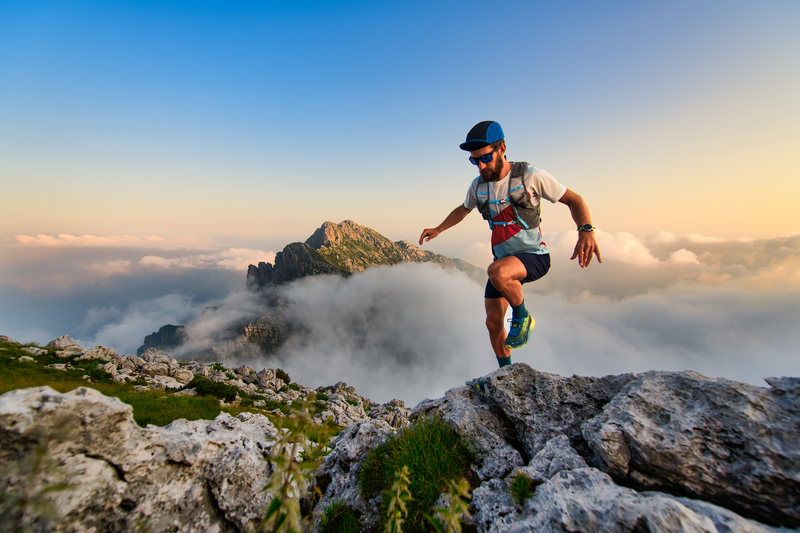
95% of researchers rate our articles as excellent or good
Learn more about the work of our research integrity team to safeguard the quality of each article we publish.
Find out more
ORIGINAL RESEARCH article
Front. Immunol. , 15 December 2022
Sec. Nutritional Immunology
Volume 13 - 2022 | https://doi.org/10.3389/fimmu.2022.1015437
This article is part of the Research Topic Targeted Innovative Immunomodulation of Allergy and Inflammation by Natural Molecules and Probiotics View all 11 articles
Introduction: Eosinophilic Esophagitis (EoE) is a chronic condition characterized by eosinophilic inflammation of the esophagus which leads to esophageal dysfunction with common symptoms including vomiting, feeding difficulty, dysphagia, abdominal pain. Current main treatment options of EoE include dietary elimination and swallowed steroids. Diet elimination approach could lead to identifying the trigger food(s), but it often requires repeated upper endoscopy with general anesthesia and potentially could negatively affect nutrition intake and growth of the child and individuals’ quality of life. Although the swallowed steroid treatment of effective, the EoE will universally recur after discontinuation of the treatment. Digestive Tea formula (DTF) has been used by the Traditional Chinese Medicine (TCM) practice to improve GI symptoms in EoE patients, including abdominal pain, GE reflux, and abnormal bowel movement. Previously, a flavonoid small molecule compound 7, 4 dihydroxy flavone (DHF) from Glycyrrhiza uralensis in DTF inhibited eotaxin, Th2 cytokine and IgE production in vitro and in vivo.
Method: This study comprehensively evaluates the potential therapeutic and immunological mechanisms underlying DHF improvement of symptoms related to EoE using computational modeling, including target mining, gene ontology enrichment, pathway analyses, protein-protein interaction analyses, in silico molecular docking and dynamic simulation followed by ex-vivo target validation by qRT-PCR using cultured human esophagus biopsy specimen with or without DHF from patients with EoE.
Results: Computational analyses defined 29 common targets of DHF on EoE, among which TNF-α, IL-6, IL1β, MAPK1, MAPK3 and AKT1 were most important. Docking analysis and dynamic simulation revealed that DHF directly binds TNF-α with a free binding energy of -7.7 kcal/mol with greater stability and flexibility. Subsequently, in the human esophagus biopsy culture system, significant reduction in levels of TNF-α, IL-6, IL-8 and IL1-β was found in the supernatant of biopsy sample cultured with DHF. Furthermore, the gene expression profile showed significant reduction in levels of TNF-α, IL1-β, IL-6, CCND and MAPK1 in the esophagus biopsy sample cultured with DHF.
Discussion: Taken together, the current study provides us an insight into the molecular mechanisms underlying multi-targeted benefits of DHF in the treatment of EoE and paves the way for facilitating more effective EoE therapies.
EoE is a chronic, immune/antigen- mediated allergic disease of the esophagus that is characterized clinically by esophageal dysfunction and histologically by eosinophil-predominant inflammation (> 15 eosinophils/HPF). The common symptoms of esophageal dysfunction include vomiting, feeding difficulty, dysphagia, and abdominal pain. The incidence of EoE is increasing with estimated prevalence in the US ranging from 40-90 cases/100000 (1). EoE predominates in Caucasian (81%) (2) and middle-aged (30–40 years) men (3) with a male-to-female ratio approaching 3:1 (4). The potential mechanism of EoE pathogenesis includes over expression of Th2 cytokines, genetic predisposition, and environmental stimulation, which initiate esophageal inflammation and subsequent tissue remodeling and fibrosis. It is possible that other molecular targets exist and yet to be identified and studied to better understand the complexity of EoE pathogenesis.
The current therapy for EoE includes the use of swallowed steroid and dietary elimination (5). The dietary approach is proven to be effective in 90% EoE patients and can lead to identifying the diet trigger(s). Sustained remission can be achieved by permanently eliminating the triggers. But multiple upper endoscopies under general anesthesia are often required and prolonged diet avoidance could negatively affect the child’s nutrition intake, physical growth, and quality of life. Systemic steroids although show significant effect in reducing esophageal eosinophils, long-term use is associated with serious side effect. Swallowed steroid treatment is effective and of less side effect, but relapse of EoE is universal after the treatment is stopped (6). Proton-pump inhibitors (PPI) are effective in 15-30% of EoE patients who are PPI-responsive, but long-term use of PPI is associated with a number of side effect (7). Several humanized antibody therapies have been designed to block IL-5 and IL-13 and have showed promising effect in treating EoE (8). These specific therapies are likely to target only particular aspects of the disease but due to complexity and involvement of multiple players in the disease, persistence of inflammation even after the blockage of these single molecules has been observed (8).
Compound 7,4-dihydroxyflavone (DHF)- a flavonoid purified from Glycyrrhiza uralensis is one the most commonly used herbs in traditional Chinese medicine (TCM). We have shown that G. uralensis significantly inhibits TNF-α production (9). Likewise, we have highlighted key effects of DHF on suppression of Th2 cytokines-IL-4, IL-5 and IL-13 and serum IgE (10) and chemo attractant protein eotaxin/CCL-11 (11). The regulatory effect of DHF was further demonstrated in another study where DHF inhibited MUC5AC mRNA and protein expression (12) Recently, we also showed a case study of an 11 yr. old boy with complete clinical and tissue remission of EoE with TCM therapy, including Digestion teas (13). Based on our previous findings, we believe that DHF improvement of symptoms of EoE may be due to its anti-inflammatory effect, which ultimately reduces GI smooth muscle spasm, reverses the tissue inflammation, facilitates epithelium repair, and restores GI bacterial homeostasis.
Given the complexity of pathological mechanisms of EoE and multiple targets of DHF on immunological responses, we utilized computational modeling—target mining, gene ontology enrichment, protein-protein interaction analyses, and in silico molecular docking to estimate the potential therapeutic mechanisms underlying the efficacy of DHF in EoE. The entire workflow of the study is shown in Figure 1. Guided by computational defined therapeutic targets, we employed human biopsy samples obtained from esophagus of EoE patients and determined DHF effects on those targets by tissue culture, ELISA, and qRT-PCR. The approaches in combination of computational modeling and ex vivo biological validation allowed us for the first time to identify potential therapeutic targets of DHF for EoE. This study provides guidance for our future in vivo experimental studies and future clinical trials.
Figure 1 The workflow of computational modeling used for analysis of DHF as one of the promising candidates for Eosinophilic Esophagitis. First, total 440 target genes for EoE were selected. Likewise, 95 biological targets of DHF were collected based on literatures and following published databases including Swiss target prediction, SEA, pub-chem and drug-bank. Mining DHF targets into obtained disease targets uncovered that DHF might potentially regulate 29 targets for EoE. The GO, KEGG pathway and PPI analysis were further conducted to uncover the regulated details of DHF. Moreover, the hub proteins were determined for further molecular docking analysis. Furthermore, the therapeutic hub targets predicted by the computational modeling were validated ex-vivo in human esophageal biopsy samples from patients with EoE by ELISA and qRT-PCR. DHF: 7,4 dihydroxy flavone; SEA, similarity ensemble approach; PPI, protein-protein interactions, GO, gene ontology; KEGG, Kyoto encyclopedia of genes and genomes; EoE, eosinophilic esophagitis.
Biological targets of DHF were identified from literature reports (14, 15) and published databases including HitPick (16), Swiss Target Prediction (17, 18), Similarity Ensemble Approach (19, 20), PubChem (21, 22), PharmMapper (23), and DrugBank (24, 25). The relevant human genes associated with EoE were selected as drug targets from various databases including Therapeutic Target Database (26, 27), Malacards (28), GeneCards (29, 30), and Open Targets Platform (31, 32). To ensure the predominance of targets, only the top 300 genes in each database were considered. Selected targets were finally mapped to UniProt Database (33, 34) for normalization. Next, the shared targets of DHF with EoE were obtained and these were considered to be potentially regulated targets of DHF for the management of patients with EoE.
Target enrichment gene ontology, pathway, and protein-protein interaction (PPI) analyses provided a molecular-level mechanistic insight into biological function. GO was introduced by mapping potential targets to the DAVID database (35, 36). The GO biological process terms with a false discovery rate of (FDR) <0.01 were selected. Pathways were obtained by mapping targets to KOBAS 3.0 (37, 38) and the significant pathways with FDR <0.01 were selected. Potential targets were mapped to String database, obtaining their interaction. The protein interactions were further used to construct the PPI network using Cytoscape (v3.2.1).
With obtained targets and significant pathways, C-T-P-D biological networks were constructed using Cytoscape (v3.2.1). This network, containing DHF, its related targets for EoE, and significant principal pathways were constructed to comprehensively elucidate the complex relationship among compound, targets, and disease related pathways. This analysis provided general information about pharmacological mechanisms of DHF for the treatment and management of EoE at a molecular level. The properties of C-T-P-D networks were validated by NetworkAnalyzer (39), a plugin of Cytoscape.
The binding modes of DHF with critical targets were predicted through molecular docking by AutoDock Vina (40). Protein crystal structures including IL1β (PDB:5R85), IL6 (PDB: 1ALU) (41), TNF (PDB:2AZ5) (42), and CCND1 (PDB:2W96) with excellent resolution were obtained from RCSB protein data bank (43, 44). The structure of DHF was directly downloaded from PubChemwithout further optimization. The molecular graphics were displayed by PyMOL system (45) (46), and Discovery Studio (47). Generally, all hydrogens and Gasteriger charges were added to each molecule. Docking areas and Autogrid parameters were set based on the binding pockets of proteins.
The molecular dynamic simulations were carried out by Groningen Machine for Chemicals Simulations (GROMACS) with amber99sb-ildn force field and tip3p water model. 50 ns molecular dynamics simulation was performed for protein-ligand complex. The Root Mean Square Deviation (RMSD) analysis, and the toot-mean-square fluctuation (RMSF) were carried out using Xmgrace software.
Pediatric subjects (aged 0-18 years old) with potential or known EoE were recruited under an IRB approved protocol at Mount Sinai Medical Center when undergoing an endoscopy as part of their routine clinical care. All subjects were determined to have active EoE based on clinical criteria including symptoms consistent with EoE and biopsies showing >15 eosinophils per high power field. Subjects ‘characteristics are listed in Table 1.
Biopsies were digested with DNases I and Collagenase IV as previously described48 and divided equally into two wells then cultured with or without DHF (0.02mg/ml) in complete RPMI with 10% FBS overnight. Cytokines were measured by ELISA (BD Biosciences, NJ) as per the manufacturer’s instructions.
RNA was extracted from precipitation of esophageal biopsy culture using TRIZOL (Invitrogen, Carlsbad, CA) followed by isopropanol precipitation. RNA was then reverse transcribed to complementary DNA (cDNA) using a PrimeScriptTM RT Reagent Kit (TaKaRa, Mountain View, CA). Real-time PCR was performed using SYBRTM Green Master Mix (Thermo Fisher Scientific, Fair lawn, NJ) as previously described (48). The target gene mRNA expression was normalized to the control group and calculated using the ΔΔCT method. The primer sequences are shown in Table S1.
The Venn diagram shows that 440 target genes for EoE were selected. Likewise, 95 biological targets of DHF were collected from the literature (14, 15), and published databases. Among them, 29 shared targets between DHF and EoE were discovered, which were finally selected as the potential therapeutic targets of DHF in the treatment and management of EoE (Figure 2A).
Figure 2 Computational identification and KEGG pathway analysis of the therapeutic targets. (A) Venn diagram showing the number of targets predicted using computational modeling. Among them 29 targets were shared between DHF and EoE. (B) KEGG Pathway analysis. Y-axis: The top 15 pathway relevant to the enriched targets (left) and genes associated with each pathway (right); X-axis: the significance of each term ranked with –log FDR. DHF, 7,4-dihydroxyflavone, EoE, Eosinophilic Esophagitis, FDR, False discovery rate.
Further, to clarify the potential pharmacological effects of DHF on EoE from the pathway level, the pathway enriched analysis was performed by connecting the predicted targets to DAVID database. The top 15 significant KEGG pathways with False Discovery Rate (FDR) <0.01 and genes associated with each pathway were ranked and picked out by enrichment score –log (FDR) as shown in Figure 2B. Most pathways were related to host immune responses to inflammation and included “IL-17 signaling pathway”, “TNF-signaling pathway”, “AGE-RAGE signaling pathway in diabetic complications” “TH17 cell differentiation”, “Tuberculosis”, “Leishmaniasis”, “C-type lectin receptor signaling pathway” and “Pathway in cancer”. One of the main features of EoE is the accumulation of activated eosinophil at the site of inflammation. Eosinophils, generally, have a short life span of about 4 days and prolonged survival of eosinophil at the site of inflammation is mediated by IL-5, a potent type 2 cytokine (16). IL-5 along with IL-3 and GM-CSF mediate prolonged eosinophilic survival and release their cationic granular proteins, oxygen radicals and lipid mediators to cause tissue damage (16). Similarly, other pathways like “Human cytomegalovirus infection”, “Hepatitis B”, “Kaposi sarcoma-associated herpesvirus infection”, “Proteoglycans in cancer”, and “osteoclast differentiation” are involved in cell survival, proliferation, and progression. Overall, multiple pathways associated with EoE that were regulated by DHF were identified.
The GO biological process terms in DAVID database were obtained with the identified targets as an enriched gene-set. The top 15 biological processes GO terms with False Discovery Rate (FDR) <0.01 were ranked by enrichment score (–log FDR) (Figure S1). The most significant GO biological process terms were closely associated with anti-apoptotic, anti-inflammatory, and anti-oxidative and anti-tumor properties. The most important pathways associated with these properties were “positive regulation of specific DNA binding transcription factor activity”, “positive regulation of transcription DNA-template”, “lipopolysaccharide-mediated signaling pathway”, “positive regulation of nitric oxide biosynthesis”, “positive regulation of transcription from RNA polymerase II promoter”, “inflammatory response”, “positive regulation of fever generation”, and “activation of MAPK”. Interestingly, most of the genes associated with these pathways (Figure S1) were inflammatory genes and were consistent with the KEGG pathway analysis. This further allowed us to explore the mechanism of DHF in treating EoE with respect to associated processes and function.
The C-T-P-D network containing DHF, selected targets, top 15 pathways and EoE was developed to interpret the potential pharmacological mechanisms of DHF for management and treatment of EoE at the molecular level (Figures 3A, B). The C-T-P-D network provides general information about the complex interactions of compound, targets, and their related diseases. The frequency of targets appearing in the top 15 pathways implies their influence and importance. Node color from green to red is proportional to degree value, displaying importance from high to low in the network. The most important protein targets based on its degree from the C-T-P-D network were TNF, MAPK1, MAPK3, IL6, IL1B, AKT1 and CASP3 and the most important pathways associated with these protein targets were “Pathways in cancer”, “IL-17 signaling pathway”, and “Human cytomegalovirus infection”. Overall, the C-T-P-D network helped to simplify the complex interaction between proteins, and pathways associated with EoE and DHF. Furthermore, it helped to select key target proteins which could potentially be targeted by DHF in treating EoE.
Figure 3 Compound-target- pathway-disease network of DHF for EoE. (A) By computational modeling, the role of DHF was deciphered through the drug-target network, and the target-disease network from the molecular level to the systems level. Triangle, circle, and diamond represent DHF, key targets and pathways, respectively. Node color from green to red is proportional to its degree. Black lines stand for interaction between the nodes The most important targets were TNF, MAPK1, MAPK3, IL6, IL1B, AKT1 and CASP3 (Red). The most important pathways associated with the protein targets were “Pathways in cancer” (P1), “IL-17 signaling pathway” (P2), and “Human cytomegalovirus infection” (P3). (B) Pathways associated in the compound-target-disease network.
The PPI network was constructed by mapping potential targets to the String database (17). The size of the node from large to small is proportional to its degree value in the network. It is well known that protein-protein interactions are critical to a wide range of biological processes, including cell-to-cell interaction and metabolic and developmental control (18). A deeper understanding of such complex relationships among disease-related proteins provides new opportunities to investigate the molecular mechanisms of diseases (19). Recently, PPI has become a reliable tool to evaluate protein functions in the network and determine hub proteins in the regulation of diseases. In this study, we found TNF occupying the central position in the network along with IL6, MAPK3, MAPK1 and AKT1 (Figure 4). The branching lines from these proteins represent its interaction with other proteins and we found highest interaction of these key proteins with others in the protein-protein interaction analysis. The other important proteins in the network were IL1B, IL4, CASP3, CCND1, and PTGS2 and these proteins showed moderate rank in the PPI network. Some of the less important proteins formed the outer layer of the network and showed lowest interaction with others. Overall, the PPI network led to specifically select key proteins with the highest interaction index to better understand how DHF could potentially regulate these proteins in management of EoE.
Figure 4 Protein-protein interactions. The PPI network was constructed by mapping potential targets to the Strings database. The size of the node from large to small is proportional to its degree value in the network. The circles represent the therapeutic targets, and the purple lines represent the interaction between the nodes.
We assumed that DHF regulated the crucial targets by interfering in gene expression or binding with proteins directly. Therefore, a molecular docking was introduced to calculate the binding energy and evaluate binding favorability between DHF, and crucial targets. The binding energy indicated that DHF might be a promising regulator for each of the selected targets with binding affinities between -6.3 to -7.7 Kcal/mol (Table S2). The optimal binding modes of DHF with targets including TNF-α, MAPK1, IL1B, IL-6, and CCND1 is shown in Figure 5. The best results were obtained for complex TNF-DHF with a free binding energy of -7.7 kcal/mol. The Hydrogen bonds between DHF with TYR151 and the π-π stacking between DHF and LEU120 stabilized the left structure of DHF in one chain (Figures 5A, F). Likewise, DHF displayed similar binding affinities with MAPK1 and IL1-β. For MAPK1-DHF complex, the hydrogen bond (MET108), π-alkyl interactions (ILE31, ILE84, ALA52, CYS166), and π-anion interactions (ASP111) with DHF stabilizing the configuration of DHF in the complex (Figures 5B, G). Similarly, for complex IL1B-DHF, the hydrogen bond between DHF and residues (TYR24, LEU134, THR79) significantly contributed to the stability of the complex (Figures 5C, H). For complex IL6-DHF, DHF fitted well in the binding cavity of IL-6 surrounded by hydrogen bonds (ASP34, ARG30), π-sigma (LEU33, LEU178), π-alkyl (ARG179) and π-π (ARG30) interactions (Figures 5D, I). Lastly, for the complex CCND1-DHF, the residues ARG87, LEU91, and LYS149 facilitated the binding interactions by hydrogen, π-π stacking, and π-cation effects (Figures 5E, J). To evaluate the stability of binding, TNF-DHF complex were further optimized by dynamic stimulation using Gromacs. The stability and flexibility of complex were estimated by RMSD (Figure S2A) and RMSF (Figure S2B) analysis respectively. The trend of RMSD figure indicated the stable binding of DHF with TNF. It is worth noting that the average RMSD value for TNF was 4.9 Å. The RMSF analysis implied minor deviation of protein during the simulation period. The most stable binding mode of TNF-DHF were displayed in Figures S2C, D respectively. Residues TYR59, ILE155, and LEU57 contributed to maintain the stability of DHF with TNF. Overall, the molecular docking results indicated that DHF bind with the crucial proteins directly to regulate the resulting biological effects.
Figure 5 Molecular docking analysis to predict the binding modes between DHF and its therapeutic targets. Based on the gene expression results in the esophagus, the binding explorations of complex MAPK1-DHF, IL1B-DHF, IL6-DHF, TNF-DHF, CCND1-DHF were explored. Predicted lowest-energy binding mode of DHF in 3-dimentiaonal figure with the following proteins: (A), (F) TNF-α; (B), (G) MAPK1; (C), (H) IL1B; (D), (I) IL6; (E), (J) CCND1; the carbon, oxygen and nitrogen are highlighted in yellow, red, and blue, respectively. For residents of proteins, the green, red, and blue stand for C, O and N, respectively.
To provide experimental support and validate the claim that DHF could be an effective alternative for the management of EoE patients, we evaluated the effect of DHF on key therapeutic targets obtained from the computational analysis. We evaluated the levels of different pro-inflammatory cytokines by ELISA and their expression by qRT-PCR in human esophageal biopsy cultures with or without the presence of DHF. In presence of DHF, we found significantly lower levels of pro-inflammatory cytokines- TNF-α, IL-8, IL-6, IL-1B respectively (Figures 6A–D) and slight increase in IL-10 levels (p=0.38) (Figure 6E) in the culture supernatant of esophagus biopsies. Furthermore, in presence of DHF, the gene expression analysis showed marked decrease in the expression levels of TNF-α, IL1-B, IL-6, CCND and MAPK1 respectively (Figures 7A–E). Likewise, there was a slight decrease in expression of CASP3 and PPARγ (p=0.08) respectively (Figures 7F, G). However, there was no statistical differences in the expression levels of MAPK3 and Akt respectively (Figures 7H, I).
Figure 6 Anti-inflammatory effect of DHF on esophageal biopsy sample: Esophageal biopsy samples were cultured with or without DHF for 24 hrs. and cytokine levels in the culture supernatant were measured by ELISA. Percentage inhibition of cytokines TNF-α (A), IL-8 (B), IL-6 (C), IL1-β (D) and percentage of increase in cytokine IL-10 (E) in presence of DHF were analyzed. Data represents Mean ± SEM. N=6-8 samples. ***p < 0.001; *p < 0.05 compared to Medium. Paired t test was used for statistical analysis.
Figure 7 Effect of DHF on therapeutic target gene in esophageal biopsy sample: Based on the therapeutic hub targets predicted by the computational modeling, the expression levels of key targets were validated ex-vivo using human esophageal biopsy samples cultured in the presence of DHF. The relative expression levels of TNF-α (A), IL1β (B), IL-6 (C), CCND (D) and MAPK1 (E) were significantly inhibited in the presence of compound DHF. Similarly, the expression levels of PPARγ (F), CASP3 (G), MAPK3 (H) and AKT (I) showed no significant difference as compared to the medium. Data represents Mean ± SEM. N = 6-8 samples. *p < 0.05; **p < 0.01 compared to Medium. Paired t test was used for statistical analysis.
In this study we, for the first time, used a computational approach that integrated target mining, network technology and pathway analysis to reveal the potential therapeutic pathways and targets to understand pharmacological mechanisms of DHF in treating EoE. The cutting-edge technologies allowed us to uncover key mechanisms involved in the pathogenesis of EoE and further advance our knowledge into new therapeutic applications. It is believed that mechanism of EoE is strictly driven by complex interplay between genetic and early life environmental risk factors (20), interaction and signaling between epithelial, mesenchymal, and immune cells on molecular and intracellular level (21). Based on our results, we selected top 15 pathways of which majority of them were inflammatory. This further adds to the complexity of the disease by deciphering key inflammatory molecular pathways and provide evidence that these pathways may be contributing directly or indirectly to the progression of disease. Some of the important pathways were- pathway in cancer, IL-17 signaling, TNF signaling, AGE-RAGE signaling in diabetic complications, and several other infectious disease related pathways. Furthermore, by mapping these pathways in the C-P-T-D network, we identified the key targets associated with these pathways which could potential be regulated by DHF in EoE. The most prominent targets were MAPK1, MAPK3, TNF, IL6, IL1B and AKT1, also known to as an important cytokine regulating tissue damage. The pathogenesis of EoE is still incompletely understood but it be believed to be a consequence of a complex interplay between genetic, environment and host immune cells (22). Beside these factors, it is plausible that the pathways and genes identified in this study by computational approach could be playing a role in the pathogenesis of EoE. Further studies are necessary to better understand the mechanisms at molecular level. Similarly, the molecular docking and dynamic simulation analysis revealed that DHF can directly bind to the target molecules with similar binding affinities to regulate its action in preventing inflammation. The strongest binding with superior stability and minor deviation was seen between DHF and TNF. The Molecular simulation could accurately predict many important dynamics, but sometimes these simulations are not suitable to systems where metal atoms are involved in binding requiring robust quantum mechanical calculations. Finally, we validated our computational modeling results using esophagus biopsy sample from patient with EoE. We demonstrated that in presence of DHF there was significant reduction in the levels of TNF-α, IL-8, IL-6, IL1B and in the culture supernatant and further validated its effect in the molecular level by qRT-PCR. Our gene expression data showed significant decrease in the expression of key pro-inflammatory genes TNF-α, IL-1B, IL-6, CCND and MAPK1. This study provides an evidence DHF could potentially be regulating inflammation in EoE and further studies with large sample size should be conducted to better understand the mechanisms associated with it.
At present, dupilumab is the only the FDA approved treatment for EoE (23), and highlighting the complex nature of the disease, it is crucial to advance our knowledge and strengthen the research into therapeutic agents that can target multiple aspects of the disease. Evidence of successful management of a patient with GI symptoms secondary to EoE using digestion tea containing DHF (13) opens up the area to study the role of flavonoid in treating EoE. With this study, we were able to successfully identify key targets and subsequently validate it using the human esophagus biopsy sample from patient with EoE. It could be possible that DHF mechanism of acting in successfully treating EoE patient was due to its action on the pathways and genes that we identified in our study. This study may provide a potential role of DHF as an active therapeutic candidate in the management and treatment of EoE. The direct effect of DHF in animal models of eosinophilic disease is the next step towards understanding and determining if DHF will be efficacious for the treatment of EoE.
The current study uses computational approach to identify key targets which are potentially regulated by DHF in EoE. However, the key targets obtained using this approach are not primary drivers of eosinophilic esophagitis but based on the evidence on the literature the role of TNF in EoE has been actively investigated. It has been reported that the inflamed epithelial cells prime esophageal fibroblasts to secrete the profibrogenic cytokines IL-1β and TNF-α, which in turn promote epithelial-to-mesenchymal transition and esophageal fibrosis (48). Additionally, it has been reported that cross talk between esophageal epithelial cells and fibroblast leads to robust production of TNF, contributing to fibrostenotic EoE (49). Furthermore, several TNF superfamily of proteins has been shown to be transcribed by several different subsets of T cells in the esophagus including the pathogenic effector Th2 cells (50). This makes us further believe that TNF play an important role in EoE, possibly at the chronic stage and a therapy targeting TNF in EoE would potentially contribute to one endotype of EoE. With current therapy having limited effects on treating fibrostenotic EoE, and ample evidence of production of TNF by esophageal fibroblast to contribute to inflammatory cascade in EoE (51), this study opens up a new area in EoE therapeutics by showing the role of DHF in modulating pro-inflammatory markers in EoE. In addition, most of the targets that we found in this study shared common pathways of inflammation and it plausible that all these inflammatory players could possibly be playing a role in exacerbating eosinophilic inflammation. Even though the effect of DHF on predicted target using computational approach seems promising, further studies are required to test the effect of DHF clinically for proposed activity against inflammatory EoE as well as the detailed mechanism of action on how these genes and targets interact with primary drivers of EoE, including Th2 genes- IL-4, IL-5, and IL-13 needs to be studied. This may be done either alone or in combination with an FDA approved treatment, Dupixent.
The original contributions presented in the study are included in the article/Supplementary Material. Further inquiries can be directed to the corresponding author.
The studies involving human participants were reviewed and approved by IRB approved protocol at Mount Sinai Medical Center. Written informed consent to participate in this study was provided by the participants’ legal guardian/next of kin.
All authors listed have made a substantial, direct, and intellectual contribution to the work and approved it for publication.
This study was supported by The Moss family foundation, The Weissman family, Laura Sagerman, The Robertson-Li family, The Smith family, and The Yen family (ORA LOG NO.: 16570-101 to X-ML). X-ML received research support from the National Institutes of Health (NIH)/National Center for Complementary and Alternative Medicine (NCCAM) # 1P01 AT002644725-01 “Center for Chinese Herbal Therapy (CHT) for Asthma”, and grant #1R01AT001495-01A1 and 2R01 AT001495-05A2; Food Allergy Research and Education (FARE) and Winston Wolkoff Integrative Medicine Fund for Allergies and Wellness, the Parker Foundation and Henan University of Chinese Medicine.
We thank Henry Ehrlich for proofreading the manuscript.
X-ML received consultancy fees from FARE and Johnson & Johnson Pharmaceutical Research & Development, L.L.C. Bayer Global Health LLC; received royalties from UpToDate; is an Honorary Professor of Chinese Medical University, Taichung, Taiwan; Henan University of Chinese Medicine Zhenzhou, China, and Professorial Lecture at Icahn School of Medicine at Mount Sinai, New York, NY, US; received travel expenses from the NCCAM and FARE; share US patent PCT/US05/008417 (FAHF-2), PCT 14/875,772 (XPP), PCT/US2014/012306 (S. Flavescens), PCT/US14/68396 (WL); PCT/US2017/056822) (nanoBBR, pending), and is a member of Herbs Springs LLC and General Nutraceutical Technology LLC and Health Freedom LLC; take compensation from her practice at Integrative Health and Acupuncture PC. NY shares PCT/US2014/012306 (S. Flavescens), PCT/US14/68396 (WL), is a member of General Nutraceutical Technology LLC and Health Freedom LLC.
All claims expressed in this article are solely those of the authors and do not necessarily represent those of their affiliated organizations, or those of the publisher, the editors and the reviewers. Any product that may be evaluated in this article, or claim that may be made by its manufacturer, is not guaranteed or endorsed by the publisher.
The Supplementary Material for this article can be found online at: https://www.frontiersin.org/articles/10.3389/fimmu.2022.1015437/full#supplementary-material
1. Dellon ES, Jensen ET, Martin CF, Shaheen NJ, Kappelman MD. Prevalence of eosinophilic esophagitis in the united states. Clin Gastroenterol Hepatol (2014) 12(4):589–96.e1. doi: 10.1016/j.cgh.2013.09.008
2. Hruz P, Straumann A, Bussmann C, Heer P, Simon HU, Zwahlen M, et al. Escalating incidence of eosinophilic esophagitis: a 20-year prospective, population-based study in olten county, Switzerland. J Allergy Clin Immunol (2011) 128:1349–50.e5. doi: 10.1016/j.jaci.2011.09.013
3. Sperry SL, Woosley JT, Shaheen NJ, Dellon ES. Influence of race and gender on the presentation of eosinophilic esophagitis. Am J Gastroenterol (2012) 107(2):215–21. doi: 10.1038/ajg.2011.342
4. Mansoor E, Cooper GS. The 2010-2015 prevalence of eosinophilic esophagitis in the USA: A population-based study. Digestive Dis Sci (2016) 61(10):2928–34. doi: 10.1007/s10620-016-4204-4
5. D'Alessandro A, Esposito D, Pesce M, Cuomo R, De Palma GD, Sarnelli G. Eosinophilic esophagitis: From pathophysiology to treatment. World J Gastrointest Pathophysiol (2015) 6(4):150–8. doi: 10.4291/wjgp.v6.i4.150
6. Butz BK, Wen T, Gleich GJ, Furuta GT, Spergel J, King E, et al. Efficacy, dose reduction, and resistance to high-dose fluticasone in patients with eosinophilic esophagitis. Gastroenterology (2014) 147(2):324–33.e5. doi: 10.1053/j.gastro.2014.04.019
7. Yibirin M, De Oliveira D, Valera R, Plitt AE, Lutgen S. Adverse effects associated with proton pump inhibitor use. Cureus (2021) 13(1):e12759. doi: 10.7759/cureus.12759
8. Patel RV, Hirano I, Gonsalves N. Eosinophilic esophagitis: Etiology and therapy. Annu Rev Med (2021) 72:183–97. doi: 10.1146/annurev-med-052819-023848
9. Patil SP, Liu C, Yang N, Li X-M. Glycyrrhiza uralensis flavonoids inhibit brain microglial cell TNF-α secretion, p-IκB expression, and increase brain-derived neurotropic factor (BDNF) secretion. J Traditional Chin Med Sci (2014) 1(1):28–37. doi: 10.1016/j.jtcms.2014.11.004
10. Yang N, Patil S, Zhuge J, Wen MC, Bolleddula J, Doddaga S, et al. Glycyrrhiza uralensis flavonoids present in anti-asthma formula, ASHMI™, inhibit memory Th2 responses in vitro and in vivo. Phytother Res (2013) 27(9):1381–91. doi: 10.1002/ptr.4862
11. Jayaprakasam B, Doddaga S, Wang R, Holmes D, Goldfarb J, Li XM. Licorice flavonoids inhibit eotaxin-1 secretion by human fetal lung fibroblasts in vitro. J Agric Food Chem (2009) 57(3):820–5. doi: 10.1021/jf802601j
12. Liu C, Weir D, Busse P, Yang N, Zhou Z, Emala C, et al. The flavonoid 7,4'-dihydroxyflavone inhibits MUC5AC gene expression, production, and secretion via regulation of NF-kappaB, STAT6, and HDAC2. Phytother Res (2015) 29(6):925–32. doi: 10.1002/ptr.5334
13. Soffer G, Kaman K, Li XM. Successful management of eosinophilic esophagitis using traditional Chinese medicine: A case report. Yale J Biol Med (2020) 93(5):685–8.
14. Chen XW, Di YM, Zhang J, Zhou ZW, Li CG, Zhou SF. Interaction of herbal compounds with biological targets: a case study with berberine. ScientificWorldJournal (2012) 2012:708292. doi: 10.1100/2012/708292
15. Liu H, You L, Wu J, Zhao M, Guo R, Zhang H, et al. Berberine suppresses influenza virus-triggered NLRP3 inflammasome activation in macrophages by inducing mitophagy and decreasing mitochondrial ROS. J leukocyte Biol (2020) 108(1):253–26. doi: 10.1002/JLB.3MA0320-358RR
16. HitPick. mips.helmholtz-muenchen.de/hitpick/. Accessed June 2022.
17. Swiss Target prediction. swisstargetprediction.ch/. Accessed June 2022.
18. Daina A, Michielin O, Zoete V. SwissTargetPrediction: updated data and new features for efficient prediction of protein targets of small molecules. Nucleic Acids Res (2019) 47(W1):W357–w64. doi: 10.1093/nar/gkz382
19. Similarity ensemble approach. sea.bkslab.org/. Accessed June 2022.
20. Keiser MJ, Roth BL, Armbruster BN, Ernsberger P, Irwin JJ, Shoichet BK. Relating protein pharmacology by ligand chemistry. Nat Biotechnol (2007) 25(2):197–206. doi: 10.1038/nbt1284
21. PubChem. pubchem.ncbi.nlm.nih.gov/. Accessed June 2022.
22. Kim S, Chen J, Cheng T, Gindulyte A, He J, He S, et al. PubChem 2019 update: improved access to chemical data. Nucleic Acids Res (2019) 47(D1):D1102–d9. doi: 10.1093/nar/gky1033
23. Wang X, Shen Y, Wang S, Li S, Zhang W, Liu X, et al. PharmMapper 2017 update: a web server for potential drug target identification with a comprehensive target pharmacophore database. Nucleic Acids Res (2017) 45(W1):W356–w60. doi: 10.1093/nar/gkx374
24. DrugBank. drugbank.ca. Accessed June 2022.
25. Wishart DS, Knox C, Guo AC, Cheng D, Shrivastava S, Tzur D, et al. DrugBank: A knowledgebase for drugs, drug actions and drug targets. Nucleic Acids Res (2008) 36(Database issue):D901–6. doi: 10.1093/nar/gkm958
26. Therapeutic target database. db.idrblab.net/ttd/. Accessed June 2022.
27. Wang Y, Zhang S, Li F, Zhou Y, Zhang Y, Wang Z, et al. Therapeutic target database 2020: enriched resource for facilitating research and early development of targeted therapeutics. Nucleic Acids Res (2020) 48(D1):D1031–d41. doi: 10.1093/nar/gkz981
28. Rappaport N, Twik M, Plaschkes I, Nudel R, Iny Stein T, Levitt J, et al. MalaCards: an amalgamated human disease compendium with diverse clinical and genetic annotation and structured search. Nucleic Acids Res (2017) 45(D1):D877–d87. doi: 10.1093/nar/gkw1012
29. GeneCards. genecards.org/. Accessed June 2022.
30. Safran M, Solomon I, Shmueli O, Lapidot M, Shen-Orr S, Adato A, et al. GeneCards 2002: towards a complete, object-oriented, human gene compendium. Bioinformatics (2002) 18(11):1542–3. doi: 10.1093/bioinformatics/18.11.1542
31. . Open targets platform. targetvalidation.org/. Accessed June 2022.
32. Carvalho-Silva D, Pierleoni A, Pignatelli M, Ong C, Fumis L, Karamanis N, et al. Open targets platform: New developments and updates two years on. Nucleic Acids Res (2019) 47(D1):D1056–d65. doi: 10.1093/nar/gky1133
33. . UniProt: A worldwide hub of protein knowledge. Nucleic Acids Res (2019) 47(D1):D506–d15. doi: 10.1093/nar/gky1049
34. UniProt: A worldwide hub of protein knowledge. Nucleic Acids Res (2019) 47(D1):D506–d15. doi: 10.1093/nar/gky1049
35. Huang DW, Sherman BT, Lempicki RA. Bioinformatics enrichment tools: paths toward the comprehensive functional analysis of large gene lists. Nucleic Acids Res (2008) 37(1):1–13. doi: 10.1093/nar/gkn923
36. DAVID. david.ncifcrf.gov/. Accessed June 2022.
37. Xie C, Mao X, Huang J, Ding Y, Wu J, Dong S, et al. KOBAS 2.0: a web server for annotation and identification of enriched pathways and diseases. Nucleic Acids Res (2011) 39(suppl_2):W316–W22. doi: 10.1093/nar/gkr483
38. KOBAS 3.0. kobas.cbi.pku.edu.cn/. Accessed June 2022.
39. Assenov Y, Ramírez F, Schelhorn S-E, Lengauer T, Albrecht M. Computing topological parameters of biological networks. Bioinformatics (2007) 24(2):282–4. doi: 10.1093/bioinformatics/btm554
40. Trott O, Olson AJ. AutoDock vina: improving the speed and accuracy of docking with a new scoring function, efficient optimization, and multithreading. J Comput Chem (2010) 31(2):455–61. doi: 10.1002/jcc.21334
41. Somers W, Stahl M, Seehra JS. 1.9 a crystal structure of interleukin 6: implications for a novel mode of receptor dimerization and signaling. EMBO J (1997) 16(5):989–97. doi: 10.1093/emboj/16.5.989
42. He MM, Smith AS, Oslob JD, Flanagan WM, Braisted AC, Whitty A, et al. Small-molecule inhibition of TNF-alpha. Science (2005) 310(5750):1022–5. doi: 10.1126/science.1116304
44. Berman HM, Westbrook J, Feng Z, Gilliland G, Bhat TN, Weissig H, et al. The protein data bank. Nucleic Acids Res (2000) 28(1):235–42. doi: 10.1093/nar/28.1.235
45. DeLano WL. Pymol: An open-source molecular graphics tool. CCP4 Newslett On Protein Crystallography (2002) 40:82–92.
48. Hill DA, Spergel JM. The immunologic mechanisms of eosinophilic esophagitis. Curr Allergy Asthma Rep (2016) 16(2):9. doi: 10.1007/s11882-015-0592-3
49. Kasagi Y, Dods K, Wang JX, Chandramouleeswaran PM, Benitez AJ, Gambanga F, et al. Fibrostenotic eosinophilic esophagitis may reflect epithelial lysyl oxidase induction by fibroblasts-derived tumor necrosis factor-alpha. J Allergy Clin Immunol (2019) 144(1):171–82. doi: 10.1016/j.jaci.2018.10.067
50. Manresa MC, Miki H, Miller J, Okamoto K, Dobaczewska K, Herro R, et al. A deficiency in the cytokine TNFSF14/LIGHT limits inflammation and remodeling in murine eosinophilic esophagitis. J Immunol (2022) 26:ji2200326. doi: 10.4049/jimmunol.2200326
51. Manresa MC, Chiang AWT, Kurten RC, Dohil R, Brickner H, Dohil L, et al. Increased production of LIGHT by T cells in eosinophilic esophagitis promotes differentiation of esophageal fibroblasts toward an inflammatory phenotype. Gastroenterology (2020) 159(5):1778–92.e13. doi: 10.1053/j.gastro.2020.07.035
Keywords: 7, 4 dihydroxy flavone (DHF), eosinophilic esophagitis, anti-inflammation, computational modelling, molecular docking
Citation: Maskey AR, Wang Z-Z, Chen X, Dunkin D, Yang N, Soffer G, Yuan Q and Li X-M (2022) Computational analysis to define efficacy & molecular mechanisms of 7, 4’- Dihydroxyflavone on eosinophilic esophagitis: Ex-vivo validation in human esophagus biopsies. Front. Immunol. 13:1015437. doi: 10.3389/fimmu.2022.1015437
Received: 09 August 2022; Accepted: 21 November 2022;
Published: 15 December 2022.
Edited by:
Simon Patrick Hogan, Michigan Medicine, University of Michigan, United StatesReviewed by:
Zhenwen Zhou, Guangzhou Medical University, ChinaCopyright © 2022 Maskey, Wang, Chen, Dunkin, Yang, Soffer, Yuan and Li. This is an open-access article distributed under the terms of the Creative Commons Attribution License (CC BY). The use, distribution or reproduction in other forums is permitted, provided the original author(s) and the copyright owner(s) are credited and that the original publication in this journal is cited, in accordance with accepted academic practice. No use, distribution or reproduction is permitted which does not comply with these terms.
*Correspondence: Xiu-Min Li, WGl1TWluX0xpQG55bWMuZWR1
†These authors have contributed equally to this work
Disclaimer: All claims expressed in this article are solely those of the authors and do not necessarily represent those of their affiliated organizations, or those of the publisher, the editors and the reviewers. Any product that may be evaluated in this article or claim that may be made by its manufacturer is not guaranteed or endorsed by the publisher.
Research integrity at Frontiers
Learn more about the work of our research integrity team to safeguard the quality of each article we publish.