- 1Department of Science and Innovation (DSI)-National Research Foundation (NRF) Centre of Excellence for Biomedical Tuberculosis Research, South African Medical Research Council Centre for Tuberculosis Research, Division of Molecular Biology and Human Genetics, Faculty of Medicine and Health Sciences, Stellenbosch University, Cape Town, South Africa
- 2Department of Molecular and Cell Biology, University of Cape Town, Cape Town, South Africa
Mycobacterium tuberculosis (Mtb) “a human adapted pathogen” has found multiple ways to manipulate the host immune response during infection. The human immune response to Mtb infection is a highly complex cascade of reactions, with macrophages as preferred intracellular location. Interaction with the host through infection gives rise to expression of specific gene products for survival and multiplication within the host. The signals that the pathogens encounter during infection cause them to selectively express genes in response to signals. One strategy to identify Mtb antigens with diagnostic potential is to identify genes that are specifically induced during infection or in specific disease stages. The shortcomings of current immunodiagnostics include the failure to detect progression from latent infection to active tuberculosis disease, and the inability to monitor treatment efficacy. This highlights the need for new tuberculosis biomarkers. These biomarkers should be highly sensitive and specific diagnosing TB infection, specifically distinguishing between latent infection and active disease. The regulation of iron levels by the host plays a crucial role in the susceptibility and outcome of Mtb infection. Of interest are the siderophore biosynthetic genes, encoded by the mbt-1 and mbt-2 loci and the SUF (mobilization of sulphur) operon (sufR-sufB-sufD-sufC-csd-nifU-sufT), which encodes the primary iron-sulphur cluster biogenesis system. These genes are induced during iron limitation and intracellular growth of Mtb, pointing to their importance during infection.
Introduction
TB disease is a major cause of poor health even in the 20th century. In 2019, approximately 10 million people fell ill with TB, while 1.2 million deaths amongst HIV-negative individuals and 208 000 deaths amongst HIV-positive individuals are estimated (1).
The innocuous or asymptomatic nature of TB infection contributes to its elusiveness (2). In individuals where the host immune response contains the infection, Mtb has the ability to establish a latent TB infection (LTBI), allowing long persistence of viable bacilli in the host without any clinical symptoms (3, 4). Following exposure to Mtb, 90-95% of infected individuals remain healthy (asymptomatic) and are latently infected (5). Between 3-10% of all LTBI individuals develop active disease during their lifespan (6). LTBI individuals therefore represents a long-lived pool from which active TB disease will continue to develop (7, 8). The risk of developing active disease decreases exponentially over time, the first 2 years after infection being crucial with an 80% chance of developing TB (2).
Where TB does develop, the non-specific nature of disease often delays diagnosis and treatment, increasing the risk of infecting others (9). A further challenge facing global TB control is the emergence and spread of multi-drug resistant Mtb (MDR-TB) strains. MDR-TB is defined as infection with an Mtb strain that is resistant to, at least, rifampicin and isoniazid (10). In 2017, an estimated 558 000 individuals developed drug-resistant TB and 230 000 died (11). Alarmingly, more than a third of the MDR cases are not enrolled for MDR treatment, with this percentage approaching two-thirds in some areas in Asia and Africa (2).
Iron Homeostasis and Mtb Pathogenesis
Iron is an essential micronutrient which is important for the host and Mtb metabolic processes (12, 13). The regulation of iron levels by the host influences the susceptibility to and outcome of Mtb infection (14, 15), since high macrophage iron stores and nutritional iron overload are associated with a higher chance of developing TB and increased severity of the disease (16–18). Most of the iron in the human body is stored as protein-bound heme (70-75%), while the remainder is associated with the plasma transport proteins transferrin and lactoferrin or bound to the intracellular storage protein, ferritin (19). Since intestinal absorption provides less than 10% of the host’s iron requirement, macrophages play a key role in maintaining serum levels by recycling iron from aged erythrocytes (20). Ferroportin (FPN-1) exports iron from macrophages into the bloodstream and is therefore central to maintaining iron homeostasis. During infection, inflammatory cytokines, primarily IL-6, induce expression of the hormone hepcidin to trigger FPN-1 internalization, preventing iron export (21); a response which can lead to anemia in chronic infections like TB (22). While macrophages represent a significant iron reservoir in the host (23, 24), inflammatory cytokines also induce ferritin synthesis in macrophages thereby reducing the labile iron pool.
Mtb employs several mechanisms to acquire iron from the host during infection, including siderophores (mycobactin and carboxymycobactin), which sequester iron from host iron-binding proteins, heme-binding proteins and specialized iron transporters (23, 25). The genes encoding the core Mtb siderophore biosynthetic machinery are organized in two clusters, mbt-1, and the mbt-2 loci. The mbt-1 locust consists of 10 genes mbtA - mbtJ and mbt-2 locus consist of 4 genes mbtN – mbtK (12, 26). An mbtB Mtb mutant, defective in the first step of mycobactin synthesis, is impaired for growth in low-iron media and macrophages (25, 27), highlighting the essential role of siderophores in obtaining iron during intracellular growth (26). This is consistent with the induction of mtbA-J during growth in IFN-γ activated macrophages and increased mbtB mRNA levels detected during Mtb infection in mice (16). The mbt operon is downregulated under iron replete conditions by the iron-dependent regulator (IdeR) (28), which concomitantly induces the iron storage genes bfrA (bacterioferritin) and bfrB (ferritin like protein) (26). The transcription of the mbt and bfr genes are also dependent on HupB, a 28-kDa DNA-binding protein which is induced under iron-limiting conditions. Binding of HupB to the mbtB is iron-dependent and is thought to induce expression in the absence of IdeR binding (Figure 1A) (28). HupB was identified in the cell wall fraction of Mtb, and recently a novel role in the transfer of iron between extracellular ferri-carboxymycobactin and membrane-bound mycobactin was proposed (30). A hupB knockout strain of Mtb showed a marked reduction in mycobactin and carboxymycobactin levels and was unable to survive in macrophages (28), likely due to impaired iron acquisition during intracellular growth.
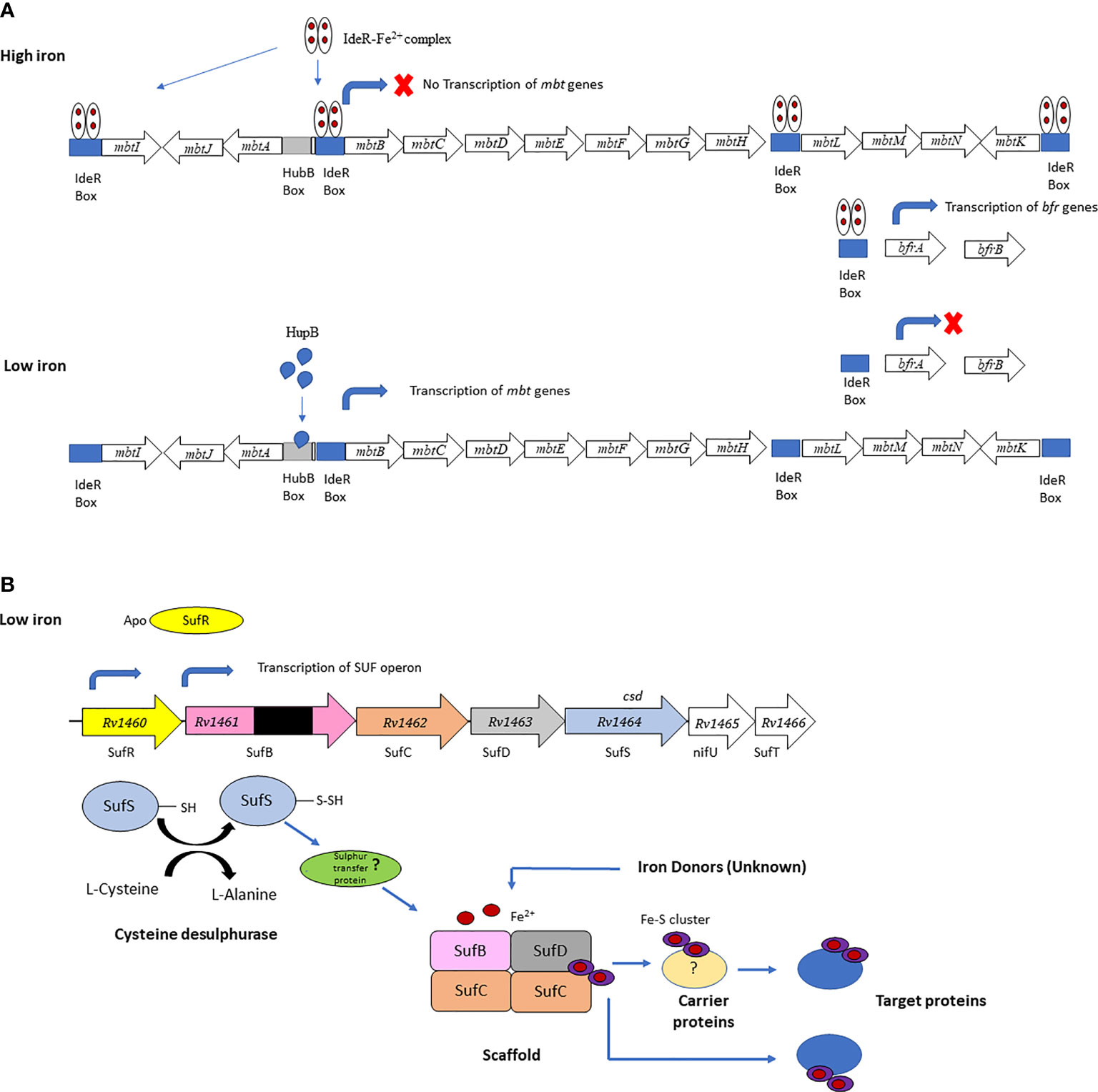
Figure 1 (A) IderR regulates Mtb siderophore biosynthesis and iron storage. The mbtA-J genes synthesize the siderphore core and the mbtL-K genes synthesize the mbtK-acyl complex. Transcription of the siderphore biosynthesis genes is inhibited under iron-sufficient conditions and synthesis of the iron storage proteins is activated when the IdeR-Fe2+ complex binds to the IdeR boxes in the mbt locus and upstream of the bfrA gene. Under iron limiting conditions the IdeR-Fe2+ complex does not form and does not bind to the IdeR box in the mbtB locus, allowing HupB to bind to the hupB box upstream of the IdeR box activating transcription of the mbt genes. In the absence of the IdeR-Fe2+ complex transcription of the iron storage genes bfrA and bfrB is repressed. Panel (A) is adapted from Sritharan (12). (B) Fe-S biogenesis machinery in Mtb encoded by a single operon (sufR-sufB-sufD-sufC-csd-nifU-sufT). All the genes in the operon, except for sufR, were predicted to be essential by forward genetic screens. This figure is adapted from Willemse (29).
A recent study by Pisu et al. (31) demonstrated a correlation between bacterial replication and iron availability in the mouse model of infection. Using dual RNA-seq they showed two distinct host and bacterial transcriptional signatures for alveolar, and monocyte-derived interstitial macrophages. Alveolar macrophage-derived bacteria had high expression of genes suggestive of iron replete conditions, e.g. iron storage proteins (BfrB), while bacteria derived from interstitial macrophages had high expression of genes associated with iron limitation, such as siderophore biosynthesis (31). In agreement with the bacterial response, interstitial macrophages expressed genes associated with iron sequestration, while alveolar macrophages showed a phenotype associated with iron release. Notably, alveolar macrophages were more permissive for bacterial growth than interstitial macrophages (31). A study investigating Mtb disease progression in knockout mice lacking the ferritin heavy chain (FtH) in myeloid-derived cell populations (32) demonstrated the importance of host iron homeostasis in disease. Fth-/- mice showed increased bacterial organ burden, increased bacterial dissemination, excessive inflammation, and decreased survival due to altered immune cell metabolism. Furthermore, Mtb infection was shown to impair iron absorption and alter host iron homeostasis. The observation that iron levels and deposition patterns are altered in lung tissue from human TB patients suggested that these finding have relevance for human disease (32).
The Current Tuberculosis Diagnostic Paradigm
The existing diagnostic tools for TB have several limitations. The most important criteria for confirming TB diagnosis are acid-fast bacilli (AFB) in sputum smears, radiographic signs, clinical symptoms and risk factors, or a combination of these (33). The gold standard is the detection of Mtb in human specimens by microbiological culture, which has a high sensitivity for detecting Mtb, but requires 2 to 6 weeks for interpretation. Sputum smear microscopy is an inexpensive tool, which is rapid and simple for diagnosing pulmonary TB, however it has low and variable sensitivity when the mycobacterial load is low, when the site of infection is not easily accessible, or when patients fail to produce a sputum sample (34). These limitations result in reduced success in identifying individuals at risk for developing extrapulmonary TB (EPTB), pediatric TB and pulmonary TB in people living with HIV (35).
Chest X-rays are also used in diagnosis, but can present with atypical radiological finding that are indistinguishable from community–acquired pneumonia (36). The intradermal purified protein derivative (PPD) test or tuberculin test (TST), which detects an immune response to bacterial proteins, is limited by the fact that it is not Mtb-specific, since it shares Mtb antigens with other mycobacterial strains (37). Another logistical limitation of the TST test is that a second patient visit is required to evaluate the test results (38). The Interferon Gamma Release Assay (IGRA) measures the levels of interferon-gamma (IFN-γ) released from T-lymphocytes after in vitro stimulation with the Mtb-specific antigens, early secreted antigenic target 6 kDa (ESAT-6; Rv3875), and culture filtrate protein 10 kDa (CFP-10; Rv3874) (38, 39). It has the advantage over TST in that it uses antigens in the region of difference-1 (RD-1), found only in Mtb, thereby erasing cross-reactivity with the BCG vaccine strain. IGRA as diagnostic tool however fails to differentiate between LTBI and active TB and has limited predictive value, failing to identify individuals at risk of progressing to active TB (40–42).
The most recent and widely implemented new diagnostic test, namely the GeneXpert MTB/RIF, has revolutionized the diagnosis of TB. Xpert MTB/RIF is an automated molecular test, using real-time polymerase chain reaction (PCR) to amplify the rpoB gene (Mtb specific sequence), and probe it with molecular beacons for mutations within the rifampicin resistance determining region (RRDR) (43). It detects 75% of smear negative pulmonary TB cases, as well as rifampicin resistance with results within 2 hours. Although GeneXpert MTB/RIF improves TB case detection, two recent randomized trials in Southern Africa suggest that on its own, GeneXpert may not be able to significantly reduce tuberculosis-related morbidity and mortality (44, 45).
Advances in Mtb Biomarker Research and Why They Are Not Good Enough
Improved TB control requires the development of sensitive and specific diagnostic tests for the identification of TB infection, and specifically to distinguishing between latent and active disease (46). Differential expression of immunological markers by the host in response to Mtb infection may enable us to distinguish the stages of TB infection and disease, and therefore hold promise as diagnostic biomarkers (47). Multiplex assays and advances in ‘omics’ technology have facilitated the study of immune biomarkers in samples that are easy to access, such as peripheral blood, saliva, or urine (48). In the following sections, we highlight studies aimed at identifying host biomarkers that differentiate between stages of Mtb infection and monitor disease progression. The use of infection-stage specific Mtb antigens in these assays is also discussed.
Host Biomarkers That Differentiate Between the Different Stages of Mtb Infection and Monitor Disease Progression
Stimulating whole blood from Mtb-infected individuals with Mtb specific antigens causes the release of several host cytokines and chemokines (49). Several studies have sought to identify cytokines (Table 1) and chemokines that might better discriminate between LTBI and active TB disease (58). Chegou et al. (58), showed an increase in multiple host markers other than IFN-γ after stimulating whole blood cells from TB patients and household contacts (HHCs) with Mtb antigens hypothesized to be differentially expressed during different phases of Mtb infection. The most promising diagnostic candidates were IL-12(p40), IP-10, IL-10 and TNF-α, which were able to discriminate between TB cases and HHC with 100% sensitivity and specificity following stimulation with Rv0081 (58). Rv0081 is a Mtb protein in the dormancy survival (DosR) regulon, which is induced during oxygen limitation and is required for anaerobic survival (59). Using a training sample set (n = 491) Chegou et al. (51) identified a seven-serum host protein biosignature (C reactive protein (CRP), transthyretin, IFN-g, complement factor H, apolipoprotein-A1, inducible protein 10 and serum amyloid A) that could diagnose TB disease. In a test set (n = 210), the signature had a sensitivity of 93.8% (95% CI 84.0% to 98.0%), specificity of 73.3% (95% CI 65.2% to 80.1%), and positive predictive value (PPV) and negative predictive value (NPV) of 60.6% (95% CI 50.3% to 70.1%) and 96.4% (95% CI 90.5% to 98.8%), respectively, irrespective of HIV infection status or ethnicity. The biosignature correctly classified 74% (17/23) of patients who were smear-negative but culture-positive and 67% (6/9) of patients who were both smear-negative and culture-negative (51). Wang et al. (52) identified a six-cytokine biosignature that could distinguish between active TB and LTBI with high levels of accuracy. In the screening group (n = 88) IFN-γ, IP-10, and IL-1Ra were identified following stimulation with ESAT-6/CFP-10, while IP-10, VEGF, and IL-12 (p70) were measured in unstimulated samples. Validation of the six-cytokine biosignature in a biomarker validation cohort (n = 216) showed a sensitivity of 88.2% and specificity of 92.1% and in a clinical validation cohort (n = 194) a sensitivity of 85.7%, a specificity of 91.3% with an accuracy of 88.7% (52).
Transcriptional profiling of blood cells from TB, healthy uninfected and/or LTBI individuals has also shown promise in identifying TB biomarkers (60). A study by Zak et al. (53) identified a 16-gene blood transcriptional correlate of risk (COR) signature that predicted the risk of progression to TB in HIV-negative South African adolescents with LTBI with a sensitivity and specificity of 66.1% and 80.6% (with a 95% confidence interval (CI) 63.2 – 68.9 and 7.2 – 82.0), respectively, 12 months preceding TB. Validation in an independent group of adolescents from South African and Gambian cohorts reported a sensitivity of 53.7% (42.6 – 64.3) and a specificity of 82.8% (76.7–86) (53). Using saliva samples from suspected pulmonary TB individuals, Jacobs et al. (54) identified a seven-marker biosignature of CRP, ferritin, SAP, MCP-1, A2M, fibrinogen and TPA, that might be useful in the diagnosis of TB disease. TB disease was diagnosed with a sensitivity of 78.1% (95% CI, 59.6–90.1%) and specificity of 83.3% (95% CI, 72.3–90.7%) after leave-one-out cross validation (54). Cao et al. (39) screened for novel serum biomarkers that can discriminate between LTBI and active TB individuals using an Mtb proteome microarray containing 4,262 antigens. Significantly higher levels of 152 Mtb antigen-specific IgG antibodies was seen in the active TB compared to the LTBI group (p < 0.05). ELISA analysis of 11 candidate antigens were consistent with the microarray analysis. Antibodies specific for Rv2031c, Rv1408, and Rv2421c had highest areas under the curve (AUCs) of 0.8520, 0.8152, and 0.7970, respectively. The authors identified several antigens with potential as serum biomarkers for discriminating between active TB and LTBI (39).
Apart from diagnosis, biomarkers can be used for monitoring treatment response in TB patients. It is believed that people with active TB may have a flawed Mtb-specific T cell response due to the increasing bacterial load. One mechanism that gives rise to the inhibition of antigen-specific T cell effector function is the expression of inhibitory receptors such as PD-1, CTLA-4, LAG-3, TIM-3, and BTLA on CD4 T cells (55). Day et al. (55) showed the expression of programmed cell death protein 1 (PD-1) on Mtb specific CD4 T cells, but not CD8 T cells. The expression of PD-1 characterizes a population of effector cells, that have engaged their cognate antigen and have the capacity to produce Th1 cytokines. This study has provided novel insight into the function of PD-1 pathway in regulating T cell response during Mtb infection and the expression of PD-1 on antigen specific CD4 T cells as a biomarker for bacterial load and treatment response in human TB (55). A pilot study by van Rensburg et al. (56) evaluating the transcriptome of B-cells showed the downregulation of FASLG and IL5RA in TB cases at diagnosis compared to healthy controls (HCs) and the upregulation of these genes in TB cases after month 6 of treatment. These genes have the potential to be used as a biosignature to monitor treatment response but need to be validated in a larger cohort (56). Using an integrated approach Ronacher et al. (61) identified a six-marker model consisting of time to positivity (TTP), body mass index (BMI), TNF-β, sIL-6R, IL-12p40 and IP-10 measured at baseline, which predicted relapse with a 75% (95% CI: 0.38–1.0) sensitivity and a 85% (95% CI: 0.75–0.93) specificity in the discovery cohort and 83% (95% CI 0.58–1) sensitivity and 61% (95% CI 0.39–0.83) specificity in the validation cohort (61). Cilliers et al. (62) investigated the early TB treatment response of patients who subsequently remained cured or who relapsed. They investigated the use of individual and multi-marker models to predict treatment outcome and responder classification. Top performing multi-variable models at diagnosis using unstimulated values predicted outcome at 24 months after treatment completion with a sensitivity of 75.0% (95% CI, 42.8–94.5%) and specificity of 72.4% (95% CI, 52.8–87.3%) in leave-one-out cross validation. Month two treatment responder classification was correctly predicted with a sensitivity of 79.2% (95% CI, 57.8–92.9%) and specificity of 92.3% (95% CI, 64.0–99.8%) (62).
Mtb Antigens With Potential to Differentiate Between the Stages of TB Infection
A determinant for immunodiagnostic test sensitivity, specificity and predictive potential is the antigen(s) that is used for stimulation (42). Mtb responds to the conditions encountered in the host and is therefore hypothesized to express different antigens during different phases of infection. These include DosR regulon encoded antigens, TB reactivation antigens, resuscitation promoting factors (rpfs) and starvation-induced proteins. To date, however, none of these antigens, single or in combination have the ability to discriminate active TB or LTBI with 100% accuracy (58). A study done by Chegou et al. (50) assessed the diagnostic potential of 118 Mtb antigens in TB patients and HHCs in a high-TB burden population. Using a 7-day diluted whole blood assay (WBA) they screened the antigens to assess their diagnostic potential for identifying active TB. The antigens that could distinguish between active TB and LTBI were the rpfs (Rv0867c, Rv2389c, Rv2450c, Rv1009 and Rv1884c), with AUCs between 0.72 and 0.80. A combination of Mtb specific ESAT-6/CFP-10 fusion protein, Rv2624c and Rv0867c accurately predicted 73% of the TB patients and 80% of the non-TB cases after cross validation (50). Loxton et al. (57) studied the ability of Mtb surface protein, heparin-binding hemagglutinin (HBHA) to induce multiple cytokines in peripheral blood mononuclear cell (PBMCs) and whole bood from TB index cases and HHCs (57). HBHA is a 28 kD heparin-binding protein produced by Mtb and Mycobacterium bovis (63), present at the outermost layer of the cell and mediates the bacteria-epithelial cell interaction (63, 64). Induction of multifunctional INF-γ-, IL-2-, and IL-17-coexpressing CD4+ T cells was observed in HHCs, but not in active TB cases (57).
Using a sera from 561 TB suspects, Kunnath-Velayudhan et al. (65) found that only about 10% of the Mtb proteome is recognized by antibodies in these individuals; a protein subset that is enriched for secreted and membrane proteins (65). Although an association was observed between the antibody response and the bacterial burden in patients with active disease, the relative response to different antigens varied between individuals. It is currently unclear if the level of antigen expression correlates with relative antigen responses. A study investigating the antibody response to LAM and 7 mycobacterial protein antigens (ESAT-6, Tpx, PstS1, AlaDH, MPT64, 16kDa and 19kDa) and 2 antigen cocktails (TUB: PstS1, 16kDa and APA; TB-LTBI: Tpx, L16) found that individually anti-16 kDa IgA and anti-MPT64 IgA were best able to differentiate between LTBI active TB disease (66). In combination anti-TB-LTBI IgG, anti-Tpx IgG, anti-MPT64 IgA and anti-LAM IgA classified both groups (TB disease or LTBI) with an accuracy of 100% in the re-substitution classification matrix and an accuracy of 95.2% after leave-one-out cross validation. Using antigen combinations may therefore be useful to overcome the variation observed between individuals.
The Diagnostic Potential of Host Iron Metabolism Indicators
Perturbations in host iron homeostasis due to inflammation occurs following infection with a range of pathogens and indicators of iron metabolism may therefore lack the specificity required for diagnostic biomarkers. A recent study investigating the etiology of community-acquired pneumonia demonstrated that ferritin levels could distinguish between atypical and typical bacterial agents (odds ratio [OR], 2.26; 95% CI, 1.18–4.32; p = 0.014), while hepcidin and ferritin distinguished between atypical bacterial and viral etiology (hepcidin: OR = 3.12, 95% CI = 1.34–7.28, p = 0.008; ferritin: OR = 2.38, 95% CI = 1.28–4.45, p = 0.006) (67). The authors proposed that these differences may be due to the intracellular location of atypical bacteria, and unique aspects of the response to viruses, suggesting that host-pathogen interactions are reflected to some extent by iron metabolism indicators.
Recent studies have investigated using iron metabolism indicators in combination to improve specificity. Dai et al. (68) built a TB prediction model using three iron biomarkers (serum iron, ferritin and transferrin levels) and a training set which included PTB, HC, HHC, LTBI and cured patients. Validation on an independent cohort showed that the model successfully differentiated TB from non-TB, with 77% sensitivity (95% CI 74 to 80), 92% specificity (95% CI87 to 96), 98% PPV (95% CI 97 to 99) and 40% NPV (95% CI 35 to 45) (68). Another approach to improve specificity is to use iron metabolism indicators in combination with Mtb-specific biomarkers. A predictive model to discriminate between TB and LTBI was developed by Luo et al. (69), using iron metabolism indicators (serum ferritin, serum iron, transferrin, total iron binding capacity, unsaturated iron binding capacity, and soluble transferrin receptor) in combination with the T-spot assay (TBAg/PHA ratio). Validation of the model on an independent cohort showed an AUC of 0.965 (95% CI, 0.934–0.997) with 92.42% sensitivity and 90.57% specificity (69).
Given that host iron status impacts TB disease severity, indicators of host iron metabolism may be useful for predicting disease progression. Panda et al. observed plasma iron and hemoglobin levels were low in PTB patients compared to HHC and HCs, suggesting anemia of inflammation due to active disease (21). In an earlier study, Minchella et al. (70) observed higher ferritin and hepcidin concentrations among early TB-progressors compared to delayed progressors (mean ferritin 50.2 vs. 26.2 ng/ml; p = 0.027; mean hepcidin 37.7 vs. 5.6 ng/ml; p = 0.036), while low transferrin around the time of Mtb exposure was identified as a risk factor for all progressors (70). The authors speculated that higher ferritin and hepcidin levels provide intracellular bacteria with increased access to iron, resulting in increased replication and active disease.
The Diagnostic Potential of Mtb Antigens Induced by Iron Limitation
Given that Mtb must compete with the host for iron during infection, it is conceivable that Mtb proteins involved in maintaining iron homeostasis may have utility as biomarkers. Sivakolundu et al. (71) showed high levels of anti-HupB antibodies in TB patients compared with HHC and HCs (71). In a follow up study, Sritharan et al. (28) observed a significantly higher level of anti-rHupB-F2 (aa 63–161) antibodies in all groups with TB compared to controls (p < 0.05), including relapse and EPTB patients (28). Both studies showed a negative correlation between serum iron levels and the titre of anti-HupB antibodies in the active TB groups, suggesting that HupB may be induced in response to iron limitation in these patients (28, 71).
In addition to iron-acquisition gene transcripts, iron-starved Mtb upregulates iron-sulphur (Fe-S) cluster biosynthesis genes (sufR-sufB-sufD-sufC-csd-nifU-sufT) (Figure 1B) and several Fe-S containing proteins. Fe-S biogenesis therefore seems to be prioritized by Mtb in its iron sparing strategy, to ensure that essential Fe-S cluster proteins remain functional (72). Induction of the sufR-sufB-sufD-sufC-csd-nifU-sufT operon also occurs in response to oxidative stress and intracellular growth (73, 74), and transcription is regulated by the Fe-S containing protein SufR (75, 76) Kumar et al. (77) detected anti-SufR antibodies in serum of TB patients suggesting that this regulator is expressed in Mtb during growth within the human host (77). This was further supported by the observation that sufR expression was induced in Mtb in sputum from TB patients, relative to expression in vitro (77). Rv2204c is a A-type carrier protein predicted function in Fe-S cluster biogenesis (78). A study investigating antigen-specific memory and regulatory T cells found a higher proportion of Rv2204c reactive CD4+ Treg in active TB patients than LTBI individuals (79). The finding is however preliminary given the small number of individuals included in the study (15 per group).
The observation by Pisu et al. (31) that Mtb may be experiencing different levels of iron limitation in different cell types raises an important question for biomarker discovery; if bacteria reside in different niches within the host, how would this influence the global immune response, and by implication the choice of diagnostic antigens? Yang et al. (80) assessed BfrB-specific cellular responses in human cohorts, including HCs, LTBI and PTB patients and showed that BfrB is capable of inducing moderate cellular responses in TB patients and LTBI individuals (80). Interestingly, BfrB-specific IFN-γ release in PTB patients was lower than for ESAT-6 or CFP-10 stimulation, while it was comparable in the LTBI cohort, suggesting that BfrB becomes immunodominant during latent infection. Similar trends have been observed for dormancy-associated antigens (81, 82), suggesting that the comparing immunodominance of different antigens may allow discrimination between disease stages.
Conclusion
The shortcomings of current immunodiagnostics for the detection of progression from LTBI to active TB, diagnosis and treatment monitoring of TB highlights the need for new TB biomarkers. Performance targets for point-of-care tests in adults with smear-positive pulmonary TB include a sensitivity and specificity of 95%, while sensitivity in smear-negative cases should attain 60-80% and specificity of 95%. It is difficult to predict which biomarkers will attain this level of discrimination, however given the importance of host and bacterial iron metabolism during infection we believe this area warrants further investigation for TB biomarker development.
Author Contributions
MW and AL conceptualized the study. LB wrote the manuscript with support from AL and MW. AL and MW edited the manuscript and gave critical comments. All authors contributed to the article and approved the submitted version.
Funding
This work was supported by the South African Medical Research Association (SAMRC), Centre of Excellence for Biomedical Tuberculosis Research (CBTBR). MW was supported by a research career award from the National Research Foundation (Grant number: 91424).
Conflict of Interest
The authors declare that the research was conducted in the absence of any commercial or financial relationships that could be construed as a potential conflict of interest.
Publisher’s Note
All claims expressed in this article are solely those of the authors and do not necessarily represent those of their affiliated organizations, or those of the publisher, the editors and the reviewers. Any product that may be evaluated in this article, or claim that may be made by its manufacturer, is not guaranteed or endorsed by the publisher.
Acknowledgments
The authors acknowledge Dr Danicke Willemse and Miss Jasmine Arries for constructing Figure 1B.
References
1. 9789240013131-Eng.Pdf. Available at: https://apps.who.int/iris/bitstream/handle/10665/336069/9789240013131-eng.pdf (Accessed June 10, 2021).
2. Cardona P-J. What We Have Learned and What We Have Missed in Tuberculosis Pathophysiology for a New Vaccine Design: Searching for the “Pink Swan.” Front Immunol (2017) 8:556. doi: 10.3389/fimmu.2017.00556
3. Chaves AS, Rodrigues MF, Mattos AMM, Teixeira HC. Challenging Mycobacterium Tuberculosis Dormancy Mechanisms and Their Immunodiagnostic Potential. Braz J Infect Dis (2015) 19:636–42. doi: 10.1016/j.bjid.2015.08.004
4. Flynn JL, Chan J. Tuberculosis: Latency and Reactivation. Infect Immun (2001) 69:4195–201. doi: 10.1128/IAI.69.7.4195-4201.2001
5. Gideon HP, Flynn JL. Latent Tuberculosis: What the Host “Sees”? Immunol Res (2011) 50:202–12. doi: 10.1007/s12026-011-8229-7
6. Ottenhoff THM. New Pathways of Protective and Pathological Host Defense to Mycobacteria. Trends Microbiol (2012) 20:419–28. doi: 10.1016/j.tim.2012.06.002
7. Raviglione M, Sulis G. Tuberculosis 2015: Burden, Challenges and Strategy for Control and Elimination. Infect Dis Rep (2016) 8:33–7. doi: 10.4081/idr.2016.6570
8. Kasprowicz VO, Churchyard G, Lawn SD, Squire SB, Lalvani A. Diagnosing Latent Tuberculosis in High-Risk Individuals: Rising to the Challenge in High-Burden Areas. J Infect Dis (2011) 204:S1168–78. doi: 10.1093/infdis/jir449
9. Biya O, Gidado S, Abraham A, Waziri N, Nguku P, Nsubuga P, et al. Knowledge, Care-Seeking Behavior, and Factors Associated With Patient Delay Among Newly-Diagnosed Pulmonary Tuberculosis Patients, Federal Capital Territory, Nigeria, 2010. Pan Afr Med J (2014) 18:6. doi: 10.11694/pamj.supp.2014.18.1.4166
10. Seung KJ, Keshavjee S, Rich ML. Multidrug-Resistant Tuberculosis and Extensively Drug-Resistant Tuberculosis. Cold Spring Harb Perspect Med (2015) 5:a017863. doi: 10.1101/cshperspect.a017863
11. Vekemans J, O’Brien KL, Farrar J. Tuberculosis Vaccines: Rising Opportunities. PloS Med (2019) 16:e1002791. doi: 10.1371/journal.pmed.1002791
12. Sritharan M. Iron Homeostasis in Mycobacterium Tuberculosis: Mechanistic Insights Into Siderophore-Mediated Iron Uptake. J Bacteriol (2016) 198:2399–409. doi: 10.1128/JB.00359-16
13. Ratledge C. Iron, Mycobacteria and Tuberculosis. Tuberc Edinb Scotl (2004) 84:110–30. doi: 10.1016/j.tube.2003.08.012
14. Moyo VM, Gangaidzo IT, Gordeuk VR, Kiire CF, Macphail AP. Tuberculosis and Iron Overload in Africa: A Review. Cent Afr J Med (1997) 43:334–9.
15. Gordeuk VR, McLaren CE, MacPhail AP, Deichsel G, Bothwell TH. Associations of Iron Overload in Africa With Hepatocellular Carcinoma and Tuberculosis: Strachan’s 1929 Thesis Revisited. Blood (1996) 87:3470–6. doi: 10.1182/blood.V87.8.3470.bloodjournal8783470
16. Boelaert JR, Vandecasteele SJ, Appelberg R, Gordeuk VR. The Effect of the Host’s Iron Status on Tuberculosis. J Infect Dis (2007) 195:1745–53. doi: 10.1086/518040
17. Gomes MS, Boelaert JR, Appelberg R. Role of Iron in Experimental Mycobacterium Avium Infection. J Clin Virol (2001) 20:117–22. doi: 10.1016/S1386-6532(00)00135-9
18. de Monyé C, Karcher DS, Boelaert JR, Gordeuk VR. Bone Marrow Macrophage Iron Grade and Survival of HIV-Seropositive Patients. AIDS (1999) 13:375–80. doi: 10.1097/00002030-199902250-00010
19. Aisen P, Leibman A. Lactoferrin and Transferrin: A Comparative Study. Biochim Biophys Acta BBA - Protein Struct (1972) 257:314–23. doi: 10.1016/0005-2795(72)90283-8
20. Hentze MW, Muckenthaler MU, Galy B, Camaschella C. Two to Tango: Regulation of Mammalian Iron Metabolism. Cell (2010) 142:24–38. doi: 10.1016/j.cell.2010.06.028
21. Panda S, Faisal S, Kumar K, Seelan DM, Sharma A, Gupta NK, et al. Role of Regulatory Proteins Involved in Iron Homeostasis in Pulmonary Tuberculosis Patients and Their Household Contacts. Indian J Clin Biochem (2021). doi: 10.1007/s12291-020-00947-w
22. Wang C-Y, Babitt JL. Hepcidin Regulation in the Anemia of Inflammation. Curr Opin Hematol (2016) 23:189–97. doi: 10.1097/MOH.0000000000000236
23. Kurthkoti K, Amin H, Marakalala MJ, Ghanny S, Subbian S, Sakatos A, et al. The Capacity of Mycobacterium Tuberculosis To Survive Iron Starvation Might Enable It To Persist in Iron-Deprived Microenvironments of Human Granulomas. mBio (2017) 8:e01092–17. doi: 10.1128/mBio.01092-17
24. Zondervan NA, van Dam JCJ, Schaap PJ, Martins Dos Santos VAP, Suarez-Diez M. Regulation of Three Virulence Strategies of Mycobacterium Tuberculosis: A Success Story. Int J Mol Sci (2018) 19:347. doi: 10.3390/ijms19020347
25. Rodriguez GM. Control of Iron Metabolism in Mycobacterium Tuberculosis. Trends Microbiol (2006) 14:320–7. doi: 10.1016/j.tim.2006.05.006
26. Pandey SD, Choudhury M, Yousuf S, Wheeler PR, Gordon SV, Ranjan A, et al. Iron-Regulated Protein HupB of Mycobacterium Tuberculosis Positively Regulates Siderophore Biosynthesis and Is Essential for Growth in Macrophages. J Bacteriol (2014) 196:1853–65. doi: 10.1128/JB.01483-13
27. De Voss JJ, Rutter K, Schroeder BG, Su H, Zhu Y, Barry CE. The Salicylate-Derived Mycobactin Siderophores of Mycobacterium Tuberculosis Are Essential for Growth in Macrophages. Proc Natl Acad Sci USA (2000) 97:1252–7. doi: 10.1073/pnas.97.3.1252
28. Sritharan N, Choudhury M, Sivakolundu S, Chaurasia R, Chouhan N, Rao PP, et al. Highly Immunoreactive Antibodies Against the Rhup-F2 Fragment (Aa 63–161) of the Iron-Regulated HupB Protein of Mycobacterium Tuberculosis and Its Potential for the Serodiagnosis of Extrapulmonary and Recurrent Tuberculosis. Eur J Clin Microbiol Infect Dis (2015) 34:33–40. doi: 10.1007/s10096-014-2203-y
29. Willemse D. Regulation of Iron-Sulphur Cluster Biogenesis in Mycobacterium Tuberculosis (2016). Available at: https://scholar.sun.ac.za:443/handle/10019.1/100385 (Accessed July 2, 2021).
30. Choudhury M, Koduru TN, Kumar N, Salimi S, Desai K, Prabhu NP, et al. Iron Uptake and Transport by the Carboxymycobactin-Mycobactin Siderophore Machinery of Mycobacterium Tuberculosis Is Dependent on the Iron-Regulated Protein HupB. BioMetals (2021) 34:511–28. doi: 10.1007/s10534-021-00292-2
31. Pisu D, Huang L, Grenier JK, Russell DG. Dual RNA-Seq of Mtb-Infected Macrophages In Vivo Reveals Ontologically Distinct Host-Pathogen Interactions. Cell Rep (2020) 30:335–50.e4. doi: 10.1016/j.celrep.2019.12.033
32. Reddy VP, Chinta KC, Saini V, Glasgow JN, Hull TD, Traylor A, et al. Ferritin H Deficiency in Myeloid Compartments Dysregulates Host Energy Metabolism and Increases Susceptibility to Mycobacterium Tuberculosis Infection. Front Immunol (2018) 9:860. doi: 10.3389/fimmu.2018.00860
33. Catanzaro A, Perry S, Clarridge JE, Dunbar S, Goodnight-White S, LoBue PA, et al. The Role of Clinical Suspicion in Evaluating a New Diagnostic Test for Active Tuberculosis: Results of a Multicenter Prospective Trial. JAMA (2000) 283:639–45. doi: 10.1001/jama.283.5.639
34. Abel L, El-Baghdadi J, Bousfiha AA, Casanova J-L, Schurr E. Human Genetics of Tuberculosis: A Long and Winding Road. Philos Trans R Soc B Biol Sci (2014) 369:20130428. doi: 10.1098/rstb.2013.0428
35. Ghiasi M, Pande T, Pai M. Advances in Tuberculosis Diagnostics. Curr Trop Med Rep (2015) 2:54–61. doi: 10.1007/s40475-015-0043-1
36. Ryu YJ. Diagnosis of Pulmonary Tuberculosis: Recent Advances and Diagnostic Algorithms. Tuberc Respir Dis (2015) 78:64–71. doi: 10.4046/trd.2015.78.2.64
37. Teixeira HC, Abramo C, Munk ME. Immunological Diagnosis of Tuberculosis: Problems and Strategies for Success. J Bras Pneumol Publicacao Of Soc Bras Pneumol E Tisilogia (2007) 33:323–34. doi: 10.1590/s1806-37132007000300015
38. Abraham PR, Devalraju KP, Jha V, Valluri VL, Mukhopadhyay S. PPE17 (Rv1168c) Protein of Mycobacterium Tuberculosis Detects Individuals With Latent TB Infection. PloS One (2018) 13:e0207787. doi: 10.1371/journal.pone.0207787
39. Cao SH, Chen YQ, Sun Y, Liu Y, Zheng SH, Zhang ZG, et al. Screening of Serum Biomarkers for Distinguishing Between Latent and Active Tuberculosis Using Proteome Microarray. BioMed Environ Sci BES (2018) 31:515–26. doi: 10.3967/bes2018.069
40. Kiazyk S, Ball T. Latent Tuberculosis Infection: An Overview. Can Commun Dis Rep (2017) 43:62–6. doi: 10.14745/ccdr.v43i34a01
41. Sharma SK, Vashishtha R, Chauhan LS, Sreenivas V, Seth D. Comparison of TST and IGRA in Diagnosis of Latent Tuberculosis Infection in a High TB-Burden Setting. PloS One (2017) 12:e0169539. doi: 10.1371/journal.pone.0169539
42. Chegou NN, Heyckendorf J, Walzl G, Lange C, Ruhwald M. Beyond the IFN-γ Horizon: Biomarkers for Immunodiagnosis of Infection With Mycobacterium Tuberculosis. Eur Respir J (2014) 43:1472–86. doi: 10.1183/09031936.00151413
43. Boehme CC, Nabeta P, Hillemann D, Nicol MP, Shenai S, Krapp F, et al. Rapid Molecular Detection of Tuberculosis and Rifampin Resistance. N Engl J Med (2010) 363:1005–15. doi: 10.1056/NEJMoa0907847
44. Churchyard GJ, Stevens WS, Mametja LD, McCarthy KM, Chihota V, Nicol MP, et al. Xpert MTB/RIF Versus Sputum Microscopy as the Initial Diagnostic Test for Tuberculosis: A Cluster-Randomised Trial Embedded in South African Roll-Out of Xpert MTB/RIF. Lancet Glob Health (2015) 3:e450–7. doi: 10.1016/S2214-109X(15)00100-X
45. Theron G, Zijenah L, Chanda D, Clowes P, Rachow A, Lesosky M, et al. Feasibility, Accuracy, and Clinical Effect of Point-of-Care Xpert MTB/RIF Testing for Tuberculosis in Primary-Care Settings in Africa: A Multicentre, Randomised, Controlled Trial. Lancet (2014) 383:424–35. doi: 10.1016/S0140-6736(13)62073-5
46. Nema V. Tuberculosis Diagnostics: Challenges and Opportunities. Lung India Off Organ Indian Chest Soc (2012) 29:259–66. doi: 10.4103/0970-2113.99112
47. Goletti D, Petruccioli E, Joosten SA, Ottenhoff THM. Tuberculosis Biomarkers: From Diagnosis to Protection. Infect Dis Rep (2016) 8:6568. doi: 10.4081/idr.2016.6568
48. Walzl G, McNerney R, du Plessis N, Bates M, McHugh TD, Chegou NN, et al. Tuberculosis: Advances and Challenges in Development of New Diagnostics and Biomarkers. Lancet Infect Dis (2018) 18:e199–210. doi: 10.1016/S1473-3099(18)30111-7
49. Gunjan K, Mohsin R K. TB. Interferon-Gamma (IFN-γ): Exploring Its Implications in Infectious Diseases. Biomol Concepts (2018) 9:64–79. doi: 10.1515/bmc-2018-0007
50. Chegou NN, Black GF, Loxton AG, Stanley K, Essone PN, Klein MR, et al. Potential of Novel Mycobacterium Tuberculosis Infection Phase-Dependent Antigens in the Diagnosis of TB Disease in a High Burden Setting. BMC Infect Dis (2012) 12:10. doi: 10.1186/1471-2334-12-10
51. Chegou NN, Sutherland JS, Malherbe S, Crampin AC, Corstjens PLAM, Geluk A, et al. Diagnostic Performance of a Seven-Marker Serum Protein Biosignature for the Diagnosis of Active TB Disease in African Primary Healthcare Clinic Attendees With Signs and Symptoms Suggestive of TB. Thorax (2016) 71:785–94. doi: 10.1136/thoraxjnl-2015-207999
52. Wang S, Li Y, Shen Y, Wu J, Gao Y, Zhang S, et al. Screening and Identification of a Six-Cytokine Biosignature for Detecting TB Infection and Discriminating Active From Latent TB. J Transl Med (2018) 16:206. doi: 10.1186/s12967-018-1572-x
53. Zak DE, Penn-Nicholson A, Scriba TJ, Thompson E, Suliman S, Amon LM, et al. A Blood RNA Signature for Tuberculosis Disease Risk: A Prospective Cohort Study. Lancet (2016) 387:2312–22. doi: 10.1016/S0140-6736(15)01316-1
54. Jacobs R, Tshehla E, Malherbe S, Kriel M, Loxton AG, Stanley K, et al. Host Biomarkers Detected in Saliva Show Promise as Markers for the Diagnosis of Pulmonary Tuberculosis Disease and Monitoring of the Response to Tuberculosis Treatment. Cytokine (2016) 81:50–6. doi: 10.1016/j.cyto.2016.02.004
55. Day CL, Abrahams DA, Bunjun R, Stone L, de Kock M, Walzl G, et al. PD-1 Expression on Mycobacterium Tuberculosis-Specific CD4 T Cells Is Associated With Bacterial Load in Human Tuberculosis. Front Immunol (2018) 9:1995. doi: 10.3389/fimmu.2018.01995
56. van Rensburg IC, Wagman C, Stanley K, Beltran C, Ronacher K, Walzl G, et al. Successful TB Treatment Induces B-Cells Expressing FASL and IL5RA mRNA. Oncotarget (2017) 8:2037–43. doi: 10.18632/oncotarget.12184
57. Loxton AG, Black GF, Stanley K, Walzl G. Heparin-Binding Hemagglutinin Induces IFN-γ+ IL-2+ IL-17+ Multifunctional CD4+ T Cells During Latent But Not Active Tuberculosis Disease. Clin Vaccine Immunol CVI (2012) 19:746–51. doi: 10.1128/CVI.00047-12
58. Chegou NN, Essone PN, Loxton AG, Stanley K, Black GF, van der Spuy GD, et al. Potential of Host Markers Produced by Infection Phase-Dependent Antigen-Stimulated Cells for the Diagnosis of Tuberculosis in a Highly Endemic Area. PloS One (2012) 7:e38501. doi: 10.1371/journal.pone.0038501
59. Park H-D, Guinn KM, Harrell MI, Liao R, Voskuil MI, Tompa M, et al. Rv3133c/dosR Is a Transcription Factor That Mediates the Hypoxic Response of Mycobacterium Tuberculosis. Mol Microbiol (2003) 48:833–43. doi: 10.1046/j.1365-2958.2003.03474.x
60. Suliman S, Thompson EG, Sutherland J, Weiner J, Ota MOC, Shankar S, et al. Four-Gene Pan-African Blood Signature Predicts Progression to Tuberculosis. Am J Respir Crit Care Med (2018) 197:1198–208. doi: 10.1164/rccm.201711-2340OC
61. Ronacher K, Chegou NN, Kleynhans L, Djoba Siawaya JF, du Plessis N, Loxton AG, et al. Distinct Serum Biosignatures Are Associated With Different Tuberculosis Treatment Outcomes. Tuberculosis (2019) 118:101859. doi: 10.1016/j.tube.2019.101859
62. Cilliers K, Menezes A, Webber T, Dockrell HM, Cliff JM, Kleynhans L, et al. Mycobacterium Tuberculosis-Stimulated Whole Blood Culture to Detect Host Biosignatures for Tuberculosis Treatment Response. Tuberc Edinb Scotl (2021) 128:102082. doi: 10.1016/j.tube.2021.102082
63. Menozzi FD, Rouse JH, Alavi M, Laude-Sharp M, Muller J, Bischoff R, et al. Identification of a Heparin-Binding Hemagglutinin Present in Mycobacteria. J Exp Med (1996) 184:993–1001. doi: 10.1084/jem.184.3.993
64. Raze D, Verwaerde C, Deloison G, Werkmeister E, Coupin B, Loyens M, et al. Heparin-Binding Hemagglutinin Adhesin (HBHA) Is Involved in Intracytosolic Lipid Inclusions Formation in Mycobacteria. Front Microbiol (2018) 9:2258. doi: 10.3389/fmicb.2018.02258
65. Kunnath-Velayudhan S, Salamon H, Wang H-Y, Davidow AL, Molina DM, Huynh VT, et al. Dynamic Antibody Responses to the Mycobacterium Tuberculosis Proteome. Proc Natl Acad Sci USA (2010) 107:14703–8. doi: 10.1073/pnas.1009080107
66. Awoniyi DO, Baumann R, Chegou NN, Kriel B, Jacobs R, Kidd M, et al. Detection of a Combination of Serum IgG and IgA Antibodies Against Selected Mycobacterial Targets Provides Promising Diagnostic Signatures for Active TB. Oncotarget (2017) 8:37525–37. doi: 10.18632/oncotarget.16401
67. Oppen K, Ueland T, Siljan WW, Skadberg Ø, Brede C, Lauritzen T, et al. Hepcidin and Ferritin Predict Microbial Etiology in Community-Acquired Pneumonia. Open Forum Infect Dis (2021) 8:ofab082. doi: 10.1093/ofid/ofab082
68. Dai Y, Shan W, Yang Q, Guo J, Zhai R, Tang X, et al. Biomarkers of Iron Metabolism Facilitate Clinical Diagnosis in M Ycobacterium Tuberculosis Infection. Thorax (2019) 74:1161–7. doi: 10.1136/thoraxjnl-2018-212557
69. Luo Y, Xue Y, Lin Q, Tang G, Yuan X, Mao L, et al. Sun Z. A Combination of Iron Metabolism Indexes and Tuberculosis-Specific Antigen/Phytohemagglutinin Ratio for Distinguishing Active Tuberculosis From Latent Tuberculosis Infection. Int J Infect Dis (2020) 97:190–6. doi: 10.1016/j.ijid.2020.05.109
70. Minchella PA, Donkor S, McDermid JM, Sutherland JS. Iron Homeostasis and Progression to Pulmonary Tuberculosis Disease Among Household Contacts. Tuberc Edinb Scotl (2015) 95:288–93. doi: 10.1016/j.tube.2015.02.042
71. Sivakolundu S, Mannela UD, Jain S, Srikantam A, Peri S, Pandey SD, et al. Serum Iron Profile and ELISA-Based Detection of Antibodies Against the Iron-Regulated Protein HupB of Mycobacterium Tuberculosis in TB Patients and Household Contacts in Hyderabad (Andhra Pradesh), India. Trans R Soc Trop Med Hyg (2013) 107:43–50. doi: 10.1093/trstmh/trs005
72. Phelan JJ, Basdeo SA, Tazoll SC, McGivern S, Saborido JR, Keane J. Modulating Iron for Metabolic Support of TB Host Defense. Front Immunol (2018) 9:2296. doi: 10.3389/fimmu.2018.02296
73. Rohde KH, Veiga DFT, Caldwell S, Balázsi G, Russell DG. Linking the Transcriptional Profiles and the Physiological States of Mycobacterium Tuberculosis During an Extended Intracellular Infection. PloS Pathog (2012) 8:e1002769. doi: 10.1371/journal.ppat.1002769
74. Rohde KH, Abramovitch RB, Russell DG. Mycobacterium Tuberculosis Invasion of Macrophages: Linking Bacterial Gene Expression to Environmental Cues. Cell Host Microbe (2007) 2:352–64. doi: 10.1016/j.chom.2007.09.006
75. Willemse D, Weber B, Masino L, Warren RM, Adinolfi S, Pastore A, et al. Rv1460, A SufR Homologue, Is a Repressor of the Suf Operon in Mycobacterium Tuberculosis. PloS One (2018) 13:e0200145. doi: 10.1371/journal.pone.0200145
76. Anand K, Tripathi A, Shukla K, Malhotra N, Jamithireddy AK, Jha RK, et al. Mycobacterium Tuberculosis SufR Responds to Nitric Oxide via Its 4Fe-4S Cluster and Regulates Fe-S Cluster Biogenesis for Persistence in Mice. Redox Biol (2021) 46:102062. doi: 10.1016/j.redox.2021.102062
77. Kumar M, Khan FG, Sharma S, Kumar R, Faujdar J, Sharma R, et al. Identification of Mycobacterium Tuberculosis Genes Preferentially Expressed During Human Infection. Microb Pathog (2011) 50:31–8. doi: 10.1016/j.micpath.2010.10.003
78. Dosanjh NS, Rawat M, Chung J-H, Av-Gay Y. Thiol Specific Oxidative Stress Response in Mycobacteria. FEMS Microbiol Lett (2005) 249:87–94. doi: 10.1016/j.femsle.2005.06.004
79. Pathakumari B, Devasundaram S, Raja A. Altered Expression of Antigen-Specific Memory and Regulatory T-Cell Subsets Differentiate Latent and Active Tuberculosis. Immunology (2018) 153:325–36. doi: 10.1111/imm.12833
80. Yang X, Wu J-B, Liu Y, Xiong Y, Ji P, Wang S-J, et al. Identification of Mycobacterial Bacterioferritin B for Immune Screening of Tuberculosis and Latent Tuberculosis Infection. Tuberc Edinb Scotl (2017) 107:119–25. doi: 10.1016/j.tube.2017.08.005
81. Schuck SD, Mueller H, Kunitz F, Neher A, Hoffmann H, Franken KLCM, et al. Identification of T-Cell Antigens Specific for Latent Mycobacterium Tuberculosis Infection. PloS One (2009) 4:e5590. doi: 10.1371/journal.pone.0005590
Keywords: tuberculosis, diagnosis, biomarkers, Fe-S clusters, siderophores, iron
Citation: Baatjies L, Loxton AG and Williams MJ (2021) Host and Bacterial Iron Homeostasis, an Underexplored Area in Tuberculosis Biomarker Research. Front. Immunol. 12:742059. doi: 10.3389/fimmu.2021.742059
Received: 15 July 2021; Accepted: 14 October 2021;
Published: 29 October 2021.
Edited by:
Julia Kzhyshkowska, Heidelberg University, GermanyReviewed by:
Kyle Rohde, University of Central Florida, United StatesSelvakumar Subbian, Public Health Research Institute (PHRI), United States
Copyright © 2021 Baatjies, Loxton and Williams. This is an open-access article distributed under the terms of the Creative Commons Attribution License (CC BY). The use, distribution or reproduction in other forums is permitted, provided the original author(s) and the copyright owner(s) are credited and that the original publication in this journal is cited, in accordance with accepted academic practice. No use, distribution or reproduction is permitted which does not comply with these terms.
*Correspondence: Lucinda Baatjies, bGJhYXRqaWVzQHN1bi5hYy56YQ==
†These authors have contributed equally to this work and share last authorship