- 1Department of Thoracic Surgery, National Cancer Center/National Clinical Research Center for Cancer/Cancer Hospital, Chinese Academy of Medical Sciences and Peking Union Medical College, Beijing, China
- 2Department of Pathology, National Cancer Center/National Clinical Research Center for Cancer/Cancer Hospital, Chinese Academy of Medical Sciences and Peking Union Medical College, Beijing, China
Tumor necrosis factor (TNF) family members participate in the body’s antitumor immunity response and influence tumor prognosis and treatment response. However, little is known about the roles of TNF family members in small cell lung cancer (SCLC). Therefore, we conducted the first comprehensive investigation of TNF family members in patients with SCLC, with the goal of using them to predict prognosis and chemotherapy benefit. Abnormal genetic alterations and expression of TNF family members were found to be widespread in SCLC patients. Using LASSO Cox regression analysis, we constructed a TNF family-based signature that separated SCLC patients in the training set (n=77) into high- and low-risk groups with distinct survival and chemotherapy benefit, and the signature was well-validated in the validation set (n=137) by RT-qPCR. Importantly, the signature exhibited superior predictive performance and was identified as a novel independent prognostic factor. Additionally, different immune phenotypes were found between the low-risk and high-risk groups, and high-risk patients had higher CMTM6 expression, suggesting that these patients could benefit from therapeutic methods targeting CMTM6. We constructed the first clinically applicable TNF family-based signature for predicting prognosis and chemotherapy benefit for patients with SCLC. The findings reported here provide a new method for predicting the prognosis of SCLC patients and optimizing clinical management.
Introduction
Small cell lung cancer (SCLC) is a recalcitrant malignancy that accounts for 13–15% of all lung cancers (1). It is an aggressive neuroendocrine tumor characterized by rapid growth and early and widespread hematogenous metastases (2). Patients with SCLC generally receive dismal prognosis, with an average 5-year overall survival (OS) of less than 5%, and median survival of only 7–12 months (3). Most SCLC patients are diagnosed in the advanced stage and are thus not candidates for surgery. Chemotherapy has been the standard treatment for first- and second-line management of SCLC for more than three decades (4, 5). However, patients often rapidly develop drug resistance or relapse after remission, even if they are initially sensitive to chemotherapy (6). The scarcity of SCLC tissue samples has hindered the process of investigating the mechanism underlying its rapid progression and treatment resistance and identifying tumor-specific prognosis predictive biomarkers in large-scale studies. Therefore, there is an urgent and unmet need to identify biomarkers that can be used to accurately predict prognosis and chemotherapy benefit so that clinical management of SCLC patients can be improved.
Immunotherapy has revolutionized cancer treatment over the past decade and has extended the life expectancy of patients with malignant tumors, including SCLC (7, 8). It has been reported that a high mutational burden is closely related to immunotherapy efficacy (9). SCLC is characterized by a high mutational burden, and these mutations can give rise to immunogenic neoantigens, which can be presented by the major histocompatibility complex and recognized by T cells, leading to tumor-specific CD8+ T cell activity (10). In addition, a highly activated host tumor immune microenvironment (TIME) is closely associated with better clinical outcomes for SCLC patients, indicating that immunotherapy could be a promising treatment for SCLC (11). In recent years, immunotherapy has achieved some encouraging results in SCLC patients. The combination of PD-L1 inhibitors like atezolizumab and durvalumab with chemotherapy has enhanced the OS of SCLC patients by 2–3 months since they were approved as first-line treatments for SCLC by the Food and Drug Administration (FDA) (12, 13). However, therapeutic interventions targeting the two best-described B7-CD28 family immunotherapy targets—T-lymphocyte protein 4 (CTLA-4) and programmed cell death protein-1 (PD-1)—have failed to achieve favorable results in patients with SCLC (14–16).Therefore, a better understanding of the TIME and immune checkpoint blockades has the potential to lead to promising new SCLC treatments. The immune molecular expression profile in the TIME is associated with conventional chemotherapy efficacy (17). Tumors influence the TIME by suppressing extracellular signals; the immunologic milieu can also affect malignant behavior and progression (18). Our previous study confirmed that TIME-related biomarkers could be used to effectively predict radiotherapy and chemotherapy benefit in ESCC patients (19). Hence, understanding the complex and diverse molecular profile of the immunologic genome in the TIME is also indispensable for maximizing the benefits of SCLC therapy.
Tumor necrosis factor (TNF) family is a master mediator of survival signaling in inflammation, which plays an important role at many stages of the immune response (20). The TNF superfamily (TNFSF) and TNF receptor superfamily (TNFRSF) include nineteen ligands and twenty-nine receptors, which play important roles in modulating cellular functions. T cell-related diseases may be treated by manipulating ligand-receptor interactions involved in inflammatory and autoimmune diseases (21). TNFSF is a type II transmembrane protein featuring a TNF homology domain. TNFRSF members can stimulate T-cell responsiveness and define niches for T cell memory (21, 22). Several TNFRSF members have been identified as emerging cancer immunotherapy targets (23–25). Unfortunately, the expression profile and clinical relevance of the TNF family in SCLC remain poorly understood.
We focused on TNF family members and sought to determine their expression profiles and clinical significance to SCLC. Survival and multivariate analyses were used to identify the genes with the greatest prognostic value. We constructed a predictive model based on TNF family members for patients with SCLC and used information extracted from public datasets to validate our novel signature in different cohorts and clinical subsets. Finally, we determined the predictive value of immune markers for adjuvant chemotherapy (ACT) in patients with SCLC. To the best of our knowledge, this signature is the first prognostic indicator based on the TNF family for SCLC. Our results may help define new therapeutic strategies, optimize the application of precision medicine, and offer renewed hope for patients with SCLC.
Materials and Methods
Public mRNA Expression Datasets and Clinical Information
We compiled comprehensive genomic profiles with complete clinical parameters and survival information for SCLC patients included within a training cohort (https://www.cbioportal.org/study/summary?id=sclc_ucologne_2015) (26). Microarray dataset GSE40275 was used to identify genes with different expression levels between SCLC and matching normal lung tissues (downloaded from the Gene Expression Omnibus (GEO) at https://www.ncbi.nlm.nih.gov/geo/). For the validation cohort, samples from 137 patients with biopsy-proven SCLC tissues were obtained from the National Cancer Center (NCC). The samples included archived formalin-fixed paraffin-embedded (FFPE) blocks and were originally collected from patients from January 2009 to November 2018. All patients had primary tumors at stages I–IV, and the diagnoses were independently reviewed by two expert pathologists from the NCC. The protocol of this study was approved by the Ethics Committee of the National Cancer Center/Cancer Hospital of the Chinese Academy of Medical Sciences. All participants provided written informed consent prior to completion of any study-related procedures. Detailed information about the samples is listed in Table 1.
RNA Extraction, cDNA Synthesis, and Quantitative Reverse-Transcriptase Polymerase Chain Reaction (qRT-PCR) Validation
Total RNA was extracted from SCLC tissue samples using the RNAiso Plus reagent (Takara, #9109) according to the manufacturer’s protocol. cDNA was synthesized from total RNA using the FastKing RT Kit (with gDNase) (Tiangen, KR116) in two steps. qRT-PCT was performed using the QuantiNova SYBR Green PCR Kit (Qiagen, 208054) with a reaction mixture containing 1 μl complementary DNA, 5 μl SYBR Green, 1.4 μl PCR primers (0.7 μl forward primer and 0.7 μl reverse primer), 1 μl QN ROX Reference Dye, and 1.6 μl RNase-Free water, totaling 10 μl in volume. GAPDH was selected as a control gene. Detailed sequences of the primer pairs are listed in Table S2. All data were log2-transformed, and expression levels were calculated using the 2-ΔΔCt method.
Functional Enrichment Analyses
To further explore the biological functions of TNF family-related genes, Gene Ontology (GO) and Kyoto Encyclopedia of Genes and Genomes (KEGG) analyses were carried out using DAVID 6.8 (http://david.abcc.ncifcrf.gov).
Gene Set Enrichment Analysis (GSEA)
GSEA was performed to interpret high-dimensional gene expression data (http://www.broadinstitute.org/gsea) (27). The enrichment score was the maximum distance from the middle of the ranking used to test gene set significance. An FDR<0.05 was regarded as indicating significant enrichment.
Gene Set Variation Analysis (GSVA)
GSVA is a non-parametric unsupervised method that allows gene set enrichment of expression data and gene sets in each sample (28). GSVA was implemented with the GSVA package (http://www.bioconductor.org) under R software version 3.5.1 to reveal the biological activity of selected signaling pathways over a sample population.
Estimate of Immune-Microenvironment and Tumor-Infiltrating Immune Cells
We used the ESTIMATE algorithm to infer the constituent cellular fraction, and calculated stromal and immune scores to assess tumor tissue purity (29). The proportion of immune cells in the TIME was quantitatively estimated by CIBERSORT (http://cibersort.stanford.edu/) based on gene expression profiles available from a public dataset. The LM22 signature algorithm containing 547 genes was used to distinguish 22 types of immune cells.
Statistical Analysis
Social demographics and clinicopathological features are presented as mean ± standard deviation or median (interquartile range) for continual variables and frequency (n) and proportion (%) for categorical variables. We used Kaplan-Meier survival analysis and least absolute shrinkage and selection operator (LASSO) Cox regression analysis to identify molecules of the TNF family with high prognostic value. The signature and risk formula were constructed using the selected genes through linear combination of gene expression levels. Patients were separated into high- or low-risk groups based on the optimal cutoff point. In the survival analysis, relapse-free survival (RFS) and OS were calculated using the Kaplan-Meier method, and between-group differences were compared using the log-rank test. Receiver operating characteristic (ROC) curves were used to show the predictive accuracy of the gene signature, and the performance of the model was determined based on the values of the area under the curve (AUC) and concordance index (C-index). Univariate and multivariable Cox proportional hazards regression models were used to determine whether the signature could independently predict the clinical outcomes of patients with SCLC after controlling for potential confounders. The relationship between TNF family molecules and immune checkpoints was also evaluated using a correlational analysis, in which correlation coefficients, CIs, and P values were generated. All statistical analyses were performed using R 3.5.1, and a two-tail P value <0.05 was considered statistically significant.
Results
The Expression Profiles of TNF Family Genes Display Significant Differences Between SCLC and Adjacent Normal Tissues
According to previous literature, we systematically explored the landscape of TNFSF/TNFRSF; 19 ligands and 29 receptors were included in our study. The interactions between ligands and receptors are summarized in Figure 1A. Firstly, we conducted a full assessment of different biological processes based on differentially expressed genes between SCLC samples and adjacent normal lung samples using GSEA. The results showed that the immune response was depressed in SCLC samples, especially T cell activation (Figure 1B). This result suggests that TNF family members—one of the most significant regulator families in T cell activation—may exhibit remarkable abnormality in SCLC patients. Next, we visualized the somatic mutation profile landscape of TNFSF/TNFRSF members in SCLC patients (Figure 1C). As depicted by the waterfall plot, we observed a mutation frequency of 20.91% (23/110), with non-synonymous mutations being the most common.
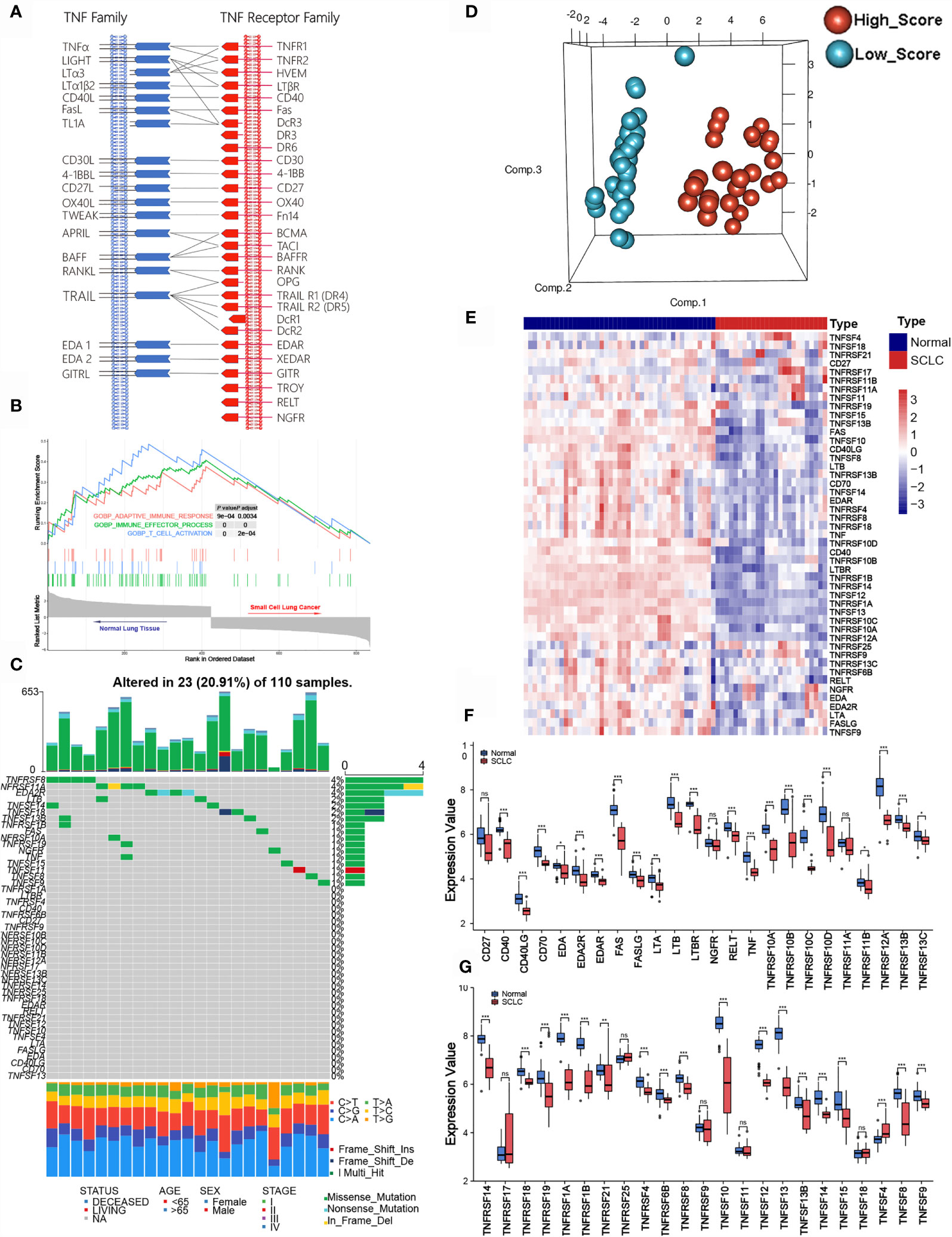
Figure 1 Molecular characteristic and expression profile of tumor necrosis factor (TNF) family members in small cell lung cancer. (A) Interaction between the ligands and receptors of the TNF family. Ligands and receptors are shown at the left (blue) and right (red) in the diagram. The vertical lines represent the cytoplasmic membrane of corresponding cells, and the horizontal lines with arrows represent ligand-receptor pairs. (B) GSEA of normal lung tissues and SCLC tissues. (C) The mutation landscape waterfall plot of TNFSF/TNFRSF members in 110 patients with SCLC from the training cohort. Each bar represents the mutation for each sample. (D) Principal component analysis (PCA) based on the expression levels of TNF family members between adjacent normal tissue and tumor tissue in the training set. (E) The expression details of TNF family members between SCLC samples and normal lung tissue. (F, G) Boxplots show differences in the expression values of TNF family members. *p < 0.05; **p < 0.01; ***p < 0.00; ns, no significance.
Next, we investigated whether the expression levels of TNF family molecules could be used to distinguish SCLC and normal tissue samples by principal component analysis (PCA), and our results indicated significant between-group heterogeneity (Figure 1D). Moreover, the heatmap showed low expression of the TNF family in SCLC samples (Figure 1E); detailed expression level information is displayed in the boxplots (Figures 1F, G). Additionally, we used Pearson correlation analysis to explore the relationships between TNFSF/TNFRSF members (Figure S1). Nearly all of the selected molecules were positively associated with each other, with the exceptions of TNFRSF25, TNFRSF11A, and TNFRSF19.
Identification of Prognostic TNF Family Genes in SCLC Patients
To identify clinically significant TNF family genes, we analyzed all TNFSF/TNFRSF members and selected 21 genes that were statistically associated with prognosis for the training cohort (Figures 2A, B and Table S1). Next, we used a LASSO Cox regression model to reduce dimensionality and identify the TNF family members with the greatest prognostic value. TNFRSF10B, CD40, TNFSF13B, TNFRSF21, TNFRSF25, TNFRSF1B, RELT, and TNFSF14 were selected by the model and used to construct a TNF family-based signature (Figures 2C, D). The weighting coefficients are presented in Figure 2E. The results indicated that TNFRSF10B, CD40, and TNFSF13B were risk factors for SCLC patients, while TNFRSF21, TNFRSF25, TNFRSF1B, RELT, and TNFSF14 were protective prognostic factors. The relationships between the TNF family-based signature and the eight genes are presented in Figure 2F.
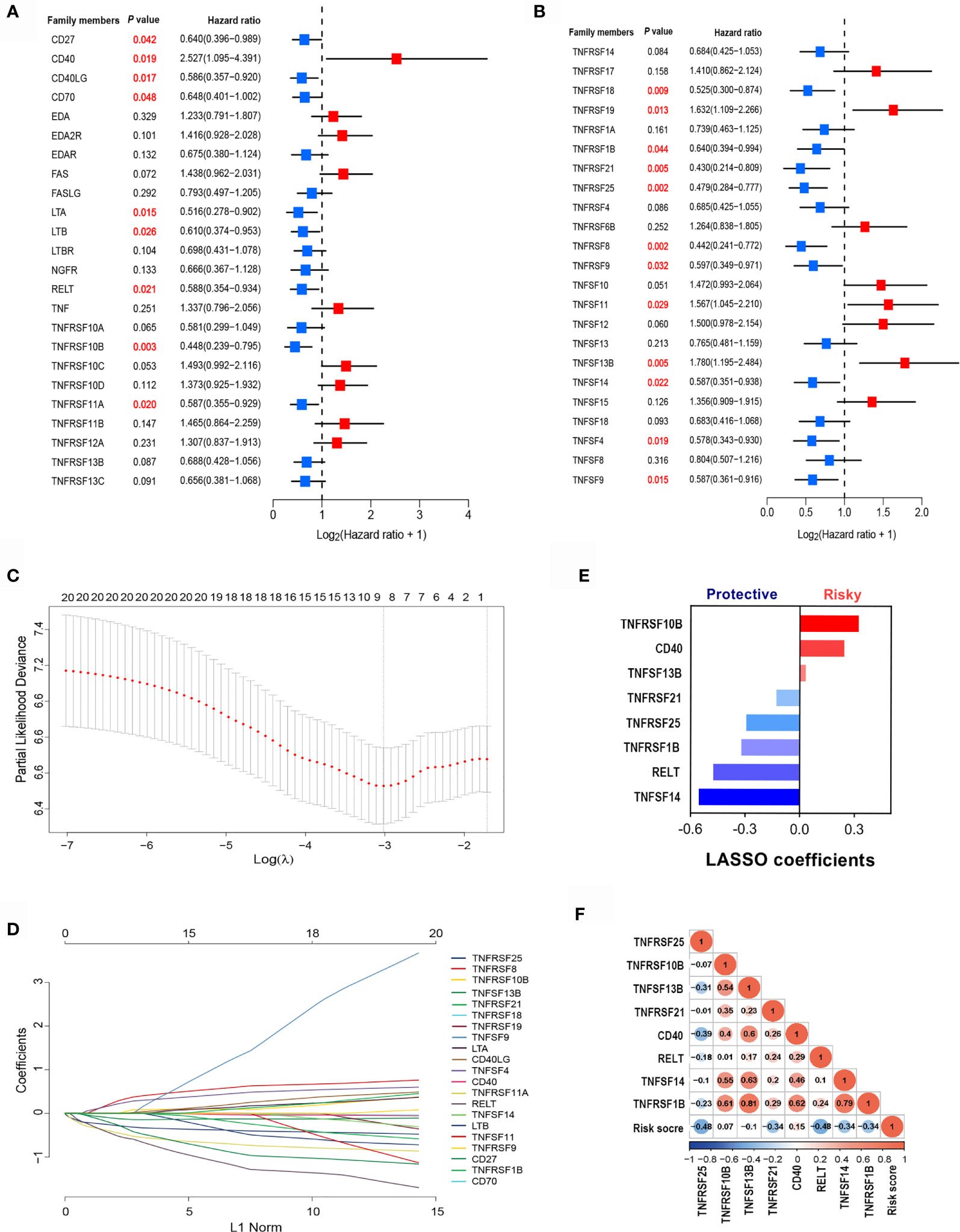
Figure 2 The clinical significance of TNF family members in SCLC patients. (A, B) The forest plots of hazard ratios (HRs) show the prognostic values of TNF members. (C) Tuning parameter (λ) selection cross-validation (n = 100) error curve for relevant prognostic genes. Red dots represent the partial likelihood deviance values, and grey lines represent the standard error (SE). The vertical lines are the optimal values according to the minimum and the 1-SE criteria. (D) The LASSO coefficient profiles of the most useful prognostic genes from the TNF family. (E) The weighting coefficient values for each prognostic gene. (F) Correlation matrix of the risk score and eight selected genes.
Construction and Validation of the TNF Family Based-Signature With a Training Cohort of SCLC Patients
The essential role of T cell-related TNF family molecules and their strong predictive value for SCLC inspired us to construct our prognostic model. We developed a TNF-family signature based on the expression levels of eight genes to predict OS for SCLC patients. The risk score was calculated as follows: risk score = (0.3224 × TNFRSF10B) + (0.2429 × CD40) + (0.0316 × TNFSF13B) + (-0.1292 × TNFRSF21) + (-0.2941 × TNFRSF25) + (-0.3208 × TNFRSF1B) + (-0.4752 × RELT) + (-0.5538 × TNFSF14). The risk score for individuals was calculated using this formula, and all patients with SCLC were separated into high- (n=45) and low-risk groups (n=32) using the optimal cutoff point. The distributions of risk scores and gene expression profiles are displayed in Figure 3A. PCA indicated obvious between-group heterogeneity (Figure 3B). Kaplan-Meier survival curves of OS revealed that high-risk patients had significantly worse OS (HR=3.38, 95% CI: 1.91, 5.97, P<0.001) (Figure 3C). We further evaluated the predictive performance of the signature using a time-dependent ROC curve; the AUCs were 0.798, 0.748, 0.72 after 1, 3, and 5 years, respectively (Figure 3D). Additionally, the predictive accuracy of the signature for 3-year survival was significantly better than that of several common clinicopathological parameters, including sex, age, smoking history, and cancer stage (Figure 3E). The C-index of the signature was as high as 0.845, indicating excellent discriminatory power (Figure 3F).
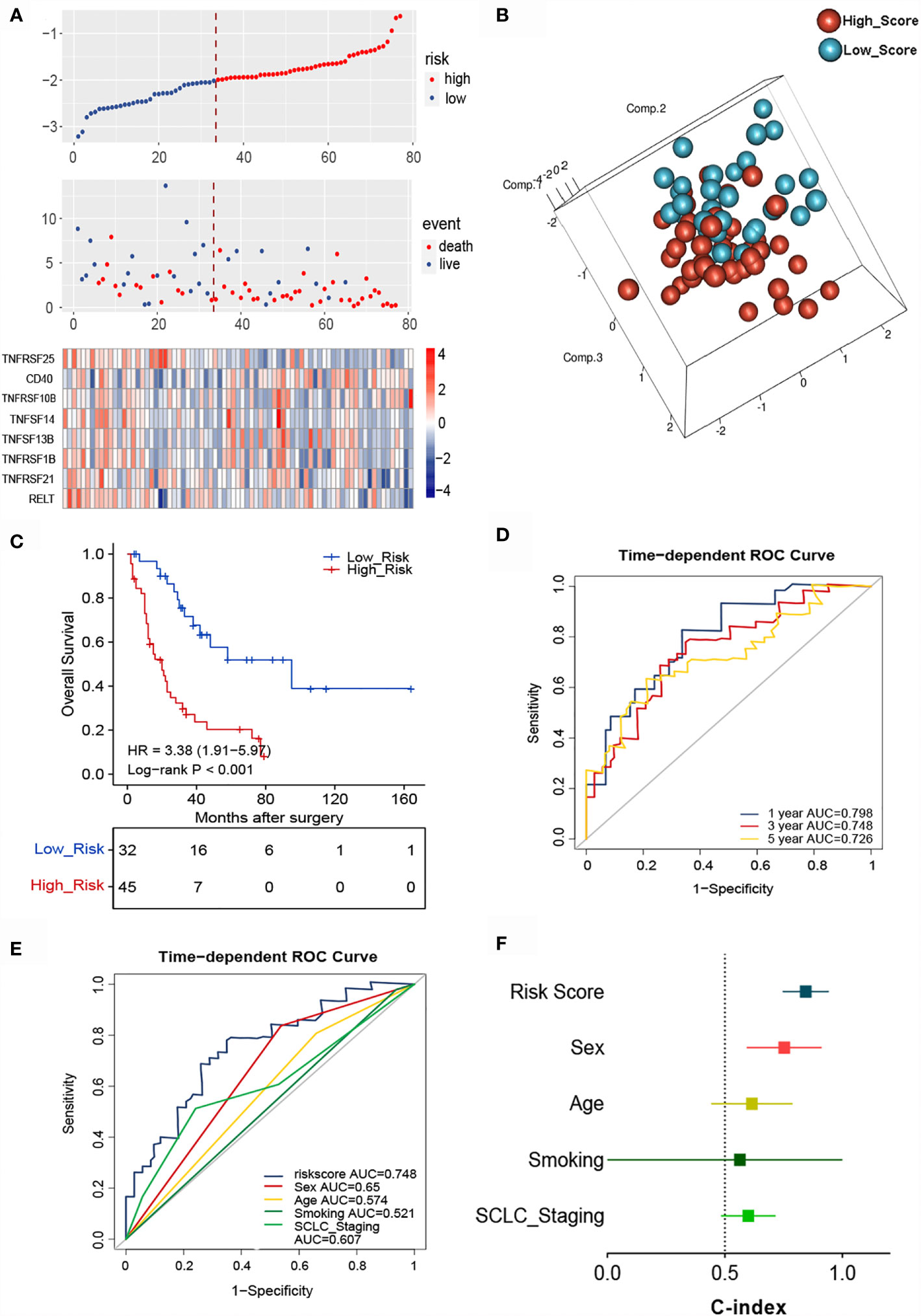
Figure 3 Identification of the TNF family-based signature in SCLCs from the training cohort. (A) The distribution of risk score, survival status, and TNF family gene expression profiles. (B) PCA of patients with SCLC based on the expression levels of the eight selected genes. (C) The Kaplan-Meier curve of OS for patients with SCLC based on the risk score. (D) Time-dependent ROC curves of the risk score for 1-, 3-, and 5-year OS prediction for the training cohort. (E) ROC analysis for prediction of 3-year survival compared with other clinicopathological factors. (F) C-indexes of models based on the risk score and other clinicopathological factors.
To determine whether the signature could independently predict the OS of patients with SCLC, we conducted univariate and multivariate Cox regression analyses using the training cohort. We confirmed that the risk score functioned as an independent risk factor (HR = 3.734, 95% CI: 1.871, 7.449, P<0.001) compared with other “traditional” clinical parameters like age, sex, smoking history, and tumor stage (Table 2).
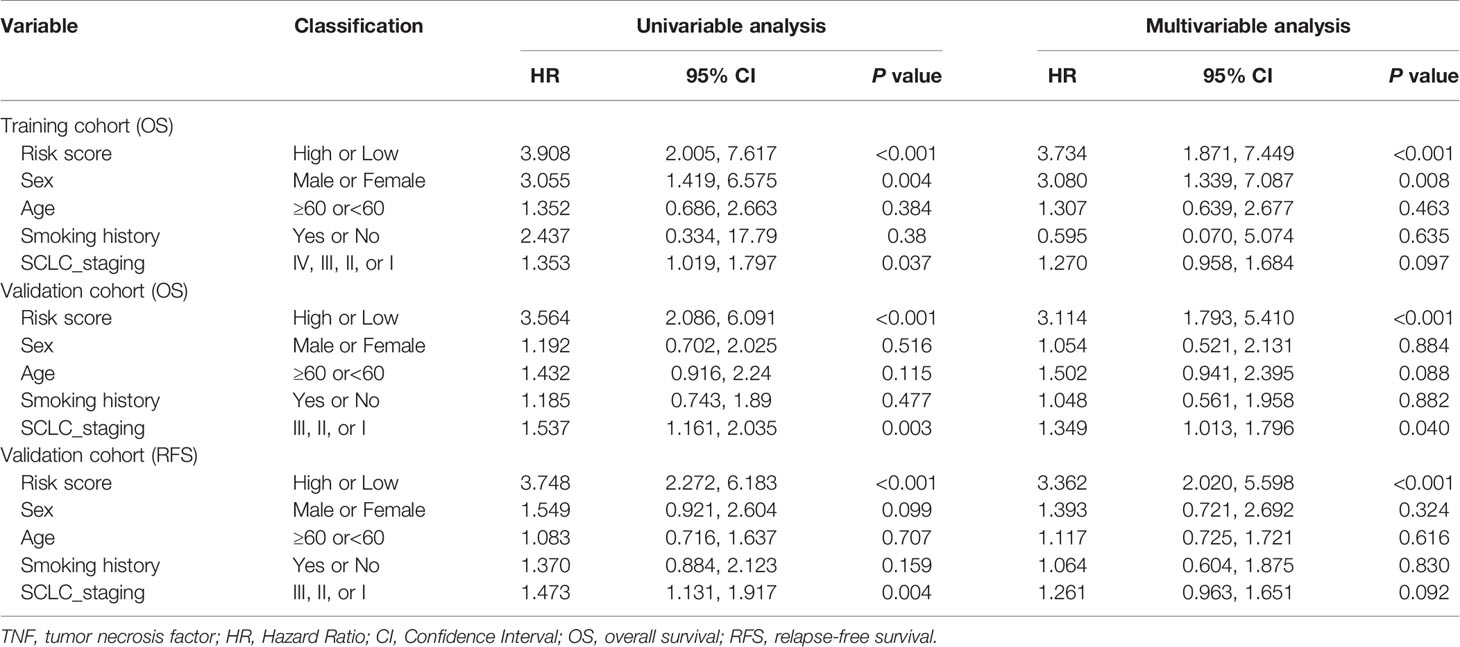
Table 2 Univariate and multivariable Cox regression analysis of TNF family-based signature and outcomes of OS and RFS in training cohort (N=77) and validation cohort (N = 137).
Validation of the Signature in a Validation Cohort of SCLC Patients
To confirm whether the TNF family-based signature derived from the training cohort was sufficiently robust for clinical application, we validated the signature in an independent validation cohort. The samples from the validation cohort were obtained from the NCC and consisted of 137 SCLC FFPE samples. We measured the gene expression levels of the FFPE samples using RT-qPCR, after which the patients’ risk scores were calculated, and patients were divided into high- (n=82) and low-risk (n=55) groups according to the optimal risk score cutoff point. As shown in Figure 4A, high and low-scoring patients had significantly different outcomes, with high-risk patients more often experiencing an unfavorable OS (HR=3.19, 95% CI: 2.05, 4.97, P<0.001). We also verified the signature’s discrimination and calibration using ROC curves and the C-index (Figures 4B–D). The signature exhibited good performance for predicting survival at 1, 3, and 5 years, with AUCs of 0.717, 0.667, and 0.702, respectively. In comparison, sex, age, smoking status, and cancer stage had AUCs of 0.509, 0.527, and 0.625, respectively. Similarly, patients with a low risk score had a longer period of RFS in comparison with those with a high score (HR=3.29, 95% CI: 2.18, 4.97, P<0.001) (Figure 4E). Subsequently, ROC and C-index analyses confirmed the robustness of the risk score (Figures 4F–H). The multivariate analysis indicated that the risk score was independently correlated with OS (HR=3.114, 95% CI: 1.793, 5.410, P<0.001) and RFS (HR=3.362, 95% CI: 2.020, 5.598, P<0.001). These results confirm that our novel signature is a reliable and effective tool for SCLC prognosis prediction (Table 2).
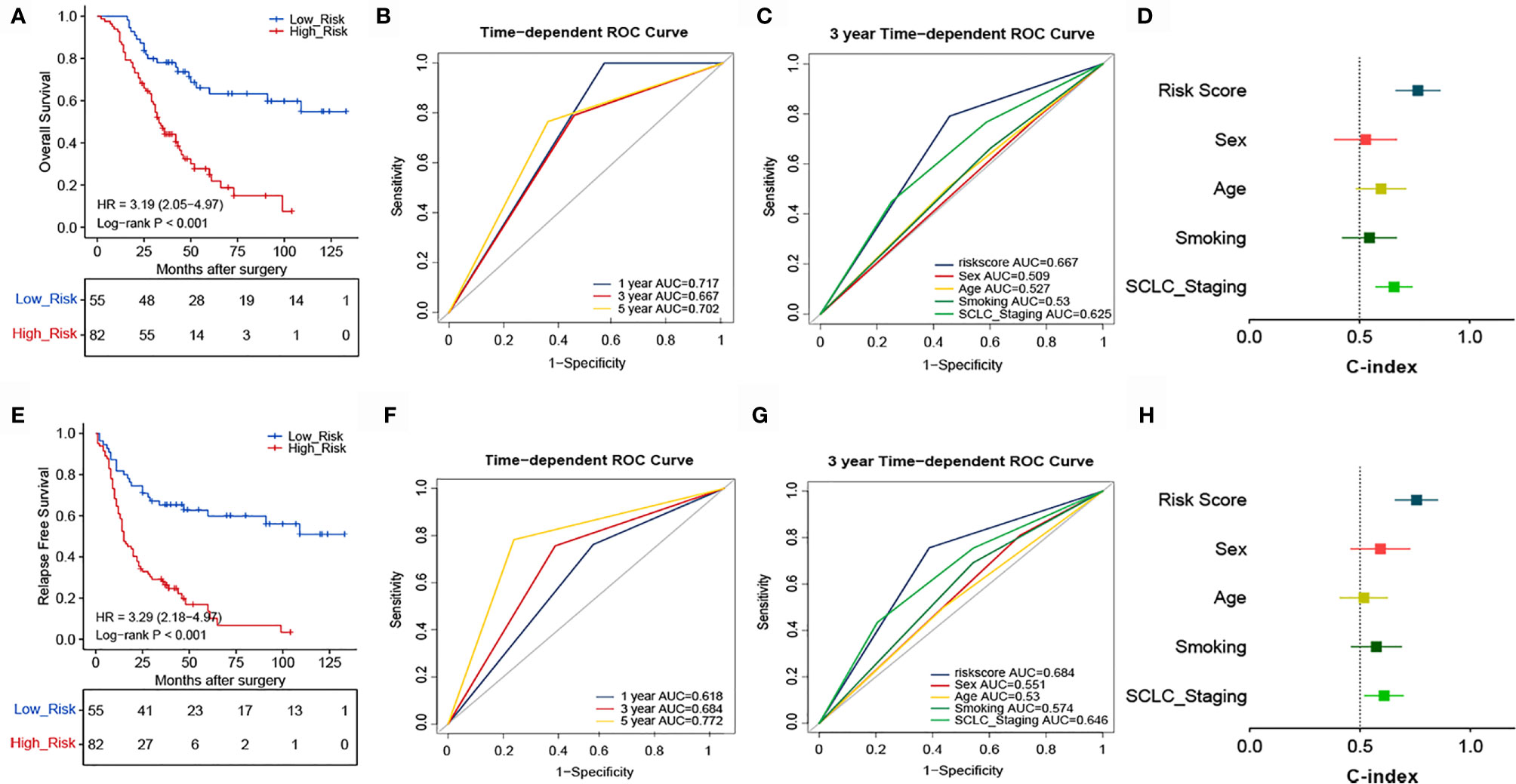
Figure 4 Validation of the prognostic performance of the TNF family based-signature in the validation cohort. (A, E) Kaplan-Meier curves of OS and RFS according to risk-score groups in the validation cohort. (B, F) ROC curves of the TNF family-based signature for predicting OS and RFS after 1, 3, and 5 years. (C, G) ROC curves of the TNF family-based signature for predicting 3-year outcomes of OS and RFS. (D, H) C-index for the predictive performance of the signature for OS and RFS.
Evaluation and Validation of the TNF Family-Based Signature in Different Clinical Subsets
To further explore the applicability of the TNF-family-based signature in different clinical settings, we analyzed its predictive efficiency for different clinical subgroups based on age, sex, and smoking status. The results indicated that high-risk patients in nearly all subgroups showed statistically significant worse OS, with the exception of non-smokers (Figures S2A-F). As expected, regarding sex, age, and smoking status, the signature remained an independent prognostic biomarker for the prediction of OS and RFS for patients with SCLC in the validation cohort (Figures S2G-Q).
Predicting ACT Benefit in Patients With SCLC
Considering that the TIME is an essential factor affecting the efficacy of chemotherapy, we determined whether the risk score could predict the benefit of ACT in patients with SCLC. The association between risk score and chemotherapy benefit was analyzed among patients who received ACT. In the training cohort, patients were classified into high- (n=27) and low-risk (n=23) groups according to the optimal cutoff point of the risk score. There were significant between-group differences in the Kaplan-Meier survival curves, suggesting that the OS of high-risk patients was worse than that of low-risk patients (Figure 5A). The ROC analysis showed that the 1-, 3-, and 5-year AUCs were 0.735, 0.727, and 0.653, respectively (Figure 5B). These findings indicate that our novel signature demonstrated good accuracy for predicting the prognosis of SCLC patients. The AUC of the risk score (AUC=0.727) was higher than that of other common clinicopathological parameters like sex (AUC=0.615), age (AUC=0.527), smoking status (AUC=0.503), or cancer stage (AUC=0.693) (Figure 5C). The novel signature also predicted ACT benefit in the validation cohort. Patients were divided into high- (n=49) and low-risk (n=69) groups, and high-risk patients demonstrated worse OS (Figure 5E) and RFS (Figure 5I). In addition, the ROC curves for different survival years and various clinical factors also confirmed the predictive value of the signature for ACT response (Figures 5F, G, J, K). The C-indexes of the training and validation cohorts also demonstrated the accuracy and discriminatory power of our signature (Figures 5D, H, L).
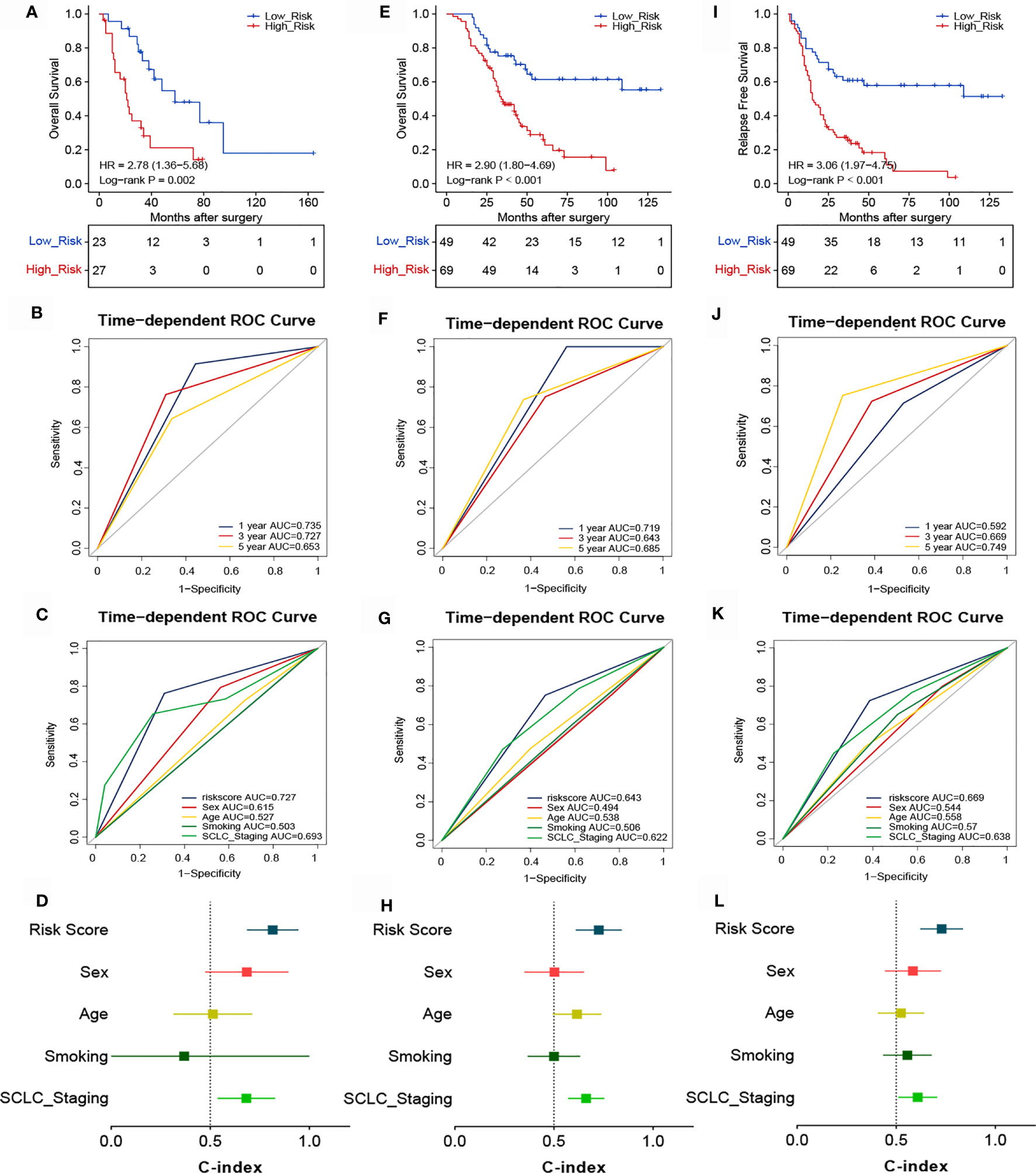
Figure 5 Association between risk score and the survival benefit of adjuvant chemotherapy in patients with SCLC. Kaplan-Meier (A) and ROC analyses of OS according to different risk groups in the training cohort (B, C). (D) C-indexes for evaluating the performance of the signature for predicting the OS of the training cohort. Kaplan-Meier (E) and ROC analyses of OS according to different risk groups in the validation cohort (F, G). (H) C-indexes for evaluating the performance of the signature for predicting the OS of the validation cohort. Kaplan-Meier (I) and ROC analyses of RFS according to different risk groups in the validation cohort (J, K). (L) C-indexes for evaluating the performance of the signature for predicting the RFS of the validation cohort.
Biological Pathway and Inflammatory Response Analysis of the TNF Family-Based Signature
The ability of the TNF family-based signature to predict SCLC prognosis inspired our subsequent exploration of signature-related biological pathways. We first selected genes that were closely related to the signature (Pearson |R| > 0.40), and 172 positive and 525 negative genes were filtered out (Figure 6A). Next, GO and KEGG analyses were performed to determine the biological roles of the selected genes. The genes in the TNF family-based signature tended to be involved in immune-specific pathways, especially the T cell-related immune response (Figures 6B, C). We also determined the association between the risk score and inflammatory activity using a seven-metagene cluster (consisting of genes that are highly correlated and provide robust clustering of samples). The expression patterns and risk scores are presented in Figure 6D. In addition, after subjecting the selected genes and metagene cluster to gene set enrichment analysis (GSVA), we found that the risk score was negatively related to LCK, MHC-I, and MHC-II. These results suggest that high-risk patients are more likely to show immunosuppression (Figure 6E).
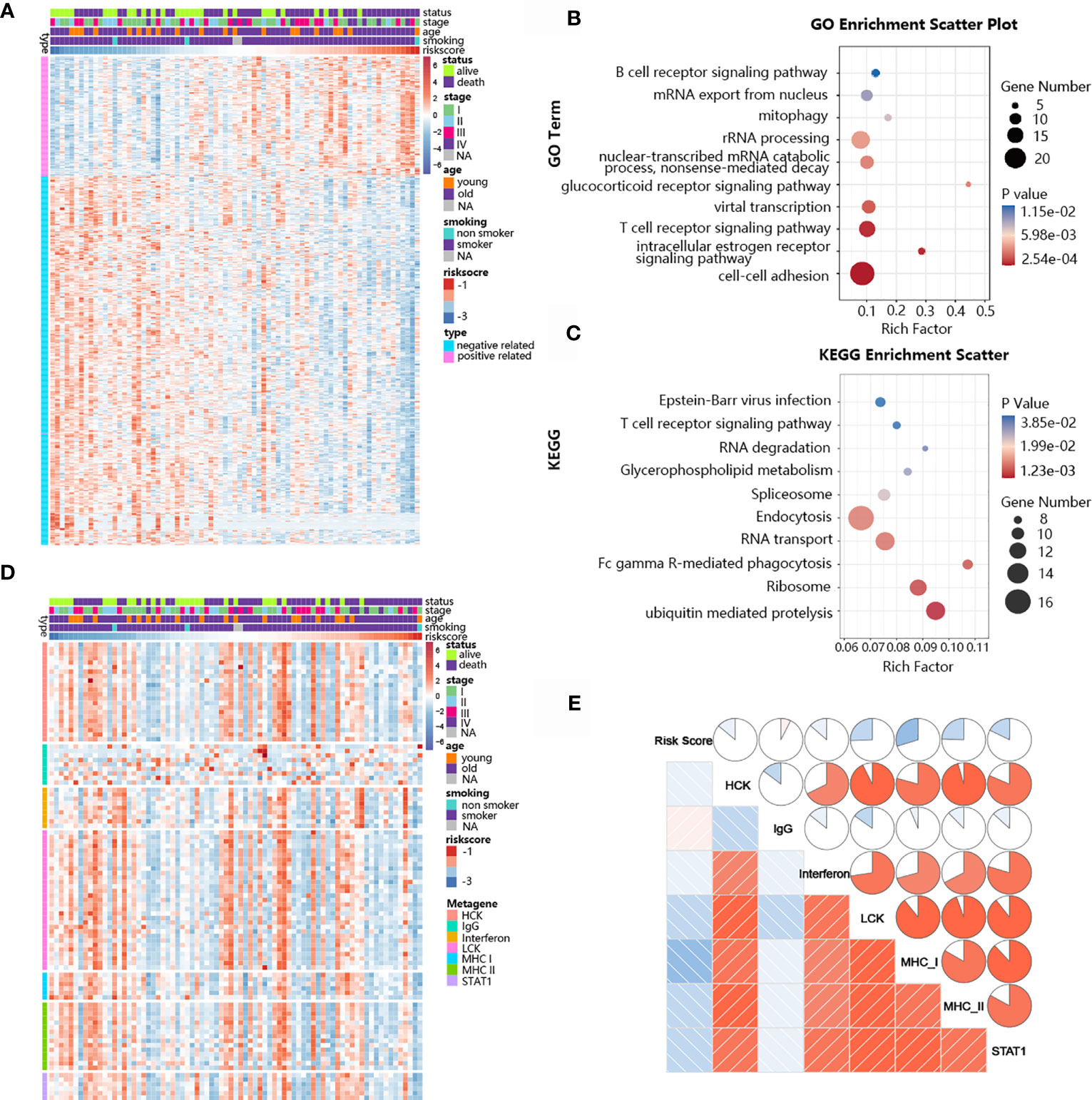
Figure 6 Biological pathways and inflammatory activities of the TNF family-based signature in SCLC from GO and KEGG pathway analyses. (A) The most highly associated genes from the TNF family based-signature in SCLC patients from training cohort. (B) Gene enrichment with GO terms of the identified genes. (C) Gene enrichment with KEGG terms of the identified genes. (D) Heatmap of the expression profiles of seven metagenes. (E) Correlations between the risk score and seven clusters of metagenes.
Immune Cell Infiltration and Immune Checkpoints Associated With the Risk Score
Immune cells and the TNF family play vital roles in immune responses and inflammatory reactions. Considering that the immune score and tumor purity have immunotherapeutic implications for cancer patients, we analyzed the relationship between the risk score and immune infiltration status by determining the ESTIMATE score, immune score, stromal score, and tumor purity for the high-risk and low-risk groups. As shown in Figure 7A, the high-risk group had lower stromal and immune scores in comparison with the low-risk group, while the former group had greater SCLC tumor purity. We further evaluated the immune infiltration of 22 types of immune cells using CIBERSORT. The relative proportion and composition of immune cells were determined for the high- and low-risk groups of the training cohort, which was stratified using the TNF family based-signature (Figure 7B). The density plots showed that the low-risk and high-risk groups had different immune landscapes. For example low-risk patients exhibited significantly greater infiltration of CD8+ T cells (P= 0.0166) (Figures 7C–F).
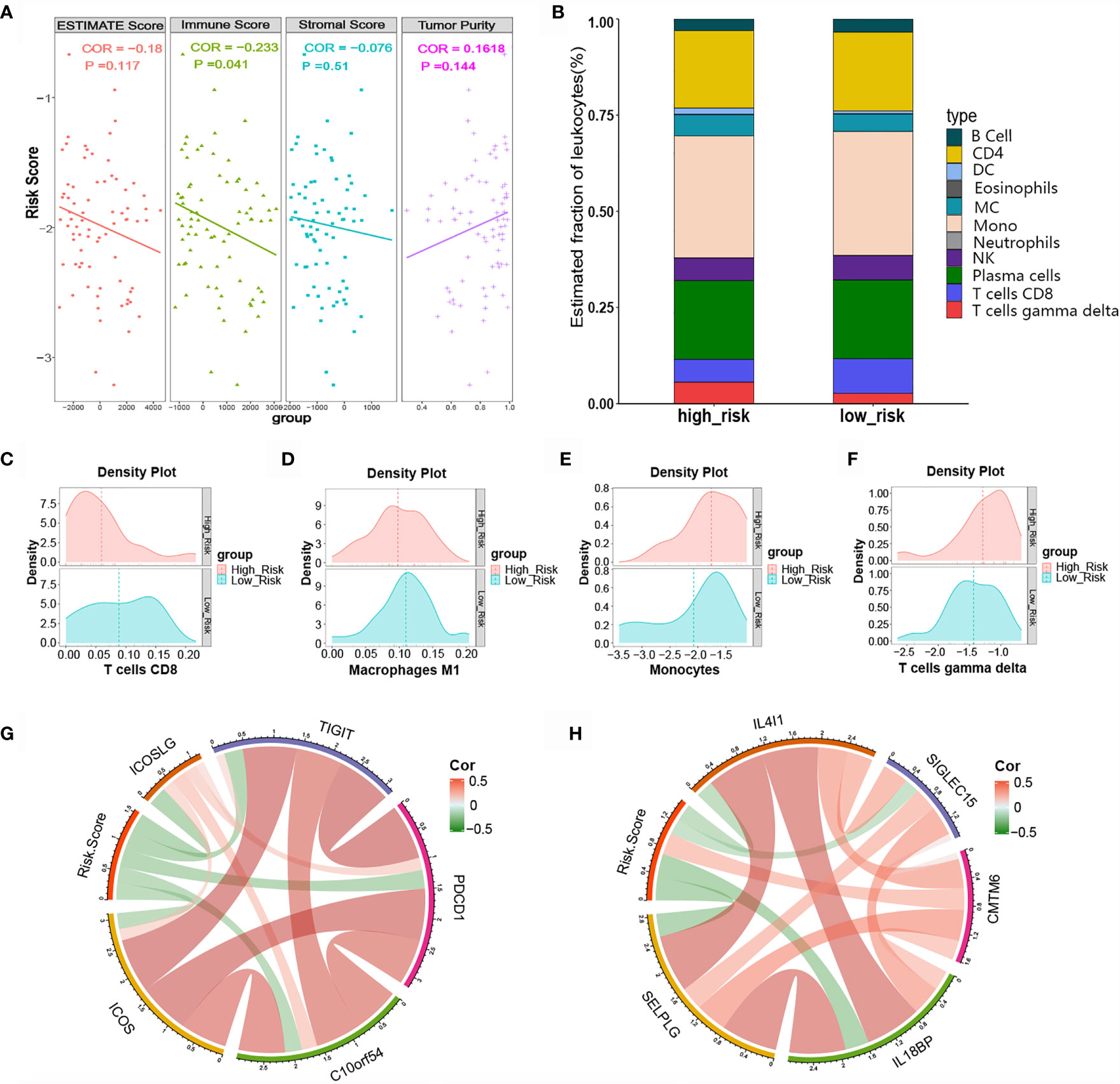
Figure 7 Relationship between the risk score and tumor-infiltrating immune cells as well as immune checkpoints. (A) ESTIMATE score, immune score, stromal score, and tumor purity in high- and low-risk patients. (B) Difference in tumor-infiltrating immune cells between the high- and low-risk groups. (C-F) Difference in immune cell infiltration abundance between the high- and low-risk groups. (G, H) Correlation chord chart showing the correlations between risk scores and other immune checkpoint molecules.
Various novel immune checkpoint molecules have been identified recent years; however, the clinical effects of ICIs, like monoclonal antibodies targeting PD-1 and PD-L1, remain unsatisfactory for SCLC patients. We therefore explored the association between the TNF family based-signature and other classic and novel immunotherapy checkpoint targets. The correlation chord chart showed that the risk score was negatively correlated with the expression levels of immune checkpoints ICOS, ICOSLG, TIGIT, PDCD1, B7-H5, IL4l1, SIGLEC15, IL18BP, and SELPLG (Figure 7G). Of note, the risk score was significantly positively correlated with the expression level of CKLF-like MARVEL transmembrane domain-containing 6 (CMTM6), a potential immunotherapy target for high-risk patients (Figure 7H).
Discussion
Due to rapid growth, early metastasis and limited treatment options, SCLC is one of the most lethal tumors. Chemotherapy remains the key backbone of SCLC treatment, and accurate prediction of prognosis and treatment response would surely help to further optimize the choice of personalized treatment and prognosis management for SCLC. Recently, the field of cancer genomics has developed rapidly, and various novel therapeutic targets and prognostic markers have been discovered for multiple types of malignant tumors. Unfortunately, the relative scarcity of tumor tissue samples has limited investigations of potential predictive markers for treatment response that could be applied in clinical settings to patients with SCLC. Immunotherapy has achieved success as a treatment for patients with many different types of tumors, but its efficacy has been limited in SCLC patients (7). Considering the limited knowledge of immune heterogeneity and the anti-tumor status of the TIME of SCLC, exploration of immune mechanisms and TIME regulators are essential for the development of improved treatments for SCLC patients.
The TNF family is expressed in nearly all cells and regulates a wide range of cellular activities, including cell survival, tissue remodeling, and immunity (30). Notably, TNF family members are immunoregulators that play pivotal roles in immune responses, particularly in co-stimulation of T cell responses (31, 32). TNF receptors are implicated in immune surveillance in pancreatic β cell cancer (33). TNF is also present in the TIME of many cancers, where it is thought to enhance cancer growth. TNF produced by myeloid cells was found to promote inflammation-associated tumors (34), and TNF derived from macrophages was implicated in inflammation and subsequent tumor development (35, 36). In addition, TNF in the TIME can cause genetic damage and have other direct effects on malignant cells; for instance, TNF in the TIME can induce tumor cells to undergo the epithelial-mesenchymal transition (EMT), thus facilitating metastasis (37–39). Before these mechanisms were fully appreciated, clinical trials of TNF were initiated, but these trials failed due to severe toxicity (40–43). To move forward with the clinical application of TNF treatment, additional studies are needed to illuminate the roles of TIME-derived TNF in human cancers and its relative importance in tumor prognosis and treatment for early and late stage SCLC patients.
This study was the first retrospective study of SCLC patients to construct an immune-specific signature based on TNF family members for prognosis and treatment benefit prediction. The eight-gene TNF-signature was constructed using a training cohort and verified with qPCR using FFPE specimens from a validation cohort. The signature was an independent prognostic factor for patients with SCLC and showed precise predictive value in several distinct clinical subgroups. Notably, the signature was also useful for predicting chemotherapy response. Our exploration of the association between the signature and immune-inflammatory responses showed that tumor-infiltrating CD8+ T cells were predominant in low-risk patients. Additionally, the risk score was positively associated with other novel immune checkpoints, suggesting that high-risk patients were more likely to benefit from ICI-based therapies.
TNF family members are promising therapeutic targets for reversing tumor immunosuppression, enhancing host antitumor immune response, and improving clinical outcomes for patients with SCLC (44). To better understand the TNF/TNFR family profiles of SCLC patients, 19 ligands and 29 receptors were selected and analyzed in our study. We compared the TNF profiles of SCLC samples and adjacent tissues, and we found that TNF family members were expressed at significantly lower levels in tumor tissues, suggesting that significant heterogeneity exists between tumor tissue and normal tissues. Based on this heterogeneity, a TNF family-based prognostic predictive signature was constructed, including TNFRSF25, CD40, TNFRSF10B, TNFSF14, TNFSF13B, TNFRSF1B, TNFRSF21, and RELT, and the signature was shown to possess excellent predictive value for predicting the prognosis of SCLC patients.
TNFRSF25 (DR3), a cell surface receptor, is mainly expressed by T cells, for which it mediates apoptotic signaling and differentiation (45). TNFRSF25 also enhances the CD4+ T cell response and promotes CD8+ T cell responses and antitumor immunity (46). TNFRSF1B (CD120b) is a membrane receptor that binds TNFα. TNFα is a proinflammatory cytokine that is synthesized by activated macrophages (47). Altered gene and/or protein expression of TNFRSF1B may be a prognostic biomarker for patients with NSCLC (48). CD40, also known as TNFRSF5,regulates cellular and humoral immunity, but the specific physiological functions of CD40 are dependent on cell type and the microenvironment. CD40 may be an effective prognostic marker for lung cancer (49). TNFRSF10B (DR5) is primarily localized on the plasma membrane, where it functions as an apoptotic receptor to induce cell apoptosis. A DR5-targeting antibody has been developed and tested in clinical trials as a therapeutic intervention for cancer patients (50, 51). TNFSF14 (LIGHT, HVEML, CD258), a secreted protein, is a highly effective stimulator of antitumor immune responses, which also influences the plasticity of the TIME (52). TNFSF14 binds the decoy receptor TNFRSF14 to deliver costimulatory signals to T cells (53). TNFSF14 is a promising cancer immunotherapy target that appears to moderate survival and apoptosis in lymphocytes and tumor cells (52). TNFSF13B (BAFF, CD257) is a cytokine with three receptors: BAFF-R, TACI, and BCMA (54). BAFF may influence the maturation, proliferation, and class switching of B cells (55). A high expression level of BAFF may lead to increased lung inflammation and worsened alveolar wall destruction (56). TNFRSF21 (DR6) is an extensively post-translationally modified type I transmembrane protein that acts as a death domain-containing receptor (57). DR6 is expressed ubiquitously in most human tissue types, and particularly in the heart, brain, pancreas, lymphoid organs, as well as by non-lymphoid cancer cell lines (58). High expression of DR6 is associated with elevated levels of anti-apoptosis molecules, and it contributes to tumor cell survival and immune evasion (59). TNFRSF19L (RELT), a type I transmembrane glycoprotein, predominates in immune cells and lymphoid tissues (60). High expression of RELT in epithelial cells induces cell death (61), and RELT-knockdown mice demonstrated negative regulation of the early T cell response phase (62). These findings illustrate that high RELT expression down-regulates immune responses.
After exploring the functions of the eight genes comprising the TNF signature, we verified its robustness by validating it using a large SCLC cohort with FFPE specimens and clinical subgroups. The results of the validation analysis confirmed the high predictive value and accuracy of the TNF family-based signature. These findings inspired us to explore the underlying mechanisms of action and elucidate the role of these genes in SCLC biology. Functional annotation of TNFSF/TNFRSF genes revealed that the signature was closely associated with immune-related processes. Considering the role of the TIME, the signature-related immune landscape was also investigated. Noticeably, we found that low-risk patients showed significantly more infiltrated CD8+ T cells, which are crucial for antitumor immunity (63). The correlations between the signature and seven metagenes were analyzed to provide additional insight into the mechanisms underlying inflammation and immune responses in SCLC patients (64). The risk score was negatively associated with monocyte/myeloid lineage-specific functions and the antigen-presenting process of T cells, thereby revealing the immunosuppressed status of high-risk patients.
In addition, the TNF family-based signature was confirmed as an independent prognostic indicator that effectively stratified patients into high- and low-risk groups with distinct prognosis and survival. Low-risk patients had more favorable outcomes than their high-risk counterparts, largely because they had a greater number of tumor-infiltrating CD8+ T cells. In agreement with our results, CD8+ T cell infiltration has been shown to be an independent positive prognostic factor that is strongly associated with better outcomes in patients with lung cancer (65), colorectal cancer (66), esophageal cancer (67), and other cancers. Noticeably, our signature can be used to predict the ACT response of SCLC patients; low-risk patients derived a greater benefit from ACT. These results were slightly consistent with prior studies (68, 69). The proportion of tumor-infiltrating CD8+ T cells was statistically higher in the low-risk group. Previously published evidence suggests that tumor-infiltrating cells have profound effects on chemotherapy efficacy against various tumors (70). CD8+ T cells are important tumor-infiltrating lymphocytes that play a vital role in antitumor immunity (71). Thus, chemotherapy may induce antitumor immunity by triggering tumor-infiltrating immune cells (72).
ICIs have shown therapeutic potential in several malignancies, including SCLC (73). The expression levels of immune checkpoints can predict the therapeutic response to immunotherapy. Well-studied biomarkers like PD-L1 and TMB are not sufficient to predict the prognosis of SCLC patients. Therefore, novel immunotherapeutic targets are needed to overcome this limitation, and immune checkpoints are being actively researched (74). Our analysis of the correlations between our TNF family-based signature and classical and novel immune checkpoints revealed that the risk score of SCLC patients was positively associated with the expression level of CMTM6. CMTM6, a member of the CMTM family, is a novel immune checkpoint that is localized at the plasma membrane of various cells. Previous studies have shown that CMTM6 influences the maintenance of cancer stem cells and the EMT (75). In addition, depletion of CMTM6 delays tumor growth, enhances tumor-specific T cell activity and reduces the number of exhausted T cells (75). CMTM6 plays an essential role in maintaining cell surface PD-L1 protein stability by preventing it from undergoing ubiquitin-mediated degradation (76). Therefore, CMTM6 may be a useful immunotherapy, especially for patients with resistance to anti-PD-1/PD-L1 treatments. Interestingly, our study suggested that our signature was positively associated with the expression level of CMTM6, but it had no significant correlation with the expression level of PD-L1. There are several potential explanations for this finding. Firstly, PD-L1 expression is usually low or absent in SCLC (77, 78). In the present study, the PD-L1 expression level of the selected samples was too low to allow correlational analysis with the TNF family-based signature. Secondly, CMTM6 regulates PD-L1 at the protein level instead of the mRNA level, so CMTM6 was not expected to affect PD-L1 mRNA expression. CMTM6 is not a general regulator of protein translation or stability (79). We focused on the transcriptional level in this study, so any effects of CMTM6 at the protein level were outside its scope. Thirdly, the effects of CMTM6 are not limited to PD-L1, and its effects in the TIME may be mediated by other mechanisms. The results described above suggest that CMTM6 may be a target for SCLC immunotherapy, especially for high-risk patients with few therapeutic options.
Several limitations of the study should be considered. Firstly, patients and clinicians mainly selected an ACT approach in real-life clinical practice settings, and no random group assignment scheme was used. Secondly, the predictive capacity of the eight-gene-related signature may not be stable within the TIME because it is a heterogeneous mixture. Finally, the study cases were collected retrospectively, and the predictive value of the signature for ACT requires further validation in large, well-designed, and prospective trials.
In conclusion, our study was the first full-scale study of the expression patterns and clinical relevance of TNF family molecules in SCLC. Our novel TNF family-based signature independently predicted the prognosis of patients with SCLC and was a useful tool for evaluating the survival benefit from ACT. Analysis of similar genetic expression patterns may be the best way to advance prognostication and the development of personalized precision medicine approaches for patients with SCLC.
Data Availability Statement
The datasets presented in this study can be found in online repositories. The names of the repository/repositories and accession number(s) can be found in the article/supplementary material.
Ethics Statement
The studies involving human participants were reviewed and approved by National Cancer Center/Cancer Hospital of the Chinese Academy of Medical Sciences. The patients/participants provided their written informed consent to participate in this study. Written informed consent was obtained from the individual(s) for the publication of any potentially identifiable images or data included in this article.
Author Contributions
NS and JH supervised the project, designed the experiments, edited the manuscript, and led the experiments. ZZ, CZ, and PW conducted the experiments and data analysis. ZZ, PW, and CZ prepared all figures and tables. ZZ and PW drafted the manuscript. CZ, GZ, YL, QZ, LW, and ZY collected clinical samples. All authors contributed to the article and approved the submitted version.
Funding
This work was supported by the CAMS Innovation Fund for Medical Sciences (2017-I2M-1-005), the National Key R&D Program of China (2016YFC1303201), the National Natural Science Foundation of China (81802299, 81502514), the Fundamental Research Funds for the Central Universities (3332018070), and the National Key Basic Research Development Plan (2018YFC1312105).
Conflict of Interest
The authors declare that the research was conducted in the absence of any commercial or financial relationships that could be construed as a potential conflict of interest.
Publisher’s Note
All claims expressed in this article are solely those of the authors and do not necessarily represent those of their affiliated organizations, or those of the publisher, the editors and the reviewers. Any product that may be evaluated in this article, or claim that may be made by its manufacturer, is not guaranteed or endorsed by the publisher.
Acknowledgments
All authors would like to thank the donors of the specimens used in this study and the research groups who provided data. All authors would also like to thank CapitalBio Technology for their kind help.
Supplementary Material
The Supplementary Material for this article can be found online at: https://www.frontiersin.org/articles/10.3389/fimmu.2021.745769/full#supplementary-material
References
1. van Meerbeeck JP, Fennell DA, De Ruysscher DK. Small-Cell Lung Cancer. LANCET (2011) 378:1741–55. doi: 10.1016/S0140-6736(11)60165-7
2. Byers LA, Rudin CM. Small Cell Lung Cancer: Where do We Go From Here? CANCER-AM Cancer Soc (2015) 121:664–72. doi: 10.1002/cncr.29098
3. Gazdar AF, Bunn PA, Minna JD. Small-Cell Lung Cancer: What We Know, What We Need to Know and the Path Forward. Nat Rev Cancer (2017) 17:725–37. doi: 10.1038/nrc.2017.87
4. Spigel DR, Socinski MA. Rationale for Chemotherapy, Immunotherapy, and Checkpoint Blockade in SCLC: Beyond Traditional Treatment Approaches. J Thorac Oncol (2013) 8:587–98. doi: 10.1097/JTO.0b013e318286cf88
5. Stinchcombe TE, Gore EM. Limited-Stage Small Cell Lung Cancer: Current Chemoradiotherapy Treatment Paradigms. ONCOLOGIST (2010) 15:187–95. doi: 10.1634/theoncologist.2009-0298
6. Ramalingam SS. Small-Cell Lung Cancer: New Directions for Systemic Therapy. J Oncol Pract (2016) 12:119–20. doi: 10.1200/JOP.2015.009225
7. Pavan A, Attili I, Pasello G, Guarneri V, Conte PF, Bonanno L. Immunotherapy in Small-Cell Lung Cancer: From Molecular Promises to Clinical Challenges. J Immunother Cancer (2019) 7:205. doi: 10.1186/s40425-019-0690-1
8. Herbst RS, Baas P, Kim DW, Felip E, Pérez-Gracia JL, Han JY, et al. Pembrolizumab Versus Docetaxel for Previously Treated, PD-L1-Positive, Advanced Non-Small-Cell Lung Cancer (KEYNOTE-010): A Randomised Controlled Trial. LANCET (2016) 387:1540–50. doi: 10.1016/S0140-6736(15)01281-7
9. Ricciuti B, Kravets S, Dahlberg SE, Umeton R, Albayrak A, Subegdjo SJ, et al. Use of Targeted Next Generation Sequencing to Characterize Tumor Mutational Burden and Efficacy of Immune Checkpoint Inhibition in Small Cell Lung Cancer. J Immunother Cancer (2019) 7:87. doi: 10.1186/s40425-019-0572-6
10. Coulie PG, Van den Eynde BJ, van der Bruggen P, Boon T. Tumour Antigens Recognized by T Lymphocytes: At the Core of Cancer Immunotherapy. Nat Rev Cancer (2014) 14:135–46. doi: 10.1038/nrc3670
11. Tian Y, Zhai X, Han A, Zhu H, Yu J. Potential Immune Escape Mechanisms Underlying the Distinct Clinical Outcome of Immune Checkpoint Blockades in Small Cell Lung Cancer. J Hematol Oncol (2019) 12:67. doi: 10.1186/s13045-019-0753-2
12. Horn L, Mansfield AS, Szczęsna A, Havel L, Krzakowski M, Hochmair MJ, et al. First-Line Atezolizumab Plus Chemotherapy in Extensive-Stage Small-Cell Lung Cancer. N Engl J Med (2018) 379:2220–9. doi: 10.1056/NEJMoa1809064
13. Paz-Ares L, Dvorkin M, Chen Y, Reinmuth N, Hotta K, Trukhin D, et al. Durvalumab Plus Platinum-Etoposide Versus Platinum-Etoposide in First-Line Treatment of Extensive-Stage Small-Cell Lung Cancer (CASPIAN): A Randomised, Controlled, Open-Label, Phase 3 Trial. LANCET (2019) 394:1929–39. doi: 10.1016/S0140-6736(19)32222-6
14. Rudin CM, Awad MM, Navarro A, Gottfried M, Peters S, Csőszi T, et al. Pembrolizumab or Placebo Plus Etoposide and Platinum as First-Line Therapy for Extensive-Stage Small-Cell Lung Cancer: Randomized, Double-Blind, Phase III KEYNOTE-604 Study. J Clin Oncol (2020) 38:2369–79. doi: 10.1200/JCO.20.00793
15. Gadgeel SM, Pennell NA, Fidler MJ, Halmos B, Bonomi P, Stevenson J, et al. Phase II Study of Maintenance Pembrolizumab in Patients With Extensive-Stage Small Cell Lung Cancer (SCLC). J Thorac Oncol (2018) 13:1393–9. doi: 10.1016/j.jtho.2018.05.002
16. Reck M, Luft A, Szczesna A, Havel L, Kim SW, Akerley W, et al. Phase III Randomized Trial of Ipilimumab Plus Etoposide and Platinum Versus Placebo Plus Etoposide and Platinum in Extensive-Stage Small-Cell Lung Cancer. J Clin Oncol (2016) 34:3740–8. doi: 10.1200/JCO.2016.67.6601
17. Binnewies M, Roberts EW, Kersten K, Chan V, Fearon DF, Merad M, et al. Understanding the Tumor Immune Microenvironment (TIME) for Effective Therapy. Nat Med (2018) 24:541–50. doi: 10.1038/s41591-018-0014-x
18. Vadakekolathu J, Minden MD, Hood T, Church SE, Reeder S, Altmann H, et al. Immune Landscapes Predict Chemotherapy Resistance and Immunotherapy Response in Acute Myeloid Leukemia. Sci Transl Med (2020) 12(546):eaaz0463. doi: 10.1126/scitranslmed.aaz0463
19. Zhang C, Zhang G, Sun N, Zhang Z, Xue L, Zhang Z, et al. An Individualized Immune Signature of Pretreatment Biopsies Predicts Pathological Complete Response to Neoadjuvant Chemoradiotherapy and Outcomes in Patients With Esophageal Squamous Cell Carcinoma. Signal Transduct Target Ther (2020) 5:182. doi: 10.1038/s41392-020-00221-8
20. Wallach D. The Tumor Necrosis Factor Family: Family Conventions and Private Idiosyncrasies. Cold Spring Harb Perspect Biol (2018) 10(10):a028431. doi: 10.1101/cshperspect.a028431
21. Croft M. The Role of TNF Superfamily Members in T-Cell Function and Diseases. Nat Rev Immunol (2009) 9:271–85. doi: 10.1038/nri2526
22. Sabbagh L, Snell LM, Watts TH. TNF Family Ligands Define Niches for T Cell Memory. Trends Immunol (2007) 28:333–9. doi: 10.1016/j.it.2007.06.001
23. Benoot T, Piccioni E, De Ridder K, Goyvaerts C. Tnfα and Immune Checkpoint Inhibition: Friend or Foe for Lung Cancer? Int J Mol Sci (2021) 22(16):8691. doi: 10.3390/ijms22168691
24. Mercogliano MF, Bruni S, Mauro F, Elizalde PV, Schillaci R. Harnessing Tumor Necrosis Factor Alpha to Achieve Effective Cancer Immunotherapy. Cancers (Basel) (2021) 13(3):564. doi: 10.3390/cancers13030564
25. Montfort A, Colacios C, Levade T, Andrieu-Abadie N, Meyer N, Ségui B. The TNF Paradox in Cancer Progression and Immunotherapy. Front Immunol (2019) 10:1818. doi: 10.3389/fimmu.2019.01818
26. George J, Lim JS, Jang SJ, Cun Y, Ozretić L, Kong G, et al. Comprehensive Genomic Profiles of Small Cell Lung Cancer. NATURE (2015) 524:47–53. doi: 10.1038/nature14664
27. Subramanian A, Tamayo P, Mootha VK, Mukherjee S, Ebert BL, Gillette MA, et al. Gene Set Enrichment Analysis: A Knowledge-Based Approach for Interpreting Genome-Wide Expression Profiles. Proc Natl Acad Sci USA (2005) 102:15545–50. doi: 10.1073/pnas.0506580102
28. Hänzelmann S, Castelo R, Guinney J. GSVA: Gene Set Variation Analysis for Microarray and RNA-Seq Data. BMC Bioinf (2013) 14:7. doi: 10.1186/1471-2105-14-7
29. Yoshihara K, Shahmoradgoli M, Martínez E, Vegesna R, Kim H, Torres-Garcia W, et al. Inferring Tumour Purity and Stromal and Immune Cell Admixture From Expression Data. Nat Commun (2013) 4:2612. doi: 10.1038/ncomms3612
30. Balkwill F. Tumour Necrosis Factor and Cancer. Nat Rev Cancer (2009) 9:361–71. doi: 10.1038/nrc2628
31. Watts TH. TNF/TNFR Family Members in Costimulation of T Cell Responses. Annu Rev Immunol (2005) 23:23–68. doi: 10.1146/annurev.immunol.23.021704.115839
32. Vinay DS, Kwon BS. TNF Superfamily: Costimulation and Clinical Applications. Cell Biol Int (2009) 33:453–65. doi: 10.1016/j.cellbi.2009.02.001
33. Calzascia T, Pellegrini M, Hall H, Sabbagh L, Ono N, Elford AR, et al. TNF-Alpha Is Critical for Antitumor But Not Antiviral T Cell Immunity in Mice. J Clin Invest (2007) 117:3833–45. doi: 10.1172/JCI32567
34. Pikarsky E, Porat RM, Stein I, Abramovitch R, Amit S, Kasem S, et al. NF-Kappab Functions as a Tumour Promoter in Inflammation-Associated Cancer. NATURE (2004) 431:461–6. doi: 10.1038/nature02924
35. Popivanova BK, Kitamura K, Wu Y, Kondo T, Kagaya T, Kaneko S, et al. Blocking TNF-Alpha in Mice Reduces Colorectal Carcinogenesis Associated With Chronic Colitis. J Clin Invest (2008) 118:560–70. doi: 10.1172/JCI32453
36. Oguma K, Oshima H, Aoki M, Uchio R, Naka K, Nakamura S, et al. Activated Macrophages Promote Wnt Signalling Through Tumour Necrosis Factor-Alpha in Gastric Tumour Cells. EMBO J (2008) 27:1671–81. doi: 10.1038/emboj.2008.105
37. Orosz P, Echtenacher B, Falk W, Rüschoff J, Weber D, Männel DN. Enhancement of Experimental Metastasis by Tumor Necrosis Factor. J Exp Med (1993) 177:1391–8. doi: 10.1084/jem.177.5.1391
38. Kim S, Takahashi H, Lin WW, Descargues P, Grivennikov S, Kim Y, et al. Carcinoma-Produced Factors Activate Myeloid Cells Through TLR2 to Stimulate Metastasis. NATURE (2009) 457:102–6. doi: 10.1038/nature07623
39. Egberts JH, Cloosters V, Noack A, Schniewind B, Thon L, Klose S, et al. Anti-Tumor Necrosis Factor Therapy Inhibits Pancreatic Tumor Growth and Metastasis. Cancer Res (2008) 68:1443–50. doi: 10.1158/0008-5472.CAN-07-5704
40. Selby P, Hobbs S, Viner C, Jackson E, Jones A, Newell D, et al. Tumour Necrosis Factor in Man: Clinical and Biological Observations. Br J Cancer (1987) 56:803–8. doi: 10.1038/bjc.1987.294
41. Furman WL, Strother D, McClain K, Bell B, Leventhal B, Pratt CB. Phase I Clinical Trial of Recombinant Human Tumor Necrosis Factor in Children With Refractory Solid Tumors: A Pediatric Oncology Group Study. J Clin Oncol (1993) 11:2205–10. doi: 10.1200/JCO.1993.11.11.2205
42. Feldmann M, Maini RN. Anti-TNF Alpha Therapy of Rheumatoid Arthritis: What Have We Learned? Annu Rev Immunol (2001) 19:163–96. doi: 10.1146/annurev.immunol.19.1.163
43. Blick M, Sherwin SA, Rosenblum M, Gutterman J. Phase I Study of Recombinant Tumor Necrosis Factor in Cancer Patients. Cancer Res (1987) 47:2986–9.
44. Croft M, Benedict CA, Ware CF. Clinical Targeting of the TNF and TNFR Superfamilies. Nat Rev Drug Discovery (2013) 12:147–68. doi: 10.1038/nrd3930
45. Kitson J, Raven T, Jiang YP, Goeddel DV, Giles KM, Pun KT, et al. A Death-Domain-Containing Receptor That Mediates Apoptosis. NATURE (1996) 384:372–5. doi: 10.1038/384372a0
46. Slebioda TJ, Rowley TF, Ferdinand JR, Willoughby JE, Buchan SL, Taraban VY, et al. Triggering of TNFRSF25 Promotes CD8⁺ T-Cell Responses and Anti-Tumor Immunity. Eur J Immunol (2011) 41:2606–11. doi: 10.1002/eji.201141477
47. Aggarwal BB. Signalling Pathways of the TNF Superfamily: A Double-Edged Sword. Nat Rev Immunol (2003) 3:745–56. doi: 10.1038/nri1184
48. Guan X, Liao Z, Ma H, Qian J, Liu Z, Yuan X, et al. TNFRSF1B +676 T>G Polymorphism Predicts Survival of Non-Small Cell Lung Cancer Patients Treated With Chemoradiotherapy. BMC Cancer (2011) 11:447. doi: 10.1186/1471-2407-11-447
49. Mu CY, Qin PX, Qu QX, Chen C, Huang JA. Soluble CD40 in Plasma and Malignant Pleural Effusion With Non-Small Cell Lung Cancer: A Potential Marker of Prognosis. Chronic Dis Transl Med (2015) 1:36–41. doi: 10.1016/j.cdtm.2015.02.010
50. Dubuisson A, Favreau C, Fourmaux E, Lareure S, Rodrigues-Saraiva R, Pellat-Deceunynck C, et al. Generation and Characterization of Novel Anti-DR4 and Anti-DR5 Antibodies Developed by Genetic Immunization. Cell Death Dis (2019) 10:101. doi: 10.1038/s41419-019-1343-5
51. Dubuisson A, Micheau O. Antibodies and Derivatives Targeting DR4 and DR5 for Cancer Therapy. Antibodies (Basel) (2017) 6(4):16. doi: 10.3390/antib6040016
52. Skeate JG, Otsmaa ME, Prins R, Fernandez DJ, Da SD, Kast WM. TNFSF14: Lighting the Way for Effective Cancer Immunotherapy. Front Immunol (2020) 11:922. doi: 10.3389/fimmu.2020.00922
53. Giles DA, Zahner S, Krause P, van der Gracht E, Riffelmacher T, Morris V, et al. The Tumor Necrosis Factor Superfamily Members Tnfsf14 (Light), Lymphotoxin β and Lymphotoxin β Receptor Interact to Regulate Intestinal Inflammation. Front Immunol (2018) 9:2585. doi: 10.3389/fimmu.2018.02585
54. Dostert C, Grusdat M, Letellier E, Brenner D. The TNF Family of Ligands and Receptors: Communication Modules in the Immune System and Beyond. Physiol Rev (2019) 99:115–60. doi: 10.1152/physrev.00045.2017
55. Sakai J, Akkoyunlu M. The Role of BAFF System Molecules in Host Response to Pathogens. Clin Microbiol Rev (2017) 30:991–1014. doi: 10.1128/CMR.00046-17
56. Fan H, Lu B, Cao C, Li H, Yang D, Huang L, et al. Plasma TNFSF13B and TNFSF14 Function as Inflammatory Indicators of Severe Adenovirus Pneumonia in Pediatric Patients. Front Immunol (2020) 11:614781. doi: 10.3389/fimmu.2020.614781
57. Kasof GM, Lu JJ, Liu D, Speer B, Mongan KN, Gomes BC, et al. Tumor Necrosis Factor-Alpha Induces the Expression of DR6, a Member of the TNF Receptor Family, Through Activation of NF-Kappab. ONCOGENE (2001) 20:7965–75. doi: 10.1038/sj.onc.1204985
58. Pan G, Bauer JH, Haridas V, Wang S, Liu D, Yu G, et al. Identification and Functional Characterization of DR6, a Novel Death Domain-Containing TNF Receptor. FEBS Lett (1998) 431:351–6. doi: 10.1016/s0014-5793(98)00791-1
59. Benschop R, Wei T, Na S. Tumor Necrosis Factor Receptor Superfamily Member 21: TNFR-Related Death Receptor-6, DR6. Adv Exp Med Biol (2009) 647:186–94. doi: 10.1007/978-0-387-89520-8_13
60. Sica GL, Zhu G, Tamada K, Liu D, Ni J, Chen L. RELT, a New Member of the Tumor Necrosis Factor Receptor Superfamily, Is Selectively Expressed in Hematopoietic Tissues and Activates Transcription Factor NF-Kappab. BLOOD (2001) 97:2702–7. doi: 10.1182/blood.v97.9.2702
61. Cusick JK, Mustian A, Goldberg K, Reyland ME. RELT Induces Cellular Death in HEK 293 Epithelial Cells. Cell Immunol (2010) 261:1–8. doi: 10.1016/j.cellimm.2009.10.013
62. Choi BK, Kim SH, Kim YH, Lee DG, Oh HS, Han C, et al. RELT Negatively Regulates the Early Phase of the T-Cell Response in Mice. Eur J Immunol (2018) 48:1739–49. doi: 10.1002/eji.201847633
63. Leclerc M, Voilin E, Gros G, Corgnac S, de Montpréville V, Validire P, et al. Regulation of Antitumour CD8 T-Cell Immunity and Checkpoint Blockade Immunotherapy by Neuropilin-1. Nat Commun (2019) 10:3345. doi: 10.1038/s41467-019-11280-z
64. Rody A, Holtrich U, Pusztai L, Liedtke C, Gaetje R, Ruckhaeberle E, et al. T-Cell Metagene Predicts a Favorable Prognosis in Estrogen Receptor-Negative and HER2-Positive Breast Cancers. Breast Cancer Res (2009) 11:R15. doi: 10.1186/bcr2234
65. An N, Wang H, Jia W, Jing W, Liu C, Zhu H, et al. The Prognostic Role of Circulating CD8(+) T Cell Proliferation in Patients With Untreated Extensive Stage Small Cell Lung Cancer. J Transl Med (2019) 17:402. doi: 10.1186/s12967-019-02160-7
66. Saleh R, Sasidharan NV, Toor SM, Taha RZ, Murshed K, Al-Dhaheri M, et al. Differential Gene Expression of Tumor-Infiltrating CD8(+) T Cells in Advanced Versus Early-Stage Colorectal Cancer and Identification of a Gene Signature of Poor Prognosis. J Immunother Cancer (2020) 8(2):e001294. doi: 10.1136/jitc-2020-001294
67. Cho Y, Miyamoto M, Kato K, Fukunaga A, Shichinohe T, Kawarada Y, et al. CD4+ and CD8+ T Cells Cooperate to Improve Prognosis of Patients With Esophageal Squamous Cell Carcinoma. Cancer Res (2003) 63:1555–9.
68. Zhu CQ, Ding K, Strumpf D, Weir BA, Meyerson M, Pennell N, et al. Prognostic and Predictive Gene Signature for Adjuvant Chemotherapy in Resected Non-Small-Cell Lung Cancer. J Clin Oncol (2010) 28:4417–24. doi: 10.1200/JCO.2009.26.4325
69. Jiang Y, Xie J, Huang W, Chen H, Xi S, Han Z, et al. Tumor Immune Microenvironment and Chemosensitivity Signature for Predicting Response to Chemotherapy in Gastric Cancer. Cancer Immunol Res (2019) 7:2065–73. doi: 10.1158/2326-6066.CIR-19-0311
70. Casares N, Pequignot MO, Tesniere A, Ghiringhelli F, Roux S, Chaput N, et al. Caspase-Dependent Immunogenicity of Doxorubicin-Induced Tumor Cell Death. J Exp Med (2005) 202:1691–701. doi: 10.1084/jem.20050915
71. St PM, Ohashi PS. The Roles of CD8(+) T Cell Subsets in Antitumor Immunity. Trends Cell Biol (2020) 30:695–704. doi: 10.1016/j.tcb.2020.06.003
72. Galluzzi L, Buqué A, Kepp O, Zitvogel L, Kroemer G. Immunological Effects of Conventional Chemotherapy and Targeted Anticancer Agents. Cancer Cell (2015) 28:690–714. doi: 10.1016/j.ccell.2015.10.012
73. Pietanza MC, Byers LA, Minna JD, Rudin CM. Small Cell Lung Cancer: Will Recent Progress Lead to Improved Outcomes? Clin Cancer Res (2015) 21:2244–55. doi: 10.1158/1078-0432.CCR-14-2958
74. Qin S, Xu L, Yi M, Yu S, Wu K, Luo S. Novel Immune Checkpoint Targets: Moving Beyond PD-1 and CTLA-4. Mol Cancer (2019) 18:155. doi: 10.1186/s12943-019-1091-2
75. Chen L, Yang QC, Li YC, Yang LL, Liu JF, Li H, et al. Targeting CMTM6 Suppresses Stem Cell-Like Properties and Enhances Antitumor Immunity in Head and Neck Squamous Cell Carcinoma. Cancer Immunol Res (2020) 8:179–91. doi: 10.1158/2326-6066.CIR-19-0394
76. Burr ML, Sparbier CE, Chan YC, Williamson JC, Woods K, Beavis PA, et al. CMTM6 Maintains the Expression of PD-L1 and Regulates Anti-Tumour Immunity. NATURE (2017) 549:101–5. doi: 10.1038/nature23643
77. Lu S, Stein JE, Rimm DL, Wang DW, Bell JM, Johnson DB, et al. Comparison of Biomarker Modalities for Predicting Response to PD-1/PD-L1 Checkpoint Blockade: A Systematic Review and Meta-Analysis. JAMA Oncol (2019) 5:1195–204. doi: 10.1001/jamaoncol.2019.1549
78. Thommen DS, Koelzer VH, Herzig P, Roller A, Trefny M, Dimeloe S, et al. A Transcriptionally and Functionally Distinct PD-1(+) CD8(+) T Cell Pool With Predictive Potential in non-Small-Cell Lung Cancer Treated With PD-1 Blockade. Nat Med (2018) 24:994–1004. doi: 10.1038/s41591-018-0057-z
Keywords: small cell lung cancer, antitumor immunity, tumor necrosis factor family, prognostic prediction, chemotherapy response
Citation: Zhang Z, Wu P, Zhang C, Luo Y, Zhang G, Zeng Q, Wang L, Yang Z, Sun N and He J (2021) Tumor Necrosis Factor Family Member Profile Predicts Prognosis and Adjuvant Chemotherapy Benefit for Patients With Small-Cell Lung Cancer. Front. Immunol. 12:745769. doi: 10.3389/fimmu.2021.745769
Received: 22 July 2021; Accepted: 01 November 2021;
Published: 18 November 2021.
Edited by:
Karine Rachel Prudent Breckpot, Vrije University Brussel, BelgiumReviewed by:
Ying Ma, Tianjin Medical University Cancer Institute and Hospital, ChinaCleo Goyvaerts, Vrije University Brussel, Belgium
Akseli Hemminki, University of Helsinki, Finland
Copyright © 2021 Zhang, Wu, Zhang, Luo, Zhang, Zeng, Wang, Yang, Sun and He. This is an open-access article distributed under the terms of the Creative Commons Attribution License (CC BY). The use, distribution or reproduction in other forums is permitted, provided the original author(s) and the copyright owner(s) are credited and that the original publication in this journal is cited, in accordance with accepted academic practice. No use, distribution or reproduction is permitted which does not comply with these terms.
*Correspondence: Nan Sun, sunnan@vip.126.com; Jie He, prof.jiehe@gmail.com
†These authors have contributed equally to this work