- School of Pharmacy, Key Laboratory of Nano-carbon Modified Film Technology of Henan Province, Diagnostic Laboratory of Animal Diseases, Xinxiang University, Xinxiang, Henan, China
Background: The severe coronavirus disease 2019 (COVID-19) is an infectious disease caused by the severe acute respiratory syndrome coronavirus 2 (SARS-CoV-2), which has resulted in the most devastating pandemic in modern history. Human immunodeficiency virus (HIV) destroys immune system cells and weakens the body’s ability to resist daily infections and diseases. Furthermore, HIV-infected individuals had double COVID-19 mortality risk and experienced worse COVID-related outcomes. However, the existing research still lacks the understanding of the molecular mechanism underlying crosstalk between COVID-19 and HIV. The aim of our work was to illustrate blood transcriptome crosstalk between COVID-19 and HIV and to provide potential drugs that might be useful for the treatment of HIV-infected COVID-19 patients.
Methods: COVID-19 datasets (GSE171110 and GSE152418) were downloaded from Gene Expression Omnibus (GEO) database, including 54 whole-blood samples and 33 peripheral blood mononuclear cells samples, respectively. HIV dataset (GSE37250) was also obtained from GEO database, containing 537 whole-blood samples. Next, the “Deseq2” package was used to identify differentially expressed genes (DEGs) between COVID-19 datasets (GSE171110 and GSE152418) and the “limma” package was utilized to identify DEGs between HIV dataset (GSE37250). By intersecting these two DEG sets, we generated common DEGs for further analysis, containing Kyoto Encyclopedia of Genes and Genomes (KEGG) pathway and Gene Ontology (GO) functional enrichment analysis, protein-protein interaction (PPI) analysis, transcription factor (TF) candidate identification, microRNAs (miRNAs) candidate identification and drug candidate identification.
Results: In this study, a total of 3213 DEGs were identified from the merged COVID-19 dataset (GSE171110 and GSE152418), and 1718 DEGs were obtained from GSE37250 dataset. Then, we identified 394 common DEGs from the intersection of the DEGs in COVID-19 and HIV datasets. GO and KEGG enrichment analysis indicated that common DEGs were mainly gathered in chromosome-related and cell cycle-related signal pathways. Top ten hub genes (CCNA2, CCNB1, CDC20, TOP2A, AURKB, PLK1, BUB1B, KIF11, DLGAP5, RRM2) were ranked according to their scores, which were screened out using degree algorithm on the basis of common DEGs. Moreover, top ten drug candidates (LUCANTHONE, Dasatinib, etoposide, Enterolactone, troglitazone, testosterone, estradiol, calcitriol, resveratrol, tetradioxin) ranked by their P values were screened out, which maybe be beneficial for the treatment of HIV-infected COVID-19 patients.
Conclusion: In this study, we provide potential molecular targets, signaling pathways, small molecular compounds, and promising biomarkers that contribute to worse COVID-19 prognosis in patients with HIV, which might contribute to precise diagnosis and treatment for HIV-infected COVID-19 patients.
Introduction
The COVID-19 virus caused by the SARS-CoV-2 virus emerged in Wuhan, China, in December 2019, resulting in a large loss of human life all over the world and posing a serious threat to public health, food systems and the world of work (1–4). A recent World Health Organization (WHO) report conducted in China showed fever, diarrhea, sore throat, dry cough, and fatigue were listed as the most common COVID-19 symptoms, while musculoskeletal symptoms, including arthralgia and muscular aches may also occur by infecting coronavirus (5–9). It is common for patients suffering from severe COVID-19 cases to suffer from acute respiratory distress syndrome (ARDS) and respiratory failure, organ manifestations that lead to the majority of fatalities resulting from COVID-19 (10). Due to COVID’s rapidly evolving mutant strains, the difficulties of manufacturing vaccines, and the lack of vaccines, the fight against COVID-19 remains highly challenging (11).
Acquired immunodeficiency syndrome (AIDS) is defined as less than 200 CD4 T-cells per liter of blood or as a disease that defines AIDS (12, 13). Infection with HIV causes human immunodeficiency virus infection and acquired immunodeficiency syndrome (HIV/AIDS) (14). The World Health Organization estimates that as of 2021, HIV/AIDS has killed about 36.3 million people and affected approximately 37.7 million people worldwide (15).
Previous studies showed that HIV-infected individuals had double COVID-19 mortality risk and experienced worse COVID-related outcomes (16–18). According to a recent study, HIV was also an independent risk factor for both severe/critical COVID-19 at admission and in-hospital mortality (19). Therefore, it is essential to explore potential molecular mechanisms and screen out potential small molecular compounds for the treatment of HIV-infected COVID-19 patients.
In addition, white blood cells circulating around the blood help the immune system fight off infections and act as a first line defense against disease-causing microorganisms. HIV infection is characterized by CD4+ T cell depletion, CD8+ T cell expansion, and chronic immune activation that leads to immune dysfunction (20). COVID-19 can trigger a cytokine storm in pulmonary tissues through hyperactivation of the immune system and the uncontrolled release of cytokines (21). These results indicate that blood cells may play important role in the progression of COVID-19 in HIV-infected individuals. The purpose of our work was to illustrate blood transcriptome crosstalk between COVID-19 and HIV and to provide potential drugs that might be useful for the treatment of HIV-infected COVID-19 patients.
Herein, we identified 394 common DEGs from the intersection of the DEGs in COVID-19 and HIV datasets. Next, the common DEGs were analyzed using KEGG and GO functional enrichment analysis to identify potential biological pathways. Then, PPI network was developed to identify hub genes that may serve as diagnostic markers for disease. Subsequently, for the advancement of COVID-19 and HIV clinical diagnosis and treatment, we analyzed TFs, miRNAs on the basis of the common DEGs. Finally, we explored possible small molecule compounds that may be profitable for treating HIV-infected COVID-19 patients.
Methods
Data acquisition
One COVID-19 dataset (GEO accession ID: GSE171110) consisted of 44 COVID-19-infected whole-blood samples and 10 healthy whole-blood samples with Illumina HiSeq 2000 (22). Another COVID-19 dataset (GEO accession ID: GSE152418) contained 16 peripheral blood mononuclear cells samples and 17 healthy samples (23). Similarly, the HIV dataset (GEO accession ID: GSE37250) consisted of 274 positive HIV-infected whole-blood samples, 263 negative HIV-infected whole-blood samples.
Identification of common DEGs
The “Deseq2” package in RStudio software (version 4.1.2) was performed to select DEGs with |Log2 Fold Change| > 0.585 and | adj.P.Val. | < 0.05 for COVID-19 datasets (GSE171110 and GSE152418). Meanwhile, the “limma” package was used to identify DEGs with |Log2 Fold Change| ≥ 0.1 and | adj.P.Val. | <0.001 for HIV dataset (GSE37250). Using “venn” package in R software, we obtained COVID-19 and HIV common DEGs.
Functional enrichment analysis of common DEGs
The “clusterProfiler” package in RStudio software (version 4.1.2) was performed to explore the possible biological pathways of the common DEGs with GO and KEGG enrichment analysis. P-value < 0.05 was used for quantifying the top listed functional items and pathways of common DEGs.
Analysis of PPI network based on common DEGs
The interactions among proteins are represented by PPI networks, which are crucial to understanding cell physiology in health and disease. It is essential to understand and gain insight into the cellular machine process by examining PPI network function and its interaction with cellular machinery (24). A set of common DEGs was also uploaded to the STRING website (https://string-db.org/) so that the interactions among proteins could be assessed critically. The PPI network of these common DEGs was constructed based on a combined score larger than 0.9. Then, the PPI networks were visualized using Cytoscape (version 3.9.1) software.
Extraction of hub genes
Using Cytoscape’s plug-in CytoHubba, we identified the top 10 hub genes by ranking them (25). Using network metrics, Cytoscape allows users to evaluate and identify biological network modulators (26). Furthermore, receiver operating characteristic curve (ROC) analyses based on degree algorithms were conducted on the top 10 hub genes.
Recognition of transcription factors and MiRNAs
Proteins called TFs can bind to specific DNA sequences and form a complex regulatory system that controls genome expression (27). Enrichr is a web-based enrichment analysis tool that provides different types of visual summary summaries for gene lists (28). We used the Enrichr Transcription Factor PPIs library to identify TFs in common DEGs and developed a TF-gene interaction map using Cytoscape software (version 3.9.1). In addition, target gene-miRNA interaction analysis was performed to detect miRNAs that could successfully attach to target gene transcripts and negatively have an influence on the expression of protein through destabilizing mature messenger RNA and reducing corresponding translation efficiency (29). MiRTarBase provides information about miRNA-target interactions (MTIs) that have been experimentally validated (30). Then, we used the Enrichr miRTarBase 2017 library to identify miRNAs in common DEGs and developed a miRNA-gene interaction map using Cytoscape software (version 3.9.1).
Evaluation of applicant drugs
An online resource, Drug Signatures Database (DSigDB), connects drugs/compounds to their target genes (31). To study the drug molecular properties of COVID-19 and HIV, we used the Drug Signatures Database (DSigDB) library under the Diseases/Drugs function in Enrichr (https://maayanlab.cloud/Enrichr/enrich).
Gene-disease association analysis
The DisGeNET database contains one of the most comprehensive collections of genes and variants associated with human disease (32–35). Based on common DEGs, we identified diseases and chronic health problems using DisGeNET database under the Diseases/Drugs function in Enrichr.
Results
Identification of DEGs and common DEGs between COVID-19 and HIV
Datasets
A flowchart was created to depict all the critical and significant processes of our study (Figure 1). In order to investigate the interactions between COVID-19 and HIV, we analyzed the blood samples downloaded from GEO database. Box plots of the gene expression of COVID-19 and HIV datasets before and after normalization were shown in Supplementary Figures 4-6. In the COVID-19 dataset, we identified 3213 DEGs with|Log2 Fold Change| > 0.585 and | adj.P.Val. | < 0.05, whereas in the HIV dataset, we identified 1718 DEGs with |Log2 Fold Change| ≥ 0.1 and | adj.P.Val. | <0.001 (Figure 2A; Supplementary Tables 1, 2). Moreover, the volcano plots depicted DEGs of COVID-19 and HIV, respectively (Figures 2B, C; Supplementary Tables 3, 4). Venn diagram revealed that 394 DEGs were common in both COVID-19 and HIV datasets (Figure 2D). According to these findings, HIV and COVID-19 have a large number of common genes and are closely related.
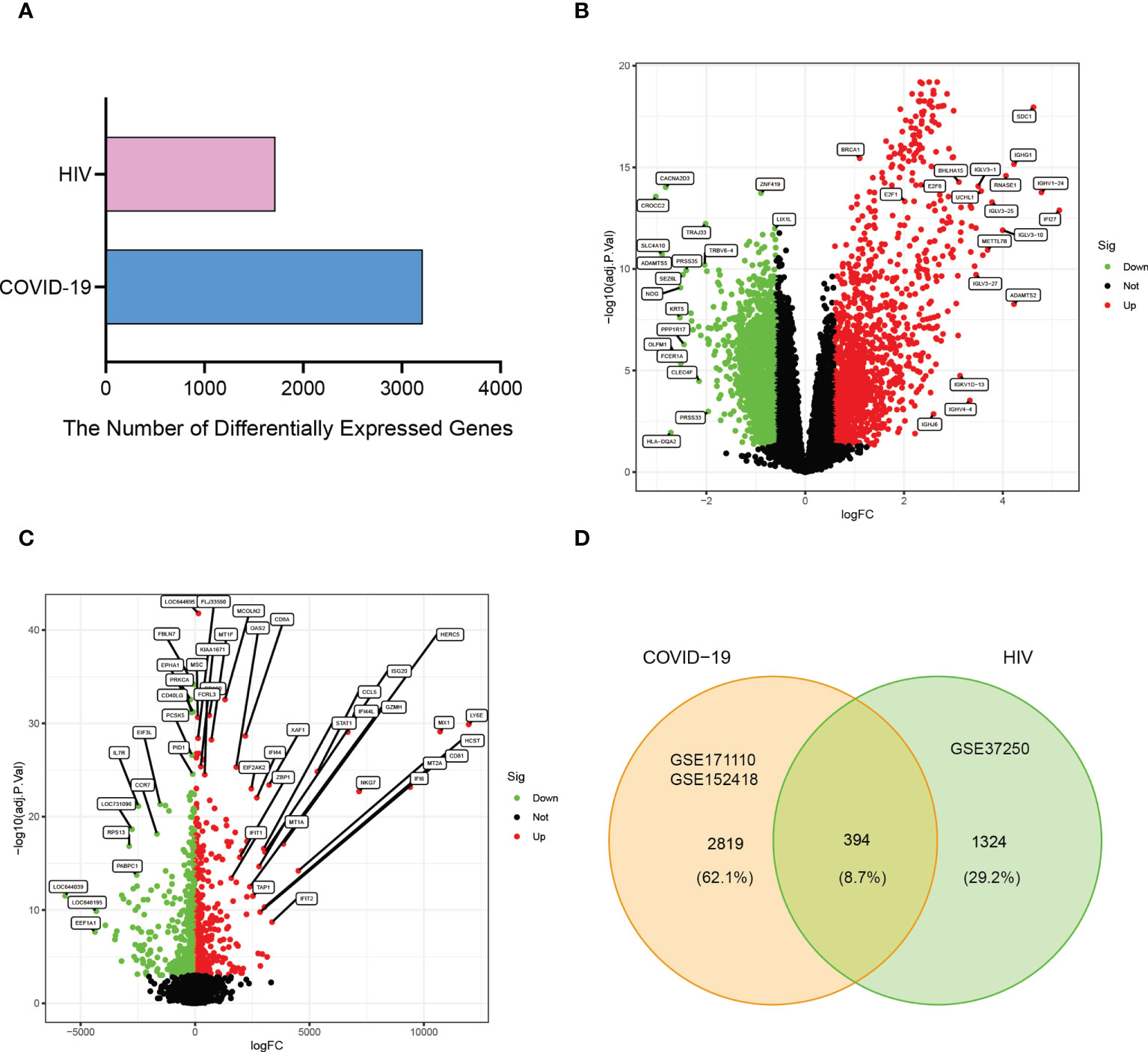
Figure 2 Visualization the number of common differentially expressed genes between two datasets, COVID-19 (GSE171110 and GSE152418) and HIV (GSE37250). (A) Comparing the number of differentially expressed genes between COVID-19 and HIV. (B) The volcano plot of differentially expressed genes in COVID-19 datasets. (C) The volcano plot of differentially expressed genes in the HIV dataset. (D) Venn diagram showing the overlap of differentially expressed genes between COVID-19 and HIV.
Functional enrichment analysis of common DEGs
The GO enrichment analyses are commonly used to show the interactions between genes and terms, whereas KEGG enrichment analyses can illustrate the relationship between genes and patterns of function (36). “ClusterProfiler” package was used to discover biological features and pathways that were enriched in this work as common DEGs. GO enrichment analysis showed significantly enriched pathways (p-value < 0.05), biological process (BP), cell composition (CC), and molecular function (MF) are included. Notable pathways among BP category were mitotic nuclear division, mitotic sister chromatid segregation, and sister chromatid segregation. In the CC category, the top three terms were cytosolic ribosome, chromosome, centromeric region and chromosomal region. Moreover, in the MF aspect, structural constituent of ribosome, coreceptor activity, and C−C chemokine receptor activity were the top three statistically significant GO terms (Figures 3A, B). The top three pathways of KEGG enrichment analysis were Coronavirus disease-COVID-19, Cytokine−cytokine receptor interaction, and Cell cycle (Figures 3C, D). These results suggest that these common DEGs have strong relationships with cell cycle, which may lead to a more effective treatment for COVID-19 and HIV.
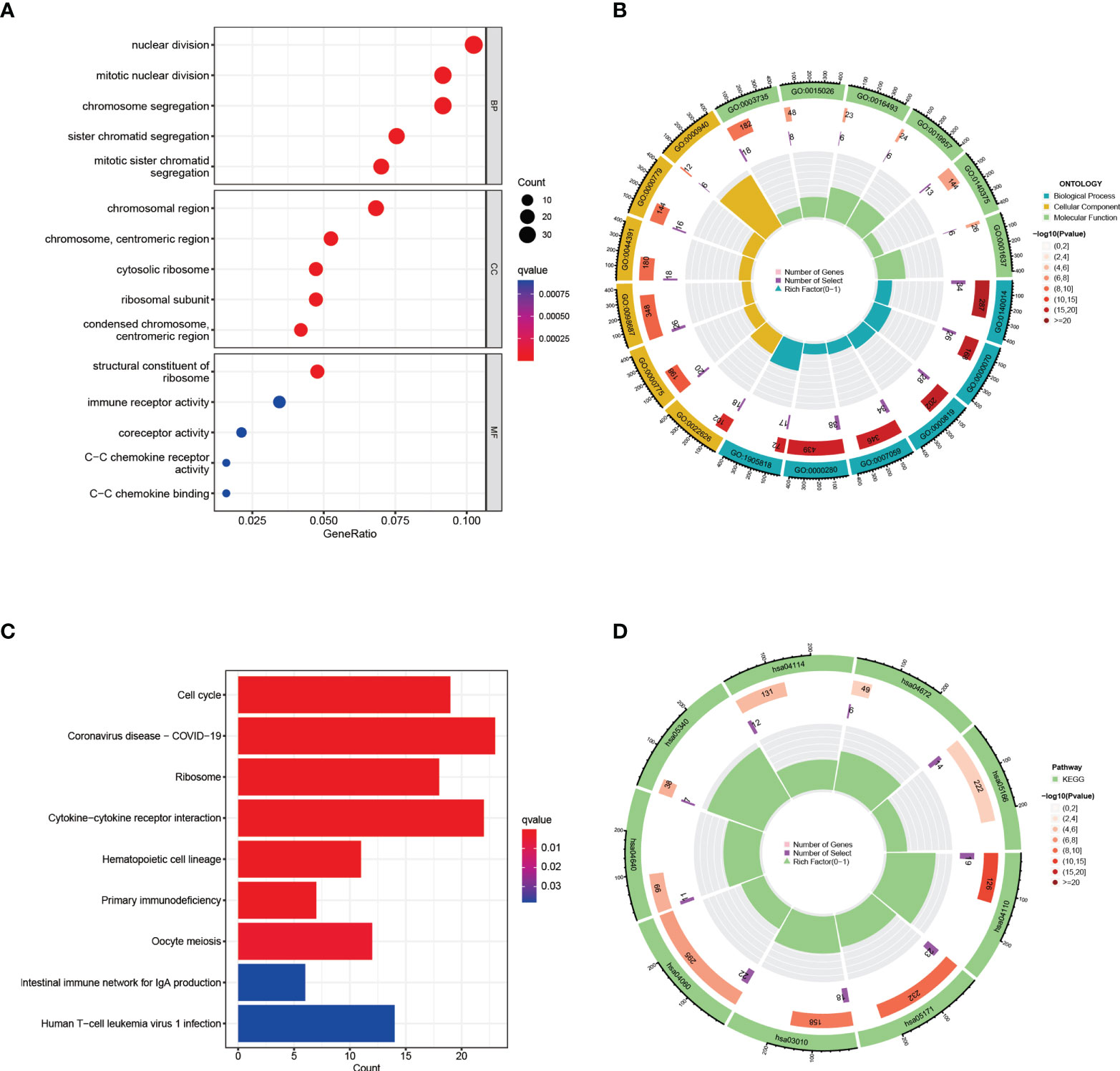
Figure 3 GO and KEGG functional enrichment analysis of the common differentially expressed genes between COVID-19 and HIV. (A) The bubble graphs of GO enrichment analysis. (B) Circle diagram of GO enrichment analysis. (C) The bar graphs of KEGG enrichment analysis. (D) Circle diagram of KEGG enrichment analysis.
PPI network and hub genes extraction of common DEGs
A comprehensive analysis of these common DEGs between COVID-19 and HIV was conducted using STRING to explore protein-protein interactions. Figure 4 illustrates the interactions between the common DEGs in HIV and COVID-19. As shown in Figure 5, top 10 hub genes were ranked according to their scores, which were identified using degree method based on the results of PPI network in Cytoscape, including CCNA2, CCNB1, CDC20, TOP2A, AURKB, PLK1, BUB1B, KIF11, DLGAP5, RRM2. Next, ROC analysis was conducted for HIV and COVID-19, respectively. Area under curve (AUC) values for all hub genes in the HIV dataset were greater than 0.616, whereas AUC values for all hub genes in the COVID-19 dataset were greater than 0.973 (Supplementary Figures 2, 3). According to these results, it is potential to develop novel targeted therapies against COVID-19 by targeting these hub genes.
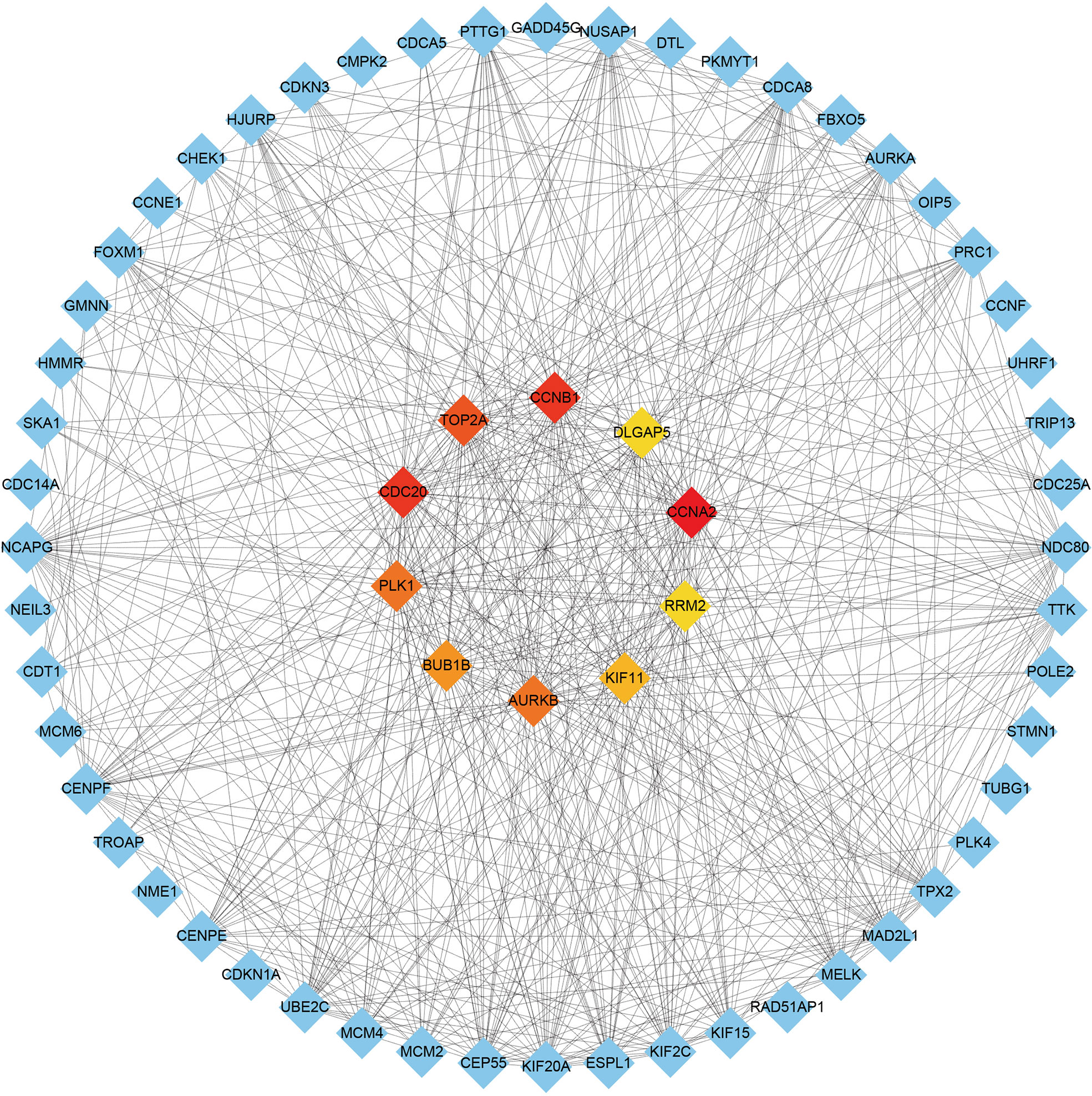
Figure 5 Identification of the top 10 hub genes ranked by their scores. In the middle circle, the 10 central genes represent the top 10 hub genes ranked by their scores and their interactions with other common DEGs.
Construction of regulatory networks
There are two types of gene expression regulators: transcription factors (TFs) and miRNAs. TFs modulate transcription by binding the promoter regions, whereas miRNAs regulate post-transcriptional gene expression (37). Analysis of interactions between TFs and miRNAs revealed that 165 TFs and 2466 miRNAs coordinate these common DEGs, indicating that they cooperate heavily. As shown in Figure 6, the top ten TFs were ranked according to their P values, including ILF3, RAD21, ILF2, TP53, CCNE1, E2F4, E2F1, HDAC8, ESR1, and HSF1. The top ten miRNAs were also ranked by their P values, containing hsa-miR-193b-3p, hsa-miR-192-5p, hsa-miR-215-5p, hsa-miR-146a-5p, hsa-miR-10a-5p, hsa-miR-216b-5p, hsa-miR-212-3p, hsa-miR-34a-5p, hsa-miR-1260b, and hsa-miR-23b-5p (Figure 7). These findings indicated that there was strong relationship between common DEGs and TFs, miRNAs.
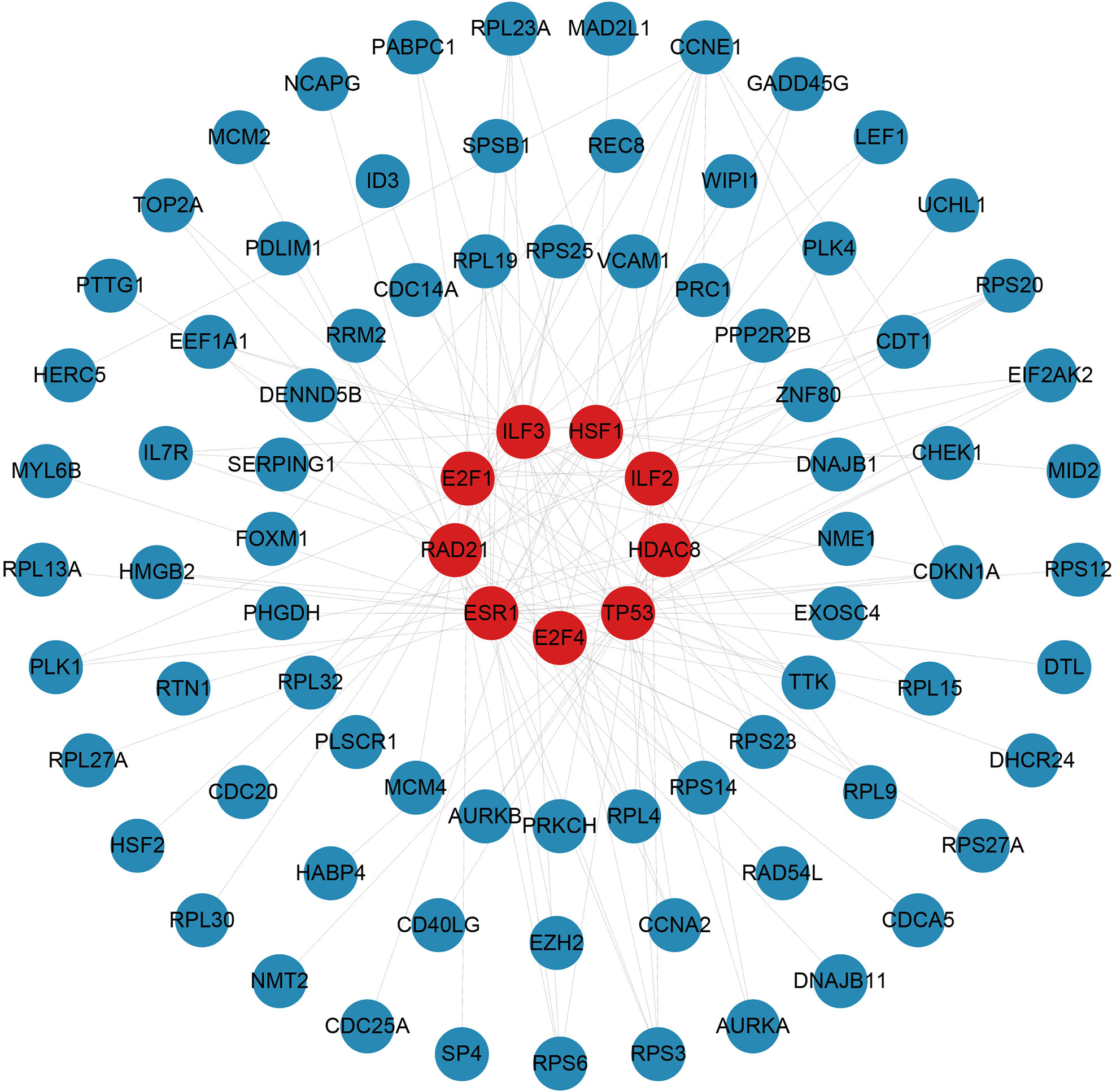
Figure 6 The top 10 transcription factors ranked according to their P values and their interactions with common differentially expressed genes. In this network, the red circles represent transcription factors with the top 10 lowest P values in the network, while the blue circles represent common differentially expressed genes correlated with transcription factors.
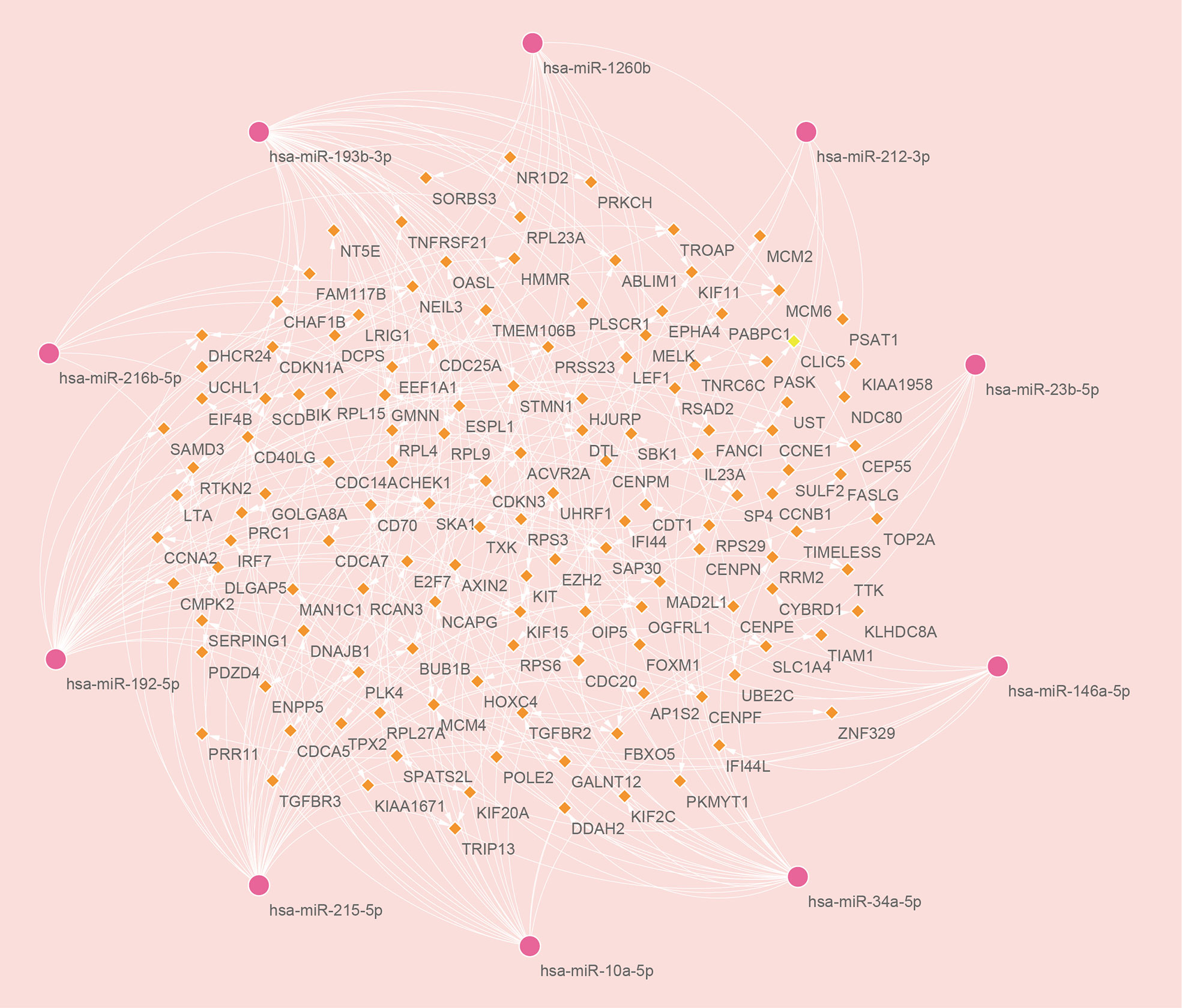
Figure 7 The top ten microRNAs with the top 10 lowest P values and their interactions with common differentially expressed genes. In this network, the pink circles represent miRNAs with the top 10 lowest P values, while the orange diamonds represent common differentially expressed genes correlated with miRNAs.
Identification of candidate drugs
As shown in Figure 8, top ten drugs were ranked according to their P values (LUCANTHONE, Dasatinib, etoposide, Enterolactone, troglitazone, testosterone, estradiol, calcitriol, resveratrol, tetradioxin), which were identified from the DSigDB library in Enrichr based on P-value. These potential small molecular compounds might serve as therapeutic targets and treatment for COVID-19 and HIV.
Identification of disease association
It has been shown that various diseases are linked and must have at least one or more genes in common (38). Based on DisGeNET library in Enrichr, we screened out five diseases that has strong relationship with common DEGs, including malignant neoplasm of breast, breast carcinoma, malignant lymphoma, lymphocytic, intermediate differentiation, diffuse, nasopharyngeal carcinoma, and leukemia (Supplementary Figure 1). These results indicated these diseases have something in common with HIV and COVID-19.
Discussion
A growing number of studies have demonstrated possible connections between various diseases in recent years. Interactions between different diseases are therefore a highly promising field that needs to be investigated in the future (23, 39, 40). There have been dramatic deaths worldwide due to COVID-19, which has presented a major challenge to public health, the food system, and the workforce. HIV is a member of the family Retrovirae within the genus Lentivirus (41), which has a wide-ranging impact on individuals’ health, households, communities, and nations’ economic and social well-being (42). People living with HIV have badly compromised immune system. The recent studies showed that patients living with HIV had a higher risk of SARS-CoV-2 infection and mortality risk from COVID-19 than people without HIV (19, 43–45). HIV was identified as an independent risk factor for both severe-type and critical-type COVID-19 (46). However, the molecular mechanisms that contribute to worse COVID-19 prognosis in patients with HIV are still unclear. In this study, we downloaded COVID-19 datasets (GSE171110 and GSE152418) and HIV dataset (GSE37250) from GEO database. Next, we performed differential analysis on COVID-19 and HIV datasets, respectively. Then, by intersecting these two DEG sets, we generated common DEGs for further analysis, and identified 10 hub genes ranked according to their scores (CCNA2, CCNB1, CDC20, TOP2A, AURKB, PLK1, BUB1B, KIF11, DLGAP5, and RRM2) using degree method. Hub proteins could be used for the development of therapeutic intervention. Cyclin A2 (CCNA2) regulates the cell cycle and contributes to tumor growth (47). Cyclin B1 (CCNB1) is a crucial mitosis initiator and controller (48). CDC20 is a well-known regulator of cell division, regulating chromosome segregation during mitosis (49). The mitotic chromosome condensation is established by topoisomerase IIα (TOP2A), a core component of mitotic chromosomes (50). Aurora kinase B (AURKB) has been identified in Caco-2 cells as a DEG of SARS-CoV-2 (51). The Polo-like Kinase 1 (PLK1) is an essential enzyme in mitosis, which initiates, maintains, and completes it (52). Moreover, knockdown of BUB1B caused acute chromosomal abnormalities as well as impaired chromosome alignment (53). A kinesin called KIF11 is responsible for intracellular vesicle transport and mitosis, as well as being overexpressed in tumors (54). DLGAP5, a gene mapped to chromosome 14q22.3, plays an important role in cancer formation (55). During DNA replication, RRM2 is essential for synthesis of Deoxynucleoside triphosphate (dNTP) in S phase of cell cycle (56).
As previous studies indicated that lower CD4 count in people living with HIV strongly correlated with increased odds of SARS-CoV-2 positivity (46). These data highlight the urgent need for mechanism studies to better illustrate how HIV-associated immunocompromise influences infection acquisition and clearance. In this study, we conducted GO and KEGG analyses to examine the association between COVID-19 and HIV. GO analysis was performed using the “clusterProfiler” package for three types of BP, CC, and MF. The BPs of these common DEGs were primarily enriched in mitotic nuclear division, mitotic sister chromatid segregation, and sister chromatid segregation. The process of chromosome segregation is crucial to the cell cycle (57). SARS-CoV-2 infection may modulate several proteins involved in inflammatory responses and chromosomal segregation (51). The CCs of these common DEGs were mainly enriched in cytosolic ribosome, chromosome, centromeric region and chromosomal region. The MF results indicated that these common DEGs were primarily enriched in structural constituent of ribosome, coreceptor activity, and C−C chemokine receptor activity. Cancer, developmental disorders, and virus infections all affect ribosome production, which is universally important in biology (58). Virus relies solely on polypeptide synthesis by ribosomes to produce polypeptide molecules (59, 60). Coronavirus disease-COVID-19, Cytokine−cytokine receptor interaction, and Cell cycle were the top 3 KEGG pathways. In a cell cycle, a specific sequence of events takes place such as cell division, DNA replication, nuclear membrane rupture, spindle formation, and preparation for chromosome segregation (61). A large number of studies have shown that viral replication and survival are facilitated by viruses regulating host cell cycle processes (62–65). Identifying significant gene ontology and molecular pathways improved our understanding of the mechanism for how HIV infection is a risk factor for increased mortality from COVID-19.
We also explored the relationships between TFs, miRNAs and common DEGs. MiRNAs, which are small non-coding RNAs with 20-24 nucleotides, are known to suppress the expression of target genes via binding to complementary target sites in the 3’ untranslated region of mRNA post-transcriptionally (37). In many biological processes, miRNAs regulate target genes, and some of these genes may promote cancer or suppress tumor growth (66). A TF is a protein that binds specific DNA sequences to regulate transcription and gene expression (67). In many biological processes, TFs play a key role by binding specific sequences of genes, for example, regulating gene transcription, controlling metabolism, and influencing immunity (68). The top 10 TFs ranked by their P values were ILF3, RAD21, ILF2, TP53, CCNE1, E2F4, E2F1, HDAC8, ESR1, and HSF1. The top ten miRNAs ranked according to their P values were hsa-miR-193b-3p, hsa-miR-192-5p, hsa-miR-215-5p, hsa-miR-146a-5p, hsa-miR-10a-5p, hsa-miR-216b-5p, hsa-miR-212-3p, hsa-miR-34a-5p, hsa-miR-1260b, and hsa-miR-23b-5p. HIV infection is promoted by ILF3 and ILF2 via direct interaction with the vRNA (69). The ILF3 suppresses HIV-1 innate sensing as well as other PAMPs that stimulate TLR7/8 and cGAS (70). Inhibition of HDAC does not enhance HIV spread ability, since it does not increase HIV infection susceptibility in peripheral blood mononuclear cells (71). TP53 regulates viral replication and induces G2 cell cycle arrest by interacting with HIV-1 viral infectivity factor (72). The estrogen receptor-1 (ESR-1) regulates HIV-1 latency based on the results of unbias shRNA library screening (73). HSF1 plays an important role in HIV transcription and HIV latent reactivation (74). Among all the miRNAs, SARS-COV infection has been linked to hsa-miR-193b-3p (75) in studies, the effect of other miRNAs for COVID-19 and HIV need to be further explored.
Moreover, we conducted gene-disease analyses to identify common DEGs associated with diseases. According to the results, common DEGs are associated with various types of diseases in HIV and COVID-19, including malignant neoplasm of breast, breast carcinoma, malignant lymphoma, lymphocytic, intermediate differentiation, diffuse, nasopharyngeal carcinoma, and leukemia. It has been recognized for more than 100 years that aneuploidy is a hallmark of tumorigenesis (76). Human cancer is characterized by chromosomal instability, which is associated with poor prognosis, metastasis, and therapeutic resistance (77). Studies showed COVID-19 was highly related to a variety of neoplasms, including mammary, colonic, stomach, and prostatic neoplasms (25). This was consistent with our above results. As a result of the severe nature of cancer and compromised immunity, COVID-19 patients were at a higher risk of dying (78).
Although molnupiravir, paxlovid, and remdesivir are approved by FDA for the treatment of COVID-19, at this time there is no evidence to suggest that any particular antiretroviral therapy agent improves or worsens COVID-19 clinical outcomes in PLHIV, or can be used for prevention of SARS-CoV-2 infection (79). Developing a safe and effective drug for the treatment of HIV-infected COVID-19 patients still has a high priority. In this study, we identified a variety of chemical compounds and drugs that could potentially treat COVID-19 and HIV, including LUCANTHONE, Dasatinib, etoposide, Enterolactone, troglitazone, testosterone, estradiol, calcitriol, resveratrol, tetradioxin. A TOP II inhibitor, Etoposide, prevents intracellular replication of the structural proteins of SARS-CoV-2 while rescuing COVID-19’s cytokine storm (80, 81). There was an early, nondurable clinical benefit associated with immediate etoposide treatment in adults with Kaposi sarcoma and HIV infection (82). The kinase signaling pathway inhibitor Dasatinib is effective against SARS-CoV (83), HIV replication could also be suppressed by Dasatinib by blocking reverse transcription and integration (84, 85). It has been found that troglitazone inhibits SARS-CoV-2 NSP9, which is crucial for viral replication (86). According to increasing evidence, Estradiol participates in the regulation of HIV infection (87), and shows a significant improvement regarding fatality in COVID-19 (88). A recent study found that resveratrol significantly reduced HIV-1 infection in CD4 T cells by cutting off the production of reverse transcripts (89). The use of calcitriol as a treatment for COVID-19 has recently been proposed (90). It has been shown that testosterone reduces COVID-19 levels by inhibiting proinflammatory cytokines, increasing antiinflammatory cytokines, modulating immune system and attenuating oxidative stress and endothelial dysfunction via its action on inflammatory cytokines (91). A deficiency of testosterone might contribute to HIV-associated lipodystrophy, or be involved in its pathogenesis (92).
Actually, blood cells are a diverse group of immune cells that act as a first line defense against infections and disease-causing microorganisms. Commonly, People living with HIV who have a CD4+ cell count below 200 are at high risk of developing serious illnesses (93). Consistently, a significant decrease in CD3+ T cells, CD4+ T cells, CD8+ T cells and natural killer cells in PBMCs indicates the severity of COVID-19 patients compared to moderate patients (94). To the best of our knowledge, the molecular blood biomarkers for HIV-infected COVID-19 patients are not reported yet. In this study, the top ten hub genes ranked according to their scores (CCNA2, CCNB1, CDC20, TOP2A, AURKB, PLK1, BUB1B, KIF11, DLGAP5, and RRM2) were analyzed by ROC analysis. All these hub genes in the cohort HIV have an AUC value above 0.616 as a result of our analysis, while in the cohort COVID-19 these hub genes had AUC values above 0.973. The discovery of these molecular blood biomarkers may provide new insights into HIV-infected COVID-19 patients’ diagnosis, care, and treatment.
In summary, our study had many advantages. Firstly, we utilized blood samples of COVID-19 and HIV from GEO database to identify hub genes, which may play crucial roles in the occurrence and development of COVID-19 and HIV. Secondly, we clarified the interactions between CVOID-19 and HIV, which could provide novel insights into the molecular mechanism underlying viral infection with COVID-19 and HIV. Thirdly, we screened out ten candidate drugs ranked by their P values, which might serve as biomarkers for the treatment of COVID-19 and HIV patients.
However, despite the strength of our study, there are several limitations. Firstly, we just derived the research data from the GEO public database. Secondly, the biological functions of hub genes as well as the effectiveness and safety of candidate drugs should be confirmed through basic experiments or clinical trials. Thirdly, the molecular mechanism of COVID-19 and HIV are required to be further investigate.
Conclusion
In this study, we provide potential molecular targets, signaling pathways, small molecular compounds, and promising biomarkers that contribute to worse COVID-19 prognosis in patients with HIV, which might contribute to precise diagnosis and treatment for HIV-infected COVID-19 patients.
Data availability statement
The original contributions presented in the study are included in the article/Supplementary Material. Further inquiries can be directed to the corresponding authors.
Author contributions
CY and XW contributed to the conception of the study and data analysis. YN performed the data analyses and wrote the manuscript. All authors contributed to the article and approved the submitted version.
Funding
This work was supported by the Natural Science Foundation for Young Scientists of Henan Province, China (Grant No. 222300420261).
Acknowledgments
The authors thank participants and staff of Xinxiang University for their contributions.
Conflict of interest
The authors declare that the research was conducted in the absence of any commercial or financial relationships that could be construed as a potential conflict of interest.
Publisher’s note
All claims expressed in this article are solely those of the authors and do not necessarily represent those of their affiliated organizations, or those of the publisher, the editors and the reviewers. Any product that may be evaluated in this article, or claim that may be made by its manufacturer, is not guaranteed or endorsed by the publisher.
Supplementary material
The Supplementary Material for this article can be found online at: https://www.frontiersin.org/articles/10.3389/fimmu.2022.1008653/full#supplementary-material
Supplementary Figure 1 | Regulatory interactions between common DEGs and diseases.
Supplementary Figure 2 | ROC analysis of the hub DEGs in the HIV dataset.
Supplementary Figure 3 | ROC analysis of the hub DEGs in the COVID-19 dataset.
Supplementary Figure 4 | Box plots of the gene expression of GSE37250 dataset before (A) and after (B) normalization.
Supplementary Figure 5 | Box plots of the gene expression of GSE171110 dataset before (A) and after (B) normalization.
Supplementary Figure 6 | Box plots of the gene expression of GSE152418 dataset before (A) and after (B) normalization.
Supplementary Table 1 | The expression of COVID-19 differentially expressed genes.
Supplementary Table 2 | The expression of HIV differentially expressed genes.
Supplementary Table 3 | Volcano plots of COVID-19 differentially expressed genes.
Supplementary Table 4 | Volcano plots of HIV differentially expressed genes.
Supplementary Table 5 | Common DEGs between COVID-19 and HIV.
Supplementary Table 6 | The TFs and their interactions with DEGs.
Supplementary Table 7 | The microRNAs and their interactions with DEGs.
Supplementary Table 8 | The potential drugs for the treatment of COVID-19 and HIV.
Supplementary Table 9 | Regulatory interactions between common DEGs and diseases.
Abbreviations
COVID-19, severe coronavirus disease 2019; SARS-CoV-2, severe acute respiratory syndrome coronavirus 2; HIV, human immunodeficiency virus; GEO, Gene Expression Omnibus; DEG, differentially expressed gene; PPI, protein-protein interaction; TF, transcription factor; miRNAs, microRNAs; ARDS, acute respiratory distress syndrome; AIDS, Acquired immunodeficiency syndrome; GO, Gene Ontology; KEGG, Kyoto Encyclopedia of Genes and Genomes; BP, biological process; CC, cell composition; MF, molecular function; DSigDB, Drug Signatures Database; AUC, area under curve.
References
1. Cooper TJ, Woodward BL, Alom S, Harky A. Coronavirus disease 2019 (COVID-19) outcomes in HIV/AIDS patients: A systematic review. HIV Med (2020) 21(9):567–77. doi: 10.1111/hiv.12911
2. Finkel Y, Mizrahi O, Nachshon A, Weingarten-Gabbay S, Morgenstern D, Yahalom-Ronen Y, et al. The coding capacity of SARS-CoV-2. Nature (2021) 589(7840):125–30. doi: 10.1038/s41586-020-2739-1
3. Lu R, Zhao X, Li J, Niu P, Yang B, Wu H, et al. Genomic characterisation and epidemiology of 2019 novel coronavirus: implications for virus origins and receptor binding. Lancet (2020) 395(10224):565–74. doi: 10.1016/S0140-6736(20)30251-8
4. Zhu N, Zhang D, Wang W, Li X, Yang B, Song J, et al. A novel coronavirus from patients with pneumonia in China, 2019. N Engl J Med (2020) 382(8):727–33. doi: 10.1056/NEJMoa2001017
5. Abd El-Aziz TM, Stockand JD. Recent progress and challenges in drug development against COVID-19 coronavirus (SARS-CoV-2) - an update on the status. Infect Genet Evol (2020) 83:104327. doi: 10.1016/j.meegid.2020.104327
6. Calabrese LH, Naides SJ. Viral arthritis. Infect Dis Clin North Am (2005) 19(4):963–80. doi: 10.1016/j.idc.2005.09.002
7. Franssila R, Hedman K. Infection and musculoskeletal conditions: Viral causes of arthritis. Best Pract Res Clin Rheumatol. (2006) 20(6):1139–57. doi: 10.1016/j.berh.2006.08.007
8. Hu B, Guo H, Zhou P, Shi ZL. Characteristics of SARS-CoV-2 and COVID-19. Nat Rev Microbiol (2021) 19(3):141–54. doi: 10.1038/s41579-020-00459-7
9. Sohrabi C, Alsafi Z, O'Neill N, Khan M, Kerwan A, Al-Jabir A, et al. World health organization declares global emergency: A review of the 2019 novel coronavirus (COVID-19). Int J Surg (2020) 76:71–6. doi: 10.1016/j.ijsu.2020.02.034
10. Eckermann M, Frohn J, Reichardt M, Osterhoff M, Sprung M, Westermeier F, et al. 3D virtual pathohistology of lung tissue from covid-19 patients based on phase contrast X-ray tomography. Elife (2020) 9:e60408. doi: 10.7554/eLife.60408
11. Kim JH, Marks F, Clemens JD. Looking beyond COVID-19 vaccine phase 3 trials. Nat Med (2021) 27(2):205–11. doi: 10.1038/s41591-021-01230-y
12. Jordan RE, Adab P, Cheng KK. Covid-19: risk factors for severe disease and death. BMJ (2020) 368:m1198. doi: 10.1136/bmj.m1198
13. Siedner MJ, Triant V. Undetectable = untransmittable and your health: The personal benefits of early and continuous therapy for HIV infection. J Infect Dis (2019) 219(2):173–6. doi: 10.1093/infdis/jiy445
14. Lasry A, Medley A, Behel S, Mujawar MI, Cain M, Diekman ST, et al. Scaling up testing for human immunodeficiency virus infection among contacts of index patients - 20 countries, 2016-2018. MMWR Morb Mortal Wkly Rep (2019) 68(21):474–7. doi: 10.15585/mmwr.mm6821a2
15. HIV/AIDS factsheet. World Health Organization (2022). Available at: https://www.who.int/news-room/fact-sheets/detail/hiv-aids.
16. Tesoriero JM, Swain CE, Pierce JL, Zamboni L, Wu M, Holtgrave DR, et al. et al: COVID-19 outcomes among persons living with or without diagnosed HIV infection in new York state. JAMA Netw Open (2021) 4(2):e2037069.doi: 10.1001/jamanetworkopen.2020.37069
17. Davies MA. HIV And risk of COVID-19 death: a population cohort study from the Western cape province, south Africa. medRxiv (2020). doi: 10.1101/2020.07.02.20145185
18. Dong Y, Li Z, Ding S, Liu S, Tang Z, Jia L, et al. HIV Infection and risk of COVID-19 mortality: A meta-analysis. Med (Baltimore) (2021) 100(26):e26573. doi: 10.1097/MD.0000000000026573
19. Bertagnolio S, Silva R, Nagarajan S, Thwin S, Jassat W, Fowler R, et al. Are people living with HIV at higher risk of severe and fatal COVID-19? In: Journal of the international aids society, vol. 2022. (West Sussex, England:John Wiley & Sons Ltd_ (2022). p. 17–8. The Atrium, Southern Gate, Chichester Po19 8sq, W.
20. Paiardini M, Müller-Trutwin M. HIV-Associated chronic immune activation. Immunol Rev (2013) 254(1):78–101. doi: 10.1111/imr.12079
21. Ye Q, Wang B, Mao J. Cytokine storm in COVID-19 and treatment. J Infect (2020) 80(6):607–13. doi: 10.1016/j.jinf.2020.03.037
22. Levy Y, Wiedemann A, Hejblum BP, Durand M, Lefebvre C, Surenaud M, et al. CD177, a specific marker of neutrophil activation, is associated with coronavirus disease 2019 severity and death. iScience (2021) 24(7):102711. doi: 10.1016/j.isci.2021.102711
23. Rahman MR, Islam T, Shahjaman M, Islam MR, Lombardo SD, Bramanti P, et al. Discovering common pathogenetic processes between COVID-19 and diabetes mellitus by differential gene expression pattern analysis. Brief Bioinform (2021) 22(6):bbab262. doi: 10.1093/bib/bbab262
24. Szklarczyk D, Gable AL, Lyon D, Junge A, Wyder S, Huerta-Cepas J, et al. STRING v11: protein-protein association networks with increased coverage, supporting functional discovery in genome-wide experimental datasets. Nucleic Acids Res (2019) 47(D1):D607–13. doi: 10.1093/nar/gky1131
25. Hu H, Tang N, Zhang F, Li L, Li L. Bioinformatics and system biology approach to identify the influences of COVID-19 on rheumatoid arthritis. Front Immunol (2022) 13:860676. doi: 10.3389/fimmu.2022.860676
26. Chin CH, Chen SH, Wu HH, Ho CW, Ko MT, Lin CY. cytoHubba: identifying hub objects and sub-networks from complex interactome. BMC Syst Biol (2014) 8 Suppl 4:S11. doi: 10.1186/1752-0509-8-S4-S11
27. Lambert SA, Jolma A, Campitelli LF, Das PK, Yin Y, Albu M, et al. The human transcription factors. Cell (2018) 172(4):650–65. doi: 10.1016/j.cell.2018.01.029
28. Chen EY, Tan CM, Kou Y, Duan Q, Wang Z, Meirelles GV, et al. Enrichr: Interactive and collaborative HTML5 gene list enrichment analysis tool. BMC Bioinf (2013) 14:128. doi: 10.1186/1471-2105-14-128
29. Papadopoulos GL, Reczko M, Simossis VA, Sethupathy P, Hatzigeorgiou AG. The database of experimentally supported targets: a functional update of TarBase. Nucleic Acids Res (2009) 37(Database issue):D155–8. doi: 10.1093/nar/gkn809
30. Chou CH, Shrestha S, Yang CD, Chang NW, Lin YL, Liao KW, et al. et al: miRTarBase update 2018: a resource for experimentally validated microRNA-target interactions. Nucleic Acids Res (2018) 46(D1):D296–302. doi: 10.1093/nar/gkx1067
31. Yoo M, Shin J, Kim J, Ryall KA, Lee K, Lee S, et al. DSigDB: Drug signatures database for gene set analysis. Bioinformatics (2015) 31(18):3069–71. doi: 10.1093/bioinformatics/btv313
32. Pinero J, Bravo A, Queralt-Rosinach N, Gutierrez-Sacristan A, Deu-Pons J, Centeno E, et al. DisGeNET: A comprehensive platform integrating information on human disease-associated genes and variants. Nucleic Acids Res (2017) 45(D1):D833–9. doi: 10.1093/nar/gkw943
33. Pinero J, Queralt-Rosinach N, Bravo A, Deu-Pons J, Bauer-Mehren A, Baron M, et al. DisGeNET: A discovery platform for the dynamical exploration of human diseases and their genes. Database (Oxford) (2015) 2015:bav028. doi: 10.1093/database/bav028
34. Pinero J, Ramirez-Anguita JM, Sauch-Pitarch J, Ronzano F, Centeno E, Sanz F, et al. The DisGeNET knowledge platform for disease genomics: 2019 update. Nucleic Acids Res (2020) 48(D1):D845–55.doi: 10.1093/nar/gkz1021
35. Pinero J, Sauch J, Sanz F, Furlong LI. The DisGeNET cytoscape app: Exploring and visualizing disease genomics data. Comput Struct Biotechnol J (2021) 19:2960–7. doi: 10.1016/j.csbj.2021.05.015
36. Chen L, Zhang YH, Lu G, Huang T, Cai YD. Analysis of cancer-related lncRNAs using gene ontology and KEGG pathways. Artif Intell Med (2017) 76:27–36. doi: 10.1016/j.artmed.2017.02.001
37. Bartel DP. MicroRNAs: genomics, biogenesis, mechanism, and function. Cell (2004) 116(2):281–97. doi: 10.1016/S0092-8674(04)00045-5
38. Mahmud SMH, Al-Mustanjid M, Akter F, Rahman MS, Ahmed K, Rahman MH, et al. Bioinformatics and system biology approach to identify the influences of SARS-CoV-2 infections to idiopathic pulmonary fibrosis and chronic obstructive pulmonary disease patients. Brief Bioinform (2021) 22(5):bbab115. doi: 10.1093/bib/bbab115
39. Peng WF, Bai F, Shao K, Shen LS, Li HH, Huang S. The key genes underlying pathophysiology association between the type 2-diabetic and colorectal cancer. J Cell Physiol (2018) 233(11):8551–7. doi: 10.1002/jcp.26440
40. Ahmed F, Ansari JA, Ansari ZE, Alam Q, Gan SH, Kamal MA, et al. A molecular bridge: connecting type 2 diabetes and alzheimer's disease. CNS Neurol Disord Drug Targets (2014) 13(2):312–21. doi: 10.2174/18715273113126660133
41. German Advisory Committee Blood SAoPTbB. Human immunodeficiency virus (HIV). Transfus Med Hemother. (2016) 43(3):203–22.doi: 10.1159/000445852
42. Global HIV/AIDS overview (2021). Available at: https://www.hiv.gov/federal-response/pepfar-global-aids/global-hiv-aids-overview.
43. Bertagnolio STS, Silva R, Nagarajan S, Jassat W, Fowler R, Haniffa R, et al. Clinical features of, and risk factors for, severe or fatal COVID-19 among people living with HIV admitted to hospital: analysis of data from the WHO global clinical platform of COVID-19. Lancet HIV (2022) 9:E486–95. doi: 10.1016/S2352-3018(22)00097-2
44. Ssentongo P, Heilbrunn ES, Ssentongo AE, Advani S, Chinchilli VM, Nunez JJ, et al. Epidemiology and outcomes of COVID-19 in HIV-infected individuals: A systematic review and meta-analysis. Sci Rep (2021) 11(1):6283. doi: 10.1038/s41598-021-85359-3
45. Varshney K, Ghosh P, Stiles H, Iriowen R. Risk factors for COVID-19 mortality among people living with HIV: a scoping review. AIDS Behav (2022) 2022:1–10. doi: 10.1007/s10461-022-03578-9
46. Tapley A AJ, Fisher L, Huang Y, Ketter N, Villaran M, Gilbert P, et al. he National AIDS Treatment Advocacy Progra: High prevalence of asymptomatic Omicron carriage and correlation with CD4 T cell count among adults with HIV enrolling in COVPN 3008 Ubuntu clinical trial in sub-Saharan Africa. J Int AIDS Soc (2022) 2022:218–9. Available at: https://www.natap.org/2022/IAC/IAC_122.htm. Accessed 21 Aug 2022.
47. Lu Y, Su F, Yang H, Xiao Y, Zhang X, Su H, et al. E2F1 transcriptionally regulates CCNA2 expression to promote triple negative breast cancer tumorigenicity. Cancer Biomark (2022) 33(1):57–70. doi: 10.3233/CBM-210149
48. Bao B, Yu X, Zheng W. MiR-139-5p targeting CCNB1 modulates proliferation, migration, invasion and cell cycle in lung adenocarcinoma. Mol Biotechnol (2022) 64(8):852–60. doi: 10.1007/s12033-022-00465-5
49. Bruno S, Ghelli Luserna di Rora A, Napolitano R, Soverini S, Martinelli G, Simonetti G. CDC20 in and out of mitosis: a prognostic factor and therapeutic target in hematological malignancies. J Exp Clin Cancer Res (2022) 41(1):159. doi: 10.1186/s13046-022-02363-9
50. Nielsen CF, Zhang T, Barisic M, Kalitsis P, Hudson DF. Topoisomerase IIalpha is essential for maintenance of mitotic chromosome structure. Proc Natl Acad Sci USA (2020) 117(22):12131–42. doi: 10.1073/pnas.2001760117
51. Bock JO, Ortea I. Re-analysis of SARS-CoV-2-infected host cell proteomics time-course data by impact pathway analysis and network analysis: A potential link with inflammatory response. Aging (Albany NY) (2020) 12(12):11277–86. doi: 10.18632/aging.103524
52. Liu Z, Sun Q, Wang X. PLK1, a potential target for cancer therapy. Transl Oncol (2017) 10(1):22–32. doi: 10.1016/j.tranon.2016.10.003
53. Koyuncu D, Sharma U, Goka ET, Lippman ME. Spindle assembly checkpoint gene BUB1B is essential in breast cancer cell survival. Breast Cancer Res Treat (2021) 185(2):331–41. doi: 10.1007/s10549-020-05962-2
54. Li Z, Yu B, Qi F, Li F. KIF11 serves as an independent prognostic factor and therapeutic target for patients with lung adenocarcinoma. Front Oncol (2021) 11:670218. doi: 10.3389/fonc.2021.670218
55. Xu T, Dong M, Li H, Zhang R, Li X. Elevated mRNA expression levels of DLGAP5 are associated with poor prognosis in breast cancer. Oncol Lett (2020) 19(6):4053–65. doi: 10.3892/ol.2020.11533
56. Abdel-Rahman MA, Mahfouz M, Habashy HO. RRM2 expression in different molecular subtypes of breast cancer and its prognostic significance. Diagn Pathol (2022) 17(1):1. doi: 10.1186/s13000-021-01174-4
57. Pioro M, Jakimowicz D. Chromosome segregation proteins as coordinators of cell cycle in response to environmental conditions. Front Microbiol (2020) 11:588. doi: 10.3389/fmicb.2020.00588
58. Bianco C, Mohr I. Ribosome biogenesis restricts innate immune responses to virus infection and DNA. Elife (2019) 8:e49551. doi: 10.7554/eLife.49551
59. Mohr I, Sonenberg N. Host translation at the nexus of infection and immunity. Cell Host Microbe (2012) 12(4):470–83. doi: 10.1016/j.chom.2012.09.006
60. Stern-Ginossar N, Thompson SR, Mathews MB, Mohr I. Translational control in virus-infected cells. Cold Spring Harb Perspect Biol (2019) 11(3):a033001. doi: 10.1101/cshperspect.a033001
61. Fan Y, Sanyal S, Bruzzone R. Breaking bad: How viruses subvert the cell cycle. Front Cell Infect Microbiol (2018) 8:396. doi: 10.3389/fcimb.2018.00396
62. Li FQ, Tam JP, Liu DX. Cell cycle arrest and apoptosis induced by the coronavirus infectious bronchitis virus in the absence of p53. Virology (2007) 365(2):435–45. doi: 10.1016/j.virol.2007.04.015
63. Wu W, Munday DC, Howell G, Platt G, Barr JN, Hiscox JA. Characterization of the interaction between human respiratory syncytial virus and the cell cycle in continuous cell culture and primary human airway epithelial cells. J Virol (2011) 85(19):10300–9. doi: 10.1128/JVI.05164-11
64. Berger G, Lawrence M, Hue S, Neil SJ. G2/M cell cycle arrest correlates with primate lentiviral vpr interaction with the SLX4 complex. J Virol (2015) 89(1):230–40. doi: 10.1128/JVI.02307-14
65. Yi R, Saito K, Isegawa N, Shirasawa H. Alteration of cell cycle progression by sindbis virus infection. Biochem Biophys Res Commun (2015) 462(4):426–32. doi: 10.1016/j.bbrc.2015.04.148
66. Seo J, Jin D, Choi CH, Lee H. Integration of MicroRNA, mRNA, and protein expression data for the identification of cancer-related MicroRNAs. PloS One (2017) 12(1):e0168412. doi: 10.1371/journal.pone.0168412
67. Chen K, Rajewsky N. The evolution of gene regulation by transcription factors and microRNAs. Nat Rev Genet (2007) 8(2):93–103. doi: 10.1038/nrg1990
68. Fernandez Esmerats J, Villa-Roel N, Kumar S, Gu L, Salim MT, Ohh M, et al. Disturbed flow increases UBE2C (Ubiquitin E2 ligase c) via loss of miR-483-3p, inducing aortic valve calcification by the pVHL (von hippel-lindau protein) and HIF-1alpha (Hypoxia-inducible factor-1alpha) pathway in endothelial cells. Arterioscler Thromb Vasc Biol (2019) 39(3):467–81. doi: 10.1161/ATVBAHA.118.312233
69. Garcia-Moreno M, Jarvelin AI, Castello A. Unconventional RNA-binding proteins step into the virus-host battlefront. Wiley Interdiscip Rev RNA (2018) 9(6):e1498. doi: 10.1002/wrna.1498
70. Nazitto R, Amon LM, Mast FD, Aitchison JD, Aderem A, Johnson JS, et al. ILF3 is a negative transcriptional regulator of innate immune responses and myeloid dendritic cell maturation. J Immunol (2021) 206(12):2949–65. doi: 10.4049/jimmunol.2001235
71. Ylisastigui L, Archin N, Lehrman G, Bosch R, Margolis D. Coaxing human immunodeficiency virus type 1 from resting CD4+ T cells: can the reservoir of HIV be purged. AIDS (2004) 18(8):1101–8. doi: 10.1097/00002030-200405210-00003
72. Izumi T, Io K, Matsui M, Shirakawa K, Shinohara M, Nagai Y, et al. HIV-1 viral infectivity factor interacts with TP53 to induce G2 cell cycle arrest and positively regulate viral replication. Proc Natl Acad Sci USA (2010) 107(48):20798–803. doi: 10.1073/pnas.1008076107
73. Das B, Dobrowolski C, Luttge B, Valadkhan S, Chomont N, Johnston R, et al. Estrogen receptor-1 is a key regulator of HIV-1 latency that imparts gender-specific restrictions on the latent reservoir. Proc Natl Acad Sci USA (2018) 115(33):E7795–804. doi: 10.1073/pnas.1803468115
74. Pan XY, Zhao W, Zeng XY, Lin J, Li MM, Shen XT, et al. Heat shock factor 1 mediates latent HIV reactivation. Sci Rep (2016) 6:26294. doi: 10.1038/srep26294
75. Yousefi H, Poursheikhani A, Bahmanpour Z, Vatanmakanian M, Taheri M, Mashouri L, et al. SARS-CoV infection crosstalk with human host cell noncoding-RNA machinery: An in-silico approach. BioMed Pharmacother (2020) 130:110548. doi: 10.1016/j.biopha.2020.110548
76. Vasudevan A, Schukken KM, Sausville EL, Girish V, Adebambo OA, Sheltzer JM. Aneuploidy as a promoter and suppressor of malignant growth. Nat Rev Cancer (2021) 21(2):89–103. doi: 10.1038/s41568-020-00321-1
77. Bakhoum SF, Cantley LC. The multifaceted role of chromosomal instability in cancer and its microenvironment. Cell (2018) 174(6):1347–60. doi: 10.1016/j.cell.2018.08.027
78. Pathania AS, Prathipati P, Abdul BA, Chava S, Katta SS, Gupta SC, et al. COVID-19 and cancer comorbidity: Therapeutic opportunities and challenges. Theranostics (2021) 11(2):731–53. doi: 10.7150/thno.51471
79. Basu D, Chavda VP, Mehta AA. Therapeutics for COVID-19 and post COVID-19 complications: An update. Curr Res Pharmacol Drug Discov (2022) 2022:100086. doi: 10.1016/j.crphar.2022.100086
80. Lovetrue B. The AI-discovered aetiology of COVID-19 and rationale of the irinotecan+ etoposide combination therapy for critically ill COVID-19 patients. Med Hypotheses (2020) 144:110180. doi: 10.1016/j.mehy.2020.110180
81. Patel M, Dominguez E, Sacher D, Desai P, Chandar A, Bromberg M, et al. Etoposide as salvage therapy for cytokine storm due to coronavirus disease 2019. Chest (2021) 159(1):e7–11. doi: 10.1016/j.chest.2020.09.077
82. Hosseinipour MC, Kang M, Krown SE, Bukuru A, Umbleja T, Martin JN, et al. As-needed vs immediate etoposide chemotherapy in combination with antiretroviral therapy for mild-to-Moderate AIDS-associated kaposi sarcoma in resource-limited settings: A5264/AMC-067 randomized clinical trial. Clin Infect Dis (2018) 67(2):251–60. doi: 10.1093/cid/ciy044
83. Abruzzese E, Luciano L, D'Agostino F, Trawinska MM, Pane F, De Fabritiis P. SARS-CoV-2 (COVID-19) and chronic myeloid leukemia (CML): A case report and review of ABL kinase involvement in viral infection. Mediterr J Hematol Infect Dis (2020) 12(1):e2020031. doi: 10.4084/mjhid.2020.031
84. Bermejo M, Lopez-Huertas MR, Garcia-Perez J, Climent N, Descours B, Ambrosioni J, et al. Dasatinib inhibits HIV-1 replication through the interference of SAMHD1 phosphorylation in CD4+ T cells. Biochem Pharmacol (2016) 106:30–45. doi: 10.1016/j.bcp.2016.02.002
85. Pogliaghi M, Papagno L, Lambert S, Calin R, Calvez V, Katlama C, et al. The tyrosine kinase inhibitor dasatinib blocks in-vitro HIV-1 production by primary CD4+ T cells from HIV-1 infected patients. AIDS (2014) 28(2):278–81. doi: 10.1097/QAD.0000000000000073
86. Zhang F, Yu C, Xu W, Li X, Feng J, Shi H, et al. Identification of critical genes and molecular pathways in COVID-19 myocarditis and constructing gene regulatory networks by bioinformatic analysis. PloS One (2022) 17(6):e0269386. doi: 10.1371/journal.pone.0269386
87. Cabrera-Munoz E, Hernandez-Hernandez OT, Camacho-Arroyo I. Role of estradiol and progesterone in HIV susceptibility and disease progression. Mini Rev Med Chem (2012) 12(11):1049–54. doi: 10.2174/138955712802762185
88. Seeland U, Coluzzi F, Simmaco M, Mura C, Bourne PE, Heiland M, et al. Evidence for treatment with estradiol for women with SARS-CoV-2 infection. BMC Med (2020) 18(1):369. doi: 10.1186/s12916-020-01851-z
89. Chan CN, Trinite B, Levy DN. Potent inhibition of HIV-1 replication in resting CD4 T cells by resveratrol and pterostilbene. Antimicrob Agents Chemother (2017) 61(9):e00408–17. doi: 10.1128/AAC.00408-17
90. Heidari S, Mohammadi S, Fathi M, Cigary S, Alisamir M, Mirkarimi M, et al. Association of vitamin d status with COVID-19 disease severity in pediatric patients: A retrospective observational study. Health Sci Rep (2022) 5(3):e569. doi: 10.1002/hsr2.569
91. Al-Kuraishy HM, Al-Gareeb AI, Faidah H, Alexiou A, Batiha GE. Testosterone in COVID-19: An adversary bane or comrade boon. Front Cell Infect Microbiol (2021) 11:666987. doi: 10.3389/fcimb.2021.666987
92. Gomes AR, Souteiro P, Silva CG, Sousa-Pinto B, Almeida F, Sarmento A, et al. Prevalence of testosterone deficiency in HIV-infected men under antiretroviral therapy. BMC Infect Dis (2016) 16(1):628. doi: 10.1186/s12879-016-1892-5
93. Antinori A, Coenen T, Costagiola D, Dedes N, Ellefson M, Gatell J, et al. Late presentation of HIV infection: a consensus definition. HIV Med (2011) 12(1):61–4. doi: 10.1111/j.1468-1293.2010.00857.x
Keywords: COVID-19, HIV, common differentially expressed genes, hub genes, small molecular compounds
Citation: Yan C, Niu Y and Wang X (2022) Blood transcriptome analysis revealed the crosstalk between COVID-19 and HIV. Front. Immunol. 13:1008653. doi: 10.3389/fimmu.2022.1008653
Received: 01 August 2022; Accepted: 29 September 2022;
Published: 28 October 2022.
Edited by:
Arif Ansori, Airlangga University, IndonesiaReviewed by:
Mohammad Rizki Fadhil Pratama, Universitas Muhammadiyah Palangkaraya, IndonesiaArli Aditya Parikesit, Indonesia International Institute for Life-Sciences (i3L), Indonesia
Ibrahim Ahmed Shaikh, Najran University, Saudi Arabia
Copyright © 2022 Yan, Niu and Wang. This is an open-access article distributed under the terms of the Creative Commons Attribution License (CC BY). The use, distribution or reproduction in other forums is permitted, provided the original author(s) and the copyright owner(s) are credited and that the original publication in this journal is cited, in accordance with accepted academic practice. No use, distribution or reproduction is permitted which does not comply with these terms.
*Correspondence: Cheng Yan, yancheng907@xxu.edu.cn; Xuannian Wang, wangxuannian@163.com
†These authors have contributed equally to this work