- 1Department of Cardiology, The First Affiliated Hospital of Soochow University, Suzhou, China
- 2Department of Dermatology, The First Affiliated Hospital of Nanjing Medical University, Nanjing, China
- 3Department of Plastic and Burn Surgery, The Second Affiliated Hospital of Chengdu Medical College, China National Nuclear Corporation 416 Hospital, Chengdu, China
Background: Although increasing evidence has reported an increased risk of atherosclerosis (AS) in rheumatoid arthritis (RA), the communal molecular mechanism of this phenomenon is still far from being fully elucidated. Hence, this article aimed to explore the pathogenesis of RA complicated with AS.
Methods: Based on the strict inclusion/exclusion criteria, four gene datasets were downloaded from the Gene Expression Omnibus (GEO) database. After identifying the communal differentially expressed genes (DEGs) and hub genes, comprehensive bioinformatics analysis, including functional annotation, co-expression analysis, expression validation, drug-gene prediction, and TF-mRNA-miRNA regulatory network construction, was conducted. Moreover, the immune infiltration of RA and AS was analyzed and compared based on the CIBERSORT algorithm, and the correlation between hub genes and infiltrating immune cells was evaluated in RA and AS respectively.
Results: A total of 54 upregulated and 12 downregulated communal DEGs were screened between GSE100927 and GSE55457, and functional analysis of these genes indicated that the potential pathogenesis lies in immune terms. After the protein-protein interaction (PPI) network construction, a total of six hub genes (CCR5, CCR7, IL7R, PTPRC, CD2, and CD3D) were determined as hub genes, and the subsequent comprehensive bioinformatics analysis of the hub genes re-emphasized the importance of the immune system in RA and AS. Additionally, three overlapping infiltrating immune cells were found between RA and AS based on the CIBERSORT algorithm, including upregulated memory B cells, follicular helper T cells and γδT cells.
Conclusions: Our study uncover the communal central genes and commonness in immune infiltration between RA and AS, and the analysis of six hub genes and three immune cells profile might provide new insights into potential pathogenesis therapeutic direction of RA complicated with AS.
Introduction
Rheumatoid arthritis (RA) is a chronic systemic inflammatory disorder characterized by articular and extra-articular involvement, with a global prevalence of 0.24% (1, 2). RA patients were reported to have higher cardiovascular morbidity and mortality (3, 4). Atherosclerosis (AS) is defined as the formation of fibrofatty lesions in the artery wall, commonly found in the cardiovascular and cerebrovascular systems, that has been the major cause of disability and death worldwide (5, 6). Clinic evidence has shown that patients with RA had a 68% increased risk of myocardial infarction (MI), and the risk of developing silent MI was twofold higher in RA patients compared with normal (7, 8). Even in groups without a history of coronary heart disease, patients with RA had more coronary plaques and were more likely to form vulnerable plaques (7, 9, 10). The association between the two diseases has not been only reported from a clinical perspective. Nearly 10 years of research have also found that RA and AS have similar pathological processes and shared risk factors (11, 12), the most pivotal among which are chronic inflammation and immune activation (13, 14). Innate and adaptive immune system activation promotes higher cumulative inflammation that involves cytokines, immune cells, and non-immune cells, such as tumor necrosis factor-α (TNF-α) and interleukin-6 (IL-6) production, T and B cells activation, and the increase in epithelial cells, fibroblasts, and smooth muscle cell, etc. (11, 15, 16). In addition, chronic inflammation not only increases the traditional risk factors but also interacts with their mechanisms (11). The above-complicated biological process leads to endothelial dysfunction, arterial stiffness, and atherosclerotic plaque formation and progression, ultimately accelerating the atherogenic process in RA (12, 13, 17, 18). However, the exact biological pathway and molecular mechanism behind the above-mentioned biological process between RA and AS is still far from being elucidated. Meanwhile, some biological medications, such as anti-TNF medications and IL-1 inhibitors, have been reported to improve vascular function and reduce the risk of MI by easing the inflammatory burden to some extent (9, 19, 20). Hence, there is an urgent need to uncover more and exact biological targets in patients with RA to prevent AS occurrence and development.
Microarray technology is an effective analysis that is widely adopted to compare genes that are differentially expressed in biological models or patients under different diseases state (21, 22). Microarray technology is also used to better understanding gene association, mapping, expression, and linkage studies. At present, some articles have analyzed the RA or AS datasets from a single disease perspective, and dig out the potential targets and regulatory biological pathway of RA or AS respectively (21, 23). These studies provide new insights and research directions for single diseases of RA or AS, yet there is still a lack of studies to systematically explore the commonality of RA and AS and provide exact research directions. The common transcription signatures may indicate new insights into the common pathogenesis and immune mechanism of RA and AS. Hence, the purpose of this research was to uncover the biological mechanism and relevant immune pathway of RA complicated with AS, and more significantly, to explore the potential biomarkers and therapeutic directions of the two diseases. In this study, GSE55457 and GSE100927 were downloaded from the Gene Expression Omnibus (GEO) database to identify the communal differentially expressed genes (DEGs) between RA and AS. Subsequently, we construct the protein-protein interaction (PPI) network of communal DEGs to determine hub genes via STRING database and Cytoscape software. Comprehensive bioinformatics analysis, including functional annotation, co-expression analysis, expression validation, drug-gene prediction, and TF-mRNA-miRNA regulatory network construction, was performed to uncover the biological characteristic of hub genes. Additionally, we analyzed and compared the immune infiltration of RA and AS based on the CIBERSORT algorithm and evaluated the correlation between hub genes and infiltrating immune cells. Finally, a total of 66 communal DEGs, six hub genes, and three infiltrating immune cells that might indicate new insights into the relevant immune mechanisms and therapeutic directions of RA complicated with AS, were identified.
Materials and methods
Data source
GEO (http://www.ncbi.nlm.nih.gov/geo) is a microarray and high-throughput sequencing database created by NCBI (24). RA and AS were used as keywords to screen for qualified gene datasets based on strict inclusion/exclusion criteria. The inclusion criteria were as follows: (1) Sporadic RA or AS; (2) datasets containing patients and healthy controls and including the largest possible sample size; (3) the test specimens in datasets derived from human tissues. Exclusion criterium was: patients who participated in a clinical trial for drugs or other treatments. After filtering and comparing, four gene datasets were chosen from GEO based on strict inclusion/exclusion criteria. GSE55457 (13 RA patients and 10 controls) and GSE55235 (10 RA patients and 10 controls) were screened as RA datasets (25). Meanwhile, in the AS group, GSE100927 (69 AS human tissues and 35 controls) and GSE28829 (13 early atherosclerotic plaques and 16 advanced atherosclerotic plaques) were selected from GEO (26, 27). GSE55457 and GSE100927 were used to screen DEGs and perform Immune Infiltration analysis, while GSE55235 and GSE28829 were used for hub gene expression validation.
Identification and enrichment analyses of overlapped DEGs
GEO2R (www.ncbi.nlm.nih.gov/geo/ge2r) is a network tool that works via the Limma package and GEOquery (28). DEGs of RA and AS were respectively identified using GEO2R with the condition of P-value < 0.05 and |logFC| > 1. After that, the overlap DEGs between RA and AS were detected and visualized by constructing VENN diagrams.
Based on the DAVID database (https://david.ncifcrf.gov/), Gene Ontology (GO) enrichment was conducted to analyze the biological characteristics of the overlap DEGs at the functional and molecular levels (29). The enrichment results are sorted separately by P-value and gene count in order to reveal the more meaningful biological processes. In addition, we performed pathway enrichment analysis of overlap DEGs from five pathway databases (KEGG PATHWAY, PID, BioCyc, Reactome and Panther) via network platform KOBAS 3.0 (30). P-value < 0.05 was considered statistically significant.
PPI network construction, hub genes selection and analyses
A PPI network could reveal protein interactions and unearth the core protein genes. Upon the condition of interaction combined score > 0.4, the PPI network of overlap DEGs was constructed based on the STRING database (http://string-db.org) and visualized using Cytoscape software (31, 32).
CytoHubba is a Cytoscape plugin that could calculate the core protein genes of the PPI network (33). There are up to 12 algorithms in CytoHubba, all of which are already proven to be effective in screening hub genes. Five of the 12 algorithms were selected randomly in CytoHubba to calculate the top 10 core genes and determine the hub genes by taking the intersection of five algorithms running outcomes.
Metascape (https://metascape.org) platform was adopted here to perform functional annotation analysis of hub genes, and further pathway enrichment was performed by KOBAS 3.0 (34) from five above-mentioned pathway databases. Subsequently, we constructed and analyzed the co-expression network of hub genes via the GeneMANIA (http://www.genemania.org/) platform which is a reliable network tool for analyzing gene list function and exploring internal associations (35). Additionally, we predicted the drug-gene pairs of hub genes using DGIdb 3.0 (http://www.dgidb.org) database based on the condition that a predicted drug was FDA-approved (36). After the prediction, the network map of drug-gene pairs was visualized via Cytoscape software.
Validation of hub gene expression
The expression of hub genes was verified in GSE55235 (10 RA patients and 10 controls) and GSE28829 (13 early atherosclerotic plaques and 16 advanced atherosclerotic plaques) respectively, and the comparison between the two sets of data was conducted with the T-test. A P value of < 0.05 was considered statistically significant.
Construction of the TF-mRNA–miRNA regulatory network
Mirwalk is a credible database basically focused on miRNA-target interactions (37). In this research, we predicted the miRNA of hub genes via the Mirwalk database under the strict condition that the predicted interactions could be verified by experiments or other databases. Transcriptional Regulatory Relationships Unraveled by Sentence-based Text mining (TRRUST) database consisting of the target genes corresponding to TFs and the regulatory relationships between TFs, was adopted to predict the TFs that regulate the hub genes (38). After the prediction, the regulatory network of TF-mRNA-miRNA was constructed and visualized using Cytoscape software.
Evaluation of immune cell infiltration and correlation analysis
On the ground of the CIBERSORT algorithm, we analyzed the relative percentage of 22 immune cell subpopulations in AS and control samples from the GSE100927. Besides, violin diagrams were generated to compare and visualize the difference in the immune cell between AS and control via the ggplot2 package. Consistent with the GSE100927, the percentage of immune cells and the difference between RA and control from GSE55457 were analyzed and visualized in the same way.
Person correlation analysis was carried out on hub genes and infiltrating immune cells by the ggstatsplot package, and the results were visualized by ggplot2 package.
Result
Identification and enrichment analyses of overlapped DEGs
The research design flow chart is shown in Figure 1. After standardizing the microarray results, DEGs of RA and AS were screened respectively. In the RA group, there were 1163 genes (447 upregulated and 716 downregulated genes) in GSE55457 identified as DEGs (Figure 2A). Meanwhile, 565 genes (418 upregulated and 147 downregulated genes) in GSE100927 were screened as DEGs in AS patients compared with controls (Figure 2B).After taking the intersection of the DEGs in GSE100927 and GSE55457, we obtained the communal DEGs (54 upregulated and 12 downregulated genes) between RA and AS (Figures 2C, D).
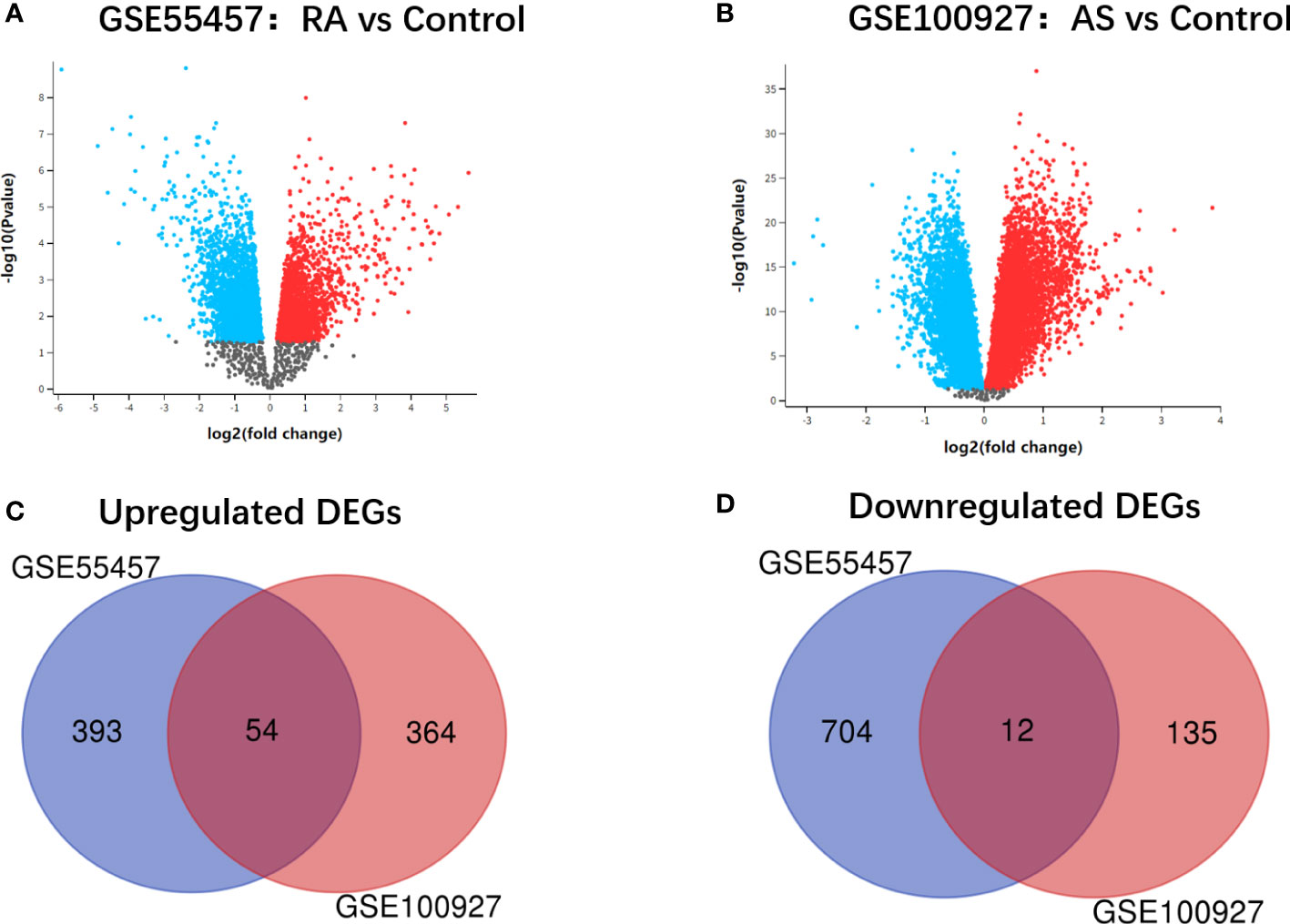
Figure 2 (A) Volcano plot of GSE55457. (B) Volcano plot of GSE100927. Upregulated genes are marked in red; downregulated genes are marked in bule. (C) Venn diagram of the 54 upregulated communal DEGs between RA and AS. (D) Venn diagram of the 12 downregulated communal DEGs between RA and AS.
We performed GO enrichment analysis using the David database to unearth the biological characteristic of the communal DEGs, and the result was divided into three functional parts consisting of biological processes (BP), cell component (CC), and molecular function (MF) (Figures 3A, B). The enrichment results of the overlap DEGs are mainly ranked in terms of enrichment P-value in three functional parts respectively. In the BP category, DEGs were mainly enriched in immune response (GO:0006955), positive regulation of T cell proliferation (GO:0042102), chemotaxis (GO:0006935) and positive regulation of T cell activation (GO:0050870). Regarding the CC category, DEGs were significantly involved in external side of the plasma membrane (GO:0009897), immunological synapse (GO:0001772), cell surface (GO:0009986) and clathrin-coated endocytic vesicle membrane (GO: 0030669). As for the MF category, DEGs were significantly involved in C-C chemokine receptor activity (GO:0016493), MHC class II protein complex binding (GO:0023026), CCR chemokine receptor binding (GO:0048020) and interleukin-7 receptor activity (GO: 0004917). Moreover, according to KOBAS 3.0, the pathway enrichment analysis of overlapped DEGs showed that the genes were mainly enriched in the Immune System, Cytokine Signaling in the Immune system, Cytokine-cytokine receptor interaction(Figure 3C).
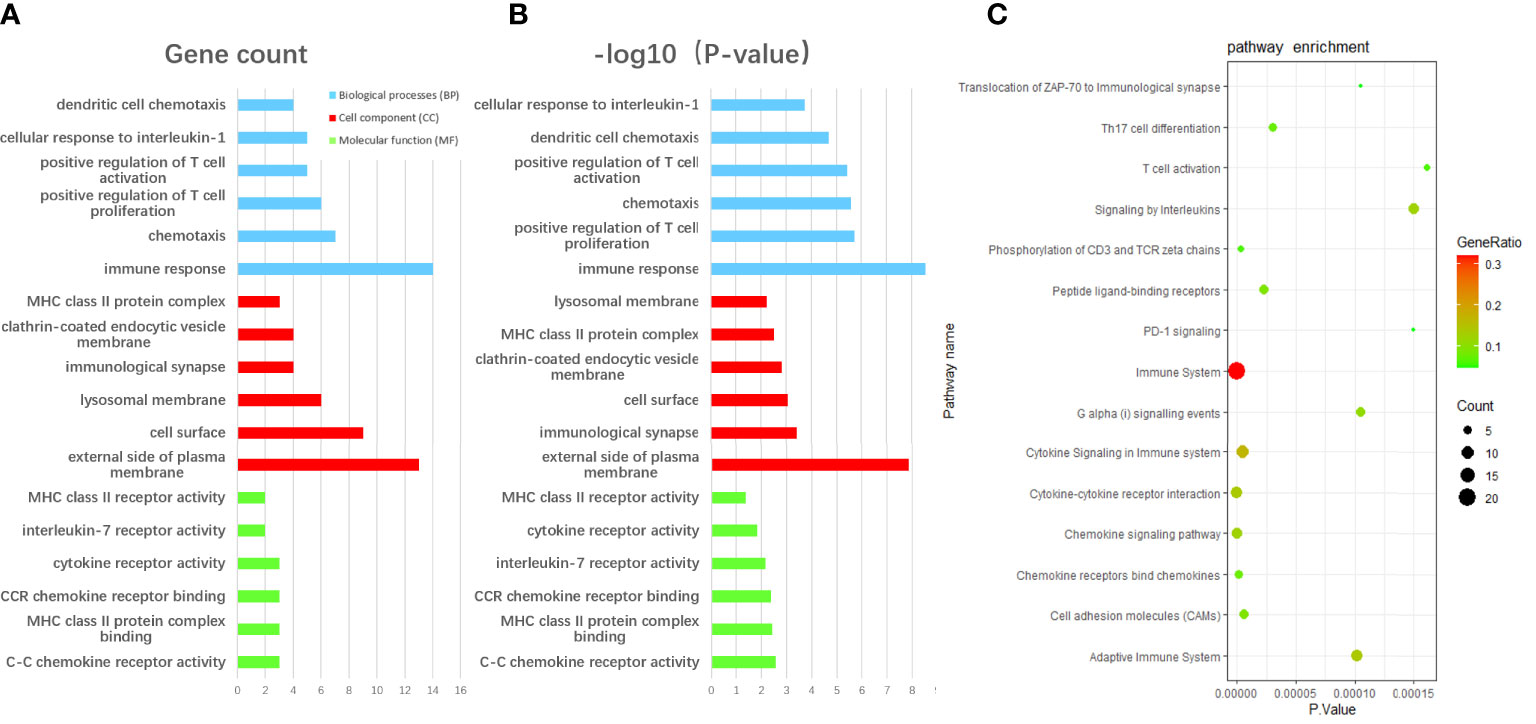
Figure 3 The enrichment analysis of communal DEGs between RA and AS. (A) The GO enrichment analyses of communal DEGs with gene count and (B) P-value based on the DAVID database. (C) The pathway enrichment analysis of communal DEGs on the ground of KOBAS 3.0. P-value < 0.05 was considered significant.
PPI network construction and hub gene selection
On the ground of the STRING database, the PPI network of overlap DEGs was constructed using Cytoscape with the condition of combined scores of >0.4 points, consisting of 45 nodes and 162 edges (Figure 4). After that, five algorithms (Degree, EPC, Radiality, Stress, and Betweenness) in the CytoHubba plugin were selected to identify hub genes. The top 10 genes calculated by the five above-mentioned algorithms are listed in Table 1. Finally, by taking the intersection of the top 10 genes in five algorithms, six central genes (CCR5, CCR7, IL7R, PTPRC, CD2, and CD3D) were determined as hub genes (Figure 5). All six hub genes were upregulated genes.
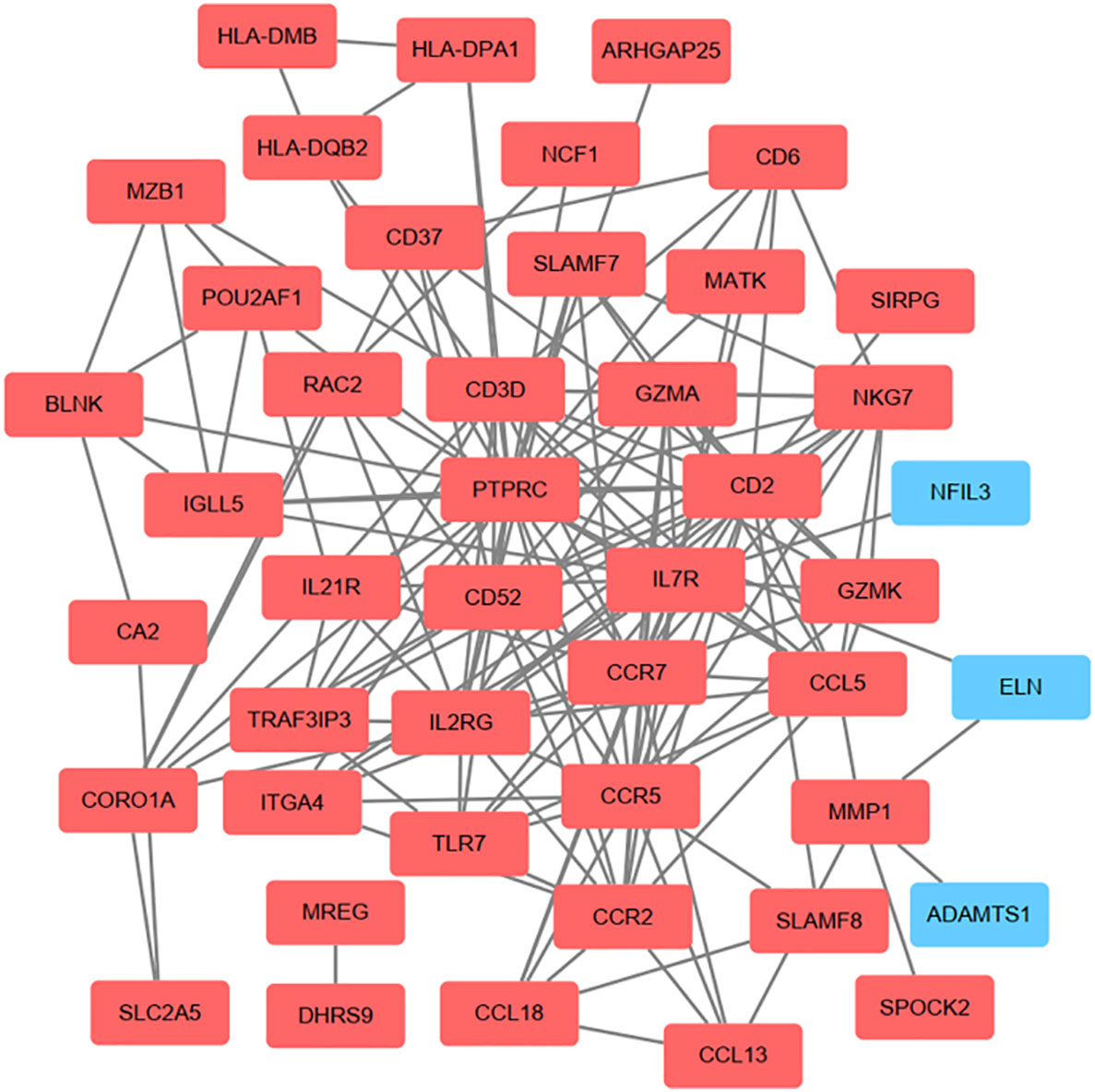
Figure 4 On the ground of STRING database, PPI networks of the communal DEGs were constructed by Cytoscape software. The red round rectangle represents upregulated genes and the blue round rectangle represents downregulated genes.
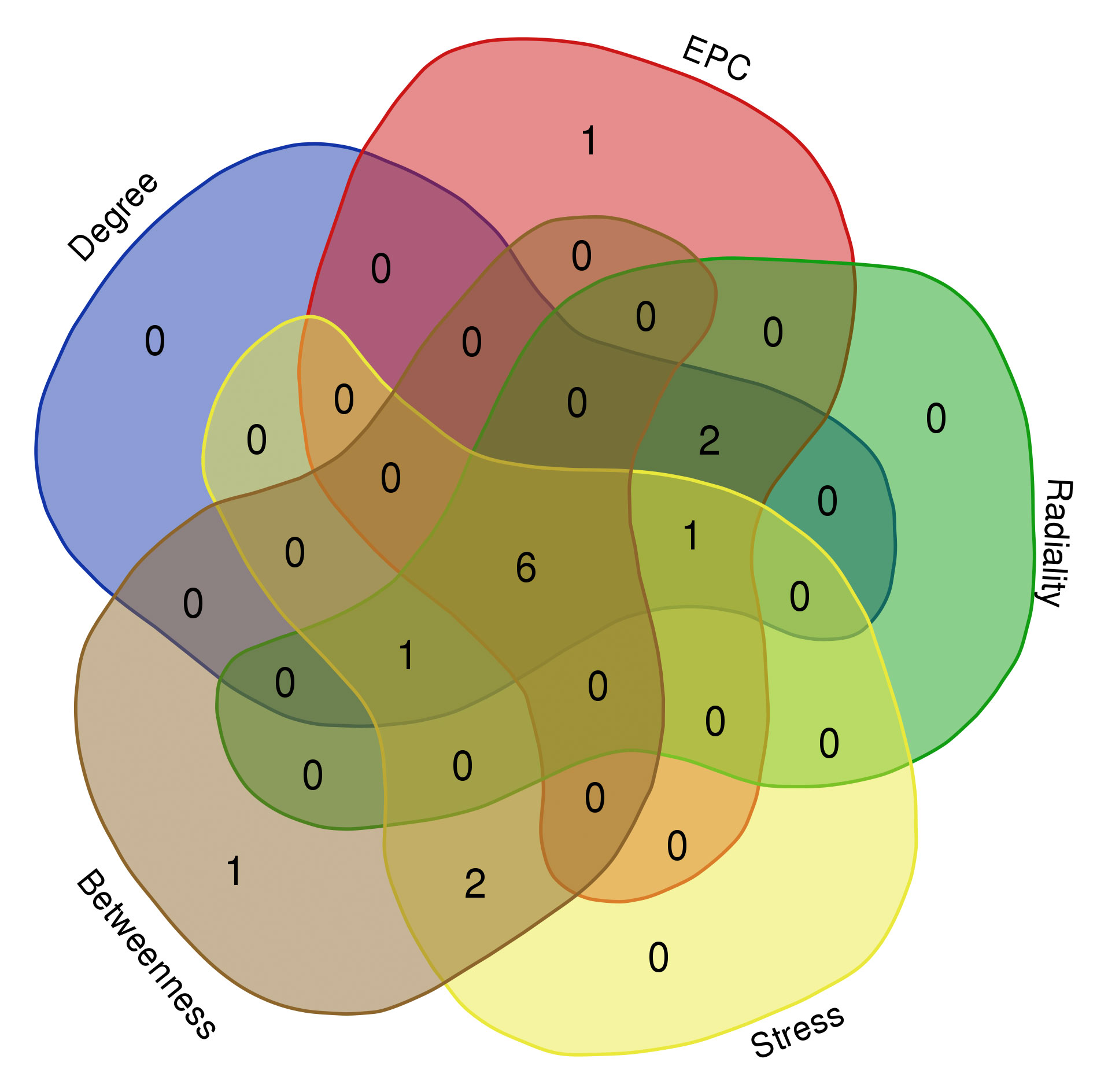
Figure 5 Six hub genes were determined by taking the intersection of the first 10 genes in the five algorithms of cytoHubba plugin (Degree, EPC, Radiality, Stress, and Betweenness).
Hub gene analyses and validation
We conducted the functional annotation analysis of hub genes via Metascape to better uncover the biological characteristics and mechanism of the six hub genes (Figure 6A). Hub genes were mainly involved in T cell activation (GO:0042110) and thymic T cell selection (GO:0045061). Besides, further pathway enrichment analysis was performed by KOBAS 3.0, and hub genes were significantly involved in the Immune System, Primary immunodeficiency, and Cytokine-Cytokine receptor interaction (Figure 6B). Similar to the analysis outcome of overlap DEGs, the gene annotation analysis revealed that hub genes were associated with the process of the immune system reacting. Subsequently, the network of the hub genes and their co-expression genes were generated on the ground of the GeneMANIA platform (Figure 6C). Six hub genes showed the complex PPI network with the Co-expression of 69.90%, Physical interactions of 10.20%, Pathway of 9.28%, Co-localization of 5.83%, Shared protein domains of 3.22% and Predicted of 1.57%. As expected, the biological function and roles of the hub genes re-emphasized the importance of the immune system in RA and AS. In addition, on the grounds of the DGIdb database, 15 drug-gene pairs were obtained with the condition that the drug should be FDA-approved, including 4 hub genes (PTPRC, CD3D, CCR5 and CD2) and 15 drugs. The categories of predicted drug are varied and all are FDA-approved drugs. Among them, there were a total of 10 potential drugs that could have effect on PTPRC, yet no drug was found to interact with multiple genes at the same time. These outcomes might reveal clues of potential therapeutic direction (Figure 7).
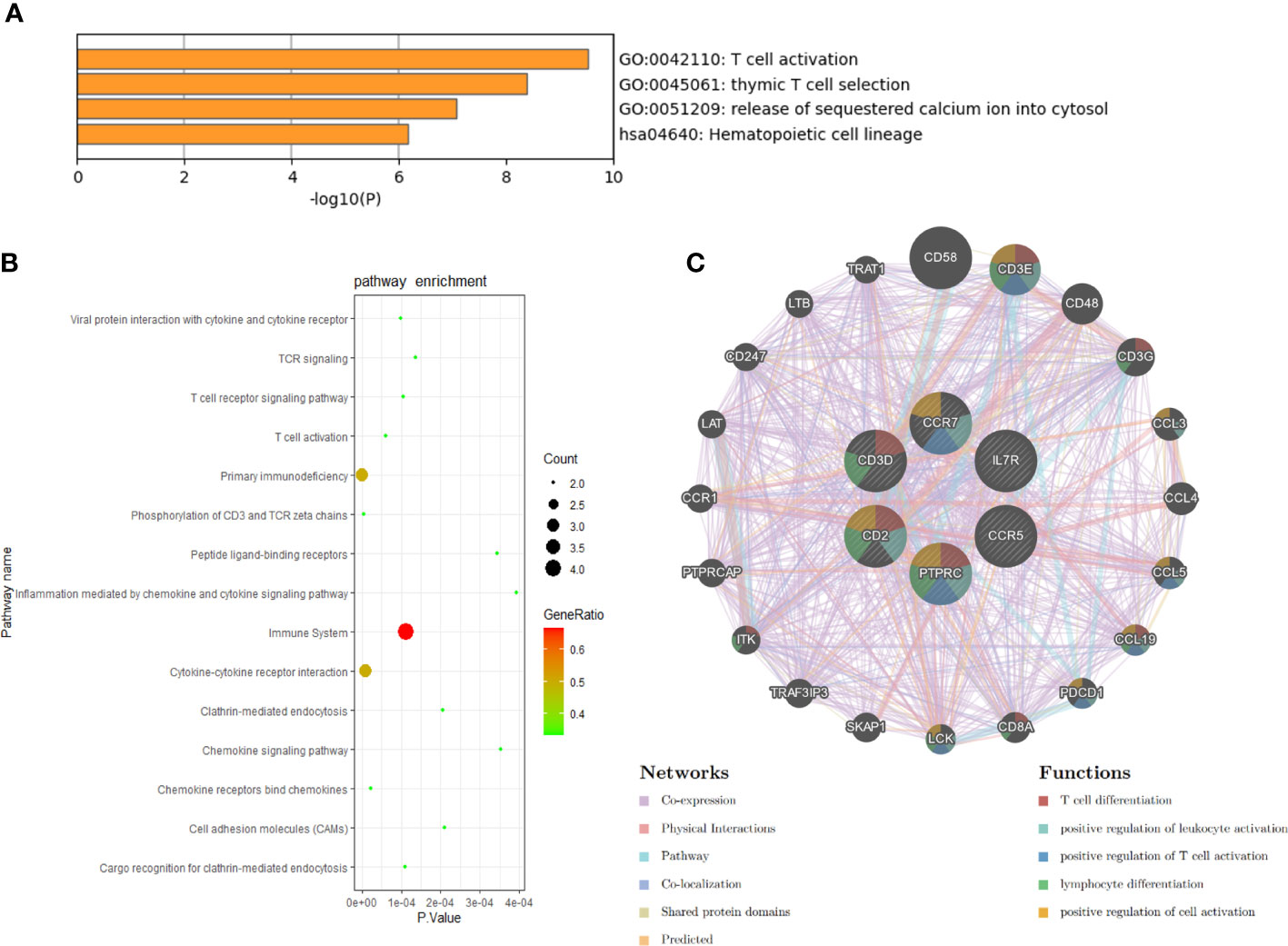
Figure 6 Biological process, pathway, and co-expression analysis of the hub genes. (A) The functional annotation analysis of hub genes via Metascape. (B) The further pathway enrichment analysis of hub genes by KOBAS 3.0. P-value < 0.05 was considered significant. (C) The network of hub genes and their co-expression genes were constructed and analyzed by GeneMANIA.
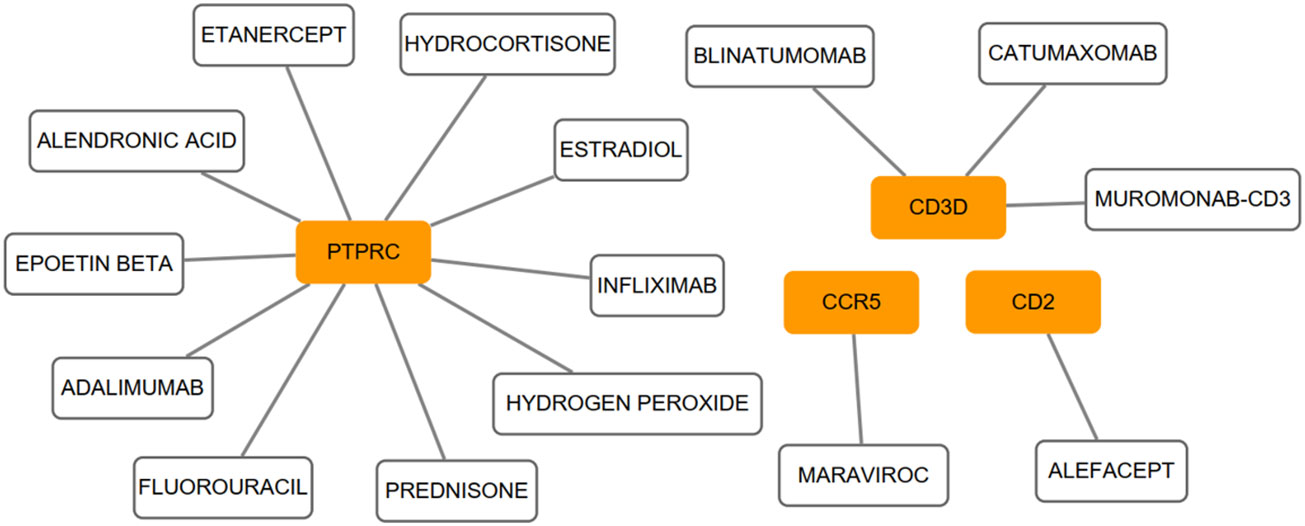
Figure 7 On the ground of the DGIdb database, the drug-gene pairs network was generated via Cytoscape, consisting of 4 hub genes (PTPRC, CD3D, CCR5 and CD2) and 15 drugs. Yellow circle indicates the hub gene and blank square indicates the drug.
In order to ensure the reliability and accuracy of bioinformatics analysis results, GSE55235 and GSE28829 were adopted to verify the expression of hub genes in RA and AS samples by independence testing analysis respectively (Figure 8). Encouragingly, compared with controls, all 6 hub genes were significantly upregulated in AS and RA samples.
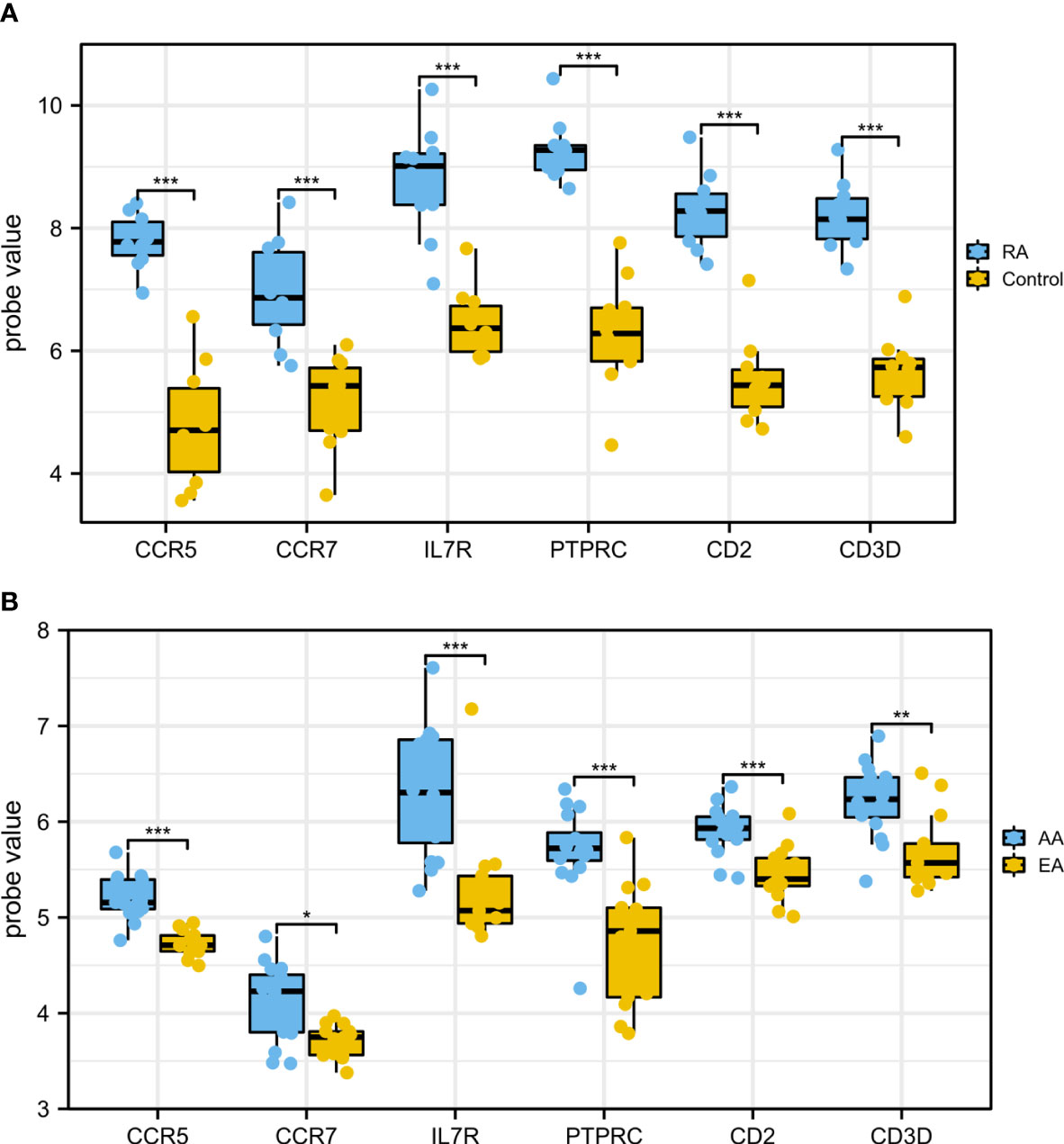
Figure 8 The expression of hub genes in (A) GSE55235 and (B) GSE28829. The comparison between the two datasets used the mean t-test. P < 0.05 was considered to be statistically significant. EA, early atherosclerotic plaques; AA, advanced atherosclerotic plaques. *P < 0.05; **P < 0.01; ***P < 0.001.
Construction of the TF-mRNA–miRNA regulatory network
With the strict condition predicting that miRNA of hub genes could be verified by experiments or other databases, a total of 162 miRNAs were screened based on the MiRwalk database. Meanwhile, on the ground of the TRRUST database predictions of six hub genes, up to 13 TFs that could regulate hub genes were obtained. Then, based on the outcome of the prediction, the regulatory network of TF-mRNA-miRNA was constructed using Cytoscape software (Figure 9).
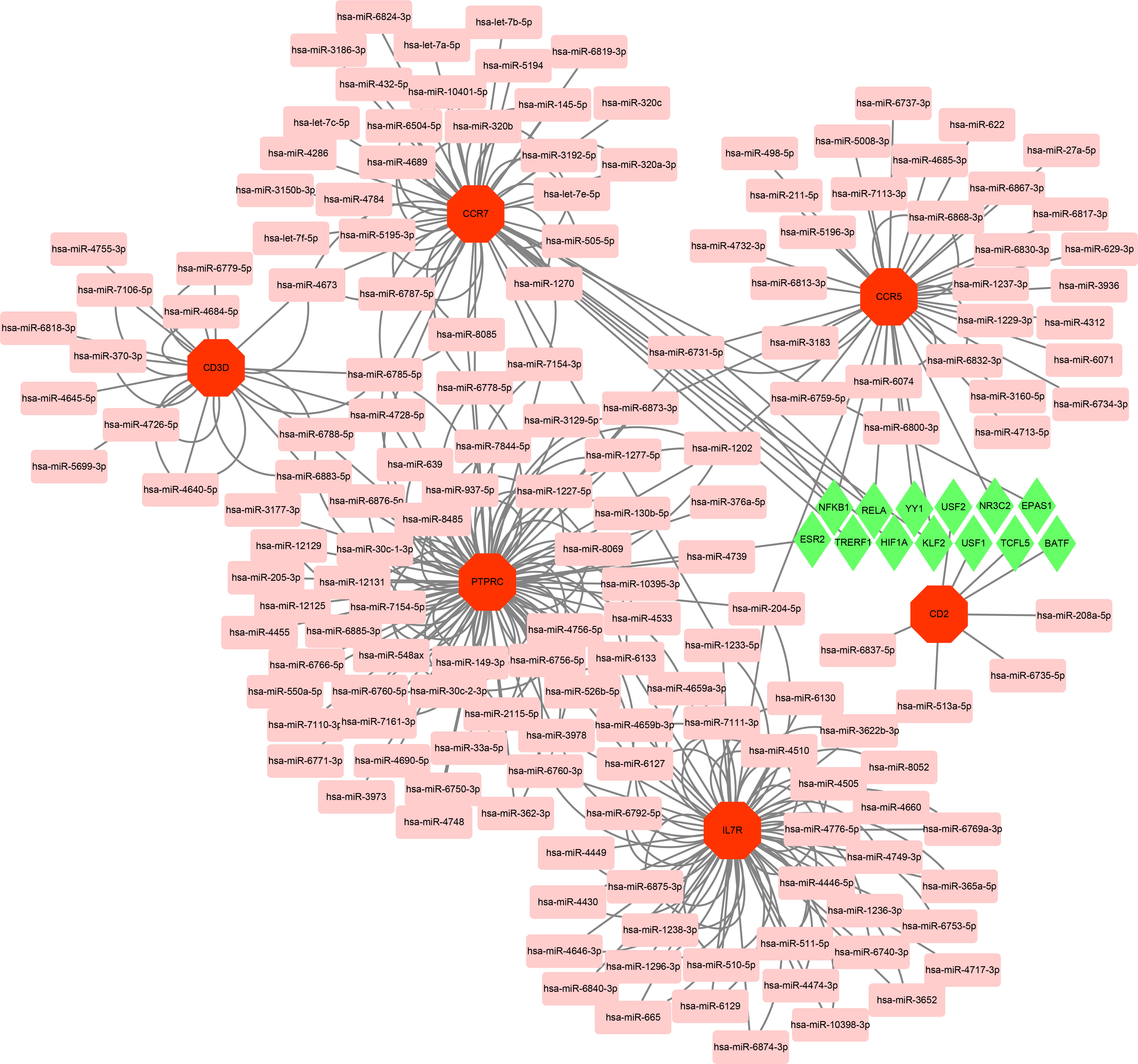
Figure 9 Based on MiRwalk and TRRUST database, TF-mRNA–miRNA regulation network of hub genes was constructed using Cytoscape. Hub genes were marked in red octagon; miRNAs were marked in pink round rectangle; TFs were marked in green diamond.
Evaluation of immune cell infiltration
Through the CIBERSORT algorithm, the relative percentage of 22 immune cell subpopulations analysis was conducted in GSE100927 and GSE55457 respectively (Figures 10A, 11A). Subsequently, we analyzed 22 immune cell subgroups difference between disease samples and control samples. In terms of AS, there were more memory B cells, CD4+ naïve T cells, γδT cells, follicular helper T cells, M0 macrophages, and mast cells activated in AS tissue compared to control tissue, but fewer B naïve cells, plasma cells, CD4+ activated memory T cells, monocytes, M1 macrophages, M2 macrophages, activated dendritic cells, and resting mast cells (Figure 10B). As for RA, the violin chart showed that compared with the normal control sample, there were more memory B cells, plasma cells, CD8+ T cells, follicular helper T cells, γδT cells, and M1 macrophages in the RA samples, but fewer CD4+ resting memory T cells, activated NK cells, and resting dendritic cells (Figure 11B). Comprehensive analysis of immune infiltration outcome revealed that upregulated memory B cells, follicular helper T cells, and γδT cells could be the common immune process and mechanism between RA and AS.
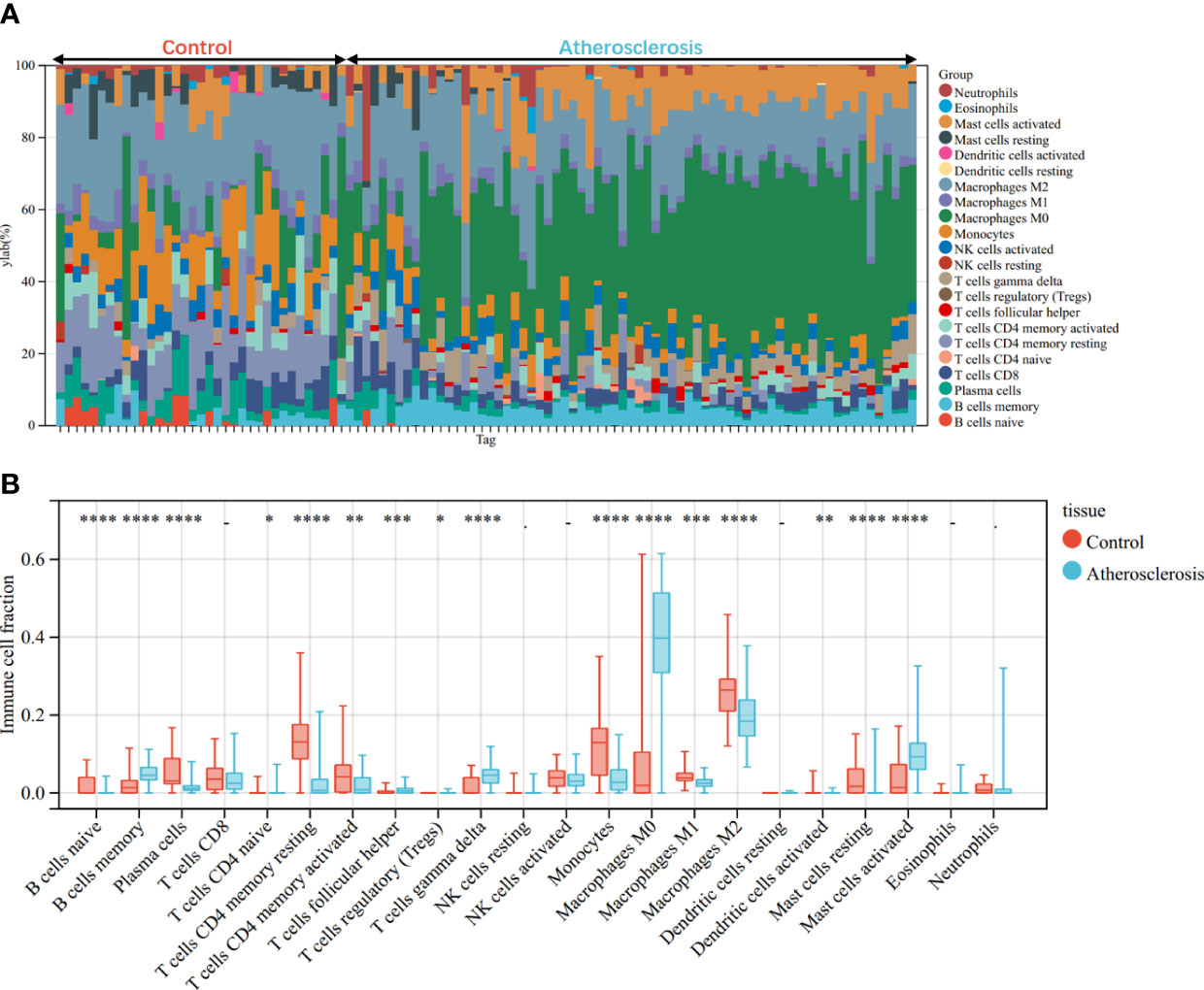
Figure 10 Immune cell infiltration mode in AS. (A) The relative percentage of 22 immune cell subpopulations out of 104 samples from the GSE100927. (B) The ratio of 22 immune cells between AS and control in GSE100927. *p < 0.05; **p < 0.01; ***p < 0.001; ****p < 0.0001.
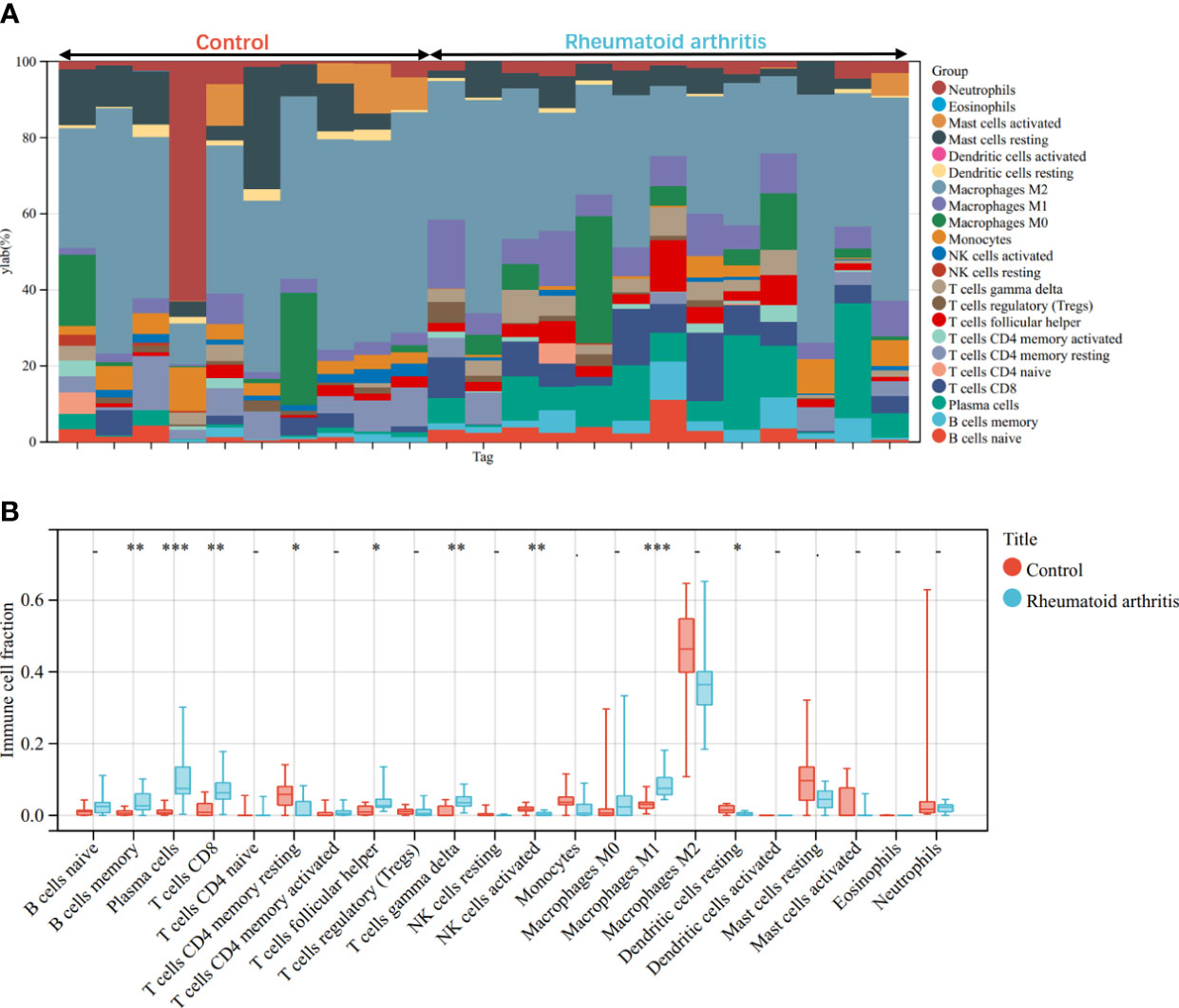
Figure 11 Immune cell infiltration mode in RA. (A)The relative percentage of 22 immune cell subpopulations out of 23 samples from the GSE55457. (B) The ratio of 22 immune cells between RA and control in GSE55457. *p < 0.05; **p < 0.01; ***p < 0.001.
Correlation analysis of hub genes and infiltrating immune cells
The correlation heatmap of 22 types of immune cells showed that γδT cells had a significant positive correlation with follicular helper T cells and memory B cells, but the correlation between memory B cells and follicular helper T cells is statistically insignificant in AS (Figure 12A). As for RA, the correlations between γδT cells, follicular helper T cells and memory B cells were all significantly positive (Figure 13A).
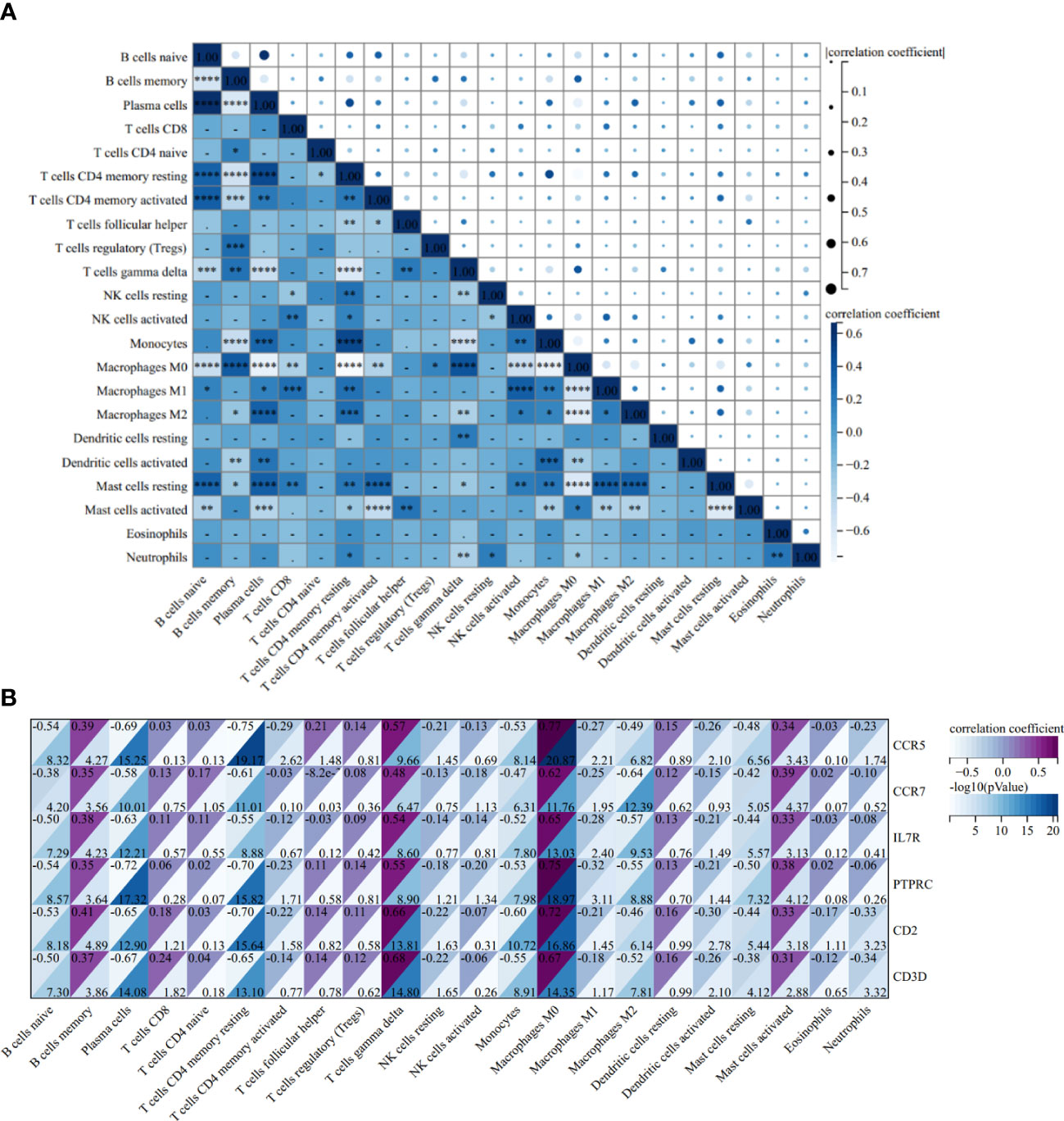
Figure 12 Correlation Analysis in AS. (A) Related heat maps of 22 immune cells in GSE100927. (B) Correlation heat map between hub genes and immune cells in GSE100927. *p < 0.05; **p < 0.01; ***p < 0.001, ****p < 0.0001.
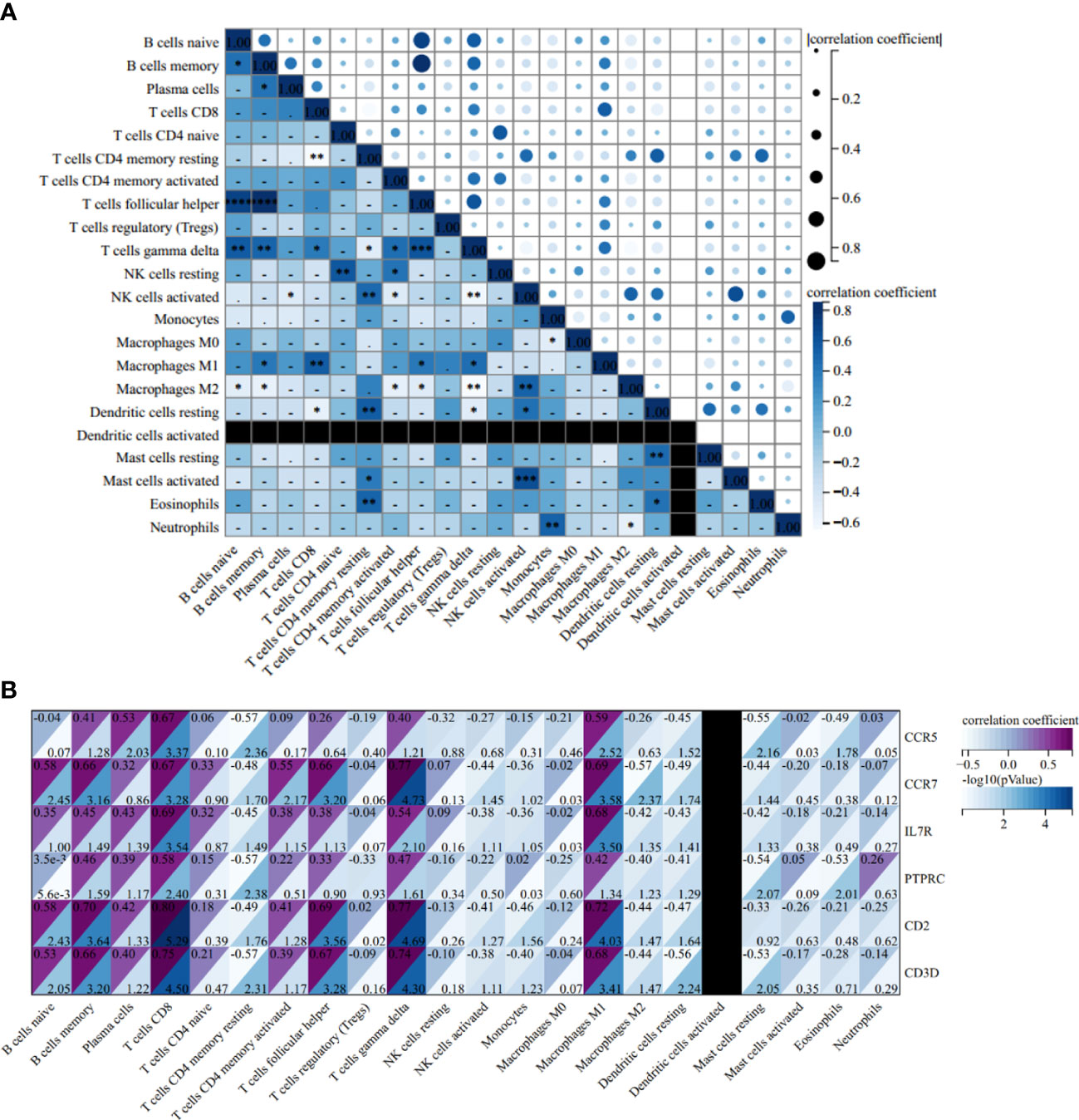
Figure 13 Correlation Analysis in RA. (A) Related heat maps of 22 immune cells in GSE55457. (B) Correlation heat map between hub genes and immune cells in GSE55457. *p < 0.05; **p < 0.01; ***p < 0.001.
In the AS group, the correlation analysis showed that all six hub genes were positively correlated with memory B cells and γδT cells, yet only CCR5 was positively correlated with follicular helper T cells. Additionally, and the correlation between other hub genes and follicular helper T cells was statistically insignificant (Figure 12B). As for RA, CCR7, IL7R, PTPRC, CD2, and CD3D were positively correlated with memory B cells and γδT cells, and CCR7, CD2, and CD3D were positively correlated with follicular helper T cells (Figure 13B).
Discussion
In this study, we identified 54 upregulated and 12 downregulated communal DEGs in RA and AS. Enrichment analyses showed that these genes were significantly involved in the immune system and related immune signaling pathways. Subsequently, six hub genes (CCR5, CCR7, IL7R, PTPRC, CD2, and CD3D) were determined in the PPI network based on five algorithms (Degree, EPC, Radiality, Stress, and Betweenness) in the Cytohubba plugin. The function annotation analysis of hub genes and analysis of the co-expression network re-emphasized the importance of the immune system in RA and AS. Besides, we also predicted the drug-gene pairs and constructed the TF-mRNA-miRNA regulatory network to further elucidate the potential biological role of hub genes. Finally, the immune infiltration of RA and AS was analyzed and compared based on the CIBERSORT algorithm, and the correlation between hub genes and infiltrating immune cells was evaluated to reveal the relevant immune mechanisms.
Based on the results of the immune infiltrating landscape between AS and RA, we can dig out that the B memory cells, γδT cells, and follicular helper T cells were both higher in the disease group. Thus, we hypothesize that the pathogenesis between AS and RA shared some commonness, which was correlated with memory B cells and γδT cells. B cells play an essential role in RA pathogenesis. On the one hand, B cells can locally infiltrate the affected joints synovial membranes with autoantibodies, such as the rheumatoid factor (RF) and anti-cyclic citrullinated peptide (ACPA) (39). On the other hand, B cells serve as the antigen presentation cell, various inflammatory cytokines, and chemokines producers and CD4+T cells co-stimulator (40–42). However, B cells in the RA patient synovial tissue express memory B cell marker CD27 rather than naïve B-cell markers (43). Moreover, RA patients have an expansion of memory B cell subsets at the time of clinical episodes, which has been associated with worse long-term clinical outcomes (44). For the AS, both cellular and humoral B cell immunity play an important role in atherosclerotic plaque formation (45). Clinical studies have demonstrated a negative association between atherosclerotic outcomes and the unswitched memory B cells, which mainly express immunoglobulin (Ig)M antibodies. Interestingly, the switched memory cells mainly express IgG or IgA antibodies, and univariate analysis uncovered that serum level of IgG has been positively associated with atherosclerosis (46). Additionally, Hamze et al. has identified that B cells secreting IgG and IgA are present in the human atherosclerotic plaques located in the vascular wall (47). However, the characteristics of memory B cells in AS are intricacy, and memory B cells may participate in AS and RA development. Different from B memory cells, there was still lack direct evidence of the position and role of γδT cells in two diseases. γδT cells, a subtype of T cell, are different from αβ T cells because they cannot recognize specific antigens (48). Despite little evidence on the role of γδT cells in AS, γδT cells could modulate AS via IL-17 production owing to it being a rich source of IL-17. IL-17 was known related to favoring plaque stability (49), and increased IL-17 is one of the key molecules in RA pathogenesis (50). Notably, studies have shown that γδT17 cells are the main innate cellular source of IL-17 in collagen-induced arthritis models (51, 52). Meanwhile, the number of γδT17 cells is equal to regular T-helper 17 (Th17) cells in mice synovium, and the proportion of γδT17 cells in the joints has been more dramatically increased than Th17 cells (53). Thus, the mechanism of γδT cells between RA and AS remains unclear, and more basic and clinical research is needed to understand the mechanism. In addition, correlation analysis indicated that all 6 hub genes are positively correlated with at least one communal high-expressed immune cell in both diseases. Meanwhile, both the functional annotation analysis of hub genes and analysis of co-expression network point to the immune system and associated biological pathway. Based on the above analysis, we speculate that hub genes may act as mediator in associated immune pathways and affect immune cell action.
CCR5, a seven-transmembrane-spanning G protein-coupled receptor, is the chemokine receptor for CCL3, CCL4, CCL5, CCL8 and CCL3L1 (54, 55). Strikingly, although CCR5 is predominantly expressed on stimulated macrophages, it is also expressed on osteoclasts and vascular smooth muscle cells (56, 57). In RA pathogenesis, CCR5 induces osteoclast formation to influence osteoclast function. Clinically, CCR5 loss function decreased incidence and/or severity of human RA (58–62), and CCR5 intervention has been negatively related to human RA (63). In AS animal models, inhibiting CCR5 has been identified as a safeguard for lesion size, macrophage infiltration and plaque stability (54). In clinical practice, CCR5Δ32 polymorphism, causing a truncated nonfunction receptor, has been demonstrated as a protective influence on the risk of cardiovascular disease (64). Therefore, CCR5 may be the risk factor for both RA and AS and is involved in both RA and AS development. CC-chemokine receptor 7 (CCR7), also a G protein receptor, is the sole receptor for CCL19 and CCL21 (65). In RA, CCL21 and CCR7 are highly co-expressed and play a role throughout RA pathogenesis (66). CCR7 is overexpressed on RA dendritic cells, which has been closely related to levels of RF and C-reactive protein (67). What’s more, CCR7 expression is also elevated in RA synovial tissue macrophages, fibroblasts, and endothelial cells (66, 68). Intriguingly, activated CCR7 in these cells can directly or indirectly facilitate RA angiogenesis (69). Meanwhile, for AS, CD68+ macrophages elevate CCR7 expression-promoted low-density lipoprotein (LDL) binding and foam cell formation in both asymptomatic and symptomatic carotid plaques (70). CCR7 plays a critical part in cell migration; therefore, the deficiency of CCR7 reduces atherosclerotic plaque content and disturbs the T cells entry or exit in the inflamed vessel wall (71). Nevertheless, considering the pleiotropic nature of CCR7 in RA and AS, therapy targeting CCR7 would be a potential strategy to ameliorate both RA and AS.
IL-7R, a heterodimeric complex, is a receptor for IL-2, IL-4, IL-7, IL-9, IL-15, and IL-21. Pickens et al. have discovered that the proportion of M1 macrophages is increased in RA synovial fluid, with elevated IL-7R expression (72). IL-7 provokes RA-naïve myeloid cells to remodel into M1 macrophages, which are known as pro-inflammatory immune cells. Meanwhile, IL-7 induces osteoclast formation in M1 macrophages that express high IL-7R levels and are more responsive than naïve and M2 macrophages (73). Moreover, IL-7R ligation by IL-7 can also maintain T cell homeostasis and promote T cell proliferation, selection, activation, and cytokine production. Consistently, blocking IL-7 or IL-7R function can attenuate collagen-induced arthritis monocyte recruitment and osteoclast differentiation (74). Orchestrally, IL-7R antibody treatment in ApoE-/- mice has significantly reduced monocyte/macrophage cell infiltration and lipid content in the atherosclerotic plaque (75). Hence, the increased IL-7R expression may be required for AS and RA, and IL-R blocking would be valuable to hold back the development of AS and RA.
PTPRC, also known as CD45, encodes a protein that is a member of the protein tyrosine phosphatase (PTP) family. PTPs are known to be signaling molecules that regulate various cellular processes, and has been confirmed to be an essential regulator of T-cell and B-cell antigen receptor signaling (76, 77). Previous numerous studies have reported that PTPRC was densely associated with the anti-TNF therapy response in RA patients, meaning that PTPRC could effectively predict and guide personalized medicine in RA therapy (78–80). Consistent with our result, Xia et al. have demonstrated that PTPRC could act as a regulatory T cell (Treg)-related gene in the progression of atherosclerosis in previous bioinformatics analysis (81), yet there is still a lack of directly experiment evidence that PTPRC is correlated with atherosclerotic plaque evolution. The protein encoded by CD2 is a surface antigen found on all peripheral blood T cells. CD2 interacts with LFA3 (CD58) on antigen-presenting cells to optimize immune recognition (82, 83). Based on the GRAIL2 computational method, CD2/CD58 has been predicted to be new RA risk loci (84). Meanwhile, Fernandez Lahore G et al. found that the expression of CD2 was obviously upregulated in RA synovial tissue compared with osteoarthritis or healthy synovium and speculated that CD2 is densely involved in joint inflammation and CD2 polymorphisms because affecting its expression led to the development or perpetuation of joint autoimmunity (85). The interaction between CD2 and CD58 could promote T cells and macrophages to secrete chemokines and cytokines establishing the inflammatory environment, resulting in the formation of atherosclerotic plaque in psoriasis patients, yet the role of CD2/CD58 in the communal pathogenesis of RA and AS still lacks relevant evidence and needs to be further explored (86). CD3D encodes a part of the T-cell receptor/CD3 complex that takes part in T-cell development and signal transduction (87). Nowadays, for the association between CD3 and two diseases only bioinformatic analysis evidence exists, and more experiments are needed to uncover and verify the potential mechanism (88).
At present, drug treatment options for RA contains nonsteroidal anti-inflammatory drugs (NSAIDs), corticosteroids, disease-modifying antirheumatic drugs (DMARDs) and biologics (2, 89, 90). Cause the heterogeneous factors and complex pathological mechanisms in RA, there are still many patients have an unsatisfactory or poor clinical response under above therapy (90). In addition to new biologics and DMARDs with low side effects keeping in continuous developing states, some novel therapy directions such as GPCR-targeted drug and RNA therapeutics are positively explored and developed (89, 91). The potential therapy target of RA complicated with AS could lies in above explored directions. On the ground of our analyze outcome, the functional annotation analysis of DEGs and hub genes both indicated the role of chemokine receptors in two diseases. Chemokine receptors are a class of GPCRs that regulate immunity, and two above-mentioned hub genes (CCR5 and CCR7) are one type of this class (92). Currently, chemokine receptors are the most intensively studied GPCRs in RA, and the two main drug strategies of RA in chemokines and chemokine receptors as follow: corresponding ligands that can be selected to inhibit chemokine receptors and direct inhibition of chemokine receptors (1, 89). Because the chemokine is widely expressed in numerus human cells and the expression of chemokine might have diverse functional roles at different disease stages of RA, the development of GPCRs was still full of challenge (89). Nowadays, the main aspect of RNA therapeutics is RNA interference (RNAi). RNAi is an endogenous mechanism of mRNA silencing involving miRNA, and siRNA-based Interventions have revealed promising prospective in treatment of RA (93, 94). Based on the MiRwalk database and TRRUST database, the TF-mRNA–miRNA regulatory network of hub genes was predicted and constructed and might provide the potential exploring clues for RNA therapeutics.
The highlight of this article is to explore the communal immune pathway and identify the hub gene and immune infiltration profiles in RA and AS. However, our research also had some limitations as follows: First, although the sample size was slightly large and the hub gene was successfully verified in other gene datasets, this article is a retrospective study that still requires external verification to further verify findings. Second, the drug-genes interaction pairs and TF-mRNA–miRNA regulatory network could provide the primary clues for further exploring core genes, yet the exact and truly association and effect still needs more experiment to uncover. Third, the function of hub genes and immune cells needs to be validated and further explored in in vitro and in vivo models. The above-described experiments will be the focus of our future work.
Conclusion
In summary, a total of 66 communal DEGs, six hub genes and three infiltrating immune cells were screened based on the comprehensive biological analysis. The evidence of overlapping pathogenesis between AS and RA points to relevant immune pathways, which might be mediated by specific hub genes and infiltrating immune cells. Furthermore, the analysis of these hub genes and immune cells may indicate new insights in potential therapeutic direction of RA complicated with AS.
Data availability statement
The datasets presented in this study can be found in online repositories. The names of the repository/repositories and accession number(s) can be found in the article/Supplementary Material.
Author contributions
All authors made substantial contributions to conception and design, acquisition of data, or analysis and interpretation of data; took part in drafting the article or revising it critically for important intellectual content; agreed to submit to the current journal; gave final approval of the version to be published; and agree to be accountable for all aspects of the work.
Conflict of interest
Author WS was employed by China National Nuclear Corporation 416 Hospital.
The remaining authors declare that the research was conducted in the absence of any commercial or financial relationships that could be construed as a potential conflict of interest.
Publisher’s note
All claims expressed in this article are solely those of the authors and do not necessarily represent those of their affiliated organizations, or those of the publisher, the editors and the reviewers. Any product that may be evaluated in this article, or claim that may be made by its manufacturer, is not guaranteed or endorsed by the publisher.
Supplementary material
The Supplementary Material for this article can be found online at: https://www.frontiersin.org/articles/10.3389/fimmu.2022.1013531/full#supplementary-material
References
1. Otón T, Carmona L. The epidemiology of established rheumatoid arthritis. Best Pract Res Clin Rheumatol (2019) 33(5):101477. doi: 10.1016/j.berh.2019.101477
2. Smolen JS, Aletaha D, McInnes IB. Rheumatoid arthritis. Lancet (2016) 388(10055):2023–38. doi: 10.1016/s0140-6736(16)30173-8
3. Aviña-Zubieta JA, Choi HK, Sadatsafavi M, Etminan M, Esdaile JM, Lacaille D. Risk of cardiovascular mortality in patients with rheumatoid arthritis: A meta-analysis of observational studies. Arthritis Rheum (2008) 59(12):1690–7. doi: 10.1002/art.24092
4. Wolfe F, Mitchell DM, Sibley JT, Fries JF, Bloch DA, Williams CA, et al. The mortality of rheumatoid arthritis. Arthritis Rheum (1994) 37(4):481–94. doi: 10.1002/art.1780370408
5. Milutinović A, Šuput D, Zorc-Pleskovič R. Pathogenesis of atherosclerosis in the tunica intima, media, and adventitia of coronary arteries: An updated review. Bosnian J Basic Med Sci (2020) 20(1):21–30. doi: 10.17305/bjbms.2019.4320
6. Libby P, Buring JE, Badimon L, Hansson GK, Deanfield J, Bittencourt MS, et al. Atherosclerosis. Nat Rev Dis Primers (2019) 5(1):56. doi: 10.1038/s41572-019-0106-z
7. Jagpal A, Navarro-Millán I. Cardiovascular Co-morbidity in patients with rheumatoid arthritis: A narrative review of risk factors, cardiovascular risk assessment and treatment. BMC Rheumatol (2018) 2:10. doi: 10.1186/s41927-018-0014-y
8. Avina-Zubieta JA, Thomas J, Sadatsafavi M, Lehman AJ, Lacaille D. Risk of incident cardiovascular events in patients with rheumatoid arthritis: A meta-analysis of observational studies. Ann Rheumatic Dis (2012) 71(9):1524–9. doi: 10.1136/annrheumdis-2011-200726
9. Adawi M, Firas S, Blum A. Rheumatoid arthritis and atherosclerosis. Israel Med Assoc Journal: IMAJ (2019) 21(7):460–3.
10. Humphreys JH, Warner A, Chipping J, Marshall T, Lunt M, Symmons DP, et al. Mortality trends in patients with early rheumatoid arthritis over 20 years: Results from the Norfolk arthritis register. Arthritis Care Res (2014) 66(9):1296–301. doi: 10.1002/acr.22296
11. Karpouzas GA, Bui VL, Ronda N, Hollan I, Ormseth SR. Biologics and atherosclerotic cardiovascular risk in rheumatoid arthritis: A review of evidence and mechanistic insights. Expert Rev Clin Immunol (2021) 17(4):355–74. doi: 10.1080/1744666x.2021.1899809
12. Skeoch S, Bruce IN. Atherosclerosis in rheumatoid arthritis: Is it all about inflammation? Nat Rev Rheumatol (2015) 11(7):390–400. doi: 10.1038/nrrheum.2015.40
13. Ozbalkan Z, Efe C, Cesur M, Ertek S, Nasiroglu N, Berneis K, et al. An update on the relationships between rheumatoid arthritis and atherosclerosis. Atherosclerosis (2010) 212(2):377–82. doi: 10.1016/j.atherosclerosis.2010.03.035
14. Hannawi S, Hannawi H, Al Salmi I. Cardiovascular disease and subclinical atherosclerosis in rheumatoid arthritis. Hypertension Res: Off J Japanese Soc Hypertension (2020) 43(9):982–4. doi: 10.1038/s41440-020-0483-4
15. Libby P. Role of inflammation in atherosclerosis associated with rheumatoid arthritis. Am J Med (2008) 121(10 Suppl 1):S21–31. doi: 10.1016/j.amjmed.2008.06.014
16. Pasceri V, Yeh ET. A tale of two diseases: Atherosclerosis and rheumatoid arthritis. Circulation (1999) 100(21):2124–6. doi: 10.1161/01.cir.100.21.2124
17. Kerekes G, Szekanecz Z, Dér H, Sándor Z, Lakos G, Muszbek L, et al. Endothelial dysfunction and atherosclerosis in rheumatoid arthritis: A multiparametric analysis using imaging techniques and laboratory markers of inflammation and autoimmunity. J Rheumatol (2008) 35(3):398–406.
18. Roman MJ, Devereux RB, Schwartz JE, Lockshin MD, Paget SA, Davis A, et al. Arterial stiffness in chronic inflammatory diseases. Hypertension (Dallas Tex: 1979) (2005) 46(1):194–9. doi: 10.1161/01.HYP.0000168055.89955.db
19. Ikonomidis I, Tzortzis S, Andreadou I, Paraskevaidis I, Katseli C, Katsimbri P, et al. Increased benefit of interleukin-1 inhibition on vascular function, myocardial deformation, and twisting in patients with coronary artery disease and coexisting rheumatoid arthritis. Circ Cardiovasc Imaging (2014) 7(4):619–28. doi: 10.1161/circimaging.113.001193
20. Daïen CI, Duny Y, Barnetche T, Daurès JP, Combe B, Morel J. Effect of tnf inhibitors on lipid profile in rheumatoid arthritis: A systematic review with meta-analysis. Ann Rheumatic Dis (2012) 71(6):862–8. doi: 10.1136/annrheumdis-2011-201148
21. Udhaya Kumar S, Thirumal Kumar D, Bithia R, Sankar S, Magesh R, Sidenna M, et al. Analysis of differentially expressed genes and molecular pathways in familial hypercholesterolemia involved in atherosclerosis: A systematic and bioinformatics approach. Front Genet (2020) 11:734. doi: 10.3389/fgene.2020.00734
22. Hephzibah Cathryn R, Udhaya Kumar S, Younes S, Zayed H, George Priya Doss C. A review of bioinformatics tools and web servers in different microarray platforms used in cancer research. Adv Protein Chem Struct Biol (2022) 131:85–164. doi: 10.1016/bs.apcsb.2022.05.002
23. Balasundaram A, Udhaya Kumar S, George Priya Doss C. A computational model revealing the immune-related hub genes and key pathways involved in rheumatoid arthritis (Ra). Adv Protein Chem Struct Biol (2022) 129:247–73. doi: 10.1016/bs.apcsb.2021.11.006
24. Edgar R, Domrachev M, Lash AE. Gene expression omnibus: Ncbi gene expression and hybridization array data repository. Nucleic Acids Res (2002) 30(1):207–10. doi: 10.1093/nar/30.1.207
25. Woetzel D, Huber R, Kupfer P, Pohlers D, Pfaff M, Driesch D, et al. Identification of rheumatoid arthritis and osteoarthritis patients by transcriptome-based rule set generation. Arthritis Res Ther (2014) 16(2):R84. doi: 10.1186/ar4526
26. Steenman M, Espitia O, Maurel B, Guyomarch B, Heymann MF, Pistorius MA, et al. Identification of genomic differences among peripheral arterial beds in atherosclerotic and healthy arteries. Sci Rep (2018) 8(1):3940. doi: 10.1038/s41598-018-22292-y
27. Döring Y, Manthey HD, Drechsler M, Lievens D, Megens RT, Soehnlein O, et al. Auto-antigenic protein-DNA complexes stimulate plasmacytoid dendritic cells to promote atherosclerosis. Circulation (2012) 125(13):1673–83. doi: 10.1161/circulationaha.111.046755
28. Barrett T, Wilhite SE, Ledoux P, Evangelista C, Kim IF, Tomashevsky M, et al. Ncbi geo: Archive for functional genomics data sets–update. Nucleic Acids Res (2013) 41(Database issue):D991–5. doi: 10.1093/nar/gks1193
29. Huang da W, Sherman BT, Lempicki RA. Systematic and integrative analysis of Large gene lists using David bioinformatics resources. Nat Protoc (2009) 4(1):44–57. doi: 10.1038/nprot.2008.211
30. Wu J, Mao X, Cai T, Luo J, Wei L. Kobas server: A web-based platform for automated annotation and pathway identification. Nucleic Acids Res (2006) 34(Web Server issue):W720–4. doi: 10.1093/nar/gkl167
31. Franceschini A, Szklarczyk D, Frankild S, Kuhn M, Simonovic M, Roth A, et al. String V9.1: Protein-protein interaction networks, with increased coverage and integration. Nucleic Acids Res (2013) 41(Database issue):D808–15. doi: 10.1093/nar/gks1094
32. Smoot ME, Ono K, Ruscheinski J, Wang PL, Ideker T. Cytoscape 2.8: New features for data integration and network visualization. Bioinf (Oxford England) (2011) 27(3):431–2. doi: 10.1093/bioinformatics/btq675
33. Chin CH, Chen SH, Wu HH, Ho CW, Ko MT, Lin CY. Cytohubba: Identifying hub objects and Sub-networks from complex interactome. BMC Syst Biol (2014) 8 Suppl 4(Suppl 4):S11. doi: 10.1186/1752-0509-8-s4-s11
34. Zhou Y, Zhou B, Pache L, Chang M, Khodabakhshi AH, Tanaseichuk O, et al. Metascape provides a biologist-oriented resource for the analysis of systems-level datasets. Nat Commun (2019) 10(1):1523. doi: 10.1038/s41467-019-09234-6
35. Warde-Farley D, Donaldson SL, Comes O, Zuberi K, Badrawi R, Chao P, et al. The genemania prediction server: Biological network integration for gene prioritization and predicting gene function. Nucleic Acids Res (2010) 38(Web Server issue):W214–20. doi: 10.1093/nar/gkq537
36. Cotto KC, Wagner AH, Feng YY, Kiwala S, Coffman AC, Spies G, et al. Dgidb 3.0: A redesign and expansion of the drug-gene interaction database. Nucleic Acids Res (2018) 46(D1):D1068–d73. doi: 10.1093/nar/gkx1143
37. Sticht C, de la Torre C, Parveen A, Gretz N. Mirwalk: An online resource for prediction of microrna binding sites. PloS One (2018) 13(10):e0206239. doi: 10.1371/journal.pone.0206239
38. Han H, Cho JW, Lee S, Yun A, Kim H, Bae D, et al. Trrust V2: An expanded reference database of human and mouse transcriptional regulatory interactions. Nucleic Acids Res (2018) 46(D1):D380–d6. doi: 10.1093/nar/gkx1013
39. Silverman GJ, Carson DA. Roles of b cells in rheumatoid arthritis. Arthritis Res Ther (2003) 5 Suppl 4(Suppl 4):S1–6. doi: 10.1186/ar1010
40. Scherer HU, Huizinga TWJ, Krönke G, Schett G, Toes REM. The b cell response to citrullinated antigens in the development of rheumatoid arthritis. Nat Rev Rheumatol (2018) 14(3):157–69. doi: 10.1038/nrrheum.2018.10
41. Marston B, Palanichamy A, Anolik JH. B cells in the pathogenesis and treatment of rheumatoid arthritis. Curr Opin Rheumatol (2010) 22(3):307–15. doi: 10.1097/BOR.0b013e3283369cb8
42. Dörner T. Crossroads of b cell activation in autoimmunity: Rationale of targeting b cells. J Rheumatol Supplement (2006) 77:3–11.
43. Cantaert T, Kolln J, Timmer T, van der Pouw Kraan TC, Vandooren B, Thurlings RM, et al. B lymphocyte autoimmunity in rheumatoid synovitis is independent of ectopic lymphoid neogenesis. J Immunol (Baltimore Md: 1950) (2008) 181(1):785–94. doi: 10.4049/jimmunol.181.1.785
44. Silverman GJ, Pelzek A. Rheumatoid arthritis clinical benefits from abatacept, cytokine blockers, and rituximab are all linked to modulation of memory b cell responses. J Rheumatol (2014) 41(5):825–8. doi: 10.3899/jrheum.140022
45. Srikakulapu P, McNamara CA. B cells and atherosclerosis. Am J Physiol Heart Circulatory Physiol (2017) 312(5):H1060–h7. doi: 10.1152/ajpheart.00859.2016
46. Tsimikas S, Brilakis ES, Lennon RJ, Miller ER, Witztum JL, McConnell JP, et al. Relationship of igg and igm autoantibodies to oxidized low density lipoprotein with coronary artery disease and cardiovascular events. J Lipid Res (2007) 48(2):425–33. doi: 10.1194/jlr.M600361-JLR200
47. Hamze M, Desmetz C, Berthe ML, Roger P, Boulle N, Brancherau P, et al. Characterization of resident b cells of vascular walls in human atherosclerotic patients. J Immunol (Baltimore Md: 1950) (2013) 191(6):3006–16. doi: 10.4049/jimmunol.1202870
48. Nielsen MM, Witherden DA, Havran WL. Γδ T cells in homeostasis and host defence of epithelial barrier tissues. Nat Rev Immunol (2017) 17(12):733–45. doi: 10.1038/nri.2017.101
49. Lu X. The impact of il-17 in atherosclerosis. Curr Medicinal Chem (2017) 24(21):2345–58. doi: 10.2174/0929867324666170419150614
50. Chabaud M, Durand JM, Buchs N, Fossiez F, Page G, Frappart L, et al. Human interleukin-17: A T cell-derived proinflammatory cytokine produced by the rheumatoid synovium. Arthritis Rheum (1999) 42(5):963–70. doi: 10.1002/1529-0131(199905)42:5<963::Aid-anr15>3.0.Co;2-e
51. McKenzie DR, Kara EE, Bastow CR, Tyllis TS, Fenix KA, Gregor CE, et al. Il-17-Producing Γδ T cells switch migratory patterns between resting and activated states. Nat Commun (2017) 8:15632. doi: 10.1038/ncomms15632
52. Roark CL, French JD, Taylor MA, Bendele AM, Born WK, O’Brien RL. Exacerbation of collagen-induced arthritis by oligoclonal, il-17-Producing gamma delta T cells. J Immunol (Baltimore Md: 1950) (2007) 179(8):5576–83. doi: 10.4049/jimmunol.179.8.5576
53. Andersson A, Grahnemo L, Engdahl C, Stubelius A, Lagerquist MK, Carlsten H, et al. Il-17-Producing Γδt cells are regulated by estrogen during development of experimental arthritis. Clin Immunol (Orlando Fla) (2015) 161(2):324–32. doi: 10.1016/j.clim.2015.09.014
54. Zhao Q. Dual targeting of Ccr2 and Ccr5: Therapeutic potential for immunologic and cardiovascular diseases. J Leukocyte Biol (2010) 88(1):41–55. doi: 10.1189/jlb.1009671
55. Mueller A, Strange PG. The chemokine receptor, Ccr5. Int J Biochem Cell Biol (2004) 36(1):35–8. doi: 10.1016/s1357-2725(03)00172-9
56. Oba Y, Lee JW, Ehrlich LA, Chung HY, Jelinek DF, Callander NS, et al. Mip-1alpha utilizes both Ccr1 and Ccr5 to induce osteoclast formation and increase adhesion of myeloma cells to marrow stromal cells. Exp Hematol (2005) 33(3):272–8. doi: 10.1016/j.exphem.2004.11.015
57. Schecter AD, Calderon TM, Berman AB, McManus CM, Fallon JT, Rossikhina M, et al. Human vascular smooth muscle cells possess functional Ccr5. J Biol Chem (2000) 275(8):5466–71. doi: 10.1074/jbc.275.8.5466
58. Pokorny V, McQueen F, Yeoman S, Merriman M, Merriman A, Harrison A, et al. Evidence for negative association of the chemokine receptor Ccr5 D32 polymorphism with rheumatoid arthritis. Ann Rheumatic Dis (2005) 64(3):487–90. doi: 10.1136/ard.2004.023333
59. Zapico I, Coto E, Rodríguez A, Alvarez C, Torre JC, Alvarez V. Ccr5 (Chemokine receptor-5) DNA-polymorphism influences the severity of rheumatoid arthritis. Genes Immun (2000) 1(4):288–9. doi: 10.1038/sj.gene.6363673
60. Gómez-Reino JJ, Pablos JL, Carreira PE, Santiago B, Serrano L, Vicario JL, et al. Association of rheumatoid arthritis with a functional chemokine receptor, Ccr5. Arthritis Rheum (1999) 42(5):989–92. doi: 10.1002/1529-0131(199905)42:5<989::Aid-anr18>3.0.Co;2-u
61. Garred P, Madsen HO, Petersen J, Marquart H, Hansen TM, Freiesleben Sørensen S, et al. Cc chemokine receptor 5 polymorphism in rheumatoid arthritis. J Rheumatol (1998) 25(8):1462–5.
62. Cooke SP, Forrest G, Venables PJ, Hajeer A. The Delta32 deletion of Ccr5 receptor in rheumatoid arthritis. Arthritis Rheum (1998) 41(6):1135–6. doi: 10.1002/1529-0131(199806)41:6<1135::Aid-art24>3.0.Co;2-n
63. Yang L, Liu YF, Wu G, Zhang JL, Wang FS, Fu DZ, et al. Blocking the cc chemokine receptor 5 pathway by antisense peptide nucleic acid prolongs islet allograft survival. Transplant Proc (2007) 39(1):185–90. doi: 10.1016/j.transproceed.2006.10.193
64. Szalai C, Duba J, Prohászka Z, Kalina A, Szabó T, Nagy B, et al. Involvement of polymorphisms in the chemokine system in the susceptibility for coronary artery disease (Cad). coincidence of elevated lp(a) and mcp-1 -2518 G/G genotype in cad patients. Atherosclerosis (2001) 158(1):233–9. doi: 10.1016/s0021-9150(01)00423-3
65. Rot A, von Andrian UH. Chemokines in innate and adaptive host defense: Basic chemokinese grammar for immune cells. Annu Rev Immunol (2004) 22:891–928. doi: 10.1146/annurev.immunol.22.012703.104543
66. Van Raemdonck K, Umar S, Palasiewicz K, Volkov S, Volin MV, Arami S, et al. Ccl21/Ccr7 signaling in macrophages promotes joint inflammation and Th17-mediated osteoclast formation in rheumatoid arthritis. Cell Mol Life Sci: CMLS (2020) 77(7):1387–99. doi: 10.1007/s00018-019-03235-w
67. Pang J, Yu KQ. Detection and its clinical value of Ccr5 and Ccr7 in dendritic cells from patients with active rheumatoid arthritis. Nan fang yi ke da xue xue bao = J South Med Univ (2010) 30(9):2067–9.
68. Pickens SR, Chamberlain ND, Volin MV, Pope RM, Mandelin AM 2nd, Shahrara S. Characterization of Ccl19 and Ccl21 in rheumatoid arthritis. Arthritis Rheum (2011) 63(4):914–22. doi: 10.1002/art.30232
69. Pickens SR, Chamberlain ND, Volin MV, Pope RM, Talarico NE, Mandelin AM 2nd, et al. Role of the Ccl21 and Ccr7 pathways in rheumatoid arthritis angiogenesis. Arthritis Rheum (2012) 64(8):2471–81. doi: 10.1002/art.34452
70. Halvorsen B, Dahl TB, Smedbakken LM, Singh A, Michelsen AE, Skjelland M, et al. Increased levels of Ccr7 ligands in carotid atherosclerosis: Different effects in macrophages and smooth muscle cells. Cardiovasc Res (2014) 102(1):148–56. doi: 10.1093/cvr/cvu036
71. Schieffer B, Luchtefeld M. Emerging role of chemokine receptor 7 in atherosclerosis. Trends Cardiovasc Med (2011) 21(8):211–6. doi: 10.1016/j.tcm.2012.05.012
72. Pickens SR, Chamberlain ND, Volin MV, Pope RM, Talarico NE, Mandelin AM 2nd, et al. Characterization of interleukin-7 and interleukin-7 receptor in the pathogenesis of rheumatoid arthritis. Arthritis Rheum (2011) 63(10):2884–93. doi: 10.1002/art.30493
73. Kim SJ, Chang HJ, Volin MV, Umar S, Van Raemdonck K, Chevalier A, et al. Macrophages are the primary effector cells in il-7-Induced arthritis. Cell Mol Immunol (2020) 17(7):728–40. doi: 10.1038/s41423-019-0235-z
74. Chen Z, Kim SJ, Chamberlain ND, Pickens SR, Volin MV, Volkov S, et al. The novel role of il-7 ligation to il-7 receptor in myeloid cells of rheumatoid arthritis and collagen-induced arthritis. J Immunol (Baltimore Md: 1950) (2013) 190(10):5256–66. doi: 10.4049/jimmunol.1201675
75. Mihailovic PM, Lio WM, Yano J, Zhou J, Zhao X, Chyu KY, et al. Il-7r blockade reduces post-myocardial infarction-induced atherosclerotic plaque inflammation in apoe(-/-) mice. Biochem Biophys Rep (2019) 19:100647. doi: 10.1016/j.bbrep.2019.100647
76. Al Barashdi MA, Ali A, McMullin MF, Mills K. Protein tyrosine phosphatase receptor type c (Ptprc or Cd45). J Clin Pathol (2021) 74(9):548–52. doi: 10.1136/jclinpath-2020-206927
77. Rheinländer A, Schraven B, Bommhardt U. Cd45 in human physiology and clinical medicine. Immunol Lett (2018) 196:22–32. doi: 10.1016/j.imlet.2018.01.009
78. Bek S, Bojesen AB, Nielsen JV, Sode J, Bank S, Vogel U, et al. Systematic review and meta-analysis: Pharmacogenetics of anti-tnf treatment response in rheumatoid arthritis. Pharmacogenomics J (2017) 17(5):403–11. doi: 10.1038/tpj.2017.26
79. Lee YH, Bae SC. Associations between ptprc Rs10919563 a/G and Fcgr2a R131h polymorphisms and responsiveness to tnf blockers in rheumatoid arthritis: A meta-analysis. Rheumatol Int (2016) 36(6):837–44. doi: 10.1007/s00296-016-3476-5
80. Higgs R. Ptprc mutation associated with response to anti-tnf therapy in rheumatoid arthritis. Nat Rev Rheumatol (2010) 6(6):311. doi: 10.1038/nrrheum.2010.69
81. Xia M, Wu Q, Chen P, Qian C. Regulatory T cell-related gene biomarkers in the deterioration of atherosclerosis. Front Cardiovasc Med (2021) 8:661709. doi: 10.3389/fcvm.2021.661709
82. Binder C, Cvetkovski F, Sellberg F, Berg S, Paternina Visbal H, Sachs DH, et al. Cd2 immunobiology. Front Immunol (2020) 11:1090. doi: 10.3389/fimmu.2020.01090
83. Makgoba MW, Sanders ME, Shaw S. The Cd2-Lfa-3 and lfa-1-Icam pathways: Relevance to T-cell recognition. Immunol Today (1989) 10(12):417–22. doi: 10.1016/0167-5699(89)90039-x
84. Raychaudhuri S, Thomson BP, Remmers EF, Eyre S, Hinks A, Guiducci C, et al. Genetic variants at Cd28, Prdm1 and Cd2/Cd58 are associated with rheumatoid arthritis risk. Nat Genet (2009) 41(12):1313–8. doi: 10.1038/ng.479
85. Fernandez Lahore G, Förster M, Johannesson M, Sabatier P, Lönnblom E, Aoun M, et al. Polymorphic estrogen receptor binding site causes Cd2-dependent sex bias in the susceptibility to autoimmune diseases. Nat Commun (2021) 12(1):5565. doi: 10.1038/s41467-021-25828-5
86. Ghazizadeh R, Shimizu H, Tosa M, Ghazizadeh M. Pathogenic mechanisms shared between psoriasis and cardiovascular disease. Int J Med Sci (2010) 7(5):284–9. doi: 10.7150/ijms.7.284
87. Gil J, Busto EM, Garcillán B, Chean C, García-Rodríguez MC, Díaz-Alderete A, et al. A leaky mutation in Cd3d differentially affects Aβ and Γδ T cells and leads to a tαβ-Tγδ+B+Nk+ human scid. J Clin Invest (2011) 121(10):3872–6. doi: 10.1172/jci44254
88. Lu J, Bi Y, Zhu Y, Huipeng S, Duan W, Zhou J. Cd3d, gzmk, and Klrb1 are potential markers for early diagnosis of rheumatoid arthritis, especially in anti-citrullinated protein antibody-negative patients. Front Pharmacol (2021) 12:726529. doi: 10.3389/fphar.2021.726529
89. Zhao J, Wei K, Jiang P, Chang C, Xu L, Xu L, et al. G-Protein-Coupled receptors in rheumatoid arthritis: Recent insights into mechanisms and functional roles. Front Immunol (2022) 13:907733. doi: 10.3389/fimmu.2022.907733
90. Scott DL, Wolfe F, Huizinga TW. Rheumatoid arthritis. Lancet (Lond Engl) (2010) 376(9746):1094–108. doi: 10.1016/s0140-6736(10)60826-4
91. Ben Mrid R, Bouchmaa N, Ainani H, El Fatimy R, Malka G, Mazini L. Anti-rheumatoid drugs advancements: New insights into the molecular treatment of rheumatoid arthritis. Biomed Pharmacother = Biomed Pharmacother (2022) 151:113126. doi: 10.1016/j.biopha.2022.113126
92. Charo IF, Ransohoff RM. The many roles of chemokines and chemokine receptors in inflammation. N Engl J Med (2006) 354(6):610–21. doi: 10.1056/NEJMra052723
93. Sajid MI, Moazzam M, Kato S, Yeseom Cho K, Tiwari RK. Overcoming barriers for sirna therapeutics: From bench to bedside. Pharm (Basel Switzerland) (2020) 13(10):294. doi: 10.3390/ph13100294
Keywords: atherosclerosis, rheumatoid arthritis, immune infiltration, hub genes, memory B cells, follicular helper T cells, γδT cells
Citation: Wang Z, Xia Q, Su W, Zhang M, Gu Y, Xu J, Chen W and Jiang T (2022) The commonness in immune infiltration of rheumatoid arthritis and atherosclerosis: Screening for central targets via microarray data analysis. Front. Immunol. 13:1013531. doi: 10.3389/fimmu.2022.1013531
Received: 07 August 2022; Accepted: 30 September 2022;
Published: 13 October 2022.
Edited by:
Jindong Ni, Guangdong Medical University, ChinaReviewed by:
Udhaya Kumar. S, Vellore Institute of Technology, IndiaNoha Mousaad Elemam, University of Sharjah, United Arab Emirates
Copyright © 2022 Wang, Xia, Su, Zhang, Gu, Xu, Chen and Jiang. This is an open-access article distributed under the terms of the Creative Commons Attribution License (CC BY). The use, distribution or reproduction in other forums is permitted, provided the original author(s) and the copyright owner(s) are credited and that the original publication in this journal is cited, in accordance with accepted academic practice. No use, distribution or reproduction is permitted which does not comply with these terms.
*Correspondence: Weixiang Chen, Y2hlbndlaXhpYW5nQHN1ZGEuZWR1LmNu; Tingbo Jiang, anRic2RmeXlAMTYzLmNvbQ==
†These authors have contributed equally to this work