- 1Department of Nephrology, Institute of Nephrology, Ruijin Hospital, Shanghai Jiao Tong University School of Medicine, Shanghai, China
- 2Department of Nephrology, The First Affiliated Hospital of Zhengzhou University, Zhengzhou, China
- 3Department of Nephrology, Sichuan Provincial People’s Hospital, Chengdu, China
- 4Department of Nephrology, The First People’s Hospital of Yunnan Province, Kunming, China
- 5Department of Nephrology, The First Affiliated Hospital of Wenzhou Medical University, Wenzhou, China
- 6Department of Nephrology, Qingdao Municipal Hospital, Qingdao, China
We aimed to validate three IgAN risk models proposed by an international collaborative study and another CKD risk model generated by an extended CKD cohort with our multicenter Chinese IgAN cohort. Biopsy-proven IgAN patients with an eGFR ≥15 ml/min/1.73 m2 at baseline and a minimum follow-up of 6 months were enrolled. The primary outcomes were a composite outcome (50% decline in eGFR or ESRD) and ESRD. The performance of those models was assessed using discrimination, calibration, and reclassification. A total of 2,300 eligible cases were enrolled. Of them, 288 (12.5%) patients reached composite outcome and 214 (9.3%) patients reached ESRD during a median follow-up period of 30 months. Using the composite outcome for analysis, the Clinical, Limited, Full, and CKD models had relatively good performance with similar C statistics (0.81, 0.81, 0.82, and 0.82, respectively). While using ESRD as the end point, the four prediction models had better performance (all C statistics > 0.9). Furthermore, subgroup analysis showed that the models containing clinical and pathological variables (Full model and Limited model) had better discriminatory abilities than the models including only clinical indicators (Clinical model and CKD model) in low-risk patients characterized by higher baseline eGFR (≥60 ml/min/1.73 m2). In conclusion, we validated recently reported IgAN and CKD risk models in our Chinese IgAN cohort. Compared to pure clinical models, adding pathological variables will increase performance in predicting ESRD in low-risk IgAN patients with baseline eGFR ≥60 ml/min/1.73 m2.
Introduction
Immunoglobulin A nephropathy (IgAN), first described by Berger in 1968, is the most common type of glomerulonephritis and an important cause of end-stage renal disease (ESRD) worldwide (1–3). Because of the heterogeneous prognostic nature of IgAN, it is important to identify high-risk patients at diagnosis not only for the selection for treatment strategies and clinical trials but also for patient health education (4–7).
In recent decades, dozens of clinical risk factors, including proteinuria, hypertension, and estimated glomerular filtration rate (eGFR), at the time of renal biopsy have been reported to be associated with worse renal prognosis in IgAN (8). In addition to clinical parameters at baseline, proteinuria or blood pressure during the first 2 years of follow-up after diagnosis also has clear correlations with the prognosis of IgAN (9). Among these risk factors, baseline eGFR was established as the most consistent indicator. An outstanding question in the field is whether combined pathological indicators, such as mesangial hypercellularity, endocapillary hypercellularity, segmental sclerosis, and interstitial fibrosis, can increase the accuracy of clinical indicators for prognosis prediction (9–11).
To date, several prediction models of IgAN progression have been established based on patients from different populations at different stages of renal function (9, 12–17). We previously established a clinical model (CLIN model) and a combined model containing both clinical and pathological variables (CLINPATH model), which had good performance in predicting the occurrence of ESRD at 10 years in the validation cohort (18). Later, a large-scale study of a combined multiethnic IgAN cohort performed by Barbour et al. established risk prediction models based on 3,927 IgAN patients (19). The clinical model in this study included proteinuria, blood pressure, and eGFR at renal biopsy. In addition to the clinical indicators, the limited model contained the MESTC histologic score, and the full model included age, medication, and racial/ethnic characteristics. The authors found that the limited model [area under the curve (AUC) = 0.80; 95% confidence interval (CI), 0.79–0.81] and full model (AUC = 0.82; 95% CI, 0.81–0.82) showed improved performance in predicting the composite outcome (defined as a 50% decline in eGFR or ESRD) compared to the clinical model (AUC = 0.78; 95% CI, 0.77–0.78). In addition, whether risk models of chronic kidney disease (CKD) can be used to predict the prognosis of IgAN is an interesting question. The study by Tangri developed and validated CKD risk models by including 8,391 Canadian CKD patients. Model 3 (C statistic, 0.91; 95% CI, 0.89–0.93), a clinical model, had good performance in predicting disease progression in patients with CKD stages 3 to 5 (20). Both studies by Barbour and Tangri studies have been further assessed and externally validated (21–23), but the prediction models would still benefit from additional external validation to improve confidence in using them in practice.
The objective of this study was to use our established multicenter Chinese IgAN cohort to conduct an independent external validation study of Barbour’s IgAN models and Tangri’s CKD model. We also compared the performance of pure clinical models (including clinical variables only) and combined models (including both clinical and pathological variables). We aimed to determine whether pathological parameters independently contribute to clinical models in predicting IgAN prognosis.
Materials and Methods
Ethics Approval and Consent to Participate
This study was performed in accordance with the Declaration of Helsinki and approved by the Ethics Research Committee of Ruijin Hospital, Medical School of Shanghai Jiaotong University. Written informed consent was collected from all participants prior to inclusion in the study.
Participants
A multicenter collaborative cohort (six nephrology centers from teaching hospitals throughout the country) was established to represent Chinese patients with IgAN. All patients were recruited from six renal centers from 1985 to 2018. The recruitment criteria for the IgAN patients included the following: (1) IgAN was defined by a renal biopsy demonstrating dominant IgA deposition in the mesangium of glomeruli by immuno-fluorescence microscopy; (2) IgAN was not secondary to systemic diseases, such as Henoch-Schoünlein purpura, systemic lupus erythematosus, and liver disease; (3) the eGFR was ≥15 ml/min/1.73 m2 at diagnosis; (4) the minimum follow-up time was 6 months; (5) the age at biopsy was more than 18 years; and (6) an informed consent form was signed.
Clinical and Pathologic Characteristics
All clinical and pathologic variables at the time of renal biopsy and during follow-up were collected. Age at biopsy, mean arterial blood pressure (MAP), serum creatinine (Scr), hemoglobin, eGFR (using the EPI equation), 24-h protein excretion, and renin–angiotensin system blocker (RASB) or glucocorticoid treatments were recorded. The severity of the renal damage was scored according to the Oxford MESTC classification (24). Three recently reported risk prediction models, including the clinical model, limited model, and full model with race/ethnicity (19), and one CKD risk prediction model (20), were used to calculate the risk of renal disease progression in individuals with IgAN.
Outcomes and Definitions
The start of follow-up time was considered the date of renal biopsy. The primary renal outcome of our study was the combined outcome (the first occurrence of either a 50% decline in eGFR from that at biopsy or ESRD). The secondary outcome was defined as ESRD (eGFR < 15 ml/min/1.73 m2 or the need for dialysis/renal transplantation). Patients were censored at the time of meeting the endpoint criterion or loss to follow-up.
Calculation of Predicted Risk and Risk Groups
To calculate the prediction risk of renal outcomes for each patient, the β coefficients from the original models of Barbour (19) and Tangri (20) were used (Supplementary Table 1). Patients were categorized into four risk groups by the percentiles of linear predictors: low risk: <16th; intermediate risk: 16th to 50th; higher risk: 50th to 84th; and highest risk: > 84th percentile (19).
Statistical Analysis
There are no reliable sample size recommendations for studies that validate prognostic models, but at least 100 events are recommended (25). Continuous data that are normally distributed or had a skewed distribution are expressed as the medians (interquartile range) or mean ± SD, respectively, and categorical data are expressed as the frequencies or percentages (%); probabilities of cumulative renal survival curves were generated by the Kaplan–Meier method. Prediction model performance was assessed using measures of model fit (Nagelkerke R2, Akaike information criterion (AIC), C statistic). Comparisons of the observed and predicted 5-year risk and 2-year risk of renal outcomes were analyzed separately. In addition, survival receiver operating characteristic (ROC) analysis was performed to evaluate the discriminatory ability of the scoring system after 5 years of follow-up. Reclassification improvement was quantified using the net reclassification improvement (NRI). Calibration refers to the agreement between observed outcomes and predictions, which was analyzed by the Hosmer–Lemeshow test in our study. Statistical analysis was performed using the ResourceSelection package (version 0.3-5), rms package (version 5.1-4), pROC package (version 1.16.1), and PredictABEL package (version 1.2-4) with the R statistical programming language (R, version 3.5.3; R Foundation for Statistical Computing, Vienna, Austria). Two-tailed p-values <.05 were considered statistically significant, except where otherwise indicated. The results are presented according to the TRIPOD guidelines for risk prediction models (Supplementary Table 2).
Results
Subject Characteristics
A total of 2,300 IgAN patients were finally enrolled based on the inclusion criteria (Figure 1). The characteristics of our cohort and the two original cohorts are summarized in Table 1. Our cohort included 1,106 males (48.1%), and the median age was 35 years (IQR, 28–44 years). The median values of baseline eGFR and 24-h proteinuria were 76.9 ml/min/1.73 m2 and 1.3 g/day, respectively. Among the included patients, 73.7% received RASB treatment and 59.8% received glucocorticoid treatment after diagnosis. During the median follow-up time of 2.5 years, 288 patients (12.5%) had a renal composite outcome, and 214 patients progressed to ESRD (9.3%).
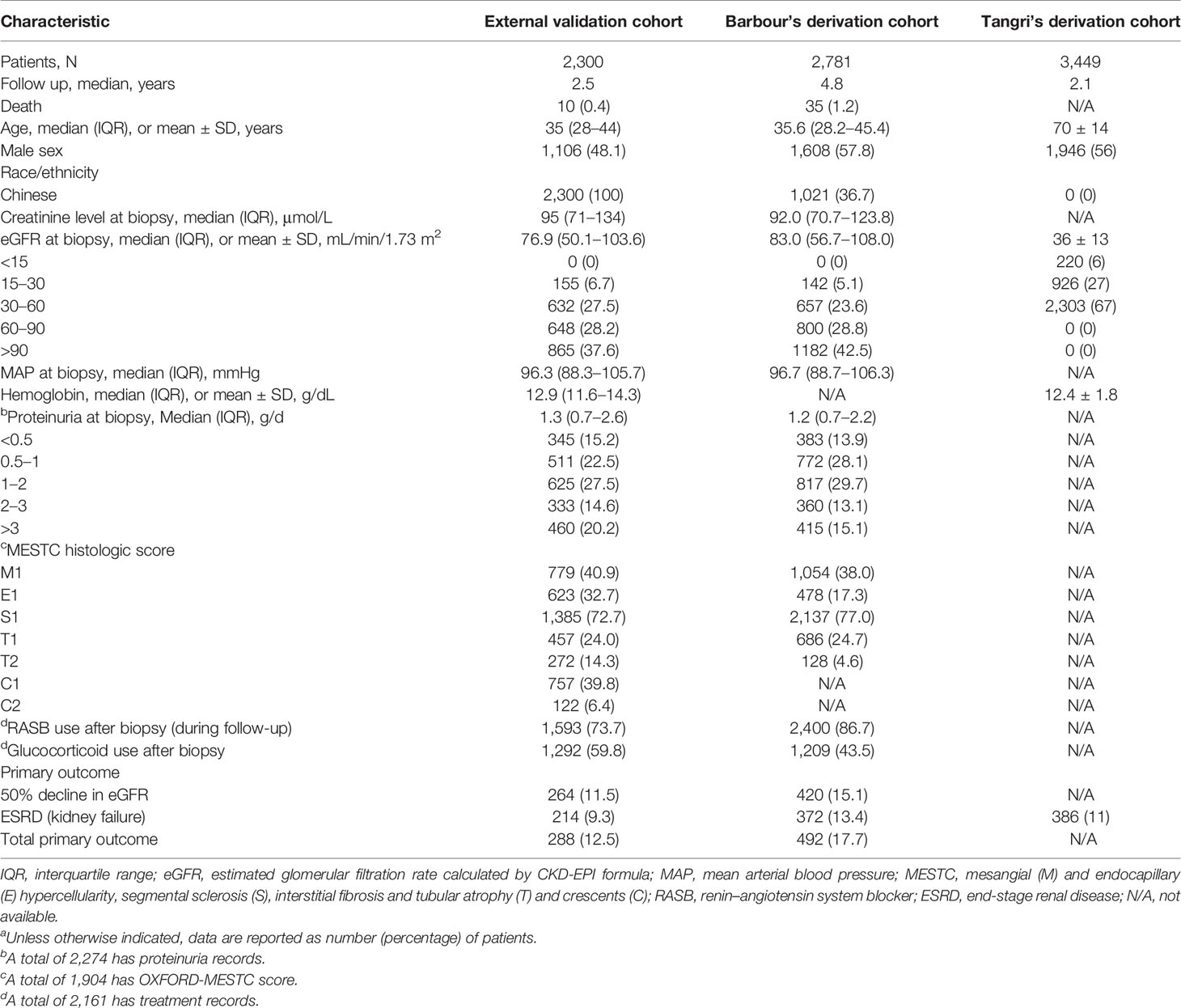
Table 1 Characteristics of patients in our external validation cohort, Barbour’s derivation cohort, and Tangri’s derivation cohort.a
Performance of the IgAN Prediction Tool in Two Renal Outcomes
The goodness of fit and statistics for discrimination for all models at 5 years after biopsy are shown in Tables 2 and 3, respectively. Using the composite outcome as an endpoint, the clinical model, including eGFR, proteinuria, and MAP, performed well (C statistic, 0.81; R2, 0.23). The C statistic and R2 were not significantly improved after adding pathological indicators in the limited model (C statistic, 0.82; R2, 0.27) or medication and other predictors in the full model (C statistic, 0.82; R2, 0.27). The AIC was also similar among the clinical, limited, and full models (712.10 and 689.33 vs. 687.68, respectively). Moreover, continuous net reclassification improvement [cNRI; full model vs. clinical model: 0.36 (95% CI, 0.18–0.55); full model vs. limited model: 0.08 (95% CI, -0.11–0.27)] indicated the significantly improved classification performance of the models that included clinical and pathological indicators compared with the models that only included clinical indicators at 5 years of follow-up (Table 3). Supplementary Figure 1 shows the mean predicted risk probability of the composite outcome against the observed risk over the follow-up period. The full model with race was calibrated well, with a mild underestimation in the low-, intermediate-, and highest-risk groups and mild overestimation in the higher-risk group.
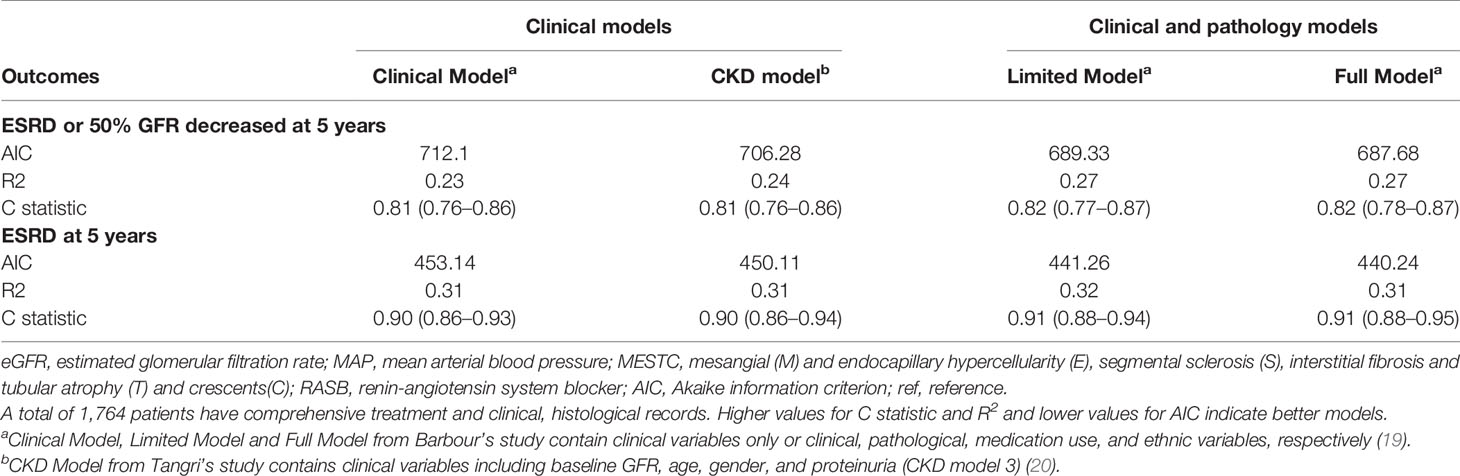
Table 2 The goodness of fit for different models predicting the composite outcome (50% GFR declined or ESRD) and ESRD at 5 years.
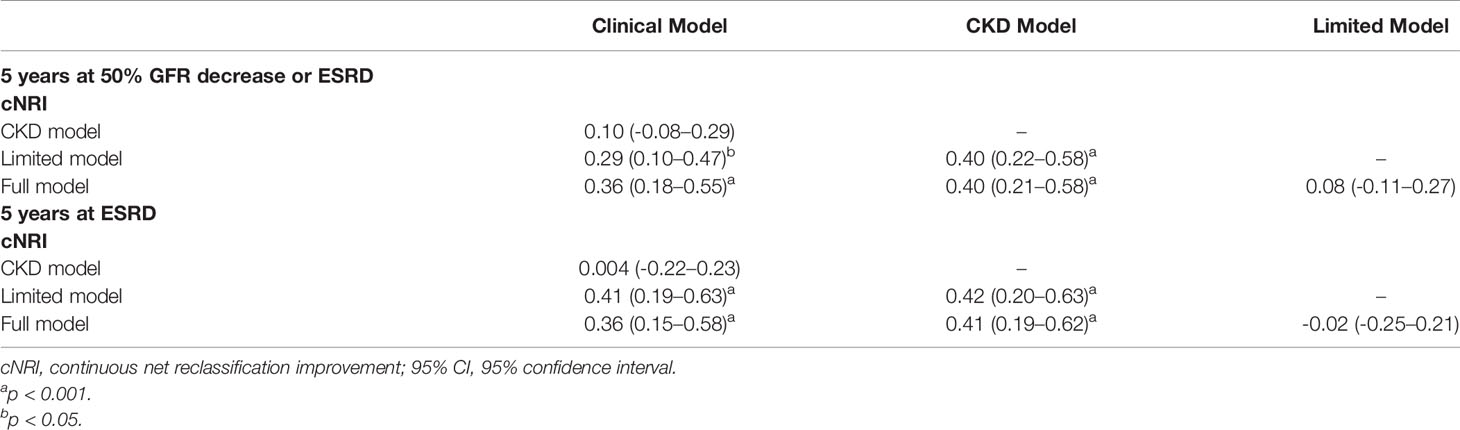
Table 3 For total group, comparison of models’ discrimination performance in the validation cohort for predicting the risk for two outcomes (ESRD or 50% GFR decreased; ESRD) at 5 years after biopsy.
Indeed, the three IgAN models used for predicting ESRD performed better (all C statistics > 0.9) than that used to predict the composite outcome (Table 2). Compared with the clinical model, the full model with race also demonstrated significant improvement in risk reclassification for predicting 5-year risk, with an NRI of 0.36 (95% CI, 0.15 to 0.58, Table 3). Overall, the three models mildly underestimated the risk within 5 years in the highest-risk group (Figure 2). In addition, we validated the performance of those models in predicting the 2-year renal outcome and found that they could also effectively predict short-term prognosis (Supplementary Tables 3 and 4).
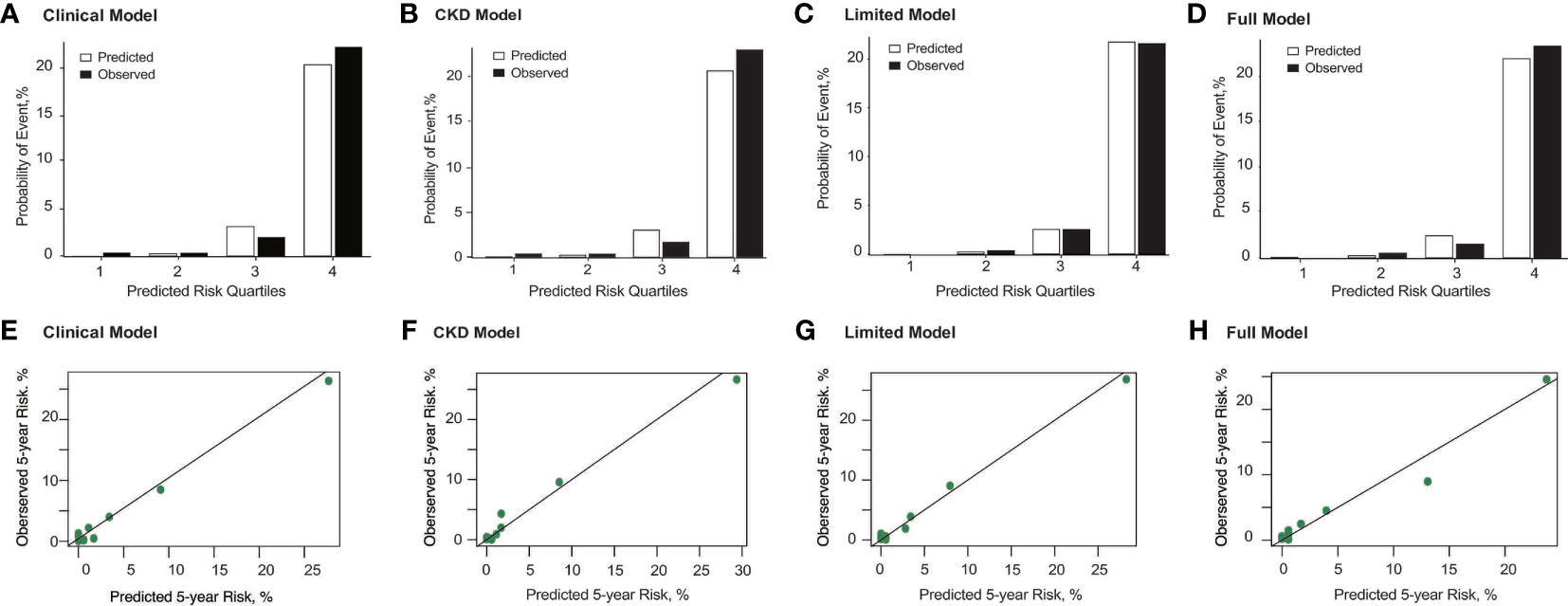
Figure 2 Observed vs. predicted probability and calibration curve of ESRD at 5 years using the Clinical Model (A, E), CKD Model (B, F), Limited Model (C, G), and Full Model (D, H). The predicted and observed event probability estimates represent the mean predicted probability from risk-prediction model and the mean observed probability from the population divided into quartiles of predicted probability. For those models, risk groups were based on the 16th (lowest risk), 16th to 50th (intermediate risk), 50th to 84th (higher risk), and higher than 84th (highest risk) percentiles of the linear predictor. The mean predicted probability (%) vs. observed probability (%) categories for quartiles 1 through 4 correspond with 0.02% vs. 0.35%, 0.25% vs. 0.33%, 3.14% vs. 2.00%, and 20.36% vs. 22.26%, respectively, for the Clinical Model; 0.03% vs. 0.35%, 0.24% vs. 0.33%, 3.01% vs. 1.67%, and 20.65% vs. 22.97%, respectively, for the CKD Model; 0.03% vs. 0.00%, 0.21% vs. 0.33%, 2.53% vs. 2.50%, and 21.74% vs. 21.55%, respectively, for the Limited Model; and 0.03% vs. 0.00%, 0.22% vs. 0.50%, 2.42% vs. 1.50%, and 21.95% vs. 23.32%, respectively, for the Full Model. In addition, the Hosmer–Lemeshow test presented that the full model with race had p value > 0.05, which means the goodness of model fit is acceptable.
Performance of the CKD Prediction Tool in Two Renal Outcomes
We next evaluated the performance of the CKD risk prediction model predicting different renal outcomes in IgAN patients given the CKD-like nature of IgAN. As a model containing only clinical indicators, CKD model 3 also had excellent performance in predicting ESRD (C statistic, 0.90; 95% CI, 0.86–0.94) and relatively good performance in predicting composite outcomes (C statistic, 0.81; 95% CI, 0.76–0.86) in our IgAN patients (Table 2). Using ESRD as the renal outcome, the R2 (0.31) and AIC (450.11) were also similar to those of the above clinical models (Table 2). The clinical models based on baseline eGFR and various other clinical parameters exhibited good performance. In addition, the difference between the observed and predictive probabilities (Figure 2) and the ROC curve (Figure 3) for predicting ESRD at 5 years in the CKD model were similar to those of the above IgAN models.
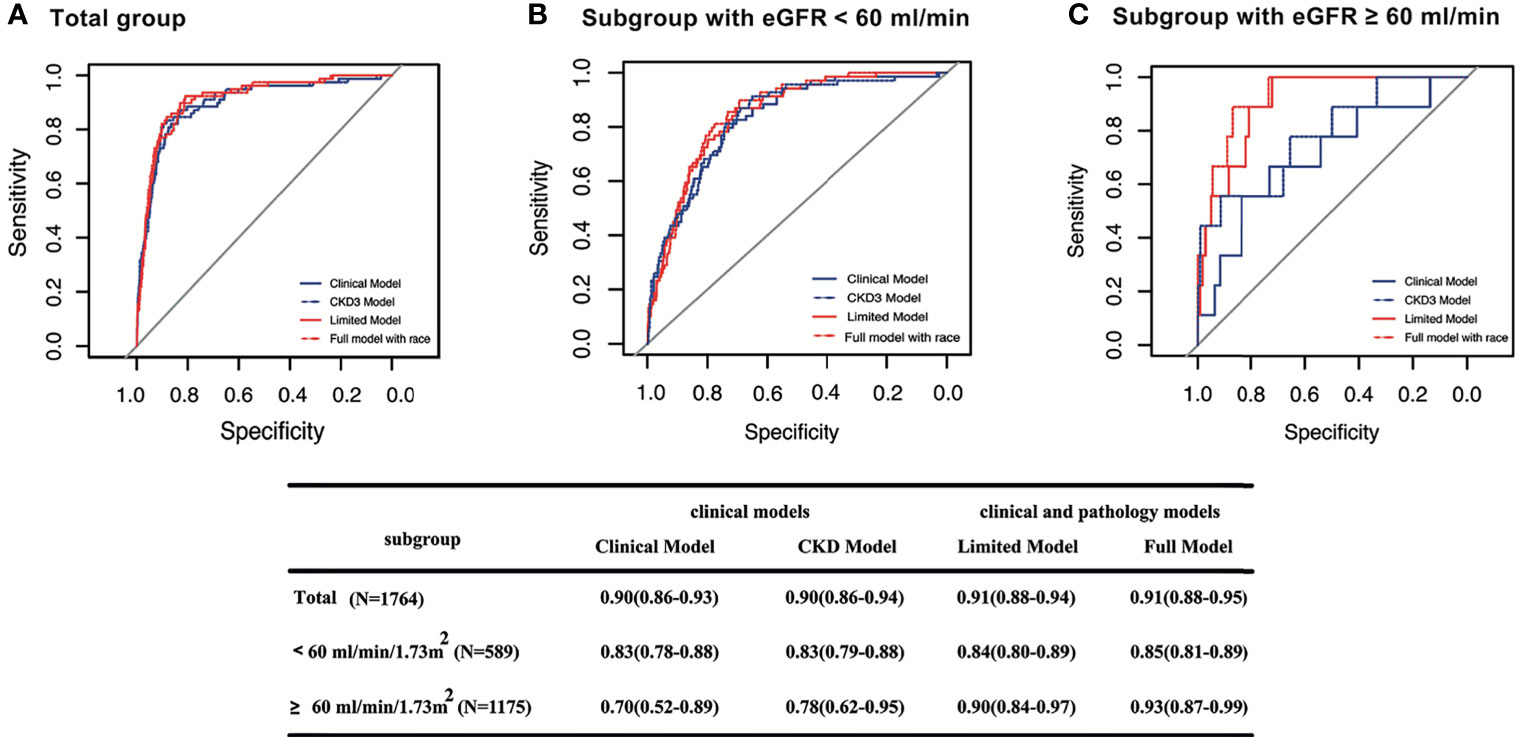
Figure 3 Survival ROC curves of four models for predicting ESRD at 5 years in different subgroups. The area the under the curve (AUC) and 95% CI of the Clinical Model, CKD Model, Limited Model, and Full Model, respectively, in total patients (A); in patients with baseline eGFR < 60 ml/min/1.73 m2 (B); in patients with baseline eGFR ≥ 60 ml/min/1.73 m2 (C).
The IgAN models and the CKD model performed better in predicting ESRD than in predicting the composite endpoint. Considering that ESRD is a robust renal outcome, it was used for further analysis.
Subgroup Analysis of the Four Models for Predicting ESRD
A subgroup analysis of the entire cohort was used to evaluate the performance of the four models in patients from different subgroups (Supplementary Figure 2). Either clinical models or clinical/pathological models had similar discriminatory abilities within subgroups defined by age, sex, proteinuria, or glucocorticoid treatment. Compared with that of the clinical models, the improved performance of the combined models after adding pathological indicators was limited, even for patients at high risk characterized by lower baseline eGFR (<60 ml/min/1.73 m2).
Comparison of Models for Predicting ESRD in Patients With Lower Risk
For patients at low risk characterized by baseline eGFR ≥60 ml/min/1.73 m2, we compared the performance of all models based on ROC analysis. The full model provided considerably improved discriminative power compared with the other three previously proposed models in predicting ESRD at 5 years of follow-up [C statistic of 0.93 (95% CI, 0.87–0.99) for the full model, 0.90 (95% CI, 0.78–0.97) for the limited model, 0.70 (95% CI, 0.52–0.89) for the clinical model, 0.78 (95% CI, 0.62–0.95) for the CKD model]. Pathological variables that added predictive value to the clinical variables were observed only in low-risk IgAN patients characterized by a baseline eGFR ≥ 60 ml/min/1.73 m2 (Figure 3).
Discussion
Clinical challenges of IgAN include accurately stratifying patients, helping clinicians to identify high-risk patients to enhance treatment, and avoiding unnecessary hormone and immunosuppressive therapies for low-risk patients. Recently, with the efforts of clinical nephrologists, multiple risk models have been established. Some models include baseline or follow-up clinical parameters, such as eGFR, proteinuria, and blood pressure, and some models add pathological parameters to the clinical models to establish combined models. These predictive models still benefit from other external validations, thereby increasing the confidence in their clinical use. In addition, whether pathological parameters, such as Oxford MEST predictors, can enhance the predictive value of clinical parameters for the prognosis of patients with IgA nephropathy remains controversial.
In this study, we assessed the performance of international IgAN prediction tools and another CKD model by external validation in a large, multicenter Chinese IgAN cohort. Relative to those in the derivation cohort of the international IgAN prediction tools, our follow-up time was shorter, and the incidence of a 50% decline in eGFR or development of ESRD was lower (12.5% versus 17.7%). Compared to the derivation cohort of the CKD model, our cohort had a lower proportion of patients with baseline eGFR < 30 ml/min/1.73 m2, and fewer patients progressed to kidney failure/ESRD (9.3% versus 11%) during the follow-up period.
Indeed, we still found that the four models performed well at predicting the 5-year risk of renal outcomes. Compared with composite outcomes, better performance of those tools used for predicting ESRD at 5 years was observed. These models also performed relatively well at predicting short-term prognosis. In addition, we found that the clinical models based on baseline eGFR and various other clinical parameters had good performance, as did the combined model that included clinical and pathological indicators. For IgAN patients at low risk characterized by higher baseline eGFR, adding pathological variables could enhance the discriminatory ability of models that contain only clinical variables. Finally, after application to patients at low risk, the full model had the best performance in predicting ESRD among the four reported models.
Among patients with IgAN, there can be considerable heterogeneity in the risk for progression to kidney failure. Risk factors associated with IgAN progression have gained increasing attention over the last two decades (26–29). The emerging literature suggests improved patient outcomes with individualized risk prediction models (30–34). The availability of these risk prediction tools has led to better adherence to treatment guidelines and encouraged individual decision making (32–34). Despite these benefits, the lack of easily applicable and externally validated models has delayed the widespread integration of risk prediction in all fields of medicine (35, 36). We confirmed that the models rely on clinical data and histological markers of IgAN severity to predict the early risk of kidney failure at 5 years. Similar to Barbour (37, 38), we also confirmed that a lower estimated eGFR, more severe proteinuria, and male gender predict faster progression to kidney failure. In addition, a higher percentage of tubular injury and segmental sclerosis also predict a higher risk of kidney failure. These markers may enable a better estimate of the underlying processes of disease (39, 40).
Considering that those laboratory and pathological markers have been associated with the progression of IgAN, risk prediction models integrate them in different combinations. Based on our data, the performances of all models according to ROC analysis were compared. The clinical models and combined models showed similar performance in predicting ESRD after 5 years of follow-up. However, for patients at low risk characterized by higher baseline eGFR (≥60 ml/min/1.73 m2), the pathological variables could add predictive value to clinical variables, likely because the contribution of pathological indicators to ESRD prediction is diminished by the subsequent use of immunosuppressive therapy in high-risk patients characterized by lower eGFR.
Risk prediction models have important implications for clinical practice, research, and public health policy. Different risk thresholds could be used to triage patients for decision-making. For example, primary care physicians could manage lower-risk patients without additional testing or treatment of complications, whereas higher-risk patients could receive more intensive testing, intervention, and early nephrology care (41). Furthermore, the risk prediction model could be used to select higher-risk patients for enrollment into clinical trials and for the evaluation of risk-treatment interactions. In addition, the risk prediction model may be useful for identifying high-risk patients for public health interventions, thereby improving the cost-effectiveness of medical care.
The strength of our study is that we added a strict primary endpoint of ESRD, which is more relevant than other common endpoints based on declined eGFR or CKD stage. Specifically, for patients at low risk characterized by higher baseline eGFR (≥60 ml/min/1.73 m2), using the full model to predict ESRD at 5 years should be more precise. Additionally, both the CKD model and IgAN model exhibited similar performance in our IgAN cohort. We still need to improve the predictive ability of the models by adding IgAN-specific biomarkers, such as HAA-IgA1 levels. Alternatively, considering the genetic background of IgAN (1, 42–45), adding genetic risk factors (29, 46) involved in disease progression could also be useful for improving risk prediction of disease progression.
Our analysis also has limitations. We did not explicitly model the risk of all-cause mortality in our IgAN population because the number of deaths in our cohort might have been underestimated. In addition, disease duration and treatment information prior to renal biopsy were incomplete, thus we did not involve these data for the analysis. Moreover, although the CKD model from the study by Tangri was evaluated and performed well in our Chinese IgAN cohort, more CKD cohorts are still needed to validate it. Additionally, as this study was a multicenter cohort study, the heterogeneity of the study population is a limitation. The lack of detailed data on systematic therapies from all the centers is another limitation.
Conclusion
In summary, we validated recently reported highly accurate predictive models for the progression of IgAN to kidney failure. Especially for patients at low risk characterized by higher baseline eGFR (≥60 ml/min/1.73 m2), an improvement in model performance was observed after adding histological indicators to these clinical indicators.
Data Availability Statement
The original contributions presented in the study are included in the article/Supplementary Material. Further inquiries can be directed to the corresponding author.
Ethics Statement
The studies involving human participants were reviewed and approved by the Ethics Research Committee from Ruijin Hospital, Medical School of Shanghai Jiaotong University. The patients/participants provided their written informed consent to participate in this study.
Author Contributions
JXi designed and was responsible for the study. YO analyzed the data and drafted the paper. ZZ, GL, HL, FX, LS, ZC, SY, YJ, JXu, MS, HH, WD, ZF, XP, WM, and NC collected the data. JXi revised the paper. All authors approved the final version of the manuscript. All authors contributed to the article and approved the submitted version.
Funding
This work was supported by the Major International (Regional) Joint Research Program of National Natural Science Foundation of China (No:8211001014), the National Natural Science Foundation of China (Nos. 81870460, 81570598, 81370015, 81900656), “Excellent Academic Leader” by Shanghai Science and Technology Commission (No: 21XD1402000). Shanghai Jiao Tong University “Jiaotong Star” Plan Medical Engineering Cross Research Key Project (No: YG2019ZDA18, YG2019QNA37), Shanghai Shenkang Hospital Development Center “Three-year Action Plan for Promoting Clinical Skills and Clinical Innovation in Municipal Hospitals” (No : SHDC2020CR6017), Science and Technology Innovation Action Plan of Shanghai Science and Technology Committee (No. 17441902200), Shanghai Municipal Education Commission, Gaofeng Clinical Medicine Grant (No. 20152207), Shanghai Jiao Tong University School of Medicine, Multi-Center Clinical Research Project (No: DLY201510), Shanghai Health and Family Planning Committee Hundred Talents Program (No: 2018BR37), and Shanghai Municipal Key Clinical Specialty (shslczdzk02502). No funding bodies had any role in study design, data collection and analysis, decision to publish, or preparation of the manuscript.
Conflict of Interest
The authors declare that the research was conducted in the absence of any commercial or financial relationships that could be construed as a potential conflict of interest.
Publisher’s Note
All claims expressed in this article are solely those of the authors and do not necessarily represent those of their affiliated organizations, or those of the publisher, the editors and the reviewers. Any product that may be evaluated in this article, or claim that may be made by its manufacturer, is not guaranteed or endorsed by the publisher.
Acknowledgments
We acknowledge the contributions of clinicians at six renal centers caring for these patients. We also acknowledge the participation of the patients involved.
Supplementary Material
The Supplementary Material for this article can be found online at: https://www.frontiersin.org/articles/10.3389/fimmu.2021.753901/full#supplementary-material
References
1. Kiryluk K, Li Y, Scolari F, Sanna-Cherchi S, Choi M, Verbitsky M, et al. Discovery of New Risk Loci for IgA Nephropathy Implicates Genes Involved in Immunity Against Intestinal Pathogens. Nat Genet (2014) 46:1187–96. doi: 10.1038/ng.3118
2. Donadio JV, Grande JP. IgA Nephropathy. N Engl J Med (2002) 347:738–48. doi: 10.1056/NEJMra020109
3. McGrogan A, Franssen CF, de Vries CS. The Incidence of Primary Glomerulonephritis Worldwide: A Systematic Review of the Literature. Nephrol Dial Transplant (2011) 26:414–30. doi: 10.1093/ndt/gfq665
4. Reich HN, Troyanov S, Scholey JW, Cattran DC. Toronto Glomerulonephritis R. Remission of Proteinuria Improves Prognosis in IgA Nephropathy. J Am Soc Nephrol (2007) 18:3177–83. doi: 10.1681/ASN.2007050526
5. Berthoux FC, Mohey H, Afiani A. Natural History of Primary IgA Nephropathy. Semin Nephrol (2008) 28:4–9. doi: 10.1016/j.semnephrol.2007.10.001
6. Taal MW, Brenner BM. Predicting Initiation and Progression of Chronic Kidney Disease: Developing Renal Risk Scores. Kidney Int (2006) 70:1694–705. doi: 10.1038/sj.ki.5001794
7. Taal MW, Brenner BM. Renal Risk Scores: Progress and Prospects. Kidney Int (2008) 73:1216–9. doi: 10.1038/ki.2008.36
8. Barbour SJ, Reich HN. Risk Stratification of Patients With IgA Nephropathy. Am J Kidney Dis (2012) 59:865–73. doi: 10.1053/j.ajkd.2012.02.326
9. Bartosik LP, Lajoie G, Sugar L, Cattran DC. Predicting Progression in IgA Nephropathy. Am J Kidney Dis (2001) 38:728–35. doi: 10.1053/ajkd.2001.27689
10. Barbour SJ, Espino-Hernandez G, Reich HN, Coppo R, Roberts IS, Feehally J, et al. The MEST Score Provides Earlier Risk Prediction in lgA Nephropathy. Kidney Int (2016) 89:167–75. doi: 10.1038/ki.2015.322
11. Moriyama T, Tanaka K, Iwasaki C, Oshima Y, Ochi A, Kataoka H, et al. Prognosis in IgA Nephropathy: 30-Year Analysis of 1,012 Patients at a Single Center in Japan. PloS One (2014) 9:e91756. doi: 10.1371/journal.pone.0091756
12. Wakai K, Kawamura T, Endoh M, Kojima M, Tomino Y, Tamakoshi A, et al. A Scoring System to Predict Renal Outcome in IgA Nephropathy: From a Nationwide Prospective Study. Nephrol Dial Transplant (2006) 21:2800–8. doi: 10.1093/ndt/gfl342
13. Goto M, Wakai K, Kawamura T, Ando M, Endoh M, Tomino Y. A Scoring System to Predict Renal Outcome in IgA Nephropathy: A Nationwide 10-Year Prospective Cohort Study. Nephrol Dial Transplant (2009) 24:3068–74. doi: 10.1093/ndt/gfp273
14. Tanaka S, Ninomiya T, Katafuchi R, Masutani K, Tsuchimoto A, Noguchi H, et al. Development and Validation of a Prediction Rule Using the Oxford Classification in IgA Nephropathy. Clin J Am Soc Nephrol (2013) 8:2082–90. doi: 10.2215/CJN.03480413
15. Pesce F, Diciolla M, Binetti G, Naso D, Ostuni VC, Di Noia T, et al. Clinical Decision Support System for End-Stage Kidney Disease Risk Estimation in IgA Nephropathy Patients. Nephrol Dial Transplant (2016) 31:80–6. doi: 10.1093/ndt/gfv232
16. Coppo R, Lofaro D, Camilla RR, Bellur S, Cattran D, Cook HT, et al. Risk Factors for Progression in Children and Young Adults With IgA Nephropathy: An Analysis of 261 Cases From the VALIGA European Cohort. Pediatr Nephrol (2017) 32:139–50. doi: 10.1007/s00467-016-3469-3
17. Chen T, Li X, Li Y, Xia E, Qin Y, Liang S, et al. Prediction and Risk Stratification of Kidney Outcomes in IgA Nephropathy. Am J Kidney Dis (2019) 74:300–9. doi: 10.1053/j.ajkd.2019.02.016
18. Xie J, Lv J, Wang W, Li G, Liu Z, Chen H, et al. Kidney Failure Risk Prediction Equations in IgA Nephropathy: A Multicenter Risk Assessment Study in Chinese Patients. Am J Kidney Dis (2018) 72:371–80. doi: 10.1053/j.ajkd.2018.01.043
19. Barbour SJ, Coppo R, Zhang H, Liu ZH, Suzuki Y, Matsuzaki K, et al. Evaluating a New International Risk-Prediction Tool in IgA Nephropathy. JAMA Intern Med (2019) 179:942–52. doi: 10.1001/jamainternmed.2019.0600
20. Tangri N, Stevens LA, Griffith J, Tighiouart H, Djurdjev O, Naimark D, et al. A Predictive Model for Progression of Chronic Kidney Disease to Kidney Failure. JAMA (2011) 305:1553–9. doi: 10.1001/jama.2011.451
21. Zhang J, Huang B, Liu Z, Wang X, Xie M, Guo R, et al. External Validation of the International IgA Nephropathy Prediction Tool. Clin J Am Soc Nephrol (2020) 15:1112–20. doi: 10.2215/CJN.16021219
22. Tangri N, Grams ME, Levey AS, Coresh J, Appel LJ, Astor BC, et al. Multinational Assessment of Accuracy of Equations for Predicting Risk of Kidney Failure: A Meta-Analysis. JAMA (2016) 315:164–74. doi: 10.1001/jama.2015.18202
23. Major RW, Shepherd D, Medcalf JF, Xu G, Gray LJ, Brunskill NJ. The Kidney Failure Risk Equation for Prediction of End Stage Renal Disease in UK Primary Care: An External Validation and Clinical Impact Projection Cohort Study. PloS Med (2019) 16:e1002955. doi: 10.1371/journal.pmed.1002955
24. Trimarchi H, Barratt J, Cattran DC, Cook HT, Coppo R, Haas M, et al. Oxford Classification of IgA Nephropathy 2016: An Update From the IgA Nephropathy Classification Working Group. Kidney Int (2017) 91:1014–21. doi: 10.1016/j.kint.2017.02.003
25. Collins GS, Ogundimu EO, Altman DG. Sample Size Considerations for the External Validation of a Multivariable Prognostic Model: A Resampling Study. Stat Med (2016) 35:214–26. doi: 10.1002/sim.6787
26. Xie J, Kiryluk K, Wang W, Wang Z, Guo S, Shen P, et al. Predicting Progression of IgA Nephropathy: New Clinical Progression Risk Score. PloS One (2012) 7:e38904. doi: 10.1371/journal.pone.0038904
27. Xie J, Kiryluk K, Li Y, Mladkova N, Zhu L, Hou P, et al. Fine Mapping Implicates a Deletion of CFHR1 and CFHR3 in Protection From IgA Nephropathy in Han Chinese. J Am Soc Nephrol (2016) 27:3187–94. doi: 10.1681/ASN.2015111210
28. Ouyang Y, Xie J, Yang M, Zhang X, Ren H, Wang W, et al. Underweight Is an Independent Risk Factor for Renal Function Deterioration in Patients With IgA Nephropathy. PloS One (2016) 11:e0162044. doi: 10.1371/journal.pone.0162044
29. Ouyang Y, Zhu L, Shi M, Yu S, Jin Y, Wang Z, et al. A Rare Genetic Defect of MBL2 Increased the Risk for Progression of IgA Nephropathy. Front Immunol (2019) 10:537. doi: 10.3389/fimmu.2019.00537
30. Batsis JA, Lopez-Jimenez F. Cardiovascular Risk Assessment–From Individual Risk Prediction to Estimation of Global Risk and Change in Risk in the Population. BMC Med (2010) 8:29. doi: 10.1186/1741-7015-8-29
31. D'Agostino RB Sr., Grundy S, Sullivan LM, Wilson P, Group CHDRP. Validation of the Framingham Coronary Heart Disease Prediction Scores: Results of a Multiple Ethnic Groups Investigation. JAMA (2001) 286:180–7. doi: 10.1001/jama.286.2.180
32. Leslie WD, Morin S, Lix LM. A Before-and-After Study of Fracture Risk Reporting and Osteoporosis Treatment Initiation. Ann Intern Med (2010) 153:580–6. doi: 10.7326/0003-4819-153-9-201011020-00007
33. Pozen MW, D'Agostino RB, Selker HP, Sytkowski PA, Hood WB Jr. A Predictive Instrument to Improve Coronary-Care-Unit Admission Practices in Acute Ischemic Heart Disease. A Prospective Multicenter Clinical Trial. N Engl J Med (1984) 310:1273–8. doi: 10.1056/NEJM198405173102001
34. Selker HP, Beshansky JR, Griffith JL, Aufderheide TP, Ballin DS, Bernard SA, et al. Use of the Acute Cardiac Ischemia Time-Insensitive Predictive Instrument (ACI-TIPI) to Assist With Triage of Patients With Chest Pain or Other Symptoms Suggestive of Acute Cardiac Ischemia. A Multicenter, Controlled Clinical Trial. Ann Intern Med (1998) 129:845–55. doi: 10.7326/0003-4819-129-11_part_1-199812010-00002
35. van Steenkiste B, van der Weijden T, Stoffers HE, Grol R. Barriers to Implementing Cardiovascular Risk Tables in Routine General Practice. Scand J Prim Health Care (2004) 22:32–7. doi: 10.1080/02813430310004489
36. Muller-Riemenschneider F, Holmberg C, Rieckmann N, Kliems H, Rufer V, Muller-Nordhorn J, et al. Barriers to Routine Risk-Score Use for Healthy Primary Care Patients: Survey and Qualitative Study. Arch Intern Med (2010) 170:719–24. doi: 10.1001/archinternmed.2010.66
37. Johnson ES, Thorp ML, Platt RW, Smith DH. Predicting the Risk of Dialysis and Transplant Among Patients With CKD: A Retrospective Cohort Study. Am J Kidney Dis (2008) 52:653–60. doi: 10.1053/j.ajkd.2008.04.026
38. Geddes CC, Fox JG, Allison ME, Boulton-Jones JM, Simpson K. An Artificial Neural Network can Select Patients at High Risk of Developing Progressive IgA Nephropathy More Accurately Than Experienced Nephrologists. Nephrol Dial Transplant (1998) 13:67–71. doi: 10.1093/ndt/13.1.67
39. Schwarz S, Trivedi BK, Kalantar-Zadeh K, Kovesdy CP. Association of Disorders in Mineral Metabolism With Progression of Chronic Kidney Disease. Clin J Am Soc Nephrol (2006) 1:825–31. doi: 10.2215/CJN.02101205
40. de Brito-Ashurst I, Varagunam M, Raftery MJ, Yaqoob MM. Bicarbonate Supplementation Slows Progression of CKD and Improves Nutritional Status. J Am Soc Nephrol (2009) 20:2075–84. doi: 10.1681/ASN.2008111205
41. Wojciechowski P, Tangri N, Rigatto C, Komenda P. Risk Prediction in CKD: The Rational Alignment of Health Care Resources in CKD 4/5 Care. Adv Chronic Kidney Dis (2016) 23:227–30. doi: 10.1053/j.ackd.2016.04.002
42. Li M, Foo JN, Wang JQ, Low HQ, Tang XQ, Toh KY, et al. Identification of New Susceptibility Loci for IgA Nephropathy in Han Chinese. Nat Commun (2015) 6:7270. doi: 10.1038/ncomms8270
43. Yu XQ, Li M, Zhang H, Low HQ, Wei X, Wang JQ, et al. A Genome-Wide Association Study in Han Chinese Identifies Multiple Susceptibility Loci for IgA Nephropathy. Nat Genet (2011) 44:178–82. doi: 10.1038/ng.1047
44. Gharavi AG, Kiryluk K, Choi M, Li Y, Hou P, Xie J, et al. Genome-Wide Association Study Identifies Susceptibility Loci for IgA Nephropathy. Nat Genet (2011) 43:321–7. doi: 10.1038/ng.787
45. Feehally J, Farrall M, Boland A, Gale DP, Gut I, Heath S, et al. HLA has Strongest Association With IgA Nephropathy in Genome-Wide Analysis. J Am Soc Nephrol (2010) 21:1791–7. doi: 10.1681/ASN.2010010076
Keywords: IgA nephropathy, disease progression, end-stage renal disease, risk prediction models, risk factor
Citation: Ouyang Y, Zhao Z, Li G, Luo H, Xu F, Shao L, Chen Z, Yu S, Jin Y, Xu J, Shi M, Hussain HMJ, Du W, Fang Z, Pan X, Wang W, Xie J and Chen N (2021) A Validation Study Comparing Risk Prediction Models of IgA Nephropathy. Front. Immunol. 12:753901. doi: 10.3389/fimmu.2021.753901
Received: 05 August 2021; Accepted: 24 September 2021;
Published: 15 October 2021.
Edited by:
Haner Direskeneli, Marmara University, TurkeyReviewed by:
Alojzija Hocevar, University Medical Centre Ljubljana, SloveniaUmmugulsum Gazel, University of Ottawa, Canada
Copyright © 2021 Ouyang, Zhao, Li, Luo, Xu, Shao, Chen, Yu, Jin, Xu, Shi, Hussain, Du, Fang, Pan, Wang, Xie and Chen. This is an open-access article distributed under the terms of the Creative Commons Attribution License (CC BY). The use, distribution or reproduction in other forums is permitted, provided the original author(s) and the copyright owner(s) are credited and that the original publication in this journal is cited, in accordance with accepted academic practice. No use, distribution or reproduction is permitted which does not comply with these terms.
*Correspondence: Jingyuan Xie, bmVwaHJveGllQDE2My5jb20=