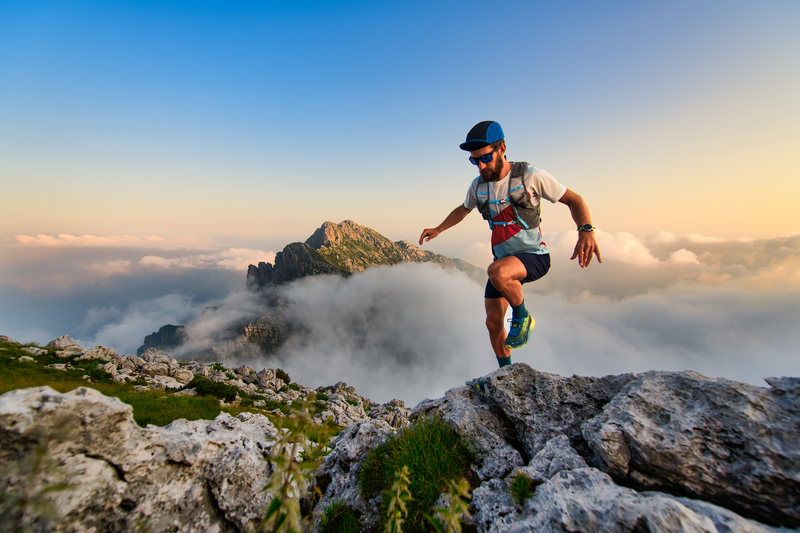
94% of researchers rate our articles as excellent or good
Learn more about the work of our research integrity team to safeguard the quality of each article we publish.
Find out more
ORIGINAL RESEARCH article
Front. Immunol. , 02 December 2022
Sec. Autoimmune and Autoinflammatory Disorders
Volume 13 - 2022 | https://doi.org/10.3389/fimmu.2022.1017444
This article is part of the Research Topic Genetic and Environmental Factors in Rheumatic Diseases View all 14 articles
Background: Epidemiological evidence suggests an association between rheumatoid arthritis (RA) and myocardial infarction (MI). However, causality remains uncertain. Therefore, this study aimed to explore the causal association between RA and MI.
Methods: Using publicly available genome-wide association study summary datasets, bidirectional two-sample Mendelian randomization (TSMR) was performed using inverse-variance weighted (IVW), weighted median, MR-Egger regression, simple mode, and weighted mode methods.
Results: The MR results for the causal effect of RA on MI (IVW, odds ratio [OR] = 1.041, 95% confidence interval [CI]: 1.007–1.076, P = 0.017; weighted median, OR = 1.027, 95% CI: 1.006–1.049, P = 0.012) supported a causal association between genetic susceptibility to RA and an increased risk of MI. MR results for the causal effect of MI on RA (IVW, OR = 1.012, 95% CI: 0.807–1.268, P = 0.921; weighted median, OR = 1.069, 95% CI: 0.855–1.338, P = 0.556) indicated that there was no causal association between genetic susceptibility to MI and an increased risk of RA.
Conclusion: Bidirectional TSMR analysis supports a causal association between genetic susceptibility to RA and an increased risk of MI but does not support a causal association between genetic susceptibility to MI and an increased risk of RA.
Rheumatoid arthritis (RA) is a chronic systemic autoimmune disease that affects several tissues and organs and causes chronic synovial inflammation, eventually leading to joint destruction, chronic disability, and reduced life expectancy (1). RA results from the interaction of genetic susceptibility, environmental factors, and immune factors, among which genetic factors determine 50–60% of the risk of RA (2, 3). Myocardial infarction (MI) is a cardiovascular disease in which the formation of plaques on the inner walls of the arteries leads to a decrease in blood flow to the heart, and long-term ischemia and hypoxia result in the death of myocardial cells (4, 5). Some observational studies have shown a close relationship between RA and MI. A cross-sectional study by Dougados et al. (6) of 4,586 RA patients enrolled in 17 countries showed a high prevalence of comorbidities among RA patients, with 6% (95% confidence interval [CI] 5.3%–6.8%) having a history of MI or stroke. In a 10-year cohort study, Lindhardsen et al. (7) found that patients with RA had an increased risk of MI of approximately 70% compared to the general population after adjusting for factors such as sex, age, and socioeconomic status. Among post-MI patients, those with RA have a poor prognosis and an increased risk of death, which is positively correlated with RA duration and steroid dosage (8). A systematic review and meta-analysis showed (9) that the risk of MI increased significantly in patients with RA (relative risk: 1.69, 95% CI 1.50–1.90). However, a cohort study by Rostami et al. (10) found that the weighted genetic risk score of RA had contributed little to the morbidity risk of MI.
Causal inferences from observational studies are susceptible to bias owing to reverse causality and potential confounders (11), which weakens our understanding of the causal association between RA and MI. Randomized controlled trials (RCTs) are the gold standard for causal inferences in epidemiological studies. Some RCTs are difficult to perform owing to medical ethics, subject selection, and extrapolation of results. Mendelian randomization (MR) is a technique that uses genetic variation as an instrumental variable (IV) to assess whether an observational association between exposure factors and outcomes is consistent with a causal effect (12). Genetic variation is not affected by the external environment, social behavior, or other factors, and it is a long-term and stable exposure factor. MR can avoid the effect of confounding factors and reverse causal association on the correlation effects in observational studies, and minimize bias. Published data were collected in this study and bidirectional TSMR analysis was used to determine whether there was a bidirectional causal association between RA and MI.
Relevant genome-wide association study (GWAS) datasets were obtained from the IEU OpenGWAS project (https://gwas.mrcieu.ac.uk). The GWAS dataset for RA was derived from GWAS analysis and included 13,838 cases and 33,742 controls of European ancestry (13). The GWAS dataset for MI was derived from another GWAS analysis and included 14,825 cases and 44,000 controls of European ancestry (14) (Supplementary Table 1).
Single nucleotide polymorphisms (SNPs) were used as IVs. P-value (P<5.0×10-8) was set. To avoid linkage disequilibrium (LD) bias, LD with significant SNPs associated with exposure factors must meet the following conditions: r2<0.001, and genetic distance of 10000 kb. Significant SNPs associated with exposure factors were extracted in the GWAS dataset of outcome variables, and the resulting IVs were recorded with information on the effect allele, allele effect sizes (beta), standard error, and p-value. The F-statistic was used to test the strength of each IV and was calculated using the following formula: F = R2(N−2)/(1−R2), where R2 is the proportion of the exposure factor variation explained by each IV, and N is the sample size of the expourse dataset (15). When F>10, there is no weak IVs bias (16).
To better estimate the causal effect, three key assumptions should be met when SNPs are used as IVs in the TSMR analysis (17) (1): IVs must be closely related to exposure factors; (2) IVs are independent of confounding factors; and (3) IVs can only influence the outcome through exposure and not through other pathways.
Summary statistics for the exposure and outcome datasets were harmonized such that the effect of SNPs on exposure and the effect of SNPs on outcome corresponded to the same alleles. TSMR analyses using inverse-variance weighted (IVW), weighted median, MR-Egger regression, simple mode, and weighted mode methods were performed to infer causal associations. We used the IVW as the primary method for MR. When each genetic variation met the IV hypothesis, the IVW method combined the Wald ratio estimates of the causal effects of different SNPs and provided a consistent estimate of the causal effect of exposure on the outcome (18). The results of the IVW method were most reliable when there was no horizontal pleiotropy of the IVs (19). When at least half of the SNPs are effective IVs, the weighted median can provide a consistent estimate of the causal effect (20). MR-Egger regression is used to confirm whether horizontal pleiotropy of IVs exists, and its intercept represents the effect estimate of horizontal pleiotropy (21). When the IVs have horizontal pleiotropy, the MR-Egger regression can still obtain an unbiased estimation of causal association. The weighted median method improves the accuracy of the results compared to the MR-Egger method (22). Simple mode and weighted mode were performed as complementary analyses (23). The Mendelian randomization pleiotropy residual sum and outlier (MR-PRESSO) test was used to detect and correct horizontal pleiotropy by removing the outliers (24). MR power analysis was performed using an online tool (http://cnsgenomics.com/shiny/mRnd/) (25). Statistical analysis was performed using R (version 4.1.0) and R packages (TwoSampleMR and MR-PRESSO). The test level α was 0.05 (P < 0.05), and the difference was statistically significant.
RA was the exposure factor, and MI was the outcome variable. In total, 15 SNPs were screened and identified as IVs, with F values greater than 10. The variance explained by these IVs was 44% for RA (Supplementary Table 2). The intercept of the MR-Egger regression can be used as an indicator to test whether horizontal pleiotropy of the IVs influences the results of TSMR analysis. The intercept was close to 0 (Egger intercept = 0.006, P = 0.384) (Table 1), indicating that there was no horizontal pleiotropy of the IVs, and it was unlikely to influence the results of the TSMR analysis (Figure 1B).
Figure 1 Forest plot (A), scatter plot (B), sensitivity analysis (C), and funnel plot (D) of the effect of RA on MI.
The MR results supported a causal association between genetic susceptibility to RA and an increased risk of MI. The MR analysis had 81% statistical power. In the absence of horizontal pleiotropy of IVs, IVW was used as the primary method to estimate the causal association between genetic susceptibility to RA and an increased risk of MI (IVW result: OR = 1.041, 95% CI: 1.007–1.076, P = 0.017). Results of the other methods included: MR-Egger, OR = 1.023, 95% CI: 0.973–1.076, P = 0.389; weighted median, OR = 1.027, 95% CI: 1.006–1.049, P = 0.012; simple mode, OR = 1.016, 95% CI: 0.968–1.065, P = 0.534; and weighted mode, OR = 1.024, 95% CI: 1.003–1.046, P = 0.042 (Table 2, Figures 1A, B).
IVW and MR-Egger regression analyses were used to detect heterogeneity between IVs. Heterogeneity was quantified using Cochran’s Q test. P < 0.05 indicated significant heterogeneity. If there was heterogeneity between the IVs, the random-effects IVW model was used to estimate causal effects (26). MR-Egger regression (Cochran’s Q = 51.36, P = 1.74E-06) and IVW (Cochran’s Q = 54.57, P = 1.03E-06) (Table 1, Figure 1D) indicated that there was heterogeneity between the IVs, and the random-effects IVW model was used to estimate the causal effect (P = 0.017). The MR-PRESSO test was used to remove the outlier SNPs (rs2228145, rs6679677, and rs71624119) and to estimate the causal effect of TSMR after correction for outliers (P =0.019) (Supplementary Table 3).
The sensitivity analysis used the leave-one-out method to remove SNPs one by one, and the causal effects of the remaining SNPs were compared with the TSMR analysis results of all SNPs to determine whether the causal association was due to a single IV, indicating that the TSMR analysis results were robust (Figure 1C).
In reverse TSMR, MI was the exposure factor, and RA was the outcome variable. In total, 9 SNPs were screened and identified as IVs, with F values greater than 10. The variance explained by these IVs was 2.6% for MI (Supplementary Table 4). The horizontal pleiotropy test (Egger intercept = −0.01, P = 0.621) (Table 1) indicated that there was no horizontal pleiotropy for the IVs. MR results did not support a causal association between genetic susceptibility to MI and an increased risk of RA (IVW, OR = 1.012, 95% CI: 0.807 – 1.268, P = 0.921). Results of other methods included: MR-Egger, OR = 1.112, 95% CI: 0.723–1.710, P = 0.643; weighted median, OR = 1.069, 95% CI: 0.855–1.338, P = 0.556; simple mode, OR = 1.161, 95% CI: 0.763–1.765, P = 0.505; and weighted mode, OR = 1.095, 95% CI: 0.871–1.377, P = 0.458 (Table 3, Figures 2A, B). Among the heterogeneity test results, MR-Egger regression showed relatively small heterogeneity (Cochran’s Q =14.62, P = 0.041), whereas IVW (Cochran’s Q = 15.18, P = 0.056) did not find heterogeneity between IVs (Table 1, Figure 2D). MR-PRESSO indicated that there was no horizontal pleiotropy for the global test (RSSobs = 18.31, P = 0.115) (Supplementary Table 3), and no outliers were observed. The leave-one-out method was used for sensitivity analysis, and the results of the TSMR analysis were reliable (Figure 2C).
Figure 2 Forest plot (A), scatter plot (B), sensitivity analysis (C), and funnel plot (D) of the effect of MI on RA.
This study used the bidirectional TSMR method to analyze published GWAS datasets and determine whether a bidirectional causal association exists between RA and MI in the European population. Our results supported a causal association between genetic susceptibility to RA and an increased risk of MI (IVW, OR = 1.041, 95% CI: 1.007 – 1.076, P = 0.017). However, our results did not support a causal association between genetic susceptibility to MI and an increased risk of RA (IVW, OR =1.012, 95% CI: 0.807 – 1.268, P = 0.921). In the sensitivity analysis, the MR results were robust and reliable.
Several possible reasons have been proposed to explain the association between RA and MI in observational studies. First, MI in RA may be associated with RA-related inflammatory reactions (27). Inflammation can promote the development of atherosclerotic plaques, increase vulnerability, and promote thrombosis (28). Acute phase reactants in patients with RA cause synovitis, and elevated levels of inflammatory cytokines such as tumor necrosis factor α (TNF-α) and interleukin 6 (IL-6) induce atherosclerotic changes and endothelial function damage, leading to an increased risk of MI (29). The TNF-α-induced signaling pathway plays an important role in cellular responses to inflammation and injury, leading to vascular dysfunction and adverse reactions to cardiac remodeling after MI (30). A case-control study showed (31) that the plasma concentrations of IL-6 and IL-6 binary complexes could predict the risk of MI. Early active RA treatment can better control the inflammation. Glucocorticoids (GC) are commonly used as immunosuppressive agents for the treatment of RA. Their anti-inflammatory and immunosuppressive effects can reduce the damage to blood vessels caused by inflammation. However, the long-term use of GC leads to a series of adverse reactions, including cardiovascular diseases (32, 33). A case-control study by Wilson et al. (34) showed that an increase in the cumulative and average daily GC doses was associated with an increased risk of MI. A cohort study by Pujase-Rodriguez et al. (33) showed that even at a lower dose of GC (< 5 mg), the risk of MI increased. Second, there is an increased risk of cardiovascular risk factors (hypertension, dyslipidemia, and diabetes) in RA patients, which, together with inflammation, can promote the formation of atherosclerosis in RA patients and may further contribute to the development of MI (35). Third, genetic factors may also play a role in the association between RA and MI. Vascular endothelial growth factor, a promoter of normal and abnormal angiogenesis, plays an important role in RA pathogenesis (36). Studies by Chen et al. (37) have shown that SNPs in the vascular endothelial growth factor A promoter regions (-2578 and -460) are associated with an increased risk of MI in patients with RA. Palomino-Morales et al. (38) found that the IL6 -174 gene polymorphism is associated with subclinical atherosclerosis, and RA patients with the IL6 -174GG genotype have severe endothelial dysfunction. Methylene tetrahydrofolate reductase 1298 A>C gene polymorphism increases the risk of atherosclerosis in patients with RA (39). Our MR results did not reveal a causal association between genetic susceptibility to MI and an increased risk of RA, and more studies should be conducted in the future.
MR uses genetic variation to estimate the health consequences of the phenotypes affected by these genetic variations (40). This is a relatively novel epidemiological approach that uses genetic variation to infer the causal association between exposure factors and outcome variables. MR provides a way to investigate associations without the typical biases inherent in observational epidemiological studies, such as reverse causal association and potential confounders. Our results differ from the MR results of Fokina et al. (41), probably because of the different GWAS datasets selected, and newer, larger GWAS studies will be necessary in the future.
This study had some limitations. First, the MR results were based on the European population, and extrapolation of the results is limited. Whether a causal relationship exists in other populations needs to be confirmed by further research. Second, SNPs used for analysis may be correlated with other traits due to genetic polymorphisms and generate confounding bias, which may affect causal inference. Third, the strength of the IV depends on the sample size of the GWAS, and a larger scale GWAS is required to determine more genetic variation for MR.
In summary, bidirectional TSMR analysis supports a causal association between genetic susceptibility to RA and an increased risk of MI, but does not support a causal association between genetic susceptibility to MI and an increased risk of RA. However, due to the limitations of the study, further research is necessary.
The original contributions presented in the study are included in the article/Supplementary Material. Further inquiries can be directed to the corresponding author.
H-YG: conceptualization, investigation, data curation, writing - original draft. WW: conceptualization, investigation, writing - original draft. HP: investigation, writing - review and editing. HY: conceptualization, writing - review and editing. All authors contributed to the article and approved the submitted version.
This work was supported by grants from Anhui Provincial Natural Science Foundation (No. 1808085QH251) and Horizontal scientific research project of Wannan Medical College (No. H2020001).
We thank all participants for the study we conducted.
The authors declare that the research was conducted in the absence of any commercial or financial relationships that could be construed as a potential conflict of interest.
All claims expressed in this article are solely those of the authors and do not necessarily represent those of their affiliated organizations, or those of the publisher, the editors and the reviewers. Any product that may be evaluated in this article, or claim that may be made by its manufacturer, is not guaranteed or endorsed by the publisher.
The Supplementary Material for this article can be found online at: https://www.frontiersin.org/articles/10.3389/fimmu.2022.1017444/full#supplementary-material
1. Song X, Lin Q. Genomics, transcriptomics and proteomics to elucidate the pathogenesis of rheumatoid arthritis. Rheumatol Int (2017) 37(8):1257–65. doi: 10.1007/s00296-017-3732-3
2. van der Woude D, van der Helm-van Mil AHM. Update on the epidemiology, risk factors, and disease outcomes of rheumatoid arthritis. Best Pract Res Clin Rheumatol (2018) 32(2):174–87. doi: 10.1016/j.berh.2018.10.005
3. Frisell T, Saevarsdottir S, Askling J. Family history of rheumatoid arthritis: an old concept with new developments. Nat Rev Rheumatol (2016) 12(6):335–43. doi: 10.1038/nrrheum.2016.52
4. Lu L, Liu M, Sun R, Zheng Y, Zhang P. Myocardial infarction: Symptoms and treatments. Cell Biochem Biophys (2015) 72(3):865–7. doi: 10.1007/s12013-015-0553-4
5. Lindahl B, Baron T, Albertucci M, Prati F. Myocardial infarction with non-obstructive coronary artery disease. EuroIntervention (2021) 17(11):e875–87. doi: 10.4244/EIJ-D-21-00426
6. Dougados M, Soubrier M, Antunez A, Balint P, Balsa A, Buch MH, et al. Prevalence of comorbidities in rheumatoid arthritis and evaluation of their monitoring: results of an international, cross-sectional study (COMORA). Ann Rheum Dis (2014) 73(1):62–8. doi: 10.1136/annrheumdis-2013-204223
7. Lindhardsen J, Ahlehoff O, Gislason GH, Madsen OR, Olesen JB, Torp-Pedersen C, et al. The risk of myocardial infarction in rheumatoid arthritis and diabetes mellitus: a Danish nationwide cohort study. Ann Rheum Dis (2011) 70(6):929–34. doi: 10.1136/ard.2010.143396
8. Palomäki A, Kerola AM, Malmberg M, Rautava P, Kytö V. Patients with rheumatoid arthritis have impaired long-term outcomes after myocardial infarction: a nationwide case-control registry study. Rheumatol (Oxford). (2021) 60(11):5205–15. doi: 10.1093/rheumatology/keab204
9. Schieir O, Tosevski C, Glazier RH, Hogg-Johnson S, Badley EM. Incident myocardial infarction associated with major types of arthritis in the general population: a systematic review and meta-analysis. Ann Rheum Dis (2017) 76(8):1396–404. doi: 10.1136/annrheumdis-2016-210275
10. Rostami S, Hoff M, Dalen H, Hveem K, Videm V. Genetic risk score associations for myocardial infarction are comparable in persons with and without rheumatoid arthritis: the population-based HUNT study. Sci Rep (2020) 10(1):20416. doi: 10.1038/s41598-020-77432-0
11. Evans DM, Davey Smith G. Mendelian randomization: New applications in the coming age of hypothesis-free causality. Annu Rev Genomics Hum Genet (2015) 16:327–50. doi: 10.1146/annurev-genom-090314-050016
12. Bae SC, Lee YH. Coffee consumption and the risk of rheumatoid arthritis and systemic lupus erythematosus: a mendelian randomization study. Clin Rheumatol (2018) 37(10):2875–9. doi: 10.1007/s10067-018-4278-9
13. Eyre S, Bowes J, Diogo D, Lee A, Barton A, Martin P, et al. High-density genetic mapping identifies new susceptibility loci for rheumatoid arthritis. Nat Genet (2012) 44(12):1336–40. doi: 10.1038/ng.2462
14. Hartiala JA, Han Y, Jia Q, Hilser JR, Huang P, Gukasyan J, et al. Genome-wide analysis identifies novel susceptibility loci for myocardial infarction. Eur Heart J (2021) 42(9):919–33. doi: 10.1093/eurheartj/ehaa1040
15. He B, Lyu Q, Yin L, Zhang M, Quan Z, Ou Y. Depression and osteoporosis: A mendelian randomization study. Calcif Tissue Int (2021) 109(6):675–84. doi: 10.1007/s00223-021-00886-5
16. Hemani G, Zheng J, Elsworth B, Wade KH, Haberland V, Baird D, et al. The MR-base platform supports systematic causal inference across the human phenome. Elife (2018) 7:e34408. doi: 10.7554/eLife.34408
17. Burgess S, Butterworth A, Thompson SG. Mendelian randomization analysis with multiple genetic variants using summarized data. Genet Epidemiol. (2013) 37(7):658–65. doi: 10.1002/gepi.21758
18. Bae SC, Lee YH. Vitamin d level and risk of systemic lupus erythematosus and rheumatoid arthritis: a mendelian randomization. Clin Rheumatol (2018) 37(9):2415–21. doi: 10.1007/s10067-018-4152-9
19. Huang S, Tian F, Yang X, Fang S, Fan Y, Bao J. Physical activity and systemic lupus erythematosus among European populations: A two-sample mendelian randomization study. Front Genet (2022) 12:784922. doi: 10.3389/fgene.2021.784922
20. Bowden J, Davey Smith G, Haycock PC, Burgess S. Consistent estimation in mendelian randomization with some invalid instruments using a weighted median estimator. Genet Epidemiol. (2016) 40(4):304–14. doi: 10.1002/gepi.21965
21. Burgess S, Thompson SG. Interpreting findings from mendelian randomization using the MR-egger method. Eur J Epidemiol. (2017) 32(5):377–89. doi: 10.1007/s10654-017-0255-x
22. Xiang K, Wang P, Xu Z, Hu YQ, He YS, Chen Y, et al. Causal effects of gut microbiome on systemic lupus erythematosus: A two-sample mendelian randomization study. Front Immunol (2021) 12:667097. doi: 10.3389/fimmu.2021.667097
23. Sun W, Zhang L, Liu W, Tian M, Wang X, Liang J, et al. Stroke and myocardial infarction: A bidirectional mendelian randomization study. Int J Gen Med (2021) 14:9537–45. doi: 10.2147/IJGM.S337681
24. Sang N, Gao RC, Zhang MY, Wu ZZ, Wu ZG, Wu GC. Causal relationship between sleep traits and risk of systemic lupus erythematosus: A two-sample mendelian randomization study. Front Immunol (2022) 13:918749. doi: 10.3389/fimmu.2022.918749
25. Chen W, Cai X, Yan H, Pan Y. Causal effect of obstructive sleep apnea on atrial fibrillation: A mendelian randomization study. J Am Heart Assoc (2021) 10(23):e022560. doi: 10.1161/JAHA.121.022560
26. Zhang K, Jia Y, Wang R, Guo D, Yang P, Sun L, et al. Rheumatoid arthritis and the risk of major cardiometabolic diseases: a mendelian randomization study. Scand J Rheumatol (2022), 1–7. doi: 10.1080/03009742.2022.2070988
27. Mal K, Kumar R, Mansoor F, Kaur N, Kumar A, Memon S, et al. Risk of major adverse cardiovascular events in patients with rheumatoid arthritis. Cureus (2020) 12(12):e12246. doi: 10.7759/cureus.12246
28. Meune C, Touzé E, Trinquart L, Allanore Y. High risk of clinical cardiovascular events in rheumatoid arthritis: Levels of associations of myocardial infarction and stroke through a systematic review and meta-analysis. Arch Cardiovasc Dis (2010) 103(4):253–61. doi: 10.1016/j.acvd.2010.03.007
29. Liu SF, Lee CK, Huang KC, Lin LY, Hsieh MY, Lin TT. Long-term effect of non-selective beta-blockers in patients with rheumatoid arthritis after myocardial infarction-a nationwide cohort study. Front Pharmacol (2021) 12:726044. doi: 10.3389/fphar.2021.726044
30. Hasan R, Siregar GA, Lindarto D. Syzygium polyanthum reduced TNF-α and ADAM17 protein expression in myocardial infarction rat model. Med Arch (2020) 74(6):416–20. doi: 10.5455/medarh.2020.74.416-420
31. Zegeye MM, Andersson JSO, Wennberg P, Repsilber D, Sirsjö A, Ljungberg LU. IL-6 as a mediator of the association between traditional risk factors and future myocardial infarction: A nested case-control study. Arterioscler Thromb Vasc Biol (2021) 41(4):1570–9. doi: 10.1161/ATVBAHA.120.315793
32. Arida A, Protogerou AD, Kitas GD, Sfikakis PP. Systemic inflammatory response and atherosclerosis: The paradigm of chronic inflammatory rheumatic diseases. Int J Mol Sci (2018) 19(7):1890. doi: 10.3390/ijms19071890
33. Pujades-Rodriguez M, Morgan AW, Cubbon RM, Wu J. Dose-dependent oral glucocorticoid cardiovascular risks in people with immune-mediated inflammatory diseases: A population-based cohort study. PloS Med (2020) 17(12):e1003432. doi: 10.1371/journal.pmed.1003432
34. Wilson JC, Sarsour K, Gale S, Pethö-Schramm A, Jick SS, Meier CR. Incidence and risk of glucocorticoid-associated adverse effects in patients with rheumatoid arthritis. Arthritis Care Res (Hoboken). (2019) 71(4):498–511. doi: 10.1002/acr.23611
35. Lazzerini PE, Capecchi PL, Laghi-Pasini F. Systemic inflammation and arrhythmic risk: lessons from rheumatoid arthritis. Eur Heart J (2017) 38(22):1717–27. doi: 10.1093/eurheartj/ehw208
36. Paleolog EM. The vasculature in rheumatoid arthritis: cause or consequence? Int J Exp Pathol (2009) 90(3):249–61. doi: 10.1111/j.1365-2613.2009.00640.x
37. Chen Y, Dawes PT, Packham JC, Mattey DL. Interaction between smoking and polymorphism in the promoter region of the VEGFA gene is associated with ischemic heart disease and myocardial infarction in rheumatoid arthritis. J Rheumatol (2011) 38(5):802–9. doi: 10.3899/jrheum.101095
38. Palomino-Morales R, Gonzalez-Juanatey C, Vazquez-Rodriguez TR, Miranda-Filloy JA, Llorca J, Martin J, et al. Interleukin-6 gene -174 promoter polymorphism is associated with endothelial dysfunction but not with disease susceptibility in patients with rheumatoid arthritis. Clin Exp Rheumatol (2009) 27(6):964–70.
39. Palomino-Morales R, Gonzalez-Juanatey C, Vazquez-Rodriguez TR, Rodriguez L, Miranda-Filloy JA, Fernandez-Gutierrez B, et al. A1298C polymorphism in the MTHFR gene predisposes to cardiovascular risk in rheumatoid arthritis. Arthritis Res Ther (2010) 12(2):R71. doi: 10.1186/ar2989
40. Riaz H, Khan MS, Siddiqi TJ, Usman MS, Shah N, Goyal A, et al. Association between obesity and cardiovascular outcomes: A systematic review and meta-analysis of mendelian randomization studies. JAMA Netw Open (2018) 1(7):e183788. doi: 10.1001/jamanetworkopen.2018.3788
Keywords: rheumatoid arthritis, myocardial infarction, bidirectional, two-sample Mendelian randomization study, causal association
Citation: Guo H-Y, Wang W, Peng H and Yuan H (2022) Bidirectional two-sample Mendelian randomization study of causality between rheumatoid arthritis and myocardial infarction. Front. Immunol. 13:1017444. doi: 10.3389/fimmu.2022.1017444
Received: 12 August 2022; Accepted: 21 November 2022;
Published: 02 December 2022.
Edited by:
Jindong Ni, Guangdong Medical University, ChinaReviewed by:
Peter Fasching, Vienna Health Association, AustriaCopyright © 2022 Guo, Wang, Peng and Yuan. This is an open-access article distributed under the terms of the Creative Commons Attribution License (CC BY). The use, distribution or reproduction in other forums is permitted, provided the original author(s) and the copyright owner(s) are credited and that the original publication in this journal is cited, in accordance with accepted academic practice. No use, distribution or reproduction is permitted which does not comply with these terms.
*Correspondence: Hui Yuan, eXVhbmh1aTA1NTNAMTI2LmNvbQ==
†These authors have contributed equally to this work and share first authorship
Disclaimer: All claims expressed in this article are solely those of the authors and do not necessarily represent those of their affiliated organizations, or those of the publisher, the editors and the reviewers. Any product that may be evaluated in this article or claim that may be made by its manufacturer is not guaranteed or endorsed by the publisher.
Research integrity at Frontiers
Learn more about the work of our research integrity team to safeguard the quality of each article we publish.