with EGFR mutant subtypes (28). Furthermore, the HLA LOH of selected HLA (A*33:03, A*31:01, and A*68:01) also did not show any difference. We next examined B2M aberrations. Specifically, we found only one form of frameshift mutation in B2M: p.L15Ffs*41 and did not find B2M aberrations to be significantly enriched in any subtypes of EGFR mutations. Since B2M is essential to the assembly of all HLA class I complexes (41), and HLA LOH may facilitate immune evasion (38), our negative findings indicated that the EGFR L858R may not have a deficiency in neoantigen presentation, at least HLA LOH and B2M mutations did not play a crucial role in the immune resistance of EGFR L858R patients.
TMB contributes to enhancing antigenic response through the generation of neoantigens (42, 43). Accordingly, we next sought to evaluate the correlation between the attributes of the TMB and EGFR mutant subtypes using our panal. Our panel analyses demonstrated that EGFR L858R and EGFR 19del had the lowest TMB compared to other EGFR rare sites mutants and EGFR WT, though no difference was noted in EGFR L858R and EGFR 19del. This is in line with the lower response rate of EGFR mutant NSCLCs treated with ICIs, for which low TMB was deemed to be a major culprit of low efficiency of immunotherapy for EGFR L858R NSCLC. However, this was different from other studies that EGFR 19del mutant lung cancers had a lower TMB compared with EGFR L858R mutant lung cancers (2, 20), might be due to, for instance, different races, histology and stages. Moreover, tumor cells are embedded in the tumor microenvironment (TME), suggesting that intercellular relationships are as important as genomic factors (44, 45). In our study, we estimated the fractions of 22 immune cell types of NSCLC from TCGA and studied the correlation between the TME and EGFR mutant subtypes. We found EGFR L858R was correlated with lower percentage CD8 cells, lower percentage activated CD4 memory T cells and higher percentage macrophage M2 compared with EGFR WT. Taken together, these revealed an inhibited TME in the EGFR L858R subgroup.
We assembled the largest cohort of NSCLC cases to explore tumor-specific somatic mutations by targeted sequencing with a 1021-gene panel for developing neoantigen vaccines. In our analysis, the EGFR L858R neoantigen was identified in an HLA subtype-specific manner that could be used to generate cancer vaccines in HLA A*33:03 subsets patients. EGFR L858R in HLA A*33:03 patients would be relevant to 2.93% of the population. Given that lung cancer is the most common cancer, the percentage of patients who may benefit is considerable. We then proposed that the lower TMB and inhibited TME may be the reason for the week immunogenicity of the EGFR L858R subset of NSCLC. There were no deficiencies in the HLA LOH and B2M mechanisms, suggesting that the process of antigen presentation of EGFR L858R is working.
Our research has some insufficiency. One limitation was that a 1021-gene panel lacks sufficient sequencing data compared with WES or WGS, and only covers a proportion of all coding regions. With the exception of B2M, it did not cover gene mutations related to the HLA presentation which have been implicated as resistance mechanisms to ICIs, like TAP1, TAP2, LMP2 and LMP7 (40, 46, 47). However, since the panel covers most concurrently mutated genomic regions, it is capable of capturing necessary information. In addition, as an indispensable component of neoantigen peptide recognition, the T-cell receptor (TCR) repertoire profiling needs to be explored (48). Recent work on NSCLC has investigated whether the TCR repertoire enables assessment of T cell diversity and T cell clonal expansion and indicated that EGFR mutant tumors exhibits lower T cell clonal expansion (49, 50). In the future, we plan to perform TCR sequencing to elucidate whether there exist significant differences in the TCR repertoire diversity in EGFR mutant subtypes, aiming to investigate the distinct characteristics of TCR repertoire patterns in EGFR L858R. Another possible limitation of this study is that we lack available sequencing data to directly compare TME in this cohort. To address this, we utilized the TCGA data source, but this data source does not represent the real tumor immunogenomic landscape in our Chinese cohort. At last, this is a retrospective study and the clinical information like stages and treatment strategies were incomplete. So we could not conduct stratified analysis to explore some underlying mechanisms.
We excluded frameshift mutations from analysis. The rationale behind this is that there is a chance to raise false positives. Such mutations often result in premature termination codons, which cause the degradation of transcripts via nonsense-mediated mRNA decay (NMD) before translation (51). An approach to assess NMD efficiency through RNA-Seq has been published (52), but is not applicable here due to the lack of RNA-Seq data. Still, we estimated to what extent our findings are biased. A total of 975 frameshift mutations (915 unique) were detected, spanning 670 samples. We recalculated the mutation frequency for each gene with frameshift taken into account. The top 6 genes by mutation frequency did not change, while the remaining genes were reordered. For some genes, the mutation frequency increased after the recalculation, such as TP53 and LRP1B. This indicates that in some patients only frameshift mutations were detected on these genes. TP53 mutation frequency increased from 40.44% to 48.34%, indicating that we probably have underestimated its potential role in neoantigen producing. However, the most frequent frameshift mutation STK11 P281Rfs*6 was shared by only six patients, which translated into a percentage of 0.3% (Supplementary Table 4). This did not serve our purpose of finding shared neoantigens.
In summary, our research identified that EGFR L858R neoantigen had the potential to generate cancer vaccines in NSCLC patients with HLA A*33:03 and revealed the possible underlying immunological features between EGFR mutant subtypes. Our finding provides the basis for further investigations into which neoantigen-based vaccines may become an effective treatment strategy for patients with EGFR L858R mutation.
Data availability statement
The original contributions presented in the study are included in the article/Supplementary Material. Further inquiries can be directed to the corresponding authors.
Ethics statement
The studies involving human participants were reviewed and approved by the Ethics Committee of Fujian Cancer Hospital. The patients/participants provided their written informed consent to participate in this study.
Author contributions
JiL, JuL, S-GH, BL carried out the whole research. X-BZ, J-NX, Y-QZ, XG, Y-TY, XY statistically analyzed all the data and graphed. LC, Y-FH, HL, J-PL help in assays. C-BC, GC and X-WZ developed the concept. X-FW and YC obtained the funds and organized the study. All authors read and approved the final manuscript. All authors contributed to the article and approved the submitted version.
Funding
The work was supported by Fujian Provincial Clinical Research Center for Cancer Radiotherapy and Immunotherapy (Grant No. 2020Y2012), the National Natural Science Foundation of China (Grant No. U1705282, 32000550), Joint Funds for the Innovation of Science and Technology, Fujian province (Grant No. 2021Y9227) and Startup Fund for scientific research, Fujian Medical University (Grant No. 2019QH1200).
Acknowledgments
We appreciate all subjects who participated in this study and all colleagues (Cancer Bio-immunotherapy Center, Fujian Cancer Hospital) for technical support.
Conflict of interest
Authors S-GH, Y-QZ, XG, Y-TY, and XY were employed by company Geneplus.
The remaining authors declare that the research was conducted in the absence of any commercial or financial relationships that could be construed as a potential conflict of interest.
Publisher’s note
All claims expressed in this article are solely those of the authors and do not necessarily represent those of their affiliated organizations, or those of the publisher, the editors and the reviewers. Any product that may be evaluated in this article, or claim that may be made by its manufacturer, is not guaranteed or endorsed by the publisher.
Supplementary material
The Supplementary Material for this article can be found online at: https://www.frontiersin.org/articles/10.3389/fimmu.2022.1022598/full#supplementary-material
Supplementary Figure 1 | The rarefaction curve shows the selected population was not biased towards certain allele types.
Abbreviations
NSCLC, non-small-cell lung cancer; ICIs, immune checkpoint inhibitors; EGFR, Epidermal growth factor receptor; 19 del, deletions in exon 19; HLA, human leukocyte antigen; HLA LOH, HLA loss of heterozygosity; TMB, Tumor mutation burden; TCGA, The Cancer Genome Atlas; TME, tumor microenvironment.
References
1. Chen W, Sun K, Zheng R, Zeng H, Zhang S, Xia C, et al. ancer incidence and mortality in China, 2014. Chin J Cancer Res (2018) 30(1):1–12. doi: 10.21147/j.issn.1000-9604.2018.01.01
2. Hastings K, Yu HA, Wei W, Sanchez-Vega F, DeVeaux M, Choi J, et al. EGFR mutation subtypes and response to immune checkpoint blockade treatment in non-small-cell lung cancer. Ann Oncol (2019) 30(8):1311–20. doi: 10.1093/annonc/mdz141
3. Liang W, Guo M, Pan Z, Cai X, Li C, Zhao Y, et al. Association between certain non-small cell lung cancer driver mutations and predictive markers for chemotherapy or programmed death-ligand 1 inhibition. Cancer Sci (2019) 110(6):2014–21. doi: 10.1111/cas.14032
4. Gou LY, Wu YL. Prevalence of driver mutations in non-small-cell lung cancers in the people's republic of China. Lung Cancer (2014) 5(default):1–9. doi: 10.2147/LCTT.S40817
5. Wu SG, Shih JY. Management of acquired resistance to EGFR TKI–targeted therapy in advanced non-small cell lung cancer. Mol Cancer (2018) 17(1):38. doi: 10.1186/s12943-018-0777-1
6. Stinchcombe TE, Borghaei H, Barker SS, Treat JA, Obasaju C. Pemetrexed with platinum combination as a backbone for targeted therapy in non-Small-Cell lung cancer. Clin Lung Cancer (2016) 17(1):1–9. doi: 10.1016/j.cllc.2015.07.002
7. Carreno BM, Magrini V, Becker-Hapak M, Kaabinejadian S, Hundal J, Petti AA, et al. Cancer immunotherapy. a dendritic cell vaccine increases the breadth and diversity of melanoma neoantigen-specific T cells. Science (2015) 348(6236):803–8.
8. Borghaei H, Paz-Ares L, Horn L, Spigel DR, Steins M, Ready NE, et al. Nivolumab versus docetaxel in advanced nonsquamous non-Small-Cell lung cancer. N Engl J Med (2015) 373(17):1627–39. doi: 10.1056/NEJMoa1507643
9. Herbst RS, Baas P, Kim DW, Felip E, Pérez-Gracia JL, Han JY, et al. Pembrolizumab versus docetaxel for previously treated, PD-L1-positive, advanced non-small-cell lung cancer (KEYNOTE-010): A randomised controlled trial. Lancet (2016) 387(10027):1540–50. doi: 10.1016/S0140-6736(15)01281-7
10. Reck M, Rodríguez-Abreu D, Robinson AG, Hui R, Csőszi T, Fülöp A, et al. Pembrolizumab versus chemotherapy for PD-L1-Positive non-small-cell lung cancer. N Engl J Med (2016) 375(19):1823–33. doi: 10.1056/NEJMoa1606774
11. Rittmeyer A, Barlesi F, Waterkamp D, Park K, Ciardiello F, von Pawel J, et al. Atezolizumab versus docetaxel in patients with previously treated non-small-cell lung cancer (OAK): A phase 3, open-label, multicentre randomised controlled trial. Lancet (2017) 389(10066):255–65. doi: 10.1016/S0140-6736(16)32517-X
12. Antonia SJ, Villegas A, Daniel D, Vicente D, Murakami S, Hui R, et al. Durvalumab after chemoradiotherapy in stage III non-Small-Cell lung cancer. N Engl J Med (2017) 377(20):1919–29. doi: 10.1056/NEJMoa1709937
13. Paz-Ares L, Luft A, Vicente D, Tafreshi A, Gümüş M, Mazières J, et al. Pembrolizumab plus chemotherapy for squamous non-Small-Cell lung cancer. N Engl J Med (2018) 379(21):2040–51. doi: 10.1056/NEJMoa1810865
14. Antonia SJ, Villegas A, Daniel D, Vicente D, Murakami S, Hui R, et al. Overall survival with durvalumab after chemoradiotherapy in stage III NSCLC. N Engl J Med (2018) 379(24):2342–50. doi: 10.1056/NEJMoa1809697
15. Socinski MA, Jotte RM, Cappuzzo F, Orlandi F, Stroyakovskiy D, Nogami N, et al. Atezolizumab for first-line treatment of metastatic nonsquamous NSCLC. N Engl J Med (2018) 378(24):2288–301. doi: 10.1056/NEJMoa1716948
16. Gandhi L, Rodríguez-Abreu D, Gadgeel S, Esteban E, Felip E, De Angelis F, et al. Pembrolizumab plus chemotherapy in metastatic non-Small-Cell lung cancer. N Engl J Med (2018) 378(22):2078–92. doi: 10.1056/NEJMoa1801005
17. Yang H, Chen H, Luo S, Li L, Zhou S, Shen R, et al. The correlation between programmed death-ligand 1 expression and driver gene mutations in NSCLC. Oncotarget (2017) 8(14):23517–28. doi: 10.18632/oncotarget.15627
18. Mhanna L, Milia J, Lusque A, Couraud S, Mascaux C, Veillon R, et al. Efficacy of immune-checkpoint inhibitors (ICI) in non-small cell lung cancer (NSCLC) patients harboring activating molecular alterations. J Clin Oncol (2018) 36(5_suppl):172–2. doi: 10.1200/JCO.2018.36.5_suppl.172
19. Mazieres J, Drilon A, Lusque A, Mhanna L, Cortot AB, Mezquita L, et al. Immune checkpoint inhibitors for patients with advanced lung cancer and oncogenic driver alterations: results from the IMMUNOTARGET registry. Ann Oncol (2019) 30(8):1321–8. doi: 10.1093/annonc/mdz167
20. Offin M, Rizvi H, Tenet M, Ni A, Sanchez-Vega F, Li BT, et al. Tumor mutation burden and efficacy of EGFR-tyrosine kinase inhibitors in patients with EGFR-mutant lung cancers. Clin Cancer Res (2019) 25(3):1063–9. doi: 10.1158/1078-0432.CCR-18-1102
21. Akbay EA, Koyama S, Carretero J, Altabef A, Tchaicha JH, Christensen CL, et al. Activation of the PD-1 pathway contributes to immune escape in EGFR-driven lung tumors. Cancer Discovery (2013) 3(12):1355–63. doi: 10.1158/2159-8290.CD-13-0310
22. Chen N, Fang W, Zhan J, Hong S, Tang Y, Kang S, et al. Upregulation of PD-L1 by EGFR activation mediates the immune escape in EGFR-driven NSCLC: Implication for optional immune targeted therapy for NSCLC patients with EGFR mutation. J Thorac Oncol (2015) 10(6):910–23. doi: 10.1097/JTO.0000000000000500
23. Wang Y, Wang H, Yao H, Li C, Fang JY, Xu J. Regulation of PD-L1: Emerging routes for targeting tumor immune evasion. Front Pharmacol (2018) 9:536. doi: 10.3389/fphar.2018.00536
24. Chan TA, Wolchok JD, Snyder A. Genetic basis for clinical response to CTLA-4 blockade in melanoma. N Engl J Med (2015) 373(20):1984. doi: 10.1056/NEJMc1508163
25. Tran E, Turcotte S, Gros A, Robbins PF, Lu YC, Dudley ME, et al. Cancer immunotherapy based on mutation-specific CD4+ T cells in a patient with epithelial cancer. Science (2014) 344(6184):641–5. doi: 10.1126/science.1251102
26. Szolek A, Schubert B, Mohr C, Sturm M, Feldhahn M, Kohlbacher O. OptiType: precision HLA typing from next-generation sequencing data. Bioinformatics (2014) 30(23):3310–6. doi: 10.1093/bioinformatics/btu548
27. Jurtz V, Paul S, Andreatta M, Marcatili P, Peters B, Nielsen M. NetMHCpan-4.0: Improved peptide-MHC class I interaction predictions integrating eluted ligand and peptide binding affinity data. J Immunol (2017) 199(9):3360–8. doi: 10.4049/jimmunol.1700893
28. McGranahan N, Rosenthal R, Hiley CT, Rowan AJ, Watkins TBK, Wilson GA, et al. Allele-specific HLA loss and immune escape in lung cancer evolution. Cell (2017) 171(6):1259–71.e11. doi: 10.1016/j.cell.2017.10.001
29. Newman AM, Liu CL, Green MR, Gentles AJ, Feng W, Xu Y, et al. Robust enumeration of cell subsets from tissue expression profiles. Nat Methods (2015) 12(5):453–7. doi: 10.1038/nmeth.3337
30. González-Galarza FF, Takeshita LY, Santos EJ, Kempson F, Maia MH, da Silva AL, et al. Allele frequency net 2015 update: New features for HLA epitopes, KIR and disease and HLA adverse drug reaction associations. Nucleic Acids Res (2015) 43(Database issue):D784–8. doi: 10.1093/nar/gku1166
31. Zhang XC, Wang J, Shao GG, Wang Q, Qu X, Wang B, et al. Comprehensive genomic and immunological characterization of Chinese non-small cell lung cancer patients. Nat Commun (2019) 10(1):1772. doi: 10.1038/s41467-019-09762-1
32. Matsushita H, Hasegawa K, Oda K, Yamamoto S, Nishijima A, Imai Y, et al. The frequency of neoantigens per somatic mutation rather than overall mutational load or number of predicted neoantigens per se is a prognostic factor in ovarian clear cell carcinoma. Oncoimmunology (2017) 6(8):e1338996. doi: 10.1080/2162402X.2017.1338996
33. Lawrence MS, Stojanov P, Polak P, Kryukov GV, Cibulskis K, Sivachenko A, et al. Mutational heterogeneity in cancer and the search for new cancer-associated genes. Nature (2013) 499(7457):214–8. doi: 10.1038/nature12213
34. Sharma GG, Mota I, Mologni L, Patrucco E, Gambacorti-Passerini C, Chiarle R. Tumor resistance against ALK targeted therapy-where it comes from and where it goes. Cancers (2018) 10(3):62. doi: 10.3390/cancers10030062
35. Lee CK, Man J, Lord S, Cooper W, Links M, Gebski V, et al. Clinical and molecular characteristics associated with survival among patients treated with checkpoint inhibitors for advanced non-small cell lung carcinoma: A systematic review and meta-analysis. JAMA Oncol (2018) 4(2):210–6. doi: 10.1001/jamaoncol.2017.4427
36. Leisegang M, Engels B, Schreiber K, Yew PY, Kiyotani K, Idel C, et al. Eradication of Large solid tumors by gene therapy with a T-cell receptor targeting a single cancer-specific point mutation. Clin Cancer Res (2016) 22(11):2734–43. doi: 10.1158/1078-0432.CCR-15-2361
37. Lu YC, Robbins PF. Cancer immunotherapy targeting neoantigens. Semin Immunol (2015) 28(1):22–7. doi: 10.1016/j.smim.2015.11.002
38. Chowell D, Morris LGT, Grigg CM, Weber JK, Samstein RM, Makarov V, et al. Patient HLA class I genotype influences cancer response to checkpoint blockade immunotherapy. Science (2017) 359(6375):582–7. doi: 10.1126/science.aao4572
39. Gao J, Shi LZ, Zhao H, Chen J, Xiong L, He Q, et al. Loss of IFN-γ pathway genes in tumor cells as a mechanism of resistance to anti-CTLA-4 therapy. Cell (2016) 167(2):397–404.e9. doi: 10.1016/j.cell.2016.08.069
40. Perea F, Bernal M, Sánchez-Palencia A, Carretero J, Torres C, Bayarri C, et al. The absence of HLA class I expression in non-small cell lung cancer correlates with the tumor tissue structure and the pattern of T cell infiltration. Int J Cancer (2017) 140(4):888–99. doi: 10.1002/ijc.30489
41. Hulpke S, Tampé R. The MHC I loading complex: A multitasking machinery in adaptive immunity. Trends Biochem Sci (2013) 38(8):412–20. doi: 10.1016/j.tibs.2013.06.003
42. Hellmann MD, Ciuleanu TE, Pluzanski A, Lee JS, Otterson GA, Audigier-Valette C, et al. Nivolumab plus ipilimumab in lung cancer with a high tumor mutational burden. N Engl J Med (2018) 378(22):2093–104. doi: 10.1056/NEJMoa1801946
43. Carbone DP, Reck M, Paz-Ares L, Creelan B, Horn L, Steins M, et al. First-line nivolumab in stage IV or recurrent non-Small-Cell lung cancer. N Engl J Med (2017) 376(25):2415–26. doi: 10.1056/NEJMoa1613493
44. Mantovani A, Marchesi F, Malesci A, Laghi L, Allavena P. Tumour-associated macrophages as treatment targets in oncology. Nat Rev Clin Oncol (2017) 14(7):399–416. doi: 10.1038/nrclinonc.2016.217
45. Kalluri R. The biology and function of fibroblasts in cancer. Nat Rev Cancer (2016) 16(9):582–98. doi: 10.1038/nrc.2016.73
46. Abele R, Tampé R. The ABCs of immunology: structure and function of TAP, the transporter associated with antigen processing. Physiol (Bethesda) (2004) 19:216–24. doi: 10.1152/physiol.00002.2004
47. El Hage F, Durgeau A, Mami-Chouaib F. TAP expression level in tumor cells defines the nature and processing of MHC class I peptides for recognition by tumor-specific cytotoxic T lymphocytes. Ann N Y Acad Sci (2013) 1283:75–80. doi: 10.1111/j.1749-6632.2012.06777.x
48. Robins HS, Campregher PV, Srivastava SK, Wacher A, Turtle CJ, Kahsai O, et al. Comprehensive assessment of T-cell receptor beta-chain diversity in alphabeta T cells. Blood (2009) 114(19):4099–107. doi: 10.1182/blood-2009-04-217604
49. Han J, Duan J, Bai H, Wang Y, Wan R, Wang X, et al. TCR repertoire diversity of peripheral PD-1(+)CD8(+) T cells predicts clinical outcomes after immunotherapy in patients with non-small cell lung cancer. Cancer Immunol Res (2020) 8(1):146–54. doi: 10.1158/2326-6066.CIR-19-0398
50. Reuben A, Zhang J, Chiou SH, Gittelman RM, Li J, Lee WC, et al. Comprehensive T cell repertoire characterization of non-small cell lung cancer. Nat Commun (2020) 11(1):603. doi: 10.1038/s41467-019-14273-0
51. Hartmaier RJ, Charo J, Fabrizio D, Goldberg ME, Albacker LA, Pao W, et al. Genomic analysis of 63,220 tumors reveals insights into tumor uniqueness and targeted cancer immunotherapy strategies. Genome Med (2017) 9(1):16. doi: 10.1186/s13073-017-0408-2
Keywords: EGFR L858R mutation, neoantigen vaccine, HLA A*33:03, immunological features, Chinese NSCLC
Citation: Lin J, Liu J, Hao S-g, Lan B, Zheng X-b, Xiong J-n, Zhang Y-q, Gao X, Chen C-b, Chen L, Huang Y-f, Luo H, Yi Y-t, Yi X, Lu J-p, Zheng X-w, Chen G, Wang X-f and Chen Y (2022) An EGFR L858R mutation identified in 1862 Chinese NSCLC patients can be a promising neoantigen vaccine therapeutic strategy. Front. Immunol. 13:1022598. doi: 10.3389/fimmu.2022.1022598
Received: 18 August 2022; Accepted: 28 September 2022;
Published: 23 November 2022.
Edited by:
Hubing Shi, Sichuan University, ChinaReviewed by:
Song Xu, Tianjin Medical University General Hospital, ChinaWeifeng Hong, Fudan University, China
Feng Wang, Jinling Hospital, China
Copyright © 2022 Lin, Liu, Hao, Lan, Zheng, Xiong, Zhang, Gao, Chen, Chen, Huang, Luo, Yi, Yi, Lu, Zheng, Chen, Wang and Chen. This is an open-access article distributed under the terms of the Creative Commons Attribution License (CC BY). The use, distribution or reproduction in other forums is permitted, provided the original author(s) and the copyright owner(s) are credited and that the original publication in this journal is cited, in accordance with accepted academic practice. No use, distribution or reproduction is permitted which does not comply with these terms.
*Correspondence: Yu Chen, Y2hleXUxOTgwQGZqbXUuZWR1LmNu; Xue-feng Wang, d2FuZ3h1ZWZlbmcyMDE5QGZveG1haWwuY29t
†These authors have contributed equally to this work