- School of Life Science, Ludong University, Yantai, Shandong, China
Introduction: Coronavirus Disease-2019 (COVID-19) is an infectious disease caused by SARS-CoV-2. Severe cases of COVID-19 are characterized by an intense inflammatory process that may ultimately lead to organ failure and patient death. Qingfei Paidu Decoction (QFPD), a traditional Chines e medicine (TCM) formula, is widely used in China as anti-SARS-CoV-2 and anti-inflammatory. However, the potential targets and mechanisms for QFPD to exert anti-SARS-CoV-2 or anti-inflammatory effects remain unclear.
Methods: In this study, Computer-Aided Drug Design was performed to identify the antiviral or anti-inflammatory components in QFPD and their targets using Discovery Studio 2020 software. We then investigated the mechanisms associated with QFPD for treating COVID-19 with the help of multiple network pharmacology approaches.
Results and discussion: By overlapping the targets of QFPD and COVID-19, we discovered 8 common targets (RBP4, IL1RN, TTR, FYN, SFTPD, TP53, SRPK1, and AKT1) of 62 active components in QFPD. These may represent potential targets for QFPD to exert anti-SARS-CoV-2 or anti-inflammatory effects. The result showed that QFPD might have therapeutic effects on COVID-19 by regulating viral infection, immune and inflammation-related pathways. Our work will promote the development of new drugs for COVID-19.
1. Introduction
The pandemic of Coronavirus Disease-2019 (COVID-19) has posed an unprecedented crisis to global public health (1–3). The main symptoms of COVID-19 infection are fever, coughing, shortness of breathing and also death (4). Traditional Chinese medicine (TCM) formulas was widely used in China against COVID-19, especially in 2020, in the absence of specific drugs and vaccines (5–7). Based on the current clinical investigation, the treatment of inflammatory storms has been proposed as a critical part of rescuing severe COVID-19 (8–11). Qingfei Paidu Decoction (QFPD) has become one of the most used compounds due to its potential role in the treatment of COVID-19 (12–14). QFPD is composed of 20 herbs (15), namely Agaric (Zhuling), Oriental waterplantain tuber (Zexie), Largehead atractylodes rhizome (Baizhu), Cassia twig (Guizhi), Chinese ephedra herb (Mahuang), Semen armeniacae amarum (Xingren), Poria cocos (Fuling), Chinese thorowax root (Chaihu), Baikal skullcap root (Huangqin), Pinellinae rhizoma praeparatum (Jiangbanxia), Common ginger rhizome (Shengjiang), Tatarian aster root and rhizomes (Ziwan), Common coltsfoot flower (Kuandonghua), Blackberrglily rhizome (Shegan), Manchurian wildginger herb (Xixin), Common yam rhizome (Shanyao), Immature bitter orange (Zhishi), Wrinkled gianthyssop herb (Huoxiang), Dried tangerine peel (Chenpi), and Baked radix glycyrrhizae (Zhigancao). The combination of these herbs is used to reduce mortality and improve cure rates in patients with COVID-19 (16).
Computer-Aided Drug Design (CADD) is a method for developing lead compounds by theoretical calculation, simulation, and prediction of the relationship between drugs and receptors (17). Network pharmacology is a powerful tool to reflect the pharmacological effects and mechanisms of TCM (18–20). The concept of holism for TCM has much in common with the major points of network pharmacology, in which the general “one target, one drug” mode is shifted to a new “multi-target, multi-component” mode (21). The research method of CADD combined with network pharmacology can be used to reveal the mystery of the “multi-target, multi-component and multi-path” of TCM formulas. This method greatly improves the success rate of drug research and saves the cost of drug development.
QFPD has the potential therapeutic effects for COVID-19 intervention in China, but how to take advantage of its anti-SARS-CoV-2 and anti-inflammatory effects deserves further exploration. Therefore, we aim to screen the antiviral or anti-inflammatory components in QFPD and their targets using Discovery Studio 2020 (DS2020) software. We compared the targets regulated by the active components in QFPD with the COVID-19 targets recorded in the Genecards database (https://www.genecards.org) and obtained their common targets. With the help of network pharmacology, we investigated how QFPD regulates the body from various aspects through multiple targets and multiple pathways. The results provided some vital information for the precise clinical medication and improved the therapeutic ability of TCM for COVID-19.
2. Experimental section
2.1. Screening the active components in QFPD from the database
Most active components of 20 herbs in QFPD were collected through the Traditional Chinese Medicines Database (TCMdb). It was a new tool with 23033 active components to support the modernization of TCM (22–24). In addition, the Traditional Chinese Medicine Systems Pharmacology Database (TCMSP database; http://tcmspw.com/tcmsp.php) was used to supplement active components. The TCMSP database integrated active components, relevant diseases, targets, and pharmacokinetic data of drugs, providing a new platform for studying the mechanism of drug action systematically (25). Only components with antiviral or anti-inflammatory effects were retained for later studies.
2.2. Ligand preparation and the prediction of absorption, distribution, metabolism, excretion, and toxicity properties
The Prepare Ligands protocol helped to prepare ligands for input components, performing tasks such as removing duplicates, enumerating isomers and tautomers. This study performed the following steps to complete this operation: changing ionization, generating tautomers, generating isomers, and fixing bad valencies. Then, the ligands of active components needed to be minimized in batches by Minimize Ligands protocol before ADMET properties’ prediction. ADMET refers to the Absorption, Distribution, Metabolism, Excretion, and Toxicity properties of a molecule within an organism (26, 27). The ADMET properties predicted in this study were hepatotoxicity, Blood-brain barrier penetration (BBB) and Human intestinal absorption (HIA) (28, 29). Optimizing these two properties during early drug discovery was crucial for reducing ADMET problems later in development.
2.3. The prediction of toxicological properties and druggability screening
Toxicity Prediction by Komputer Assisted Technology (TOPKAT) accurately and rapidly assessed the toxicity of chemicals based solely on their 2D molecular structure. It could assess the toxicological properties of candidate active components with a range of robust, cross-validated, and Quantitative Structure-Toxicity Relationship (QSTR) models (30). The toxicological properties we predicted in this study were the Ames test, Rodent Carcinogenicity, Aerobic Biodegradability, and oral LD50 in rats. After the predicted results were obtained, all active components that exceed these four properties’ optimal prediction space (OPS) needed to be deleted manually. In order to exclude active components with poor absorption, permeation, and oral bioavailability, it was necessary to ensure that the screened active components comply with Lipinski’s rule of five (31) and Veber’s rules (32, 33). The active components that did not meet these rules will be automatically deleted at the end of the calculation. The reserved active components had better pharmacokinetic properties and higher bioavailability in the metabolism of organisms.
2.4. Performing reverse finding target
The technique of reverse finding target was the core of this study. Reverse finding target process was to match the pharmacophore models with active components which had high credibility after a series of screening. In addition, the matching degree of pharmacophore models and active components can be distinguished by different colors in the “Heat map of Ligand profiler”. Generally, the higher the matching between the pharmacophore models and the active components, the higher the confidence of the targets corresponding to the pharmacophore models. Based on these pharmacophore models, we can identify the target proteins regulated by the active components of QFPD. We then used the search function in the Protein Data Bank (PDB) database to convert the target protein names to standard gene names. COVID-19 targets recorded in the Genecards database and QFPD targets regulated by active ingredients in QFPD were compared to get common targets. These common targets represented potential targets for QFPD to exert anti-SARS-CoV-2 or anti-inflammatory effects.
2.5. Construction of protein-protein interaction network
The interaction between the targets was illustrated as a PPI network. The construction of the PPI network was realized via the Search Tool for the Retrieval of Interacting Genes (STRING; https://string-db.org/). It gathered a large number of information resources, mainly for storing PPI data identified by experiments, calculating predicted data, and collecting public text (34).
2.6. Construction of “herb-active component-target” interaction network diagram
The “herb-active component-target” interaction network was plotted by the major constituents of QFPD and their targets using Cytoscape 3.8.0. The network constructed by this information was represented as nodes and edges with related data attributes, which revealed the close relationship between diseases, targets, and drugs, and provided ideas for further study of multi-target and multi-component TCM formula.
2.7. Pathway analysis of QFPD
Gene set enrichment analysis (GSEA) was performed on transcriptome sequencing data of COVID-19 from Gene Expression Omnibus (GEO) database using GSEA 4.1.0. The COVID-19 group consisted of 30 samples, which were organized into gene expression matrix. Based on the expression of the target, they were divided into target high expression and low expression groups for GSEA analysis.
The potential targets of QFPD were submitted to DAVID (https://david.ncifcrf.gov/) to analyze Gene Ontology (GO) function enrichment and Kyoto Encyclopedia of Genes and Genomes (KEGG) pathway enrichment. GO analysis was involved in terms of cellular component (CC), molecular function (MF), as well as biological process (BP) (35). CC was defined as the active sites of gene products in cells. MF was considered as the biochemical activity. BP involved the contribution of genes or genetic products to biological objectives. KEGG was a highly integrated database for biological interpretation of wholly sequenced genomes through pathway mapping (36).
3. Results and discussion
3.1. Screening the active components in QFPD from the database
A total of 108 active components in QFPD were selected from TCMdb and TCMSP database based on the above criteria about antiviral or anti-inflammatory effects. The basic information of 108 active components was showed in Table 1.
3.2. Ligand preparation and prediction of ADMET properties
Prepare Ligands and Minimize Ligands protocols in DS2020 were used to prepare and minimize the structures of 108 active components, respectively. The results showed that 107 tautomers were produced during the preparation process, so the number of active components reached 215. After minimization, the number of active components remained unchanged. Favorable ADMET properties can be considered as essential nature for a candidate drug. As shown in Figure 1, the green ellipse represents 99% of the absorption confidence interval, and the blue ellipse represents 99% of the BBB confidence interval. In general, the absorption outside the 99% ellipse tends to drop relatively quickly. If the active component is outside the 99% confidence interval of the BBB model, the prediction of the molecule is considered unreliable. In order to reduce the risk of late-stage attrition, we excluded active components outside the 99% confidence interval of the BBB model and HIA model. In this process, all the active components from the 5 herbs (Jiangbanxia, Chenpi, Fuling, Shanyao, Zhuling) were excluded. In the end, only 97 compounds from 15 herbs were left.
3.3. TOPKAT and druggability screening
We checked whether the 97 active components were in the OPS of four toxicological properties (Ames test, Rodent Carcinogenicity, Aerobic Biodegradability, and oral LD50). It can be seen from Table S1 that the number of candidate active components became 68 after excluding active components that did not meet the OPS. Due to the unsatisfactory results of the active components of Kuandonghua and Ziwan, they should not be further studied. In the process of druggability screening, the program automatically eliminated 2 unqualified active components according to Lipinski’s rule of five and Veber’s rules. Therefore, 66 active components from 13 herbs may become oral drugs.
3.4. Reverse finding target
The results showed that the corresponding targets of active components in Guizhi did not have antiviral or anti-inflammatory effects. Therefore, 64 active components from 12 herbs were retained in the reverse finding target process. The structures of 64 active components are shown in Figure S1. It can be seen from Figure 2 that the horizontal axis and the longitudinal axis represent the pharmacophore models and the active components, respectively. The color gradient trend is red, yellow, green, and blue on the Heat map. The pharmacophore models with high and low Fit Value are represented by red and blue, respectively. Red means that the matching degree is good. Based on these pharmacophore models, we found that the possible targets of the 64 active components in QFPD were Antigen peptide transporter 1 (TAP1), Retinol-binding protein 4 (RBP4), Interleukin 1 receptor antagonist (IL1RN), Centromere-associated protein E (CENPE), Beta-secretase 1 (BACE1), Transthyretin (TTR), Tyrosine-protein kinase Fyn (FYN), Thyroid hormone receptor alpha (THRA), Pulmonary surfactant-associated protein D (SFTPD), Nuclear receptor subfamily 0 group B member 2 (NR0B2), Cellular tumor antigen p53 (TP53), SRSF protein kinase 1 (SRPK1), RAC-alpha serine/threonine-protein kinase (AKT1) and Protein Mdm4 (MDM4). We compared 14 targets regulated by 64 active components in QFPD with the COVID-19 targets recorded in Genecards databases and found the common targets RBP4 (74), IL1RN (8), TTR (75), FYN (76), SFTPD (77), TP53 (78), SRPK1 (79), and AKT1 (80). The information of 8 potential targets is shown in Table 2. FYN, AKT1, SFTPD, SRPK1, and TP53 were SARS-CoV-2 specific targets.
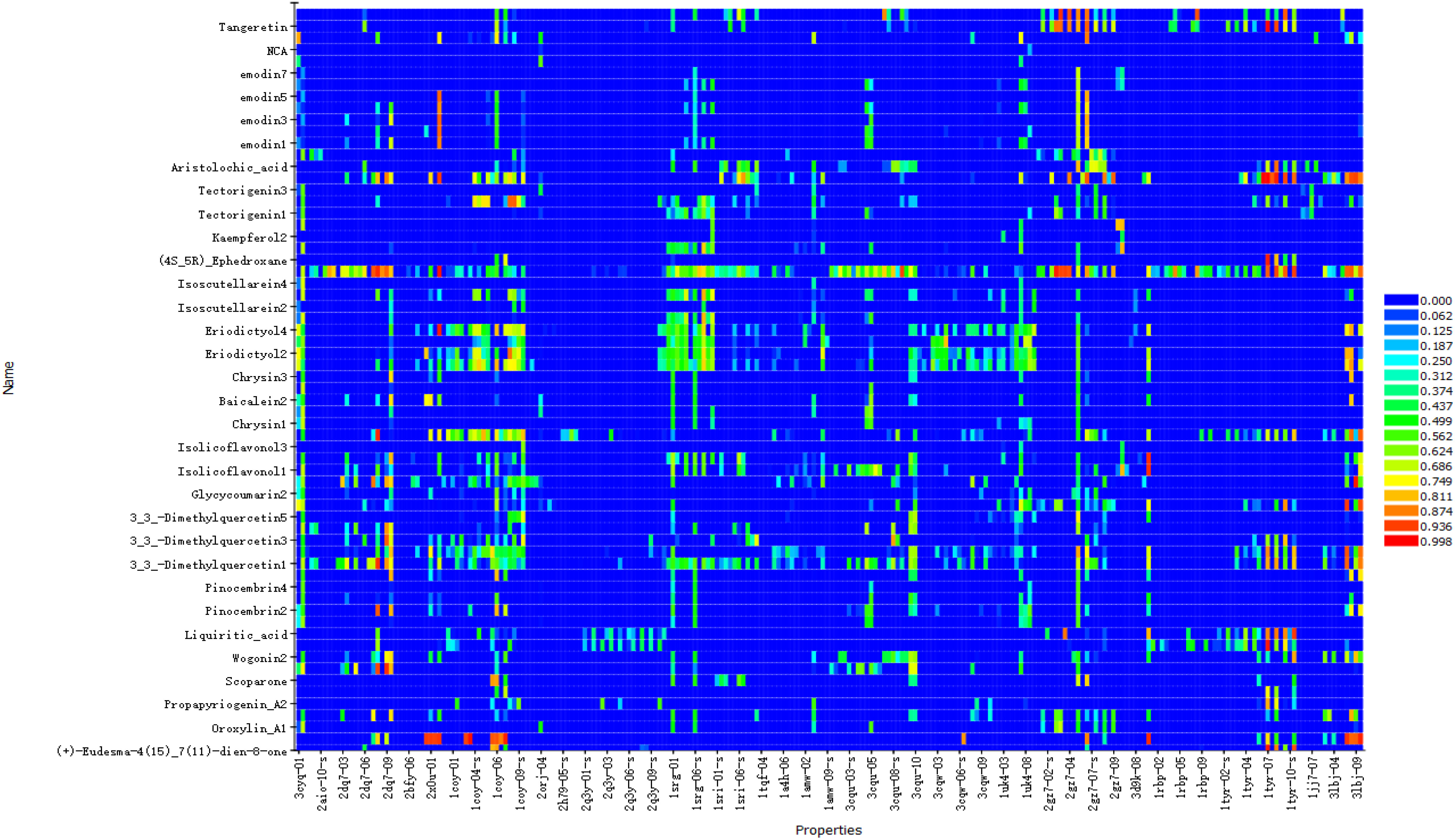
Figure 2 Reverse finding target results of QFPD. The horizontal axis represents the pharmacophore models, and the longitudinal axis represents the active components. Due to the limited size of the picture, we only show more promising pharmacophore models and active components.
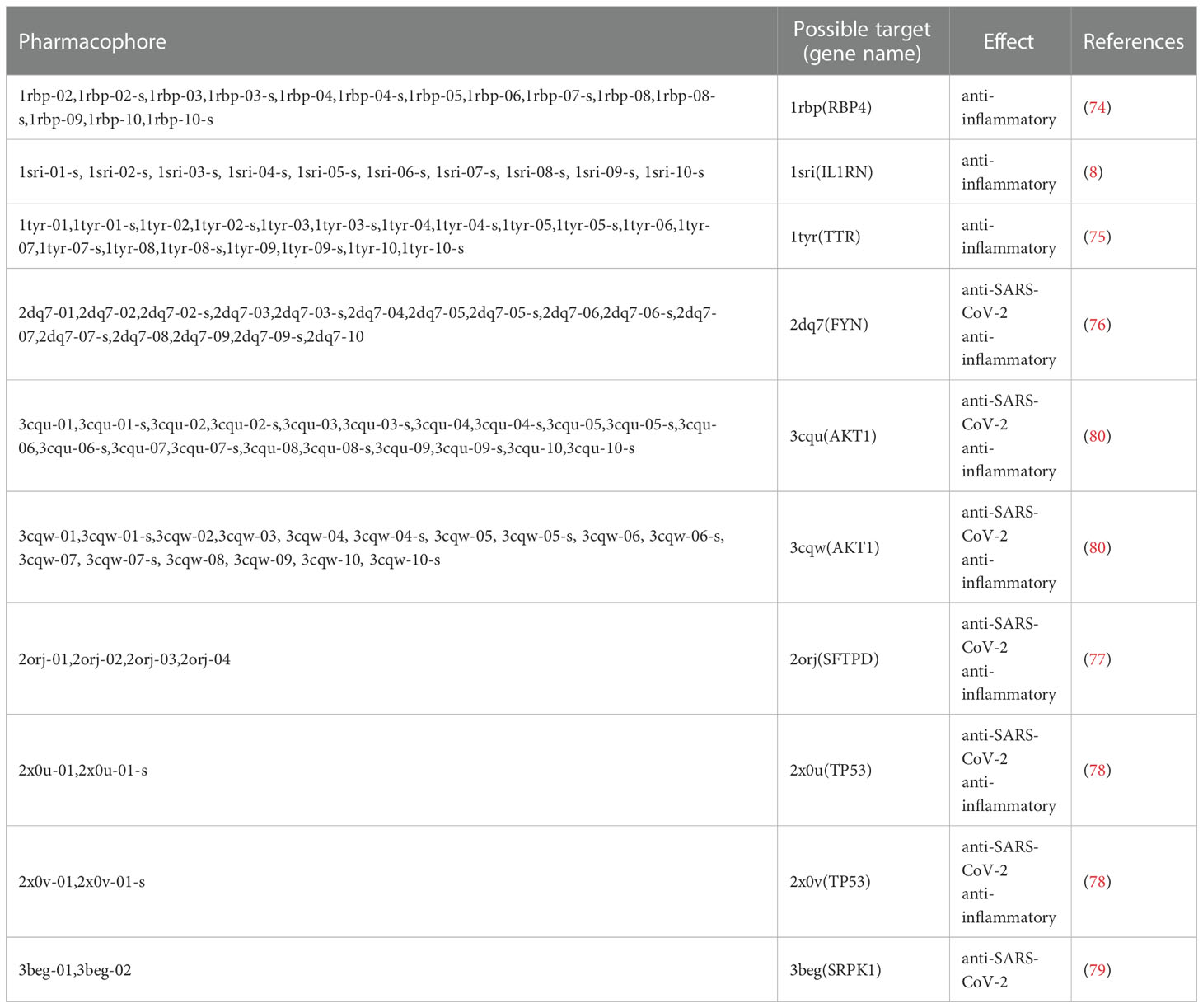
Table 2 All pharmacophores and their possible corresponding targets obtained by reverse finding target.
3.5. Construction of protein-protein interaction network
PPI network was constructed by String with the potential targets of the 62 active components in QFPD. It can be seen from Figure 3A that the network contained 8 nodes and 6 edges. The nodes represented the targets, and the edges represented the interactions between the targets. The more edges the node had, the more critical it was in the network. TP53 and AKT1, with a high degree of connectivity, were core genes that may play an essential role in treating COVID-19 with QFPD. The network in Figure 3B contained 5 nodes and 5 edges. If medium confidence≧0.4 was selected as the screening criteria, the candidate targets were TP53, AKT1, FYN, and SRPK1. If the highest confidence≧0.9 was selected as the screening criteria, the candidate targets were TP53, AKT1. The network in Figure 3C contained 7 nodes and 4 edges. If medium confidence≧0.4 was selected as the screening criteria, the candidate targets were TP53, AKT1, RBP4, TTR, and FYN. If the highest confidence≧0.9 was selected as the screening criteria, the candidate targets were TP53, AKT1, RBP4, and TTR.
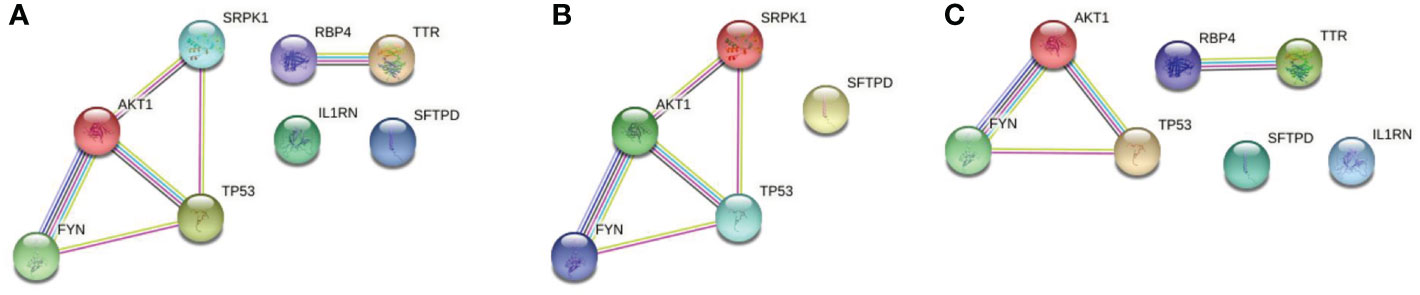
Figure 3 (A) PPI network of 8 potential targets. (B) PPI network of the SARS-CoV-2 specific targets. (C) PPI network of the inflammatory targets. Associations are meant to be specific and meaningful, i.e. proteins jointly contribute to a shared function; this does not necessarily mean they are physically binding to each other. The light-blue edges denote known interactions from curated databases. The pink edges show that the known interactions were determined by experimental methods. The yellow edges demonstrate that the predicted interactions arose from text-mining. The black edges denote that the predicted interactions arose from co-expression. The lavender edges show that the predicted interactions arose from protein homology. The dark-blue edges denote that the predicted interactions arose from gene co-occurrence.
3.6. Construction of “herb-active component-target” interaction network diagram
As shown in Figure 4, 62 active components from 12 herbs (Mahuang, Zhishi, Huoxiang, Zexie, Shegan, Shengjiang, Chaihu, Huangqin, Xingren, Baizhu, Xixin, and Zhigancao) have anti-SARS-CoV-2 or anti-inflammatory effects. Among them, Eriodictyol was common in Xixin and Huangqin. Wogonin was common in Chaihu and Huangqin. Geraniol was common in Chaihu and Shengjiang. (+)-Eudesma-4(15),7(11)-dien-8-one was common in Xixin and Baizhu. It can be seen from Table 3 that, Kaempferol2, Kaempferol3, Emodin7, and Isolicoflavonol3 only had anti-SARS-CoV-2 effects, (4S_5R) Ephedroxane and Pulegone only had anti-inflammatory effects. The other 56 components were both anti-inflammatory and anti-SARS-CoV-2. Among these components, Quercetin, Wogonin, and Emodin were able to interfere with various stages of the coronavirus entry and replication cycle (59, 81, 82). Kaempferol could be used as an antiviral drug against the 3a Channel Protein of Coronavirus (55). Baicalein had a high affinity for SARS-CoV-2 3CLpro (83). They were identified as candidate active components for COVID-19. In addition, each target was bound to two or more active components, indicating that these targets could be affected by multiple active components simultaneously to exert different effects.
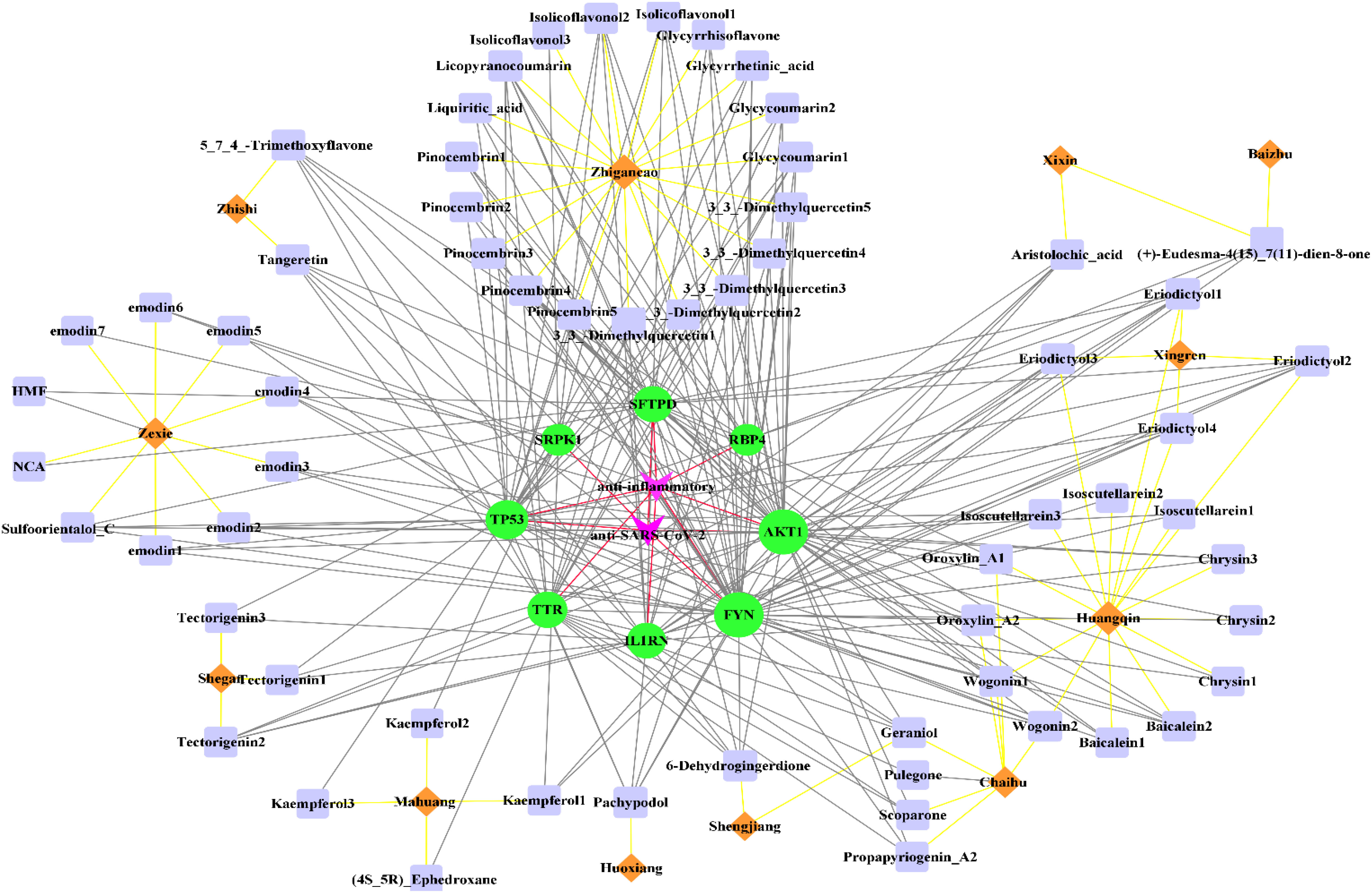
Figure 4 The “herb-active component-target” interaction network diagram for the treatment of COVID-19. 12 herbs (Mahuang, Zhishi, Huoxiang, Zexie, Shegan, Shengjiang, Chaihu, Huangqin, Xingren, Baizhu, Xixin, Zhigancao) in QFPD are marked in orange; 62 active components screened from 12 herbs with anti-SARS-CoV-2 or anti-inflammatory effects are marked in purple; 8 potential targets of QFPD are marked in green, and the properties of the targets are marked in pink. The black line represents that a certain active component comes from a certain herb; the yellow line represents the interaction between the active component and the target, and the red line represents a certain target is against inflammation or SARS-CoV-2.
3.7. Pathway analysis of QFPD
We identified TP53 and AKT1 as core targets based on the PPI network. Therefore, according to the expression of TP53 or AKT1, we divided them into high and low expression groups for GSEA analysis. P-value<0.05 was considered statistically significant. Figure 5A showed that 6 significant pathways were enriched in the TP53 high expression group: RNA polymerase, primary immunodeficiency, intestinal immune network for IGA production, systemic lupus erythematosus, allograft rejection, and autoimmune thyroid disease. 3 pathways were enriched in the TP53 low expression group: O glycan biosynthesis, regulation of autophagy, and long-term potentiation. 20 meaningful pathways were enriched in the AKT1 high expression group. We showed the first six significant enrichments in Figure 5B, which were taste transduction, ECM receptor interaction, focal adhesion, ABC transporters, long term depression, and linoleic acid metabolism. 3 pathways were enriched in the AKT1 low expression group, namely other glycan degradation, glycosylphosphatidylinositol GPI anchor biosynthesis, peroxisome, and hematopoietic cell lineage.
It can be seen from Table 4 that 8 potential targets of QFPD (RBP4, IL1RN, TTR, FYN, SFTPD, TP53, SRPK1, and AKT1) were mainly enriched in 21 BP entries. 17 BP entries were determined with P-value<0.05 (Figure 6). The core BP entries mainly included the viral process, intracellular signal transduction, innate immune response, protein phosphorylation, and cell differentiation. During SARS-CoV-2 infection, the innate immune system experienced substantial disturbance (84). Several of the cytokines involved in the reaction employ a distinct intracellular signaling pathway mediated by Janus kinases (85). Researchers studied the perturbation in protein phosphorylation during SARS-CoV-2 infection by mass spectrometry, and the results showed that large changes were observed in protein phosphorylation (86). This evidences verified that QFPD regulates diseases through a variety of biological processes. The core MF entries mainly included protein binding, identical protein binding, enzyme binding, and ATP binding. A recent study reported that SARS-CoV-2 enters the host cells through the binding of its spike protein to the cell surface-expressing angiotensin-converting enzyme 2 (ACE2) (87). Therefore, inhibiting the binding of some specific proteins or enzymes may attenuate the progression of COVID-19. The core CC entries mainly included protein complex and extracellular space. The GO analysis results showed that AKT1, FYN, TP53, TTR, and RBP4 were key targets involved in regulation. The KEGG analysis results showed that AKT1, FYN, and TP53 were key targets involved in regulation (Table 5). The top 13 KEGG pathways are shown in Figure 7. The core pathways mainly included Sphingolipid signaling pathway, Fc epsilon RI signaling pathway, Apoptosis, and Measles. Sphingolipids play a vital role in protecting the lung from damages (88). The Fc epsilon RI signaling pathway is associated with cytokine secretion and inflammatory responses (89). Based on previous data, SARS-CoV-2 may have the ability to induce endogenous and exogenous apoptotic pathways and stimulate T cell apoptosis (90). Therefore, we speculate that the active components in QFPD may play an important role in the therapeutic of COVID-19 by multiple pathways.
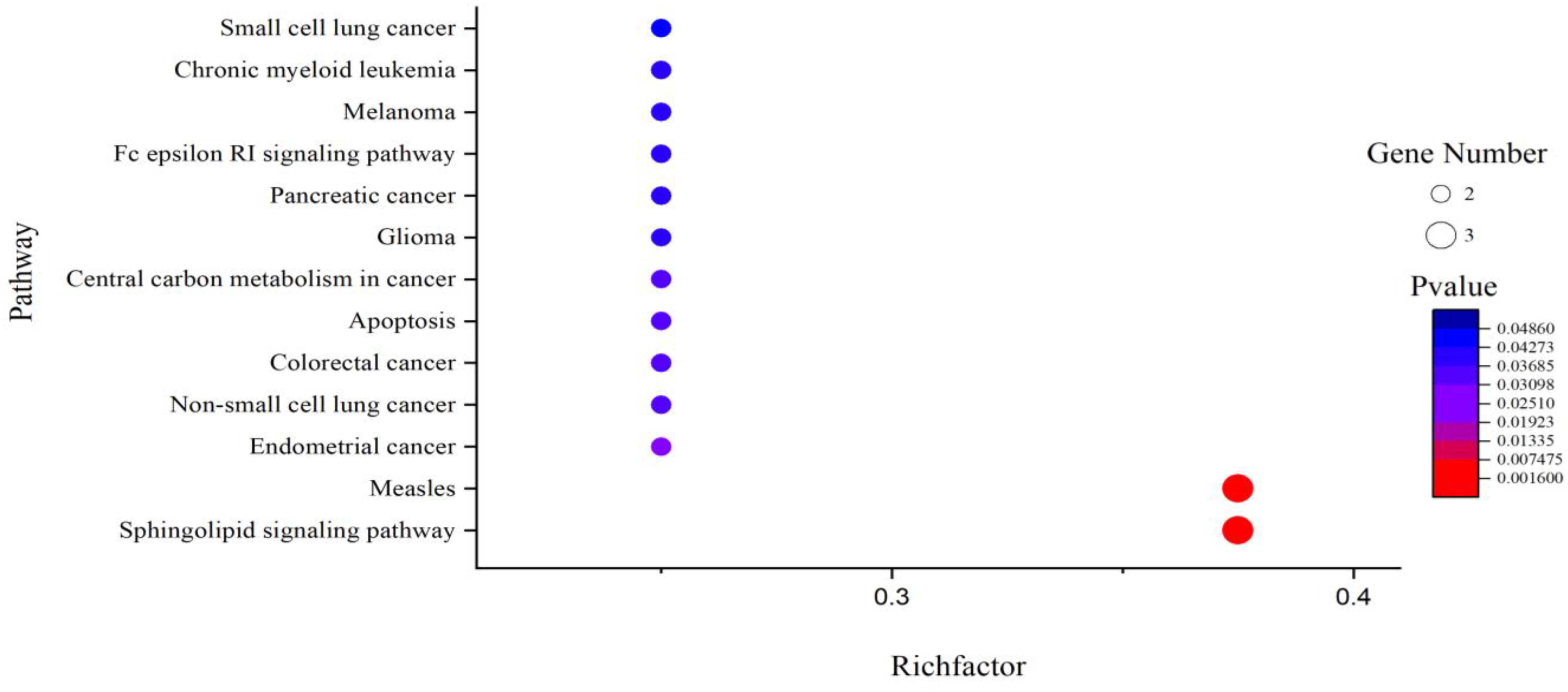
Figure 7 KEGG pathway analysis for the SARS-CoV-2 specific targets of active components in QFPD. The rich factor indicates the ratio of the number of target genes belonging to a pathway to the total number of annotated target genes in the pathway. The higher value for this ratio corresponds to a higher level of enrichment. The size and color of the bubble indicates the number and significant characters of target genes that enriched in pathways.
Conclusion
In this study, we used various network pharmacology methods combined with CADD techniques to reveal the diversity of potential targets and therapeutic pathways for QFPD against COVID-19. We found that RBP4, IL1RN, TTR, FYN, SFTPD, TP53, SRPK1, and AKT1 are highly related to COVID-19. QFPD could act on multiple pathways, including viral process, immunodeficiency, RNA polymerase, Sphingolipid signaling pathway, and taste transduction. The results showed that QFPD has “multi-component, multi-target, and multi-pathway” characteristics in regulating inflammation, viral infection, cellular damage, and immune responses. Our work helps to establish the basic theory of TCM for the treatment of COVID-19.
Data availability statement
The original contributions presented in the study are included in the article/Supplementary Material. Further inquiries can be directed to the corresponding author.
Author contributions
ZW conducted the literature review, designed the research system, collated data information, and wrote the manuscript. JZ contributed to the manuscript's revision and guided the calculation procee. HG contributed to the conception and design of the study. All authors participate in the revision, reading, and approval the submitted version.
Funding
This work was financially supported by the High-end Talent Team Construction Foundation [Grant No. 108-10000318], the Cooperation Project of University and Local Enterprise in Yantai of Shandong Province (2021XDRHXMXK23).
Conflict of interest
The authors declare that the research was conducted in the absence of any commercial or financial relationships that could be construed as a potential conflict of interest.
Publisher’s note
All claims expressed in this article are solely those of the authors and do not necessarily represent those of their affiliated organizations, or those of the publisher, the editors and the reviewers. Any product that may be evaluated in this article, or claim that may be made by its manufacturer, is not guaranteed or endorsed by the publisher.
Supplementary material
The Supplementary Material for this article can be found online at: https://www.frontiersin.org/articles/10.3389/fimmu.2022.1015271/full#supplementary-material
References
1. Pinotti F, Di Domenico L, Ortega E, Mancastroppa M, Pullano G, Valdano E, et al. Tracing and analysis of 288 early SARS-CoV-2 infections outside China: A modeling study. PloS Med (2020) 17:1003193–1003193. doi: 10.1371/journal.pmed.1003193
2. Larremore DB, Toomre D, Parker R. Modeling the effectiveness of olfactory testing to limit SARS-CoV-2 transmission. Nat Commun (2021) 12:3664–4. doi: 10.1038/s41467-021-23315-5
3. Lowery SA, Sariol A, Perlman S. Innate immune and inflammatory responses to SARS-CoV-2: Implications for COVID-19. Cell Host Microbe (2021) 29:1052–62. doi: 10.1016/j.chom.2021.05.004
4. Amat-Santos IJ, Santos-Martinez S, López-Otero D, Nombela-Franco L, Gutiérrez-Ibanes E, Del Valle R, et al. Ramipril in high-risk patients with COVID-19. J Am Coll Cardiol (2020) 76:268–76. doi: 10.1016/j.jacc.2020.05.040
5. Li Y, Liu X, Guo L, Li J, Zhong D, Zhang Y, et al. Traditional Chinese herbal medicine for treating novel coronavirus (COVID-19) pneumonia: protocol for a systematic review and meta-analysis. Systematic Rev (2020) 9:75–5. doi: 10.1186/s13643-020-01343-4
6. Constantinos G, Anna H, Dimitrios K, Taxiarchis P, Vasiliki T. Status of traditional Chinese medicine in Greece and its approach on COVID-19 pandemic. Chin Med Culture (2021) 4:78–85. doi: 10.4103/CMAC.CMAC_16_21
7. Li M, Yang Y, Liu Y, Zheng M, Li J, Chen L, et al. Progress of traditional Chinese medicine treating COVID-19. World J Traditional Chin Med (2021) 7:167–83. doi: 10.4103/wjtcm.wjtcm_68_20
8. Fricke-Galindo I, Falfan-Valencia R. Genetics insight for COVID-19 susceptibility and severity: a review. Front Immunol (2021) 12:1057–8. doi: 10.3389/fimmu.2021.622176
9. Huan−Huan T, Qi L, Pan−Hong J, Xiang−Dong Z. Clinical value of related inflammatory factors in COVID⁃19. J Hainan Med Univ (2021) 27:647–50. doi: 10.13210/j.cnki.jhmu.20210329.001
10. Vannucchi AM, Sordi B, Morettini A, Nozzoli C, Poggesi L, Pieralli F, et al. Compassionate use of JAK1/2 inhibitor ruxolitinib for severe COVID-19: a prospective observational study. Leukemia (2021) 35:1121–33. doi: 10.1038/s41375-020-01018-y
11. Wang Z, Zhang J, Zhan J, Gao H. Screening out anti-inflammatory or anti-viral targets in xuanfei baidu tang through a new technique of reverse finding target. Bioorganic Chem (2021) 116:105274–4. doi: 10.1016/j.bioorg.2021.105274
12. Shi N, Liu B, Liang N, Ma Y, Ge Y, Yi H, et al. Association between early treatment with qingfei paidu decoction and favorable clinical outcomes in patients with COVID-19: a retrospective multicenter cohort study. Pharmacol Res (2020) 161:105290–0. doi: 10.1016/j.phrs.2020.105290
13. Xin S, Cheng X, Zhu B, Liao X, Yang F, Song L, et al. Clinical retrospective study on the efficacy of qingfei paidu decoction combined with Western medicine for COVID-19 treatment. Biomed Pharmacother (2020) 129:110500–0. doi: 10.1016/j.biopha.2020.110500
14. Ren W, Ma Y, Wang R, Liang P, Sun Q, Pu Q, et al. Research advance on qingfei paidu decoction in prescription principle, mechanism analysis and clinical application. Front Pharmacol (2021) 11:2046–6. doi: 10.3389/fphar.2020.589714
15. Gao K, Song YP, Chen H, Zhao LT, Ma L. Therapeutic efficacy of qingfei paidu decoction combined with antiviral drugs in the treatment of corona virus disease 2019: A protocol for systematic review and meta analysis. Medicine (2020) 99:20489–9. doi: 10.1097/MD.0000000000020489
16. Junhua M, Yang H, Qian C, Qiang G, Yonggang C, Jing A. A retrospective study on the treatment of COVID-19 type common/type severe with qingfei paidu decoction. Chin J Hosp Pharm (2020) 40:2152–7. doi: 10.3969/j.issn.2097-0005.2021.12.009
17. Silva C.H.T.D.P.D., Silva V, Resende J, Rodrigues PF, Bononi FC, Benevenuto CG, et al. Computer-aided drug design and ADMET predictions for identification and evaluation of novel potential farnesyltransferase inhibitors in cancer therapy. J Mol Graphics Model (2010) 28:513–23. doi: 10.1016/j.jmgm.2009.11.011
18. Zhang A, Hui S, Yang B, Wang X. Predicting new molecular targets for rhein using network pharmacology. BMC Syst Biol (2012) 6:20–0. doi: 10.1186/1752-0509-6-20
19. Shao L, Zhang B. Traditional Chinese medicine network pharmacology: theory, methodology and application. Chin J Natural Medicines (2013) 11:110–20. doi: 10.1016/S1875-5364(13)60037-0
20. Chang-Xiao L, Liu R, Fan H-R, Ng X-F, Xiao X-P, Chen. Network pharmacology bridges traditional application and modern development of traditional Chinese medicine. Chin Herbal Medicines (2015) 7:3–17. doi: 10.1016/S1674-6384(15)60014-4
21. Yang Y, Ding Z, Wang Y, Zhong R, Feng Y, Xia T, et al. Systems pharmacology reveals the mechanism of activity of physalis alkekengi l. var. franchetii against lipopolysaccharide-induced acute lung injury. J Cell Mol Med (2020) 24:5039–56. doi: 10.1111/jcmm.15126
22. Liu Y, Sun Y. China Traditional Chinese medicine (TCM) patent database. World Patent Inf (2004) 26:91–6. doi: 10.1016/S0172-2190(03)00110-8
23. Hsin-Yi C. Discovery of novel insomnia leads from screening traditional Chinese medicine database. J Biomolecular Structure Dynamics (2013) 32:776–91. doi: 10.1080/07391102.2013.790849
24. Arya H, Coumar MS. Virtual screening of traditional Chinese medicine (TCM) database: identification of fragment-like lead molecules for filariasis target asparaginyl-tRNA synthetase. J Mol Modeling (2014) 20:2266–7. doi: 10.1007/s00894-014-2266-9
25. Ru J, Li P, Wang J, Zhou W, Li B, Huang C, et al. TCMSP: a database of systems pharmacology for drug discovery from herbal medicines. J Cheminform (2014) 6:13–4. doi: 10.1186/1758-2946-6-13
26. Ma LY, Zhou QL, Yang XB, Wang HP, Yang XW. Metabolism of 20(S)-ginsenoside Rg2 by rat liver microsomes: Bioactivation to SIRT1-activating metabolites. Molecules (2016) 21:757–8. doi: 10.3390/molecules21060757
27. Tailong L, Youyong Li, Yunlong S, Dan Li, Huiyong, Sun. ADMET evaluation in drug discovery: 15. accurate prediction of rat oral acute toxicity using relevance vector machine and consensus modeling. J Cheminformatics (2016) 8:6–8. doi: 10.1186/s13321-016-0117-7
28. Qidwai T. QSAR modeling, docking and ADMET studies for exploration of potential anti-malarial compounds against plasmodium falciparum. In Silico Pharmacol (2017) 5:6–7. doi: 10.1007/s40203-017-0026-0
29. Alam S, Khan F. Virtual screening, docking, ADMET and system pharmacology studies on garcinia caged xanthone derivatives for anticancer activity. Sci Rep (2018) 8:5524–5. doi: 10.1038/s41598-018-23768-7
30. Ruiz P, Begluitti G, Tincher T, Wheeler J, Mumtaz M. Prediction of acute mammalian toxicity using QSAR methods: a case study of sulfur mustard and its breakdown products. Molecules (Basel Switzerland) (2012) 17:8982–9001. doi: 10.3390/molecules17088982
31. Bahadur Gurung A, Ajmal Ali M, Lee J, Abul Farah M, Mashay Al-Anazi K. Structure-based virtual screening of phytochemicals and repurposing of FDA approved antiviral drugs unravels lead molecules as potential inhibitors of coronavirus 3C-like protease enzyme. J King Saud University. Sci (2020) 32:2845–53. doi: 10.1016/j.jksus.2020.07.007
32. Alam S, Khan F. 3D-QSAR, docking, ADME/Tox studies on flavone analogs reveal anticancer activity through tankyrase inhibition. Sci Rep (2019) 9:5414–4. doi: 10.1038/s41598-019-41984-7
33. Zhou Y, Lu X, Yang H, Chen Y, Wang F, Li J, et al. Discovery of selective butyrylcholinesterase (BChE) inhibitors through a combination of computational studies and biological evaluations. Molecules (Basel Switzerland) (2019) 24:4217–7. doi: 10.3390/molecules24234217
34. Damian S, Andrea F, Stefan W, Kristoffer F, Davide H, Jaime HC, et al. STRING v10: protein–protein interaction networks, integrated over the tree of life. Nucleic Acids Res (2015) 43:447–52. doi: 10.1093/nar/gku1003
35. Grindrod P, Kibble M. Review of uses of network and graph theory concepts within proteomics. Expert Rev Proteomics (2004) 1:229–38. doi: 10.1586/14789450.1.2.229
36. Kanehisa M, Goto S, Sato Y, Furumichi M, Tanabe M. KEGG for integration and interpretation of large-scale molecular data sets. Nucleic Acids Res (2012) 40:D109–14. doi: 10.1093/nar/gkr988
37. Shen Y-C, Chiou W-F, Chou Y-C, Chen C-F. Mechanisms in mediating the anti-inflammatory effects of baicalin and baicalein in human leukocytes. Eur J Pharmacol (2003) 465:171–81. doi: 10.1016/S0014-2999(03)01378-5
38. Lee JY, Park W. Anti-inflammatory effects of oroxylin a on RAW 264.7 mouse macrophages induced with polyinosinic-polycytidylic acid. Exp Ther Med (2016) 12:151–6. doi: 10.3892/etm.2016.3320
39. Paniagua-Pérez R, Flores-Mondragón G, Reyes-Legorreta C, Herrera-López B, Cervantes-Hernández I, Madrigal-Santillán O, et al. Evaluation of the anti-inflammatory capacity of beta-sitosterol in rodent assays. Afr J Traditional Complementary Altern Medicines (2017) 14:123–30. doi: 10.21010/ajtcam.v14i1.13
40. Kroes BV, Van Den Berg A, Van Ufford HQ, Van Dijk H, Labadie R. Anti-inflammatory activity of gallic acid. Planta Med (1992) 58:499–504. doi: 10.1055/s-2006-961535
41. Selloum L, Bouriche H, Tigrine C, Boudoukha C. Anti-inflammatory effect of rutin on rat paw oedema, and on neutrophils chemotaxis and degranulation. Exp Toxicologic Pathol (2003) 54:313–8. doi: 10.1078/0940-2993-00260
42. Lee S, Jung SH, Lee YS, Yamada M, Kim B-K, Ohuchi K, et al. Antiinflammatory activity of hyperin fromAcanthopanax chiisanensis roots. Arch pharmacal Res (2004) 27:628–32. doi: 10.1007/BF02980162
43. Pan C-H, Kim ES, Jung SH, Nho CW, Lee JK. Tectorigenin inhibits IFN-γ/LPS-induced inflammatory responses in murine macrophage RAW 264.7 cells. Arch pharmacal Res (2008) 31:1447–56. doi: 10.1007/s12272-001-2129-7
44. Saha S, Sadhukhan P, Sil PC. Mangiferin: A xanthonoid with multipotent anti-inflammatory potential. Biofactors (2016) 42:459–74. doi: 10.1002/biof.1292
45. Hart P, Brand C, Carson C, Riley T, Prager R, Finlay-Jones J. Terpinen-4-ol, the main component of the essential oil of melaleuca alternifolia (tea tree oil), suppresses inflammatory mediator production by activated human monocytes. Inflammation Res (2000) 49:619–26. doi: 10.1007/s000110050639
46. Cosyns J-P. Aristolochic acid and ‘Chinese herbs nephropathy’. Drug Saf (2003) 26:33–48. doi: 10.2165/00002018-200326010-00004
47. Fanhchaksai K, Kodchakorn K, Pothacharoen P, Kongtawelert P. Effect of sesamin against cytokine production from influenza type a H1N1-induced peripheral blood mononuclear cells: Computational and experimental studies. In Vitro Cell Dev Biology-Animal (2016) 52:107–19. doi: 10.1007/s11626-015-9950-7
48. Xu J-J, Liu Z, Tang W, Wang G-C, Chung HY, Liu Q-Y, et al. Tangeretin from citrus reticulate inhibits respiratory syncytial virus replication and associated inflammation in vivo. J Agric Food Chem (2015) 63:9520–7. doi: 10.1021/acs.jafc.5b03482
49. Haggag YA, El-Ashmawy NE, Okasha KM. Is hesperidin essential for prophylaxis and treatment of COVID-19 infection? Med Hypotheses (2020) 144:109957–8. doi: 10.1016/j.mehy.2020.109957
50. Tutunchi H, Naeini F, Ostadrahimi A, Hosseinzadeh-Attar MJ. Naringenin, a flavanone with antiviral and anti-inflammatory effects: A promising treatment strategy against COVID-19. Phytotherapy Res (2020) 34:3137–47. doi: 10.1002/ptr.6781
51. Chiang LC, Ng LT, Cheng PW, Chiang W, Lin CC. Antiviral activities of extracts and selected pure constituents of ocimum basilicum. Clin Exp Pharmacol Physiol (2005) 32:811–6. doi: 10.1111/j.1440-1681.2005.04270.x
52. Bhattacharjee B, Chatterjee J. Identification of proapoptopic, anti-inflammatory, anti-proliferative, anti-invasive and anti-angiogenic targets of essential oils in cardamom by dual reverse virtual screening and binding pose analysis. Asian Pacific J Cancer Prev (2013) 14:3735–42. doi: 10.7314/APJCP.2013.14.6.3735
53. Carballo-Villalobos A, González-Trujano M, López-Muñoz F. Evidence of mechanism of action of anti-inflammatory/antinociceptive activities of acacetin. Eur J Pain (2014) 18:396–405. doi: 10.1002/j.1532-2149.2013.00378.x
54. Rogerio A, Kanashiro A, Fontanari C, Da Silva E, Lucisano-Valim Y, Soares E, et al. Anti-inflammatory activity of quercetin and isoquercitrin in experimental murine allergic asthma. Inflammation Res (2007) 56:402–8. doi: 10.1007/s00011-007-7005-6
55. Schwarz S, Sauter D, Wang K, Zhang R, Sun B, Karioti A, et al. Kaempferol derivatives as antiviral drugs against the 3a channel protein of coronavirus. Planta Med (2014) 80:177–82. doi: 10.1055/s-0033-1360277
56. Murbach Teles Andrade BF, Conti BJ, Santiago KB, Fernandes A, Sforcin JM. C ymbopogon martinii essential oil and geraniol at noncytotoxic concentrations exerted immunomodulatory/anti-inflammatory effects in human monocytes. J Pharm Pharmacol (2014) 66:1491–6. doi: 10.1111/jphp.12278
57. Lee JK. Anti-inflammatory effects of eriodictyol in lipopolysaccharidestimulated raw 264.7 murine macrophages. Arch Pharmacal Res (2011) 34:671–9. doi: 10.1007/s12272-011-0418-3
58. Freire RS, Morais SM, Catunda-Junior FEA, Pinheiro DC. Synthesis and antioxidant, anti-inflammatory and gastroprotector activities of anethole and related compounds. Bioorganic medicinal Chem (2005) 13:4353–8. doi: 10.1016/j.bmc.2005.03.058
59. Agrawal PK, Agrawal C, Blunden G. Quercetin: antiviral significance and possible COVID-19 integrative considerations. Natural Product Commun (2020) 15:1–10. doi: 10.1177/1934578X20976293
60. Sin KS, Kim HP, Lee WC, Pachaly P. Pharmacological activities of the constituents of atractylodes rhizomes. Arch Pharmacal Res (1989) 12:236–8. doi: 10.1007/BF02911051
61. Huang H, Cheng Z, Shi H, Xin W, Wang TT, Yu L. Isolation and characterization of two flavonoids, engeletin and astilbin, from the leaves of engelhardia roxburghiana and their potential anti-inflammatory properties. J Agric Food Chem (2011) 59:4562–9. doi: 10.1021/jf2002969
62. Lee CW, Park NH, Kim JW, Um BH, Shpatov A, Shults E, et al. Study of skin anti-ageing and anti-inflammatory effects of dihydroquercetin, natural triterpenoinds, and their synthetic derivatives. Russian J Bioorganic Chem (2012) 38:328–34. doi: 10.1134/S1068162012030028
63. Hwa JS, Jin YC, Lee YS, Ko YS, Kim YM, Shi LY, et al. 2-methoxycinnamaldehyde from cinnamomum cassia reduces rat myocardial ischemia and reperfusion injury in vivo due to HO-1 induction. J ethnopharmacol (2012) 139:605–15. doi: 10.1016/j.jep.2011.12.001
64. Kim D-H, Yoo T-H, Lee SH, Kang HY, Nam BY, Kwak SJ, et al. Gamma linolenic acid exerts anti-inflammatory and anti-fibrotic effects in diabetic nephropathy. Yonsei Med J (2012) 53:1165–75. doi: 10.3349/ymj.2012.53.6.1165
65. Pan P-H, Lin S-Y, Ou Y-C, Chen W-Y, Chuang Y-H, Yen Y-J, et al. Stearic acid attenuates cholestasis-induced liver injury. Biochem Biophys Res Commun (2010) 391:1537–42. doi: 10.1016/j.bbrc.2009.12.119
66. Xia S, Ni Y, Zhou Q, Xiang H, Sui H, Shang D. Emodin attenuates severe acute pancreatitis via antioxidant and anti-inflammatory activity. Inflammation (2019) 42:2129–38. doi: 10.1007/s10753-019-01077-z
67. Shang X, He X, Liu H, Wen B, Tan T, Xu C, et al. Stachyose prevents intestinal mucosal injury in the immunosuppressed mice. Starch-Stärke (2020) 72:1900073–1900074. doi: 10.1002/star.201900073
68. Zhou Y-Z, Li X, Gong W-X, Tian J-S, Gao X-X, Gao L, et al. Protective effect of isoliquiritin against corticosterone-induced neurotoxicity in PC12 cells. Food Funct (2017) 8:1235–44. doi: 10.1039/C6FO01503D
69. Abdizadeh R, Hadizadeh F, Abdizadeh T. In silico analysis and identification of antiviral coumarin derivatives against 3-chymotrypsin-like main protease of the novel coronavirus SARS-CoV-2. Mol Diversity (2021) 16:1053–76. doi: 10.1007/s11030-021-10230-6
70. Richard SA. Exploring the pivotal immunomodulatory and anti-inflammatory potentials of glycyrrhizic and glycyrrhetinic acids. Mediators Inflammation (2021) 2021:1–15. doi: 10.1155/2021/6699560
71. Lee JY, Park W. Anti-inflammatory effect of wogonin on RAW 264.7 mouse macrophages induced with polyinosinic-polycytidylic acid. Molecules (2015) 20:6888–900. doi: 10.3390/molecules20046888
72. Roy A, Park H-J, Abdul QA, Jung HA, Choi JS. Pulegone exhibits anti-inflammatory activities through the regulation of NF-κB and nrf-2 signaling pathways in LPS-stimulated RAW 264.7 cells. Natural Product Sci (2018) 24:28–35. doi: 10.20307/nps.2018.24.1.28
73. Hui Y, Wang X, Yu Z, Fan X, Cui B, Zhao T, et al. Scoparone as a therapeutic drug in liver diseases: Pharmacology, pharmacokinetics and molecular mechanisms of action. Pharmacol Res (2020) 160:105170–2. doi: 10.1016/j.phrs.2020.105170
74. Leng L, Li M, Li W, Mou D, Liu G, Ma J, et al. Sera proteomic features of active and recovered COVID-19 patients: potential diagnostic and prognostic biomarkers. Signal transduction targeted Ther (2021) 6:1–3. doi: 10.1038/s41392-021-00612-5
75. Tahery N, Khodadost M, Sherafat SJ, Tavirani MR, Ahmadi N, Montazer F, et al. C-reactive protein as a possible marker for severity and mortality of COVID-19 infection. Gastroenterol Hepatol From Bed to Bench (2021) 14:S118–22.
76. Vavougios GD, Breza M, Mavridis T, Krogfelt KA. FYN, SARS-CoV-2, and IFITM3 in the neurobiology of alzheimer's disease. Brain Disord (2021) 3:100022–3. doi: 10.1016/j.dscb.2021.100022
77. Chen L, Zheng S. Understand variability of COVID-19 through population and tissue variations in expression of SARS-CoV-2 host genes. Inf Med unlocked (2020) 21:100443–3. doi: 10.1016/j.imu.2020.100443
78. Harford JB, Kim SS, Pirollo KF, Chang EH. TP53 gene therapy as a potential treatment for patients with COVID-19. Viruses (2022) 14:739–40. doi: 10.3390/v14040739
79. Savastano A, Ibáñez De Opakua A, Rankovic M, Zweckstetter M. Nucleocapsid protein of SARS-CoV-2 phase separates into RNA-rich polymerase-containing condensates. Nat Commun (2020) 11:1–10. doi: 10.1038/s41467-020-19843-1
80. Xia QD, Xun Y, Lu JL, Lu YC, Yang YY, Zhou P, et al. Network pharmacology and molecular docking analyses on lianhua qingwen capsule indicate Akt1 is a potential target to treat and prevent COVID-19. Cell proliferation (2020) 53:12949–9. doi: 10.1111/cpr.12949
81. Shah T, Shah Z, Xia K-Y, Baloch Z. Therapeutic mechanisms and impact of traditional Chinese medicine on COVID-19 and other influenza diseases. Pharmacol Research-Modern Chin Med (2021) 2:100029–9. doi: 10.1016/j.prmcm.2021.100029
82. Zhang X, Gao R, Zhou Z, Tang X, Lin J, Wang L, et al. A network pharmacology based approach for predicting active ingredients and potential mechanism of lianhuaqingwen capsule in treating COVID-19. Int J Med Sci (2021) 18:1866–7. doi: 10.7150/ijms.53685
83. Tao Q, Du J, Li X, Zeng J, Tan B, Xu J, et al. Network pharmacology and molecular docking analysis on molecular targets and mechanisms of huashi baidu formula in the treatment of COVID-19. Drug Dev Ind Pharm (2020) 46:1345–53. doi: 10.1080/03639045.2020.1788070
84. Kuri-Cervantes L, Pampena MB, Meng W, Rosenfeld AM, Ittner CA, Weisman AR, et al. Comprehensive mapping of immune perturbations associated with severe COVID-19. Sci Immunol (2020) 5:eabd7114. doi: 10.1126/sciimmunol.abd7114
85. Luo W, Li Y-X, Jiang L-J, Chen Q, Wang T, Ye D-W. Targeting JAK-STAT signaling to control cytokine release syndrome in COVID-19. Trends Pharmacol Sci (2020) 41:531–43. doi: 10.1016/j.tips.2020.06.007
86. Bouhaddou M, Memon D, Meyer B, White KM, Rezelj VV, Marrero MC, et al. The global phosphorylation landscape of SARS-CoV-2 infection. Cell (2020) 182:685–712.e619. doi: 10.1016/j.cell.2020.06.034
87. Kiew L-V, Chang C-Y, Huang S-Y, Wang P-W, Heh C-H, Liu C-T, et al. Development of flexible electrochemical impedance spectroscopy-based biosensing platform for rapid screening of SARS-CoV-2 inhibitors. Biosensors Bioelectronics (2021) 183:113213–3. doi: 10.1016/j.bios.2021.113213
88. Abu-Farha M, Thanaraj TA, Qaddoumi MG, Hashem A, Abubaker J, Al-Mulla F. The role of lipid metabolism in COVID-19 virus infection and as a drug target. Int J Mol Sci (2020) 21:3544–5. doi: 10.3390/ijms21103544
89. Barh D, Aljabali AA, Tambuwala MM, Tiwari S, Serrano-Aroca Á., Alzahrani KJ, et al. Predicting COVID-19–comorbidity pathway crosstalk-based targets and drugs: towards personalized COVID-19 management. Biomedicines (2021) 9:556–8. doi: 10.3390/biomedicines9050556
Keywords: COVID-19, herb, target, active component, anti-inflammatory, anti-SARS-CoV-2
Citation: Wang Z, Zhan J and Gao H (2022) Computer-aided drug design combined network pharmacology to explore anti-SARS-CoV-2 or anti-inflammatory targets and mechanisms of Qingfei Paidu Decoction for COVID-19. Front. Immunol. 13:1015271. doi: 10.3389/fimmu.2022.1015271
Received: 09 August 2022; Accepted: 05 December 2022;
Published: 23 December 2022.
Edited by:
Alfonso J. Rodriguez-Morales, Fundacion Universitaria Autónoma de las Américas, ColombiaReviewed by:
Zhonglei Wang, Qufu Normal University, ChinaTrina Ekawati Tallei, Sam Ratulangi University, Indonesia
Copyright © 2022 Wang, Zhan and Gao. This is an open-access article distributed under the terms of the Creative Commons Attribution License (CC BY). The use, distribution or reproduction in other forums is permitted, provided the original author(s) and the copyright owner(s) are credited and that the original publication in this journal is cited, in accordance with accepted academic practice. No use, distribution or reproduction is permitted which does not comply with these terms.
*Correspondence: Hongwei Gao, gaohongw369@ldu.edu.cn