- 1Huaxi MR Research Center (HMRRC), Department of Radiology, Functional and Molecular Imaging Key Laboratory of Sichuan Province, West China Hospital, Sichuan University, Chengdu, China
- 2Department of Clinic Medical Center, Dazhou Central Hospital, Dazhou, China
- 3Laboratory of Clinical Nuclear Medicine, Department of Nuclear Medicine, West China Hospital, Sichuan University, Chengdu, China
- 4Department of Neurology, West China Hospital, Sichuan University, Chengdu, China
- 5Department of Clinical Research Management, West China Hospital, Sichuan University, Chengdu, China
Glioblastoma (GBM) is the most common malignant tumor of the central nervous system with poor prognosis. Although the field of immunotherapy in glioma is developing rapidly, glioblastoma is still prone to recurrence under strong immune intervention. The major challenges in the process of immunotherapy are evaluating the curative effect, accurately distinguishing between treatment-related reactions and tumor recurrence, and providing guidance for clinical decision-making. Since the conventional magnetic resonance imaging (MRI) is usually difficult to distinguish between pseudoprogression and the true tumor progression, many studies have used various advanced imaging techniques to evaluate treatment-related responses. Meanwhile, criteria for efficacy evaluation of immunotherapy are constantly updated and improved. A standard imaging scheme to evaluate immunotherapeutic response will benefit patients finally. This review mainly summarizes the application status and future trend of several advanced imaging techniques in evaluating the efficacy of GBM immunotherapy.
Introduction
Glioblastoma is the most common malignant brain tumor in adult and is extremely aggressive. The current standard treatment involves maximal safe resection, followed by radiotherapy and adjuvant chemotherapy (1). Despite this active treatment, the prognosis remains poor, with a median survival of less than 2 years (2). The main reason is that glioblastoma is strongly aggressive and grows rapidly, specifically, tumor cells are prone to infiltrate the normal brain parenchyma aside the lesion (3, 4). Thus, there is a risk of tumor recurrence once tumor stem cells remain after the resection and follow-up treatment. Many other treatments have been studied, such as immunotherapy, aiming to stimulate or mobilize the immune system and enhance the antitumor immunity in the tumor microenvironment, so as to control and kill tumor cells (5). This treatment concept has derived a variety of treatment strategies, and remarkable progress of those methods has been made in the treatment of patients with intractable solid tumors such as melanoma and nonsmall cell lung cancer (6, 7). There are also many immunotherapy studies of glioma not only basic but also clinical. Due to the existence of blood-brain barrier (BBB) in the central nervous system (8), obvious loss of lymphatic reflux system (9), and the strong heterogeneity of GBM (10), the effectiveness of immunotherapy for brain tumors might be limited. Fortunately, it has been found that immunotherapy has the potential to induce immune changes in brain tumors (11, 12).
One of the challenges in the treatment of GBM is how to assess the treatment response accurately in order to make more informed clinical decisions. It is important to evaluate the treatment response to immunotherapy in an early stage by using noninvasive imaging, which can reduce unnecessary clinical complications. However, conventional imaging techniques are usually difficult to distinguish between pseudoprogression and tumor recurrence. Immune response is usually accompanied with inflammatory reaction characterized by the enlargement of enhanced foci, which is easily confused with the behavior of tumor relapse. Effective immunotherapy may be mistakenly terminated if being misdiagnosed, thus causing a negative impact on the prognosis. To solve this problem, researchers have carried out a lot of researches on advanced imaging techniques. This review describes the definition and clinical significance of pseudoprogression, generalizes the response evaluation criteria of GBM, summarizes the status and future development direction of advanced imaging techniques relevant to immunotherapy in GBM, and discusses the strengths and deficiencies of artificial intelligence (AI) in monitoring therapeutic response in GBM.
Pseudoprogression of GBM
About 30% of GBM patients who received radiotherapy and adjuvant temozolomide-based chemotherapy had pseudoprogression, which mainly occurred within 3 months after treatment (13). According to the Response Assessment in Neuro-Oncology (RANO) criteria, pseudoprogression was defined as the appearance of new lesion or an increase in contrast-enhancing areas, but these changes gradually faded or stabilized without changing the treatment (14). At present, it is believed that the enlargement of enhanced foci may be caused by the infiltration of inflammatory factors after radiotherapy and chemotherapy, but the real cause of pseudoprogression remains to be further studied. In addition, the methylation status of the O6-methylguanine-DNA methyltransferase (MGMT) promoter was associated with pseudoprogression, and about 2/3 of GBM patients with MGMT methylation exhibited pseudoprogression (15).
Patients with pseudoprogression usually have no clinical symptoms and only show new or enlarged enhanced lesions on images. Such patients usually only need symptomatic treatment and do not need to change the treatment project, while patients with tumor recurrence probably need to resect the lesion again or find another cure. If there is no accurate distinction between them, the effectiveness of treatment may be reduced. Therefore, correct identification of pseudoprogression and tumor recurrence is of great significance to guide clinical decision-making.
Response Evaluation Criteria of GBM
Noninvasive imaging for GBM can help define widely applicable treatment response criteria to assess disease progression and make clinical decisions. In order to address imaging challenges such as pseudoprogression, multidisciplinary experts developed RANO criteria (14), which suggested that the original treatment regimen can be maintained for patients with no clear clinical symptoms and only tumor progression on imaging. These patients only need regular follow-up. At present, the RANO criteria have been widely accepted in the field of neuro-oncology and applied in clinical and scientific researches. However, evaluating the therapeutic response to immunotherapy only by RANO criteria may not be sufficient. For example, the mechanism of pseudoprogression caused by immunotherapy may be different from that of standard therapy, which may be due to the infiltration of immune cells and inflammatory cells. It is necessary to establish corresponding imaging response criteria for immunotherapy in GBM.
Based on the important factors above, experts developed immunotherapy Response Assessment in Neuro-Oncology (iRANO) criteria for patients with GBM receiving immunotherapy to provide guidance for imaging changes in the early stage of progression (16). According to the iRANO criteria, the time window for pseudoprogression after immunotherapy is 6 months. Hence, the criteria recommend that patients with no significant clinical symptoms and evidence of early imaging progress within 6 months after immunotherapy should continue to receive immunotherapy before follow-up imaging confirms the tumor progression. In other words, patients with evidence of imaging progress outside the time window after immunotherapy will have a higher probability of potential true tumor progression, and these patients should be advised to discontinue ongoing immunotherapy.
Application of Advanced Imaging in Immunotherapy of GBM
At present, the researches of glioma immunotherapy strategy mainly include the following: (1) specific peptide vaccine; (2) immunotoxin therapy; (3) immune checkpoint inhibitors (ICIs) therapy; (4) dendritic cell (DC) therapy; and (5) chimeric antigen receptor T-cell (CAR-T) Immunotherapy (17–21). The feasibility and safety of DC vaccine in the treatment of glioma have been proved, and it could induce immune response (20). It is worth noting that a new type of gamma delta T (γδ T)-cell therapy is becoming a rising star of cancer immunotherapy (22). Unlike the alpha beta T (αβ T) cells involved in most T-cell researches and clinical applications, γδ T cells recognize their target cells independently of major histocompatibility complex (MHC) and do not cause graft-versus-host disease. γδ T cells infiltrate in a variety of tissues, which can quickly respond to the target cells and release effector cytokines. Furthermore, the recognition and killing of tumor by γδ T cells do not depend on the expression of single antigen (23). Based on the advantages of γδ T cells, a new CAR-T therapy can be developed to break through the limited application of αβ T-cell-based CAR-T-cell therapy in solid tumors (including gliomas) (24, 25). Currently, γδ T-cell therapy has been studied in the treatment and prevention of recurrence of solid tumors including head and neck cancer, breast cancer, and lung cancer (26–28). The therapeutic effect in glioma still needs to be verified in a large number of clinical trials.
Advanced imaging techniques based on physiological or metabolic characteristics may reflect the state of tumor more accurately, so various advanced imaging techniques are being studied to correctly identify immunotherapy-related changes and tumor progression and provide a credible basis for the treatment of patients. The advanced imaging techniques used in GBM currently include perfusion-weighted imaging (PWI), diffusion imaging, amide proton transfer (APT), magnetic resonance spectroscopy (MRS), positron emission tomography (PET) (i.e., Table 1). Some of these imaging techniques have been used to evaluate the immunotherapy efficacy of glioma. The following will introduce the basic concepts of these imaging techniques and describe the latest research progress and future application prospects that support them in the evaluation of therapeutic response to immunotherapy.
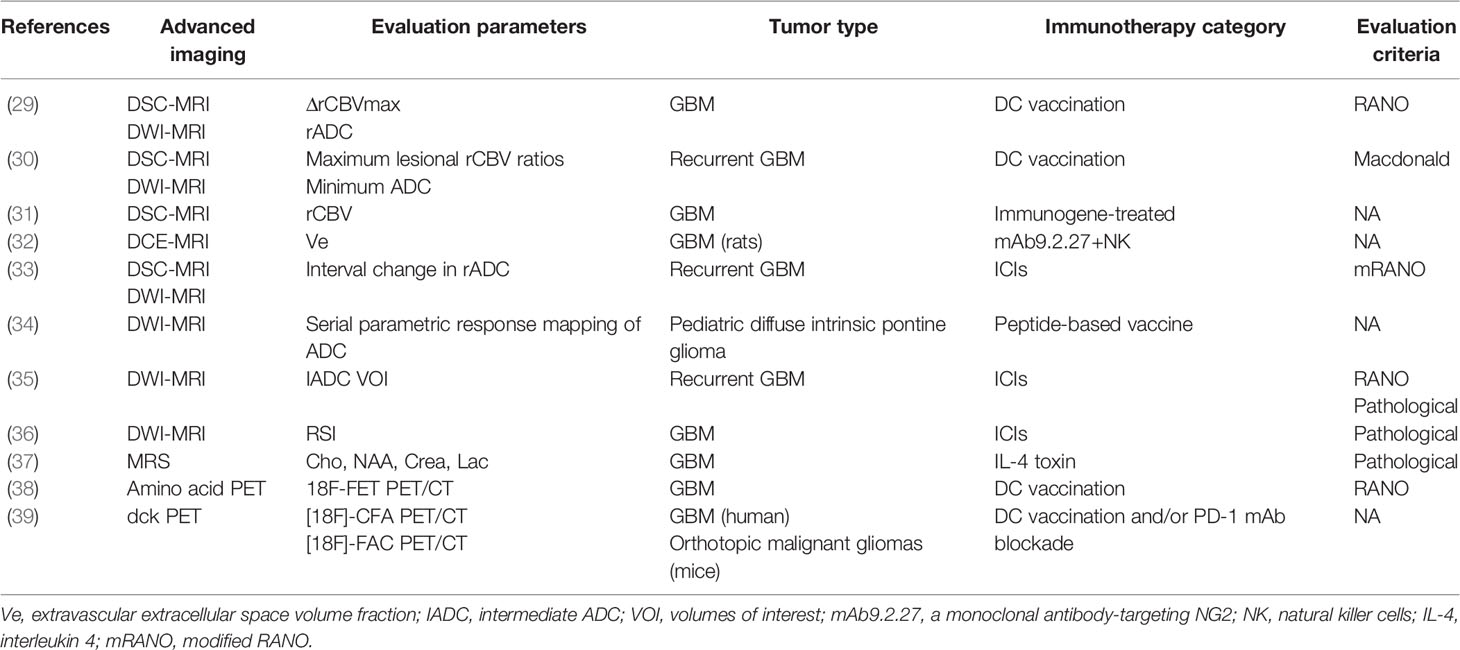
Table 1 Studies of applying advanced imaging techniques to assess immunotherapeutic responses in GBM.
Perfusion-Weighted Imaging
PWI can reflect tissue perfusion by quantitatively calculating perfusion parameters including relative cerebral blood volume (rCBV), relative cerebral blood flow (rCBF), mean transit time (MTT), and time to peak (TTP). When the tumor progresses, neovascularization and increased perfusion could be observed in the lesion area. As pseudoprogression is usually caused by inflammation, there is no neovascularization and the perfusion is relatively low. As a consequence, these perfusion parameters can be used to distinguish between pseudoprogression and tumor recurrence in GBM patients receiving standard treatment or immunotherapy (30, 40).
DSC-MRI is the most commonly used perfusion technique in clinic. Evidence has shown that adding perfusion imaging to conventional MRI in patients with gliomas is helpful for clinical decision-making (41, 42). A recent meta-analysis including 35 studies on the role of various advanced imaging techniques in evaluating the therapeutic response of high-grade gliomas indicated that the diagnostic accuracy of perfusion imaging was only second to MR spectroscopy (MRS). The sensitivity and specificity of DSC were 87% and 86%, respectively, while the sensitivity and specificity of DCE were 92% and 85%, respectively (43). In addition, a retrospective study comparing the value of DSC-MRI and DCE-MRI combined with T1WI enhancement and DWI imaging in predicting the recurrence of GBM revealed that both the two perfusion imaging could significantly improve the diagnostic accuracy, and there was no significant difference in diagnostic performance (42). Similarly, some studies have compared the diagnostic accuracy of DSC-MRI with three-dimensional pseudocontinuous arterial spin labeling (3D-pcASL) and suggested that the ability of 3D-pcASL perfusion imaging in distinguishing between pseudoprogression and tumor recurrence in GBM patients is almost the same as that of DSC, but 3D-pcASL is superior to DSC when the lesions are disturbed by magnetic susceptibility artifacts (44, 45). The reason is that the fast spin echo (FSE) technology used in GE 3D-ASL can effectively overcome the disadvantages of DSC being vulnerable to susceptibility artifacts. The artifacts can attenuate the imaging signal, usually when the focus is on the skull base, paranasal sinuses or large surgical resection cavity with blood residue. Another deficiency of DSC imaging is that the contrast medium may leak into the space where the BBB is destroyed. When it happens, the values of rCBV parameters cannot reflect the real perfusion level (46). Also, there is no unified standard between different imaging parameters and postprocessing methods. These factors will affect the diagnostic accuracy of DSC perfusion imaging to varying degrees.
So far, there are still few researches about the application of PWI on assessing the immunotherapeutic response of GBM. In a study of advanced MRI assessing dendritic cell immunotherapy against GBM, it was found that the difference of relative cerebral blood volume (△rCBVmax) could effectively differentiate tumor recurrence from pseudoprogression, with a sensitivity of 67% and specificity of 75% (p = 0.004), suggesting that the value of △rCBV might be more helpful to distinguish them than the absolute value of rCBV during follow-up (29) (Figure 1). Research by Vrabec et al. showed that the maximal rCBV ratios in the contrast-enhancing area were potential radiological indicators to distinguish between inflammatory response induced by immunotherapy and tumor recurrence (30). Another follow-up study on immunogene-treated glioblastoma multiforme with DSC perfusion imaging combined with contrast-enhanced MR imaging also supported this view (31).
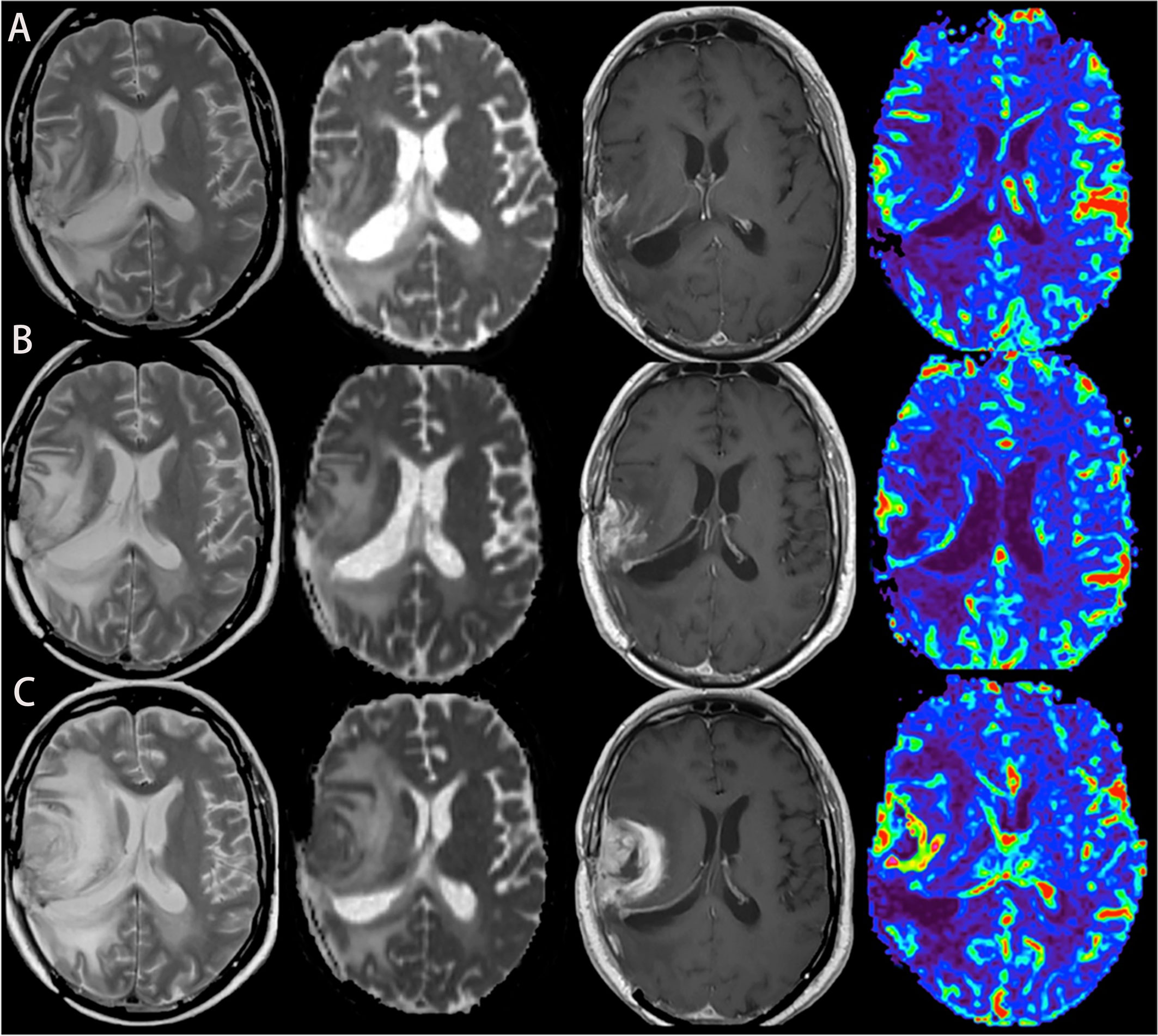
Figure 1 A case of glioblastoma relapsed during immunotherapy, T2, ADC map, T1-enhanced, and CBV map from left to right. (A–C) MRI was performed in the 2nd, 6th, and 8th months of immunotherapy, respectively, showing that the edema degree of the lesion was gradually aggravated, the enhancement was more obvious, and the perfusion was higher.
It is worth noting that both DCE-MRI and ASL techniques have not been widely explored in GBM patients treated with immunotherapy, which may be due to the lack of standardized acquisition parameters of DCE-MRI and the poor image signal of ASL perfusion imaging. However, these two perfusion techniques still have their own advantages. For instance, DCE-MRI can measure vascular permeability by pharmacokinetic parameters to quantify the movement of contrast media through BBB (47, 48). Compared with DSC-MRI, the ability of DCE-MRI of quantifying the permeability can make the calculation of cerebral blood volume more precise. 3D-pcASL can avoid the influence of magnetic susceptibility artifacts. If we could combine the advantages of various perfusion imaging to make up for the shortcomings, we would have a powerful supplementary tool to evaluate immunotherapeutic response in GBM.
Diffusion Imaging
Diffusion-Weighted Imaging (DWI) reflects the diffusion of water molecules in the tissue of interest. The most widely used quantitative parameter is the apparent diffusion coefficient (ADC), which is inversely proportional to the cell density (49, 50). Based on this characteristic, it has been used in tumor identification, grading, and therapeutic response monitoring (51–54). In patients with recurrent gliomas, the diffusion of water molecules within the tumor was limited and the ADC values decreased, while treatment-related response, such as pseudoprogression, had higher ADC values than recurrent gliomas. This point of view was confirmed by a meta-analysis of diffusion magnetic resonance imaging combined with ADC measurements for distinguishing between glioma recurrence and pseudoprogression. Six cohort studies were included in the meta-analysis, and different ADC values were analyzed, including mean ADC values, relative ADC (rADC), and 5th percentile values. The results proved that the ADC values of pseudoprogression was higher than that of tumor recurrence, which provided a reliable foundation for the differentiation of the two (55). To date, some researches have applied this technique to the assessment of glioma immunotherapy and studied the evaluation effect of different ADC values. Song et al. conducted a retrospective study of 19 patients with recurrent GBM to evaluate whether the early changes in the quantitative parameters of diffusion and perfusion MRI before and after immunotherapy can determine the treatment-related changes. They calculated the rADC values and several perfusion parameters of the lesions before and after treatment and found that only the change of rADC could be used as an early marker to evaluate the response within 6 months after treatment (33). Another study also proved that rADC could help predict the immuno-therapeutic response and survival rate in patients with GBM (29) (Figure 2). Moreover, serial parametric response mapping of ADC performed at multiple time points of therapy may help identify pseudoprogression as an imaging biomarker in vaccine therapy for pediatric diffuse intrinsic pontine glioma (34). However, some studies believe that the application of the mean ADC values on differentiating pseudoprogression from tumor recurrence has some limitations, because the ADC values of cystic and necrotic areas are higher than that of solid tumors, which will affect the accuracy of the final results. It is considered that the 5th percentile values are better for the distinction (56, 57). Although ADC has good diagnostic value as a whole, the practicability of these different ADC parameters needs to be further studied. In addition, these results need to be verified in multicenter and larger cohorts.
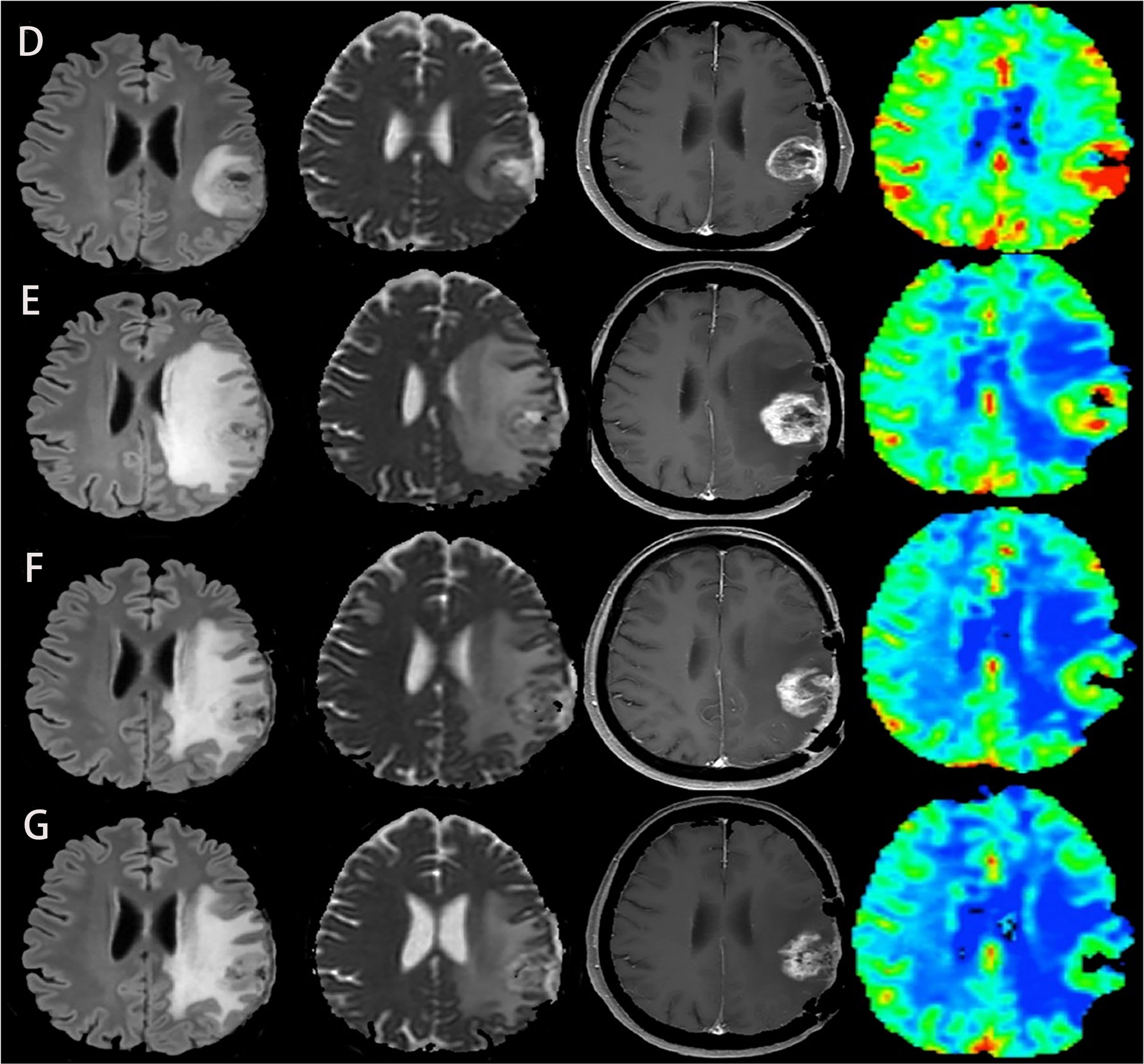
Figure 2 Another case of glioblastoma developed pseudoprogression during immunotherapy, with FLAIR, ADC map, T1-enhanced, and CBV map from left to right. (D–G) MRI was performed before immunotherapy and 2, 4, and 6 months after immunotherapy, respectively. Although tumor recurrence was suspected at the second month, the subsequent two MRI showed that the lesion became smaller, the degree of edema and imitation of diffusion alleviated, and the perfusion decreased. These two cases demonstrate that the combination of conventional MRI and advanced MRI imaging can accurately identify pseudoprogression and tumor recurrence of glioblastoma after immunotherapy. The above two figures were reproduced with the permission of (29) (Copyright at Multidisciplinary Digital Publishing Institute).
Another kind of imaging technique commonly used in clinic is diffusion tensor imaging (DTI), which uses the diffusion anisotropy of water molecules for imaging. The fractional anisotropy (FA) images can show the structure and anisotropy of white matter fibers in the brain, and the change of FA can evaluate the therapeutic effect. Wang et al. combined DTI and DSC-MRI and found that the best models to distinguish between true progression and none-true progression (pseudoprogression and mixed progression) included FA, linear anisotropy coefficient (CL), and rCBVmax. It is suggested that the combination of DTI and DSC perfusion parameters could help evaluate the therapeutic response of gliomas (58). Although DTI has not been applied to the evaluation of immunotherapy in GBM, a recent study on the association of T cell density and diffusion tensor MRI changes in brain metastases revealed that FA in the peritumoral region was closely related to the density of CD3+ T-cell infiltration, indicating that FA could reflect the tumor immune microenvironment. This finding supports future researches and can be used to detect the sensitivity of neurological tumors to immunotherapy (59).
Furthermore, researchers also explored the role of some advanced diffusion models in assessing therapeutic response of brain tumors. These techniques are mainly used in scientific researches, such as intravoxel incoherent motion (IVIM) MRI, restriction spectrum imaging (RSI) MRI, etc. Based on the double exponential model, IVIM can simultaneously obtain diffusion and perfusion parameters reflecting tumor cellularity and vascularity. Fast diffusion coefficient (D*) mainly reflects perfusion information, slow diffusion coefficient (D) represents real diffusion information, and perfusion fraction (f) reflects blood flow (60). At present, it has been successfully used in gliomas for grading and distinguishing between treatment-related changes and tumor progression (61–63). However, this technique has not been used to monitor the response to immunotherapy yet. RSI-MRI is an advanced DWI technique, which provides a direct method for measuring tumor cellularity in vivo (64). Compared with the traditional DWI model, RSI can improve the conspicuity and delineation of high-grade tumors (65), better distinguish between true and pseudoresponse in antiangiogenic therapy (66), and better display white matter tracts in peritumoral edema areas (67). These advantages indicate that RSI-MRI have a good application prospect in the immunotherapy of neurological tumors. In a case report of immunotherapy for GBM, authors demonstrated that RSI could differentiate between pseudoprogression and tumor relapse while conventional DWI imaging could not provide more information (36). Despite that these advanced DWI techniques can provide better tissue structural characteristics than traditional DWI, the potential pathophysiological mechanism of tumor is still unknown.
Amide Proton Transfer
Currently, APT is a relatively popular MR molecular imaging technique that can quantify free proteins with noninvasion and nonradiation. It reflects the changes of concentration and environment by detecting amide proton (NH) in endogenous low-concentration proteins or peptides. This technique displays its application value in a variety of central nervous system diseases (68–73) and shows great potential in glioma grading and curative effect evaluation (74, 75). Ma et al. used three-dimensional APT imaging technique combined with several conventional MRI sequences to evaluate the imaging features of tumor recurrence and pseudoprogression in 32 patients with gliomas who received standard treatment. It was found that the two kinds of progression had similar performance on conventional MRI. On the contrary, patients with tumor recurrence exhibited high signal intensity (relative to contralateral normal brain tissue) on APT-weighted (APTw) images, while patients with pseudoprogression showed equal to mild hyperintensity on APT-weighted images. Quantitative results demonstrated that compared with conventional MRI sequences, APTw could greatly improve the ability of MRI to distinguish between pseudoprogression and tumor recurrence (76). Additionally, as the therapeutic benefit and prognosis of glioma are related to its molecular subtypes and the expression of some proteins, APT imaging can detect the expression of MGMT protein before operation and provide relevant information for the possible drug resistance during treatment and the corresponding targeted therapy (77).
APT also has some shortcomings. In APTw images, red represents higher protein content, but not all red areas represent lesions or high-grade gliomas. Some tissues present high signal intensity on APTw images as well, like fat, cysts, and blood vessels. In addition to gliomas, there are other lesions that may also show high signal intensity, such as meningiomas, lymphomas, and some metastases. Most APTw images remove skull information because of the high signal of skull, which may hide potential lesions near the cerebral cortex. For this reason, using APT imaging alone to judge the nature of lesions may not be accurate enough, and it is best to combine multiple sequences to make a comprehensive diagnosis. Up to now, no research has reported the use of APT in the evaluation of immunotherapeutic response in GBM, but previous studies have shown that APT imaging is of great help to improve the diagnostic accuracy. If it is to become a powerful tool to assess immunotherapeutic response, it is necessary to continue exploiting and developing this technique and carrying out more clinical and scientific researches.
Magnetic Resonance Spectroscopy
MRS uses the phenomenon of magnetic resonance chemical shift to determine the molecular composition of substances. It can simultaneously measure the concentrations of several metabolites in brain tissue and tumors and can be used to diagnose, grade, and evaluate the curative effect of brain tumors (78). The metabolism of brain tumor is exuberant, while that of chronic inflammation is lower. From the metabolism degree of lesion, we can decide its composition and distinguish between benign and malignant tissues (79, 80). The typical proton magnetic resonance spectroscopy (1H-MRS) manifestation of glioma exhibits obvious inversion of Cho/NAA ratio, while inflammatory lesions are characterized by increased Cho/Cr ratio and normal or decreased NAA/Cr ratio (81, 82). Thus, the response induced by immunotherapy and tumor progression in glioma patients can be distinguished by the concentration of metabolites. Floeth et al. found that the metabolic data of MRS may help to distinguish between tumor recurrence and pseudoprogression after local immunotherapy of GBM and contribute to further decision-making (37). In addition, a recent meta-analysis suggested that among the advanced MRI techniques, MRS had the highest diagnostic accuracy in distinguishing between treatment-related changes and tumor recurrence, with a sensitivity and specificity of 91% and 95%, respectively, which showed the good diagnostic performance of MRS (43).
MRS has some limitations in detecting small lesions compared with other MR imaging techniques due to its low spatial resolution, and it needs to be collected in high quantities because of the low concentration of metabolites in tumor tissues, which needs more time. The determination of metabolite concentration may also be affected by MR equipment, pulse sequence and data postprocessing methods. Lastly, MRS requires experienced operators to define exactly areas of interest, which is faced with technical challenges in clinical practice (78).
Positron Emission Tomography
Positron emission tomography-computed tomography (PET-CT) is a metabolic functional imaging technique, which is applied to diagnose and analyze lesions by imaging radioactive markers. It is commonly used in clinical tumor staging, curative effect evaluation, and therapy. The most widely used PET tracer is 18F-fluorodeoxyglucose (18F-FDG) based on glycolysis, whose tracer concentration occurs in hypermetabolic lesions. Every technology has some deficiencies, and FDG-PET is no exception. First of all, the resolution of PET is relatively low, and normal brain tissue also shows high metabolism. If the lesion is close to the cerebral cortex, the measured FDG uptake value cannot reflect the true condition of the lesion. Furthermore, treatment-related necrotic reactions can also be characterized by increased glucose metabolism, resulting in increased FDG uptake (83). Although FDG-PET is widely used in clinic, it may be for some reasons above that make the accuracy of differential diagnosis of tumor recurrence and pseudoprogression not high (84, 85). Therefore, radioactive tracers with higher tumor-background uptake ratio have been studied.
Due to the increased proliferative activity and amino acid transport of malignant brain tumors, and the relatively low level of amino acid uptake in normal brain tissue, the use of amino acid-based radioactive tracers can improve the tumor-background ratio to some extent and identify tumors better (86). Till now, some radioactive tracers based on amino acids have been developed, such as 11C-methyl-L-methionine (11C-MET) and O-(2-[18F]fluoroethyl)-L-tyrosine (18F-FET). Studies have indicated that these two tracers have good accuracy in making a distinction between treatment-related response and tumor recurrence, and their manifestations are similar (87). However, 11C-MET is difficult to be commonly used in clinic owing to its short half-life and difficulties of preparation. Contrarily, 18F-FET has a long half-life, and the preparation process is relatively easy. In a study of immunotherapy with DC vaccination in GBM patients, 18F-FET PET imaging showed a more accurate identification ability than that of contrast-enhanced MRI initially (38). Although this study had several limitations such as a small sample size, it pointed out that 18F-FET PET had a potential role in monitoring the immunotherapy efficacy of GBM. In addition, Joseph et al. speculated that the PET probe for deoxycytidine kinase (dCK) could be used to distinguish between immune inflammatory response and enhancement foci caused by other factors in contrast-enhanced MRI imaging. They applied DC vaccination and/or PD-1 mAb blockade therapy to mice with orthotopic malignant gliomas model and GBM patients, and then used dCK PET probe and contrast-enhanced MRI for imaging respectively. The ratio of MRI contrast enhancement region to PET probe uptake area (immunotherapeutic response index) was used to describe the immune inflammatory activity in tumors. Finally, it was found that the accumulation of dCK PET probe in tumors and secondary lymphoid organs increased after immunotherapy, indicating that the immunotherapeutic response could be quantified by combining dCK PET probe with MRI imaging, which could be a potential biomarker for monitoring tumor immunotherapy (39).
With the gradual development of PET/MRI, the combination of PET and MRI makes full use of the good soft tissue contrast and multi-parameter evaluation ability of MRI. Compared with PET/CT, PET/MRI has superiority in the diagnosis and characterization of several diseases (88). Researchers have found the potential of PET/MRI in evaluating therapeutic response of GBM (89, 90) and the potential benefit of F-18 fluorothymidine (FLT)–PET/MRI for the diagnosis of melanoma brain metastasis and treatment monitoring of targeted therapy and immunotherapy (91). The ability of PET/MRI imaging in monitoring the treatment response to immunotherapy of GBM needs to be further studied. The future of PET/MRI is bright, and any new techniques need lots of researches to prove its value in clinical application.
Application of Artificial Intelligence in Immunotherapy of Glioma
AI has developed rapidly in medical field in the past decade, especially in image identification. Many studies have reported the application of AI in diagnosis, grading, curative effect evaluation, and overall survival prediction of glioma, showing the great superiority of AI technology (92–99). Among them, radiomics is a new field that uses automatic data mining algorithm to transform a large number of image data into high-dimensional feature space. In the identification of treatment response and tumor progression in glioma, studies have investigated that the diagnostic performance of multiparameter radiomics model is better than single parameter model. The former can find more hidden information in the image data of glioma and improve the treatment of patients (100). The expression status of genes related to the prognosis of GBM can also be predicted from the features extracted from radiomics (101). Furthermore, radiogenomics, which combines imaging features with genome maps, is also helpful to find prognosis-related immune biomarkers (102). Despite the rapid development of AI, there are still some problems. Any algorithm needs to provide a large amount of high-quality data, and many researches on AI have a small amount of data, poor quality, and lack unified standards. These are the problems that need to be solved in the future.
Conclusion
Pseudoprogression is the main problem that needs to be tackled in the treatment process of GBM, and the identification of which is also essential for the follow-up treatment. However, current assessment of treatment response of immunotherapy is still in the exploratory stage and does not meet the standard of routine clinical use. Fortunately, establishing a standard imaging scheme is the key to reverse this situation. The advanced imaging techniques have been widely studied and used as a tool to evaluate the therapeutic response in GBM. A large number of studies supported that the combination of various advanced imaging techniques can improve the diagnostic accuracy, expanding our prospective to the development of multimodal imaging. As for now, however, these imaging methods need to be further verified in multicenter and large sample clinical trials to drive them to truly become powerful diagnostic tools in the future.
Author Contributions
YL and YM provided the draft, evaluated the literature, and wrote the review. MW, BS, SL, and LC revised the review. RX, FZ, and HC contributed to the review. All authors contributed to the article and approved the submitted version.
Funding
This work was supported by the Natural Science Foundation of China (Grant No. 81501462); the Chengdu International Science and Technology Cooperation Funding (Grant No. 2019-GH02-00074-HZ); the 1·3·5 Project for Disciplines of Excellence-Clinical Research Incubation Project, West China Hospital, Sichuan University; and the Functional and Molecular Imaging Key Laboratory of Sichuan Province (Grant No. 2012JO0011).
Conflict of Interest
The authors declare that the research was conducted in the absence of any commercial or financial relationships that could be construed as a potential conflict of interest.
Publisher’s Note
All claims expressed in this article are solely those of the authors and do not necessarily represent those of their affiliated organizations, or those of the publisher, the editors and the reviewers. Any product that may be evaluated in this article, or claim that may be made by its manufacturer, is not guaranteed or endorsed by the publisher.
References
1. Weller M, van den Bent M, Preusser M, Le Rhun E, Tonn JC, Minniti G, et al. Eano Guidelines on the Diagnosis and Treatment of Diffuse Gliomas of Adulthood. Nat Rev Clin Oncol (2021) 18:170–86. doi: 10.1038/s41571-020-00447-z
2. Lieberman F. Glioblastoma Update: Molecular Biology, Diagnosis, Treatment, Response Assessment, and Translational Clinical Trials. F1000Res (2017) 6:1892. doi: 10.12688/f1000research.11493.1
3. Vollmann-Zwerenz A, Leidgens V, Feliciello G, Klein CA, Hau P. Tumor Cell Invasion in Glioblastoma. Int J Mol Sci (2020) 21:1932. doi: 10.3390/ijms21061932
4. Li C, Wang S, Yan JL, Torheim T, Boonzaier NR, Sinha R, et al. Characterizing Tumor Invasiveness of Glioblastoma Using Multiparametric Magnetic Resonance Imaging. J Neurosurg (2019) 132:1465–72. doi: 10.3171/2018.12.JNS182926
5. Abbott M, Ustoyev Y. Cancer and the Immune System: The History and Background of Immunotherapy. Semin Oncol Nurs (2019) 35:150923. doi: 10.1016/j.soncn.2019.08.002
6. Watson I, Dominguez PP, Donegan E, Charles Z, Robertson J, Adam EJ. Nice Guidance on Pembrolizumab for Advanced Melanoma. Lancet Oncol (2016) 17:21–2. doi: 10.1016/S1470-2045(15)00547-1
7. Herbst RS, Baas P, Kim DW, Felip E, Pérez-Gracia JL, Han JY, et al. Pembrolizumab Versus Docetaxel for Previously Treated, Pd-L1-Positive, Advanced Non-Small-Cell Lung Cancer (Keynote-010): A Randomised Controlled Trial. Lancet (2016) 387:1540–50. doi: 10.1016/S0140-6736(15)01281-7
8. Obermeier B, Verma A, Ransohoff RM. The Blood-Brain Barrier. Handb Clin Neurol (2016) 133:39–59. doi: 10.1016/B978-0-444-63432-0.00003-7
9. Louveau A, Harris TH, Kipnis J. Revisiting the Mechanisms of Cns Immune Privilege. Trends Immunol (2015) 36:569–77. doi: 10.1016/j.it.2015.08.006
10. Qazi MA, Vora P, Venugopal C, Sidhu SS, Moffat J, Swanton C, et al. Intratumoral Heterogeneity: Pathways to Treatment Resistance and Relapse in Human Glioblastoma. Ann Oncol (2017) 28:1448–56. doi: 10.1093/annonc/mdx169
11. Wong AJ, Ruppert JM, Bigner SH, Grzeschik CH, Humphrey PA, Bigner DS, et al. Structural Alterations of the Epidermal Growth Factor Receptor Gene in Human Gliomas. Proc Natl Acad Sci USA (1992) 89:2965–9. doi: 10.1073/pnas.89.7.2965
12. Sampson JH, Heimberger AB, Archer GE, Aldape KD, Friedman AH, Friedman HS, et al. Immunologic Escape After Prolonged Progression-Free Survival With Epidermal Growth Factor Receptor Variant Iii Peptide Vaccination in Patients With Newly Diagnosed Glioblastoma. J Clin Oncol (2010) 28:4722–9. doi: 10.1200/JCO.2010.28.6963
13. Radbruch A, Fladt J, Kickingereder P, Wiestler B, Nowosielski M, Bäumer P, et al. Pseudoprogression in Patients With Glioblastoma: Clinical Relevance Despite Low Incidence. Neuro Oncol (2015) 17:151–9. doi: 10.1093/neuonc/nou129
14. Wen PY, Macdonald DR, Reardon DA, Cloughesy TF, Sorensen AG, Galanis E, et al. Updated Response Assessment Criteria for High-Grade Gliomas: Response Assessment in Neuro-Oncology Working Group. J Clin Oncol (2010) 28:1963–72. doi: 10.1200/JCO.2009.26.3541
15. Brandes AA, Tosoni A, Spagnolli F, Frezza G, Leonardi M, Calbucci F, et al. Disease Progression or Pseudoprogression After Concomitant Radiochemotherapy Treatment: Pitfalls in Neurooncology. Neuro Oncol (2008) 10:361–7. doi: 10.1215/15228517-2008-008
16. Okada H, Weller M, Huang R, Finocchiaro G, Gilbert MR, Wick W, et al. Immunotherapy Response Assessment in Neuro-Oncology: A Report of the Rano Working Group. Lancet Oncol (2015) 16:e534–42. doi: 10.1016/S1470-2045(15)00088-1
17. Wang H, Xu T, Huang Q, Jin W, Chen J. Immunotherapy for Malignant Glioma: Current Status and Future Directions. Trends Pharmacol Sci (2020) 41:123–38. doi: 10.1016/j.tips.2019.12.003
18. Kong Z, Wang Y, Ma W. Vaccination in the Immunotherapy of Glioblastoma. Hum Vaccin Immunother (2018) 14:255–68. doi: 10.1080/21645515.2017.1388481
19. Zhu S, Liu Y, Wang PC, Gu X, Shan L. Recombinant Immunotoxin Therapy of Glioblastoma: Smart Design, Key Findings, and Specific Challenges. BioMed Res Int (2017) 2017:7929286. doi: 10.1155/2017/7929286
20. Eagles ME, Nassiri F, Badhiwala JH, Suppiah S, Almenawer SA, Zadeh G, et al. Dendritic Cell Vaccines for High-Grade Gliomas. Ther Clin Risk Manag (2018) 14:1299–313. doi: 10.2147/TCRM.S135865
21. Li L, Zhu X, Qian Y, Yuan X, Ding Y, Hu D, et al. Chimeric Antigen Receptor T-Cell Therapy in Glioblastoma: Current and Future. Front Immunol (2020) 11:594271. doi: 10.3389/fimmu.2020.594271
22. Kabelitz D, Serrano R, Kouakanou L, Peters C, Kalyan S. Cancer Immunotherapy With γδ T Cells: Many Paths Ahead of Us. Cell Mol Immunol (2020) 17:925–39. doi: 10.1038/s41423-020-0504-x
23. Wo J, Zhang F, Li Z, Sun C, Zhang W, Sun G. The Role of Gamma-Delta T Cells in Diseases of the Central Nervous System. Front Immunol (2020) 11:580304. doi: 10.3389/fimmu.2020.580304
24. Capsomidis A, Benthall G, Van Acker HH, Fisher J, Kramer AM, Abeln Z, et al. Chimeric Antigen Receptor-Engineered Human Gamma Delta T Cells: Enhanced Cytotoxicity With Retention of Cross Presentation. Mol Ther (2018) 26:354–65. doi: 10.1016/j.ymthe.2017.12.001
25. Sebestyen Z, Prinz I, Déchanet-Merville J, Silva-Santos B, Kuball J. Translating Gammadelta (γδ) T Cells and Their Receptors Into Cancer Cell Therapies. Nat Rev Drug Discovery (2020) 19:169–84. doi: 10.1038/s41573-019-0038-z
26. To WC, Wood BG, Krauss JC, Strome M, Esclamado RM, Lavertu P, et al. Systemic Adoptive T-Cell Immunotherapy in Recurrent and Metastatic Carcinoma of the Head and Neck: A Phase 1 Study. Arch Otolaryngol Head Neck Surg (2000) 126:1225–31. doi: 10.1001/archotol.126.10.1225
27. Meraviglia S, Eberl M, Vermijlen D, Todaro M, Buccheri S, Cicero G, et al. In Vivo Manipulation of Vgamma9vdelta2 T Cells With Zoledronate and Low-Dose Interleukin-2 for Immunotherapy of Advanced Breast Cancer Patients. Clin Exp Immunol (2010) 161:290–7. doi: 10.1111/j.1365-2249.2010.04167.x
28. Kakimi K, Matsushita H, Murakawa T, Nakajima J. Γδ T Cell Therapy for the Treatment of Non-Small Cell Lung Cancer. Transl Lung Cancer Res (2014) 3:23–33. doi: 10.3978/j.issn.2218-6751.2013.11.01
29. Cuccarini V, Aquino D, Gioppo A, Anghileri E, Pellegatta S, Schettino C, et al. Advanced Mri Assessment During Dendritic Cell Immunotherapy Added to Standard Treatment Against Glioblastoma. J Clin Med (2019) 8:2007. doi: 10.3390/jcm8112007
30. Vrabec M, Van Cauter S, Himmelreich U, Van Gool SW, Sunaert S, De Vleeschouwer S, et al. Mr Perfusion and Diffusion Imaging in the Follow-Up of Recurrent Glioblastoma Treated With Dendritic Cell Immunotherapy: A Pilot Study. Neuroradiology (2011) 53:721–31. doi: 10.1007/s00234-010-0802-6
31. Stenberg L, Englund E, Wirestam R, Siesjö P, Salford LG, Larsson EM. Dynamic Susceptibility Contrast-Enhanced Perfusion Magnetic Resonance (Mr) Imaging Combined With Contrast-Enhanced Mr Imaging in the Follow-Up of Immunogene-Treated Glioblastoma Multiforme. Acta Radiol (2006) 47:852–61. doi: 10.1080/02841850600815341
32. Rygh CB, Wang J, Thuen M, Gras Navarro A, Huuse EM, Thorsen F, et al. Dynamic Contrast Enhanced Mri Detects Early Response to Adoptive Nk Cellular Immunotherapy Targeting the Ng2 Proteoglycan in a Rat Model of Glioblastoma. PloS One (2014) 9:e108414. doi: 10.1371/journal.pone.0108414
33. Song J, Kadaba P, Kravitz A, Hormigo A, Friedman J, Belani P, et al. Multiparametric Mri for Early Identification of Therapeutic Response in Recurrent Glioblastoma Treated With Immune Checkpoint Inhibitors. Neuro Oncol (2020) 22:1658–66. doi: 10.1093/neuonc/noaa066
34. Ceschin R, Kurland BF, Abberbock SR, Ellingson BM, Okada H, Jakacki RI, et al. Parametric Response Mapping of Apparent Diffusion Coefficient as an Imaging Biomarker to Distinguish Pseudoprogression From True Tumor Progression in Peptide-Based Vaccine Therapy for Pediatric Diffuse Intrinsic Pontine Glioma. AJNR Am J Neuroradiol (2015) 36:2170–6. doi: 10.3174/ajnr.A4428
35. Qin L, Li X, Stroiney A, Qu J, Helgager J, Reardon DA, et al. Advanced Mri Assessment to Predict Benefit of Anti-Programmed Cell Death 1 Protein Immunotherapy Response in Patients With Recurrent Glioblastoma. Neuroradiology (2017) 59:135–45. doi: 10.1007/s00234-016-1769-8
36. Daghighi S, Bahrami N, Tom WJ, Coley N, Seibert TM, Hattangadi-Gluth JA, et al. Restriction Spectrum Imaging Differentiates True Tumor Progression From Immune-Mediated Pseudoprogression: Case Report of a Patient With Glioblastoma. Front Oncol (2020) 10:24. doi: 10.3389/fonc.2020.00024
37. Floeth FW, Wittsack HJ, Engelbrecht V, Weber F. Comparative Follow-Up of Enhancement Phenomena With Mri and Proton Mr Spectroscopic Imaging After Intralesional Immunotherapy in Glioblastoma–Report of Two Exceptional Cases. Zentralbl Neurochir (2002) 63:23–8. doi: 10.1055/s-2002-31579
38. Kristin Schmitz A, Sorg RV, Stoffels G, Grauer OM, Galldiks N, Steiger HJ, et al. Diagnostic Impact of Additional O-(2-[18f]Fluoroethyl)-L-Tyrosine ((18)F-Fet) Pet Following Immunotherapy With Dendritic Cell Vaccination in Glioblastoma Patients. Br J Neurosurg (2019) 13:1–7. doi: 10.1080/02688697.2019.1639615
39. Antonios JP, Soto H, Everson RG, Moughon DL, Wang AC, Orpilla J, et al. Detection of Immune Responses After Immunotherapy in Glioblastoma Using Pet and Mri. Proc Natl Acad Sci USA (2017) 114:10220–5. doi: 10.1073/pnas.1706689114
40. Hu LS, Eschbacher JM, Heiserman JE, Dueck AC, Shapiro WR, Liu S, et al. Reevaluating the Imaging Definition of Tumor Progression: Perfusion Mri Quantifies Recurrent Glioblastoma Tumor Fraction, Pseudoprogression, and Radiation Necrosis to Predict Survival. Neuro Oncol (2012) 14:919–30. doi: 10.1093/neuonc/nos112
41. Geer CP, Simonds J, Anvery A, Chen MY, Burdette JH, Zapadka ME, et al. Does Mr Perfusion Imaging Impact Management Decisions for Patients With Brain Tumors? A Prospective Study. AJNR Am J Neuroradiol (2012) 33:556–62. doi: 10.3174/ajnr.A2811
42. Kim HS, Goh MJ, Kim N, Choi CG, Kim SJ, Kim JH. Which Combination of Mr Imaging Modalities is Best for Predicting Recurrent Glioblastoma? Study of Diagnostic Accuracy and Reproducibility. Radiology (2014) 273:831–43. doi: 10.1148/radiol.14132868
43. van Dijken BRJ, van Laar PJ, Holtman GA, van der Hoorn A. Diagnostic Accuracy of Magnetic Resonance Imaging Techniques for Treatment Response Evaluation in Patients With High-Grade Glioma, a Systematic Review and Meta-Analysis. Eur Radiol (2017) 27:4129–44. doi: 10.1007/s00330-017-4789-9
44. Manning P, Daghighi S, Rajaratnam MK, Parthiban S, Bahrami N, Dale AM, et al. Differentiation of Progressive Disease From Pseudoprogression Using 3d Pcasl and Dsc Perfusion Mri in Patients With Glioblastoma. J Neurooncol (2020) 147:681–90. doi: 10.1007/s11060-020-03475-y
45. Xu Q, Liu Q, Ge H, Ge X, Wu J, Qu J, et al. Tumor Recurrence Versus Treatment Effects in Glioma: A Comparative Study of Three Dimensional Pseudo-Continuous Arterial Spin Labeling and Dynamic Susceptibility Contrast Imaging. Med (Baltimore) (2017) 96:e9332. doi: 10.1097/MD.0000000000009332
46. Paulson ES, Schmainda KM. Comparison of Dynamic Susceptibility-Weighted Contrast-Enhanced Mr Methods: Recommendations for Measuring Relative Cerebral Blood Volume in Brain Tumors. Radiology (2008) 249:601–13. doi: 10.1148/radiol.2492071659
47. Tofts PS, Brix G, Buckley DL, Evelhoch JL, Henderson E, Knopp MV, et al. Estimating Kinetic Parameters From Dynamic Contrast-Enhanced T(1)-Weighted Mri of a Diffusable Tracer: Standardized Quantities and Symbols. J Magn Reson Imaging (1999) 10:223–32. doi: 10.1002/(sici)1522-2586(199909)10:3<223::aid-jmri2>3.0.co;2-s
48. Tofts PS, Kermode AG. Measurement of the Blood-Brain Barrier Permeability and Leakage Space Using Dynamic Mr Imaging. 1. Fundamental Concepts. Magn Reson Med (1991) 17:357–67. doi: 10.1002/mrm.1910170208
49. Chen J, Xia J, Zhou YC, Xia LM, Zhu WZ, Zou ML, et al. Correlation Between Magnetic Resonance Diffusion Weighted Imaging and Cell Density in Astrocytoma. Zhonghua Zhong Liu Za Zhi (2005) 27:309–11. doi: 10.3760/j.issn:0253-3766.2005.05.016
50. Sugahara T, Korogi Y, Kochi M, Ikushima I, Shigematu Y, Hirai T, et al. Usefulness of Diffusion-Weighted Mri With Echo-Planar Technique in the Evaluation of Cellularity in Gliomas. J Magn Reson Imaging (1999) 9:53–60. doi: 10.1002/(sici)1522-2586(199901)9:1<53::aid-jmri7>3.0.co;2-2
51. Hilario A, Ramos A, Perez-Nuñez A, Salvador E, Millan JM, Lagares A, et al. The Added Value of Apparent Diffusion Coefficient to Cerebral Blood Volume in the Preoperative Grading of Diffuse Gliomas. AJNR Am J Neuroradiol (2012) 33:701–7. doi: 10.3174/ajnr.A2846
52. Wang S, Kim S, Chawla S, Wolf RL, Zhang WG, O’Rourke DM, et al. Differentiation Between Glioblastomas and Solitary Brain Metastases Using Diffusion Tensor Imaging. Neuroimage (2009) 44:653–60. doi: 10.1016/j.neuroimage.2008.09.027
53. Tomura N, Narita K, Izumi J, Suzuki A, Anbai A, Otani T, et al. Diffusion Changes in a Tumor and Peritumoral Tissue After Stereotactic Irradiation for Brain Tumors: Possible Prediction of Treatment Response. J Comput Assist Tomogr (2006) 30:496–500. doi: 10.1097/00004728-200605000-00024
54. Schmainda KM. Diffusion-Weighted Mri as a Biomarker for Treatment Response in Glioma. CNS Oncol (2012) 1:169–80. doi: 10.2217/cns.12.25
55. Yu Y, Ma Y, Sun M, Jiang W, Yuan T, Tong D. Meta-Analysis of the Diagnostic Performance of Diffusion Magnetic Resonance Imaging With Apparent Diffusion Coefficient Measurements for Differentiating Glioma Recurrence From Pseudoprogression. Med (Baltimore) (2020) 99:e20270. doi: 10.1097/MD.0000000000020270
56. Song YS, Choi SH, Park CK, Yi KS, Lee WJ, Yun TJ, et al. True Progression Versus Pseudoprogression in the Treatment of Glioblastomas: A Comparison Study of Normalized Cerebral Blood Volume and Apparent Diffusion Coefficient by Histogram Analysis. Korean J Radiol (2013) 14:662–72. doi: 10.3348/kjr.2013.14.4.662
57. Chu HH, Choi SH, Ryoo I, Kim SC, Yeom JA, Shin H, et al. Differentiation of True Progression From Pseudoprogression in Glioblastoma Treated With Radiation Therapy and Concomitant Temozolomide: Comparison Study of Standard and High-B-Value Diffusion-Weighted Imaging. Radiology (2013) 269:831–40. doi: 10.1148/radiol.13122024
58. Wang S, Martinez-Lage M, Sakai Y, Chawla S, Kim SG, Alonso-Basanta M, et al. Differentiating Tumor Progression From Pseudoprogression in Patients With Glioblastomas Using Diffusion Tensor Imaging and Dynamic Susceptibility Contrast Mri. AJNR Am J Neuroradiol (2016) 37:28–36. doi: 10.3174/ajnr.A4474
59. Zakaria R, Platt-Higgins A, Rathi N, Radon M, Das S, Das K, et al. T-Cell Densities in Brain Metastases are Associated With Patient Survival Times and Diffusion Tensor Mri Changes. Cancer Res (2018) 78:610–6. doi: 10.1158/0008-5472.CAN-17-1720
60. Le Bihan D, Breton E, Lallemand D, Grenier P, Cabanis E, Laval-Jeantet M. Mr Imaging of Intravoxel Incoherent Motions: Application to Diffusion and Perfusion in Neurologic Disorders. Radiology (1986) 161:401–7. doi: 10.1148/radiology.161.2.3763909
61. Hu YC, Yan LF, Wu L, Du P, Chen BY, Wang L, et al. Intravoxel Incoherent Motion Diffusion-Weighted Mr Imaging of Gliomas: Efficacy in Preoperative Grading. Sci Rep (2014) 4:7208. doi: 10.1038/srep07208
62. Kim HS, Suh CH, Kim N, Choi CG, Kim SJ. Histogram Analysis of Intravoxel Incoherent Motion for Differentiating Recurrent Tumor From Treatment Effect in Patients With Glioblastoma: Initial Clinical Experience. AJNR Am J Neuroradiol (2014) 35:490–7. doi: 10.3174/ajnr.A3719
63. Liu ZC, Yan LF, Hu YC, Sun YZ, Tian Q, Nan HY, et al. Combination of Ivim-Dwi and 3d-Asl for Differentiating True Progression From Pseudoprogression of Glioblastoma Multiforme After Concurrent Chemoradiotherapy: Study Protocol of a Prospective Diagnostic Trial. BMC Med Imaging (2017) 17:10. doi: 10.1186/s12880-017-0183-y
64. White NS, McDonald C, Farid N, Kuperman J, Karow D, Schenker-Ahmed NM, et al. Diffusion-Weighted Imaging in Cancer: Physical Foundations and Applications of Restriction Spectrum Imaging. Cancer Res (2014) 74:4638–52. doi: 10.1158/0008-5472.CAN-13-3534
65. White NS, McDonald CR, Farid N, Kuperman JM, Kesari S, Dale AM. Improved Conspicuity and Delineation of High-Grade Primary and Metastatic Brain Tumors Using “Restriction Spectrum Imaging”: Quantitative Comparison With High B-Value Dwi and Adc. AJNR Am J Neuroradiol (2013) 34:958–64, S1. doi: 10.3174/ajnr.A3327
66. Kothari P, White NS, Farid N, Chung R, Kuperman JM, Girard HM, et al. Longitudinal Restriction Spectrum Imaging is Resistant to Pseudoresponse in Patients With High-Grade Gliomas Treated With Bevacizumab. AJNR Am J Neuroradiol (2013) 34:1752–7. doi: 10.3174/ajnr.A3506
67. McDonald CR, White NS, Farid N, Lai G, Kuperman JM, Bartsch H, et al. Recovery of White Matter Tracts in Regions of Peritumoral Flair Hyperintensity With Use of Restriction Spectrum Imaging. AJNR Am J Neuroradiol (2013) 34:1157–63. doi: 10.3174/ajnr.A3372
68. Zhou J, Lal B, Wilson DA, Laterra J, van Zijl PC. Amide Proton Transfer (Apt) Contrast for Imaging of Brain Tumors. Magn Reson Med (2003) 50:1120–6. doi: 10.1002/mrm.10651
69. Sun PZ, Cheung JS, Wang E, Lo EH. Association Between Ph-Weighted Endogenous Amide Proton Chemical Exchange Saturation Transfer Mri and Tissue Lactic Acidosis During Acute Ischemic Stroke. J Cereb Blood Flow Metab (2011) 31:1743–50. doi: 10.1038/jcbfm.2011.23
70. Tietze A, Blicher J, Mikkelsen IK, Østergaard L, Strother MK, Smith SA, et al. Assessment of Ischemic Penumbra in Patients With Hyperacute Stroke Using Amide Proton Transfer (Apt) Chemical Exchange Saturation Transfer (Cest) Mri. NMR BioMed (2014) 27:163–74. doi: 10.1002/nbm.3048
71. Song G, Li C, Luo X, Zhao X, Zhang S, Zhang Y, et al. Evolution of Cerebral Ischemia Assessed by Amide Proton Transfer-Weighted Mri. Front Neurol (2017) 8:67. doi: 10.3389/fneur.2017.00067
72. Wang R, Li SY, Chen M, Zhou JY, Peng DT, Zhang C, et al. Amide Proton Transfer Magnetic Resonance Imaging of Alzheimer’s Disease at 3.0 Tesla: A Preliminary Study. Chin Med J (Engl) (2015) 128:615–9. doi: 10.4103/0366-6999.151658
73. Li C, Wang R, Chen H, Su W, Li S, Zhao X, et al. Chemical Exchange Saturation Transfer Mr Imaging is Superior to Diffusion-Tensor Imaging in the Diagnosis and Severity Evaluation of Parkinson’s Disease: A Study on Substantia Nigra and Striatum. Front Aging Neurosci (2015) 7:198. doi: 10.3389/fnagi.2015.00198
74. Togao O, Yoshiura T, Keupp J, Hiwatashi A, Yamashita K, Kikuchi K, et al. Amide Proton Transfer Imaging of Adult Diffuse Gliomas: Correlation With Histopathological Grades. Neuro Oncol (2014) 16:441–8. doi: 10.1093/neuonc/not158
75. Choi YS, Ahn SS, Lee SK, Chang JH, Kang SG, Kim SH, et al. Amide Proton Transfer Imaging to Discriminate Between Low- and High-Grade Gliomas: Added Value to Apparent Diffusion Coefficient and Relative Cerebral Blood Volume. Eur Radiol (2017) 27:3181–9. doi: 10.1007/s00330-017-4732-0
76. Ma B, Blakeley JO, Hong X, Zhang H, Jiang S, Blair L, et al. Applying Amide Proton Transfer-Weighted Mri to Distinguish Pseudoprogression From True Progression in Malignant Gliomas. J Magn Reson Imaging (2016) 44:456–62. doi: 10.1002/jmri.25159
77. Jiang S, Rui Q, Wang Y, Heo HY, Zou T, Yu H, et al. Discriminating Mgmt Promoter Methylation Status in Patients With Glioblastoma Employing Amide Proton Transfer-Weighted Mri Metrics. Eur Radiol (2018) 28:2115–23. doi: 10.1007/s00330-017-5182-4
78. Oz G, Alger JR, Barker PB, Bartha R, Bizzi A, Boesch C, et al. Clinical Proton Mr Spectroscopy in Central Nervous System Disorders. Radiology (2014) 270:658–79. doi: 10.1148/radiol.13130531
79. Schlemmer HP, Bachert P, Herfarth KK, Zuna I, Debus J, van Kaick G. Proton Mr Spectroscopic Evaluation of Suspicious Brain Lesions After Stereotactic Radiotherapy. AJNR Am J Neuroradiol (2001) 22:1316–24.
80. Dowling C, Bollen AW, Noworolski SM, McDermott MW, Barbaro NM, Day MR, et al. Preoperative Proton Mr Spectroscopic Imaging of Brain Tumors: Correlation With Histopathologic Analysis of Resection Specimens. AJNR Am J Neuroradiol (2001) 22:604–12.
81. Rabinov JD, Lee PL, Barker FG, Louis DN, Harsh GR, Cosgrove GR, et al. In Vivo 3-T Mr Spectroscopy in the Distinction of Recurrent Glioma Versus Radiation Effects: Initial Experience. Radiology (2002) 225:871–9. doi: 10.1148/radiol.2253010997
82. Zeng QS, Li CF, Zhang K, Liu H, Kang XS, Zhen JH. Multivoxel 3d Proton Mr Spectroscopy in the Distinction of Recurrent Glioma From Radiation Injury. J Neurooncol (2007) 84:63–9. doi: 10.1007/s11060-007-9341-3
83. Kubota R, Yamada S, Kubota K, Ishiwata K, Tamahashi N, Ido T. Intratumoral Distribution of Fluorine-18-Fluorodeoxyglucose In Vivo: High Accumulation in Macrophages and Granulation Tissues Studied by Microautoradiography. J Nucl Med (1992) 33:1972–80.
84. Kim YH, Oh SW, Lim YJ, Park CK, Lee SH, Kang KW, et al. Differentiating Radiation Necrosis From Tumor Recurrence in High-Grade Gliomas: Assessing the Efficacy of 18f-Fdg Pet, 11c-Methionine Pet and Perfusion Mri. Clin Neurol Neurosurg (2010) 112:758–65. doi: 10.1016/j.clineuro.2010.06.005
85. Gómez-Río M, Rodríguez-Fernández A, Ramos-Font C, López-Ramírez E, Llamas-Elvira JM. Diagnostic Accuracy of 201thallium-Spect and 18f-Fdg-Pet in the Clinical Assessment of Glioma Recurrence. Eur J Nucl Med Mol Imaging (2008) 35:966–75. doi: 10.1007/s00259-007-0661-5
86. Kato T, Shinoda J, Oka N, Miwa K, Nakayama N, Yano H, et al. Analysis of 11c-Methionine Uptake in Low-Grade Gliomas and Correlation With Proliferative Activity. AJNR Am J Neuroradiol (2008) 29:1867–71. doi: 10.3174/ajnr.A1242
87. Grosu AL, Astner ST, Riedel E, Nieder C, Wiedenmann N, Heinemann F, et al. An Interindividual Comparison of O-(2-[18f]Fluoroethyl)-L-Tyrosine (Fet)- and L-[Methyl-11c]Methionine (Met)-Pet in Patients With Brain Gliomas and Metastases. Int J Radiat Oncol Biol Phys (2011) 81:1049–58. doi: 10.1016/j.ijrobp.2010.07.002
88. Ehman EC, Johnson GB, Villanueva-Meyer JE, Cha S, Leynes AP, Larson PEZ, et al. Pet/mri: Where Might it Replace Pet/Ct? J Magn Reson Imaging (2017) 46:1247–62. doi: 10.1002/jmri.25711
89. Deuschl C, Moenninghoff C, Goericke S, Kirchner J, Köppen S, Binse I, et al. Response Assessment of Bevacizumab Therapy in Gbm With Integrated 11c-Met-Pet/Mri: A Feasibility Study. Eur J Nucl Med Mol Imaging (2017) 44:1285–95. doi: 10.1007/s00259-017-3661-0
90. Hojjati M, Badve C, Garg V, Tatsuoka C, Rogers L, Sloan A, et al. Role of Fdg-Pet/Mri, Fdg-Pet/Ct, and Dynamic Susceptibility Contrast Perfusion Mri in Differentiating Radiation Necrosis From Tumor Recurrence in Glioblastomas. J Neuroimaging (2018) 28:118–25. doi: 10.1111/jon.12460
91. Nguyen NC, Yee MK, Tuchayi AM, Kirkwood JM, Tawbi H, Mountz JM. Targeted Therapy and Immunotherapy Response Assessment With F-18 Fluorothymidine Positron-Emission Tomography/Magnetic Resonance Imaging in Melanoma Brain Metastasis: A Pilot Study. Front Oncol (2018) 8:18. doi: 10.3389/fonc.2018.00018
92. Cho HH, Lee SH, Kim J, Park H. Classification of the Glioma Grading Using Radiomics Analysis. PeerJ (2018) 6:e5982. doi: 10.7717/peerj.5982
93. Sengupta A, Ramaniharan AK, Gupta RK, Agarwal S, Singh A. Glioma Grading Using a Machine-Learning Framework Based on Optimized Features Obtained From T(1) Perfusion Mri and Volumes of Tumor Components. J Magn Reson Imaging (2019) 50:1295–306. doi: 10.1002/jmri.26704
94. Zhang B, Chang K, Ramkissoon S, Tanguturi S, Bi WL, Reardon DA, et al. Multimodal Mri Features Predict Isocitrate Dehydrogenase Genotype in High-Grade Gliomas. Neuro Oncol (2017) 19:109–17. doi: 10.1093/neuonc/now121
95. Zhou H, Chang K, Bai HX, Xiao B, Su C, Bi WL, et al. Machine Learning Reveals Multimodal Mri Patterns Predictive of Isocitrate Dehydrogenase and 1p/19q Status in Diffuse Low- and High-Grade Gliomas. J Neurooncol (2019) 142:299–307. doi: 10.1007/s11060-019-03096-0
96. Korfiatis P, Kline TL, Coufalova L, Lachance DH, Parney IF, Carter RE, et al. Mri Texture Features as Biomarkers to Predict Mgmt Methylation Status in Glioblastomas. Med Phys (2016) 43:2835–44. doi: 10.1118/1.4948668
97. Kim JY, Yoon MJ, Park JE, Choi EJ, Lee J, Kim HS. Radiomics in Peritumoral Non-Enhancing Regions: Fractional Anisotropy and Cerebral Blood Volume Improve Prediction of Local Progression and Overall Survival in Patients With Glioblastoma. Neuroradiology (2019) 61:1261–72. doi: 10.1007/s00234-019-02255-4
98. Kebir S, Khurshid Z, Gaertner FC, Essler M, Hattingen E, Fimmers R, et al. Unsupervised Consensus Cluster Analysis of [18f]-Fluoroethyl-L-Tyrosine Positron Emission Tomography Identified Textural Features for the Diagnosis of Pseudoprogression in High-Grade Glioma. Oncotarget (2017) 8:8294–304. doi: 10.18632/oncotarget.14166
99. Bacchi S, Zerner T, Dongas J, Asahina AT, Abou-Hamden A, Otto S, et al. Deep Learning in the Detection of High-Grade Glioma Recurrence Using Multiple Mri Sequences: A Pilot Study. J Clin Neurosci (2019) 70:11–3. doi: 10.1016/j.jocn.2019.10.003
100. Kim JY, Park JE, Jo Y, Shim WH, Nam SJ, Kim JH, et al. Incorporating Diffusion- and Perfusion-Weighted Mri Into a Radiomics Model Improves Diagnostic Performance for Pseudoprogression in Glioblastoma Patients. Neuro Oncol (2019) 21:404–14. doi: 10.1093/neuonc/noy133
101. Zinn PO, Singh SK, Kotrotsou A, Hassan I, Thomas G, Luedi MM, et al. A Coclinical Radiogenomic Validation Study: Conserved Magnetic Resonance Radiomic Appearance of Periostin-Expressing Glioblastoma in Patients and Xenograft Models. Clin Cancer Res (2018) 24:6288–99. doi: 10.1158/1078-0432.CCR-17-3420
Keywords: glioblastoma, immunotherapy, treatment response, pseudoprogression, tumor recurrence, advanced imaging
Citation: Li Y, Ma Y, Wu Z, Xie R, Zeng F, Cai H, Lui S, Song B, Chen L and Wu M (2021) Advanced Imaging Techniques for Differentiating Pseudoprogression and Tumor Recurrence After Immunotherapy for Glioblastoma. Front. Immunol. 12:790674. doi: 10.3389/fimmu.2021.790674
Received: 07 October 2021; Accepted: 08 November 2021;
Published: 25 November 2021.
Edited by:
Xiaoli Wu, Tianjin University, ChinaReviewed by:
Fan Yang, Affiliated Hospital of Southwest Medical University, ChinaBin Yu, First Affiliated Hospital of Zhengzhou University, China
Copyright © 2021 Li, Ma, Wu, Xie, Zeng, Cai, Lui, Song, Chen and Wu. This is an open-access article distributed under the terms of the Creative Commons Attribution License (CC BY). The use, distribution or reproduction in other forums is permitted, provided the original author(s) and the copyright owner(s) are credited and that the original publication in this journal is cited, in accordance with accepted academic practice. No use, distribution or reproduction is permitted which does not comply with these terms.
*Correspondence: Min Wu, wuminscu@scu.edu.cn
†These authors have contributed equally to this work