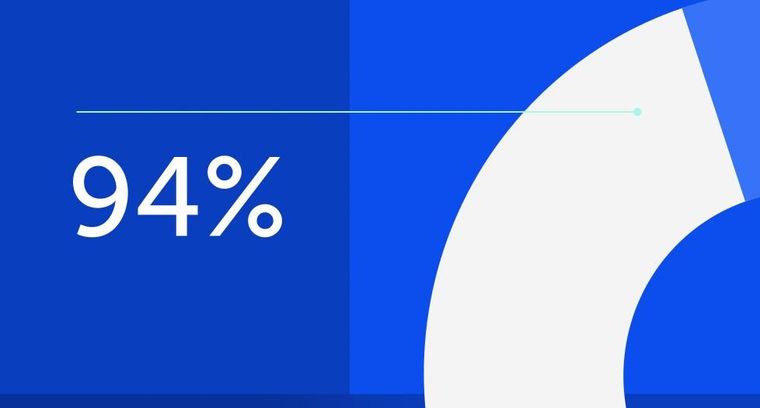
94% of researchers rate our articles as excellent or good
Learn more about the work of our research integrity team to safeguard the quality of each article we publish.
Find out more
ORIGINAL RESEARCH article
Front. Immunol., 24 January 2022
Sec. Autoimmune and Autoinflammatory Disorders
Volume 12 - 2021 | https://doi.org/10.3389/fimmu.2021.746998
This article is part of the Research TopicEmerging Insights in Controlling AutoimmunityView all 24 articles
Background: Growing evidence has shown that alterations in gut microbiota composition are associated with multiple autoimmune diseases (ADs). However, it is unclear whether these associations reflect a causal relationship.
Objective: To reveal the causal association between gut microbiota and AD, we conducted a two-sample Mendelian randomization (MR) analysis.
Materials and Methods: We assessed genome-wide association study (GWAS) summary statistics for gut microbiota and six common ADs, namely, systemic lupus erythematosus, rheumatoid arthritis, inflammatory bowel disease, multiple sclerosis, type 1 diabetes (T1D), and celiac disease (CeD), from published GWASs. Two-sample MR analyses were first performed to identify causal bacterial taxa for ADs in discovery samples. Significant bacterial taxa were further replicated in independent replication outcome samples. A series of sensitivity analyses was performed to validate the robustness of the results. Finally, a reverse MR analysis was performed to evaluate the possibility of reverse causation.
Results: Combining the results from the discovery and replication stages, we identified one causal bacterial genus, Bifidobacterium. A higher relative abundance of the Bifidobacterium genus was associated with a higher risk of T1D [odds ratio (OR): 1.605; 95% CI, 1.339–1.922; PFDR = 4.19 × 10−7] and CeD (OR: 1.401; 95% CI, 1.139–1.722; PFDR = 2.03 × 10−3), respectively. Further sensitivity analyses validated the robustness of the above associations. The results of reverse MR analysis showed no evidence of reverse causality from T1D and CeD to the Bifidobacterium genus.
Conclusion: This study implied a causal relationship between the Bifidobacterium genus and T1D and CeD, thus providing novel insights into the gut microbiota-mediated development mechanism of ADs.
Autoimmune diseases (ADs) are conditions in which an individual’s immune system mistakenly attacks its host’s tissues. Patients with ADs often endure lifelong debilitating symptoms, loss of organ function, reduced productivity at work, and high medical expenses. ADs are considered a significant cause of morbidity and mortality worldwide. Accumulating evidence demonstrates a steady rise in the incidence of ADs over the last few decades (1).
Although the etiology and pathogenesis of ADs are not fully understood, genetic components, environmental factors, and their interactions have great significance in their development. In addition, growing evidence suggests that alterations in gut microbiota composition are closely related to autoimmunity (2, 3). The gut microbiota is defined as the community of microorganisms that live in the human gastrointestinal tract. Gut microbial dysbiosis has been observed in many AD studies. For example, multiple studies reported a decrease of Firmicutes/Bacteroidetes ratio in systemic lupus erythematosus (SLE) patients and type 1 diabetes (T1D) patients (4, 5). A case-control study reported an increased abundance of Methanobrevibacter and Akkermansia and decreased abundance of Butyricimonas in patients with multiple sclerosis (MS) (6). Chen et al. (7) found that rheumatoid arthritis (RA) patients had a decrease in Faecalibacterium and expansion of Eggerthella and Collinsella.
All the above gut microbiota–AD associations were derived from cross-sectional studies, leaving the causal nature of these associations elusive. However, establishing causal relationships not only deepens the understanding of gut microbiota-derived AD pathogenesis but also has the capacity to guide microbiota-orientated interventions against AD in the clinic. Therefore, there is an urgent need to elucidate the causal relationship between the gut microbiota and various types of AD.
Mendelian randomization (MR) is a statistical approach that implies causal association from an exposure to an outcome. It uses genetic variants associated with exposure as a surrogate for exposure to assess the association between the surrogate and the outcome (8). Thanks to fruitful findings from large-scale genome-wide association studies (GWASs) conducted to date at both gut microbiota and disease levels (9–11), MR analysis has been widely applied to various scenarios, including the causal associations between gut microbiota and AD. In previous studies, García-Santisteban et al. (12) performed an MR analysis and identified a causal association between gut microbiota composition and celiac disease (CeD). Another study by Inamo (13) identified no causal association between gut microbiota composition and RA. The above two studies fall short in that they treat gut microbiota composition as a whole without distinguishing specific taxa, while different microbial taxa may have distinct effects on human health. During the preparation of this article, Zhang et al. (14) and Xiang et al. (15) investigated the causal effects of specific microbial taxa on two ADs, inflammatory bowel disease (IBD) and SLE. However, studies on other ADs are still sparse.
In the present study, aiming to investigate the causal relationship between gut microbiota and a broad range of ADs, we conducted a comprehensive two-sample MR analysis of six ADs, including SLE, RA, IBD, MS, T1D, and CeD.
Our analysis used publicly available GWAS summary statistics. No new data were collected, and no new ethical approval was required. The flowchart of the study is shown in Figure 1. Briefly, gut microbiota served as the exposure, while ADs served as the outcome. Single-nucleotide polymorphisms (SNPs) significantly associated with specific gut microbiota taxa were selected as instrumental variables (IVs) based on strict inclusion and exclusion criteria. Outcome samples included both discovery and replication samples. A series of sensitivity analyses was performed for significant associations. Finally, reverse MR analysis was performed to mitigate the potential impact of ADs on the causal gut microbiota.
Figure 1 The flowchart of the study. The whole workflow of MR analysis. MR, Mendelian randomization; SLE, systemic lupus erythematosus; RA, rheumatoid arthritis; MS, multiple sclerosis; IBD, inflammatory bowel disease; T1D, type 1 diabetes; CeD, celiac disease.
Summary statistics for gut microbial taxa were obtained from a large-scale multi-ethnic GWAS meta-analysis that included 18,340 individuals from 24 cohorts (16). The microbial composition was profiled by targeting three distinct variable regions of the 16S rRNA gene. To account for differences in sequencing depth, all datasets were rarefied to 10,000 reads per sample. Taxonomic classification was performed using direct taxonomic binning. In each cohort, only the taxa present in more than 10% of the samples were included to explore the effect of host genetics on the abundance of gut bacterial taxa. The study-wide cutoffs included an effective sample size of at least 3,000 individuals and presence in at least three cohorts. A total of 211 taxa (131 genera, 35 families, 20 orders, 16 classes, and 9 phyla) were included. After adjustment for age, sex, technical covariates, and genetic principal components, Spearman’s correlation analysis was performed to identify genetic loci that affected the covariate-adjusted abundance of bacterial taxa. More details on the microbiota data were described elsewhere (16).
In the discovery stage, GWAS summary statistics for each of the six ADs were extracted from publicly available GWAS analyses. Summary statistics for SLE were obtained from a publicly available GWAS meta-analysis, including 7,219 cases and 15,991 controls of European ancestry (17). Summary statistics for RA were extracted from a GWAS meta-analysis, including 14,361 RA cases and 43,923 controls of European ancestry from 18 studies (18). Summary statistics for IBD were obtained from a GWAS meta-analysis of 25,042 IBD cases and 34,915 controls of European ancestry (19). Summary statistics of MS were derived from the discovery stage of the latest GWAS meta-analysis of the International MS Genetics Consortium (IMSGC), including 14,802 MS cases and 26,703 controls of European ancestry (20). Summary statistics of T1D were derived from a GWAS with 6,683 T1D cases and 12,173 controls of European ancestry (21). Finally, summary statistics of CeD were obtained from a GWAS meta-analysis, including 12,041 CeD cases and 12,228 controls (22). Detailed information on the datasets is provided in Table 1.
Significant bacterial taxa identified in the discovery stage were replicated during the replication stage. The replication outcome samples for RA, IBD, MS, and T1D were obtained from the UK Biobank study, which is a large prospective cohort study with approximately 500,000 participants aged 40–69 years from 22 centers across the United Kingdom. The replication sample of SLE was a single GWAS from Spain, including 907 patients with SLE and 1,524 healthy controls (23). The replication sample for CeD is a GWAS meta-analysis of five samples, including 4,533 CeD cases and 10,750 controls of European ancestry (24). Detailed information on the replication samples is presented in Table 1.
The 211 bacterial taxa were categorized at six taxonomic levels. Of these, the genus is the smallest and most specific taxonomic level. To identify each causal bacteria group as specifically as possible, we analyzed 131 bacterial taxa at the genus level only. Fourteen taxa with unknown groups were excluded, meaning 117 bacterial taxa were included in the subsequent MR analysis.
SNPs associated with gut bacterial taxa at the genome-wide significance threshold P < 5.0 × 10−8 were selected as potential IVs. A series of quality control steps was implemented to select eligible IVs. First, SNPs with inconsistent alleles between the exposure and outcome samples (i.e., A/G vs. A/C) were excluded. Second, palindromic A/T or G/C alleles were excluded. Third, SNPs within each bacterial taxon were clumped to retain only independent SNPs. The linkage disequilibrium (LD) threshold for clumping was set to r2 < 0.01, and the clumping window size was set to 500 kb. LD was estimated based on the European-based 1,000 Genome Projects reference panel. Fourth, the MR pleiotropy residual sum and outlier (MR‐PRESSO) test was applied to detect potential horizontal pleiotropy and to eliminate the effects of pleiotropy by removing outliers (25). Finally, to assess the strength of the selected SNPs, the following equation was used to calculate the F statistics for each bacterial taxon:
where R2 is the portion of exposure variance explained by the IVs, n is the sample size, and k is the number of IVs. An F-statistic ≥10 indicates no strong evidence of weak instrument bias (26). IVs with F-statistics less than <10 were considered weak IVs and were excluded.
We performed MR analysis to estimate the causal effect of the gut microbiota on the six ADs. For bacterial genera containing only one SNP, the Wald ratio method was used for the MR analysis. The causal effect was calculated by dividing the SNP-outcome effect estimated by the SNP-exposure effect estimate. For bacterial genera containing multiple SNPs, multiple tests, including fixed-/random-effects inverse variance weighted (IVW) test (27), weighted median method, and MR-Egger regression test were performed. Cochrane’s Q test was performed to assess the heterogeneity among SNPs associated with each bacterial genus. In the presence of heterogeneity (P < 0.05), the random-effects IVW test was used instead to provide a more conservative but robust estimate. The weighted median test can generate consistent estimates when ≥50% of the weights come from valid IVs (28). The MR-Egger regression test allows pleiotropy present in more than 50% of IVs (29).
Significant genera identified in the discovery samples were replicated in replication samples. The replication MR analysis procedure was the same as that used in the discovery stage. To evaluate the robustness of the identified causal associations, we performed two sensitivity analyses, including the MR-Egger intercept test and leave-one-out analysis. The intercept of the MR-Egger regression test can provide an estimate of the degree of directional pleiotropy (29). The leave-one-out analysis was performed to evaluate whether the significant results were driven by a single SNP.
To explore whether ADs have any causal impact on the identified significant bacterial genus, we also performed a reverse MR analysis (i.e., ADs as exposure and the identified causal bacterial genus as outcome) using SNPs that are associated with ADs as IVs.
All statistical analyses were conducted using R (version 4.0.3). The IVW, weighted median, and MR-Egger regression methods were performed using the “TwoSampleMR” package (version 0.5.4). The MR-PRESSO test was performed using the “MRPRESSO” package. The statistical significance of the MR effect estimates was defined as a false discovery rate (FDR) of <5% to adjust for multiple testing.
After a series of quality control steps, 32 SNPs associated with 13 genera were selected as IVs. Specifically, 19 independent SNPs (P < 5.0 × 10−8, r2 < 0.01) were associated with 13 genera for SLE, 17 independent SNPs were associated with 12 genera for RA, 19 SNPs were associated with 13 genera for MS, 18 SNPs were associated with 12 genera for IBD, 7 SNPs were associated with 3 genera for T1D, and 6 SNPs were associated with 3 genera for CeD (Supplementary Table S1). No evidence of pleiotropic effects was detected by the MR-PRESSO global test (P > 0.05). The F-statistics of IVs ranged between 29.78 and 2,074.13, all largely >10, indicating no evidence of weak instrument bias (Supplementary Table S2).
In the discovery stage, the genetically predicted relative abundance of two genera, Bifidobacterium and Ruminococcus, was associated with the risk of SLE, MS, T1D, and CeD. Ruminococcus was also associated with the risk of IBD (Table 2). Specifically, a higher genetically predicted Bifidobacterium level was associated with a lower risk of SLE [odds ratio (OR): 0.565, 95% confidence interval (CI): 0.426–0.748, PFDR = 8.53 × 10−4]. In contrast, a higher genetically predicted Bifidobacterium was associated with a higher risk of MS (OR: 1.384, 95% CI: 1.128–1.700, PFDR = 0.012), T1D (OR: 1.605, 95% CI: 1.339–1.922, PFDR = 4.19 × 10−7), and CeD (OR: 1.401, 95% CI: 1.139–1.722, PFDR = 2.03 × 10−3). These associations were also supported by the weighted median method, as shown in Table 2. The genetically predicted Ruminococcus level was associated with a higher risk of SLE (OR: 5.593, 95% CI: 2.079–15.045, PFDR = 4.22 × 10−3), IBD (OR: 2.141, 95% CI: 1.425–3.216, PFDR = 2.92 × 10−3), and MS (OR: 2.890, 95% CI: 1.669–5.003, PFDR = 1.96 × 10−3). But its associations with T1D and CeD were negative (OR: 0.122, 95% CI: 0.0661–0.224, PFDR = 3.38 × 10−11) and CeD (OR: 0.352, 95% CI: 0.195–0.635, PFDR = 1.57 × 10−3). As shown in Supplementary Table S2, there was no evidence of a causal association between any microbial taxa and RA.
These two genera Bifidobacterium and Ruminococcus were replicated in the replication samples. The causal effects of the Bifidobacterium genus on T1D and CeD were successfully replicated, as shown in Table 3. The effect direction was consistent with that in the discovery sample, which strengthened the confidence of the true causal associations.
No evidence of heterogeneity was observed between the genetic IVs for Bifidobacterium (Supplementary Table S3). None of the MR-Egger regression intercepts deviated from null, indicating no evidence of horizontal pleiotropy (all intercept P > 0.05) (Supplementary Table S4). Additionally, the leave-one-out analysis showed no marked difference in causal estimations of Bifidobacterium on T1D and CeD, suggesting that none of the identified causal associations were driven by any single IV (Figures 2, 3). In reverse MR analysis, there was no evidence of a causal effect of T1D and CeD on Bifidobacterium (Table 4). Detailed information on the IVs used in the reverse MR analyses is shown in Supplementary Table S5.
Figure 2 Leave-one-out analysis of the causal effect of Bifidobacterium on T1D. Red lines represent estimations from the IVW test. T1D, type 1 diabetes; IVW, inverse variance weighted.
Figure 3 Leave-one-out analysis of the causal effect of Bifidobacterium on CeD. Red lines represent estimations from the IVW test. CeD, celiac disease; IVW, inverse variance weighted.
In this study, we performed two-sample MR analyses to investigate the causal association between gut microbiota and six common ADs (SLE, RA, MS, IBD, T1D, and CeD). Combining evidence from both discovery and replication samples, we identified that the bacterial genus Bifidobacterium was causally associated with the risk of T1D and CeD.
Bifidobacterium is the primary microbe that colonizes the human gut. Previous observational studies have demonstrated that Bifidobacterium plays an important role in the pathogenesis of multiple ADs. However, observational studies have yielded conflicting results regarding the effect pattern. Two case-control studies showed that the relative abundance of Bifidobacterium was higher in T1D patients than that in controls (30, 31). Similarly, a higher relative abundance of Bifidobacterium was observed in patients with CeD (32). In line with these studies, our study suggested that the increased relative abundance of Bifidobacterium was causally associated with a higher risk of T1D and CeD, indicating its harmful effect on both diseases. In contrast, several other studies observed a lower relative abundance of Bifidobacterium in T1D and CeD patients, suggesting its protective effect (33–35).
Recent studies have shown that probiotic intervention, mainly of the Lactobacillus and Bifidobacterium genera, can effectively attenuate the progression of multiple ADs, including T1D and CeD. In a double-blinded, placebo-controlled trial, probiotic intervention with Bifidobacterium breve BR03 and B. breve B632 has shown a positive effect on decreasing the production of the pro-inflammatory cytokine tumor necrosis factor-α (TNF-α) in children with CeD on a gluten-free diet (36). In contrast, Smecuol et al. (37) did not detect significant changes in TNF-α in CeD patients treated with Bifidobacterium infantis. Similarly, Groele et al. (38) reported that administration of Lactobacillus rhamnosus GG and Bifidobacterium lactis Bb12 had no significant effect on maintaining the residual pancreatic beta-cell function in children with newly diagnosed T1D. There was no significant difference in cytokine levels and intestinal permeability (zonulin levels) between the probiotics and placebo groups (38).
Some functional studies have shown evidence of the anti-inflammatory effects of Bifidobacterium, while others have reported its pro-inflammatory effects. A previous study showed that Bifidobacterium adolescentis significantly increased Th17 cell levels in several other gut-associated organs, while elevated Th17 cell responses have been associated with autoimmune/inflammatory disease in both mice and humans (38). In addition, López et al. (39) reported that some Bifidobacterium bifidum strains could induce the secretion of large amounts of interleukin IL-17 and promote Th17 cell polarization. Combining evidence from observational studies, MR analysis, clinical trials, and functional studies, we speculated that the positive and negative effects of Bifidobacterium on ADs may be species- and strain-specific. The causal relationship between Bifidobacterium and ADs needs to be further explored at more specialized levels (i.e., species level and strain level).
In previous studies, Zhang et al. (14) and Xiang et al. (15) performed MR analyses to investigate the effects of gut microbiota on IBD and SLE, respectively. Our study differs from their studies in the following three aspects: First, our study is more comprehensive in its investigation of ADs. Unlike the above two studies that analyzed two separate diseases, we comprehensively analyzed six common AD diseases. This will give us an opportunity to evaluate common gut microbiota that are causally related to multiple ADs. Second, the quality control procedure for selecting IVs was stricter in our study. We selected independent GWAS SNPs as IVs and conducted a series of sensitivity analyses, including horizontal pleiotropy assessment and reverse MR analysis, to maximally fulfill basic MR assumptions. In contrast, the above two studies used a fairly loose P-value threshold (P < 1 × 10−5) to select eligible IVs. Third, Zhang et al. (14) used summary-level data of gut microbiota in a relatively small sample size (N = 1,126 twin pairs). Instead, the sample size in the present study was much larger (N = 18,340). Meanwhile, the causal associations identified in the discovery stage were further replicated in independent replication outcome samples, which enhanced the confidence of the true causal relationship.
Nevertheless, our study had several limitations. First, while the majority of participants in the GWAS summary data used in our study were of European ancestry, a small number of the gut microbiota data were taken from sets consisting of other races, which may partially bias our estimates. Second, bacterial taxa were only analyzed at the genus level but not at a more specialized level such as species or strain levels. When microbiota GWASs use more advanced shotgun metagenomic sequencing analysis, the results can be more specific and accurate. Third, our study used gut microbiota data from a meta-analysis of mostly adult individuals, whereas the CeD study was conducted in children. Finally, most ADs are more prevalent in women than in men (e.g., SLE, RA, and MS). However, our study did not analyze the two genders separately, which may have influenced our results. It would be helpful to perform a gender-specific MR analysis in future endeavors.
In conclusion, our findings support the potentially causal effects of the Bifidobacterium genus on T1D and CeD. Although Bifidobacterium is generally considered beneficial bacteria, specific species and strains of Bifidobacterium may have varying effects on human health. Therefore, the potential mechanisms of specific species and strains of Bifidobacterium in the development of AD need to be further investigated.
The datasets presented in this study can be found in online repositories. The names of the repository/repositories and accession number(s) can be found in the article/Supplementary Material.
LZ and Y-FP designed the research. QX and J-JN collected the data. QX and BL analyzed the data. J-JN, B-XH, S-SY, X-TW, G-JF, and HZ performed the literature search. QX and BL drafted the article. Y-FP and LZ jointly supervised the study. All authors were involved in writing the paper. All authors contributed to the article and approved the submitted version.
This study was partially supported by funding from the National Natural Science Foundation of China (31771417) and a project funded by the Priority Academic Program Development (PAPD) of Jiangsu higher education institutions. The numerical calculations in this paper have been done on the supercomputing system of the National Supercomputing Center in Changsha. This study was supported by grants from Suzhou Science and Technology Bureau (No. SKY2021022), Gusu Health Top-Notch Youth Talent of Suzhou Health Commission (No. GSWS2019086) and Wujiang District Health Commission (No. WWK201806).
The authors declare that the research was conducted in the absence of any commercial or financial relationships that could be construed as a potential conflict of interest.
All claims expressed in this article are solely those of the authors and do not necessarily represent those of their affiliated organizations, or those of the publisher, the editors and the reviewers. Any product that may be evaluated in this article, or claim that may be made by its manufacturer, is not guaranteed or endorsed by the publisher.
We appreciate all the volunteers who participated in this study. We are grateful to the IMSGC for releasing the latest MS GWAS summary statistics and the MiBioGen consortium for releasing the gut microbiota GWAS summary statistics.
The Supplementary Material for this article can be found online at: https://www.frontiersin.org/articles/10.3389/fimmu.2021.746998/full#supplementary-material
1. Lerner A, Jeremias P, Matthias T. The World Incidence and Prevalence of Autoimmune Diseases is Increasing. Int J Celiac Dis (2015) 3:151–5.
2. De Luca F, Shoenfeld Y. The Microbiome in Autoimmune Diseases. Clin Exp Immunol (2019) 195:74–85. doi: 10.1111/cei.13158
3. Chen B, Sun L, Zhang X. Integration of Microbiome and Epigenome to Decipher the Pathogenesis of Autoimmune Diseases. J Autoimmun (2017) 83:31–42. doi: 10.1016/j.jaut.2017.03.009
4. López P, de Paz B, Rodríguez-Carrio J, Hevia A, Sánchez B, Margolles A, et al. Th17 Responses and Natural IgM Antibodies are Related to Gut Microbiota Composition in Systemic Lupus Erythematosus Patients. Sci Rep (2016) 6:24072. doi: 10.1038/srep24072
5. Murri M, Leiva I, Gomez-Zumaquero JM, Tinahones FJ, Cardona F, Soriguer F, et al. Gut Microbiota in Children With Type 1 Diabetes Differs From That in Healthy Children: A Case-Control Study. BMC Med (2013) 11:46. doi: 10.1186/1741-7015-11-46
6. Jangi S, Gandhi R, Cox LM, Li N, von Glehn F, Yan R, et al. Alterations of the Human Gut Microbiome in Multiple Sclerosis. Nat Commun (2016) 7:12015. doi: 10.1038/ncomms12015
7. Chen J, Wright K, Davis JM, Jeraldo P, Marietta EV, Murray J, et al. An Expansion of Rare Lineage Intestinal Microbes Characterizes Rheumatoid Arthritis. Genome Med (2016) 8(1):43. doi: 10.1186/s13073-016-0299-7
8. Emdin CA, Khera AV, Kathiresan S. Mendelian Randomization. JAMA (2017) 318(19):1925–6. doi: 10.1001/jama.2017.17219
9. Goodrich JK, Davenport ER, Beaumont M, Jackson MA, Knight R, Ober C, et al. Genetic Determinants of the Gut Microbiome in UK Twins. Cell Host Microbe (2016) 19(5):731–43. doi: 10.1016/j.chom.2016.04.017
10. Wang J, Thingholm LB, Skiecevičienė J, Rausch P, Kummen M, Hov JR, et al. Genome-Wide Association Analysis Identifies Variation in Vitamin D Receptor and Other Host Factors Influencing the Gut Microbiota. Nat Genet (2016) 48(11):1396–406. doi: 10.1038/ng.3695
11. Bonder MJ, Kurilshikov A, Tigchelaar EF, Mujagic Z, Imhann F, Vila AV, et al. The Effect of Host Genetics on the Gut Microbiome. Nat Genet (2016) 48(11):1407–12. doi: 10.1038/ng.3663
12. García-Santisteban I, Cilleros-Portet A, Moyua-Ormazabal E, Kurilshikov A, Zhernakova A, Garcia-Etxebarria K, et al. A Two-Sample Mendelian Randomization Analysis Investigates Associations Between Gut Microbiota and Celiac Disease. Nutrients (2020) 12(5):1420. doi: 10.3390/nu12051420
13. Inamo J. Non-Causal Association of Gut Microbiome on the Risk of Rheumatoid Arthritis: A Mendelian Randomisation Study. Ann Rheum Dis (2021) 81(1):e3. doi: 10.1136/annrheumdis-2019-216565
14. Zhang ZJ, Qu HL, Zhao N, Wang J, Wang XY, Hai R, et al. Assessment of Causal Direction Between Gut Microbiota and Inflammatory Bowel Disease: A Mendelian Randomization Analysis. Front Genet (2021) 12:631061. doi: 10.3389/fgene.2021.631061
15. Xiang K, Wang P, Xu Z, Hu YQ, He YS, Chen Y, et al. Causal Effects of Gut Microbiome on Systemic Lupus Erythematosus: A Two-Sample Mendelian Randomization Study. Front Immunol (2021) 12:667097. doi: 10.3389/fimmu.2021.667097
16. Kurilshikov A, Medina-Gomez C, Bacigalupe R, Radjabzadeh D, Wang J, Demirkan A, et al. Large-Scale Association Analyses Identify Host Factors Influencing Human Gut Microbiome Composition. Nat Genet (2021) 53(2):156–65. doi: 10.1038/s41588-020-00763-1
17. Bentham J, Morris DL, Graham DSC, Pinder CL, Tombleson P, Behrens TW, et al. Genetic Association Analyses Implicate Aberrant Regulation of Innate and Adaptive Immunity Genes in the Pathogenesis of Systemic Lupus Erythematosus. Nat Genet (2015) 47(12):1457–64. doi: 10.1038/ng.3434
18. Okada Y, Wu D, Trynka G, Raj T, Terao C, Ikari K, et al. Genetics of Rheumatoid Arthritis Contributes to Biology and Drug Discovery. Nature (2014) 506(7488):376–81. doi: 10.1038/nature12873
19. de Lange KM, Moutsianas L, Lee JC, Lamb CA, Luo Y, Kennedy NA, et al. Genome-Wide Association Study Implicates Immune Activation of Multiple Integrin Genes in Inflammatory Bowel Disease. Nat Genet (2017) 49(2):256–61. doi: 10.1038/ng.3760
20. International Multiple Sclerosis Genetics Consortium. Multiple Sclerosis Genomic Map Implicates Peripheral Immune Cells and Microglia in Susceptibility. Sci (New York NY) (2019) 365(6460):eaav7188. doi: 10.1126/science.aav7188
21. Onengut-Gumuscu S, Chen WM, Burren O, Cooper NJ, Quinlan AR, Mychaleckyj JC, et al. Fine Mapping of Type 1 Diabetes Susceptibility Loci and Evidence for Colocalization of Causal Variants With Lymphoid Gene Enhancers. Nat Genet (2015) 47(4):381–6. doi: 10.1038/ng.3245
22. Trynka G, Hunt KA, Bockett NA, Romanos J, Mistry V, Szperl A, et al. Dense Genotyping Identifies and Localizes Multiple Common and Rare Variant Association Signals in Celiac Disease. Nat Genet (2011) 43(12):1193–201. doi: 10.1038/ng.998
23. Julià A, López-Longo FJ, Pérez Venegas JJ, Bonàs-Guarch S, Olivé À, Andreu JL, et al. Genome-Wide Association Study Meta-Analysis Identifies Five New Loci for Systemic Lupus Erythematosus. Arthritis Res Ther (2018) 20(1):100. doi: 10.1186/s13075-018-1604-1
24. Dubois PC, Trynka G, Franke L, Hunt KA, Romanos J, Curtotti A, et al. Multiple Common Variants for Celiac Disease Influencing Immune Gene Expression. Nat Genet (2010) 42:295–302. doi: 10.1038/ng.543
25. Verbanck M, Chen CY, Neale B, Do R. Detection of Widespread Horizontal Pleiotropy in Causal Relationships Inferred From Mendelian Randomization Between Complex Traits and Diseases. Nat Genet (2018) 50(5):693–8. doi: 10.1038/s41588-018-0099-7
26. Pierce BL, Ahsan H, Vanderweele TJ. Power and Instrument Strength Requirements for Mendelian Randomization Studies Using Multiple Genetic Variants. Int J Epidemiol (2011) 40(3):740–52. doi: 10.1093/ije/dyq151
27. Burgess S, Butterworth A, Thompson SG. Mendelian Randomization Analysis With Multiple Genetic Variants Using Summarized Data. Genet Epidemiol (2013) 37(7):658–65. doi: 10.1002/gepi.21758
28. Bowden J, Davey Smith G, Haycock PC, Burgess S. Consistent Estimation in Mendelian Randomization With Some Invalid Instruments Using a Weighted Median Estimator. Genet Epidemiol (2016) 40(4):304–14. doi: 10.1002/gepi.21965
29. Bowden J, Davey Smith G, Burgess S. Mendelian Randomization With Invalid Instruments: Effect Estimation and Bias Detection Through Egger Regression. Int J Epidemiol (2015) 44(2):512–25. doi: 10.1093/ije/dyv080
30. Maffeis C, Martina A, Corradi M, Quarella S, Nori N, Torriani S, et al. Association Between Intestinal Permeability and Faecal Microbiota Composition in Italian Children With Beta Cell Autoimmunity at Risk for Type 1 Diabetes. Diabetes/Metabol Res Rev (2016) 32(7):700–9. doi: 10.1002/dmrr.2790
31. Brown CT, Davis-Richardson AG, Giongo A, Gano KA, Crabb DB, Mukherjee N, et al. Gut Microbiome Metagenomics Analysis Suggests a Functional Model for the Development of Autoimmunity for Type 1 Diabetes. PloS One (2011) (6):e25792. doi: 10.1371/journal.pone.0025792
32. Nistal E, Caminero A, Vivas S, Ruiz de Morales JM, Sáenz de Miera LE, Rodríguez-Aparicio LB, et al. Differences in Faecal Bacteria Populations and Faecal Bacteria Metabolism in Healthy Adults and Celiac Disease Patients. Biochimie (2012) 94(8):1724–9. doi: 10.1016/j.biochi.2012.03.025
33. Pinto E, Anselmo M, Calha M, Bottrill A, Duarte I, Andrew PW, et al. The Intestinal Proteome of Diabetic and Control Children Is Enriched With Different Microbial and Host Proteins. Microbiol (Read Engl) (2017) 163(2):161–74. doi: 10.1099/mic.0.000412
34. Salamon D, Sroka-Oleksiak A, Kapusta P, Szopa M, Mrozińska S, Ludwig-Słomczyńska AH, et al. Characteristics of Gut Microbiota in Adult Patients With Type 1 and Type 2 Diabetes Based on Next−Generation Sequencing of the 16S rRNA Gene Fragment. Polish Arch Intern Med (2018) 128(6):336–43. doi: 10.20452/pamw.4246
35. Leiva-Gea I, Sánchez-Alcoholado L, Martín-Tejedor B, Castellano-Castillo D, Moreno-Indias I, Urda-Cardona A, et al. Gut Microbiota Differs in Composition and Functionality Between Children With Type 1 Diabetes and MODY2 and Healthy Control Subjects: A Case-Control Study. Diabetes Care (2018) 41(11):2385–95. doi: 10.2337/dc18-0253
36. Klemenak M, Dolinšek J, Langerholc T, Di Gioia D, Mičetić-Turk D. Administration of Bifidobacterium Breve Decreases the Production of TNF-α in Children With Celiac Disease. Digest Dis Sci (2015) 60(11):3386–92. doi: 10.1007/s10620-015-3769-7
37. Smecuol E, Hwang HJ, Sugai E, Corso L, Cherñavsky AC, Bellavite FP, et al. Exploratory, Randomized, Double-Blind, Placebo-Controlled Study on the Effects of Bifidobacterium Infantis Natren Life Start Strain Super Strain in Active Celiac Disease. J Clin Gastroenterol (2013) 47(2):139–47. doi: 10.1097/MCG.0b013e31827759ac
38. Groele L, Szajewska H, Szalecki M, Świderska J, Wysocka-Mincewicz M, Ochocińska A, et al. Lack of Effect of Lactobacillus Rhamnosus GG and Bifidobacterium Lactis Bb12 on Beta-Cell Function in Children With Newly Diagnosed Type 1 Diabetes: A Randomised Controlled Trial. BMJ Open Diabetes Res Care (2021) 9(1):e001523. doi: 10.1136/bmjdrc-2020-001523
Keywords: Mendelian randomization, gut microbiota, autoimmune disease (AD), type 1 diabetes, celiac disease
Citation: Xu Q, Ni J-J, Han B-X, Yan S-S, Wei X-T, Feng G-J, Zhang H, Zhang L, Li B and Pei Y-F (2022) Causal Relationship Between Gut Microbiota and Autoimmune Diseases: A Two-Sample Mendelian Randomization Study. Front. Immunol. 12:746998. doi: 10.3389/fimmu.2021.746998
Received: 25 July 2021; Accepted: 20 December 2021;
Published: 24 January 2022.
Edited by:
Elias Toubi, Technion Israel Institute of Technology, IsraelReviewed by:
Feng Zhang, Xi’an Jiaotong University, ChinaCopyright © 2022 Xu, Ni, Han, Yan, Wei, Feng, Zhang, Zhang, Li and Pei. This is an open-access article distributed under the terms of the Creative Commons Attribution License (CC BY). The use, distribution or reproduction in other forums is permitted, provided the original author(s) and the copyright owner(s) are credited and that the original publication in this journal is cited, in accordance with accepted academic practice. No use, distribution or reproduction is permitted which does not comply with these terms.
*Correspondence: Lei Zhang, bHpoYW5nNkBzdWRhLmVkdS5jbg==; Bin Li, YmxpNDAwNEBzdWRhLmVkdS5jbg==; Yu-Fang Pei, eXBlaUBzdWRhLmVkdS5jbg==
Disclaimer: All claims expressed in this article are solely those of the authors and do not necessarily represent those of their affiliated organizations, or those of the publisher, the editors and the reviewers. Any product that may be evaluated in this article or claim that may be made by its manufacturer is not guaranteed or endorsed by the publisher.
Research integrity at Frontiers
Learn more about the work of our research integrity team to safeguard the quality of each article we publish.