- 1Fondazione The Microsoft Research – University of Trento Centre for Computational and Systems Biology (COSBI), Rovereto, Italy
- 2Preclinical, GSK, Rockville, MD, United States
- 3Department of Cellular, Computational and Integrative Biology (CIBIO), University of Trento, Povo, Italy
- 4Department of Computer Science, University of Pisa, Pisa, Italy
- 5Toscana Life Sciences Foundation, Siena, Italy
- 6Data Science and Computational Vaccinology, GSK, Siena, Italy
RNA vaccines represent a milestone in the history of vaccinology. They provide several advantages over more traditional approaches to vaccine development, showing strong immunogenicity and an overall favorable safety profile. While preclinical testing has provided some key insights on how RNA vaccines interact with the innate immune system, their mechanism of action appears to be fragmented amid the literature, making it difficult to formulate new hypotheses to be tested in clinical settings and ultimately improve this technology platform. Here, we propose a systems biology approach, based on the combination of literature mining and mechanistic graphical modeling, to consolidate existing knowledge around mRNA vaccines mode of action and enhance the translatability of preclinical hypotheses into clinical evidence. A Natural Language Processing (NLP) pipeline for automated knowledge extraction retrieved key biological evidences that were joined into an interactive mechanistic graphical model representing the chain of immune events induced by mRNA vaccines administration. The achieved mechanistic graphical model will help the design of future experiments, foster the generation of new hypotheses and set the basis for the development of mathematical models capable of simulating and predicting the immune response to mRNA vaccines.
Introduction
Since December 2019 SARS-CoV-2 virus has spread across the globe, becoming a pandemic threat and claiming millions of lives. The contagiousness combined with the mortality rate triggered unprecedented efforts to quickly design and develop a vaccine. The first two vaccines that received emergency use authorization by EMA and FDA to prevent COVID-19 disease in humans are based on messenger RNA (mRNA), a relatively new vaccine platform with several advantages over more traditional approaches for vaccine design (1–7). Because of their unique features in terms of manufacturability, mechanism of action and ability to induce potent immune responses, mRNA vaccines represent an important advancement in the history of vaccinology to defeat infectious diseases. mRNA vaccines are based on the concept that, starting from the amino acid sequence of the antigen of interest, it is possible to design a related mRNA sequence that is employed by the cells of the body as template to express the antigen in situ. mRNA vaccines are known to stimulate both arms of the humoral and cellular immunity (7), however their mechanism of action is still partially understood. In this work, we applied a systems biology approach (Figure 1) to dissect and elucidate: the delivery of mRNA vaccines, the antigen expression and the resulting vaccine-specific immune responses.
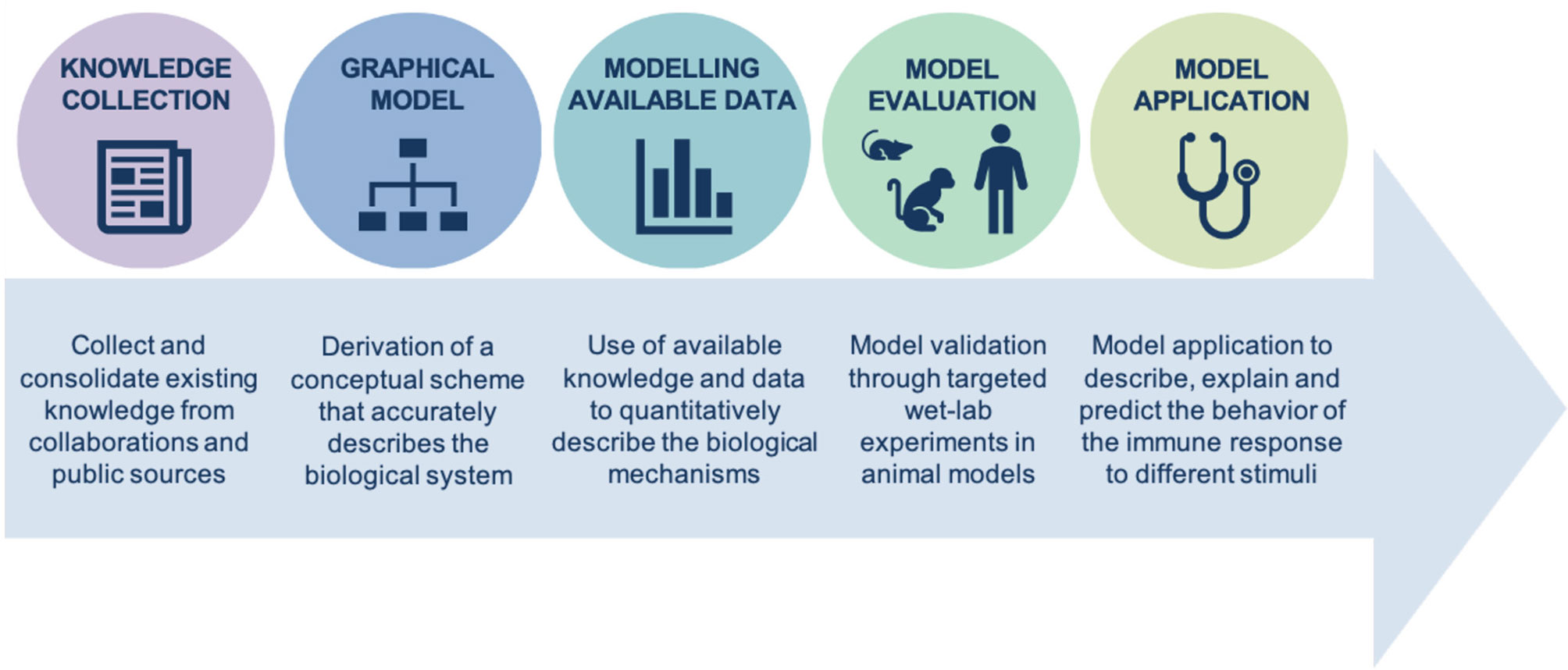
Figure 1 In silico modeling of the immune system. Integrative systems biology approach to properly describe, explain, and predict the behavior of any biological mechanism, which in our specific case study is the immune response to mRNA-based vaccines.
The strategy depicted in Figure 1 applies to several biological processes and sets the basis for building mathematical models starting from already established knowledge, mainly stored within the scientific literature. The ability for an investigator to process the vast amount of publications through automated literature mining and natural language processing (NLP) algorithms is instrumental to efficiently collect relevant information and define a comprehensive picture. Within each publication, the heterogeneity of data sources (e.g. in vitro evidence, animal models, clinical trials, etc.) requires an additional effort to integrate the retrieved information into an interactive platform that can be queried by the users. Databases are usually the preferred solution to store parameters and other numerical information, but as per RNA vaccines some processes may particularly benefit from a mechanistic graphical model that would help identify and fill in the knowledge gaps. Indeed, literature derived data could be complemented with proprietary knowledge and used to draft a mathematical model of the underlying biology. Accordingly, progressive and iterative refinement of the mechanistic process through targeted wet-lab experiments in animal models contributes to the model evaluation. The finalized model is eventually applied to specific scenarios of interest to predict, investigate and support drug development.
Although mRNA vaccines have been the focus of several studies in the last decade, they are usually employed with different components (encoded antigen, delivery system, mRNA architecture) and investigations focused on different arms of the immune response or different biological sites. Consequently, the generated knowledge tends to be rather specific to each individual platform, not always generalizable and sometimes even fragmented. This is what motivated our effort to collect and consolidate all publicly available scientific evidences, related to mRNA vaccine mode of action, into an interactive mechanistic graphical model (Figure 2, link to https://www.cosbi.eu/fx/9839203 dynamic figure) tracing the stages of the immune response to mRNA vaccines, from the innate immune activation at the site of injection up to the adaptive response, measurable in the peripheral blood system several days after vaccination. The proposed mechanistic graphical model facilitates the interpretation of what has been discovered so far and fosters interactions among investigators with very different backgrounds, such as immunologists and data scientists.
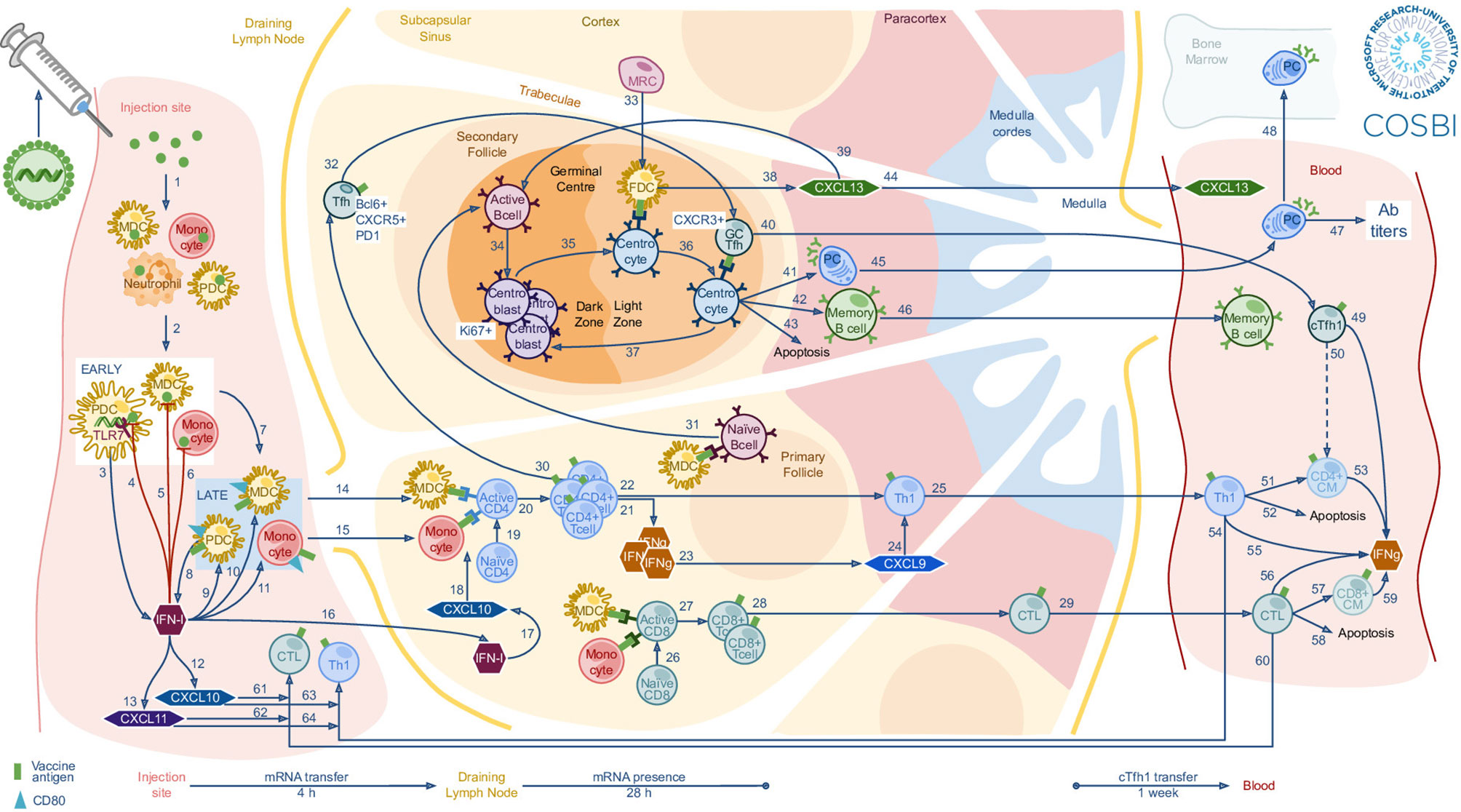
Figure 2 Mechanistic graphical model of the mRNA-based vaccine biological mechanism of action. Lipid nanoparticles encapsulating mRNA molecules are intradermal or intramuscular injected triggering immune signals that reach the draining lymph node, where other immune reactions generate the specific antibodies, eventually transferred into the circulatory system. This figure is proposed in a dynamic view at the link https://www.cosbi.eu/fx/9839203, where each connection (blue arrows) is documented by literature references as well as the involved cells measured in mouse and NHP experiments.
Literature Mining and Information Processing
Automated knowledge extraction from text is the very definition of text-mining, a process becoming more and more a necessity in researching the everyday growing amount of available body of text (8). Text mining does not just find the documents reporting the searched information, but systematically and efficiently examines those contents, a process that performed manually would be unfeasible, unrealistic and error prone. Several tools have been already developed for extracting biomedical information (9, 10), based on sophisticated NLP algorithms to read and analyze the huge number of scientific publications. Over time those methods have been largely improved, becoming suitable for annotating large experimental datasets and merging data from several studies across the world, which may lead to the discovery of global trends within the existing literature (11, 12).
In this part of the study we investigated the biological perturbations, at the level of immune cells and immunoactive peptides (cytokines/chemokines and cell-surface markers), induced by mRNA vaccines through an NLP-guided literature mining process (10). The initial search for mRNA vaccines-associated literature identified 361 scientific articles in PubMed, 245 of which were automatically annotated as providing experimental evidences generated in animal models. Given the relevance of non-human primates (NHP) as a model for human immunology studies, the pipeline selected 17 NHP-related papers, among which 6 were defined as eligible sources of information and manually confirmed (Figure 2). The 6 retrieved scientific publications have been extremely useful to understand the available information about mRNA vaccine mode of action in NHP and one described the SAM platform. To be as inclusive as possible, mice-derived data and information were also taken into consideration. These consisted in a set of 9 self-amplifying mRNA vaccines studies, 5 of which provided eligible quantitative information (Table 1). Manually curating the 361 papers of interest since the beginning would have been highly time consuming, while the properly instructed NLP pipeline was able to provide the answers to our questions in a matter of few hours, leaving to the scientists a manageable amount of information to explore.
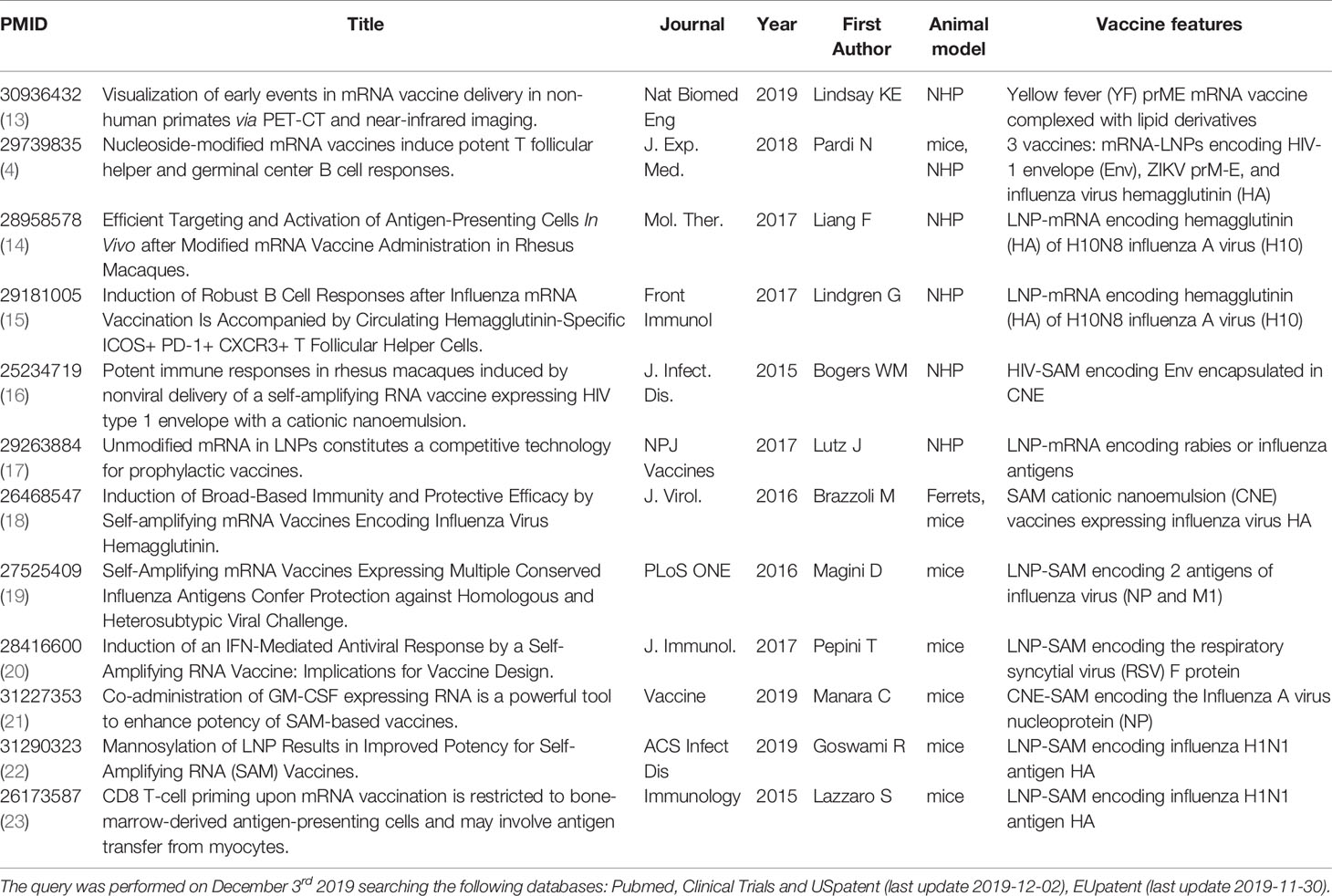
Table 1 List of relevant scientific literature studying mRNA-based vaccines in mouse and NHP animal models.
Scientific literature mining is foundational for the generation of new hypotheses as well as for driving future research and designing new studies. At this point, data and facts of different type and format are gathered and need to be integrated, pressuring data-integration methods to be efficient, in order to explicitly represent the deeply connected big picture the scientist is looking for (24).
Mechanistic Graphical Modelling
Figure 2 is a static version of the mechanistic review of the mechanisms of action of RNA vaccines, with a dynamic design made available at the link https://www.cosbi.eu/fx/9839203. Given the known similarities and discrepancies between the mouse and NHP models, we represented separately mouse and NHP data, each of them accessible by clicking on the respective black animal shape in the left upper corner of the model, overlapping the shared information when possible. The immunization process reported in Figure 2 covers the basics of biology, documented by the references attached to the arrows connecting each element of the model, which have been manually searched while integrating the novelties apported by the mRNA-based vaccine technology, discovered by literature-mining instead.
The mechanistic graphical model starts with either intramuscular or intradermal injection of mRNA, delivered through lipid nanoparticles (LNPs), which showed a more persistent protein expression than systemic intravenous delivery (4). RNA-LNPs enter the cytosol of local neutrophils, monocytes and dendritic cells (DCs), where mRNA expression begins. Immune cells subsequently migrate to the draining lymph node where they orchestrate the T cell and germinal center responses (14). A critical aspect is represented by the way the mRNA vaccine interacts with the sensors in the host’s cells (25). The signaling strength of the exogenous mRNA vector in activating pattern recognition receptors (PPRs), like RIG-I, MDA5 and members of the Toll-like receptors family, is relative to the mRNA species (26–29). This signal is de facto a self-adjuvanticity property in the SAM platform that should, at least in principle, be beneficial for the generation of potent immune responses. However, the activation of PPRs is typically associated with the production of type I interferons (IFNs) by plasmacytoid DCs (pDCs) (14) and the induction of the anti-viral state, a condition that has been proposed to severely limit the antibody titers, yet not necessarily impacting the vaccine efficacy (20, 30–32).
The mRNA-based vaccines have shown to most likely leave the injection site through vaccine loaded myeloid DCs (mDCs) making their way to the draining lymph node (dLN) (14), where the concentration of type I IFNs increases, inducing CXCL10, crucial to keep T cells and DCs in proximity, enhancing the chances of T cell activation. After encountering the antigen, both naïve CD4+ and CD8+ become activated and differentiate in mature CD4+ and CD8+ T cells, respectively (14). When CD8+ T cells encounter the antigen and differentiate into short-lived effector cytotoxic T lymphocytes (CTLs), they migrate to the peripheral tissues and to the sites of inflammation (17). In addition, RNA vaccines induce strong Tfh cell responses, which govern the germinal center reactions, including somatic hypermutation, affinity maturation, isotype switching and differentiation of the antigen-specific B cells (15). mRNA vaccines also induce CXCL13, a chemokine responsible for directing B cells efficiently into the follicles (15). From the germinal centers, antigen-specific B cells may differentiate into plasma cells, which home in the bone marrow and continuously secrete antigen-specific antibodies in the blood, or memory B cells, which recirculate in the blood until further antigen encounter (15).
Subsequently, circulating CD4+ and CD8+ T cells may undergo through two possible fates: apoptosis and survival into memory. Indeed, Th1 cells and CTLs may give rise to central memory (CM) CD4+ and CD8+ T cells, respectively. Moreover, depending on the mRNA vaccine doses, both Th1 cells and CTLs have been observed to produce IFNg (15), which is usually associated with strong anti-viral responses, underlying the efficacy of RNA-based therapeutics (15, 17).
Discussion
mRNA vaccines are emerging as one of the most promising technologies in vaccinology. Several pharmaceutical companies and research institutes are working at the development of new platforms for mRNA-based antigen delivery, trying to identify and characterize those parameters that are required for a safe and protective vaccine response. This, combined with the recent advancements in omics technologies, has resulted in the accumulation of a vast amount of data and information. With this work, we built from this unique opportunity and applied text-mining algorithms to screen and analyze scientific literature, with the aim of collecting all available experimentally validated evidence related to the mechanisms of action of mRNA vaccines. We processed and used this information to collect, in a centralized and structured fashion, the various stages of the immune response to mRNA vaccines across different organs and tissues. This knowledge base collection comes in the form of an interactive mechanistic graphical model, which allows to explore the different arms of the immune response to this kind of vaccines.
To maximize the simplicity and interpretability, the model was built using the minimal set of graphical elements, consisting of arrows (representing cells, chemotaxis, transformation or activity) and symbols representing relevant cells and immunoactive peptides. Ideally, a mechanistic graphical model should be readily available to the different stakeholders, should provide some level of interactivity for exploration of the underlying data and be readily upgradable to incorporate new evidences and information. Indeed, an interactive version of the model was made accessible, even remotely, using a web browser, by a proprietary javascript framework. Hosting a copy of the mechanistic graphical model on a remote server ensures future updates to be readily available, avoiding the need of sharing files and risk of misalignments among different versions. Furthermore, all the elements of the mechanistic graphical model can be clicked upon to access the original reference describing that specific evidence. The knowledge gathered during the development of the mechanistic graphical model provides an updated description of the biological phenomena underlying the immune response to mRNA vaccines. The graphical modelling platform can also facilitate the interaction among scientists from different areas, highlight potential gaps in data availability and knowledge and guide the design of new experiments. The natural evolution of this work would be to leverage on the quantitative information acquired during the process (e.g., kinetic parameters, cells concentrations, etc.) to develop a mathematical model describing the immune response, across different biological compartments (e.g. injection site and lymph node) over time (manuscript in preparation). Ideally, this will help highlighting the key elements of an immune response to mRNA vaccines that are responsible for a protective response or, conversely, elements that may lead to suboptimal responses or undesirable effects. Provided an accurate mathematical model is achieved, this could be used to support the experimental design, by allowing to simulate multiple scenarios and predict their outcome. An example could be that of predicting a presumably safe dose range in a dose finding, first time in human clinical study.
As mentioned before, modelling, and prospectively predicting, the behavior of the immune system is a highly challenging task. Consequently, the presented mechanistic graphical model should not be regarded as an endpoint but rather as a milestone within a broader modelling roadmap that we propose as a promising strategy to achieve a better understanding of the human immune system and how it responds to vaccination. The design of future studies for unraveling mRNA mechanism of action will have new pace through the use of literature mining and mathematical modelling, brought together by the power of modern technology.
Author Contributions
LL, GL, GS and SP computed the results presented in the paper. DT and SG provided technical support in implementing the interactive version of the graphical model. DM, ES and LM conceived the study. ES, GL, LL and LM wrote the manuscript. LL and GL share first authorship. ES and LM share last authorship. All authors contributed to the article and approved the submitted version.
Funding
This research project was funded by GlaxoSmithKline Biologicals SA.
Conflict of Interest
GL, HS, DM, and ES were all employees of the GSK group of companies at the time of the study. The “Fondazione The Microsoft Research – University of Trento Centre for Computational and Systems Biology (COSBI)” institute received financial remuneration for conducting the activates described in this study.
The authors declare that this study received funding from GlaxoSmithKline Biologicals SA. The funder had the following involvement in the study: study design, interpretation of data, the writing of this article and the decision to submit it for publication.
The remaining authors declare that the research was conducted in the absence of any commercial or financial relationships that could be construed as a potential conflict of interest.
Publisher’s Note
All claims expressed in this article are solely those of the authors and do not necessarily represent those of their affiliated organizations, or those of the publisher, the editors and the reviewers. Any product that may be evaluated in this article, or claim that may be made by its manufacturer, is not guaranteed or endorsed by the publisher.
References
1. Thess A, Grund S, Mui BL, Hope MJ, Baumhof P, Fotin-Mleczek M, et al. Sequence-Engineered mRNA Without Chemical Nucleoside Modifications Enables an Effective Protein Therapy in Large Animals. Mol Ther (2015) 23:1456–64. doi: 10.1038/mt.2015.103
2. Karikó K, Muramatsu H, Welsh FA, Ludwig J, Kato H, Akira S, et al. Incorporation of Pseudouridine Into mRNA Yields Superior Nonimmunogenic Vector With Increased Translational Capacity and Biological Stability. Mol Ther (2008) 16:1833–40. doi: 10.1038/mt.2008.200
3. Kauffman KJ, Webber MJ, Anderson DG. Materials for non-Viral Intracellular Delivery of Messenger RNA Therapeutics. J Control Release (2016) 240:227–34. doi: 10.1016/j.jconrel.2015.12.032
4. Pardi N, Hogan MJ, Naradikian MS, Parkhouse K, Cain DW, Jones L, et al. Nucleoside-Modified mRNA Vaccines Induce Potent T Follicular Helper and Germinal Center B Cell Responses. J Exp Med (2018) 215:1571–88. doi: 10.1084/jem.20171450
5. Guan S, Rosenecker J. Nanotechnologies in Delivery of mRNA Therapeutics Using Nonviral Vector-Based Delivery Systems. Gene Ther (2017) 24:133–43. doi: 10.1038/gt.2017.5
6. Ulmer JB, Geall AJ. Recent Innovations in mRNA Vaccines. Curr Opin Immunol (2016) 41:18–22. doi: 10.1016/j.coi.2016.05.008
7. Pardi N, Hogan MJ, Porter FW, Weissman D. mRNA Vaccines - a New Era in Vaccinology. Nat Rev Drug Discov (2018) 17:261–79. doi: 10.1038/nrd.2017.243
8. Renganathan V. Text Mining in Biomedical Domain With Emphasis on Document Clustering. Healthc Inform Res (2017) 23:141–6. doi: 10.4258/hir.2017.23.3.141
9. Ananiadou S MJ. Text Mining for Biology and Biomedicine. Artech House Publishers; Illustrated Edition (2006).
10. Ramponi A, Giampiccolo S, Tomasoni D, Priami C, Lombardo R. High-Precision Biomedical Relation Extraction for Reducing Human Curation Efforts in Industrial Applications. IEEE Access (2020) 8:150999–1011. doi: 10.1109/access.2020.3014862
11. Schuster J, Superdock M, Agudelo A, Stey P, Padbury J, Sarkar IN, et al. Machine Learning Approach to Literature Mining for the Genetics of Complex Diseases. Database (2019) 2019:1–12. doi: 10.1093/database/baz124
12. Simon C, Davidsen K, Hansen C, Seymour E, Barnkob MB, Olsen LR. BioReader: A Text Mining Tool for Performing Classification of Biomedical Literature. BMC Bioinf (2019) 19:57. doi: 10.1186/s12859-019-2607-x
13. Lindsay KE, Bhosle SM, Zurla C, Beyersdorf J, Rogers KA, Vanover D, et al. Visualization of Early Events in mRNA Vaccine Delivery in Non-Human Primates via PET-CT and Near-Infrared Imaging. Nat BioMed Eng (2019) 3:371–80. doi: 10.1038/s41551-019-0378-3
14. Liang F, Lindgren G, Lin A, Thompson EA, Ols S, Röhss J, et al. Efficient Targeting and Activation of Antigen-Presenting Cells In Vivo After Modified mRNA Vaccine Administration in Rhesus Macaques. Mol Ther (2017) 25:2635–47. doi: 10.1016/j.ymthe.2017.08.006
15. Lindgren G, Ols S, Liang F, Thompson EA, Lin A, Hellgren F, et al. Induction of Robust B Cell Responses After Influenza mRNA Vaccination Is Accompanied by Circulating Hemagglutinin-Specific ICOS+ PD-1+ CXCR3+ T Follicular Helper Cells. Front Immunol (2017) 8:1539. doi: 10.3389/fimmu.2017.01539
16. Bogers WM, Oostermeijer H, Mooij P, Koopman G, Verschoor EJ, Davis D, et al. Potent Immune Responses in Rhesus Macaques Induced by Nonviral Delivery of a Self-Amplifying RNA Vaccine Expressing HIV Type 1 Envelope With a Cationic Nanoemulsion. J Infect Dis (2015) 211:947–55. doi: 10.1093/infdis/jiu522
17. Lutz J, Lazzaro S, Habbeddine M, Schmidt KE, Baumhof P, Mui BL, et al. Unmodified mRNA in LNPs Constitutes a Competitive Technology for Prophylactic Vaccines. NPJ Vaccines (2017) 2:29. doi: 10.1038/s41541-017-0032-6
18. Brazzoli M, Magini D, Bonci A, Buccato S, Giovani C, Kratzer R, et al. Induction of Broad-Based Immunity and Protective Efficacy by Self-Amplifying mRNA Vaccines Encoding Influenza Virus Hemagglutinin. J Virol (2016) 90:332–44. doi: 10.1128/JVI.01786-15
19. Magini D, Giovani C, Mangiavacchi C, Maccari S, Cecchi R, Ulmer JB, et al. Self-Amplifying mRNA Vaccines Expressing Multiple Conserved Influenza Antigens Confer Protection Against Homologous and Heterosubtypic Viral Challenge. PLoS One (2016) 11:e0161193. doi: 10.1371/journal.pone.0161193
20. Pepini T, Pulichino A-P, Carsillo T, Carlson AL, Sari-Sarraf F, Ramsauer K, et al. Induction of an IFN-Mediated Antiviral Response by a Self-Amplifying RNA Vaccine: Implications for Vaccine Design. J Immunol (2017) 198:4012–24. doi: 10.4049/jimmunol.1601877
21. Manara C, Brazzoli M, Piccioli D, Taccone M, D'Oro U, Maione D, et al. Co-Administration of GM-CSF Expressing RNA Is a Powerful Tool to Enhance Potency of SAM-Based Vaccines. Vaccine (2019) 37:4204–13. doi: 10.1016/j.vaccine.2019.04.028
22. Goswami R, Chatzikleanthous D, Lou G, Giusti F, Bonci A, Taccone M, et al. Mannosylation of LNP Results in Improved Potency for Self-Amplifying RNA (SAM) Vaccines. ACS Infect Dis (2019) 5:1546–58. doi: 10.1021/acsinfecdis.9b00084
23. Lazzaro S, Giovani C, Mangiavacchi S, Magini D, Maione D, Baudner B, et al. CD8 T-Cell Priming Upon mRNA Vaccination Is Restricted to Bone-Marrow-Derived Antigen-Presenting Cells and may Involve Antigen Transfer From Myocytes. Immunology (2015) 146:312–26. doi: 10.1111/imm.12505
24. Zhao S, Su C, Lu Z, Wang F. Recent Advances in Biomedical Literature Mining. Briefings Bioinf (2020) 22(3):1–19. doi: 10.1093/bib/bbaa057
25. Chen N, Xia P, Li S, Zhang T, Wang TT, Zhu J. RNA Sensors of the Innate Immune System and Their Detection of Pathogens. IUBMB Life (2017) 69:297–304. doi: 10.1002/iub.1625
26. Hornung V, Ellegast J, Kim S, Brzózka K, Jung A, Kato H, et al. 5’-Triphosphate RNA is the Ligand for RIG-I. Science (2006) 314:994–7. doi: 10.1126/science.1132505
27. Pichlmair A, Schulz O, Tan C-P, Rehwinkel J, Kato H, Takeuchi O, et al. Activation of MDA5 Requires Higher-Order RNA Structures Generated During Virus Infection. J Virol (2009) 83:10761–9. doi: 10.1128/jvi.00770-09
28. Diebold SS, Kaisho T, Hemmi H, Akira S, Reis e Sousa C. Innate Antiviral Responses by Means of TLR7-Mediated Recognition of Single-Stranded RNA. Science (2004) 303:1529–31. doi: 10.1126/science.1093616
29. Edwards DK, Jasny E, Yoon H, Horscroft N, Schanen B, Geter T, et al. Adjuvant Effects of a Sequence-Engineered mRNA Vaccine: Translational Profiling Demonstrates Similar Human and Murine Innate Response. J Trans Med (2017) 15:1. doi: 10.1186/s12967-016-1111-6
30. Kim YG, Baltabekova AZ, Zhiyenbay EE, Aksambayeva AS, Shagyrova ZS, Khannanov R, et al. Recombinant Vaccinia Virus-Coded Interferon Inhibitor B18R: Expression, Refolding and a Use in a Mammalian Expression System With a RNA-Vector. PLoS One (2017) 12:e0189308. doi: 10.1371/journal.pone.0189308
31. Beissert T, Koste L, Perkovic M, Walzer KC, Erbar S, Selmi A, et al. Improvement of In Vivo Expression of Genes Delivered by Self-Amplifying RNA Using Vaccinia Virus Immune Evasion Proteins. Hum Gene Ther (2017) 28:1138–46. doi: 10.1089/hum.2017.121
Keywords: mRNA vaccines, natural language processing, graphical modeling, scientific literature mining, mechanisms of action
Citation: Leonardelli L, Lofano G, Selvaggio G, Parolo S, Giampiccolo S, Tomasoni D, Domenici E, Priami C, Song H, Medini D, Marchetti L and Siena E (2021) Literature Mining and Mechanistic Graphical Modelling to Improve mRNA Vaccine Platforms. Front. Immunol. 12:738388. doi: 10.3389/fimmu.2021.738388
Received: 08 July 2021; Accepted: 23 August 2021;
Published: 07 September 2021.
Edited by:
Jurjen Tel, Eindhoven University of Technology, NetherlandsReviewed by:
Kashish Chetal, Massachusetts General Hospital and Harvard Medical School, United StatesJennifer Oyler-Yaniv, Harvard Medical School, United States
Copyright © 2021 Leonardelli, Lofano, Selvaggio, Parolo, Giampiccolo, Tomasoni, Domenici, Priami, Song, Medini, Marchetti and Siena. This is an open-access article distributed under the terms of the Creative Commons Attribution License (CC BY). The use, distribution or reproduction in other forums is permitted, provided the original author(s) and the copyright owner(s) are credited and that the original publication in this journal is cited, in accordance with accepted academic practice. No use, distribution or reproduction is permitted which does not comply with these terms.
*Correspondence: Duccio Medini, d.medini@toscanalifesciences.org
†ORCID: Luca Marchetti, orcid.org/0000-0001-9043-7705
‡These authors share first authorship
§These authors share last authorship