- 1Department of Orthopedics, 900th Hospital of Joint Logistics Support Force, Fuzhou, China
- 2Arthritis Clinical and Research Center, Peking University People’s Hospital, Beijing, China
Background: Severe acute respiratory syndrome coronavirus 2 (SARS-CoV-2) caused a global pandemic of severe coronavirus disease 2019 (COVID-19). Staphylococcus aureus is one of the most common pathogenic bacteria in humans, rheumatoid arthritis (RA) is among the most prevalent autoimmune conditions. RA is a significant risk factor for SARS-CoV-2 and S. aureus infections, although the mechanism of RA and SARS-CoV-2 infection in conjunction with S. aureus infection has not been elucidated. The purpose of this study is to investigate the biomarkers and disease targets between RA and SARS-CoV-2 and S. aureus infections using bioinformatics analysis, to search for the molecular mechanisms of SARS-CoV-2 and S. aureus immune escape and potential drug targets in the RA population, and to provide new directions for further analysis and targeted development of clinical treatments.
Methods: The RA dataset (GSE93272) and the S. aureus bacteremia (SAB) dataset (GSE33341) were used to obtain differentially expressed gene sets, respectively, and the common differentially expressed genes (DEGs) were determined through the intersection. Functional enrichment analysis utilizing GO, KEGG, and ClueGO methods. The PPI network was created utilizing the STRING database, and the top 10 hub genes were identified and further examined for functional enrichment using Metascape and GeneMANIA. The top 10 hub genes were intersected with the SARS-CoV-2 gene pool to identify five hub genes shared by RA, COVID-19, and SAB, and functional enrichment analysis was conducted using Metascape and GeneMANIA. Using the NetworkAnalyst platform, TF-hub gene and miRNA-hub gene networks were built for these five hub genes. The hub gene was verified utilizing GSE17755, GSE55235, and GSE13670, and its effectiveness was assessed utilizing ROC curves. CIBERSORT was applied to examine immune cell infiltration and the link between the hub gene and immune cells.
Results: A total of 199 DEGs were extracted from the GSE93272 and GSE33341 datasets. KEGG analysis of enrichment pathways were NLR signaling pathway, cell membrane DNA sensing pathway, oxidative phosphorylation, and viral infection. Positive/negative regulation of the immune system, regulation of the interferon-I (IFN-I; IFN-α/β) pathway, and associated pathways of the immunological response to viruses were enriched in GO and ClueGO analyses. PPI network and Cytoscape platform identified the top 10 hub genes: RSAD2, IFIT3, GBP1, RTP4, IFI44, OAS1, IFI44L, ISG15, HERC5, and IFIT5. The pathways are mainly enriched in response to viral and bacterial infection, IFN signaling, and 1,25-dihydroxy vitamin D3. IFI44, OAS1, IFI44L, ISG15, and HERC5 are the five hub genes shared by RA, COVID-19, and SAB. The pathways are primarily enriched for response to viral and bacterial infections. The TF-hub gene network and miRNA-hub gene network identified YY1 as a key TF and hsa-mir-1-3p and hsa-mir-146a-5p as two important miRNAs related to IFI44. IFI44 was identified as a hub gene by validating GSE17755, GSE55235, and GSE13670. Immune cell infiltration analysis showed a strong positive correlation between activated dendritic cells and IFI44 expression.
Conclusions: IFI144 was discovered as a shared biomarker and disease target for RA, COVID-19, and SAB by this study. IFI44 negatively regulates the IFN signaling pathway to promote viral replication and bacterial proliferation and is an important molecular target for SARS-CoV-2 and S. aureus immune escape in RA. Dendritic cells play an important role in this process. 1,25-Dihydroxy vitamin D3 may be an important therapeutic agent in treating RA with SARS-CoV-2 and S. aureus infections.
Introduction
Rheumatoid arthritis (RA) (1) is one of the most prevalent chronic inflammatory autoimmune diseases and has been the focus of intense study for decades (1–5). The prevalence of RA is about 1% (6). The clinical presentation of RA is characterized by chronic persistent synovitis, which, in turn, destroys bone and cartilage, leading to joint bone destruction and chronic disability (7–9). Therefore, patients with RA are more prone than the general population to requiring hip and knee replacements (10). There are three main categories of factors that influence the progression of RA: genetic, environmental, and immune (11, 12), with microbial infections (e.g., bacteria and viruses) constituting a significant subset of environmental factors that can trigger, induce, and exacerbate the disease process in RA (13–17). The balance between the impact of microorganisms on the host and the immune response of the host to microorganisms is crucial for maintaining the regular functioning of the body’s immune system, and an imbalance between these reactions can exacerbate autoimmune inflammation in RA (18). In addition, disease-modifying antirheumatic drugs (DMARDs) and glucocorticoids, commonly used for RA, can affect the immune system to varying degrees (19–21). Although emerging biologic medicines (e.g., TNF inhibitors) have been employed in recent years to treat patients with RA with an inadequate response to DMARDs (22–25), studies have shown that their usage is linked with an increased risk of infection in patients with RA (26–28). Therefore, microbial infection is dangerous for individuals with RA, either in the illness itself or with the associated medicine, as well as after arthroplasty (29–31).
Staphylococcus aureus is a gram-positive human opportunistic pathogen (32) that frequently colonizes the human nasal cavity (33, 34) and can cause severe systemic or local infections if the immune system is compromised (35). First, S. aureus bacteremia (SAB) is a frequent systemic infection that is characterized by significant morbidity and mortality (36), and the majority of SAB are endogenous infections, predominantly from nasally colonized colonies (37). Second, local infections with S. aureus are prevalent in postoperative surgical-site infection (SSI) and prosthetic joint infection (PJI) (38, 39), which are not only the most prevalent postoperative complications (40) but also catastrophic consequences of joint replacement surgery (41, 42). According to studies, nasal carriage of S. aureus is also a common source of postoperative infections (43, 44). Patients with RA are more likely than the general population to carry S. aureus in their nasal vestibules (45), and RA medications enhance nasal S. aureus carriage (46). In a Danish national observational cohort study, RA was identified as a significant risk factor for SAB, and intra-articular orthopedic implants enhanced the chance of infection (31). Another prospective cohort study found that patients with RA had a greater incidence of SAB and death and that RA-induced osteoarthritic damage made S. aureus more vulnerable to osteoarthritic infection (47). Patients with RA are susceptible to S. aureus primarily due to the following factors: First, the immune system disorder of patients with RA makes S. aureus easy to invade the host. Second, for the local situation of patients with RA, the bone and joint damage caused by the disease makes local infection with S. aureus easier. Third, patients with RA are susceptible to carrying S. aureus. Fourth, the medication of RA makes the nasal cavity more susceptible and carries more S. aureus. Fifth, it is easy for S. aureus to cause SSI and PJI in patients with RA who have had joint replacement surgery. Therefore, we aim to investigate the RA population’s underlying susceptibility mechanism to S. aureus.
In 2019, COVID-19, caused by the severe acute respiratory syndrome coronavirus 2 (SARS-CoV-2), swiftly affected people and produced a significant public health concern, which was eventually classified as a worldwide pandemic (48–52). As of 12 June 2022, over 535 million confirmed cases and over six million deaths had been reported globally (53). COVID-19 is a systemic disease that can cause significant damage to several body systems, manifesting clinically as fever, cough, and respiratory distress, as well as skeletal and muscular symptoms, including arthralgia (54–57). SARS-CoV-2 has been reported to overstimulate the body’s immune system and contribute to autoantibody production due to potential antigenic cross-reactivity with the body (58–60). Indeed, patients with COVID-19 frequently exhibit immunological dysregulation (61) and can trigger multiple autoimmune diseases (59, 62), and, conversely, patients with autoimmune disease are more vulnerable to SARS-CoV-2 infection (63), and the course of COVID-19 is more severe in hospitalized patients with autoimmune disease (64). As one of the most prevalent autoimmune diseases, RA merits in-depth investigation. According to studies, patients with RA infected with SARS-CoV-2 had a greater likelihood of hospitalization and mortality than non-RA patients (65, 66). Moreover, viral sequelae/combined bacterial infections are not only common consequences (67–69) but also significantly exacerbate disease severity and death (70–74). Streptococcus pneumoniae, β-hemolytic streptococci, Haemophilus influenzae, Pseudomonas aeruginosa, and S. aureus are often coinfected microorganisms (75–80). In COVID-19, S. aureus was the most common bacterium for SARS-CoV-2 sequel/combination (81, 82).
The RA population is one of the most numerous in the world for autoimmune diseases, with S. aureus being one of the most common human pathogens and COVID-19 caused by SARS-CoV-2, leading to a global pandemic. These three factors affect a wide range of populations and have a poor prognosis, and their combination leads to high rates of disability and mortality, posing a serious risk to global public health. This study aims to investigate the causes of RA susceptibility to SARS-CoV-2 and Aureus infection through bioinformatics analysis, to discover the common biomarkers and disease targets among the three, to search for the mechanisms of SARS-CoV-2 and S. aureus immune escape in the RA population, and to provide new directions for further analysis of their pathogenesis and targeted development of clinical treatments.
Materials and methods
Data collection
Three RA (GSE93272, GSE17755, GSE55235) and two S. aureus infection (GSE33341 and GSE13670) datasets were included in this study (83–87), using the National Center for Biotechnology Information Gene Expression Omnibus (GEO) (https://www.ncbi.nlm.nih.gov/geo/) for screening (Table 1). As test sets, the GSE93272 dataset with 232 patients with RA and 43 healthy individuals’ whole blood samples and the GSE33341 dataset with 31 SAB patients and 43 healthy individuals’ whole blood samples were utilized to identify the differentially expressed genes (DEGs). The GSE17755 dataset contains whole-blood samples from four patients with RA and 12 healthy individuals. The GSE55235 dataset contains synovial tissue samples from 10 patients with RA and 10 healthy individuals. GSE13670 dataset contains 15 S. aureus-infected macrophage samples and 15 healthy human macrophage samples. These three datasets were utilized as validation sets for the hub genes.
Identification of DEGs
The empirical Bayesian approach of the limma R package (http://www.bioconductor.org/packages/release/bioc/html/limma.html) (88, 89) was used for differentially expressed genes between the RA and HC groups of GSE93272 and the SAB and HC groups of GSE33341 for analysis. The cutoff was |log2 FC| > 0.5 and P < 0.05. The volcano map was further drawn using the ggplot2 package to reflect the differential expression of DEGs. The common DEGs were obtained by taking the intersection of DEGs (GSE93272) and DEGs (GSE33341) using the Venn-diagram package in the R software.
GO, KEGG, and ClueGO enrichment analyses of DEGs
To explore the pathways and functions of the identified genes, GO and KEGG enrichment analyses of common-DEGs were performed with the R package “clusterProfiler” (90, 91). P < 0.05 indicates statistical significance. Finally, we visualized the common DEGs by using ClueGO (a plug-in for Cytoscape that uses the Kappa statistical analysis method) to display the interactive gene network map and analyze the function of the target gene set.
PPI network analysis, machine learning, and the identification of hub genes
The STRING database (https://string-db.org/) (92) was utilized to filter and construct PPI networks based on confidence values greater than 0.40. Machine learning is used to predict the interactions of PPI networks, specifically using the k-means algorithm (network is clustered to a specified number of clusters; number of clusters: 3) for clustering. K-means is an effective unsupervised machine learning approach for predicting protein pairings that interact without prior data labeling (93, 94). We construct and visualize PPI network data using The Cytoscape platform (95), then analyze PPI molecular networks using The MCODE (a Added to abbreviations Cytoscape plug-in). The cytoHubba tool was utilized to find hub genes, analyze each gene using the maximum centrality (MCC) algorithm, rank these genes, and filter the top 10 hub genes.
Metascape and GeneMANIA enrichment analyses of hub genes
Metascape (https://metascape.org/gp/index.html#/main/step1) is a statistical approach that can use all genes in the input genome as an enrichment background. Genes are grouped into clusters using terms with a P-value of 0.01, a minimum count of 3, and an enrichment factor > 1.5 to look for enrichment pathways and related functional annotations of target genes. In addition, terms with a similarity of greater than 0.30 were connected point to point by the Cytoscape visual network program to generate a network diagram that further illustrates the relationships between terms. GeneMANIA (http://www.genemania.org) is another website that integrates different databases and technologies to anticipate and identify the relevant activities of individual genes in hub genes and establish gene priority and linkages.
Identification of hub genes between RA, COVID-19, and SAB and functional enrichment analysis
The GeneCards database (https://www.genecards.org/) (96) was accessed and searched for “COVID-19” and “SARS-CoV-2” as keywords, and 4,778 and 4,055 related genes were found, respectively. There were 17, 28, and 25 SARS-CoV-2–associated genes from Ziegler et al. (97), Jain et al. (98), and Xiong et al. (99), respectively (Table 2). Finally, 5,103 related genes were obtained after summarizing these five parts of genes and removing duplicate data. Hub genes were obtained from the intersection of the top 10 hub genes and 5,103 SARS-CoV-2–related genes using the Venn Diagram package in R software. Finally, Metascape and GeneMANIA enrichment analyses of the hub gene were used.
Construction of TF-hub genes and miRNA-hub gene network
TF-hub gene and miRNAs-hub gene regulation networks were constructed utilizing NetworkAnalyst (https://www.networkanalyst.ca/) (100). We submitted the hub genes between RA, COVID-19, and SAB to NetworkAnalyst to acquire TFs from the ENCODE database and miRNA from miRTarBase v8.0 and TarBase v8.0 for hub genes. Cytoscape was used to display the networks of TF-hub genes and miRNA-hub genes.
Validation of hub genes and identification of hub genes
The datasets GSE17755, GSE55235, and GSE13670 were included in our study as validation sets to strengthen the reliability and correctness of the results. The genes from the three validation sets were also individually processed using the limma R package to generate the volcano map of the corresponding DEGs. The hub genes were identified using the Venn Diagram tool, and the expression of the hub genes in each dataset was visualized using a box plot. The subject Receiver Operating Characteristic Curve (ROC) was then used to estimate the test’s effect to determine the hub gene’s sensitivity and specificity in various datasets (101). A value of 0.7 was regarded as diagnostically significant.
Analysis of immune cell infiltration and correlation analysis between immune cells
The CIBERSORT algorithm (http://CIBERSORT.stanford.edu/) is a method based on linear support vector regression (102). It was applied to evaluate the composition and quantity of immune cells in RA and HC. P < 0.05 prompted us to submit the data to CIBERSORT and receive the immune cell infiltration matrix. The ggplot2 package was used to display the distribution of LM22, whereas the corrplot package was utilized to display its correlation. Finally, we used Pearson’s correlation coefficient analysis to reveal the relationship between the expression of target genes and the abundance of immune cells in RA to find immune cells associated with the target genes. The Github page for this study is HTTPS(https://github.com/zheng5862/IFI44).
Results
Identification of DEGs
The flowchart shows all our study’s key and important procedures (Figure 1). A total of 338 DEGs were obtained from the GSE93272 dataset, of which 322 were upregulated genes and 16 were downregulated genes. In addition, 3,174 DEGs were obtained from the GSE33341 dataset, of which 1,429 were upregulated genes and 1,745 were downregulated genes. The distribution of DEGs for the two datasets was visualized using a volcano plot (Figure 2) and clustered heat map analysis (Figure 3). The analysis results of these two datasets were intersected using the Venn Diagram package to obtain a total of 199 DEGs (Figure 4A). The 199 DEGs had 192 upregulated genes in GSE93272, seven downregulated genes in GSE33341, 188 upregulated genes in GSE33341, and 11 downregulated genes in GSE33341. The distribution of the 199 DEGs in the microarray datasets GSE93272 and GSE33341, respectively, can be seen using the clustered heat map (Figures 4B,C).
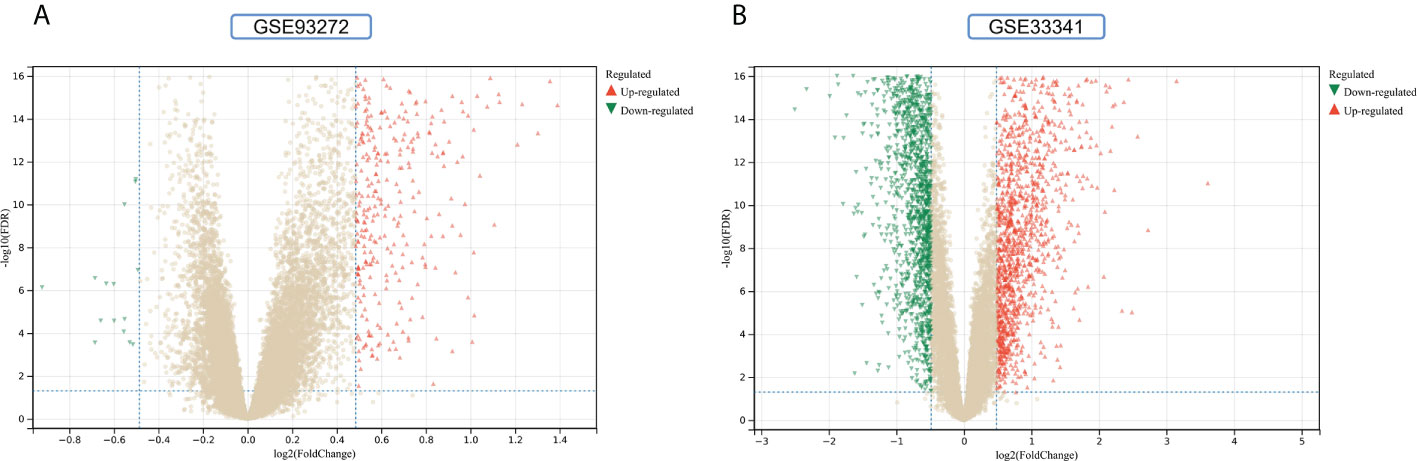
Figure 2 DEGs identification. (A) Gray dots represent genes not substantially differently expressed in RA and HC groups (P > 0.05), red triangles represent upregulated genes (P < 0.05), and green triangles represent downregulated genes (P < 0.05) in the GSE93272 dataset. (B) Gray dots represent genes not substantially differently expressed in S. aureus and HC groups (P > 0.05), red triangles represent upregulated genes (P < 0.05), and green triangles represent downregulated genes (P < 0.05) in the GSE33341 dataset.
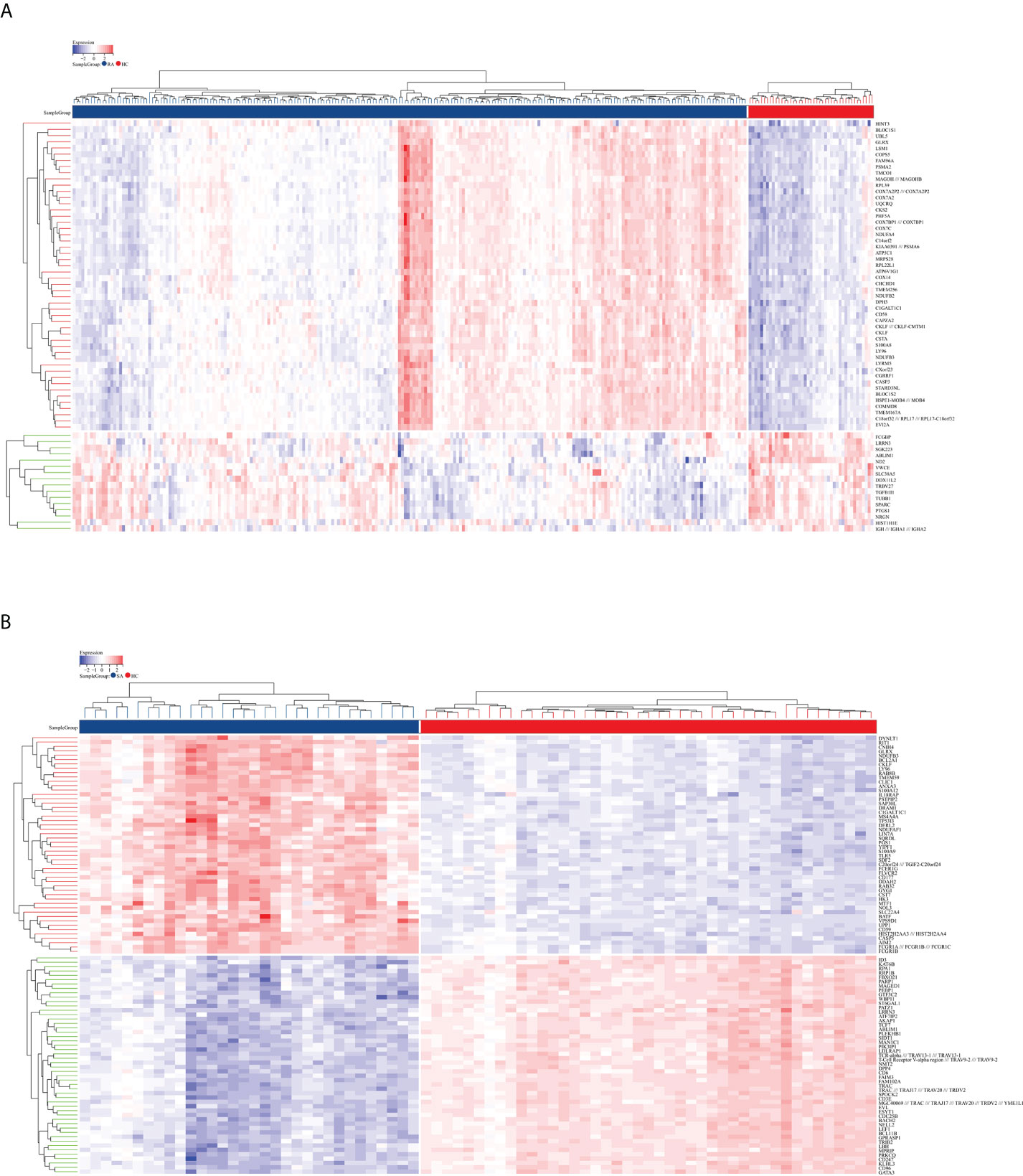
Figure 3 DEG distribution. (A) The clustering heat map shows the DEGs in the GSE93272 dataset. The RA group’s samples are colored blue, whereas the HC group’s samples are colored red. Red rectangles indicate elevated genes (P < 0.05), whereas blue rectangles indicate downregulated genes (P < 0.05). (B) The clustering heat map shows the intersection of DEGs in the GSE33341 dataset. The SA group’s samples are colored blue, whereas the HC group’s samples are colored red. Red rectangles indicate elevated genes (P < 0.05), whereas blue rectangles indicate downregulated genes (P < 0.05).
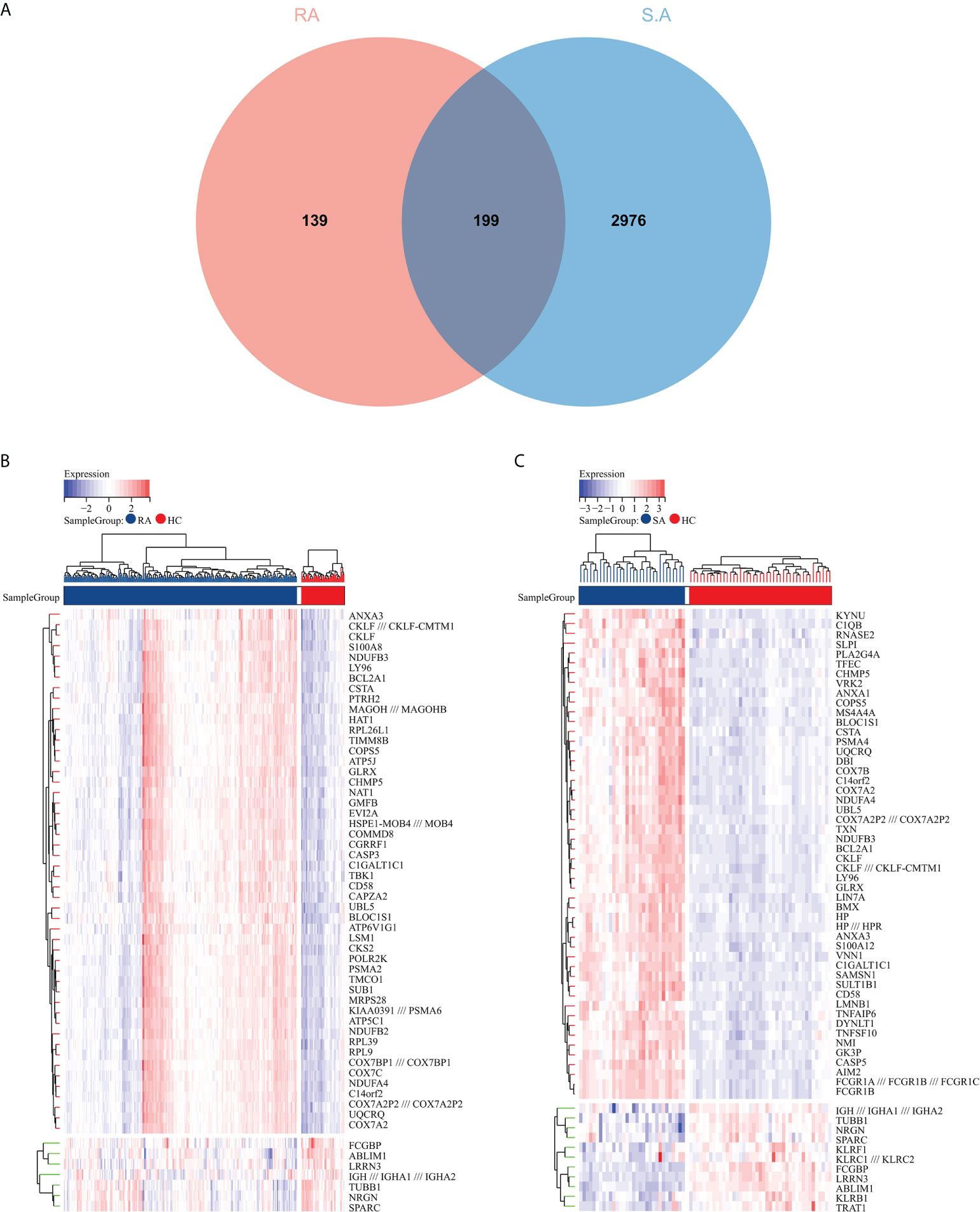
Figure 4 Common DEG screening. (A) Venn diagram on GSE93272 DEGs and GSE33341 DEGs. (B) Clustered heat map of common DEGs belonging to the RA group. (C) Clustered heat map of common DEGs in the SA group, with the RA/SA group colored blue and the HC group colored red. Red rectangles indicate elevated genes (P < 0.05), whereas blue rectangles indicate downregulated genes (P < 0.05).
Functional enrichment analyses of DEGs
The GO and KEGG methods were used to explore the functional correlation between the 199 DEGs sets of RA and SAB. From the GO analysis, it is clear that BP is mainly manifested in immune system process, immune response, defense response, immune effector process, innate immune response, response to biotic stimulus, response to other organisms, response to external biotic stimulus, defense response to other organism, and response to a virus (Figure 5A). CC is mainly enriched in the cytosol and cytosolic part (Figure 5B). MF mainly manifests in oxidoreductase activity, cytochrome-oxidase activity, pantetheine hydrolase activity, and immunoglobulin receptor activity (Figure 5C). The KEGG analysis shows the main enrichment in the NOD-like receptor signaling pathway, influenza A, oxidative phosphorylation, Epstein–Barr virus infection, and cytosolic DNA-sensing pathway (Figure 5D). From the ClueGO analysis, it can be visualized that the main enrichment is in the following pathways. First, regulation of innate immune responses includes IFN-I production, regulation of IFN-I production, regulation of IFN-I–mediated signaling pathway, IFN-I signaling pathway, IFN-α/β production, regulation IFN-α/β production, negative regulation of immune response, and negative regulation of innate immune response. Second, controlling viral infections involves regulating the viral replication process and immune, cellular, and defensive responses to a virus (Figure 5E).
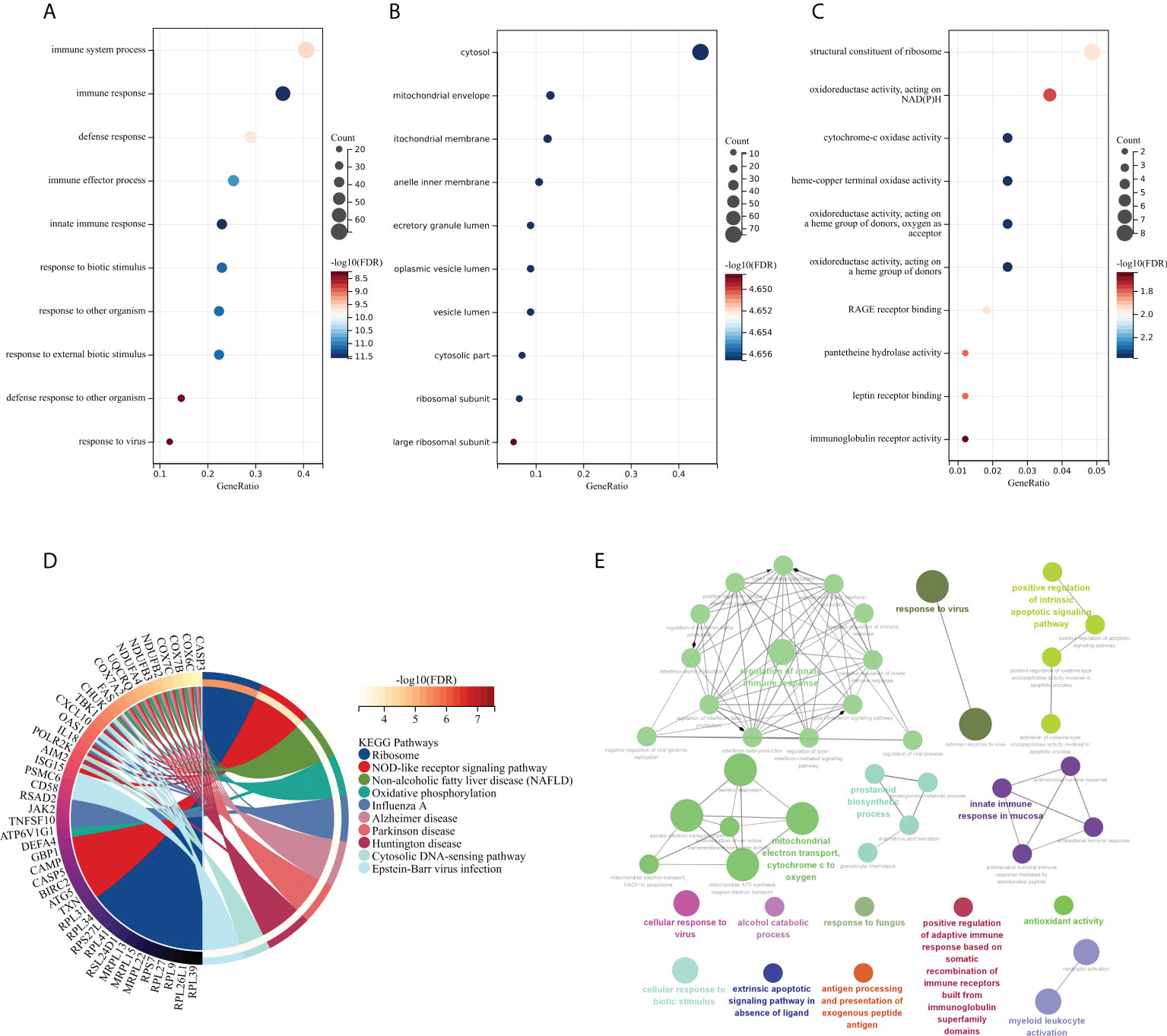
Figure 5 Functional enrichment analysis: GO, KEGG, and ClueGO analysis of DEGs. (A) BP gene enrichment of DEGs. (B) CC gene enrichment of DEGs. (C) MF gene enrichment of DEGs. (D) Analysis of DEGs using KEGG. (E) Analysis of DEGs using ClueGO.
PPI network, machine learning, and the identification of top 10 hub genes
PPI network data based on the STRING database were processed using Cytoscape software to further investigate the pathogenesis between RA and SAB. The results show that this PPI network has 184 nodes, 750 edges, an average node degree of 8.15, and an average local clustering coefficient of 0.461. The k-means cluster analysis graph based on the unsupervised machine learning algorithm of the PPI network can be seen: the green hexagon in the lower right corner is exactly the top 10 hub genes derived using the CytoHubba analysis method (Figure 6A). We then identified the top 10 genes in the enrichment ranking by the MCC algorithm of the CytoHubba package in Cytoscape software: RSAD2, IFIT3, GBP1, RTP4, IFI44, OAS1, IFI44L, ISG15, HERC5, and IFIT5 (Figure 6B), consistent with the PPI network using a k-means clustering algorithm to obtain the same results. Tables 3, 4 give information about the top 10 hub genes in the GSE93272 and GSE33341 datasets, respectively.
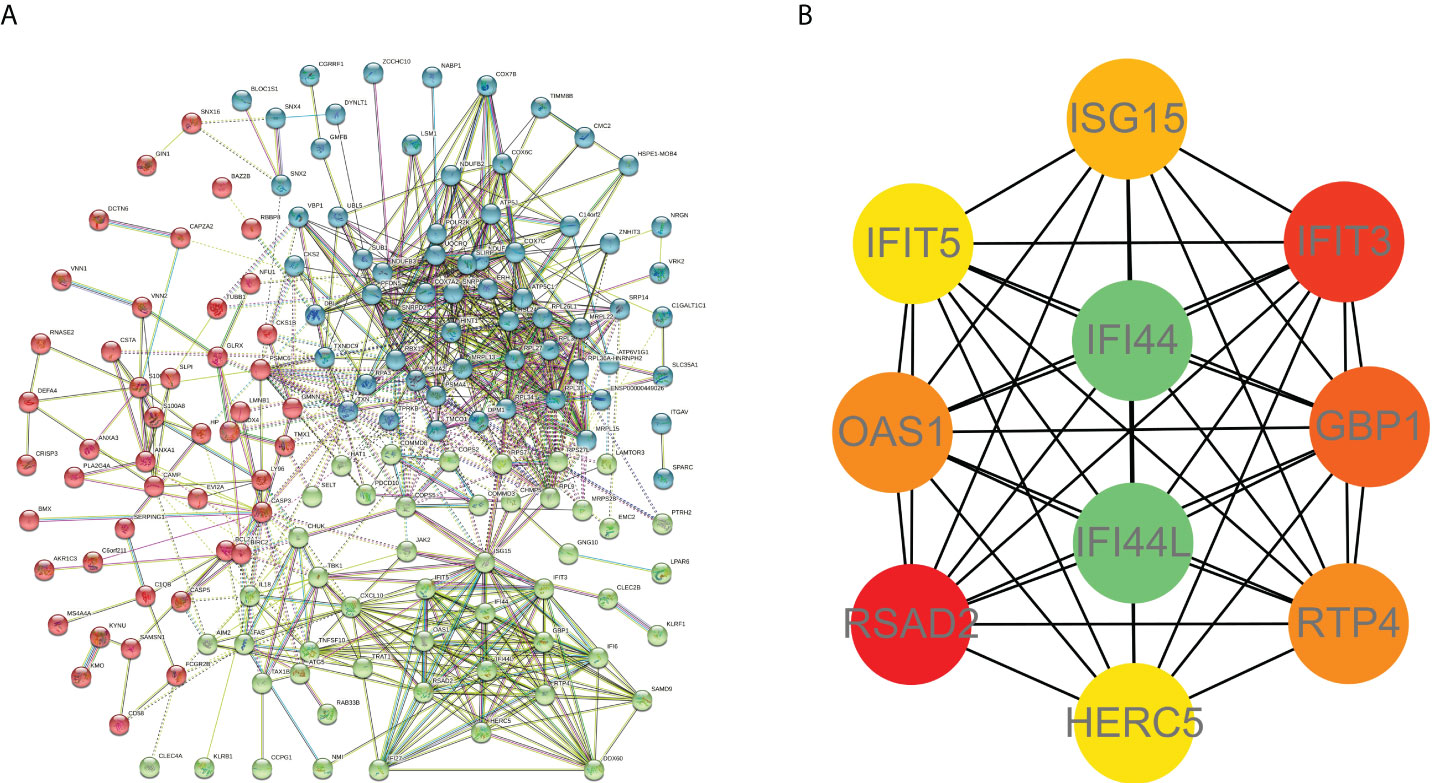
Figure 6 PPI interworking networks. (A) PPI network with 184 nodes and 750 edges. The green hexagon in the lower right corner is the top 10 hub genes derived using the CytoHubba analysis method. (B) Analysis of the top 10 hub genes with CytoHubba of Cytoscape.
Functional enrichment analyses of the top 10 hub genes
The top 10 hub genes were analyzed by the Metascape platform with the following findings. First, pathway and process enrichment analysis is mainly enriched in response to a virus, defense response to a virus, interferon (IFN) signaling, non-genomic actions of 1,25-dihydroxy vitamin D3, and cellular response to cytokine stimulus (Figure 7A). Second, DisGeNET13 was mainly enriched in influenza A, bacterial infections, rhinovirus infections, and hepatitis C (chronic) (Figure 7B). Further network connection diagrams are used to visualize the connections between the pathways (Figure 7C). Finally, GeneMANIA was used to visualize the link between the 10 core genes and the most closely related genes (Figure 7D).
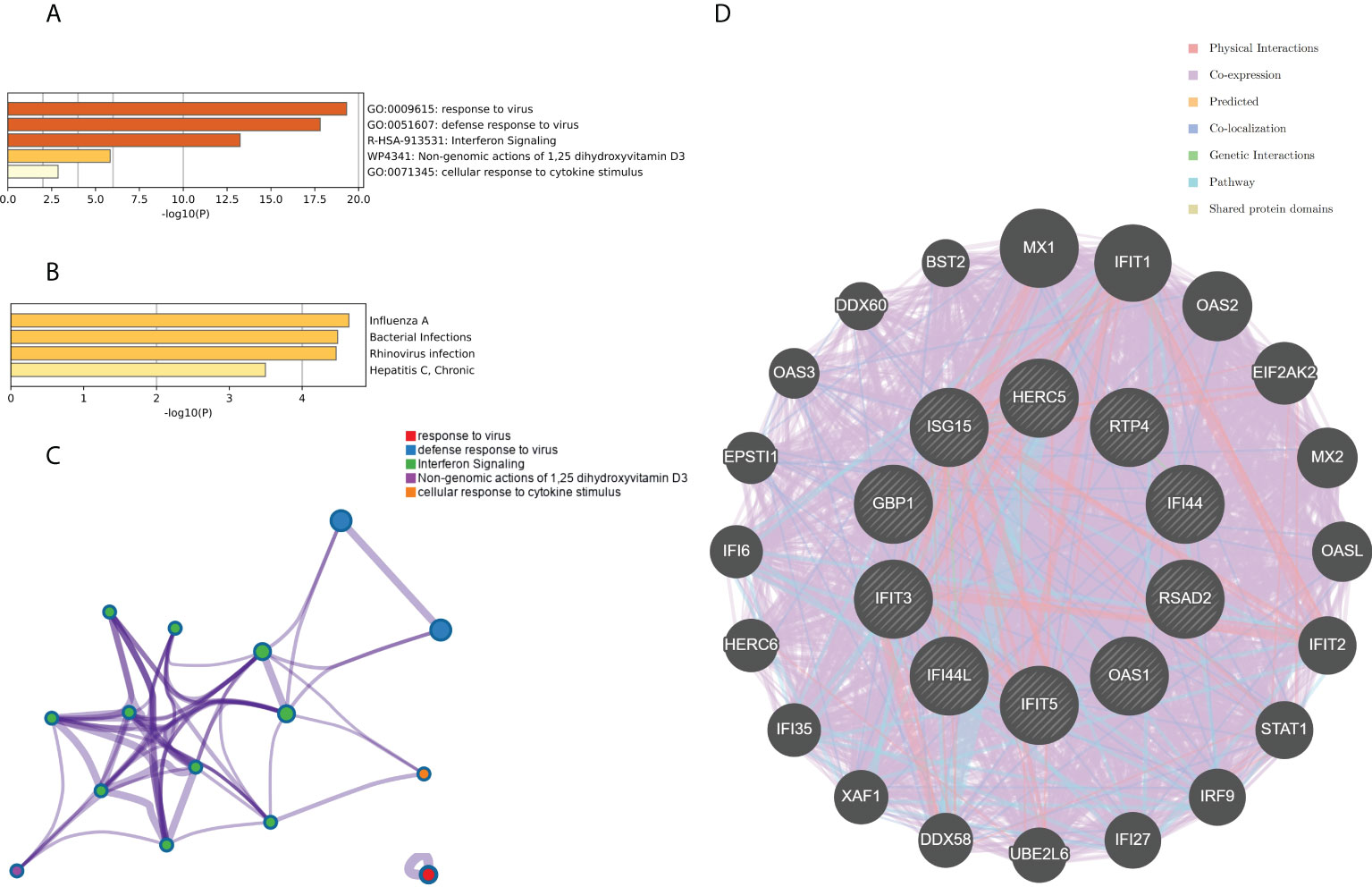
Figure 7 Functional enrichment analysis: Metascape and GeneMANIA of top 10 hub genes. (A) Pathway and process richness analysis of the Metascape platform. (B) Summary of enrichment analysis of DisGeNET 13 on Metascape platform. (C) The network is visualized using Cytoscape 5, colored by cluster IDs, and nodes sharing the same cluster ID are usually close to each other. (D) The gene–gene interaction network of the top 10 hub genes with the 20 most adjacent genes was analyzed using the GeneMANIA database. Each node represents a gene. The color of the linkage of the nodes represents the linkage between the corresponding genes.
Identification of the hub genes between RA, COVID-19, and SAB and functional enrichment analysis
The genes associated with SARS-CoV-2 were selected from the Genecard database and related literature, and 5,103 genes were obtained after summarizing and removing duplicate data. The top 10 hub genes intersected with the SARS-CoV-2 gene set with five genes: IFI44, OAS1, IFI44L, ISG15, and HERC5 (Figure 8A). The expression of these five genes in the GSE93272 and GSE33341 datasets was analyzed using split-face violin plots, and it can be seen that the expression of all five genes in the RA and SAB datasets was significantly higher than that in the control group (P < 0.01) (Figures 8B, C). The functional enrichment analysis results using the Metascape platform are as follows. First, pathway and process enrichment analysis is mainly enriched in response to a virus, defense response to a virus, and response to a bacterium (Figure 9A). Second, DisGeNET13 was mainly enriched in bacterial infections (Figure 9B). Further network connection diagrams are utilized to more precisely depict the links between the channels (Figure 9C). Finally, GeneMANIA was utilized to illustrate the relationship between the five hub genes and their closest relatives (Figure 9D).
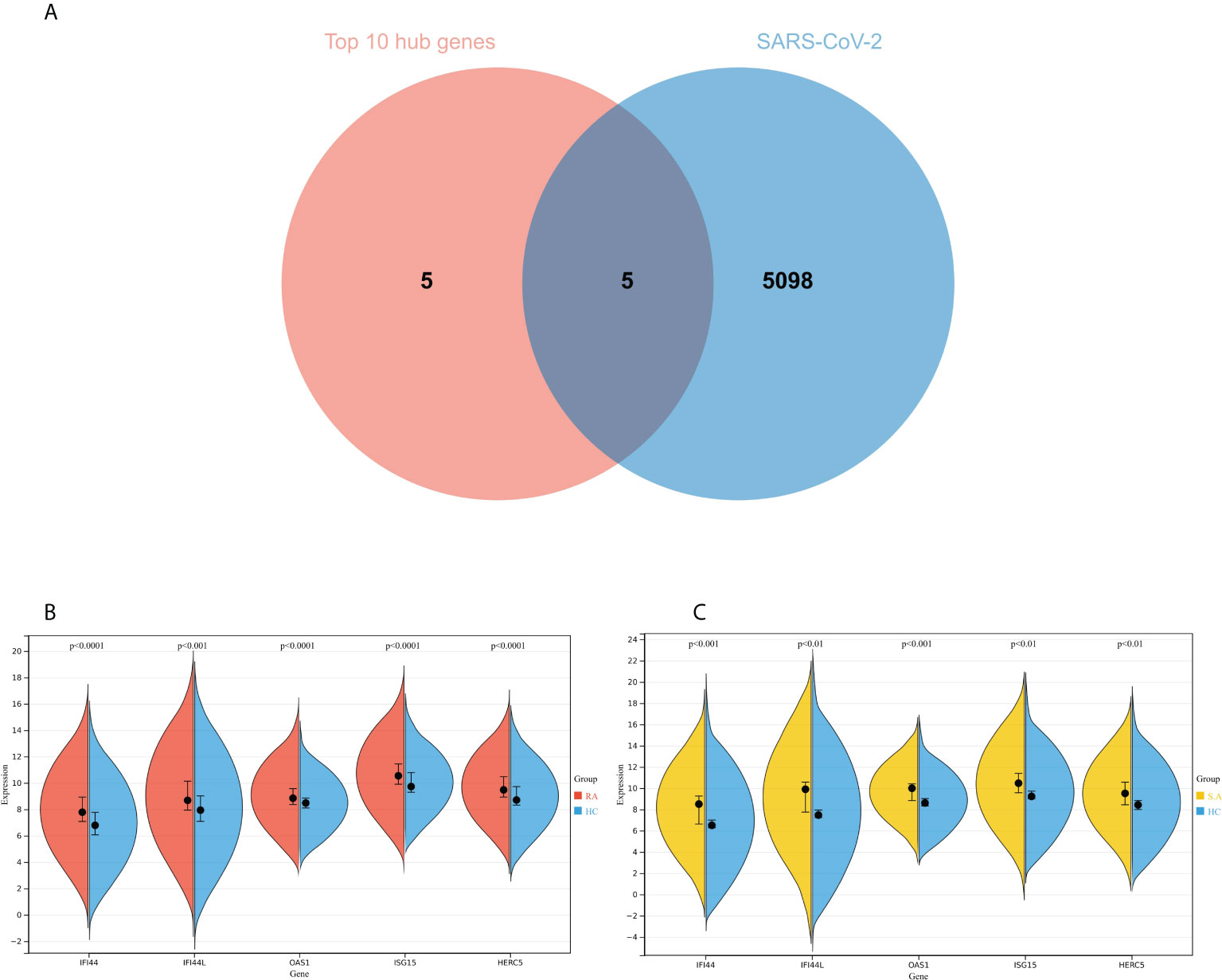
Figure 8 Identification of the hub gene between RA, COVID-19, and SAB. (A) Venn diagram of the top 10 hub genes and the SARS-CoV-2 gene set. (B, C) The expression of IFI44, OAS1, IFI44L, ISG15, and HERC5 in the GSE93272 and GSE33341 datasets was analyzed using split-face violin plots. Red indicates the RA group, yellow indicates the S. aureus group, and blue indicates the HC group.
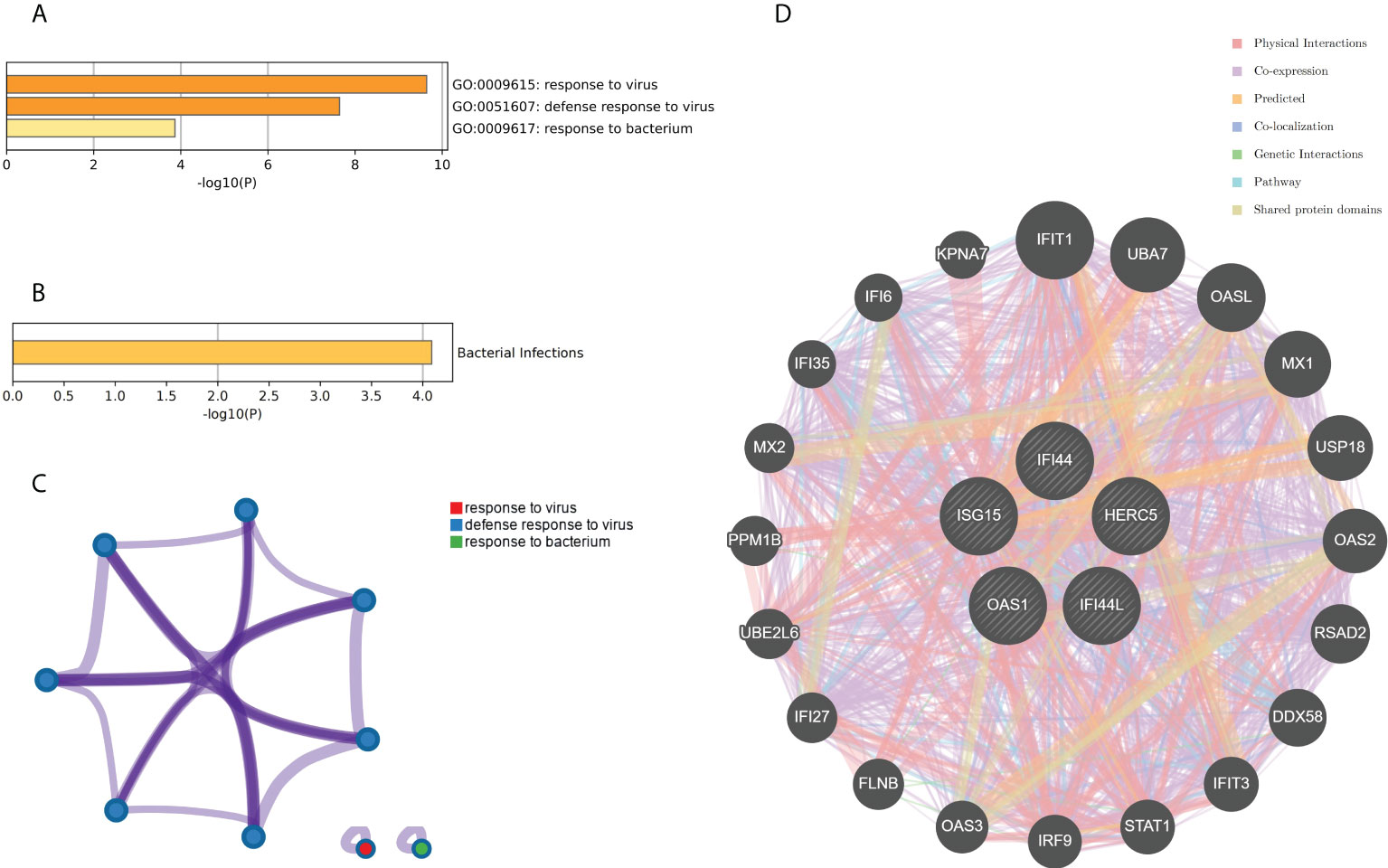
Figure 9 Functional enrichment analysis: Metascape and GeneMANIA of five hub genes. (A) Pathway and process richness analysis of the Metascape platform. (B) Summary of enrichment analysis of DisGeNET 13 on Metascape platform. (C) The network is visualized using Cytoscape 5, colored by cluster IDs, and nodes sharing the same cluster ID are usually close to each other. (D) The gene–gene interaction network of the five hub genes with the 20 most adjacent genes was analyzed using the GeneMANIA database. Each node represents a gene. The color of the linkage of the nodes represents the linkage between the corresponding genes.
Analyses of the network of TF-hub genes and miRNA-hub genes
The TF of five hub genes was predicted using the ENCODE database and the NetworkAnalyst web tool. The miRNAs of five hub genes were analyzed using the miRTarBase v8.0 package and the TarBase v8.0 package of the NetworkAnalyst web tool to build the networks of TF-hub genes and miRNA-hub genes, respectively. The TF-hub gene network includes three seeds, 81 edges, and 81 nodes (Figure 10A), and the simplified minimum network includes three seeds, four edges, and five nodes (Figure 10B). YY1 has the potential to regulate ISG15 and IFI44, and SIN3A and ZNF580 have the potential to regulate ISG15 and HERC5. The network structure of miRNA-hub genes analyzed using the miRTarBase v8.0 package includes four seeds, 26 edges, and 26 nodes (Figure 10C). The simplified minimum network includes four seeds, six edges, and six nodes (Figure 10D). The network structure of miRNA-hub genes analyzed by the TarBase v8.0 package includes five seeds, 188 edges, and 94 nodes (Figure 10E). The simplified minimum network includes five seeds, 16 edges, and 10 nodes (Figure 10F). The intersection of these two miRNA-hub gene networks could reveal that hsa-mir-1-3p and hsa-mir-146a-5p may play an important role in the expression of IFI44.
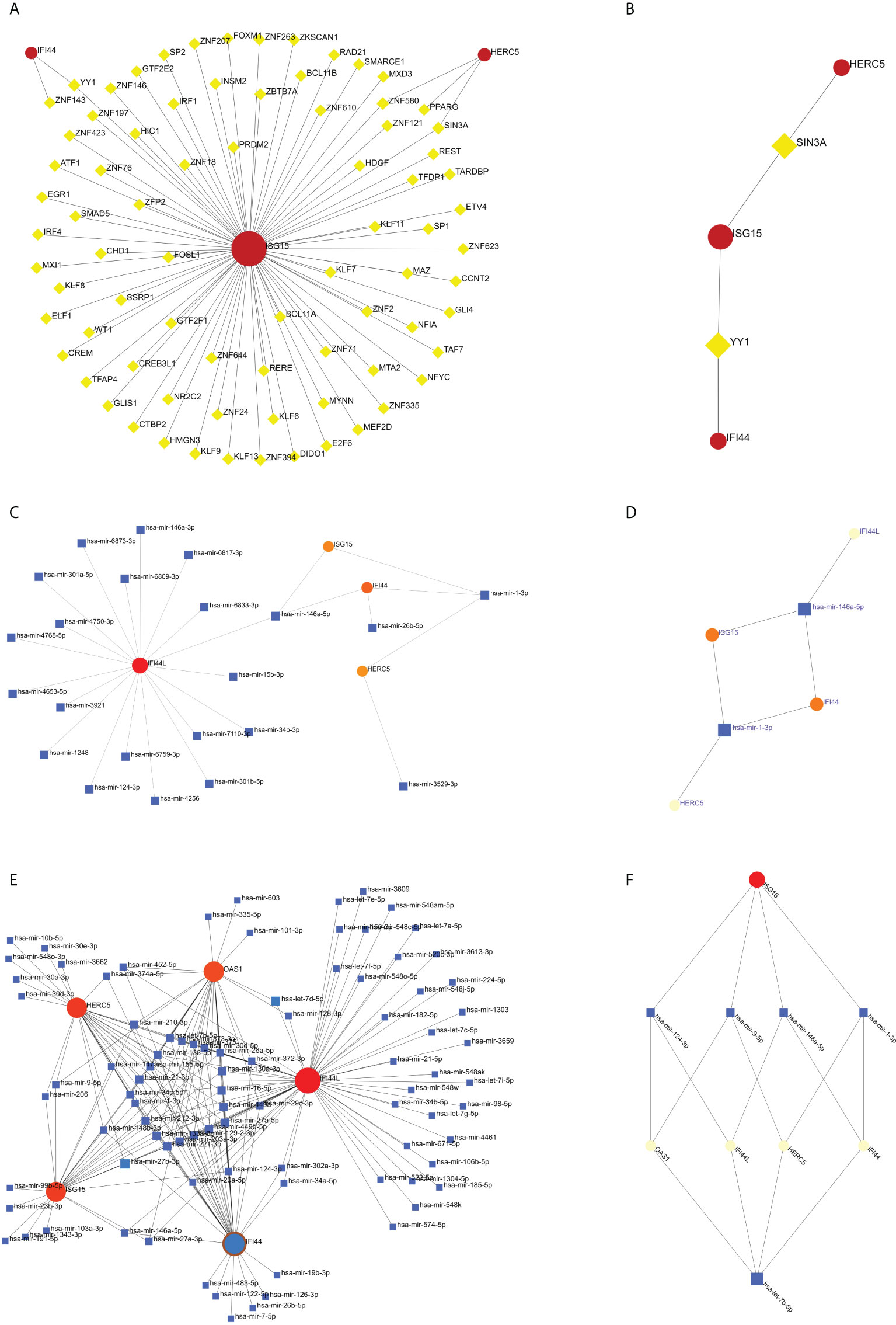
Figure 10 Construction of TF-hub gene and miRNA-hub gene network using NetworkAnalyst. (A, B) TF-hub gene network and simplified diagram. Red circles are genes, and yellow squares are TF. (C, D) miRNA-hub gene network (miRTarBase v8.0) and simplified diagram. (E, F) miRNA-hub gene network (TarBase v8.0) and simplified diagram. Circles are genes, and squares are miRNAs.
Validation of hub genes
To improve the reliability and reproducibility of the results, we used the datasets GSE17755, GSE55235, and GSE13670 for validation. A total of 669 DEGs were obtained from the GSE17755 dataset, of which 471 were upregulated genes and 198 were downregulated genes. In addition, 2,230 DEGs were obtained from the GSE55235 dataset, of which 1,279 were upregulated genes and 951 were downregulated genes. A total of 3063 DEGs were obtained from the GSE13670 dataset, of which 1,100 upregulated genes and 1,963 downregulated genes were used. The distribution of DEGs in these three datasets was visualized using a volcano map, respectively (Figures 11A–C). The Venn diagram of five hub genes with the three validation sets of DEGs shows that IFI44 is the only intersection result (Figure 11D). IFI44 was highly expressed in all three validation sets (P < 0.01) (Figures 12A–C). Finally, the diagnostic validity of IFI44 as a biomarker was verified by ROC curves, which showed that the AUC values of IFI44 on the datasets GSE17755, GSE55235, and GSE13670 were 0.96 (95% CI, 0.95–0.96), 0.90 (95% CI, 0.77–1.00), and 0.79 (95% CI, 0.59–0.98). All had high sensitivity and high specificity (Figures 12D–F).
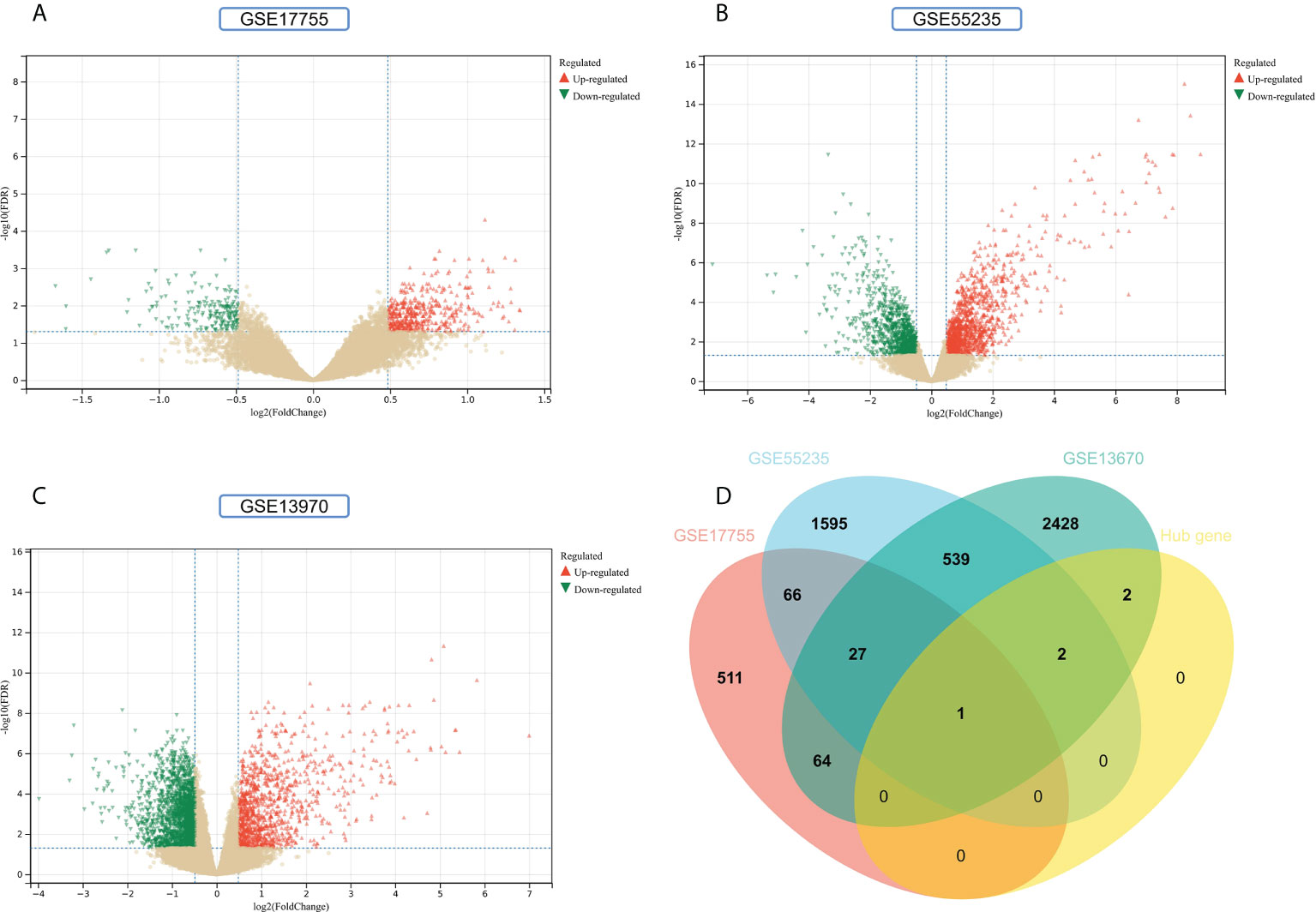
Figure 11 Screening for key genes. (A, B) Gray dots represent genes not substantially differently expressed in RA and HC groups (P > 0.05), red triangles represent upregulated genes (P < 0.05), and green triangles represent downregulated genes (P < 0.05) in GSE17755 and GSE55235 datasets. (C) Gray dots represent genes not substantially differently expressed in S. aureus and HC groups (P > 0.05), red triangles represent upregulated genes (P < 0.05), and green triangles represent downregulated genes (P < 0.05) in the GSE13670 dataset. (D) The Venn diagram of five hub genes with the three validation sets of DEGs.
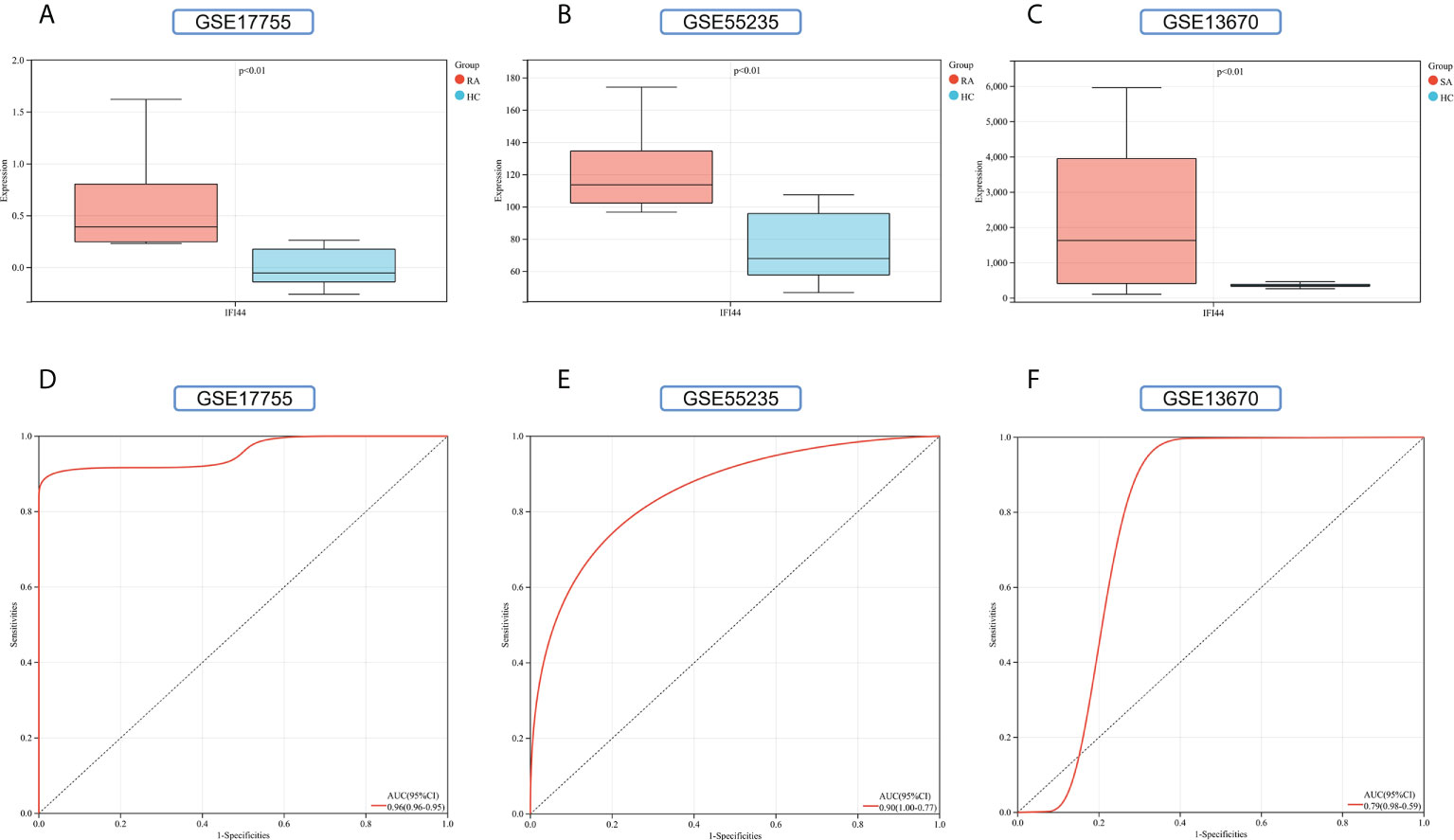
Figure 12 Validation of key genes. (A–C) The expression of IFI44 in GSE17755, GSE55235, and GSE13670. Red for RA/S. aureus group, and cyan for HC group. (D–F) The AUC of the ROC curve verifies the diagnostic validity of IFI44 in GSE17755, GSE55235, and GSE13670 (P < 0.05).
Immune infiltration analysis
We mapped 22 immune cell proportions in RA samples using CIBERSORT (Figure 13A) and then analyzed the differences in immune cell infiltration between RA and HC using box plots (Figure 13B). The results indicated that RA enriched four types of immune cells: B-cell memory, T-cell gamma delta, activated dendritic cells (DCs), and neutrophils (P < 0.05). Further correlation matrix analysis revealed that activated DCs were positively correlated with B-cell memory and T-cell gamma delta and negatively correlated with neutrophils (P < 0.05) (Figure 13C). Finally, we revealed the relationship between the expression of IFI44 and the abundance of immune cells in RA by Pearson’s correlation coefficient analysis (Figure 13D), which showed that only activated DCs were closely and positively correlated with IFI44 (R = 0.68, P = 3.7e-39), and activated DCs were highly enriched in RA. Thus, IFI44 may be involved in RA progression by regulating immune cell infiltration, and activated DCs may play an important role in this regard.
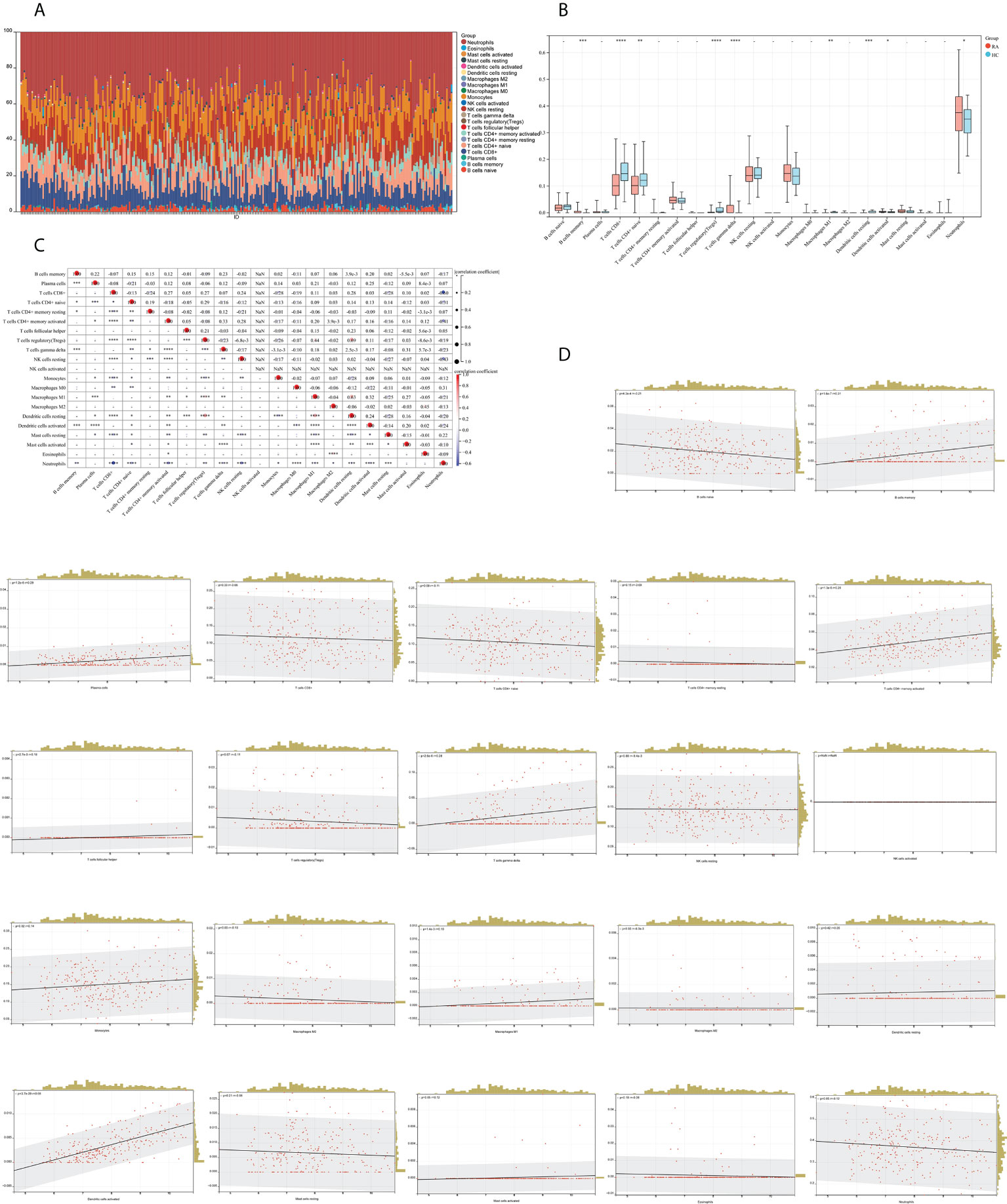
Figure 13 Analysis of immune cell infiltration. (A) A histogram of the proportion of LM22 in RA samples is depicted using the CIBERSORT algorithm, with the horizontal coordinate representing the sample and the vertical coordinate representing the percentage of individual immune cells. (B) Comparison of immune infiltrating cells between the RA and HC groups; red represents RA and cyan represents HC. (C) Correlation matrix between immune cells within the RA group. The horizontal and vertical coordinates are LM22, with red representing positive correlations and blue representing negative correlations (*P < 0.05, ** P < 0.01, and *** P < 0.001). (D) Correlation analysis between the expression of IFI44 and LM22.
Discussion
In this study, a total of 199 DEGs were obtained using a dataset of whole blood samples from RA and SAB (GSE93272 and GSE33341), and they were found to be closely associated with positive/negative regulation of the immune system and regulation of the IFN-I (IFN-α/β) pathway and related pathways of the immune system response to a virus by KEGG, GO, and ClueGO analyses. Ten hub genes were obtained using the PPI network and Cytoscape platform: RSAD2, IFIT3, GBP1, RTP4, IFI44, OAS1, IFI44L, ISG15, HERC5, and IFIT5, which were analyzed by Metascape platform and found to be associated with IFN signaling regulation and immune system response to viral infection and bacterial infection and were closely related. Five hub genes shared by RA, COVID-19, and SAB were IFI44, OAS1, IFI44L, ISG15, and HERC5, and they were found to be closely associated with the immune system response to viral infection and bacterial infection using Metascape analysis. TF-hub gene network and miRNA-hub gene network was constructed for these five hub genes, and one important TF (YY1) and two important miRNAs (hsa-mir-1-3p and hsa-mir-146a-5p) associated with IFI44 were obtained. To verify the reliability and comprehensiveness of the results, not only whole blood samples from RA (GSE17755) but also synovial tissue samples from RA (GSE55235) and blood samples from S. aureus-infected human mononuclear cells (GSE13670) were used to validate a core gene, which was obtained as IFI44. IFI44 was highly expressed in all five datasets, and its test efficacy was verified using ROC. Immune infiltration analysis reveals that the immune cells closely associated with IFI44 are activated DCs, which may play a significant connection between RA, SARS-CoV-2, and S. aureus infection. The pathway enrichment analysis revealed that 1,25-dihydroxy vitamin D3 might be an effective therapeutic agent for RA’s SARS-CoV-2 and S. aureus infections.
Association of this study with The IFN-ISG pathways
IFNs are a family of cytokines having pleiotropic effects in humans (103, 104)—first recognized by Isaacs and Lindenmann in 1957 (105, 106) and characterized as antiviral inhibitors (107, 108). After more than 50 years of research by biologists, it was discovered that IFN is an essential regulator of the body’s immune system (109), which plays a crucial role not only in viral infections (110–112) but also in bacterial infections (113, 114) and autoimmune illnesses (115, 116). There are three types of IFNs: IFN-I (IFN-α, β, ϵ, κ, and ω), IFN-II (IFN-γ), and IFN-III (IFN-λ), with IFN signaling through the Janus kinase (JAK)/STAT pathway (117).
Pattern recognition receptors (PRRs) recognize pathogen-associated molecular patterns (PAMPs) (118–120) and can activate transcription factors like IRF-3 and IRF-7, as well as the NF-κB pathway of B cells (121–124). NOD-like receptors are among the important PRRs that initiate the IFN pathway. TBK-1 and IKKϵ phosphorylate IRF-3 and IRF-7 to stimulate the transcription of IFN and proinflammatory genes (125, 126), with STING serving as the upstream signaling molecule that recruits TBK-1 and IKK (127). cGAS is an important cytosolic DNA sensing that can induce IFN formation by generating the cGAMP pathway that activates STING to form the cGAS-STING pathway (128–130). Activation of the cGAS-STING pathway is a double-edged sword that plays not only a crucial function in fighting viruses (131, 132) and bacteria (133, 134) but also an aberrant activation of cGAS by its DNA, which can provoke autoimmune disorders (135). The NOD-like receptor signaling pathway, oxidative phosphorylation, and cytosolic DNA sensing in the KEGG pathway of the intersecting genes of RA and SAB in this study are reflected in the IFN pathway. In cells that are not activated by the signal, NF-κB is prevented in the cytoplasm by IκBs, and only when IκBs are phosphorylated and hydrolyzed by proteases does NF-κB migrate to the nucleus to induce the production of IFN and proinflammatory genes (136, 137). IKK is responsible for the phosphorylation of IκBs, and it consists of two kinase subunits (IKKα and IKKβ) and one regulatory subunit (IKKγ) (138, 139). Notably, FKBP5 activates IKKϵ (140), interacts with the three subunits of IKK, and promotes IKK synthesis, leading to phosphorylation of IκBs, activation of NF-κB, and its migration into the nucleus, which eventually initiates the IFN signaling pathway (141, 142).
The receptor that binds IFN-I is composed of IFNAR1 and IFNAR2 subunits (143–145), whereas the receptor that binds IFN-III is composed of IFNLR1 and IL-10R subunits (146–148). By interacting with the receptor, IFN activates JAK1 and tyrosine kinase 2 (TYK2) (149–151). Activated JAK1 and TYK2 phosphorylate and activate STAT1 and STAT2 (152–154), whereas active STAT1 and STAT2 recruit and bind IRF-9 to form ISGF3 (155, 156). The ISGF3 complex can move from the cytoplasm to the nucleus and bind to the ISRE region in the ISG promoter, thereby beginning ISG transcription (143, 157, 158). ISGs influence cell activation and death in addition to viral aspects (159), and the antiviral, antiproliferative, and immunological stress actions of ISGs allow cells and organisms to survive (160). Although IFN-I and IFN-III produce ISGs through the same mechanism, the IFN-I pathway can induce ISG expression earlier, more swiftly, and more efficiently (157, 161), and IFN-I has been the subject of most studies, triggering the production of more than 300 ISGs (162). In this study, ClueGO was used to analyze the intersectional gene enrichment pathways of RA and SAB in IFN-I production, regulation of IFN-I production, regulation of IFN-I–mediated signaling pathway, IFN-I signaling pathway, IFN-α/β production, regulation IFN-α/β production, negative regulation of immune response, negative regulation of innate immune response, regulation of viral replication process, response to a virus, defense response to viruses, and cellular response to a virus, which is reflected in the IFN-I pathway.
IFN, ISG, and IFI44 in RA
On the basis of the findings of this study, a portion of the route of the top 10 hub genes of RA and SAB was enriched in IFN signaling and cellular response to cytokine stimulus; therefore, the association between RA and the IFN signaling pathway piqued our interest. Studies have shown that enhanced autoimmune responses can be detected in the presence of disease treated with IFN-α (163) and that 34% of patients have elevated rheumatoid factors (164), and that IFN-α treatment can contribute to the progression of RA (165, 166). In addition, the use of IFN-β1 in the treatment of MS promotes the development of RA (167). In contrast, TNF, a key driver of RA, enhances mtDNA release and initiates a cGAS/STING-dependent IFN response in inflammatory arthritis (168), and prolonged TNF therapy induces the creation of high quantities of IFN-I via a mechanism that stimulates IRF1 and IRF3 (169, 170). It has also been shown that significant amounts of IFN-I can be discovered in the peripheral blood of both patients with preclinical and clinical RA (115) and the synovial fluid of patients with RA (171). In reality, it dates back to 1979, when it was discovered that IFN levels were elevated in individuals with AID and positively linked with the disease’s activity (172). The possible reason for this is that PAMPs are recognized by PRRs that produce IFN-I. These PRRs include TLR, RLR, and cGAS receptors that can sense nucleic acids (173, 174).
Interestingly, these PRRs can recognize viral nucleic acids and their nucleic acids to trigger AID (175). RA is one of the most common AIDs, and IFN-I plays an important role in contributing to the development of RA (115, 176). Furthermore, IFN-I can be used as an RA biomarker and a predictor of disease progression in patients with RA (177). Recent investigations have identified a significant expression of IFN-I–induced ISGs in the peripheral blood of patients with RA (176), and this elevated expression of ISGs induced by the IFN-I signaling pathway is referred to as the IFN signature of RA (178). In peripheral blood (179, 180) and synovial fluid of patients with RA (171, 176), elevated amounts of ISGs were found. Although patients with RA correlate unequally with IFN-I and ISGs (181), IFN-I and ISGs play a role in RA susceptibility (177), and thus, IFN and ISGs are considered biomarkers and disease targets for RA (179, 182, 183).
In combating pathogenic infections, many ISGs act directly on the signaling pathways of the pathogen’s life cycle to inhibit its proliferation (158, 184). However, in RA, the excessive innate immune response and signaling dysregulation produce large amounts of IFNs that damage the organism (185). IFN desensitization is, therefore, of particular importance (158). The first aspect is cell intrinsic, which reduces signaling by blocking the JAK-STAT pathway via endocytosis and turnover of IFN receptors (186–189). The second aspect is that, during the immune response, some ISGs function as negative feedback regulators to maintain cellular homeostasis (158, 190, 191), and some ISGs can act as inhibitory proteins to reduce IFN pathway transduction (192). Common ISGs with negative regulatory functions include SOCS and USP18. Increased SOCS protein levels decrease the sensitivity of the JAK-STAT system, whose mechanism of action is to suppress JAK activity by binding to IFN receptors and tyrosine residues on JAK, thus preventing STAT-1 activation (193). By binding to the IFN-I receptor, USP18 can also prevent JAK activation and induce IFN-I desensitization (194). In addition, it was reported for the first time in 2019 that IFI44 also functions as a negative regulator of the IFN signaling pathway and that IFN-α treatment induces high expression of IFI44 (195) and also triggers the development of RA (196), which corresponds to our study’s finding of high expression of IFI44 in patients with RA.
IFI44 is one of the IFN-I–induced ISGs (197, 198), which was initially found in hepatitis C virus–associated microtubule aggregation protein isolation (199). Therefore, we also observed hepatitis C (chronic) pathway enrichment in the top 10 hub genes of RA versus SAB. IFI44, with the assistance of FKBP5, is capable of exhibiting the two actions listed below. First, IFI44 significantly decreases the kinase activity of IKKβ, which inhibits the phosphorylation of IκBs, which, in turn, limits NF-κB activation and restricts its migration into the nucleus (200). Second, IFI44 can reduce the kinase activity of IKKϵ, resulting in the inhibition of IRF-3 phosphorylation (125), the restriction of STAT1 phosphorylation, and the reduction of ISG production (153). The reason for the high expression of IFI44 in patients with RA is that the high expression of IFNs and ISGs in patients with RA leads to an increase in the expression of IFI44 as an ISG, and it is the negative feedback regulation of IFI44 that makes its expression significantly higher than that of the healthy population. In the results of this study, a portion of the pathways of the top 10 hub genes of RA and SAB were enriched in immune responses to viral and bacterial infections. A portion of the pathways of the top five hub genes of RA, SAB, and COVID-19 was also enriched in immune responses to viral and bacterial infections. Therefore, we followed this thought regarding the IFN pathway and continued exploring the relationship between RA, SAB, and COVID-19.
Crosstalk between RA and SAB in terms of IFN, ISG, and IFI44
The average life expectancy of the RA population is reported to be shortened by 8 to 15 years, with infections, cardiovascular disease, and kidney disease being the three leading reasons (201–203). S. aureus seems inseparable from the topic of infection in patients with RA, as studies from the 1950s indicate that patients with RA are at a significantly increased risk of infection with S. aureus (201) and that invasion of patients with RA by S. aureus can result in severe deep bone and joint infections, as well as high rates of disability and mortality (47, 204). IFN-I has a crucial role in bacterial invasion of the host (205, 206), which can be both useful and damaging to the organism (207, 208), depending on the type of invading bacteria and the organism’s regulatory mechanisms (113, 209). IFN-I generated by S. aureus exacerbates the recruitment of leukocytes and the release of inflammatory cytokines, with detrimental effects on the organism (210–212). Because RA is an autoimmune disease capable of producing large levels of cytokines such as IFNs and ISGs, the relationship between RA and SAB via the IFN-I pathway can be described as follows.
On the one hand, the following points are of interest from the perspective of IFN-I–positive signaling. First, the TLR9 receptor identifies the DNA of S. aureus, causing DCs to produce IFN-I (213). Second, S. aureus detects TLR9-IRF1 via the Xr domain of SpA to activate the JAK-STAT pathway and NF-κB signaling pathway, resulting in the production of inflammatory cytokines such as TNF and IL-6, which promote inflammation and contribute to the progression of RA (211). Third, the autolysis process of S. aureus that produces peptidoglycan, among others, activates the NOD2/IRF5 pathway of DCs to mediate the IFN-I pathway, which enhances the virulence of S. aureus in the host to increase bacterial pathogenicity and also over-recruits neutrophils to promote inflammatory responses (210). Therefore, when patients with RA are infected with S. aureus, it leads to a severe proinflammatory response, probably because the superposition of the two proinflammatory mechanisms leads to an excessive inflammatory response and a severe imbalance in the immune system, followed by a collapse of the immune system, leading to a decrease in the body’s defenses and further aggravating the S. aureus infection, thus creating a vicious circle. On the other hand, examining the issue from the standpoint of ISGs with a negative feedback regulatory effect on the IFN-I pathway yields the following conclusions. First, SOCS has a pro-bacterial effect because it makes it easier for S. aureus to invade an organism’s defenses (214). SOCS not only inhibits the MYD88 molecule in macrophages to affect their antimicrobial effect (215, 216) but also inhibits the NF-κB pathway to reduce TNF release to act as an inhibitor of inflammation, thereby causing problems for host clearance of S. aureus (217), and an increase in phagocytosis and killing of S. aureus by the organism is observed when SOCS is inhibited (214). Second, USP18 can boost the susceptibility of S. aureus by negatively regulating the IFN-I pathway to reduce TNF-α signaling, and inhibition of USP18 can improve the body’s bacterial infection status (218).
SOCS and USP18 proteins have been reported to promote bacterial infection, whereas few IFI44 proteins have been studied. In our study, IFI44 was found to be a key crosstalk gene between RA and SAB, and IFI44 is also an IFN-I–negative regulator, which can give a decrease in antimicrobial inflammatory factors by negatively regulating the NF-κB pathway and can also inhibit STAT1 activation from blocking the production of IFN-I and ISGs (195). Thus, IFI44 may also potentially promote RA susceptibility to S. aureus. Many studies have suggested that the IFN-I pathway acts as a paradoxical immune response during bacterial infection of the host (218), which may be due to the different focus of the IFN-I pathway on the different stages of bacterial infection. The high expression of IFI44 protein in patients with early RA facilitates further invasion of the organism by S. aureus, which is one of the reasons for S. aureus susceptibility, and the vicious cycle of immune imbalance in the organism resulting from the excessive IFN-I cascade response prompted by late RA and S. aureus stimulation is one of the reasons for the poor prognosis and high mortality. We, therefore, suggest that the negative regulation of the IFN-I pathway by IFI44 expression may be one of the mechanisms of immune escape from S. aureus. However, most of the functions of IFI44 are unknown, and further investigation of its mechanisms in bacterial infection is a direction of interest.
Crosstalk between RA, COVID-19, and SAB in terms of IFN, ISG, and IFI44
The coronavirus class is typically characterized by pandemic transmission and high pathogenicity; SARS-COV-2 is the ninth coronavirus identified as a severe threat to human health in 2019 (219–221). SARS-COV-2 is an enveloped virus of the genus Betacoronavirus with a positive-stranded single-stranded RNA genome of 26–32 kb in length (222–225). A virus is divided into four genera: α-, β-, δ-, and γ-CoV, characterized by high mutation rates and diverse recombination rates (226–229), and from 2019 to November 2021, the World Health Organization (WHO) has published Alpha (B.1.1.7), Beta (B.1.351), Gamma (P.1), Delta (B.1.617.2), and Omicron (B.1.1.529) for a total of five variants of concern (VOCs) (230). RA is associated with COVID-19 in the following points. On the one hand, SARS-CoV-2 can overstimulate the body’s immune system and has the potential for antigenic cross-reactivity with the body to trigger the creation of autoantibodies (58, 60, 62). Thus, SARS-CoV-2 infection is considered a trigger for autoimmune disease and results in a worse prognosis (231–235). On the other hand, studies indicate that patients with rheumatic disorders are at a larger risk of SARS-COV-2 infection than the general population, with a worse prognosis and increased mortality (236, 237). In the COVID-19 Global Rheumatology Alliance (C19-GRA) Global Registry and other studies, the most common rheumatic disease among patients with COVID-19 was RA (238–241). Therefore, we prefer to propose that SARS-CoV-2 infection triggers the progression of RA, that patients with RA are more susceptible to SARS-CoV-2 infection, and that the crosstalk between the two results in a vicious cycle of mutual disease progression that increases the risk of hospitalization and death (242–244), and that the crosstalk mechanism cannot be separated from the immune system and related inflammatory pathways (245).
In addition, COVID-19 combined/secondary S. aureus infection results in a considerable increase in mortality (246) primarily due to the following factors. First, patients with COVID-19 on admission had fewer coinfections with bacteria (3.5%) due to preventive administration of antibiotics, the most prevalent of which was S. aureus (81, 247–250). Second, in literature comprising 10 studies, a total of 132 bacterial species were reported as coinfections/secondary infections in patients with COVID-19 after admission, with S. aureus being the most common (n = 41.31%) (251). Third, according to a French study, 28% of critically ill COVID-19 patients admitted to the ICU had coinfections with bacteria, primarily S. aureus (252). We list a portion of the relevant literatures between RA, SAB, and COVID-19 (Table 5). In another bioinformatics investigation, S. aureus infection was shown to be the second highest in the KEGG analysis pathway enrichment order table for RA and COVID-19 (253), a result that was confirmed in our work, suggesting that there may be a connection between the IFN-I pathway in RA, COVID-19, and SAB.
IFN-I is among the most effective cytokines secreted by the organism against SARS-CoV-2 (254, 255). However, it is not always protective for the organism. In the late stage of COVID-19, the continual strong expression of IFN-I causes inflammatory damage to the immune system and many organs, increasing the organism’s burden (256–258). It is undeniable that the IFN-I pathway had an important role in antagonizing the early stages of COVID-19 infection by secreting ISGs during the SARS-CoV-2 invasion (259, 260). However, the ISGs are not the only antiviral factors. Although most ISGs encode proteins capable of inhibiting different stages of the SARS-CoV-2 replication cycle (143, 261, 262), a few ISGs, including SOCS, USP18, and IFI44, can promote viral infection of the host (263–268). It was shown that silencing of IFI44 inhibits viral replication and overexpression of IFI44 promotes viral production due to negative regulation of the IFN-I pathway by IFI44 (195). Viruses mentioned in this study are not limited to SeV, LCMV, VSV, and IAV. Therefore, we suggest that the negative regulation of the IFN-I pathway by the expression of IFI44 may be one of the mechanisms of SARS-CoV-2 immune escape.
High expression of IFN-α in RA contributed to elevated levels of IFI44, promoted viral replication during the early stages of SARS-CoV-2 invasion, and increased susceptibility of S. aureus. Therefore, IFI44 may be an important target for the immune escape of SARS-CoV-2 and S. aureus infection in RA. Of course, we still need basic experiments and clinical trials to validate the results of our bioinformatics analysis.
1,25(OH)2VD3 may be an effective therapeutic agent in treating RA with SARS-CoV-2 and S. aureus infections
Finally, we also found that part of the pathway of the top 10 hub genes of RA and SAB was enriched in non-genomic actions of 1,25-dihydroxy vitamin D3 and that IFI44 was positively correlated with DCs in an immune infiltration correlation analysis in RA. We put 1,25(OH)2VD3 in series with RA, S. aureus infection, COVID-19, IFI44, and DCs (Figure 14). First, in RA, 1,25(OH)2VD3 insufficiency is commonly reported among patients with RA (269–271). In a meta-analysis of 24 studies, 1,25(OH)2VD3 was found to be inversely linked with RA disease activity (272), and the degree of deficiency was utilized as an indication of RA progression (273). Second, in SAB, 1,25(OH)2VD3 was able to prevent the invasion of S. aureus by boosting the expression of mature macrophages, upregulating macrophage complement receptor immunoglobulin (CRIg), and encouraging macrophage phagocytosis (274, 275). Studies have shown that 1,25(OH)2VD3 levels are significantly lower in S. aureus-infected populations than in non–S. aureus–infected populations (276), and 1,25(OH)2VD3 analogs reduce the incidence of PJI in S. aureus infections (277, 278). Third, in COVID-19, according to a study conducted in Israel, 1,25(OH)2VD3 levels were adversely correlated with COVID-19 (279), and COVID-19 populations were frequently associated with vitamin D deficiency (280–282). 1,25(OH)2VD3 insufficiency is positively associated with the severity and complications of COVID-19 and increases the chance of SARS-CoV-2 infection (283–286). The main reason for this is the ability to inhibit the cytokine storm and excessive inflammatory response in COVID-19 (287); thus, vitamin D can play a role in the prevention (288, 289), mitigation (285, 290), and treatment (291, 292) of COVID-19 (293). Fourth, in IFI44, the addition of 1,25(OH)2VD3 to MDDCs in autoimmune diseases (SLE) resulted in a 34% reduction in IFI44 expression and the concentration of 1,25(OH)2VD3 was negatively correlated with the activity of MDDCs in SLE (294). In our study, the expression of IFI44 was found to be positively correlated with DCs, so 1,25(OH)2VD3 may also have some correlation with DCs. Fifth, in DCs, it was discovered that 1,25(OH)2VD3 and its analogs inhibited DC chemotactic activity and IFN-α production, which decreased the expression of ISGs (295, 296). In addition, it has also been shown that DCs are potential target cells of 1,25(OH)2VD3 for RA inhibition (297). Therefore, in this study, 1,25(OH)2VD3 was found to be a drug target through the enrichment pathway of the shared genes of RA and SAB, and 1,25(OH)2VD3 was found to be negatively associated with the expression of RA, COVID-19, SAB, IFI44, and the production and chemotactic activity of IFN-α in DCs from a new perspective.
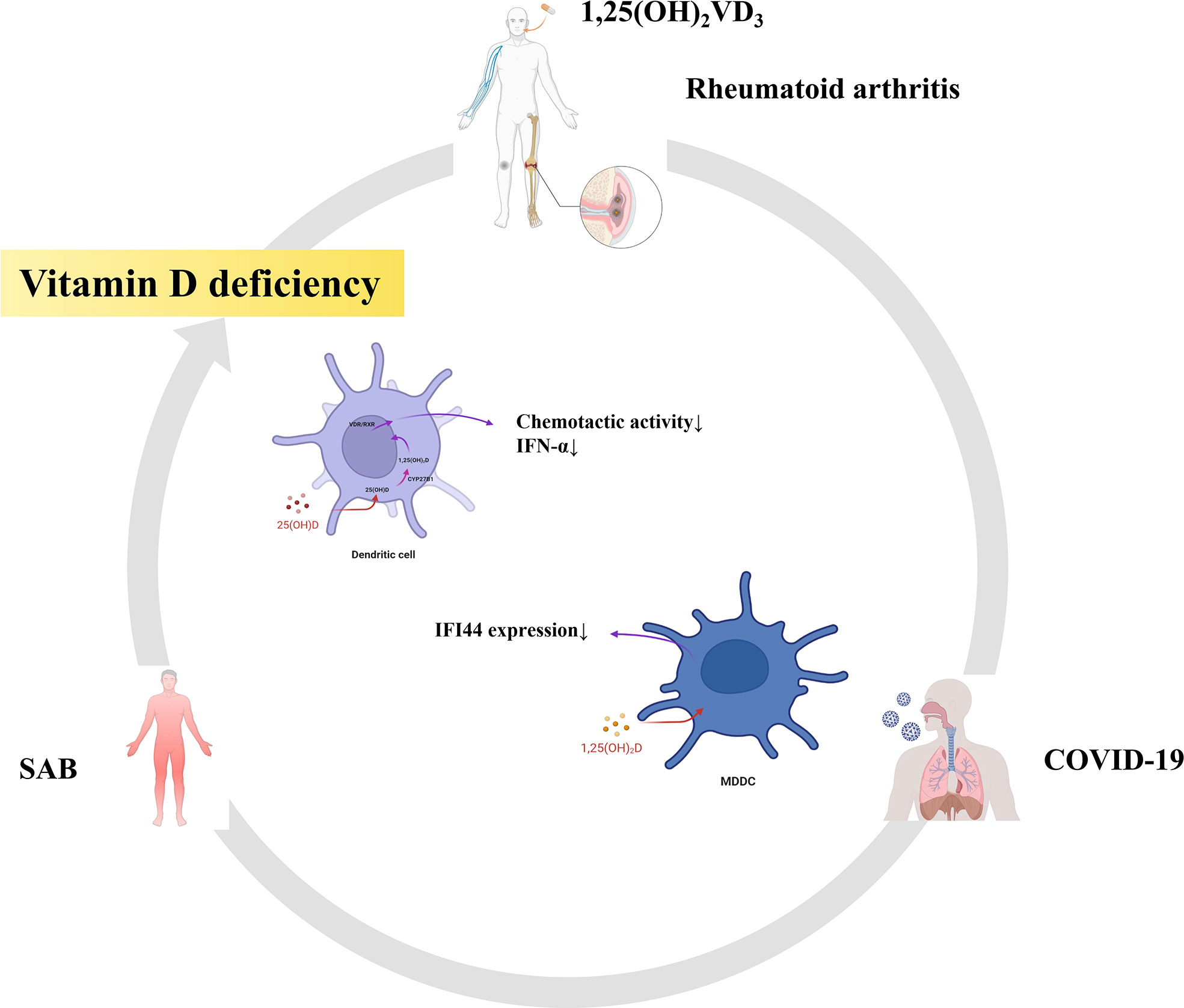
Figure 14 RA, SAB, and COVID-19 are often associated with vitamin D deficiency. This diagram shows that 1,25(OH)2VD3 is the common target drug for RA, SAB, COVID-19, IFI44, and dendritic cells.
Conclusions
In our present study, we screened the shared DEGs based on two datasets of RA (GSE93272) and SAB (GSE33341) and identified pathways associated with immunity and viral infection by multi-platform functional enrichment analysis. The following intersections were taken with the COVID-19 gene library to obtain hub genes, and functional enrichment analysis was performed to validate the pathway linkage of hub genes associated with RA, COVID-19, and SAB. The biomarker and disease target shared by RA, COVID-19, and SAB were validated and identified as IFI144 by GSE17755, GSE55235, and GSE13670 datasets. IFI44, a negative regulator of the IFN signaling pathway, promotes viral replication and bacterial proliferation and is an important molecular target for SARS-CoV-2 and S. aureus immune escape in RA. DC activation was positively correlated with the expression of IFI44. 1,25(OH)2VD3 may be an important therapeutic agent in treating RA with SARS-CoV-2 and S. aureus infections. Our research can provide new directions for further analysis of its pathogenesis and targeted development of clinical treatments.
Data availability statement
The datasets presented in this study can be found in online repositories. The names of the repository/repositories and accession number(s) can be found in the article/supplementary material.
Author contributions
QZ analyzed and wrote the manuscript. DW designed the experiments and analyzed the data. WW devised the concept and supervised the study. All authors contributed to the article and approved the submitted version.
Acknowledgments
We acknowledge the GEO and Genecards databases for providing their platforms and contributors for uploading meaningful datasets.
Conflict of interest
The authors declare that the research was conducted in the absence of any commercial or financial relationships that could be construed as a potential conflict of interest.
Publisher’s note
All claims expressed in this article are solely those of the authors and do not necessarily represent those of their affiliated organizations, or those of the publisher, the editors and the reviewers. Any product that may be evaluated in this article, or claim that may be made by its manufacturer, is not guaranteed or endorsed by the publisher.
Glossary
References
1. Lee DM, Weinblatt ME. Rheumatoid arthritis. Lancet. (2001) 358(9285):903–11. doi: 10.1016/S0140-6736(01)06075-5
2. Scott DL, Wolfe F, Huizinga TW. Rheumatoid arthritis. Lancet. (2010) 376(9746):1094–108. doi: 10.1016/S0140-6736(10)60826-4
3. Smolen JS, Aletaha D, McInnes IB. Rheumatoid arthritis. Lancet. (2016) 388(10055):2023–38. doi: 10.1016/S0140-6736(16)30173-8
4. McInnes IB, Schett G. The pathogenesis of rheumatoid arthritis. N Engl J Med (2011) 365(23):2205–19. doi: 10.1056/NEJMra1004965
5. Smolen JS, Aletaha D, Barton A, Burmester GR, Emery P, Firestein GS, et al. Rheumatoid arthritis. Nat Rev Dis Primers. (2018) 4:18001. doi: 10.1038/nrdp.2018.1
6. van der Woude D, van der Helm-van Mil AHM. Update on the epidemiology, risk factors, and disease outcomes of rheumatoid arthritis. Best Pract Res Clin Rheumatol (2018) 32:174–87. doi: 10.1016/j.berh.2018.10.005
7. McInnes IB, Schett G. Pathogenetic insights from the treatment of rheumatoid arthritis. Lancet. (2017) 389(10086):2328–37. doi: 10.1016/S0140-6736(17)31472-1
8. Choy E. Understanding the dynamics: pathways involved in the pathogenesis of rheumatoid arthritis. Rheumatol (Oxford) (2012) 51(Suppl 5):v3–11. doi: 10.1093/rheumatology/kes113
9. Liu L, Wong CW, Han M, Farhoodi HP, Liu G, Liu Y, et al. Meta-analysis of preclinical studies of mesenchymal stromal cells to treat rheumatoid arthritis. EBioMedicine. (2019) 47:47:563–577. doi: 10.1016/j.ebiom.2019.08.073
10. Cordtz RL, Hawley S, Prieto-Alhambra D, Højgaard P, Zobbe K, Overgaard S, et al. Incidence of hip and knee replacement in patients with rheumatoid arthritis following the introduction of biological DMARDs: an interrupted time-series analysis using nationwide Danish healthcare registers. Ann Rheum Dis (2018) 77(5):684–9. doi: 10.1136/annrheumdis-2017-212424
11. Firestein GS, McInnes IB. Immunopathogenesis of rheumatoid arthritis. Immunity (2017) 46:183–96. doi: 10.1016/j.immuni.2017.02.006
12. Karami J, Aslani S, Jamshidi A, Garshasbi M, Mahmoudi M. Genetic implications in the pathogenesis of rheumatoid arthritis; an updated review. Gene (2019) 702:8–16. doi: 10.1016/j.gene.2019.03.033
13. Joo YB, Lim YH, Kim KJ, Park KS, Park YJ. Respiratory viral infections and the risk of rheumatoid arthritis. Arthritis Res Ther (2019) 21(1):199. doi: 10.1186/s13075-019-1977-9
14. Bartold PM, Marino V, Cantley M, Haynes DR. Effect of porphyromonas gingivalis-induced inflammation on the development of rheumatoid arthritis. J Clin Periodontol (2010) 37:405–11. doi: 10.1111/j.1600-051X.2010.01552.x
15. Puntis D, Malik S, Saravanan V, Rynne M, Heycock C, Hamilton J, et al. Urinary tract infections in patients with rheumatoid arthritis. Clin Rheumatol (2013) 32:355–60. doi: 10.1007/s10067-012-2129-7
16. Klatt T, Ouyang Q, Flad T, Koetter I, Bühring HJ, Kalbacher H, et al. Expansion of peripheral CD8+ CD28– T cells in response to Epstein-Barr virus in patients with rheumatoid arthritis. J Rheumatol (2005) 32:239–51.
17. Kawahito Y, Ichinose S, Sano H, Tsubouchi Y, Kohno M, Yoshikawa T, et al. Mycoplasma fermentans glycolipid-antigen as a pathogen of rheumatoid arthritis. Biochem Biophys Res Commun (2008) 369:561–6. doi: 10.1016/j.bbrc.2008.02.079
18. Hitchon CA, El-Gabalawy HS. Infection and rheumatoid arthritis: still an open question. Curr Opin Rheumatol (2011) 23(4):352–7. doi: 10.1097/BOR.0b013e3283477b7b
19. Rimoin DL, Wennberg JE, John E. Acute septic arthritis complicating chronic rheumatoid arthritis. JAMA (1966) 196:617–13. doi: 10.1001/jama.1966.03100200057018
20. Baum J. Infection in rheumatoid arthritis. Arthritis Rheum (1971) 14:135–7. doi: 10.1002/art.1780140119
21. McLean-Tooke A, Aldridge C, Waugh S, Spickett GP, Kay L. Methotrexate, rheumatoid arthritis and infection risk: what is the evidence? Rheumatol (Oxford). (2009) 48(8):867–71. doi: 10.1093/rheumatology/kep101
22. Singh JA, Furst DE, Bharat A, Curtis JR, Kavanaugh AF, Kremer JM, et al. Update of the 2008 American college of rheumatology recommendations for the use of disease-modifying antirheumatic drugs and biologic agents in the treatment of rheumatoid arthritis. Arthritis Care Res (Hoboken) (2012) 64(5):625–39. doi: 10.1002/acr.21641
23. Lahiri M, Dixon WG. Risk of infection with biologic antirheumatic therapies in patients with rheumatoid arthritis. Best Pract Res Clin Rheumatol (2015) 29:290–305. doi: 10.1016/j.berh.2015.05.009
24. Smolen JS, Landewe R, Breedveld FC, Buch M, Burmester G, Dougados M, et al. EULAR recommendations for the management of rheumatoid arthritis with synthetic and biological disease-modifying antirheumatic drugs: 2013 update. Ann Rheum Dis (2014) 73(3):492–509. doi: 10.1136/annrheumdis-2013-204573
25. Ramiro S, Sepriano A, Chatzidionysiou K, Nam JL, Smolen JS, van der Heijde D, et al. Safety of synthetic and biological DMARDs: a systematic literature review informing the 2016 update of the EULAR recommendations for management of rheumatoid arthritis. Ann Rheum Dis (2017) 76:1101–36. doi: 10.1136/annrheumdis-2016-210708
26. Singh JA, Cameron C, Noorbaloochi S, Cullis T, Tucker M, Christensen R, et al. Risk of serious infection in biological treatment of patients with rheumatoid arthritis: a systematic review and meta-analysis. Lancet. (2015) 386(9990):258–65. doi: 10.1016/S0140-6736(14)61704-9
27. Dixon WG. Rheumatoid arthritis: biological drugs and risk of infection. Lancet. (2015) 386(9990):224–5. doi: 10.1016/S0140-6736(14)61907-3
28. Bongartz T, Sutton AJ, Sweeting MJ, Buchan I, Matteson EL, Montori V. Anti-TNF antibody therapy in rheumatoid arthritis and the risk of serious infections and malignancies: systematic review and meta-analysis of rare harmful effects in randomized controlled trials. JAMA: J Am Med Assoc (2006) 295(19):2275–85. doi: 10.1001/jama.295.19.2275
29. Arleevskaya MI, Larionova RV, Brooks WH, Bettacchioli E, Renaudineau Y. Toll-like receptors, infections, and rheumatoid arthritis. Clin Rev Allergy Immunol (2020) 58(2):172–81. doi: 10.1007/s12016-019-08742-z
30. Carty SM, Snowden N, Silman AJ. Should infection still be considered as the most likely triggering factor for rheumatoid arthritis? J Rheumatol (2003) 30(3):425–9.
31. Dieperink SS, Glintborg B, Oestergaard LB, Nørgaard M, Benfield T, Mehnert F, et al. Risk of staphylococcus aureus bacteraemia in patients with rheumatoid arthritis and the effect of orthopaedic implants on the risk: a nationwide observational cohort study. Scand J Rheumatol (2022) 20:1–9. doi: 10.1080/03009742.2022.2049057
32. Lowy FD. Staphylococcus aureus infections. N Engl J Med (1998) 339(8):520–32. doi: 10.1056/NEJM199808203390806
33. Foster TJ. Nasal colonization by staphylococcus aureus. Nat Med (2004) 10(5):447. doi: 10.1038/nm0504-447a
34. Wertheim HF, Melles DC, Vos MC, van Leeuwen W, van Belkum A, Verbrugh HA, et al. The role of nasal carriage in staphylococcus aureus infections. Lancet Infect Dis (2005) 5:751–62. doi: 10.1016/S1473-3099(05)70295-4
35. Lowy FD. How staphylococcus aureus adapts to its host. N Engl J Med (2011) 364(21):1987–90. doi: 10.1056/NEJMp1100251
36. Naber CK. Staphylococcus aureus bacteremia: epidemiology, pathophysiology, and management strategies. Clin Infect Dis (2009) 48 Suppl 4:S231–7. doi: 10.1086/598189
37. von Eiff C, Becker K, Machka K, Stammer H, Peters G. Nasal carriage as a source of staphylococcus aureus bacteremia. study group. N Engl J Med (2001) 344(1):11–6. doi: 10.1056/NEJM200101043440102
38. Hidron AI, Edwards JR, Patel J, Horan TC, Sievert DM, Pollock DA, et al. NHSN annual update: antimicrobial-resistant pathogens associated with healthcare-associated infections: annual summary of data reported to the national healthcare safety network at the centers for disease control and prevention, 2006–2007. Infect Control Hosp Epidemiol (2008) 29:996–1011. doi: 10.1086/591861
39. Lauderdale KJ, CL M, BR B, Morcuende J, Horswill AR. Biofilm dispersal of community-associated methicillin-resistant staphylococcus aureus on orthopedic implant material. J Orthop Res (2010) 28:55–61. doi: 10.1002/jor.20943
40. Magill SS, Hellinger W, Cohen J, Kay R, Bailey C, Boland B, et al. Prevalence of healthcare-associated infections in acute care hospitals in Jacksonville, Florida. Infect Control Hosp Epidemiol (2012) 33:283–91. doi: 10.1086/664048
41. Kapadia BH, Berg RA, Daley JA, Fritz J, Bhave A, Mont MA. Periprosthetic joint infection. Lancet. (2016) 387(10016):386–94. doi: 10.1016/S0140-6736(14)61798-0
43. Herwaldt LA. Staphylococcus aureus nasal carriage and surgical-site infections. Surgery. (2003) 134(5 Suppl):S2–9. doi: 10.1016/s0039-6060(03)00390-8
44. Kluytmans J, van Belkum A, Verbrugh H. Nasal carriage of staphylococcus aureus: epidemiology, underlying mechanisms, and associated risks. Clin Microbiol Rev (1997) 10(3):505–20. doi: 10.1128/CMR.10.3.505
45. Tabarya D, Hoffman WL. Staphylococcus aureus nasal carriage in rheumatoid arthritis: antibody response to toxic shock syndrome toxin-1. Ann Rheum Dis (1996) 55(11):823–8. doi: 10.1136/ard.55.11.823
46. Goodman SM, Nocon AA, Selemon NA, Shopsin B, Fulmer Y, Decker ME, et al. Increased staphylococcus aureus nasal carriage rates in rheumatoid arthritis patients on biologic therapy. J Arthroplasty. (2019) 34(5):954–8. doi: 10.1016/j.arth.2019.01.025
47. Joost I, Kaasch A, Pausch C, Peyerl-Hoffmann G, Schneider C, Voll RE, et al. Staphylococcus aureus bacteremia in patients with rheumatoid arthritis - data from the prospective INSTINCT cohort. J Infect (2017) 74(6):575–84. doi: 10.1016/j.jinf.2017.03.003
48. Chen N, Zhou M, Dong X, Qu J, Gong F, Han Y, et al. Epidemiological and clinical characteristics of 99 cases of 2019 novel coronavirus pneumonia in wuhan, China: a descriptive study. Lancet. (2020) 395(10223):507–13. doi: 10.1016/S0140-6736(20)30211-7
49. Zhu N, Zhang D, Wang W, Li X, Yang B, Song J, et al. China Novel coronavirus investigating and research team. a novel coronavirus from patients with pneumonia in China, 2019. n. Engl J Med (2020) 382(8):727–33. doi: 10.1056/NEJMoa2001017
50. Huang C, Wang Y, Li X, Ren L, Zhao J, Hu Y, et al. Clinical features of patients infected with 2019 novel coronavirus in wuhan. China. Lancet (2020) 395(10223):497–506. doi: 10.1016/S0140-6736(20)30183-5
51. Liang W, Guan W, Chen R, Wang W, Li J, Xu K, et al. Cancer patients in SARS-CoV-2 infection: a nationwide analysis in China. Lancet Oncol (2020) 21(3):335–7. doi: 10.1016/S1470-2045(20)30096-6
52. Pollard CA, Morran MP, Nestor-Kalinoski AL. The COVID-19 pandemic: a global health crisis. Physiol Genomics (2020) 52(11):549–57. doi: 10.1152/physiolgenomics.00089.2020
53. Johns Hopkins Coronavirus Resource Center. COVID-19 dashboard. JHU (2021). Available at: https://coronavirus.jhu.edu/map.html.
54. Harrison AG, Lin T, Wang P. Mechanisms of SARS-CoV-2 transmission and pathogenesis. Trends Immunol (2020) 41(12):1100–15. doi: 10.1016/j.it.2020.10.004
55. Abd El-Aziz TM, Stockand JD. Recent progress and challenges in drug development against COVID-19 coronavirus (SARS-CoV-2) - an update on the status. Infect Genet Evol (2020) 83:104327. doi: 10.1016/j.meegid.2020.104327
56. Sohrabi C, Alsafi Z, O’Neill N, Khan M, Kerwan A, Al-Jabir A, et al. World health organization declares global emergency: A review of the 2019 novel coronavirus (COVID-19). Int J Surg (2020) 76:71–6. doi: 10.1016/j.ijsu.2020.02.034
57. Hu B, Guo H, Zhou P, Shi ZL. Characteristics of SARS-CoV-2 and COVID-19. Nat Rev Microbiol (2021) 19(3):141–54. doi: 10.1038/s41579-020-00459-7
58. Ruan Q, Yang K, Wang W, Jiang L, Song J. Clinical predictors of mortality due to COVID-19 based on an analysis of data of 150 patients from wuhan, China. Intensive Care Med (2020) 46(5):846–8. doi: 10.1007/s00134-020-05991-x
59. Dotan A, Muller S, Kanduc D, David P, Halpert G, Shoenfeld Y. The SARS-CoV-2 as an instrumental trigger of autoimmunity. Autoimmun Rev (2021) 20(4):102792. doi: 10.1016/j.autrev.2021.102792
60. Vojdani A, Kharrazian D. Potential antigenic cross-reactivity between SARS-CoV-2 and human tissue with a possible link to an increase in autoimmune diseases. Clin Immunol (2020) 217:108480. doi: 10.1016/j.clim.2020.108480
61. Kim H, Byun JE, Yoon SR, Koohy H, Jung H, Choi I. SARS-CoV-2 peptides bind to NKG2D and increase NK cell activity. Cell Immunol (2022) 371:104454. doi: 10.1016/j.cellimm.2021.104454
62. Liu Y, Sawalha AH, Lu Q. COVID-19 and autoimmune diseases. Curr Opin Rheumatol (2021) 33(2):155–62. doi: 10.1097/BOR.0000000000000776
63. Zhong J, Shen G, Yang H, Huang A, Chen X, Dong L, et al. COVID-19 in patients with rheumatic disease in hubei province, China: a multicentre retrospective observational study. Lancet Rheumatol (2020) 2(9):e557–64. doi: 10.1016/S2665-9913(20)30227-7
64. Pablos JL, Galindo M, Carmona L, Lledó A, Retuerto M, Blanco R, et al. RIER investigators group; RIER investigators group. clinical outcomes of hospitalised patients with COVID-19 and chronic inflammatory and autoimmune rheumatic diseases: a multicentric matched cohort study. Ann Rheum Dis (2020) 79(12):1544–9. doi: 10.1136/annrheumdis-2020-218296
65. Raiker R, DeYoung C, Pakhchanian H, Ahmed S, Kavadichanda C, Gupta L, et al. Outcomes of COVID-19 in patients with rheumatoid arthritis: A multicenter research network study in the united states. Semin Arthritis Rheumatol (2021) 51(5):1057–66. doi: 10.1016/j.semarthrit.2021.08.010
66. England BR, Roul P, Yang Y, Kalil AC, Michaud K, Thiele GM, et al. Risk of COVID-19 in rheumatoid arthritis: A national veterans affairs matched cohort study in At-risk individuals. Arthritis Rheumatol (2021) 73(12):2179–88. doi: 10.1002/art.41800
67. Paget C, Trottein F. Mechanisms of bacterial superinfection post-influenza: A role for unconventional T cells. Front Immunol (2019) 10:336. doi: 10.3389/fimmu.2019.00336
68. McArdle AJ, Turkova A, Cunnington AJ. When do co-infections matter? Curr Opin Infect Dis (2018) 31(3):209–15. doi: 10.1097/QCO.0000000000000447
69. Gupta RK, George R, Nguyen-Van-Tam JS. Bacterial pneumonia and pandemic influenza planning. Emerg Infect Dis (2008) 14(8):1187–92. doi: 10.3201/eid1408.070751
70. Metzger DW, Sun K. Immune dysfunction and bacterial coinfections following influenza. J Immunol (2013) 191(5):2047–52. doi: 10.4049/jimmunol.1301152
71. Jia L, Xie J, Zhao J, Cao D, Liang Y, Hou X, et al. Mechanisms of severe mortality-associated bacterial Co-infections following influenza virus infection. Front Cell Infect Microbiol (2017) 7:338. doi: 10.3389/fcimb.2017.00338
72. Quah J, Jiang B, Tan PC, Siau C, Tan TY. Impact of microbial aetiology on mortality in severe community-acquired pneumonia. BMC Infect Dis (2018) 18(1):451. doi: 10.1186/s12879-018-3366-4
73. Katsurada N, Suzuki M, Aoshima M, Yaegashi M, Ishifuji T, Asoh N, et al. Adult pneumonia study group-japan. the impact of virus infections on pneumonia mortality is complex in adults: a prospective multicentre observational study. BMC Infect Dis (2017) 17(1):755. doi: 10.1186/s12879-017-2858-y
74. Beadling C, Slifka MK. How do viral infections predispose patients to bacterial infections? Curr Opin Infect Dis (2004) 17(3):185–91. doi: 10.1097/00001432-200406000-00003
75. Morens DM, Taubenberger JK, Fauci AS. Predominant role of bacterial pneumonia as a cause of death in pandemic influenza: implications for pandemic influenza preparedness. J Infect Dis (2008) 198(7):962–70. doi: 10.1086/591708
76. Rowe HM, Meliopoulos VA, Iverson A, Bomme P, Schultz-Cherry S, Rosch JW. Direct interactions with influenza promote bacterial adherence during respiratory infections. Nat Microbiol (2019) 4(8):1328–36. doi: 10.1038/s41564-019-0447-0
77. Mulcahy ME, McLoughlin RM. Staphylococcus aureus and influenza a virus: Partners in coinfection. mBio. (2016) 7(6):e02068–16. doi: 10.1128/mBio.02068-16
78. Sun K, Metzger DW. Influenza and staphylococcus aureus coinfection: TLR9 at play. Trends Microbiol (2019) 27(5):383–4. doi: 10.1016/j.tim.2019.02.006
79. Voiriot G, Visseaux B, Cohen J, Nguyen LB, Neuville M, Morbieu C, et al. Viral-bacterial coinfection affects the presentation and alters the prognosis of severe community-acquired pneumonia. Crit Care (2016) 20(1):375. doi: 10.1186/s13054-016-1517-9
80. Abelenda-Alonso G, Rombauts A, Gudiol C, Meije Y, Ortega L, Clemente M, et al. Influenza and bacterial coinfection in adults with community-acquired pneumonia admitted to conventional wards: Risk factors, clinical features, and outcomes. Open Forum Infect Dis (2020) 7(3):ofaa066. doi: 10.1093/ofid/ofaa066
81. Garcia-Vidal C, Sanjuan G, Moreno-García E, Puerta-Alcalde P, Garcia-Pouton N, Chumbita M, et al. COVID-19 researchers group. incidence of co-infections and superinfections in hospitalized patients with COVID-19: a retrospective cohort study. Clin Microbiol Infect (2021) 27(1):83–8. doi: 10.1016/j.cmi.2020.07.041
82. Hughes S, Troise O, Donaldson H, Mughal N, Moore LSP. Bacterial and fungal coinfection among hospitalized patients with COVID-19: a retrospective cohort study in a UK secondary-care setting. Clin Microbiol Infect (2020) 26(10):1395–9. doi: 10.1016/j.cmi.2020.06.025
83. Tasaki S, Suzuki K, Kassai Y, Takeshita M, Murota A, Kondo Y, et al. Multi-omics monitoring of drug response in rheumatoid arthritis in pursuit of molecular remission. Nat Commun (2018) 9(1):2755. doi: 10.1038/s41467-018-05044-4
84. Ahn SH, Tsalik EL, Cyr DD, Zhang Y, van Velkinburgh JC, Langley RJ, et al. Gene expression-based classifiers identify staphylococcus aureus infection in mice and humans. PloS One (2013) 8(1):e48979. doi: 10.1371/journal.pone.0048979
85. Lee HM, Sugino H, Aoki C, Nishimoto N. Underexpression of mitochondrial-DNA encoded ATP synthesis-related genes and DNA repair genes in systemic lupus erythematosus. Arthritis Res Ther (2011) 13(2):R63. doi: 10.1186/ar3317
86. Woetzel D, Huber R, Kupfer P, Pohlers D, Pfaff M, Driesch D, et al. Identification of rheumatoid arthritis and osteoarthritis patients by transcriptome-based rule set generation. Arthritis Res Ther (2014) 16(2):R84. doi: 10.1186/ar4526
87. Koziel J, Maciag-Gudowska A, Mikolajczyk T, Bzowska M, Sturdevant DE, Whitney AR, et al. Phagocytosis of staphylococcus aureus by macrophages exerts cytoprotective effects manifested by the upregulation of antiapoptotic factors. PloS One (2009) 4(4):e5210. doi: 10.1371/journal.pone.0005210
88. Diboun I, Wernisch L, Orengo CA, Koltzenburg M. Microarray analysis after RNA amplification can detect pronounced differences in gene expression using limma. BMC Genomics (2006) 7:252. doi: 10.1186/1471-2164-7-252
89. Ritchie ME, Phipson B, Wu D, Hu Y, Law CW, Shi W, et al. Limma powers differential expression analyses for RNA-sequencing and microarray studies. Nucleic Acids Res (2015) 43(7):e47. doi: 10.1093/nar/gkv007
90. Huang da W, Sherman BT, Lempicki RA. Systematic and integrative analysis of large gene lists using DAVID bioinformatics resources. Nat Protoc (2009) 4:44–57. doi: 10.1038/nprot.2008.211
91. Yu G, Wang LG, Han Y, He QY. Clusterprofiler: An r package for comparing biological themes among gene clusters. OMICS (2012) 16(5):284–7. doi: 10.1089/omi.2011.0118
92. Szklarczyk D, Gable AL, Lyon D, Junge A, Wyder S, Huerta-Cepas J, et al. STRING v11: protein-protein association networks with increased coverage, supporting functional discovery in genome-wide experimental datasets. Nucleic Acids Res (2019) 47(D1):D607–13. doi: 10.1093/nar/gky1131
93. Sarkar D, Saha S. Machine-learning techniques for the prediction of protein-protein interactions. J Biosci (2019) 44(4):104. doi: 10.1007/s12038-019-9909-z
94. Choi J, Yun JS, Song H, Kim NH, Kim HS, Yook JI. Exploring the chemical space of protein-protein interaction inhibitors through machine learning. Sci Rep (2021) 11(1):13369. doi: 10.1038/s41598-021-92825-5
95. Shannon P, Markiel A, Ozier O, Baliga NS, Wang JT, Ramage D, et al. Cytoscape: a software environment for integrated models of biomolecular interaction networks. Genome Res (2003) 13:2498–504. doi: 10.1101/gr.1239303
96. Safran M, Dalah I, Alexander J, Rosen N, Iny Stein T, Shmoish M, et al. GeneCards version 3: the human gene integrator. Database. (2010) 2010:baq020. doi: 10.1093/database/baq020
97. Ziegler CG, Allon SJ, Nyquist SK, Mbano IM, Miao VN, Tzouanas CN, et al. SARS-CoV-2 receptor ACE2 is an interferon-stimulated gene in human airway epithelial cells and is detected in specific cell subsets across tissues. Cell (2020) 181:1016–35:e1019. doi: 10.1016/j.cell.2020.04.035
98. Jain R, Ramaswamy S, Harilal D, Uddin M, Loney T, Nowotny N, et al. Host transcriptomic profiling of COVID-19 patients with mild, moderate, and severe clinical outcomes. Comput Struct Biotechnol J (2021) 19:153–60. doi: 10.1016/j.csbj.2020.12.016
99. Xiong Y, Liu Y, Cao L, Wang D, Guo M, Jiang A, et al. Transcriptomic characteristics of bronchoalveolar lavage fluid and peripheral blood mononuclear cells in COVID-19 patients. Emerg Microbes Infect (2020) 9:761–70. doi: 10.1080/22221751.2020.1747363
100. Zhou G, Soufan O, Ewald J, Hancock REW, Basu N, Xia J. NetworkAnalyst 3.0: a visual analytics platform for comprehensive gene expression profiling and meta-analysis. Nucleic Acids Res (2019) 47(W1):W234–41. doi: 10.1093/nar/gkz240
101. Robin X, Turck N, Hainard A, Tiberti N, Lisacek F, Sanchez JC, et al. pROC: an open-source package for r and s+ to analyze and compare ROC curves. BMC Bioinf (2011) 12:77. doi: 10.1186/1471-2105-12-77
102. Chen B, Khodadoust MS, Liu CL, Newman AM, Alizadeh AA. Profiling tumor infiltrating immune cells with CIBERSORT. Methods Mol Biol (2018) 1711:243–59. doi: 10.1007/978-1-4939-7493-1_12
103. Maher SG, Romero-Weaver AL, Scarzello AJ, Gamero AM. Interferon: cellular executioner or white knight? Curr Med Chem (2007) 14(12):1279–89. doi: 10.2174/092986707780597907
104. Pestka S, Krause CD, Walter MR. Interferons, interferon-like cytokines, and their receptors. Immunol Rev (2004) 202:8–32. doi: 10.1111/j.0105-2896.2004.00204.x
105. Isaacs A, Lindenmann J. Virus interference. i. the interferon. Proc R Soc Lond B Biol Sci (1957) 147(927):258–67. doi: 10.1098/rspb.1957.0048
106. Isaacs A, Lindenmann J, Valentine RC. Virus interference. II. some properties of interferon. Proc R Soc Lond B Biol Sci (1957) 147:268–73. doi: 10.1098/rspb.1957.0049
107. Pestka S, Langer JA, Zoon KC, Samuel CE. Interferons and their actions. Annu Rev Biochem (1987) 56:727–77. doi: 10.1146/annurev.bi.56.070187.003455
108. Pestka S. Interferon from 1981 to 1986. Methods Enzymol (1986) 119:3–14. doi: 10.1016/0076-6879(86)19003-3
109. Duerr CU, Fritz JH. Editorial: Immunoregulatory mechanisms of interferon. Front Immunol (2020) 11:187. doi: 10.3389/fimmu.2020.00187
111. Fensterl V, Chattopadhyay S, Sen GC. No love lost between viruses and interferons. Annu Rev Virol (2015) 2(1):549–72. doi: 10.1146/annurev-virology-100114-055249
112. Samuel CE. Antiviral actions of interferons. Clin Microbiol Rev (2001) 14(4):778–809. doi: 10.1128/CMR.14.4.778-809.2001
113. Kovarik P, Castiglia V, Ivin M, Ebner F. Type I interferons in bacterial infections: A balancing act. Front Immunol (2016) 7:652. doi: 10.3389/fimmu.2016.00652
114. Perry AK, Chen G, Zheng D, Tang H, Cheng G. The host type I interferon response to viral and bacterial infections. Cell Res (2005) 15(6):407–22. doi: 10.1038/sj.cr.7290309
115. Barrat FJ, Crow MK, Ivashkiv LB. Interferon target-gene expression and epigenomic signatures in health and disease. Nat Immunol (2019) 20(12):1574–83. doi: 10.1038/s41590-019-0466-2
116. Wahren-Herlenius M, Dörner T. Immunopathogenic mechanisms of systemic autoimmune disease. Lancet. (2013) 382(9894):819–31. doi: 10.1016/S0140-6736(13)60954-X
117. Chow KT, Gale M Jr. SnapShot: Interferon signaling. Cell. (2015) 163(7):1808–1808.e1. doi: 10.1016/j.cell.2015.12.008
118. Takeuchi O, Akira S. Pattern recognition receptors and inflammation. Cell. (2010) 140(6):805–20. doi: 10.1016/j.cell.2010.01.022
119. Jensen S, Thomsen AR. Sensing of RNA viruses: a review of innate immune receptors involved in recognizing RNA virus invasion. J Virol (2012) 86(6):2900–10. doi: 10.1128/JVI.05738-11
120. Iwasaki A. A virological view of innate immune recognition. Annu Rev Microbiol (2012) 66:177–96. doi: 10.1146/annurev-micro-092611-150203
121. Yoneyama M, Suhara W, Fukuhara Y, Fukuda M, Nishida E, Fujita T. Direct triggering of the type I interferon system by virus infection: activation of a transcription factor complex containing IRF-3 and CBP/p300. EMBO J (1998) 17(4):1087–95. doi: 10.1093/emboj/17.4.1087
122. Honda K, Yanai H, Negishi H, Asagiri M, Sato M, Mizutani T, et al. IRF-7 is the master regulator of type-I interferon-dependent immune responses. Nature. (2005) 434(7034):772–7. doi: 10.1038/nature03464
123. Honda K, Ohba Y, Yanai H, Negishi H, Mizutani T, Takaoka A, et al. Spatiotemporal regulation of MyD88-IRF-7 signalling for robust type-I interferon induction. Nature. (2005) 434(7036):1035–40. doi: 10.1038/nature03547
124. Visvanathan KV, Goodbourn S. Double-stranded RNA activates binding of NF-kappa b to an inducible element in the human beta-interferon promoter. EMBO J (1989) 8(4):1129–38. doi: 10.1002/j.1460-2075.1989.tb03483.x
125. Sharma S, tenOever BR, Grandvaux N, Zhou GP, Lin R, Hiscott J. Triggering the interferon antiviral response through an IKK-related pathway. Science. (2003) 300(5622):1148–51. doi: 10.1126/science.1081315
126. Fitzgerald KA, McWhirter SM, Faia KL, Rowe DC, Latz E, Golenbock DT, et al. IKKepsilon and TBK1 are essential components of the IRF3 signaling pathway. Nat Immunol (2003) 4(5):491–6. doi: 10.1038/ni921
127. Chen Q, Sun L, Chen ZJ. Regulation and function of the cGAS-STING pathway of cytosolic DNA sensing. Nat Immunol (2016) 17(10):1142–9. doi: 10.1038/ni.3558
128. Lio CW, McDonald B, Takahashi M, Dhanwani R, Sharma N, Huang J, et al. cGAS-STING signaling regulates initial innate control of cytomegalovirus infection. J Virol (2016) 90(17):7789–97. doi: 10.1128/JVI.01040-16
129. Zhang G, Chan B, Samarina N, Abere B, Weidner-Glunde M, Buch A, et al. Cytoplasmic isoforms of kaposi sarcoma herpesvirus LANA recruit and antagonize the innate immune DNA sensor cGAS. Proc Natl Acad Sci U S A. (2016) 113(8):E1034–43. doi: 10.1073/pnas.1516812113
130. Schoggins JW, MacDuff DA, Imanaka N, Gainey MD, Shrestha B, Eitson JL, et al. Pan-viral specificity of IFN-induced genes reveals new roles for cGAS in innate immunity. Nature. (2014) 505(7485):691–5. doi: 10.1038/nature12862
131. Li XD, Wu J, Gao D, Wang H, Sun L, Chen ZJ. Pivotal roles of cGAS-cGAMP signaling in antiviral defense and immune adjuvant effects. Science. (2013) 341(6152):1390–4. doi: 10.1126/science.1244040
132. Paijo J, Döring M, Spanier J, Grabski E, Nooruzzaman M, Schmidt T, et al. cGAS senses human cytomegalovirus and induces type I interferon responses in human monocyte-derived cells. PloS Pathog (2016) 12(4):e1005546. doi: 10.1371/journal.ppat.1005546
133. Watson RO, Bell SL, MacDuff DA, Kimmey JM, Diner EJ, Olivas J, et al. The cytosolic sensor cGAS detects mycobacterium tuberculosis DNA to induce type I interferons and activate autophagy. Cell Host Microbe (2015) 17(6):811–9. doi: 10.1016/j.chom.2015.05.004
134. Storek KM, Gertsvolf NA, Ohlson MB, Monack DM. cGAS and Ifi204 cooperate to produce type I IFNs in response to francisella infection. J Immunol (2015) 194(7):3236–45. doi: 10.4049/jimmunol.1402764
135. Crow YJ. Type I interferonopathies: mendelian type I interferon up-regulation. Curr Opin Immunol (2015) 32:7–12. doi: 10.1016/j.coi.2014.10.005
136. Karin M, Ben-Neriah Y. Phosphorylation meets ubiquitination: the control of NF-[kappa]B activity. Annu Rev Immunol (2000) 18:621–63. doi: 10.1146/annurev.immunol.18.1.621
137. Yaron A, Hatzubai A, Davis M, Lavon I, Amit S, Manning AM, et al. Identification of the receptor component of the IkappaBalpha-ubiquitin ligase. Nature. (1998) 396(6711):590–4. doi: 10.1038/25159
138. Karin M. How NF-kappaB is activated: the role of the IkappaB kinase (IKK) complex. Oncogene. (1999) 18(49):6867–74. doi: 10.1038/sj.onc.1203219
139. Zandi E, Chen Y, Karin M. Direct phosphorylation of IkappaB by IKKalpha and IKKbeta: discrimination between free and NF-kappaB-bound substrate. Science. (1998) 281(5381):1360–3. doi: 10.1126/science.281.5381.1360
140. Bouwmeester T, Bauch A, Ruffner H, Angrand PO, Bergamini G, Croughton K, et al. A physical and functional map of the human TNF-alpha/NF-kappa b signal transduction pathway. Nat Cell Biol (2004) 6(2):97–105. doi: 10.1038/ncb1086
141. Romano S, Xiao Y, Nakaya M, D’Angelillo A, Chang M, Jin J, et al. FKBP51 employs both scaffold and isomerase functions to promote NF-κB activation in melanoma. Nucleic Acids Res (2015) 43(14):6983–93. doi: 10.1093/nar/gkv615
142. Erlejman AG, De Leo SA, Mazaira GI, Molinari AM, Camisay MF, Fontana V, et al. NF-κB transcriptional activity is modulated by FK506-binding proteins FKBP51 and FKBP52: a role for peptidyl-prolyl isomerase activity. J Biol Chem (2014) 289(38):26263–76. doi: 10.1074/jbc.M114.582882
143. Schoggins JW, Rice CM. Interferon-stimulated genes and their antiviral effector functions. Curr Opin Virol (2011) 1(6):519–25. doi: 10.1016/j.coviro.2011.10.008
144. de Weerd NA, Vivian JP, Nguyen TK, Mangan NE, Gould JA, Braniff SJ, et al. Structural basis of a unique interferon-β signaling axis mediated via the receptor IFNAR1. Nat Immunol (2013) 14(9):901–7. doi: 10.1038/ni.2667
145. Shemesh M, Lochte S, Piehler J, Schreiber G. IFNAR1 and IFNAR2 play distinct roles in initiating type I interferon-induced JAK-STAT signaling and activating STATs. Sci Signal (2021) 14(710):eabe4627. doi: 10.1126/scisignal.abe4627
146. Kotenko SV, Rivera A, Parker D, Durbin JE. Type III IFNs: Beyond antiviral protection. semin. Immunol. (2019) 43:101303. doi: 10.1016/j.smim.2019.101303
147. Kotenko SV, Gallagher G, Baurin VV, Lewis-Antes A, Shen M, Shah NK, et al. IFN-lambdas mediate antiviral protection through a distinct class II cytokine receptor complex. Nat Immunol (2003) 4(1):69–77. doi: 10.1038/ni875
148. Sheppard P, Kindsvogel W, Xu W, Henderson K, Schlutsmeyer S, Whitmore TE, et al. IL-28, IL-29 and their class II cytokine receptor IL-28R. Nat Immunol (2003) 4(1):63–8. doi: 10.1038/ni873
149. Stark GR, Darnell JE Jr. The JAK-STAT pathway at twenty. Immunity. (2012) 36(4):503–14. doi: 10.1016/j.immuni.2012.03.013
150. Schindler C, Plumlee C. Inteferons pen the JAK-STAT pathway. Semin Cell Dev Biol (2008) 19(4):311–8. doi: 10.1016/j.semcdb.2008.08.010
151. Schnepf D, Crotta S, Thamamongood T, Stanifer M, Polcik L, Ohnemus A, et al. Selective janus kinase inhibition preserves interferon-λ-mediated antiviral responses. Sci Immunol (2021) 6(59):eabd5318. doi: 10.1126/sciimmunol.abd5318
152. Li X, Leung S, Qureshi S, Darnell JE Jr, Stark GR. Formation of STAT1-STAT2 heterodimers and their role in the activation of IRF-1 gene transcription by interferon-alpha. J Biol Chem (1996) 271(10):5790–4. doi: 10.1074/jbc.271.10.5790
153. Wang Y, Song Q, Huang W, Lin Y, Wang X, Wang C, et al. Stark GR. a virus-induced conformational switch of STAT1-STAT2 dimers boosts antiviral defenses. Cell Res (2021) 31(2):206–18. doi: 10.1038/s41422-020-0386-6
154. Ho J, Pelzel C, Begitt A, Mee M, Elsheikha HM, Scott DJ, et al. STAT2 is a pervasive cytokine regulator due to its inhibition of STAT1 in multiple signaling pathways. PloS Biol (2016) 14(10):e2000117. doi: 10.1371/journal.pbio.2000117
155. Platanitis E, Demiroz D, Schneller A, Fischer K, Capelle C, Hartl M, et al. A molecular switch from STAT2-IRF9 to ISGF3 underlies interferon-induced gene transcription. Nat Commun (2019) 10(1):2921. doi: 10.1038/s41467-019-10970-y
156. Rengachari S, Groiss S, JM D, Caron E, Grandvaux N, Panne D. Structural basis of STAT2 recognition by IRF9 reveals molecular insights into ISGF3 function. Proc Natl Acad Sci U S A. (2018) 115(4):E601–9. doi: 10.1073/pnas.1718426115
157. Zhou Z, Hamming OJ, Ank N, Paludan SR, Nielsen AL, Hartmann R. Type III interferon (IFN) induces a type I IFN-like response in a restricted subset of cells through signaling pathways involving both the jak-STAT pathway and the mitogen-activated protein kinases. J Virol (2007) 81(14):7749–58. doi: 10.1128/JVI.02438-06
158. Schneider WM, Chevillotte MD, Rice CM. Interferon-stimulated genes: a complex web of host defenses. Annu Rev Immunol (2014) 32:513–45. doi: 10.1146/annurev-immunol-032713-120231
159. Platanias LC. Mechanisms of type-i- and type-II-interferon-mediated signalling. Nat Rev Immunol (2005) 5(5):375–86. doi: 10.1038/nri1604
160. Schindler C, Levy DE, Decker T. JAK-STAT signaling: from interferons to cytokines. J Biol Chem (2007) 282(28):20059–63. doi: 10.1074/jbc.R700016200
161. Lazear HM, Schoggins JW, Diamond MS. Shared and distinct functions of type I and type III interferons. Immunity. (2019) 50(4):907–23. doi: 10.1016/j.immuni.2019.03.025
162. Der SD, Zhou A, Williams BR, Silverman RH. Identification of genes differentially regulated by interferon alpha, beta, or gamma using oligonucleotide arrays. Proc Natl Acad Sci U S A. (1998) 95(26):15623–8. doi: 10.1073/pnas.95.26.15623
163. Biggioggero M, Gabbriellini L, Meroni PL. Type I interferon therapy and its role in autoimmunity. Autoimmunity. (2010) 43(3):248–54. doi: 10.3109/08916930903510971
164. Borg FA, Isenberg DA. Syndromes and complications of interferon therapy. Curr Opin Rheumatol (2007) 19(1):61–6. doi: 10.1097/BOR.0b013e328010c547
165. Ionescu C, Micu L, Constantinescu I, Hortopan M, Ursaciuc C, Voiculescu M. Prolonged treatment with interferon alpha and peginterferon induces rheumatoid arthritis syndrome and erythema nodosum. J Gastrointestin Liver Dis (2008) 17(2):211–2. doi: 10.1007/s11749-008-0114-x
167. Hojjati SM, Heidari B, Babaei M. Development of rheumatoid arthritis during treatment of multiple sclerosis with interferon beta 1-a. coincidence of two conditions or a complication of treatment: A case report. J Adv Res (2016) 7(5):611–3. doi: 10.1016/j.jare.2016.06.004
168. Willemsen J, Neuhoff MT, Hoyler T, Noir E, Tessier C, Sarret S, et al. TNF leads to mtDNA release and cGAS/STING-dependent interferon responses that support inflammatory arthritis. Cell Rep (2021) 37(6):109977. doi: 10.1016/j.celrep.2021.109977
169. Venkatesh D, Ernandez T, Rosetti F, Batal I, Cullere X, Luscinskas FW, et al. Endothelial TNF receptor 2 induces IRF1 transcription factor-dependent interferon-β autocrine signaling to promote monocyte recruitment. Immunity. (2013) 38(5):1025–37. doi: 10.1016/j.immuni.2013.01.012
170. Yarilina A, Park-Min KH, Antoniv T, Hu X, Ivashkiv LB. TNF activates an IRF1-dependent autocrine loop leading to sustained expression of chemokines and STAT1-dependent type I interferon-response genes. Nat Immunol (2008) 9(4):378–87. doi: 10.1038/ni1576
171. Hopkins SJ, Meager A. Cytokines in synovial fluid: II. the presence of tumour necrosis factor and interferon. Clin Exp Immunol (1988) 73(1):88–92.
172. Hooks JJ, Moutsopoulos HM, Geis SA, Stahl NI, Decker JL, Notkins AL. Immune interferon in the circulation of patients with autoimmune disease. N Engl J Med (1979) 301(1):5–8. doi: 10.1056/NEJM197907053010102
173. Tan X, Sun L, Chen J, Chen ZJ. Detection of microbial infections through innate immune sensing of nucleic acids. Annu Rev Microbiol (2018) 8:72:447–478. doi: 10.1146/annurev-micro-102215-095605
174. Levy DE, Marié IJ, Durbin JE. Induction and function of type I and III interferon in response to viral infection. Curr Opin Virol (2011) 1(6):476–86. doi: 10.1016/j.coviro.2011.11.001
175. Roers A, Hiller B, Hornung V. Recognition of endogenous nucleic acids by the innate immune system. Immunity. (2016) 44(4):739–54. doi: 10.1016/j.immuni.2016.04.002
176. Higgs BW, Liu Z, White B, Zhu W, White WI, Morehouse C, et al. Patients with systemic lupus erythematosus, myositis, rheumatoid arthritis and scleroderma share activation of a common type I interferon pathway. Ann Rheum Dis (2011) 70(11):2029–36. doi: 10.1136/ard.2011.150326
177. Rodríguez-Carrio J, López P, Suárez A. Type I IFNs as biomarkers in rheumatoid arthritis: towards disease profiling and personalized medicine. Clin Sci (Lond). (2015) 128(8):449–64. doi: 10.1042/CS20140554
178. Rönnblom L, Eloranta ML. The interferon signature in autoimmune diseases. Curr Opin Rheumatol (2013) 25(2):248–53. doi: 10.1097/BOR.0b013e32835c7e32
179. van der Pouw Kraan TC, van Baarsen LG, Wijbrandts CA, Voskuyl AE, Rustenburg F, Baggen JM, et al. Expression of a pathogen-response program in peripheral blood cells defines a subgroup of rheumatoid arthritis patients. Genes Immun (2008) 9(1):16–22. doi: 10.1038/sj.gene.6364438
180. Rodríguez-Carrio J, de Paz B, López P, Prado C, Alperi-López M, Ballina-García FJ, et al. IFNα serum levels are associated with endothelial progenitor cells imbalance and disease features in rheumatoid arthritis patients. PloS One (2014) 9(1):e86069. doi: 10.1371/journal.pone.0086069
181. Rodríguez-Carrio J, Alperi-López M, López P, Ballina-García FJ, Suárez A. Heterogeneity of the type I interferon signature in rheumatoid arthritis: A potential limitation for its use as a clinical biomarker. Front Immunol (2018) 8:2007. doi: 10.3389/fimmu.2017.02007
182. van der Pouw Kraan TC, Wijbrandts CA, van Baarsen LG, Voskuyl AE, Rustenburg F, Baggen JM, et al. Rheumatoid arthritis subtypes identified by genomic profiling of peripheral blood cells: assignment of a type I interferon signature in a subpopulation of patients. Ann Rheum Dis (2007) 66(8):1008–14. doi: 10.1136/ard.2006.063412
183. Muskardin TLW, Niewold TB. Type I interferon in rheumatic diseases. Nat Rev Rheumatol (2018) 14(4):214–28. doi: 10.1038/nrrheum.2018.31
184. Iwasaki A, Pillai PS. Innate immunity to influenza virus infection. Nat Rev Immunol (2014) 14(5):315–28. doi: 10.1038/nri3665
185. Rönnblom L. The type I interferon system in the etiopathogenesis of autoimmune diseases. Ups J Med Sci (2011) 116(4):227–37. doi: 10.3109/03009734.2011.624649
186. Coccia EM, Uzé G, Pellegrini S. Negative regulation of type I interferon signaling: facts and mechanisms. Cell Mol Biol (Noisy-le-grand). (2006) 52(1):77–87.
187. ten Hoeve J, de Jesus Ibarra-Sanchez M, Fu Y, Zhu W, Tremblay M, David M, et al. Identification of a nuclear Stat1 protein tyrosine phosphatase. Mol Cell Biol (2002) 22(16):5662–8. doi: 10.1128/MCB.22.16.5662-5668.2002
188. Irie-Sasaki J, Sasaki T, Matsumoto W, Opavsky A, Cheng M, Welstead G, et al. CD45 is a JAK phosphatase and negatively regulates cytokine receptor signalling. Nature. (2001) 409(6818):349–54. doi: 10.1038/35053086
189. Altman JB, Taft J, Wedeking T, Gruber CN, Holtmannspötter M, Piehler J, et al. Type I IFN is siloed in endosomes. Proc Natl Acad Sci U S A. (2020) 117(30):17510–2. doi: 10.1073/pnas.1921324117
190. Richards KH, Macdonald A. Putting the brakes on the anti-viral response: negative regulators of type I interferon (IFN) production. Microbes Infect (2011) 13(4):291–302. doi: 10.1016/j.micinf.2010.12.007
191. Komuro A, Bamming D, Horvath CM. Negative regulation of cytoplasmic RNA-mediated antiviral signaling. Cytokine. (2008) 43(3):350–8. doi: 10.1016/j.cyto.2008.07.011
192. Larner AC, Chaudhuri A, Darnell JE Jr. Transcriptional induction by interferon. new protein(s) determine the extent and length of the induction. J Biol Chem (1986) 261(1):453–9. doi: 10.1016/S0021-9258(17)42492-6
193. Hong XX, Carmichael GG. Innate immunity in pluripotent human cells: attenuated response to interferon-β. J Biol Chem (2013) 288(22):16196–205. doi: 10.1074/jbc.M112.435461
194. Malakhova OA, Kim KI, Luo JK, Zou W, Kumar KG, Fuchs SY, et al. UBP43 is a novel regulator of interferon signaling independent of its ISG15 isopeptidase activity. EMBO J (2006) 25(11):2358–67. doi: 10.1038/sj.emboj.7601149
195. DeDiego ML, Nogales A, Martinez-Sobrido L, Topham DJ. Interferon-induced protein 44 interacts with cellular FK506-binding protein 5, negatively regulates host antiviral responses, and supports virus replication. mBio. (2019) 10(4):e01839-19. doi: 10.1128/mBio.01839-19
196. Nesher G, Ruchlemer R. Alpha-interferon-induced arthritis: clinical presentation treatment, and prevention. Semin Arthritis Rheumatol (1998) 27(6):360–5. doi: 10.1016/s0049-0172(98)80015-2
197. Bain VG, Yoshida EM, Kaita KD, Swain MG, Heathcote EJ, Garcia A, et al. Dynamics of interferon-specific gene expression in peripheral blood of interferon alfa-naïve patients with genotype 1 chronic hepatitis c infection treated with albumin-interferon alfa. Hepatol Res (2006) 35(4):256–62. doi: 10.1016/j.hepres.2006.04.005
198. Kitamura A, Takahashi K, Okajima A, Kitamura N. Induction of the human gene for p44, a hepatitis-c-associated microtubular aggregate protein, by interferon-alpha/beta. Eur J Biochem (1994) 224(3):877–83. doi: 10.1111/j.1432-1033.1994.00877.x
199. Honda Y, Kondo J, Maeda T, Yoshiyama Y, Yamada E, Shimizu YK, et al. Isolation and purification of a non-a, non-b hepatitis-associated microtubular aggregates protein. J Gen Virol (1990) 71(Pt 9):1999–2004. doi: 10.1099/0022-1317-71-9-1999
200. Zandi E, Rothwarf DM, Delhase M, Hayakawa M, Karin M. The IkappaB kinase complex (IKK) contains two kinase subunits, IKKalpha and IKKbeta, necessary for IkappaB phosphorylation and NF-kappaB activation. Cell. (1997) 91(2):243–52. doi: 10.1016/s0092-8674(00)80406-7
201. COBB S, ANDERSON F, BAUER W. Length of life and cause of death in rheumatoid arthritis. N Engl J Med (1953) 249(14):553–6. doi: 10.1056/NEJM195310012491402
202. England BR, Thiele GM, Anderson DR, Mikuls TR. Increased cardiovascular risk in rheumatoid arthritis: mechanisms and implications. BMJ. (2018) 361:k1036. doi: 10.1136/bmj.k1036
203. Suzuki A, Ohosone Y, Obana M, Mita S, Matsuoka Y, Irimajiri S, et al. Cause of death in 81 autopsied patients with rheumatoid arthritis. J Rheumatol (1994) 21(1):33–6.
204. Yeganeh MH, Kheir MM, Shahi A, Parvizi J. Rheumatoid arthritis, disease modifying agents, and periprosthetic joint infection: What does a joint surgeon need to know? J Arthroplasty. (2018) 33(4):1258–64. doi: 10.1016/j.arth.2017.11.031
205. Mancuso G, Midiri A, Biondo C, Beninati C, Zummo S, Galbo R, et al. Type I IFN signaling is crucial for host resistance against different species of pathogenic bacteria. J Immunol (2007) 178(5):3126–33. doi: 10.4049/jimmunol.178.5.3126
206. Manca C, Tsenova L, Freeman S, Barczak AK, Tovey M, Murray PJ, et al. Hypervirulent m. tuberculosis W/Beijing strains upregulate type I IFNs and increase expression of negative regulators of the jak-stat pathway. J Interferon Cytokine Res (2005) 25(11):694–701. doi: 10.1089/jir.2005.25.694
207. Decker T, Müller M, Stockinger S. The yin and yang of type I interferon activity in bacterial infection. Nat Rev Immunol (2005) 5(9):675–87. doi: 10.1038/nri1684
208. McNab F, Mayer-Barber K, Sher A, Wack A, O’Garra A. Type I interferons in infectious disease. Nat Rev Immunol (2015) 15(2):87–103. doi: 10.1038/nri3787
209. Boxx GM, Cheng G. The roles of type I interferon in bacterial infection. Cell Host Microbe (2016) 19(6):760–9. doi: 10.1016/j.chom.2016.05.016
210. Parker D, Planet PJ, Soong G, Narechania A, Prince A. Induction of type I interferon signaling determines the relative pathogenicity of staphylococcus aureus strains. PloS Pathog (2014) 10(2):e1003951. doi: 10.1371/journal.ppat.1003951
211. Martin FJ, Gomez MI, Wetzel DM, Memmi G, O’Seaghdha M, Soong G, et al. Staphylococcus aureus activates type I IFN signaling in mice and humans through the xr repeated sequences of protein a. J Clin Invest. (2009) 119(7):1931–9. doi: 10.1172/jci35879
212. Stifter SA, Feng CG. Interfering with immunity: detrimental role of type I IFNs during infection. J Immunol (2015) 194(6):2455–65. doi: 10.4049/jimmunol.1402794
213. Parker D, Prince A. Staphylococcus aureus induces type I IFN signaling in dendritic cells. via TLR9. J Immunol (2012) 189(8):4040–6. doi: 10.4049/jimmunol.1201055
214. Klopfenstein N, Brandt SL, Castellanos S, Gunzer M, Blackman A, Serezani CH. SOCS-1 inhibition of type I interferon restrains staphylococcus aureus skin host defense. PloS Pathog (2021) 17(3):e1009387. doi: 10.1371/journal.ppat.1009387
215. Serezani CH, Lewis C, Jancar S, Peters-Golden M. Leukotriene B4 amplifies NF-κB activation in mouse macrophages by reducing SOCS1 inhibition of MyD88 expression. J Clin Invest. (2011) 121(2):671–82. doi: 10.1172/JCI43302
216. Piñeros Alvarez AR, Glosson-Byers N, Brandt S, Wang S, Wong H, Sturgeon S, et al. SOCS1 is a negative regulator of metabolic reprogramming during sepsis. JCI Insight (2017) 2(13):e92530. doi: 10.1172/jci.insight.92530
217. Duncan SA, Baganizi DR, Sahu R, Singh SR, Dennis VA. SOCS proteins as regulators of inflammatory responses induced by bacterial infections: A review. Front Microbiol (2017) 8:2431. doi: 10.3389/fmicb.2017.02431
218. Shaabani N, Honke N, Nguyen N, Huang Z, Arimoto KI, Lazar D, et al. The probacterial effect of type I interferon signaling requires its own negative regulator USP18. Sci Immunol (2018) 3(27):eaau2125. doi: 10.1126/sciimmunol.aau2125
219. Holmes EC, Goldstein SA, Rasmussen AL, Robertson DL, Crits-Christoph A, Wertheim JO, et al. The origins of SARS-CoV-2: A critical review. Cell. (2021) 184(19):4848–56. doi: 10.1016/j.cell.2021.08.017
220. Vlasova AN, Diaz A, Damtie D, Xiu L, Toh TH, Lee JS, et al. Novel canine coronavirus isolated from a hospitalized patient with pneumonia in East Malaysia. Clin Infect Dis (2022) 74(3):446–54. doi: 10.1093/cid/ciab456
221. Lednicky JA, Tagliamonte MS, White SK, Elbadry MA, Alam MM, Stephenson CJ, et al. Independent infections of porcine deltacoronavirus among Haitian children. Nature. (2021) 600(7887):133–7. doi: 10.1038/s41586-021-04111-z
222. Masters PS. The molecular biology of coronaviruses. Adv Virus Res (2006) 66:193–292. doi: 10.1016/S0065-3527(06)66005-3
223. Lu R, Zhao X, Li J, Niu P, Yang B, Wu H, et al. Genomic characterisation and epidemiology of 2019 novel coronavirus: implications for virus origins and receptor binding. Lancet. (2020) 395(10224):565–74. doi: 10.1016/S0140-6736(20)30251-8
224. Mittal A, Manjunath K, Ranjan RK, Kaushik S, Kumar S, Verma V. COVID-19 pandemic: Insights into structure, function, and hACE2 receptor recognition by SARS-CoV-2. PloS Pathog (2020) 16(8):e1008762. doi: 10.1371/journal.ppat.1008762
225. Chan JF, Yuan S, Kok KH, To KK, Chu H, Yang J, et al. A familial cluster of pneumonia associated with the 2019 novel coronavirus indicating person-to-person transmission: a study of a family cluster. Lancet. (2020) 395(10223):514–23. doi: 10.1016/S0140-6736(20)30154-9
226. Cui J, Li F, Shi ZL. Origin and evolution of pathogenic coronaviruses. Nat Rev Microbiol (2019) 17(3):181–92. doi: 10.1038/s41579-018-0118-9
227. Dai L, Gao GF. Viral targets for vaccines against COVID-19. Nat Rev Immunol (2021) 21(2):73–82. doi: 10.1038/s41577-020-00480-0
228. Jin Y, Yang H, Ji W, Wu W, Chen S, Zhang W, et al. Virology, epidemiology, pathogenesis, and control of COVID-19. Viruses. (2020) 12(4):372. doi: 10.3390/v12040372
229. Almazán F, Dediego ML, Galán C, Escors D, Alvarez E, Ortego J, et al. Construction of a severe acute respiratory syndrome coronavirus infectious cDNA clone and a replicon to study coronavirus RNA synthesis. J Virol (2006) 80(21):10900–6. doi: 10.1128/JVI.00385-06
230. WHO. Tracking SARS-CoV-2 variants (2021). Available at: https://www.who.int/en/activities/tracking-SARS-CoV-2-variants.
231. Sacchi MC, Tamiazzo S, Stobbione P, Agatea L, De Gaspari P, Stecca A, et al. SARS-CoV-2 infection as a trigger of autoimmune response. Clin Transl Sci (2021) 14(3):898–907. doi: 10.1111/cts.12953
232. Liew IY, Mak TM, Cui L, Vasoo S. Lim XR. a case of reactive arthritis secondary to coronavirus disease 2019 infection. J Clin Rheumatol (2020) 26(6):233. doi: 10.1097/RHU.0000000000001560
233. Ono K, Kishimoto M, Shimasaki T, Uchida H, Kurai D, Deshpande GA, et al. Reactive arthritis after COVID-19 infection. RMD Open (2020) 6(2):e001350. doi: 10.1136/rmdopen-2020-001350
234. Alivernini S, Cingolani A, Gessi M, Paglionico A, Pasciuto G, Tolusso B, et al. Comparative analysis of synovial inflammation after SARS-CoV-2 infection. Ann Rheum Dis (2021) 80(6):e91. doi: 10.1136/annrheumdis-2020-218315
235. Talarico R, Stagnaro C, Ferro F, Carli L, Mosca M. Symmetric peripheral polyarthritis developed during SARS-CoV-2 infection. Lancet Rheumatol (2020) 2(9):e518–9. doi: 10.1016/S2665-9913(20)30216-2
236. Conway R, Grimshaw AA, Konig MF, Putman M, Duarte-García A, Tseng LY, et al. COVID-19 global rheumatology alliance. SARS-CoV-2 infection and COVID-19 outcomes in rheumatic diseases: A systematic literature review and meta-analysis. Arthritis Rheumatol (2022) 74(5):766–75. doi: 10.1002/art.42030
237. Akiyama S, Hamdeh S, Micic D, Sakuraba A. Prevalence and clinical outcomes of COVID-19 in patients with autoimmune diseases: A systematic review and meta-analysis. Ann Rheumatol Dis (2020) 80:384–91. doi: 10.1136/annrheumdis-2020-218946
238. The COVID-19 global rheumatology alliance global registry (2020). Available at: https://rheum-covid.org/updates/combined-data.html.
239. Favalli EG, Ingegnoli F, Cimaz R, Caporali R. What is the true incidence of COVID-19 in patients with rheumatic diseases? Ann Rheum Dis (2021) 80(2):e18. doi: 10.1136/annrheumdis-2020-217615
240. Haberman R, Axelrad J, Chen A, Castillo R, Yan D, Izmirly P, et al. Covid-19 in immune-mediated inflammatory diseases - case series from new York. N Engl J Med (2020) 383(1):85–8. doi: 10.1056/NEJMc2009567
241. Sarzi-Puttini P, Marotto D, Caporali R, Montecucco CM, Favalli EG, Franceschini F, et al. Prevalence of COVID infections in a population of rheumatic patients from Lombardy and marche treated with biological drugs or small molecules: A multicentre retrospective study. J Autoimmun (2021) 116:102545. doi: 10.1016/j.jaut.2020.102545
242. Wang Y, D’Silva KM, Jorge AM, Li X, Lyv H, Wei J, et al. Increased risk of COVID-19 in patients with rheumatoid arthritis: A general population-based cohort study. Arthritis Care Res (Hoboken). (2022) 74(5):741–7. doi: 10.1002/acr.24831
243. Dewanjee S, Kandimalla R, Kalra RS, Valupadas C, Vallamkondu J, Kolli V, et al. COVID-19 and rheumatoid arthritis crosstalk: Emerging association, therapeutic options and challenges. Cells. (2021) 10(12):3291. doi: 10.3390/cells10123291
244. Favalli EG, Maioli G, Biggioggero M, Caporali R. Clinical management of patients with rheumatoid arthritis during the COVID-19 pandemic. Expert Rev Clin Immunol (2021) 17(6):561–71. doi: 10.1080/1744666X.2021.1908887
245. Schett G, Manger B, Simon D, Caporali R. COVID-19 revisiting inflammatory pathways of arthritis. Nat Rev Rheumatol (2020) 16(8):465–70. doi: 10.1038/s41584-020-0451-z
246. Mirzaei R, Goodarzi P, Asadi M, Soltani A, Aljanabi HAA, Jeda AS, et al. Bacterial co-infections with SARS-CoV-2. IUBMB Life (2020) 72(10):2097–111. doi: 10.1002/iub.2356
247. Vaughn VM, Gandhi TN, Petty LA, Patel PK, Prescott HC, Malani AN, et al. Empiric antibacterial therapy and community-onset bacterial coinfection in patients hospitalized with coronavirus disease 2019 (COVID-19): A multi-hospital cohort study. Clin Infect Dis (2021) 72(10):e533–41. doi: 10.1093/cid/ciaa1239
248. Karami Z, Knoop BT, Dofferhoff ASM, Blaauw MJT, Janssen NA, van Apeldoorn M, et al. Few bacterial co-infections but frequent empiric antibiotic use in the early phase of hospitalized patients with COVID-19: results from a multicentre retrospective cohort study in the Netherlands. Infect Dis (Lond). (2021) 53(2):102–10. doi: 10.1080/23744235.2020.1839672
249. Adler H, Ball R, Fisher M, Mortimer K, Vardhan MS. Low rate of bacterial co-infection in patients with COVID-19. Lancet Microbe (2020) 1(2):e62. doi: 10.1016/S2666-5247(20)30036-7
250. Fu Y, Yang Q, Xu M, Kong H, Chen H, Fu Y, et al. Secondary bacterial infections in critical ill patients with coronavirus disease 2019. Open Forum Infect Dis (2020) 7(6):ofaa220. doi: 10.1093/ofid/ofaa220
251. Westblade LF, Simon MS, Satlin MJ. Bacterial coinfections in coronavirus disease 2019. Trends Microbiol (2021) 29(10):930–41. doi: 10.1016/j.tim.2021.03.018
252. Contou D, Claudinon A, Pajot O, Micaëlo M, Longuet Flandre P, Dubert M, et al. Bacterial and viral co-infections in patients with severe SARS-CoV-2 pneumonia admitted to a French ICU. Ann Intensive Care (2020) 10(1):119. doi: 10.1186/s13613-020-00736-x
253. Hu H, Tang N, Zhang F, Li L, Li L. Bioinformatics and system biology approach to identify the influences of COVID-19 on rheumatoid arthritis. Front Immunol (2022) 13:860676. doi: 10.3389/fimmu.2022.860676
254. Lowery SA, Sariol A, Perlman S. Innate immune and inflammatory responses to SARS-CoV-2: Implications for COVID-19. Cell Host Microbe (2021) 29(7):1052–62. doi: 10.1016/j.chom.2021.05.004
255. Park A, Iwasaki A. Type I and type III interferons - induction, signaling, evasion, and application to combat COVID-19. Cell Host Microbe (2020) 27(6):870–8. doi: 10.1016/j.chom.2020.05.008
256. Wilk AJ, Rustagi A, Zhao NQ, Roque J, Martínez-Colón GJ, McKechnie JL, et al. Blish CA. a single-cell atlas of the peripheral immune response in patients with severe COVID-19. Nat Med (2020) 26(7):1070–6. doi: 10.1038/s41591-020-0944-y
257. Lucas C, Wong P, Klein J, Castro TBR, Silva J, Sundaram M, et al. Longitudinal analyses reveal immunological misfiring in severe COVID-19. Nature. (2020) 584(7821):463–9. doi: 10.1038/s41586-020-2588-y
258. Lee JS, Park S, Jeong HW, Ahn JY, Choi SJ, Lee H, et al. Immunophenotyping of COVID-19 and influenza highlights the role of type I interferons in development of severe COVID-19. Sci Immunol (2020) 5(49):eabd1554. doi: 10.1126/sciimmunol.abd1554
259. Vanderheiden A, Ralfs P, Chirkova T, Upadhyay AA, Zimmerman MG, Bedoya S, et al. Type I and type III interferons restrict SARS-CoV-2 infection of human airway epithelial cultures. J Virol (2020) 94(19):e00985-20. doi: 10.1128/JVI.00985-20
260. Mantlo E, Bukreyeva N, Maruyama J, Paessler S, Huang C. Antiviral activities of type I interferons to SARS-CoV-2 infection. Antiviral Res (2020) 179:104811. doi: 10.1016/j.antiviral.2020.104811
261. Cheemarla NR, Watkins TA, Mihaylova VT, Wang B, Zhao D, Wang G, et al. Dynamic innate immune response determines susceptibility to SARS-CoV-2 infection and early replication kinetics. J Exp Med (2021) 218(8):e20210583. doi: 10.1084/jem.20210583
262. Martin-Sancho L, Lewinski MK, Pache L, Stoneham CA, Yin X, Becker ME, et al. Functional landscape of SARS-CoV-2 cellular restriction. Mol Cell (2021) 81(12):2656–68. doi: 10.1016/j.molcel.2021.04.008
263. Low ZY, Wen Yip AJ, Chow VTK, Lal SK. The suppressor of cytokine signalling family of proteins and their potential impact on COVID-19 disease progression. Rev Med Virol (2022) 32(3):e2300. doi: 10.1002/rmv.2300
264. Johnson HM, Lewin AS, Ahmed CM. SOCS, Factors IV, Treatment of COVID-19, Front ImmunAhmed CM, et al. SOCS1 mimetics and antagonists: A complementary approach to positive and negative regulation of immune function. Front Immunol (2015) 6:183. doi: 10.3389/fimmu.2015.00183
266. Gold IM, Reis N, Glaser F, Glickman MH. Coronaviral PLpro proteases and the immunomodulatory roles of conjugated versus free interferon stimulated gene product-15 (ISG15). Semin Cell Dev Biol (2022) S1084-9521(22):00215–4. doi: 10.1016/j.semcdb.2022.06.005
267. Kirby D, Parmar B, Fathi S, Marwah S, Nayak CR, Cherepanov V, et al. Determinants of ligand specificity and functional plasticity in type I interferon signaling. Front Immunol (2021) 12:748423. doi: 10.3389/fimmu.2021.748423
268. Wilmes S, Beutel O, Li Z, Francois-Newton V, Richter CP, Janning D, et al. Receptor dimerization dynamics as a regulatory valve for plasticity of type I interferon signaling. J Cell Biol (2015) 209(4):579–93. doi: 10.1083/jcb.201412049
269. Kerr GS, Sabahi I, Richards JS, Caplan L, Cannon GW, Reimold A, et al. Prevalence of vitamin d insufficiency/deficiency in rheumatoid arthritis and associations with disease severity and activity. J Rheumatol (2011) 38(1):53–9. doi: 10.3899/jrheum.100516
270. Despotović M, Jevtović Stoimenov T, Stojanović S, Bašić J, Kundalić J, Đorđević B, et al. Association of vitamin d receptor genetic variants with bone mineral density and inflammatory markers in rheumatoid arthritis. Clin Biochem (2021) 87:26–31. doi: 10.1016/j.clinbiochem.2020.10.006
271. Murdaca G, Tonacci A, Negrini S, Greco M, Borro M, Puppo F, et al. Emerging role of vitamin d in autoimmune diseases: An update on evidence and therapeutic implications. Autoimmun Rev (2019) 18(9):102350. doi: 10.1016/j.autrev.2019.102350
272. Lin J, Liu J, Davies ML, Chen W. Serum vitamin d level and rheumatoid arthritis disease activity: Review and meta-analysis. PloS One (2016) 11(1):e0146351. doi: 10.1371/journal.pone.0146351
273. Mouterde G, Gamon E, Rincheval N, Lukas C, Seror R, Berenbaum F, et al. Association between vitamin d deficiency and disease activity, disability, and radiographic progression in early rheumatoid arthritis: The ESPOIR cohort. J Rheumatol (2020) 47(11):1624–8. doi: 10.3899/jrheum.190795
274. Small AG, Harvey S, Kaur J, Putty T, Quach A, Munawara U, et al. Vitamin d upregulates the macrophage complement receptor immunoglobulin in innate immunity to microbial pathogens. Commun Biol (2021) 4(1):401. doi: 10.1038/s42003-021-01943-3
275. Liu G, Fu Y, Yosri M, Chen Y, Sun P, Xu J, et al. CRIg plays an essential role in intravascular clearance of bloodborne parasites by interacting with complement. Proc Natl Acad Sci USA (2019) 116:24214–20. doi: 10.1073/pnas.1913443116
276. Thomason J, Rentsch C, Stenehjem EA, Hidron AI, Rimland D. Association between vitamin d deficiency and methicillin-resistant staphylococcus aureus infection. Infection. (2015) 43(6):715–22. doi: 10.1007/s15010-015-0815-5
277. Alamanda VK, Springer BD. The prevention of infection: 12 modifiable risk factors. Bone Joint J (2019) 101-B(1_Supple_A):3–9. doi: 10.1302/0301-620X.101B1.BJJ-2018-0233.R1
278. Hegde V, Dworsky EM, Stavrakis AI, Loftin AH, Zoller SD, Park HY, et al. Single-dose, preoperative vitamin-d supplementation decreases infection in a mouse model of periprosthetic joint infection. J Bone Joint Surg Am (2017) 99(20):1737–44. doi: 10.2106/JBJS.16.01598
279. Merzon E, Tworowski D, Gorohovski A, Vinker S, Golan Cohen A, Green I, et al. Low plasma 25(OH) vitamin d level is associated with increased risk of COVID-19 infection: an Israeli population-based study. FEBS J (2020) 287(17):3693–702. doi: 10.1111/febs.15495
280. Mercola J, Grant WB, Wagner CL. Evidence regarding vitamin d and risk of COVID-19 and its severity. Nutrients. (2020) 12(11):3361. doi: 10.3390/nu12113361
281. Grant WB, Lahore H, McDonnell SL, Baggerly CA, French CB, Aliano JL, et al. Evidence that vitamin d supplementation could reduce risk of influenza and covid-19 infections and deaths. Nutrients (2020) 12(4):988. doi: 10.3390/nu12040988
282. Rhodes JM, Subramanian S, Laird E, Griffin G, Kenny RA. Perspective: Vitamin d deficiency and COVID-19 severity - plausibly linked by latitude, ethnicity, impacts on cytokines, ACE2 and thrombosis. J Intern Med (2021) 289(1):97–115. doi: 10.1111/joim.13149
283. Radujkovic A, Hippchen T, Tiwari-Heckler S, Dreher S, Boxberger M, Merle U. Vitamin d deficiency and outcome of COVID-19 patients. Nutrients. (2020) 12(9):2757. doi: 10.3390/nu12092757
284. Infante M, Buoso A, Pieri M, Lupisella S, Nuccetelli M, Bernardini S, et al. Low vitamin d status at admission as a risk factor for poor survival in hospitalized patients with COVID-19: An Italian retrospective study. J Am Nutr Assoc (2022) 41(3):250–65. doi: 10.1080/07315724.2021.1877580
285. Pereira M, Dantas Damascena A, Galvão Azevedo LM, de Almeida Oliveira T, da Mota Santana J. Vitamin d deficiency aggravates COVID-19: systematic review and meta-analysis. Crit Rev Food Sci Nutr (2022) 62(5):1308–16. doi: 10.1080/10408398.2020.1841090
286. Panfili FM, Roversi M, D’Argenio P, Rossi P, Cappa M, Fintini D. Possible role of vitamin d in covid-19 infection in pediatric population. J Endocrinol Invest. (2021) 44(1):27–35. doi: 10.1007/s40618-020-01327-0
287. Gilani SJ, Bin-Jumah MN, Nadeem MS, Kazmi I. Vitamin d attenuates COVID-19 complications Via modulation of proinflammatory cytokines, antiviral proteins, and autophagy. . Expert Rev Anti Infect Ther (2022) 20(2):231–41. doi: 10.1080/14787210.2021.1941871
288. Kaufman HW, Niles JK, Kroll MH, Bi C, Holick MF. SARS-CoV-2 positivity rates associated with circulating 25-hydroxyvitamin d levels. PloS One (2020) 15(9):e0239252. doi: 10.1371/journal.pone.0239252
289. Ilie PC, Stefanescu S, Smith L. The role of vitamin d in the prevention of coronavirus disease 2019 infection and mortality. Aging Clin Exp Res (2020) 32(7):1195–8. doi: 10.1007/s40520-020-01570-8
290. Xu Y, Baylink DJ, Chen CS, Reeves ME, Xiao J, Lacy C, et al. The importance of vitamin d metabolism as a potential prophylactic, immunoregulatory and neuroprotective treatment for COVID-19. J Transl Med (2020) 18(1):322. doi: 10.1186/s12967-020-02488-5
291. Murai IH, Fernandes AL, Sales LP, Pinto AJ, Goessler KF, Duran CSC, et al. Effect of a single high dose of vitamin D3 on hospital length of stay in patients with moderate to severe COVID-19: A randomized clinical trial. JAMA. (2021) 325(11):1053–60. doi: 10.1001/jama.2020.26848
292. Annweiler G, Corvaisier M, Gautier J, Dubée V, Legrand E, Sacco G, et al. Vitamin d supplementation associated to better survival in hospitalized frail elderly COVID-19 patients: The GERIA-COVID quasi-experimental study. Nutrients. (2020) 12(11):3377. doi: 10.3390/nu12113377
293. Tomaszewska A, Rustecka A, Lipińska-Opałka A, Piprek RP, Kloc M, Kalicki B, et al. The role of vitamin d in COVID-19 and the impact of pandemic restrictions on vitamin d blood content. Front Pharmacol (2022) 13:836738. doi: 10.3389/fphar.2022.836738
294. Ben-Zvi I, Aranow C, Mackay M, Stanevsky A, Kamen DL, Marinescu LM, et al. The impact of vitamin d on dendritic cell function in patients with systemic lupus erythematosus. PloS One (2010) 5(2):e9193. doi: 10.1371/journal.pone.0009193
295. Suzuki T, Tatsuno K, Ito T, Sakabe JI, Funakoshi A, Tokura Y. Distinctive downmodulation of plasmacytoid dendritic cell functions by vitamin D3 analogue calcipotriol. J Dermatol Sci (2016) 84(1):71–9. doi: 10.1016/j.jdermsci.2016.06.003
296. Barragan M, Good M, Kolls JK. Regulation of dendritic cell function by vitamin d. Nutrients. (2015) 7(9):8127–51. doi: 10.3390/nu7095383
Keywords: SARS-CoV-2, COVID-19, Staphylococcus aureus, Rheumatoid arthritis, IFI44, dendritic cells, 1,25-dihydroxy vitamin D3
Citation: Zheng Q, Wang D, Lin R, Lv Q and Wang W (2022) IFI44 is an immune evasion biomarker for SARS-CoV-2 and Staphylococcus aureus infection in patients with RA. Front. Immunol. 13:1013322. doi: 10.3389/fimmu.2022.1013322
Received: 06 August 2022; Accepted: 29 August 2022;
Published: 15 September 2022.
Edited by:
Chenhe Su, Wistar Institute, United StatesReviewed by:
Yusha Araf, Shahjalal University of Science and Technology, BangladeshSharah Jabeen, Bangladesh Agricultural University, Bangladesh
Copyright © 2022 Zheng, Wang, Lin, Lv and Wang. This is an open-access article distributed under the terms of the Creative Commons Attribution License (CC BY). The use, distribution or reproduction in other forums is permitted, provided the original author(s) and the copyright owner(s) are credited and that the original publication in this journal is cited, in accordance with accepted academic practice. No use, distribution or reproduction is permitted which does not comply with these terms.
*Correspondence: Wanming Wang, d2FuZ3dtOTNAMTI2LmNvbQ==
†These authors have contributed equally to this work