- 1Department of Pharmacy, Suqian First Hospital, Suqian, China
- 2Department of Oncology, Changzhou Traditional Chinese Medicine Hospital, Changzhou, China
- 3Department of General Surgery, Jiangsu Cancer Hospital, Jiangsu Institute of Cancer Research, The Affiliated Cancer Hospital of Nanjing Medical University, Nanjing, China
- 4Department of Pharmacy, Jiangsu Cancer Hospital, Jiangsu Institute of Cancer Research, The Affiliated Cancer Hospital of Nanjing Medical University, Nanjing, China
Purpose: This study aimed to investigate the value of 18F-fluorodeoxyglucose positron emission tomography/computed tomography (18F-FDG PET/CT) in predicting early immunotherapy response of immune checkpoint inhibitors (ICIs) in patients with advanced or metastatic non-small-cell lung cancer (NSCLC).
Methods: A comprehensive search of PubMed, Web of science, Embase and the Cochrane library was performed to examine the prognostic value of 18F-FDG PET/CT in predicting early immunotherapy response of ICIs in patients with NSCLC. The main outcomes for evaluation were overall survival (OS) and progression-free survival (PFS). Detailed data from each study were extracted and analyzed using STATA 14.0 software.
Results: 13 eligible articles were included in this systematic review. Compared to baseline 18F-FDG PET/CT imaging, the pooled hazard ratios (HR) of maximum and mean standardized uptake values SUVmax, SUVmean, MTV and TLG for OS were 0.88 (95% CI: 0.69-1.12), 0.79 (95% CI: 0.50-1.27), 2.10 (95% CI: 1.57-2.82) and 1.58 (95% CI: 1.03-2.44), respectively. The pooled HR of SUVmax, SUVmean, MTV and TLG for PFS were 1.06 (95% CI: 0.68–1.65), 0.66 (95% CI: 0.48-0.90), 1.50 (95% CI: 1.26-1.79), 1.27 (95% CI: 0.92-1.77), respectively. Subgroup analysis showed that high MTV group had shorter OS than low MTV group in both first line group (HR: 1.97, 95% CI: 1.39-2.79) and undefined line group (HR: 2.11, 95% CI: 1.61-2.77). High MTV group also showed a shorter PFS in first line group (HR: 1.85, 95% CI: 1.28-2.68), and low TLG group had a longer OS in undefined group (HR: 1.37, 95% CI: 1.00-1.86). No significant differences were in other subgroup analysis.
Conclusion: Baseline MTV and TLG may have predictive value and should be prospectively studied in clinical trials. Baseline SUVmax and SUVmean may not be appropriate prognostic markers in advanced or metastatic NSCLC patients treated with ICIs.
Systematic Review Registration: https://www.crd.york.ac.uk/prospero/display_record.php?RecordID=323906, identifier CRD42022323906.
Introduction
Lung cancer is the second most common malignant tumor with the highest mortality rate worldwide, of which non-small-cell lung cancer (NSCLC) accounts for about 85% (1, 2). Due to the relatively insidious symptoms of early NSCLC, most diagnosis presents local spread or distant metastasis, resulting in poor prognosis. As one of the most important breakthroughs in oncology in recent years, immune checkpoint inhibitors (ICIs), characterized by programmed death 1 (PD-1), programmed death ligand 1 (PD-L1) and cytotoxic T lymphocyte associated antigen 4 inhibitors, have dramatically changed the treatment landscape, and even advanced into the first-line treatment of advanced or metastatic NSCLC (3–5). Immunotherapy can significantly improve the patient’s clinical outcome and for the first time offer hope of long-term survival for NSCLC patients. However, not all patients can benefit from immunotherapy, because only a small percentage of NSCLC patients are sensitive to ICIs (6, 7). The response rate for unscreened NSCLC patients treated with ICIs alone is usually less than 20% (3). On the other hand, unlike most traditional treatments, immunotherapy usually shows delayed response, pseudoprogression, hyperprogression and other phenomena, as well as immune related adverse events (8, 9), which brings difficulties and challenges to clinical treatment decision-making.
The complexity of immunotherapy response challenges the monitor of treatment assessment using conventional methods. Predictive biomarkers are required to determine immunotherapy achievement and survival benefit. PD-L1 expression is a typical and predictive biomarker for ICIs treatment, and patients who benefit most from immunotherapy can be selected (10). However, predictions based on PD-L1 levels are sometimes biased. Some patients without PD-L1 expression can achieve certain remission and control of lesions after immunotherapy, while some patients with PD-L1 expression cannot benefit from immunotherapy (11, 12). Tumor mutation burden is another new potential biomarker that can predict progression-free survival (PFS), but its prediction of overall survival (OS) is not reliable enough (13, 14). As the most important weapon of anti-tumor immunotherapy, tumor-infiltrating lymphocytes (TILs) CD3+, CD8+ and forkhead box protein 3 (FOXP3+) may be independent predictors of clinical benefit of ICIs (15–17). The most significant limitation, however, is the inability to predict efficacy from their density and location prior to treatment. Therefore, it is the focus and difficulty of ICIs treatment to find effective prognostic markers to predict immunotherapy response and screen out patients who really benefit.
With the progress of technical approaches and the wide application of ICIs, studies on dynamic monitor of treatment response and prognosis based on tissue specimen, liquid biopsy and imaging are gradually increasing. 18F-fluorodeoxyglucose positron emission tomography/computed tomography (18F-FDG PET/CT) is a functional noninvasive imaging modality based on glucose metabolism, which is of great value in cancer diagnosis, staging, efficacy judgment and prognosis evaluation (18). Malignant tumor cells proliferate and divide in an abnormally active manner, requiring more energy and leading to enhanced glycolysis. The high expression of glucose transporter type 1 can transport a large amount of glucose to meet its demand for rapid reproduction, resulting in the gradual aggregation of 18F-FDG in tumor cells and abnormal radioactive hyperconcentration of lesions (19). 18F-FDG PET/CT can reflect the systemic metabolism of malignant tumors at the molecular level (20, 21), so it can diagnose and evaluate the therapeutic efficacy of malignant tumors earlier and more accurately, and monitor survival prognosis. Several studies have shown that PET/CT metabolic parameters including the maximum standardized uptake values (SUVmax), the mean standardized uptake values (SUVmean), metabolic tumor volume (MTV) and total lesion glycolysis (TLG) have important predictive value in the prognostic evaluation of malignant tumors. Some studies have found that SUVmax can be used as a potential predictor of ICIs response (22, 23), while other studies have not found their relationship (24). MTV is usually considered as a typical prognostic parameter that reflects tumor volume with metabolic activity and the metabolic volume of anatomical lesions. TLG includes tumor metabolic activity and tumor metabolic volume, which can fully reflect the overall metabolic characteristics of tumor lesions. Studies have proved that baseline MTV and TLG can better predict prognosis than SUVmax for NSCLC patients undergoing surgery, radiotherapy, chemotherapy and targeted therapy (25–28). In terms of immunotherapy, the prognostic and predictive value of these metabolic parameters in NSCLC patients treated with ICIs is still unknown. This study aimed to investigate the prognostic value of baseline 18F-FDG PET/CT metabolic parameters MTV, SUVmax, SUVmean, and TLG for survival in advanced or metastatic NSCLC patients treated with ICIs.
Methods
Data source and search strategy
A comprehensive literature search for examining the value of 18F-FDG PET/CT in assessing immunotherapy response of patients with NSCLC was conducted in PubMed, Embase, the Cochrane Library and Web of Science databases. The keywords (MeSH, Emtree) include “positron emission tomography computed tomography”, “carcinoma, non-small-cell lung” and “immune checkpoint inhibitors”. The search strategy was combined with keywords and free words. The search was carried out without any language restriction from the inception of the databases to March 28, 2022. The bibliographies of the pertinent articles were searched to identify additional relevant studies. Full-text articles were further checked if abstracts did not provide sufficient information. Furthermore, the reference lists of related articles were scrutinized for additional studies. Reviews, case reports, letters to the editor, editorials comments, and conference abstracts were excluded.
Study selection
The inclusion criteria were as follows: 1) patient diagnosed as stage III or IV NSCLC patients; 2) patients treated with immune checkpoint inhibitors; 3) 18F-FDG PET or PET/CT performed before ICIs treatment as baseline; 4) reporting metabolic parameters, such as baseline SUVmax, SUVmean, MTV or TLG; 5) reporting survival data that HR and 95%CI can be directly obtained or calculated. Review articles, case reports, letters and studies with missed data or without follow-up were excluded.
Data extraction and collection
Data were extracted from searched publications by two authors of the manuscript independently (Tao Ling and Lianghui Zhang), and then the data were collected to a pre-designed data extraction form within Microsoft Excel version 2021 (Microsoft Corporation, Seattle, Washington, USA). Any difference in retrieval and filter was settled by consensus. Detailed data were extracted from each eligible study, including the first author, year of publication, country, study designing, number of patients, TNM staging, treatment protocol, and study endpoints.
Quality assessment
The risk of bias of the included studies was evaluated using the Newcastle-Ottawa Scale (NOS) based on three broad domains: selection of the study groups, comparability among different groups and ascertainment of either the exposure or outcome of interest (29). The total score of NOS uses a star system (maximum of nine stars) based on its assessment items. Two authors (Tao Ling and Lingli Huang) assessed the quality items and differences independently.
Statistical analyses
The endpoints were OS and PFS. The relationship between SUVmax, SUVmean, MTV, TLG and OS and PFS was evaluated in terms of the hazard ratio (HR) effect size. Univariate or multivariate HR estimates with 95% confidence intervals (CIs) were extracted directly from each study, if possible. For patients with high SUVmax, SUVmean, MTV or TLG, HR greater than 1 implied worse survival, whereas HR smaller than 1 implied a survival benefit. Heterogeneity was assessed by Cochran’s Q value (and its associated p value) as well as the inconsistency index (I2 index). I2 levels of 50% or less correspond to a low heterogeneity and I2 levels of 50% more correspond to a high heterogeneity. Begg’s test was used to assess publication bias. P < 0.05 was considered statistically significant. Data from each study were analyzed using STATA 14.0 software.
Results
Characteristics of the eligible studies
540 relevant articles were retrieved by the approaches mentioned in the previous section. After screening by the titles and abstracts, 234 articles were excluded. The full article evaluation for the remaining 20 studies was performed, and ultimately 13 eligible articles (30–42) were included in the systematic review. The PRISMA flowchart of the study is illustrated in Figure 1. The principal characteristics and further details of eligible articles are shown in Table 1. The assessment of study quality for all included trials is summarized in Table 2.
The value of SUVmax, SUVmean, MTV and TLG of 18F-FDG PET/CT in prediction of OS
A total of 9 studies (30, 31, 33–36, 38, 40, 42) explored the value of baseline SUVmax in prediction of OS. There was no significance in OS between high SUVmax group and low SUVmax group (HR: 0.88, 95% CI: 0.69-1.12) using random model for low heterogeneity between the studies (I2 = 16.8%, P = 0.293) (Figure 2A). Begg’s test (P = 0.602) manifested that there was no publication bias for recruited studies on OS.
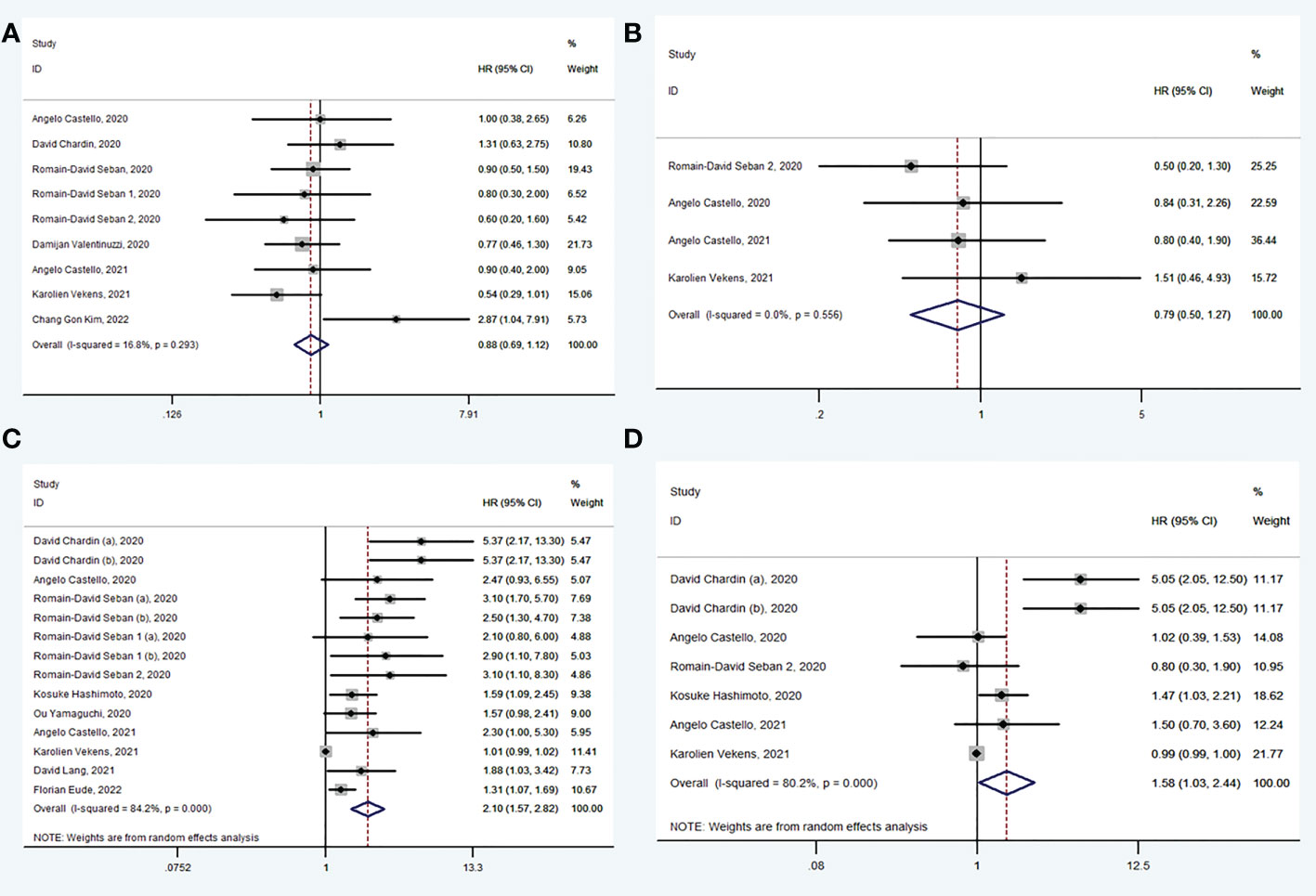
Figure 2 Forest plots of hazard ratios in studies investigating the prognostic value of SUVmax (A), SUVmean (B), MTV (C), TLG (D) for OS.
A total of 4 studies (30, 35, 38, 40) explored the value of baseline SUVmean in prediction of OS. There was no significance in OS between high SUVmean group and low SUVmean group (HR: 0.79, 95% CI: 0.50-1.27) using fixed model for low heterogeneity between the studies (I2 = 0.0%, P = 0.556) (Figure 2B). Begg’s test (P = 1.000) manifested that there was no publication bias for recruited studies on OS.
A total of 11 studies (30–35, 37–41) explored the value of baseline MTV in prediction of OS. In pooled analysis, OS was evidently prolonged in low MTV group (HR: 2.10, 95% CI: 1.57-2.82) using random model for heterogeneity between the studies (I2 = 84.2%, P < 0.001) (Figure 2C). Sensitivity analysis was performed and it was found that heterogeneity was reduced from 84.2% to 54.0% by excluding studies (Karolien Vekens, 2021), and the results were stable. Begg’s test (P = 1.000) manifested that there was no publication bias for recruited studies on OS.
A total of 6 studies (30–32, 35, 38, 40) explored the value of baseline TLG in prediction of OS. In pooled analysis, OS was evidently prolonged in low TLG group (HR: 1.58, 95% CI: 1.03-2.44) using random model for heterogeneity between the studies (I2 = 80.2%, P < 0.001) (Figure 2D). Sensitivity analysis was performed and it was found that heterogeneity was reduced from 80.2% to 69.6% by excluding studies (Karolien Vekens, 2021), and the results were stable. Begg’s test (P = 0.453) manifested that there was no publication bias for recruited studies on OS.
The value of SUVmax, SUVmean, MTV and TLG of 18F-FDG PET/CT in prediction of PFS
A total of 6 studies (33, 34, 36, 38, 40, 42) explored the value of baseline SUVmax in prediction of PFS. There was no significance in PFS between high SUVmax group and low SUVmax group (HR: 1.06, 95% CI: 0.68-1.65) and using random model for high heterogeneity between the studies (I2 = 74.7%, P < 0.001) (Figure 3A). Begg’s test (P = 0.174) manifested that there was no publication bias for recruited studies on FPS.
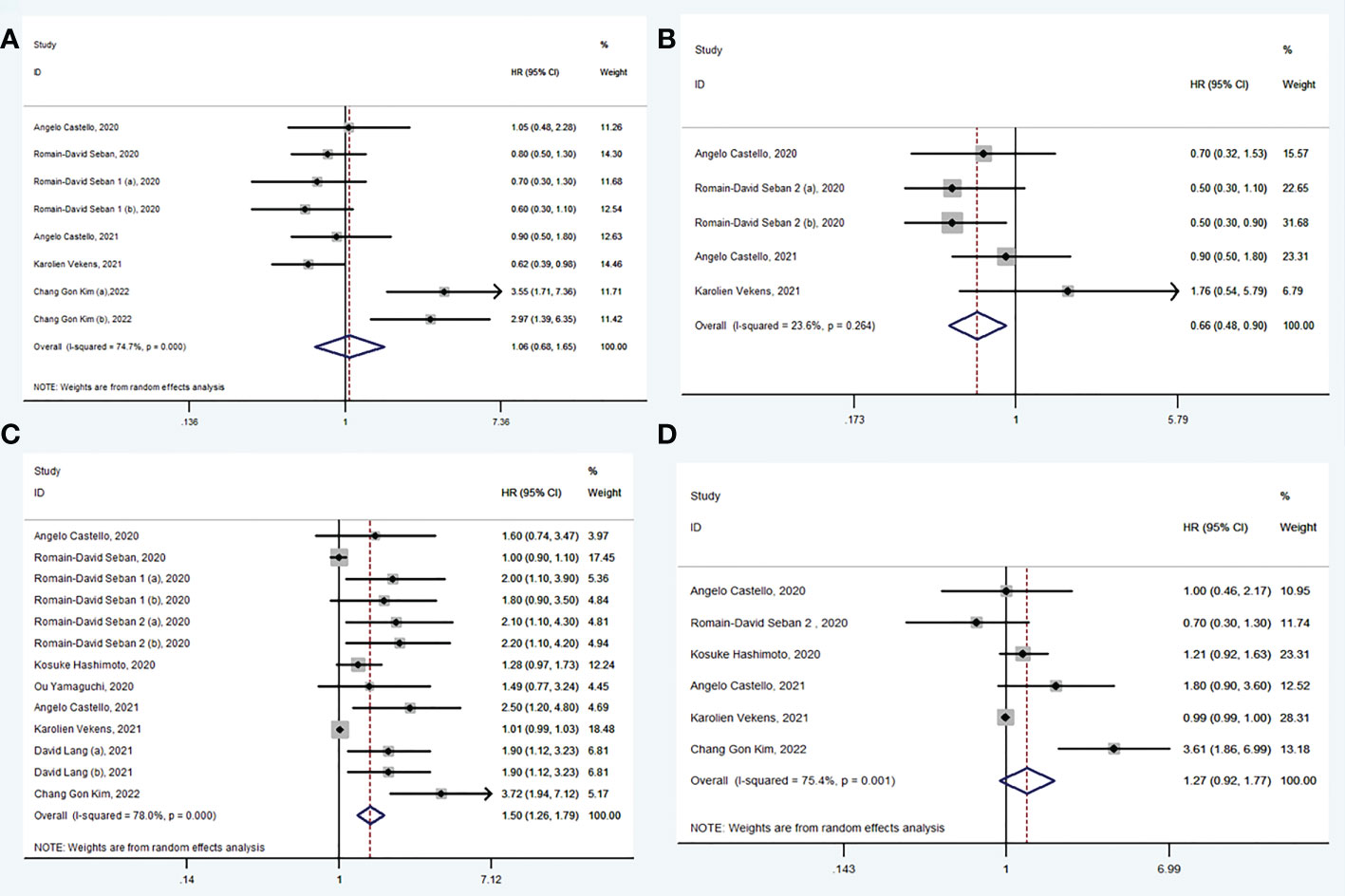
Figure 3 Forest plots of hazard ratios in studies investigating the prognostic value of SUVmax (A), SUVmean (B), MTV (C), TLG (D) for PFS.
A total of 4 studies (30, 35, 38, 40) explored the value of baseline SUVmean in prediction of PFS. In pooled analysis, PFS was evidently prolonged in low SUVmean group (HR: 0.66, 95% CI: 0.48-0.90) using fixed model for low heterogeneity between the studies (I2 = 23.6%, P = 0.264) (Figure 3B). Begg’s test (P = 0.221) manifested that there was no publication bias for recruited studies on PFS.
A total of 10 studies (30, 32–35, 37–40, 42) explored the value of baseline MTV in prediction of PFS. In pooled analysis, PFS was evidently prolonged in low MTV group (HR: 1.50, 95% CI: 1.26-1.79) using random model for heterogeneity between the studies (I2 = 78.0%, P < 0.001) (Figure 3C). Sensitivity analyses showed that heterogeneity was reduced from 78.0% to 16.7% when studies were excluded (Romain-David Seban, 2020 and Karolien Vekens, 2021), and the results were stable. Begg’s test (P = 0.625) manifested that there was no publication bias for recruited studies on PFS.
A total of 6 studies (30, 32, 35, 38, 40, 42) explored the value of baseline TLG in prediction of PFS. There was no significance in PFS between high TLG group and low TLG group (HR: 1.27, 95% CI: 0.92-1.77) using random model for high heterogeneity between the studies (I2 = 75.4%, P = 0.001) (Figure 3D). Begg’s test (P = 0.625) manifested that there was no publication bias for recruited studies on PFS.
Subgroup analysis
For patients with NSCLC, a subgroup analysis of the treatment line was performed in the study, which were first line (only including first line treatment) and undefined line (including first or later line treatment, and second or later line treatment). The results are shown in Table 3. Subgroup analysis showed that no significant differences in OS and PFS were found between high SUVmax group and low SUVmax group either in first line group (HR: 0.96, 95% CI: 0.54-1.71; HR: 1.19, 95% CI: 0.56-2.54) or in undefined line group (HR: 1.20, 95% CI: 0.81-1.77; HR: 0.87, 95% CI: 0.62-1.23). As for SUVmean, there was also no significant difference for OS and PFS between two group in first line group (HR: 0.82, 95% CI: 0.28-2.39; HR: 0.63, 95% CI: 0.35-1.14) and undefined line group (HR: 0.81, 95% CI: 0.44-1.50; HR: 0.81, 95% CI: 0.50-1.34). High MTV group displayed shorter OS than low MTV group in both first line group (HR: 1.97, 95% CI: 1.39-2.79) and undefined line group (HR: 2.11, 95% CI: 1.61-2.77), but high MTV group only showed an inferior PFS in first line group (HR: 1.85, 95% CI: 1.28-2.68). Low TLG group displayed a longer OS in undefined line group (HR: 1.37, 95% CI: 1.00-1.86), rather than in first line group (HR: 2.03, 95% CI: 0.78-5.26).
Discussion
PET/CT has been proved to be of great value in the diagnosis, clinical staging, radiotherapy localization, efficacy evaluation and prognosis of NSCLC (31, 43), but its prognostic status in ICIs treatment is unclear. This meta-analysis evaluated the prognostic value of baseline metabolic parameters of 18F-FDG PET/CT for advanced or metastasis NSCLC by analyzing the HR of PFS and OS in patients with high SUVmax, SUVmean, MTV or TLG versus those with low SUVmax, SUVmean, MTV or TLG. The pooled results showed patients with high MTV and TLG prior to ICIs treatment may had shorter OS than patients with lower metabolic rate, suggesting that MTV and TLG may be meaningful prognostic markers. High MTV may also predict worse PFS, and patients with high SUVmean had better PFS. No correlation was found between SUVmax and OS or PFS.
Unlike traditional tumor therapy by killing cancer cells, ICIs work in an immunomodulatory way maybe by modulating tumor metabolism. The proliferation rate of tumor cells is positively related to their glucose uptake (44). Higher uptake of FDG leads to more aggressive tumors and worse prognosis (45). SUV has independent and prognostic value for OS in NSCLC patients, but most studies are based on early NSCLC (46–48). SUVmax and SUVmean are associated with the presence of CD8+ TILs in patients with NSCLC (20). Takada et al. also linked high SUVmax to positivity for PD-L1 (49). These findings suggest that high FDG uptake may indicate an immunologically cold tumor. However, previous studies have also found that this may not be true for patients with advanced NSCLC. SUVmax is, to a lesser extent, considered to be an effective predictor of prognosis in patients with NSCLC, regardless of treatment or disease stage (50, 51). Our pooled results also found that baseline SUVmax did not provide any prognostic information. High SUVmean may predict longer PFS, but had no correlation with OS. The results of subgroup analysis showed no predictive value of SUVmax and SUVmean in first line group and undefined line group. It is worth noting that pseudoprogression can lead to possible misjudgment by PFS. Both SUVmax and SUVmean can represent the uptake level of FDG, but cannot reflect the overall metabolism of tumor. Especially, SUV does not consider the effect of tumor volume on prognosis, and only reflects the metabolic level of mononectin in the lesions (52, 53).
When extensive metastasis occurs, the tumor load is large, which may be more valuable than the metabolic activity for primary lung cancer prognosis. Liao S et al. (54) found that MTV and TLG were better predictors of clinical outcomes in patients with stage IV NSCLC than SUVmax and SUVmean. MTV and TLG are another type of metabolic parameters that take tumor metabolic volume and metabolic activity into comprehensive consideration. MTV can not only calculate the tumor volume, but also display the metabolic activity of the lesions. However, TLG combined with MTV and glycolysis can analyze the overall metabolic status of lesions more comprehensively. The pooled results of this study showed OS and PFS was shortened in high MTV group, indicating the larger the tumor metabolic volume and metabolic load, the shorter the possible survival, the worse the prognosis. High TLG group also showed shorter OS than low TLG group, but no significance was found for PFS. Nonetheless, significant heterogeneity was found in the analysis of MTV and TLG predicting PFS and OS. The subgroup analysis of treatment line showed that OS in high MTV group was significantly shorter than that in low MTV group in either first line group or undefined line group. However, high MTV may only predict poor PFS in first line group, but not in undefined line group. Meanwhile, high TLG was associated with poor OS in undefined line group, but not in first line group. MTV has shown good prognostic ability, but the predictive significance of TLG is unclear in the different treatment lines.
There is no theoretical explanation of the mechanism by which these metabolic parameters serve as prognostic markers for ICIs treatment. Advanced or metastatic NSCLC is often accompanied by lymph node metastasis, or liver, bone, brain and other organ metastasis. Therefore, the impact of metastasis other than the primary site of tumor should be focused on in prognosis assessment. Systemic tumor metabolic load includes the metabolic load of primary tumor, metastatic lymph node and distant metastasis, which more comprehensively reflects systemic metabolic information. MTV and TLG measure tumor volume and metabolic activity in three dimensions, providing more information about tumor aggressiveness. This study shows that NSCLC patients with high baseline MTV have a poor prognosis after ICIs treatment. MTV reflects the real volume of tumors with high metabolic activity in tumor tissues, and the high accumulation of FDG may be related to tumor necrosis or hypoxia caused by larger tumor deposition. Tumor necrosis, acidity and hypoxia can promote the establishment of immunosuppressive tumor microenvironment by recruiting a variety of immunosuppressive cells, such as myeloid-derived suppressor cells, regulatory t cells (Tregs) and tumor-associated macrophages (55, 56). These are closely associated with tumor recurrence and survival deterioration (57, 58). In this environment, FOXP3+, the major regulator of Tregs, has been reported to be positively correlated with MTV (59, 60). Whereas, in the anoxic environment caused by tumor necrosis and inflammation, no correlation has been found between PD-L1 expression and MTV (61). Studies have found that NSCLC patients with positive PD-L1 expression have significantly higher MTV than patients with negative PD-L1 expression (62), While other studies have shown that MTV is not significantly associated with PD-L1 expression (60, 63). Although clinical trials have confirmed that high expression of PD-L1 can make tumor cells more sensitive to immunotherapy (64, 65), and can also mediate immune escape (66, 67). PD-L1 may play a dual role, but it is vague what mechanism determines PD-L1 to activate drug resistance or response pathways. In summary, an environment with high MTV may form an immunosuppressive state, thus leading to drug resistance of ICIs, regardless of PD-L1 expression. MTV may be a better parameter to predict immunotherapy response and prognosis, but its mechanism needs to be clarified.
This is the first meta-analysis to evaluate ability of baseline metabolic parameters of 18F-FDG PET/CT for survival in advanced or metastatic NSCLC patients treated with ICIs. Our results suggest that MTV can be considered as a basis for patient stratification in the immunotherapy and prognosis of advanced or metastatic NSCLC patients. This provides some data support for clinical application of 18F-FDG PET/CT to judge the prognosis of NSCLC patients and guides the treatment of lung cancer. Although analysis software is available to measure these metabolism parameters (40–42), MTVand TLG are not typical components of standard PET/CT reports because of insufficient evidence of clinical application value and increased workload. As the clinical significance of these parameters is fully validated, radiologists will become aware of their potential applications. It is economical and feasible to help patients select the best treatment strategy by obtaining baseline MTV and TLG of NSCLC patients only once before ICIs treatment in the future. Early identification of patients who do not benefit from immunotherapy may lead to timely decisions and discontinuation of treatment, potentially reducing drug toxicity and the high cost of immunotherapy and initiating different treatment regimens earlier.
However, the study has several limitations. Firstly, heterogeneity was detected in the present meta-analysis. This may be due to bias in detection methods, differences in ICIs treatment, etc. Secondly, we cannot determine an optimal threshold to classify volume parameters as high or low. Different cut-off values and delineation strategies, as well as different histological methods, may influence the occurrence and survival of events. Thirdly, there are different types of NSCLC, such as adenocarcinoma and squamous cell carcinoma, with different prognosis. However, in the currently included studies, all of these subtypes are mixed and these studies can yield different results in response prediction and monitoring with 18F-FDG PET/CT imaging. Therefore, further large-scale prospective studies are necessary to evaluate whether MTV and TLG can be independent prognostic factors for clinical outcome in patients with different types of NSCLC.
Conclusion
Baseline MTV may have strong predictive power for survival, while baseline SUVmax and SUVmean may not be appropriate prognostic markers in advanced or metastatic NSCLC patients treated with ICIs. Baseline TLG may predict OS for only a subset of patients, and its predictive value remains to be examined.
Data availability statement
The raw data supporting the conclusions of this article will be made available by the authors, without undue reservation.
Author contributions
TL and LZ conducted the literatures screening. TL and RP conducted the statistical analysis. TL, LZ and LH wrote the manuscript. LH and CY revised the manuscript. All authors contributed to the article and approved the submitted version.
Conflict of interest
The authors declare that the research was conducted in the absence of any commercial or financial relationships that could be construed as a potential conflict of interest.
Publisher’s note
All claims expressed in this article are solely those of the authors and do not necessarily represent those of their affiliated organizations, or those of the publisher, the editors and the reviewers. Any product that may be evaluated in this article, or claim that may be made by its manufacturer, is not guaranteed or endorsed by the publisher.
References
1. Sung H, Ferlay J, Siegel RL, Laversanne M, Soerjomataram I, Jemal A, et al. Global cancer statistics 2020: GLOBOCAN estimates of incidence and mortality worldwide for 36 cancers in 185 countries. CA Cancer J Clin (2021) 71(3):209–49. doi: 10.3322/caac.21660
2. Duma N, Santana-Davila R, Molina JR. Non-small cell lung cancer: Epidemiology, screening, diagnosis, and treatment. Mayo Clinic Proc (2019) 94(8):1623–40. doi: 10.1016/j.mayocp.2019.01.013
3. Garon EB, Rizvi NA, Hui R, Leighl N, Balmanoukian AS, Eder JP, et al. Pembrolizumab for the treatment of non-small-cell lung cancer. N Engl J Med (2015) 372(21):2018–28. doi: 10.1056/NEJMoa1501824
4. Borghaei H, Paz-Ares L, Horn L, Spigel DR, Steins M, Ready NE, et al. Nivolumab versus docetaxel in advanced nonsquamous non-Small-Cell lung cancer. N Engl J Med (2015) 373(17):1627–39. doi: 10.1056/NEJMoa1507643
5. Fehrenbacher L, Spira A, Ballinger M, Kowanetz M, Vansteenkiste J, Mazieres J, et al. Atezolizumab versus docetaxel for patients with previously treated non-small-cell lung cancer (POPLAR): A multicentre, open-label, phase 2 randomised controlled trial. Lancet (London England). (2016) 387(10030):1837–46. doi: 10.1016/s0140-6736(16)00587-0
6. Borghaei H, Gettinger S, Vokes EE, Chow LQM, Burgio MA, de Castro Carpeno J, et al. Five-year outcomes from the randomized, phase III trials CheckMate 017 and 057: Nivolumab versus docetaxel in previously treated non-Small-Cell lung cancer. J Clin Oncol Off J Am Soc Clin Oncol (2021) 39(7):723–33. doi: 10.1200/jco.20.01605
7. Doroshow DB, Herbst RS. Treatment of advanced non-small cell lung cancer in 2018. JAMA Oncol (2018) 4(4):569–70. doi: 10.1001/jamaoncol.2017.5190
8. Zhou L, Zhang M, Li R, Xue J, Lu Y. Pseudoprogression and hyperprogression in lung cancer: a comprehensive review of literature. J Cancer Res Clin Oncol (2020) 146(12):3269–79. doi: 10.1007/s00432-020-03360-1
9. Kadono T. Immune-related adverse events by immune checkpoint inhibitors. Nihon Rinsho Men'eki Gakkai kaishi = Japanese J Clin Immunol (2017) 40(2):83–9. doi: 10.2177/jsci.40.83
10. Brody R, Zhang Y, Ballas M, Siddiqui MK, Gupta P, Barker C, et al. PD-L1 expression in advanced NSCLC: Insights into risk stratification and treatment selection from a systematic literature review. Lung Cancer. (2017) 112:200–15. doi: 10.1016/j.lungcan.2017.08.005
11. Doroshow DB, Bhalla S, Beasley MB, Sholl LM, Kerr KM, Gnjatic S, et al. PD-L1 as a biomarker of response to immune-checkpoint inhibitors. Nat Rev Clin Oncol (2021) 18(6):345–62. doi: 10.1038/s41571-021-00473-5
12. Hellmann MD, Paz-Ares L, Caro RB, Zurawski B, Kim SW, Costa EC, et al. Nivolumab plus ipilimumab in advanced non-Small-Cell lung cancer. New Engl J Med (2019) 381(21):2020–31. doi: 10.1056/NEJMoa1910231
13. Rizvi NA, Hellmann MD, Snyder A, Kvistborg P, Makarov V, Havel JJ, et al. Cancer immunology. mutational landscape determines sensitivity to PD-1 blockade in non-small cell lung cancer. Sci (New York NY). (2015) 348(6230):124–8. doi: 10.1126/science.aaa1348
14. Chan TA, Yarchoan M, Jaffee E, Swanton C, Quezada SA, Stenzinger A, et al. Development of tumor mutation burden as an immunotherapy biomarker: Utility for the oncology clinic. Ann Oncol Off J Eur Soc Med Oncol (2019) 30(1):44–56. doi: 10.1093/annonc/mdy495
15. Kim H, Kwon HJ, Han YB, Park SY, Kim ES, Kim SH, et al. Increased CD3+T cells with a low FOXP3+/CD8+T cell ratio can predict anti-PD-1 therapeutic response in non-small cell lung cancer patients. Modern Pathology. (2019) 32(3):367–75. doi: 10.1038/s41379-018-0142-3
16. Tumeh PC, Harview CL, Yearley JH, Shintaku IP, Taylor EJ, Robert L, et al. PD-1 blockade induces responses by inhibiting adaptive immune resistance. Nature (2014) 515:568–71. doi: 10.1038/nature13954
17. Geng YT, Shao YJ, He WT, Hu WW, Xu YJ, Chen J, et al. Prognostic role of tumor-infiltrating lymphocytes in lung cancer: A meta-analysis. Cell Physiol Biochem (2015) 37(4):1560–71. doi: 10.1159/000438523
18. Stroobants S, Verschakelen J, Vansteenkiste J. Value of FDG-PET in the management of non-small cell lung cancer. Eur J Radiol (2003) 45(1):49–59. doi: 10.1016/s0720-048x(02)00282-6
19. Kurahara H, Maemura K, Mataki Y, Sakoda M, Iino S, Kawasaki Y, et al. Significance of glucose transporter type 1 (GLUT-1) expression in the therapeutic strategy for pancreatic ductal adenocarcinoma. Ann Surg Oncol (2018) 25(5):1432–9. doi: 10.1245/s10434-018-6357-1
20. Lopci E, Toschi L, Grizzi F, Rahal D, Olivari L, Castino GF, et al. Correlation of metabolic information on FDG-PET with tissue expression of immune markers in patients with non-small cell lung cancer (NSCLC) who are candidates for upfront surgery. Eur J Nucl Med Mol Imaging. (2016) 43(11):1954–61. doi: 10.1007/s00259-016-3425-2
21. Donegani MI, Ferrarazzo G, Marra S, Miceli A, Raffa S, Bauckneht M, et al. Positron emission tomography-based response to target and immunotherapies in oncology. Medicina (Kaunas). (2020) 56(8):373. doi: 10.3390/medicina56080373
22. Evangelista L, Cuppari L, Menis J, Bonanno L, Reccia P, Frega S, et al. 18F-FDG PET/CT in non-small-cell lung cancer patients: A potential predictive biomarker of response to immunotherapy. Nucl Med Commun (2019) 40(8):802–7. doi: 10.1097/mnm.0000000000001025
23. Takada K, Toyokawa G, Tagawa T, Kohashi K, Akamine T, Takamori S, et al. Association between PD-L1 expression and metabolic activity on (18)F-FDG PET/CT in patients with small-sized lung cancer. Anticancer Res (2017) 37(12):7073–82. doi: 10.21873/anticanres.12180
24. Polverari G, Ceci F, Bertaglia V, Reale ML, Rampado O, Gallio E, et al. (18)F-FDG pet parameters and radiomics features analysis in advanced nsclc treated with immunotherapy as predictors of therapy response and survival. Cancers (Basel). (2020) 12(5):1163. doi: 10.3390/cancers12051163
25. Liao S, Penney BC, Wroblewski K, Zhang H, Simon CA, Kampalath R, et al. Prognostic value of metabolic tumor burden on 18F-FDG PET in nonsurgical patients with non-small cell lung cancer. Eur J Nucl Med Mol Imaging. (2012) 39(1):27–38. doi: 10.1007/s00259-011-1934-6
26. Chin AL, Kumar KA, Guo HH, Maxim PG, Wakelee H, Neal JW, et al. Prognostic value of pretreatment FDG-PET parameters in high-dose image-guided radiotherapy for oligometastatic non-small-cell lung cancer. Clin Lung Cancer. (2018) 19(5):E581–E8. doi: 10.1016/j.cllc.2018.04.003
27. Sharma A, Mohan A, Bhalla AS, Sharma MC, Vishnubhatla S, Das CJ, et al. Role of various metabolic parameters derived from baseline 18F-FDG PET/CT as prognostic markers in non-small cell lung cancer patients undergoing platinum-based chemotherapy. Clin Nucl Med (2018) 43(1):E8–E17. doi: 10.1097/rlu.0000000000001886
28. Winther-Larsen A, Fledelius J, Sorensen BS, Meldgaard P. Metabolic tumor burden as marker of outcome in advanced EGFR wild-type NSCLC patients treated with erlotinib. Lung Cancer. (2016) 94:81–7. doi: 10.1016/j.lungcan.2016.01.024
29. Wells GA, Shea B, O’Connell D, Peterson J, Welch V, Losos M, et al. The Newcastle Ottawa scale (NOS) for assessing the quality of non-randomised studies in meta-analyses. (2000). Available at: http://www.ohri.ca/programs/clinical_epidemiology/oxford.htm.
30. Castello A, Carbone FG, Rossi S, Monterisi S, Federico D, Toschi L, et al. Circulating tumor cells and metabolic parameters in NSCLC patients treated with checkpoint inhibitors. Cancers (2020) 12(2):487. doi: 10.3390/cancers12020487
31. Chardin D, Paquet M, Schiappa R, Darcourt J, Bailleux C, Poudenx M, et al. Baseline metabolic tumor volume as a strong predictive and prognostic biomarker in patients with non-small cell lung cancer treated with PD1 inhibitors: A prospective study. J Immunotherapy Cancer (2020) 8(2):10. doi: 10.1136/jitc-2020-000645
32. Hashimoto K, Kaira K, Yamaguchi O, Mouri A, Shiono A, Miura Y, et al. Potential of FDG-PET as prognostic significance after anti-pd-1 antibody against patients with previously treated non-small cell lung cancer. J Clin Med (2020) 9(3):725. doi: 10.3390/jcm9030725
33. Seban RD, Mezquita L, Berenbaum A, Dercle L, Botticella A, Le Pechoux C, et al. Baseline metabolic tumor burden on FDG PET/CT scans predicts outcome in advanced NSCLC patients treated with immune checkpoint inhibitors. Eur J Nucl Med Mol Imaging. (2020) 47(5):1147–57. doi: 10.1007/s00259-019-04615-x
34. Seban RD, Assie JB, Giroux-Leprieur E, Massiani MA, Soussan M, Bonardel G, et al. Association of the metabolic score using baseline FDG-PET/CT and dNLR with immunotherapy outcomes in advanced NSCLC patients treated with first-line pembrolizumab. Cancers (2020) 12(8). doi: 10.3390/cancers12082234
35. Seban RD, Assie JB, Giroux-Leprieur E, Massiani MA, Soussan M, Bonardel G, et al. FDG-PET biomarkers associated with long-term benefit from first-line immunotherapy in patients with advanced non-small cell lung cancer. Ann Nucl Med (2020) 34(12):968–74. doi: 10.1007/s12149-020-01539-7
36. Valentinuzzi D, Vrankar M, Boc N, Ahac V, Zupancic Z, Unk M, et al. [18F]FDG PET immunotherapy radiomics signature (iRADIOMICS) predicts response of non-small-cell lung cancer patients treated with pembrolizumab. Radiol Oncol (2020) 54(3):285–94. doi: 10.2478/raon-2020-0042
37. Yamaguchi O, Kaira K, Hashimoto K, Mouri A, Shiono A, Miura Y, et al. Tumor metabolic volume by f-18-FDG-PET as a prognostic predictor of first-line pembrolizumab for NSCLC patients with PD-L1 >= 50%. Sci Rep (2020) 10(1):14990. doi: 10.1038/s41598-020-71735-y
38. Castello A, Rossi S, Toschi L, Lopci E. Impact of antibiotic therapy and metabolic parameters in non-small cell lung cancer patients receiving checkpoint inhibitors. J Clin Med [Article]. (2021) 10(6):1–12. doi: 10.3390/jcm10061251
39. Lang D, Ritzberger L, Rambousek V, Horner A, Wass R, Akbari K, et al. First-line pembrolizumab mono- or combination therapy of non-small cell lung cancer: Baseline metabolic biomarkers predict outcomes. Cancers (Basel). (2021) 13(23):6096. doi: 10.3390/cancers13236096
40. Vekens K, Everaert H, Neyns B, Ilsen B, Decoster L. The value of 18F-FDG PET/CT in predicting the response to PD-1 blocking immunotherapy in advanced NSCLC patients with high-level PD-L1 expression. Clin Lung Cancer. (2021) 22(5):432–40. doi: 10.1016/j.cllc.2021.03.001
41. Eude F, Guisier F, Salaün M, Thiberville L, Pressat-Laffouilhere T, Vera P, et al. Prognostic value of total tumour volume, adding necrosis to metabolic tumour volume, in advanced or metastatic non-small cell lung cancer treated with first-line pembrolizumab. Ann Nucl Med (2022) 36(3):224–34. doi: 10.1007/s12149-021-01694-5
42. Kim CG, Hwang SH, Kim KH, Yoon HI, Shim HS, Lee JH, et al. Predicting treatment outcomes using (18)F-FDG PET biomarkers in patients with non-small-cell lung cancer receiving chemoimmunotherapy. Ther Adv Med Oncol (2022) 14:1–13. doi: 10.1177/17588359211068732
43. Im HJ, Pak K, Cheon GJ, Kang KW, Kim SJ, Kim IJ, et al. Prognostic value of volumetric parameters of (18)F-FDG PET in non-small-cell lung cancer: A meta-analysis. Eur J Nucl Med Mol Imaging. (2015) 42(2):241–51. doi: 10.1007/s00259-014-2903-7
44. Higashi K, Ueda Y, Yagishita M, Arisaka U, Sakurai A, Oguchi M, et al. FDG PET measurement of the proliferative potential of non-small cell lung cancer. J Nucl Med (2000) 41(1):85–92.
45. Suzawa N, Ito M, Qiao S, Uchida K, Takao M, Yamada T, et al. Assessment of factors influencing FDG uptake in non-small cell lung cancer on PET/CT by investigating histological differences in expression of glucose transporters 1 and 3 and tumour size. Lung Cancer. (2011) 72(2):191–8. doi: 10.1016/j.lungcan.2010.08.017
46. Cerfolio RJ, Bryant AS, Ohja B, Bartolucci AA. The maximum standardized uptake values on positron emission tomography of a non-small cell lung cancer predict stage, recurrence, and survival. J Thorac Cardiovasc Surgery. (2005) 130(1):151–9. doi: 10.1016/j.jtcvs.2004.11.007
47. de Jong WK, van der Heijden HFM, Pruim J, Dalesio O, Oyen WJG, Groen HJM. Prognostic value of different metabolic measurements with fluorine-18 fluorodeoxyglucose positron emission tomography in resectable non-small cell lung cancer: A two-center study. J Thorac Oncol (2007) 2(11):1007–12. doi: 10.1097/JTO.0b013e31815608f5
48. Hoang JK, Hoagland LF, Coleman RE, Coan AD, Herndon JE, Patz EF. Prognostic value of fluorine-18 fluorodeoxyglucose positron emission tomography imaging in patients with advanced-stage non-small-cell lung carcinoma. J Clin Oncol (2008) 26(9):1459–64. doi: 10.1200/jco.2007.14.3628
49. Takada K, Toyokawa G, Okamoto T, Baba S, Kozuma Y, Matsubara T, et al. Metabolic characteristics of programmed cell death-ligand 1-expressing lung cancer on 18F-fluorodeoxyglucose positron emission tomography/computed tomography. Cancer Med (2017) 6(11):2552–61. doi: 10.1002/cam4.1215
50. Houdu B, Lasnon C, Licaj I, Thomas G, Do P, Guizard AV, et al. Why harmonization is needed when using FDG PET/CT as a prognosticator: demonstration with EARL-compliant SUV as an independent prognostic factor in lung cancer. Eur J Nucl Med Mol Imaging. (2019) 46(2):421–8. doi: 10.1007/s00259-018-4151-8
51. Ceriani L, Milan L, Johnson PWM, Martelli M, Presilla S, Giovanella L, et al. Baseline PET features to predict prognosis in primary mediastinal b cell lymphoma: A comparative analysis of different methods for measuring baseline metabolic tumour volume. Eur J Nucl Med Mol Imaging. (2019) 46(6):1334–44. doi: 10.1007/s00259-019-04286-8
52. Hirata K, Tamaki N. Quantitative FDG PET assessment for oncology therapy. Cancers (Basel). (2021) 13(4):869. doi: 10.3390/cancers13040869
53. Hicks RJ. The value of the standardized uptake value (SUV) and metabolic tumor volume (MTV) in lung cancer. Semin Nucl Med (2022) S0001-2998(22):00038–1. doi: 10.1053/j.semnuclmed.2022.04.007
54. Liao SR, Penney BC, Zhang H, Suzuki K, Pu YL. Prognostic value of the quantitative metabolic volumetric measurement on 18F-FDG PET/CT in stage IV nonsurgical small-cell lung cancer. Acad Radiology. (2012) 19(1):69–77. doi: 10.1016/j.acra.2011.08.020
55. Kumar V, Gabrilovich DI. Hypoxia-inducible factors in regulation of immune responses in tumour microenvironment. Immunology (2014) 143(4):512–9. doi: 10.1111/imm.12380
56. Facciabene A, Peng XH, Hagemann IS, Balint K, Barchetti A, Wang LP, et al. Tumour hypoxia promotes tolerance and angiogenesis via CCL28 and T-reg cells. Nature (2011) 475(7355):226–U141. doi: 10.1038/nature10169
57. Garg AD, Agostinis P. Cell death and immunity in cancer: From danger signals to mimicry of pathogen defense responses. Immunol Rev (2017) 280(1):126–48. doi: 10.1111/imr.12574
58. Gkogkou C, Frangia K, Saif MW, Trigidou R, Syrigos K. Necrosis and apoptotic index as prognostic factors in non-small cell lung carcinoma: A review. Springerplus (2014) 3:120. doi: 10.1186/2193-1801-3-120
59. Schaaf MB, Garg AD, Agostinis P. Defining the role of the tumor vasculature in antitumor immunity and immunotherapy. Cell Death Dis (2018) 9(2):115. doi: 10.1038/s41419-017-0061-0
60. Wang Y, Zhao N, Wu Z, Pan N, Shen XJ, Liu T, et al. New insight on the correlation of metabolic status on (18)F-FDG PET/CT with immune marker expression in patients with non-small cell lung cancer. Eur J Nucl Med Mol Imaging. (2020) 47(5):1127–36. doi: 10.1007/s00259-019-04500-7
61. Jreige M, Letovanec I, Chaba K, Renaud S, Rusakiewicz S, Cristina V, et al. F-18-FDG PET metabolic-to-morphological volume ratio predicts PD-L1 tumour expression and response to PD-1 blockade in non-small-cell lung cancer. Eur J Nucl Med Mol Imaging. (2019) 46(9):1859–68. doi: 10.1007/s00259-019-04348-x
62. Wang DL, Li YC, Chen XL, Li P. Prognostic significance of volume-based f-18-FDG PET/CT parameters and correlation with PD-L1 expression in patients with surgically resected lung adenocarcinoma. Medicine (2021) 100(35):e27100. doi: 10.1097/md.0000000000027100
63. Ishimura M, Norikane T, Mitamura K, Yamamoto Y, Arai-Okuda H, Murota M, et al. Correlation of epidermal growth factor receptor mutation status and PD-L1 expression with f-18 FDG PET using volume-based parameters in non-small cell lung cancer. Nucl Med Commun (2022) 43(3):304–9. doi: 10.1097/mnm.0000000000001517
64. Reck M, Rodriguez-Abreu D, Robinson AG, Hui RN, Csoszi T, Fulop A, et al. Pembrolizumab versus chemotherapy for PD-L1-Positive non-Small-Cell lung cancer. New Engl J Med (2016) 375(19):1823–33. doi: 10.1056/NEJMoa1606774
65. Ott PA, Elez E, Hiret S, Kim DW, Morosky A, Saraf S, et al. Pembrolizumab in patients with extensive-stage small-cell lung cancer: Results from the phase ib KEYNOTE-028 study. J Clin Oncol (2017) 35(34):3823–+. doi: 10.1200/jco.2017.72.5069
66. Lastwika KJ, Wilson W, Li QK, Norris J, Xu HY, Ghazarian SR, et al. Control of PD-L1 expression by oncogenic activation of the AKT-mTOR pathway in non-small cell lung cancer. Cancer Res (2016) 76(2):227–38. doi: 10.1158/0008-5472.Can-14-3362
Keywords: immune checkpoint inhibitor, non-small-cell lung cancer, prognosis, 18F-FDG PET/CT, metabolic tumor volume
Citation: Ling T, Zhang L, Peng R, Yue C and Huang L (2022) Prognostic value of 18F-FDG PET/CT in patients with advanced or metastatic non-small-cell lung cancer treated with immune checkpoint inhibitors: A systematic review and meta-analysis. Front. Immunol. 13:1014063. doi: 10.3389/fimmu.2022.1014063
Received: 08 August 2022; Accepted: 20 October 2022;
Published: 17 November 2022.
Edited by:
Sarah Weiss, The State University of New Jersey, United StatesReviewed by:
Murat Fani Bozkurt, Hacettepe University, TurkeySemra Paydaş, Çukurova University, Turkey
Copyright © 2022 Ling, Zhang, Peng, Yue and Huang. This is an open-access article distributed under the terms of the Creative Commons Attribution License (CC BY). The use, distribution or reproduction in other forums is permitted, provided the original author(s) and the copyright owner(s) are credited and that the original publication in this journal is cited, in accordance with accepted academic practice. No use, distribution or reproduction is permitted which does not comply with these terms.
*Correspondence: Lingli Huang, lingli_huang@njmu.edu.cn; Chao Yue, yuechaomeng@126.com
†These authors have contributed equally to this work