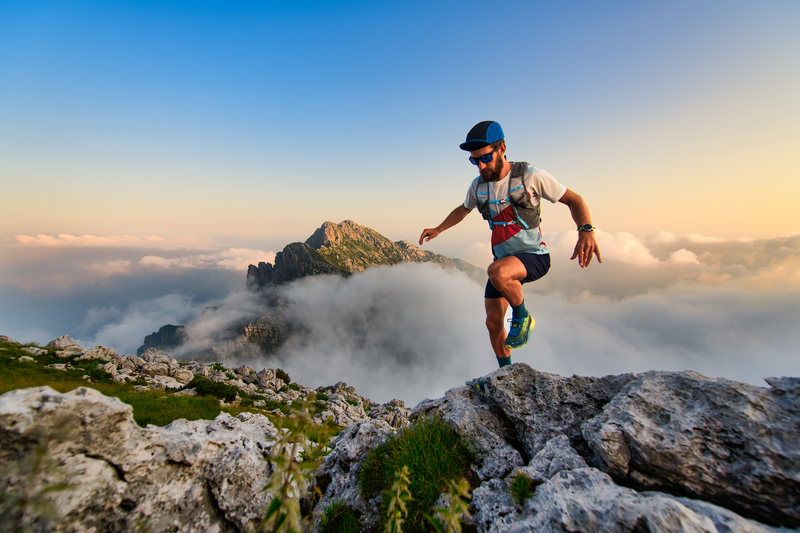
94% of researchers rate our articles as excellent or good
Learn more about the work of our research integrity team to safeguard the quality of each article we publish.
Find out more
ORIGINAL RESEARCH article
Front. Immunol. , 08 December 2021
Sec. Multiple Sclerosis and Neuroimmunology
Volume 12 - 2021 | https://doi.org/10.3389/fimmu.2021.770679
This article is part of the Research Topic Reassessing the Immune System Contribution in Multiple Sclerosis: Therapeutic Target, Biomarkers of Disease and Immune Pathogenesis View all 19 articles
Multiple sclerosis (MS) is an immune-mediated demyelinating and degenerative disease with unknown etiology. Inappropriate response of T-cells to myelin antigens has an essential role in the pathophysiology of MS. The clinical and pathophysiological complications of MS necessitate identification of potential molecular targets to understand the pathogenic events of MS. Since the functions and regulatory mechanisms of long non-coding RNAs (lncRNAs) acting as competing endogenous RNAs (ceRNAs) in MS are yet uncertain, we conducted a bioinformatics analysis to explain the lncRNA-associated ceRNA axes to clarify molecular regulatory mechanisms involved in T-cells responses in MS. Two microarray datasets of peripheral blood T-cell from subjects with relapsing-remitting MS and matched controls containing data about miRNAs (GSE43590), mRNAs and lncRNAs (GSE43591) were downloaded from the Gene Expression Omnibus database. Differentially expressed miRNAs (DEmiRNAs), mRNAs (DEmRNAs), and lncRNAs (DElncRNAs) were identified by the limma package of the R software. Protein-protein interaction (PPI) network and module were developed using the Search Tool for the Retrieval of Interacting Genes/Proteins (STRING) and the Molecular Complex Detection (MCODE) Cytoscape plugin, respectively. Using DIANA-LncBase and miRTarBase, the lncRNA-associated ceRNA axes was constructed. We conducted a Pearson correlation analysis and selected the positive correlations among the lncRNAs and mRNAs in the ceRNA axes. Lastly, DEmRNAs pathway enrichment was conducted by the Enrichr tool. A ceRNA regulatory relationship among Small nucleolar RNA host gene 1 (SNHG1), hsa-miR-197-3p, YOD1 deubiquitinase (YOD1) and zinc finger protein 101 (ZNF101) and downstream connected genes was identified. Pathway enrichment analysis showed that DEmRNAs were enriched in “Protein processing in endoplasmic reticulum” and “Herpes simplex virus 1 infection” pathways. To our knowledge, this would be the first report of a possible role of SNHG1/hsa-miR-197-3p/YOD1/ZNF101 axes in the pathogenesis of MS. This research remarks on the significance of ceRNAs and prepares new perceptions for discovering the molecular mechanism of MS.
Multiple sclerosis (MS) is the most frequent cause of non-traumatic neurological disability in young adult people (1). Estimates indicate that a total of 2.8 million individuals with MS live around the world (35.9 per 100,000 population) (2). In this degenerative disorder, the central nervous system is demyelinated through the mediation of the immune system. Yet, the main cause of MS is not known. MS presents in four clinical types: relapsing-remitting MS (RRMS), secondary progressive MS (SPMS), primary progressive MS (PPMS), and progressive relapsing MS (PRMS). RRMS is the most common subtype, which is represented by acute attacks (relapses) and then partially or fully recovered phases (remission) (3). Although the causes and etiologies underlie MS are not completely apprehended, there are proofs that it originates from multiple factors involving central and peripheral immunological tolerance mechanisms. An inappropriate T-cell response to myelin antigens is probably an important mechanism in MS pathogenesis (4). Emerging evidence indicates the potential of non-coding RNAs, especially long non-coding RNAs (lncRNAs) and the microRNAs (miRNAs), in the regulation of gene expression, providing novel prospects to understand the progression of MS (5–7). LncRNAs have major contributions to complex disorders (e.g., MS) through functioning as competing endogenous RNAs (ceRNAs) (8).
The ceRNA hypothesis suggests a cross-talk between both coding and non-coding RNAs through miRNA response elements (MREs), as miRNA complementary sequences, thereby forming a large-scale regulatory network in various parts of the transcriptome. Based on this supposition, through ceRNA regulatory mechanism, these two RNA transcripts will be indirectly correlated with miRNAs levels. Additionally, expression levels of these two RNA transcripts are positively correlated with each other (9). The consequence of disrupted balance of ceRNA cross-talk is well documented in a variety of disorders (10). Nevertheless, the roles and regulatory mechanisms of lncRNAs acting as ceRNAs in MS are still unclear.
As MS is complex in terms of both pathophysiological and clinical aspects, it is necessary to identify wide-ranging potential molecular targets to understand the pathogenic processes involved in MS. As the functions and regulatory mechanisms of lncRNAs role as ceRNAs in MS is not clear, bioinformatics analysis was done to clarify the lncRNA-associated ceRNA axes to explain molecular regulatory mechanisms involved in T-cells in MS.
In the present study, we utilized a system biology methodology for mining data of the two microarray datasets of peripheral blood T-cell (GSE43590 and GSE43591) from patients with RRMS and matched controls, which are SubSeries of the SuperSeries GSE43592 (11). We intended to identify differentially expressed miRNAs (DEmiRNAs), mRNAs (DEmRNAs), and lncRNAs (DElncRNAs) and construct lncRNA-associated ceRNA axes. Figure 1 summarizes the stages performed in the bioinformatics strategy. The study protocol was approved by Ethical Committee of Shahid Beheshti University of Medical Sciences and all methods were performed in accordance with the relevant guidelines and regulations.
The miRNA profile data GSE43590 and lncRNA/mRNA profile data GSE43591 were obtained from the NCBI Gene Expression Omnibus database (GEO, https://www.ncbi.nlm.nih.gov/geo/). The platforms GPL14613 (miRNA-2) Affymetrix Multispecies miRNA-2 Array and GPL570 (HG-U133_Plus_2) Affymetrix Human Genome U133 Plus 2.0 Array were applied for GSE43590 and GSE43591 datasets, respectively. The GSE43590 included 11 peripheral blood samples from RRMS patients and nine from control subjects. The GSE43591 contained 20 peripheral blood samples, of which ten were from RRMS patients, and ten were served as controls.
Two datasets were analyzed separately. The Robust Multichip Average (RMA) was employed for background correction and quantile normalization of the entire raw data files (12). The quality was assessed by the AgiMicroRna Bioconductor package (version 2.40.0). The principal component analysis (PCA) was applied for a dimensional reduction analysis (13) to find similarities between each sample group by the ggplot2 package in R software version 4.0.3. Differential gene expression analysis (DGEA) was performed between RRMS and normal samples by the linear models for microarray data (limma) R package (14) in Bioconductor (https://www.bioconductor.org/) (15). The miRNAmeConverter Bioconductor package (16) was used to convert all miRNA names to miRBase v22. The previously applied methodology wasfor QA employed to detect lncRNA probes (17). We downloaded the full list of lncRNA genes with the approved HUGO Gene Nomenclature Committee (HGNC) symbols from (https://www.genenames.org/) (18). Then, we compared the lncRNA gene list with our dataset gene symbols and chose the overlapped genes. Student t-test was utilized to detect statistically significant genes and the aberrantly expressed RNAs cut-off was set as: (1) a false discovery rate (adjusted P-value) < 0.05, and (2) |log2 fold change (log2FC)| ≥ 0.585. The heat map of DEmiRNAs and volcano plot of DEmRNAs/DElncRNAs were drawn using the Pheatmap (version 1.0.12) and ggplot2 packages of R.
PPIs were identified amongst the DEmRNAs using the Search Tool for the Retrieval of Interacting Genes/Proteins (STRING, https://string-db.org/) (19). For PPI network construction, a combined score of 0.4 (medium confidence) was selected. The PPI network was depicted using Cytoscape software (version 3.8.0) (20). In addition, the Molecular Complex Detection (MCODE) cytoscape plugin (version 2.0.0) was used to select most significant module in the PPI network (21). The experimentally validated interactions between miRNAs and lncRNAs were identified using DIANA-LncBase v3 (22). Homo Sapiens “Species” and high “miRNA Confidence Levels” were selected as criteria for the DIANA-LncBase query. Furthermore, we acquired the interactions between miRNAs and target mRNAs from miRTarBase (23), which were supported by experimental evidence. Next, a comparison was made between the obtained mRNAs and the previously attained mRNAs. Duplicated mRNAs were then utilized for constructing the lncRNA-miRNA-mRNA axes. LncRNAs, targeted mRNAs, and the interacted miRNAs were retrieved from the ceRNA axes based on the observed opposite expression pattern between the target mRNAs and lncRNAs. The ceRNA regulatory axes were generated by the Cytoscape software.
We performed Pearson correlation analysis to find positive correlations between lncRNAs and mRNAs that were in the ceRNA axes. We used Hmisc (version 4.5.0) and psych (version 2.1.3) packages for the calculation of the correlations and visualization.
The KEGG pathway enrichment analysis was done by the Enrichr tool (24, 25) for pathway enrichment for analyzing the DEmRNAs existing in the ceRNA axes.
Background correction and normalization were performed prior to DGEA. The AgiMicroRna Bioconductor package was employed for controlling the quality of data. The spatial distribution of samples was demonstrated by a PCA plot (Supplementary File S1), which represents information concerning the structure of the examined data, and is helpful in finding similarities between samples. PCA showed that the samples were heterogeneous. For noise reduction, few samples [GSM1065996, GSM1065997 (two control samples), and GSM1066022 (a patient sample)] were excluded from the analysis. As it is necessary to balance between noise reduction and sample size drop, only the most obvious outliers were removed. Three samples were excluded from further analysis: two control samples from GSE43590 and a RRMS sample from GSE43591.
Based on the criteria of adjusted P-value < 0.05, and (2) |log2 fold change (log2FC)| ≥ 0.585, a total of three human DEmiRNAs were identified from GSE43590, all of them being were downregulated. In the GSE43591 dataset, 19 DElncRNA (12 downregulated and seven upregulated), and 467 DEmRNA (307 downregulated and 160 upregulated) were screened. A heatmap for GSE43590 and a volcano plot for GSE43591 are shown in Figure 2. The details of DEGs are summarized in Supplementary File S1.
Figure 2 Differentially expressed genes between relapsing-remitting multiple sclerosis (RRMS) samples and control (CTL) samples. (A) Heatmap of the DEmiRNAs in dataset GSE43590. The normalized relative expression values are in a range between zero and five. High expressed genes are shown in red, while those expressed at low levels are blue. (B) Volcano plot for the DEmRNAs and DElncRNAs in dataset GSE43591. The x-axis shows the log Fold Change, and the y-axis shows the -log10 (adjusted P-value). Upregulated and downregulated genes are represented by red and blue dots, respectively. The gray dots represent genes with no significant difference. The DEGs were screened according to a |(log2FC)≥ 0.585 and an adjusted P-value < 0.05.
Protein interactions amongst DEmRNAs were identified (cutoff score ≥ 0.4) using the online STRING tool. We eliminated non-interacting genes from the PPI network to simplify it. Furthermore, the highly connected module was detected by plugin MCODE. To elucidate the regulatory mechanism in T-cells in RRMS, we made regulatory ceRNA axes based on DElncRNA-DEmiRNA and DEmiRNA-DEmRNA interactions which were obtained from DIANA-LncBase and mirTarBase, respectively. DELncRNAs, targeted DEmRNAs, and also the interacted DEmiRNAs were deleted from the ceRNA axes in the opposite expression pattern present between DElncRNAs and the targeted DEmRNAs. In total, one key lncRNA (SNHG1: small nucleolar RNA host gene 1), one key miRNA (hsa-miR-197-3p), and two key mRNAs (YOD1: YOD1 deubiquitinase and ZNF101: zinc finger protein 101) were identified. The ceRNA axes in T-cell in MS and the downstream connected genes are depicted in Figure 3.
Figure 3 The long non-coding RNA-associated competing endogenous RNA (ceRNA) axes in T-cell in Multiple sclerosis. The red and blue nodes represent the upregulation and downregulation, respectively. The hexagon nodes and the round rectangle nodes represent the lncRNA and miRNA, respectively. The diamond nodes represent miRNAs targeted mRNAs. The ellipse nodes represent downstream connected mRNAs.
The Pearson correlation analysis between lncRNA SNHG1 and target mRNAs (YOD1 and ZNF101) was conducted for the verification of the hypothesis that in the ceRNA axes, mRNA expression is positively regulated by lncRNA through interaction with miRNA (Figure 4). The results showed that expression of SNHG1 is positively correlated with YOD1 (r = 0.87, P < 0.001) and ZNF101 (r = 0.86, P < 0.001).
Figure 4 The distribution of each variable is shown on the diagonal. The lower portion of the diagonal shows bivariate scatter plots with a fitted line. On the upper part of the diagonal, the correlation coefficients plus the significance level as stars are displayed. *** is significant correlation at P-value < 0.001.
The results of KEGG pathway enrichment analyses for DEmRNAs that were in the ceRNA axes are presented in Figure 5. The related pathways were “Protein processing in endoplasmic reticulum” and “Herpes simplex virus 1 infection”.
Figure 5 Overall results of pathway enrichment analysis using Enrichr tool. The bar chart shows the enriched pathways, along with their corresponding P-values. Colored bars correspond to terms with significant P-values (<0.05). An asterisk (*) next to a P-value indicates the term also has a significant adjusted P-value (<0.05).
Multiple reports have shown that ceRNA regulatory axes and connected networks act actively in various developmental procedures and pathological conditions, such as tumor formation and wide-ranging brain-related disorders (26, 27). The ceRNA is expressed differently according to tissue-related, cellular, and subcellular situations. There may be a variety of ceRNAs, including lncRNAs, circRNAs, pseudogenes, as well as mRNAs, in a network. LncRNAs, one of the main types of RNAs, are detected in the ceRNA machinery and significantly contribute to cellular mechanisms in both physiological and pathological situations (28). There is currently full agreement that lncRNAs are expressed variously according to tissue, cellular types, and developmental levels. Such a specific tissue dependence, in addition to subcellular dispersions, is a clear indication of the tight regulation of lncRNAs expression (29). As denoted in the theoretic notions, the ceRNA regulatory axes connected to lncRNAs can have a critical contribution to MS pathogenicity. Up to now, research on the ceRNA axes involved in MS has been insufficient, and it is necessary to further examine the corresponding expression patterns and mechanisms in MS. In the current study, we utilized a public database to download the expression profiles of peripheral blood T-cells from RRMS patients to assess the DEmiRNAs, DElncRNAs, and DEmRNAs in RRMS and normal samples and then construct lncRNA-miRNA-mRNA regulatory axes. According to these ceRNA axes and lncRNA-mRNA co-expression relationships, we found the lncRNA-miRNA-mRNA axes consisting of one lncRNA (SNHG1), one miRNA (hsa-miR-197-3p), and two mRNAs (YOD1 and ZNF101).
A higher level of lncRNA SNHG1 was detected in patients with RRMS in comparison with the controls. SNHG1 is a recently described lncRNA involved in the development of several tumors and other types of disorders, including Alzheimer’s disease (AD) and Parkinson’s disease (PD). In line with our result, its upregulation has been seen in in vitro models of PD from neurons and microglia, mouse models, and AD in vitro models. It is involved in the pathogenesis of AD and PD through several complementary ceRNA mechanisms (27). To our knowledge, our research is the first to report the association between SNHG1 and MS; thus, the reported result should be validated by extra investigations.
Conversely, the level of hsa-miR-197-3p was lower in RRMS patients in comparison with controls. In a profiling study using microarray analysis and validation by real-time polymerase chain reaction, hsa-miR-197-3p was identified as a downregulated significant regulatory miRNA in T-cells in RRMS (11), consistent with our result.
The expression of YOD1 and ZNF101 genes was increased in RRMS cases compared with controls. YOD1 is a highly conserved deubiquitinase-like yeast ovarian tumor domain-containing protein 1 (OTU1) related to regulating the endoplasmic reticulum (ER)-associated degradation pathway. Indeed, YOD1 is reported to be involved in the ER stress response induced by the mislocalization of unfolded proteins in mammalian cells. YOD1 was shown to have elevated expression levels due to different stress conditions. Moreover, YOD1 level upregulation was reported to be induced by neurogenic proteins, causing Huntington’s disease and PD. The deubiquitinase YOD1 was proposed to contribute in the pathogenicity of neurodegenerative diseases by reducing ubiquitination of abnormal proteins and their degradation (30). Our result is in line with these findings. ZNF101 was another gene in the ceRNA axes. Zinc finger proteins, including ZNF101 interact with nucleic acids and have lots of crucial activities, particularly regulation of transcription (31). In line with our result, a previous study reported that single nucleotide polymorphism rs1064395 in neurocan (NCAN) gene is associated with the upregulated expression level of ZNF101 (32).
In the current study, KEGG pathway enrichment analysis also was conducted. The results showed that DEmRNAs that were in the ceRNA axes were enriched in “Protein processing in endoplasmic reticulum” and “Herpes simplex virus 1 infection” pathways, respectively. The unfolded protein response (UPR) happens to respond to ER stress resulting from the accretion of unfolded or misfolded proteins in the ER. The cytoprotective activities are promoted by the UPR to amend ER stress, but the influenced cells become apoptotic due to the UPR as a result of unresolved ER stress. The UPR is a central attribute of various disorders in humans, e.g., MS (33). Furthermore, an association between herpes simplex virus 1 (HSV-1) infection and demyelination has been reported in previous studies. Nonetheless, it is not certain whether HSV-1 is involved in MS etiology. Viruses, specifically HSV-1, may act as a risk factor for MS progression rather than a causative agent. This neurotropic pathogenic agent may mediate several molecular procedures (34).
There are some limitations in our study. Firstly, multiple parameters, including different methodologies, sample preparation, patient characteristics, platforms, and analyzing data, might influence the gene expression patterns. Secondly, a small sample size can cause low statistical power. Lastly, the present findings need to be validated by confirmative experimental approaches as well as re-analysis of microarray gene expression profiles.
In conclusion, lncRNA SNHG1 can serve as a ceRNA to regulate the expression of YOD1 and ZNF101 and downstream connected genes in T-cells in RRMS patients via sponging hsa-miR-197-3p. In our investigation, potential research targets are provided to examine molecular mechanisms that underpin the pathogenicity of MS.
The raw data supporting the conclusions of this article will be made available by the authors, without undue reservation.
MT, HS, BH, and MR wrote the manuscript and revised it. SG-F, NA, ZS, MAB, and MRA performed the bioinformatic analysis and collected the information. All authors contributed to the article and approved the submitted version.
The research protocol was approved & supported by Molecular Medicine Research Center, Tabriz University of Medical Sciences (grant number: 67290).
The authors declare that the research was conducted in the absence of any commercial or financial relationships that could be construed as a potential conflict of interest.
All claims expressed in this article are solely those of the authors and do not necessarily represent those of their affiliated organizations, or those of the publisher, the editors and the reviewers. Any product that may be evaluated in this article, or claim that may be made by its manufacturer, is not guaranteed or endorsed by the publisher.
The Supplementary Material for this article can be found online at: https://www.frontiersin.org/articles/10.3389/fimmu.2021.770679/full#supplementary-material
1. Koch-Henriksen N, Sørensen PS. The Changing Demographic Pattern of Multiple Sclerosis Epidemiology. Lancet Neurol (2010) 9(5):520–32. doi: 10.1016/S1474-4422(10)70064-8
2. Walton C, King R, Rechtman L, Kaye W, Leray E, Marrie RA, et al. Rising Prevalence of Multiple Sclerosis Worldwide: Insights From the Atlas of MS, Third Edition. Mult Scler (2020) 26(14):1816–21. doi: 10.1177/1352458520970841
3. Ghasemi N, Razavi S, Nikzad E. Multiple Sclerosis: Pathogenesis, Symptoms, Diagnoses and Cell-Based Therapy. Cell J (2017) 19(1):1–10. doi: 10.22074/cellj.2016.4867
4. Kasper LH, Shoemaker J. Multiple Sclerosis Immunology: The Healthy Immune System vs the MS Immune System. Neurology (2010) 74(Suppl 1):S2–8. doi: 10.1212/WNL.0b013e3181c97c8f
5. Yang X, Wu Y, Zhang B, Ni B. Noncoding RNAs in Multiple Sclerosis. Clin Epigenet (2018) 10(1):149. doi: 10.1186/s13148-018-0586-9
6. Sheng WH, Sheng KT, Zhao YX, Li H, Zhou JL, Yao HY, et al. Identifying the Biomarkers of Multiple Sclerosis Based on Non-Coding RNA Signature. Eur Rev Med Pharmacol Sci (2015) 19(19):3635–42.
7. Ghafouri-Fard S, Taheri M. A Comprehensive Review of Non-Coding RNAs Functions in Multiple Sclerosis. Eur J Pharmacol (2020) 879:173127. doi: 10.1016/j.ejphar.2020.173127
8. Ding Y, Li T, Yan X, Cui M, Wang C, Wang S, et al. Identification of Hub lncRNA ceRNAs in Multiple Sclerosis Based on ceRNA Mechanisms. Mol Genet Genomics (2021) 296(2):423–35. doi: 10.1007/s00438-020-01750-1
9. Salmena L, Poliseno L, Tay Y, Kats L, Pandolfi PP. A ceRNA Hypothesis: The Rosetta Stone of a Hidden RNA Language? Cell (2011) 146(3):353–8. doi: 10.1016/j.cell.2011.07.014
10. Sen R, Ghosal S, Das S, Balti S, Chakrabarti J. Competing Endogenous RNA: The Key to Posttranscriptional Regulation. ScientificWorldJournal (2014) 2014:896206. doi: 10.1155/2014/896206
11. Jernås M, Malmeström C, Axelsson M, Nookaew I, Wadenvik H, Lycke J, et al. MicroRNA Regulate Immune Pathways in T-Cells in Multiple Sclerosis (MS). BMC Immunol (2013) 14:32. doi: 10.1186/1471-2172-14-32
12. Irizarry RA, Hobbs B, Collin F, Beazer-Barclay YD, Antonellis KJ, Scherf U, et al. Exploration, Normalization, and Summaries of High Density Oligonucleotide Array Probe Level Data. Biostatistics (2003) 4(2):249–64. doi: 10.1093/biostatistics/4.2.249
13. Yeung KY, Ruzzo WL. Principal Component Analysis for Clustering Gene Expression Data. Bioinf (Oxford England) (2001) 17(9):763–74. doi: 10.1093/bioinformatics/17.9.763
14. Ritchie ME, Phipson B, Wu D, Hu Y, Law CW, Shi W, et al. Limma Powers Differential Expression Analyses for RNA-Sequencing and Microarray Studies. Nucleic Acids Res (2015) 43(7):e47. doi: 10.1093/nar/gkv007
15. Huber W, Carey VJ, Gentleman R, Anders S, Carlson M, Carvalho BS, et al. Orchestrating High-Throughput Genomic Analysis With Bioconductor. Nat Methods (2015) 12(2):115–21. doi: 10.1038/nmeth.3252
16. Haunsberger SJ, Connolly NM, Prehn JH. Mirnameconverter: An R/bioconductor Package for Translating Mature miRNA Names to Different Mirbase Versions. Bioinf (Oxford England) (2017) 33(4):592–3. doi: 10.1093/bioinformatics/btw660
17. Dashti S, Taheri M, Ghafouri-Fard S. An in-Silico Method Leads to Recognition of Hub Genes and Crucial Pathways in Survival of Patients With Breast Cancer. Sci Rep (2020) 10(1):18770. doi: 10.1038/s41598-020-76024-2
18. Braschi B, Denny P, Gray K, Jones T, Seal R, Tweedie S, et al. Genenames.org: The HGNC and VGNC Resources in 2019. Nucleic Acids Res (2019) 47(D1):D786–D92. doi: 10.1093/nar/gky930
19. Szklarczyk D, Gable AL, Lyon D, Junge A, Wyder S, Huerta-Cepas J, et al. STRING V11: Protein–Protein Association Networks With Increased Coverage, Supporting Functional Discovery in Genome-Wide Experimental Datasets. Nucleic Acids Res (2018) 47(D1):D607–13. doi: 10.1093/nar/gky1131
20. Shannon P, Markiel A, Ozier O, Baliga NS, Wang JT, Ramage D, et al. Cytoscape: A Software Environment for Integrated Models of Biomolecular Interaction Networks. Genome Res (2003) 13(11):2498–504. doi: 10.1101/gr.1239303
21. Bader GD, Hogue CWV. An Automated Method for Finding Molecular Complexes in Large Protein Interaction Networks. BMC Bioinf (2003) 4:2. doi: 10.1186/1471-2105-4-2
22. Karagkouni D, Paraskevopoulou MD, Tastsoglou S, Skoufos G, Karavangeli A, Pierros V, et al. DIANA-LncBase V3: Indexing Experimentally Supported miRNA Targets on Non-Coding Transcripts. Nucleic Acids Res (2020) 48(D1):D101–D10. doi: 10.1093/nar/gkz1036
23. Huang HY, Lin YC, Li J, Huang KY, Shrestha S, Hong HC, et al. Mirtarbase 2020: Updates to the Experimentally Validated microRNA-Target Interaction Database. Nucleic Acids Res (2020) 48(D1):D148–D54. doi: 10.1093/nar/gkz896
24. Chen EY, Tan CM, Kou Y, Duan Q, Wang Z, Meirelles GV, et al. Enrichr: Interactive and Collaborative HTML5 Gene List Enrichment Analysis Tool. BMC Bioinf (2013) 14:128. doi: 10.1186/1471-2105-14-128
25. Kuleshov MV, Jones MR, Rouillard AD, Fernandez NF, Duan Q, Wang Z, et al. Enrichr: A Comprehensive Gene Set Enrichment Analysis Web Server 2016 Update. Nucleic Acids Res (2016) 44(W1):W90–7. doi: 10.1093/nar/gkw377
26. Ala U. Competing Endogenous RNAs, Non-Coding RNAs and Diseases: An Intertwined Story. Cells (2020) 9(7):1574. doi: 10.3390/cells9071574
27. Moreno-García L, López-Royo T, Calvo AC, Toivonen JM, de la Torre M, Moreno-Martínez L, et al. Competing Endogenous RNA Networks as Biomarkers in Neurodegenerative Diseases. Int J Mol Sci (2020) 21(24):9582. doi: 10.3390/ijms21249582
28. Cai Y, Wan J. Competing Endogenous RNA Regulations in Neurodegenerative Disorders: Current Challenges and Emerging Insights. Front Mol Neurosci (2018) 11:370–. doi: 10.3389/fnmol.2018.00370
29. Gloss BS, Dinger ME. The Specificity of Long Noncoding RNA Expression. Biochim Biophys Acta (2016) 1859(1):16–22. doi: 10.1016/j.bbagrm.2015.08.005
30. Tanji K, Mori F, Miki Y, Utsumi J, Sasaki H, Kakita A, et al. YOD1 Attenuates Neurogenic Proteotoxicity Through Its Deubiquitinating Activity. Neurobiol Dis (2018) 112:14–23. doi: 10.1016/j.nbd.2018.01.006
31. Bellefroid EJ, Marine JC, Ried T, Lecocq PJ, Rivière M, Amemiya C, et al. Clustered Organization of Homologous KRAB Zinc-Finger Genes With Enhanced Expression in Human T Lymphoid Cells. EMBO J (1993) 12(4):1363–74. doi: 10.1002/j.1460-2075.1993.tb05781.x
32. Fransen NL, Crusius JBA, Smolders J, Mizee MR, van Eden CG, Luchetti S, et al. Post-Mortem Multiple Sclerosis Lesion Pathology is Influenced by Single Nucleotide Polymorphisms. Brain Pathol (2020) 30(1):106–19. doi: 10.1111/bpa.12760
33. Stone S, Lin W. The Unfolded Protein Response in Multiple Sclerosis. Front Neurosci (2015) 9:264–. doi: 10.3389/fnins.2015.00264
Keywords: bioinformatics analysis, competing endogenous RNA, long non-coding RNA, microarray, multiple sclerosis
Citation: Sabaie H, Salkhordeh Z, Asadi MR, Ghafouri-Fard S, Amirinejad N, Askarinejad Behzadi M, Hussen BM, Taheri M and Rezazadeh M (2021) Long Non-Coding RNA- Associated Competing Endogenous RNA Axes in T-Cells in Multiple Sclerosis. Front. Immunol. 12:770679. doi: 10.3389/fimmu.2021.770679
Received: 04 September 2021; Accepted: 22 November 2021;
Published: 08 December 2021.
Edited by:
Roberta Magliozzi, University of Verona, ItalyReviewed by:
Hikoaki Fukaura, Saitama Medical University, JapanCopyright © 2021 Sabaie, Salkhordeh, Asadi, Ghafouri-Fard, Amirinejad, Askarinejad Behzadi, Hussen, Taheri and Rezazadeh. This is an open-access article distributed under the terms of the Creative Commons Attribution License (CC BY). The use, distribution or reproduction in other forums is permitted, provided the original author(s) and the copyright owner(s) are credited and that the original publication in this journal is cited, in accordance with accepted academic practice. No use, distribution or reproduction is permitted which does not comply with these terms.
*Correspondence: Mohammad Taheri, TW9oYW1tYWRfODIzQHlhaG9vLmNvbQ==; Maryam Rezazadeh, UmV6YXphZGVobUB0YnptZWQuYWMuaXI=
Disclaimer: All claims expressed in this article are solely those of the authors and do not necessarily represent those of their affiliated organizations, or those of the publisher, the editors and the reviewers. Any product that may be evaluated in this article or claim that may be made by its manufacturer is not guaranteed or endorsed by the publisher.
Research integrity at Frontiers
Learn more about the work of our research integrity team to safeguard the quality of each article we publish.